- 1Department of Psychiatry, Interdisciplinary Center Psychopathology and Emotion Regulation, University Medical Center Groningen, University of Groningen, Groningen, Netherlands
- 2Department of Developmental Psychology, Faculty of Behavioural and Social Sciences, University of Groningen, Groningen, Netherlands
Scientific evidence in the field of psychiatry is mainly derived from group-based (“nomothetic”) studies that yield group-aggregated results, while often the need is to answer questions that apply to individuals. Particularly in the presence of great inter-individual differences and temporal complexities, information at the individual-person level may be valuable for personalized treatment decisions, individual predictions and diagnostics. The single-subject study design can be used to make inferences about individual persons. Yet, the single-subject study is not often used in the field of psychiatry. We believe that this is because of a lack of awareness of its value rather than a lack of usefulness or feasibility. In the present paper, we aimed to resolve some common misconceptions and beliefs about single-subject studies by discussing some commonly heard “facts and fictions.” We also discuss some situations in which the single-subject study is more or less appropriate, and the potential of combining single-subject and group-based study designs into one study. While not intending to plea for single-subject studies at the expense of group-based studies, we hope to increase awareness of the value of single-subject research by informing the reader about several aspects of this design, resolving misunderstanding, and providing references for further reading.
Introduction
Scientific evidence in the field of psychiatry mainly relies on studies that evaluate what is true on average in the population or a group. In many instances these studies yield valuable information, but particularly when the goal is to improve patient care we need to answer questions that apply to individual patients. For instance, if we want to know whether an antidepressant drug is effective in a particular patient, it will not suffice to know that this drug results in an average reduction of 0.31 SD in depressive symptoms in the population (1). Also, knowing that at the group level depressive symptoms are associated with increased levels of inflammatory markers (2) will not inform us whether for a specific patient depressive symptoms will increase when levels of inflammatory markers increase. It is increasingly being recognized that there are great inter-individual differences in causes, risk factors, and course over time of psychiatric disorders and their symptoms, and their response to treatments [e.g., (3, 4)].
To illustrate the potential magnitude of this heterogeneity, Figure 1 shows the course over time of depressive symptoms weekly assessed over a period of 3 years in 267 persons who were depressed at baseline [see for study details (5)]. The Figure shows that there are great differences between persons in the trajectories and that most persons show substantial fluctuations in symptom levels over time. It seems that for very few persons the average trajectory (left panel) applies, even were it to some extent. So, one may wonder to what extent such group-level results will give us information about what happens in most individual persons studied in that group.
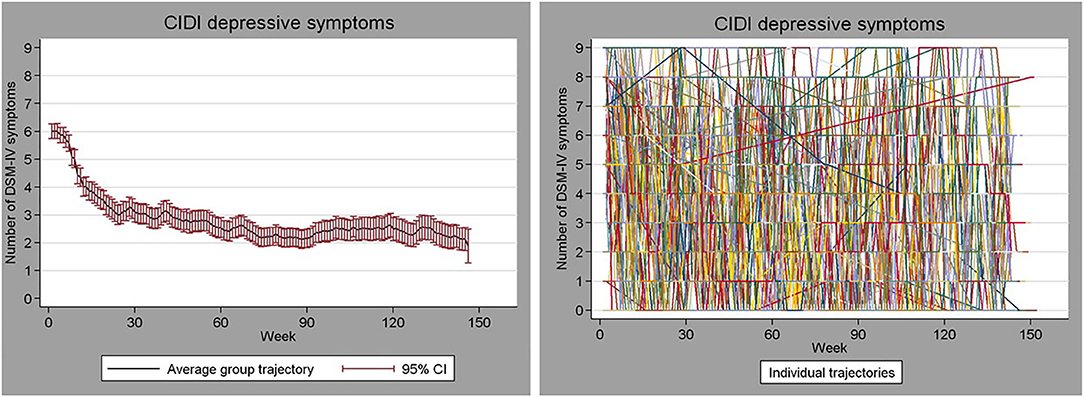
Figure 1. Weekly assessed depressive symptom severity over a period of 3 years in 267 persons who were initially depressed. Left: mean (95% CI) symptom severity. Right: trajectories of the individual persons.
What the Figure shows is not an extraordinary pattern, and many authors have noted the problem of relying on averages when no subject is average [e.g., (6–12)]. Many phenomena we study in the field of psychiatry are very heterogeneous across people, and most phenomena are not static but are highly dynamic (for example mood regulation and stress physiology). In the presence of such great inter- and intra-individual variability, information at the individual-person level may be of great value for making personalized treatment decisions or identifying personal predictors of changes in symptoms. Furthermore, in order to grasp the highly dynamic nature of certain phenomena we would need multiple repeated assessments across time. The single-subject study is a useful study design that can be used to make inferences about individual persons and to uncover the highly dynamic nature of our variables of interest. Nevertheless, this design is rarely used in the field of psychiatry. We think this may be due to a lack of awareness of its value, which may be partly due to a number of persistent misconceptions regarding single-subject studies. In the present paper, we aim to increase the recognition of the value of single-subject studies in the field of psychiatry by discussing some major facts and fictions of single-subject research.
Single-subject studies are characterized by their focus on single persons. This is in contrast to most traditional group-based (“nomothetic”) study designs, which focus on group averages and compare (groups of) individuals with other individuals (such as RCTs, cohort studies or case-control studies). In single-subject studies, data of each individual are analyzed separately and individuals are compared with themselves (13, 14). By virtue of multiple assessments collected within one individual, an individual can serve as his or her own control over time. This allows to quantitatively examine whether changes in one variable are systematically related to changes in another variable within an individual (observational single-subject design), or whether an experimental manipulation is related to a consistent change within this individual (experimental single-subject design; see Figure 2).
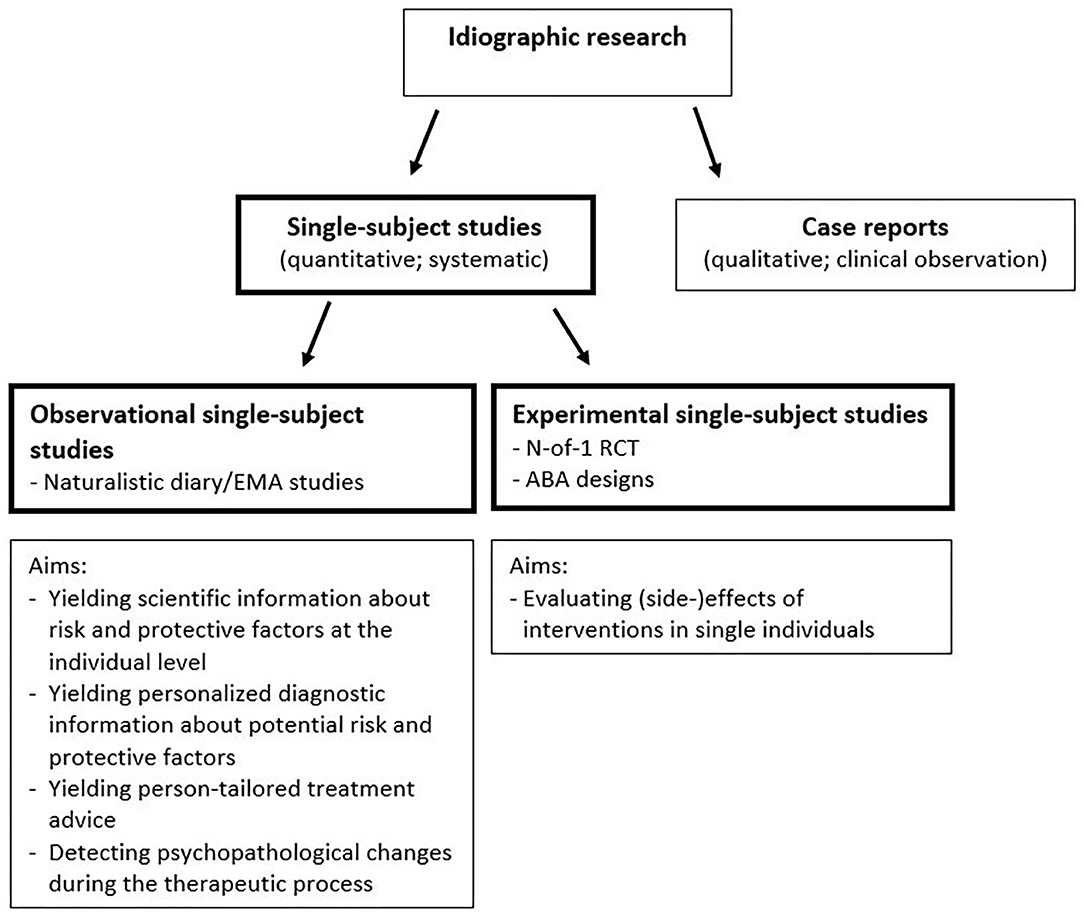
Figure 2. Schematic illustration of different sorts of single-subject studies and some examples of aims of the two types of single-subject studies.
More generalizable conclusions can be obtained by replicating multiple single-subject studies on a specific topic. In the presence of great inter- and intra-individual variability, this will only answer questions that apply to individual patients when each participant is analyzed at the intra-individual level. If the same effect is found in a series of single-subject studies, this could potentially be the basis for a generalizable conclusion. That is, the association might be true for the majority of persons [i.e., true in general; (15)]. In case of large heterogeneity, the chance of finding such commonalities for processes underlying psychiatric disorders might not be great. In that case, single-subject studies can be used to link individual-level results to certain person characteristics, or may be used in clinical practice to inform the treatment process.
The single-subject study might be rare nowadays, it has been used much in earlier centuries and has yielded important information about human behavior, physiology and pathology (see Box 1). The use of this design began to decline at the beginning of the 20th century, when people became interested in the improvement of species or races (27). In that time, scientists (and eugenicists) like Pearson and Fisher introduced statistical techniques focusing on group averages, therewith fueling a paradigm shift toward group-aggregated results. This shift toward statistics based on group averages was a logical step to make if the interest is in improving species or plant varieties. For example, if a farmer wants to know which factors improve the growth of lettuce plants, he is not interested in the growth of the individual lettuce plant, but rather in the average yield of the whole field of lettuce plants. However, as we illustrated in the first paragraph of this introduction, many questions in the field of psychiatry apply to individual patients. The almost complete disappearance of the single-subject study at the beginning of the 20th century therefore seems incompatible with the demand for information that applies to individual persons in this field.
Box 1. The single-subject study has a long history.
Single-subject research is rather unpopular nowadays, except in some specific subfields of psychology (16). However, it used to be very common. At the end of the 19th century, “the intensive study of individuals” (also called “Idiographic research”) was the most popular scientific approach (15). The term “nomothetic” had a different meaning in those days: nomothetic research was research aimed at establishing general laws and theories (10). It was thought that establishing general laws cannot be done without describing and explaining particular events and individual processes. That is, in order to find out whether something is true for all or the majority of persons, one must first describe and explain what holds for single individuals. Accordingly, nomothetic and idiographic research were seen as complementary. This changed in the beginning of the 20th century. Inspired by famous statisticians and eugenicists like Pearson and Fisher, who introduced techniques like the correlation coefficient and the normal distribution, the research focus shifted from the intensive study of individuals to the study of aggregates from large groups. The label “nomothetic” came to stand for group-based research, and instead of focusing on what is common to all, analyses became focused on what is true “on average” (10, 15).1 Although seemingly old-fashioned, several useful findings have sprouted from the study of individuals, for example from quantitative research by Ebbinghaus, Pavlov, Thorndike, Watson, and Shapiro, and qualitative research by Broca, James, Freud, Alzheimer, and Piaget, which has been described elegantly elsewhere (10, 17, 18). One of the most famous adepts of the single-subject approach was Burrhus Skinner, who said that he would rather study one rat for a thousand times than a thousand rats for 1 h each (19). Skinner studied how animal subjects (such as pigeons or rats) acquired certain behaviors in response to stimuli by rewarding or punishing the animal (20). This work on operant conditioning revealed important knowledge of human behavior that is still applied nowadays for addressing clinical problems, such as the treatment of addiction (21), and the development of cognitive behavioral and operant behavioral therapies (22). In medical sciences, single-subject studies are still used occasionally, for example to examine the benefits or side effects of a drug in individual patients. Such “n-of-1 trials” have shown their potential in terms of deciding whether a specific intervention works for a specific individual patient [e.g., (23–26)].
Although they are still relatively rare, in recent years single-subject studies have been more frequently used in the field of psychiatry, possibly due to innovations in ecological momentary assessment (EMA), analytic methods, and technologies (28, 29). Single-subject studies in the field of psychiatry have been applied for several reasons (see Figure 2). First, observational single-subject studies have been done to evaluate temporal associations between variables that may be important in processes underlying psychiatric disorders [e.g., (30–33)]. Such studies can yield very useful information about potential risk and protective factors at the individual level, which can increase scientific as well as clinical insight. Some recent studies have elaborated on this, and used single-subject research for developing personalized diagnostics (34–40) and person-tailored treatment advice (41–44). Another applicability of observational single-subject studies is the examination of the temporal dynamics of single variables, such as the variability or inertia (or autocorrelation, i.e., the degree to which successive observations are related to each other). For instance, Wichers et al. revealed that an increase in autocorrelation in negative affect preceded a relapse of depression in a single patient (45). Thus, single-subject studies can yield information that can be used for detecting psychopathological changes or early warning signals. Another application of the single-subject study is to evaluate the effects and side effects of interventions in single individuals. In the field of psychiatry such experimental single-subject studies have evaluated, for example, the person-specific effects of individualized cognitive therapy for depression in women with metastatic cancer (46), pharmacological treatment for depression (47), stimulants for ADHD in children (48), and treatments for schizophrenia (49). Taken together, single-subject studies are and can be used for different reasons in the field of psychiatry.
Despite a small recent increase in the use of the single-subject study design, it is still relatively scarce in the field of psychiatry. This is remarkable in a field that typically has to deal with a lot of inter-individual heterogeneity and intra-individual variability, and in which there is a high demand for results that apply to individual patients. We believe that this is because of a lack of awareness of the value of single-subject studies rather than a lack of usefulness or feasibility. We will discuss several facts and fictions regarding single-subject research, in order to resolve some existing misconceptions about single-subject studies and make the reader more aware of their value.
Facts and Fictions
We will now describe several statements that are often heard from, for example, reviewers, members of ethical boards, funding agencies, and colleague researchers. For each statement we will explain whether we think it is a fact or fiction, and elaborate on this.
Statement 1. Single-Subject Studies Are Case Reports and Therefore Have No Scientific Value
Fiction
Although single-subject and case reports both focus on individuals (i.e., are both idiographic; see Figure 2), there are some major differences. A case report is the presentation of an interesting observation on a patient by the treating specialist that lacks a pre-conceived design and systematic assessments. Although case reports may be very informative for generating hypotheses, the lack of systematic design elements makes them prone to bias and invalid inference (14, 50). For instance, a clinician may observe improvement in a depressed patient after a certain therapy [e.g., (51)] and may attribute this improvement to the therapy, while in reality it was due to something else or a spontaneous recovery. Experimental single-subject studies have specific design elements that help elucidate whether the improvement is really due to the therapy. They have a pre-conceived design with different cross-over periods (intervention and control) and pre-planned assessments, often done with validated instruments by an independent researcher [for guidelines see (14, 17, 50, 52)].
Observational single-subject studies are also characterized by a pre-planned design and systematic assessments of outcomes, making them valuable for scientific research and clinical diagnostics. For example, a patient might want to know whether he generally feels more depressed after seeing his mother in law. A single-subject observational study can answer questions about such dynamic associations between variables within one individual, which may go undetected in the clinical care setting.
Thus, if a single-subject study is set up properly and has enough observations to allow for statistical inference, it can yield valid and reliable scientific and clinical evidence (13, 17, 23).
Statement 2. The Sample Size of Single-Subject Studies Is Too Small to Yield Enough Statistical Power
Fiction
An often-heard objection is that the sample size of single-subject studies is too small. However, in single-subject studies time-series data are analyzed for each individual separately. Because of this, the power in single-subject studies depends on the number of repeated observations within a person instead of the number of persons. Thus, if the number of repeated observations within the individual is large enough for the planned statistical analysis, the power is sufficient. A variety of statistical methods exists for the analysis of single-subject data [e.g., (29, 53, 54)].
Statement 3. The Sample Size of Single-Subject Studies Is Often Too Small to Generalize Findings to the Population
Fact
While power of a single-subject study can be sufficient even if n = 1 (see Statement 2), the sample size of single-subject studies is still relevant for generalizability to the population. One can only generalize findings to a population if it can be demonstrated that the principle holds in all, or a large majority, of a representative sample, which is not possible if n = 1. However, in order to generalize the results of single-subject studies, multiple single-subject studies can be performed in individuals of the same population (direct replication), or in different settings or populations (systematic replication) (55, 56). Results of multiple single-subject studies may subsequently be summarized using for instance meta-analysis (24, 57, 58). If the ultimate goal is to gather scientific evidence concerning questions that apply to individual persons, researchers can build a body of single-case work, eventually leading to a large sample.
Statement 4. Group-Based Studies Are More Suitable Than Single-Subject Studies to Find Out What Is True in General
Fiction
Results from group-based studies yield information about what is “true on average” and typically end up in standardized treatment guidelines. However, in the presence of large inter- and intra-individual differences, average effects are not informative on whether a result is “true in general” (i.e., present in the majority of the sample) (15). For instance, mood disorders are on average associated with higher cortisol levels at the group level (59), but this does not necessarily mean that worse mood is associated with higher cortisol levels in the majority of individuals. A single-subject study repeated in 30 individuals found great individual differences in the within-subject association between mood and cortisol levels (31). Thus, group-aggregated, averaged results are not necessarily or very likely true for each individual in that group. Moreover, the average may also be a poor reflection of what is true for most individuals in the group, for example if the distribution of parameters is bimodal or trimodal (60). Also creating subgroups may not solve that problem, because we often do not know by which characteristics we must define subgroups.
More formally, it has been shown that results obtained from group-based studies can only be generalized to individuals when the assumption of “ergodicity” is met. Ergodicity implies that the average, variance, covariance and lagged covariance between variables should be the same for all individuals (homogeneity), and that no changes over time in these statistical characteristics should be present (stationarity) (9, 61, 62). In the absence of ergodicity, effects calculated at the group level, or even at a more homogeneous subgroup level, will not generalize to the individual level (7, 9, 12, 15, 63–65). An association found at the (sub) group level may be weaker, stronger, absent or even reversed in an individual (61). In fact, group-aggregated results may sometimes not even apply to a single individual in that group (10, 15).
Statement 5. Confounding in Single-Subject Studies Is the Same as in Group-Based Studies
Fiction
A point frequently raised by reviewers is that analyses of single-subject studies should be adjusted for relevant demographic or clinical variables. Indeed, these type of variables may confound associations in group-based studies because they may differ between individuals (between-subjects confounding). However, in single-subject studies all variance in the outcome is due to within-person variance in other variables, which may include changes in environmental variables, events, lifestyle- or other behaviors, treatments, etc. (61, 62). Therefore, in single-subject studies variables can only confound an association if they vary within the individual over time (within-subject confounding). Variables that do not show fluctuations over time, such as sex or a stable somatic condition, need (and can) not be adjusted for in single-subject studies. While in group-based studies both between- and within-subjects confounding may occur, in single-subject studies only within-subject confounding may occur. Only if one reverts to a group approach, for example by combining results from multiple single-subject studies, group-level covariates will become applicable again.
Statement 6. A Single-Subject Study Cannot Establish Causality
Fact
It is not possible to establish causality using a single-subject design. Moreover, this is also true for group-based study designs. Hypothetically, the ideal experiment to determine the causal effect of a certain treatment would be to expose an individual to this treatment, observe what happens, and then go back in time and expose the same individual to another condition (i.e., no treatment or placebo), all other things being equal (25, 66). Of course, such a design is not possible as we cannot go back in time. But interestingly, certain forms of single-subject studies come close to this ideal experimental design: the n-of-1 randomized controlled trials (n-of-1 RCTs). In these trials, various conditions (e.g., treatment and placebo, or medicines with varying dosages) are alternated over time and the order of exposure is determined randomly. In this way, each individual serves as his or her own control. The strength of the design increases if multiple cross-over periods are used and patients as well as clinicians and researchers are blinded to the treatment order. A few studies in the field of psychiatry have used such designs successfully to examine the impact of interventions in single patients [e.g., (47–49, 67, 68)]. The deviation of this n-of-1 RCT from the ideal counterfactual experiment is small and concerns only the fact that the individual may have changed over time. In the traditional group-based RCT, groups of people are compared with each other, assuming these groups are similar. This latter assumed similarity is a stronger assumption, and generally not true (69) than the assumption that an individual is similar to him- or herself somewhat earlier in time (66). Furthermore, n-of-1 RCTs often include patients that do not meet the highly selective inclusion criteria of group-based RCTs, and thus provide information for patients for whom there is currently a lack of evidence for treatment efficacy (47). Thus, the n-of-1 RCT appears at least as ideal as the group-based RCT to establish causality, and therefore deserves more attention in the scientific field (25, 66, 70).
In addition to the n-of-1 RCT, other forms of single-subject studies, such as ABA-designs or observational single-subject studies can also contribute information that is important for establishing causality, including strength of the association, consistency of the association in different contexts and times, specificity of the association, temporal precedence, and dose-response relationship (71, 72). Because an individual serves as his or her own control in all single-subject studies, it is possible to determine in a systematic way the strength, consistency, specificity and dose-response relation of an association within that individual. Furthermore, because of the multitude of repeated assessments in single-subject studies, the temporal order of associations can be revealed (30, 31, 73–75). For example, it can be revealed that changes in certain factors systematically precede changes in other variables (i.e., temporal precedence). Even though causality can probably never be completely established, these aspects of single-subject studies are particularly helpful to approach valid causal inference. Also sophisticated approaches to establish causal inference via Directed Acyclic Graphs and Structural Causal models can be applied to single-subject models (76, 77), although these have very strict assumptions that are very hard to meet in practice (77).
Statement 7. Single-Subject Research Is a Lot of Work
Fact
An often-heard statement about single-subject research is that it needs a lot of effort, which is true. Single-subject research involves frequent/repeated assessment during a relatively long period of time, which is time consuming and effortful for both the participant, the researcher, and for therapists if they are involved. However, due to recent technical innovations and increased smartphone and sensor use, collecting ambulatory time-series data has become more feasible. The feasibility has been shown for healthy individuals (37), older adults (78, 79), and also for patients with psychiatric disorders such as severe depression or bipolar disorder (80), panic disorder (36), psychosis (81), eating disorders (82) or ADHD (83). Data collection via a smartphone is more convenient for the participant than paper-and-pencil methods and makes laborious and error-prone data entry unnecessary. The researcher mainly has to focus on data cleaning, statistical analysis and (optionally) feedback generation, for which nowadays more and more automated algorithms are being developed [e.g., (84, 85)].
Single-subject research is also more feasible when participating in a single-subject study is rewarding for participants. For instance, revealing the personal treatment effects or optimal dosage of a certain drug (47), or giving diagnostic information through a personalized feedback report (37, 41, 43) appeared particularly motivating to increase compliance in clinical samples. Feedback reports can contain descriptive feedback [e.g., (41)] or information about potential triggers of symptoms based on statistical models [e.g., (36, 37)]. Although there are still many challenges that need to be resolved (86), personalized feedback may reveal valuable insights for patients and thus help to motivate them to complete the study.
Statement 8. One Can Just as Well Use Multilevel Modeling in Order to Analyze Data of a Group of Individuals
Fiction
In single-subject research, time series of each individual are analyzed separately. But why not use multilevel modeling instead? Multilevel modeling is a well-known tool for analyzing longitudinal data collected in multiple persons, that also allows to study within-person associations. While this is true, multilevel methods still yield group-aggregated results. The fixed effects, which are usually the main outcome of interest, represent the average effect in the group. The fixed effects are a mix of within- and between-person effects, but person-mean centering of the predictors can be applied if we are interested in within-person associations[(87); for examples of such studies, see (88–94)]. However, such analyses still yield average within-person associations. As discussed in Statement 4, the average may not reflect what is true in general, i.e., for the majority of individuals. If there is large heterogeneity in the sample, for example if the distribution of parameters is bimodal or trimodal, or if the functional form of the model differs across individuals, the fixed effects will be a poor reflection of what holds for individuals (60, 95, 96). As a corollary, also the random effects (the inter-individual differences in the effects) may not be appropriate. Random effects are post-hoc estimated deviations from the average effects, and are assumed to be normally distributed around these averages. If the latter are inaccurate, so will be the random effects (60, 95, 96).
Furthermore, while person-mean centering is useful for disaggregating between- and within-person effects of the predictors, with respect to other model features within- and between-person variance is more difficult to separate (for example, the error covariance matrix) (97, 98). Recently, new models like Dynamic Structural Equation Modeling (99), or Bayesian Dynamic Modeling (96, 100, 101) do offer increased possibilities to adequately model other model features within a multilevel framework. However, with increased model complexity, for example with multiple interactions, feedback loops, or non-linear effects, the problem of disaggregating within- and between-person variance in multilevel models becomes quite difficult. Analyzing data at the individual level leaves more room for modeling such complexities (56, 95, 96).
Nevertheless, there are situations in which multilevel modeling is the preferred statistical method. Multilevel models have the advantage that they can “borrow strength” from the data of other individuals (95, 101). This may be a great advantage if individual time-series data are noisy, the number of repeated measures is low, or the sample is homogeneous. In such cases, multilevel models will yield better estimates than single-subject analyses. A replicated single-subjects approach may be the statistical method of choice in case of large heterogeneity, many repeated observations, or high complexity (33, 56, 60, 95, 96, 101).
Discussion
Despite the relatively high demand for information applying to individual persons, the single-subject study is not often used in the field of psychiatry. We believe that this is because of a lack of awareness of their value rather than a lack of usefulness or feasibility. In the present paper we aimed to resolve some common misconceptions and beliefs about single-subject studies by discussing some commonly heard facts and fictions.
Single-subject studies can be particularly useful and have additional value in several situations. For example, when there are large inter-individual differences in the processes under study, when these processes are very complex or nonlinear, or can change over time. Additionally, single-subject studies have the advantage that they may include any patient (also those with complex or rare diseases), and (in the case of experimental single-subject studies) are able to adjust the treatment when deemed necessary (102, 103). This increases the ecological validity of the single-subject study, and makes it particularly useful in situations when large-group studies are not feasible; for instance because the disease or event under study is rare, the patient is complex (e.g., many comorbidities), the setting is complex (e.g., palliative care), the intervention is highly expensive or controversial, or the intervention contains person-specific elements (46, 56, 102, 104, 105). Furthermore, single-subject studies may involve patients more in their treatment process, thereby increasing patient empowerment and shared decision making (68). The single-subject study may also contribute in the diagnostic process, to evaluate factors contributing to treatment responses, or to evaluate efficacy of certain treatments (34, 38–41, 43, 44). Practically, this has led to the recent development of algorithms and clinical care applications that implement single-subject analyses in the diagnostic process in clinical care settings [(42, 44, 106) conference abstract]. Single-subject studies can also be used to obtain a detailed description of a particular approach applied to an individual in order to test an existing clinical theory [theory exemplification; (107)]. While group-based studies often only study a limited number of aspects belonging to a theory, a single-subject study can map in detail all its aspects together in one person. For example, a single-subject study could detail all processes underlying response to cognitive therapy in a specific patient [e.g. (108)], thereby showing how to optimally apply an existing theory underlying cognitive therapy. Complex statistical models can be linked to processes underlying psychiatric disorders [for example how a panic attack evolves in a specific patient, (109)], which may help in understanding their mechanism in individual patients.
Despite these advantages of single-subject studies, group-based studies are more appropriate if one wants to make inferences about average tendencies in the population, such as the prevalence, incidence or average risk of a disorder, or the average effect of certain treatments in the whole population or a certain subpopulation. For instance, if one wants to know whether legalizing cannabis helps in reducing the prevalence or incidence of psychotic disorders in the population. Moreover, group-based studies are more appropriate if individual time-series data are very short or noisy, or the process under study is homogeneous across individuals (33, 95, 101). Thus, group-based and single-subject studies can both be useful and are appropriate in different situations.
In some circumstances, the group-based and single-subject approach may be combined. A first reason for combining these designs is to identify commonalities across persons, in order to increase generalizability to the population. Practically this can be done by combining group- and individual-level analyses in one model, for example using Group Iterative Multiple Model Estimation [GIMME (60)], or meta-analytical techniques for pooling data from multiple single-subject studies (57, 58, 110). Related to this, pooling data from multiple single-subject studies can be used to link individual-level results to certain between-subjects characteristics. For instance, results from multiple experimental single-subject studies may be pooled and linked to patient characteristics in order to identify which patient characteristics are associated with better outcomes of a certain treatment (103). Likewise, data from multiple observational single-subject studies may be pooled in order to identify whether person-specific associations between variables can be linked to certain patient characteristics such as the presence of a depressive disorder [e.g. (31, 73, 111)], or different severity of depressive symptoms (112). Another reason to combine the single-subject with the group-based approach would be to examine the group-level effectiveness of an individualized treatment or lifestyle advice that is based on single-subject analyses of diary observations [e.g., (43, 44)]. In this way, the single-subject study design can be of added value to the increasing urge for personalized patient care in mental health care settings (29, 113–115).
Conclusion
In the field of psychiatry, single-subject studies are still relatively scarce. We hope that we have resolved some misunderstandings surrounding single-subject studies and have increased the reader's awareness of possibilities and impossibilities of the single-subject design. Although single-subject studies are definitely not suitable in all circumstances, we believe that they deserve more attention in the field of psychiatry, especially in view of the current urge for personalized patient care, increased importance of shared decision making, increased availability of electronic devices and sensors, and recent advancements in analytic methods for time-series data.
Author Contributions
MZ, HR, ES, SB, MW, and EB: conceptualization, review, and editing. ES, SB, and MW wrote sections of the manuscript. MZ, HR, and EB: wrote first draft of major parts of the manuscript. All authors contributed to the article and approved the submitted version.
Funding
MW received an ERC consolidator grant (project No. 681466; TRANS-ID, 2015). HR received a grant of the Foundation VCVGZ (Stichting tot Steun VCVGZ, grant no. 239). HR and MW received a grant of the Innovatiefonds De Friesland (grant no. DS81). The sponsors had no role in the design and content of the present paper.
Conflict of Interest
The authors declare that the research was conducted in the absence of any commercial or financial relationships that could be construed as a potential conflict of interest.
Acknowledgments
We thank Dr. Conradi and Prof. Dr. de Jonge for using the data of the INSTEL study. This project was initiated by the iLab of the Department of Psychiatry, University Medical Center Groningen, Groningen, Netherlands (http://ilab-psychiatry.nl).
Footnote
1. ^In view of the ambiguous meaning of the word “nomothetic,” we use the term “group-based” throughout the paper when we refer to a study that focuses on group-aggregated results.
References
1. Turner EH, Matthews AM, Linardatos E, Tell RA, Rosenthal R. Selective publication of antidepressant trials and its influence on apparent efficacy. N Engl J Med. (2008) 358:252–60. doi: 10.1056/NEJMsa065779
2. Howren MB, Lamkin DM, Suls J. Associations of depression with C-reactive protein, IL-1, and IL-6: a meta-analysis. Psychosom Med. (2009) 71:171–86. doi: 10.1097/PSY.0b013e3181907c1b
3. Ozomaru U, Wahlestedt C, Nemeroff CB. Personalized medicine in psychiatry: problems and promises. BMC Med. (2013) 11:132. doi: 10.1186/1741-7015-11-132
4. Fernandes BS, Williams LM, Steiner J, Leboyer M, Carvalho AF, Berk M. The new field of ‘precision psychiatry'. BMC Med. (2017) 15:80. doi: 10.1186/s12916-017-0849-x
5. Conradi HJ, de Jonge P, Kluiter H, Smit A, Van der Meer K, Jenner JA, et al. Enhanced treatment for depression in primary care: long-term outcomes of a psycho-educational prevention program alone and enriched with psychiatric consultation or cognitive behavioral therapy. Psychol Med. (2007) 37:849–62. doi: 10.1017/S0033291706009809
6. Estes WK, Maddox WT. Risks of drawing inferences about cognitive processes from model fits to individual versus average performance. Psychonomic Bull Rev. (2005) 12:403–8. doi: 10.3758/BF03193784
7. Hamaker EL, Dolan CV, Molenaar PCM. Statistical modeling of the individual: Rationale and application of multivariate stationary time series analysis. Multivariate Behav Res. (2005) 40:207–33. doi: 10.1207/s15327906mbr4002_3
8. Navarro DJ, Griffiths TL, Steyvers M, Lee MD. Modeling individual differences using Dirichlet Processes. J Math Psychol. (2006) 50:101–22. doi: 10.1016/j.jmp.2005.11.006
9. Molenaar PCM, Campbell CG. The new person-specific paradigm in psychology. Curr Dir Psychol Sci. (2009) 18:112–7. doi: 10.1111/j.1467-8721.2009.01619.x
10. Robinson OC. The idiographic/nomothetic dichotomy: tracing historical origins of contemporary confusions. History Philos Psychol. (2011) 13:32–9.
11. Liew SX, Howe PDL, Little DR. The appropriacy of averaging in the study of context effects. Psychon Bull Rev. (2016) 23:1639–46. doi: 10.3758/s13423-016-1032-7
12. Fisher AJ, Medaglia JD, Jeronimus BF. Lack of group-to-individual generalizability is a threat to human subjects research. Proc Natl Acad Sci USA. (2018) 115:E6106–15. doi: 10.1073/pnas.1711978115
13. Hilliard RB. Single-case methodology in psychotherapy process and outcome research. J Consult Clin Psychol. (1993) 61:373–80. doi: 10.1037/0022-006X.61.3.373
15. Lamiell JT. “Nomothetic” and “idiographic”: Contrasting Windelband's understanding with contemporary usage. Theory Psychol. (1998) 8:23–38. doi: 10.1177/0959354398081002
16. Little DR, Smith PL. Replication is already mainstream: Lessons from small-N designs. Behav Brain Sci. (2018) 41:e141. doi: 10.1017/S0140525X18000766
17. Cohen LL, Feinstein A, Masuda A, Vowles KE. Single-case research design in pediatric psychology: considerations regarding data analysis. J Pediatr Psychol. (2014) 39:124–37. doi: 10.1093/jpepsy/jst065
18. Barlow DH, Nock MK. Why can't we be more idiographic in our research? Perspect Psychol Sci. (2009) 4:19–21. doi: 10.1111/j.1745-6924.2009.01088.x
19. Skinner BF. Operant behavior. In: Honig WK, editor. Operant Behavior: Areas of Research and Application. New York, NY: Appleton-Century-Crofts (1966). p.12–32.
21. Silverman K. Exploring the limits and utility of operant conditioning in the treatment of drug addiction. Behavior Analyst. (2004) 27:209–30. doi: 10.1007/BF03393181
22. Rachman S. The evolution of behaviour therapy and cognitive behaviour therapy. Behav Res Ther. (2015) 64:1–8. doi: 10.1016/j.brat.2014.10.006
24. Stunnenberg BC, Woertman W, Raaphorst J, Statland JM, Griggs RC, Timmermans J. Combined n-of-1 trials to investigate mexiletine in non-dystrophic myotonia using a Bayesian approach; study rationale and protocol. BMC Neurol. (2015) 15:43. doi: 10.1186/s12883-015-0294-4
25. Madhok V, Fahey T. N-of-1 trials: an opportunity to tailor treatment in individual patients. Br J Gen Pract. (2005) 55:171–2.
26. Mahon J, Laupacis A, Donner A, Wood T. Randomised study of n of 1 trials versus standard practice. BMJ. (1996) 312:1069–74. doi: 10.1136/bmj.312.7038.1069
28. Hamaker EL, Wichers M. No time like the present: discovering the hidden dynamics in intensive longitudinal data. Curr Dir Psycholog Sci. (2017) 26:10–5. doi: 10.1177/0963721416666518
29. Wright AGC, Woods WC. Personalized models of psychopathology. Annu Rev Clin Psychol. (2020) 16:49–74. doi: 10.1146/annurev-clinpsy-102419-125032
30. Rosmalen JGM, Wenting AM, Roest AM, De Jonge P, Bos EH. Revealing causal heterogeneity using time series analysis of ambulatory assessments: application to the association between depression and physical activity after myocardial infarction. Psychosom Med. (2012) 74:377–86. doi: 10.1097/PSY.0b013e3182545d47
31. Booij SH, Bos EH, de Jonge P, Oldehinkel AJ. The temporal dynamics of cortisol and affective states in depressed and non-depressed individuals. Psychoneuroendocrinology. (2016) 69:16–25. doi: 10.1016/j.psyneuen.2016.03.012
32. Cheung YK, Hsueh PS, Qian M, Yoon S, Meli L, Diaz KM, et al. Are nomothetic or ideographic approaches superior in predicting daily exercise behaviors? Methods Inf Med. (2017) 56:452–60. doi: 10.3414/ME16-02-0051
33. Rozet A, Kronish IM, Schwartz JE, Davidson KW. Using machine learning to derive just-in-time personalized predictors of stress: observational study bridging the gap between nomothetic and ideographic approaches. J Med Internet Res. (2019) 21:e12910. doi: 10.2196/12910
34. Bak M, Drukker M, Hasmi L, van Os J. An n=1 clinical network analysis of symptoms and treatment in psychosis. PLoS ONE. (2016) 11:e0162811. doi: 10.1371/journal.pone.0162811
35. Fisher AJ, Reeves JW, Lawyer G, Medaglia JD, Rubel JA. Exploring the idiographic dynamics of mood and anxiety via network analysis. J Abnorm Psychol. (2017) 126:1044–56. doi: 10.1037/abn0000311
36. Kroeze R, van der Veen DC, Servaas MN, Bastiaansen JA, Oude Voshaar RC, Borsboom D, et al. Personalized feedback on symptom dynamics of psychopathology: a proof-of-principle study. J Person Oriented Res. (2017) 3:1–10. doi: 10.17505/jpor.2017.01
37. Van der Krieke L, Blaauw FJ, Emerencia AC, Schenk HM, Slaets JP, Bos EH, et al. Temporal dynamics of health and well-being: a crowdsourcing approach to momentary assessments and automated generation of personalized feedback. Psychosom Med. (2017) 79:213–23. doi: 10.1097/PSY.0000000000000378
38. David SJ, Marshall AJ, Evanovich EK, Mumma GH. Intraindividual dynamic network analysis – implications for clinical assessment. J Psychopathol Behav Assess. (2018) 40:235–48. doi: 10.1007/s10862-017-9632-8
39. Epskamp S, van Borkulo CD, van der Veen DC, Servaas MN, Isvoranu AM, Riese H, et al. Personalized network modeling in psychopathology: the importance of contemporaneous and temporal connections. Clin Psychol Sci. (2018) 6:416–27. doi: 10.1177/2167702617744325
40. Voigt ALA, Kreiter DJ, Jacobs CJ, Revenich EGM, Serafras N, Wiersma M, et al. Clinical network analysis in a bipolar patient using an experience sampling mobile health tool: An n=1 study. Bipolar Disord. (2018) 4:1. doi: 10.4172/2472-1077.1000121
41. Kramer I, Simons CJ, Hartmann JA, Menne-Lothmann C, Viechtbauer W, Peeters F, et al. A therapeutic application of the experience sampling method in the treatment of depression: a randomized controlled trial. World Psychiatry. (2014) 13:68–77. doi: 10.1002/wps.20090
42. Fernandez KC, Fisher AJ, Chi C. Development and initial implementation of the Dynamic Assessment Treatment Algorithm (DATA). PLoS ONE. (2017) 12:e0178806. doi: 10.1371/journal.pone.0178806
43. Van Roekel E, Vrijen C, Heininga VE, Masselink M, Bos EH, Oldehinkel AJ. An exploratory randomized controlled trial of personalized lifestyle advice and tandem skydives as means to reduce anhedonia. Behav Ther. (2017) 48:76–96. doi: 10.1016/j.beth.2016.09.009
44. Fisher AJ, Bosley HG, Fernandez KC, Reeves JW, Soyster PD, Diamond AE, et al. Open trial of a personalized modular treatment for mood and anxiety. Behav Res Ther. (2019) 116:69–79. doi: 10.1016/j.brat.2019.01.010
45. Wichers M, Groot PC, Psychosystems, ESM Group EWS Group. (2016). Critical slowing down as a personalized early warning signal for depression. Psychother. Psychosom. 85:114–6. doi: 10.1159/000441458
46. Lévesque M, Savard J, Simard S, Gauthier JG, Ivers H. Efficacy of cognitive therapy for depression among women with metastatic cancer: a single-case experimental study. J Beh Ther Exp Psychiatry. (2004) 35:287–305. doi: 10.1016/j.jbtep.2004.05.002
47. Kronish IM, Hampsey M, Falzon L, Konrad B, Davidson KW. Personalized (N-of-1) trials for depression. A systematic review. Clin Psychopharmacol. (2018) 38:218–25. doi: 10.1097/JCP.0000000000000864
48. Mordijck E, Danckaerts M, Onghena P. [N-of-1 trials in child and adolescent psychiatry: a closer look at stimulants]. Tijdschrift Voor Psychiatrie. (2018) 60:315–25.
49. Marwick KFM, Stevenson AJ, Davies C, Lawrie SM. Application of n-of-1 treatment trials in schizophrenia: systematic review. Br J Psychiatry. (2018) 213:398–403. doi: 10.1192/bjp.2018.71
50. Kravitz RL, Duan N, (eds) the DEcIDE Methods Center N-of-1 Guidance Panel (Duan N Eslick I Gabler NB Kaplan HC Kravitz RL Larson EB.). Design and Implementation of N-of-1 Trials: A User's Guide. AHRQ Publication No. 13(14)-EHC122-EF. Rockville, MD: Agency for Healthcare Research and Quality (2014).
51. Tavormina R, Tavormina MGM. Overcoming depression with dance movement therapy: a case report. Psychiatria Danubina. (2018) 30:515–20.
52. Vohra S, Shamseer L, Sampson M, Bukutu C, Schmid CH, Tate R, et al. CONSORT extension for reporting N-of 1 trials (CENT) 2015: explanation and elaboration. J Clin Epidemiol. (2016) 76:9–17. doi: 10.1016/j.jclinepi.2015.05.004
53. Hamaker EL, Ceulemans E, Grasman RPPP, Tuerlinckx F. Modeling affect dynamics: state of the art and future challenges. Emot Rev. (2015) 7:316–22. doi: 10.1177/1754073915590619
54. Velicer WF, Molenaar P. Time series analysis. Research methods in psychology. In: Schinka J, Velicer WF, editors. Volume 2 of Handbook of Psychology (I. B. Weiner, Editor-in-Chief). 2nd ed. New York, NY: John Wiley & Sons (2013). p. 628–60.
55. Ottenbacher KJ, Hinderer SR. Evidence-based practice: methods to evaluate individual patient improvement. Am J Phys Med Rehabil. (2001) 80:786–96. doi: 10.1097/00002060-200110000-00014
56. Smith PL, Little DR. Small is beautiful: in defense of the small-N design. Psychonomic Bull Rev. (2018) 25:2083–101. doi: 10.3758/s13423-018-1451-8
57. Zucker DR, Schmid CH, McIntosh MW, D'Agostino RB, Selker HP, Lau J. Combining single patient (N-of-1) trials to estimate population treatment effects and to evaluate individual patient responses to treatment. J Clin Epidemiol. (1997) 50:401–10. doi: 10.1016/S0895-4356(96)00429-5
58. Huber AM, Tomlinson GA, Koren G, Feldman BM. Amitriptyline to relieve pain in juvenile idiopathic arthritis: a pilot study using Bayesian meta-analysis of multiple n-of-1 clinical trials. J Rheumatol. (2007) 34:1125–32.
59. Stetler C, Miller GE. Depression and hypothalamic-pituitary-adrenal activation: a quantitative summary of four decades of research. Psychosom Med. (2011) 73:114–26. doi: 10.1097/PSY.0b013e31820ad12b
60. Gates KM, Molenaar PC. Group search algorithm recovers effective connectivity maps for individuals in homogeneous and heterogeneous samples. Neuroimage. (2012) 63:310–9. doi: 10.1016/j.neuroimage.2012.06.026
61. Hamaker EL. Why researchers should think ‘within-person': a paradigmatic rationale. In: Mehl MR, Conner TS, editors. Handbook of Research Methods for Studying Daily Life. New York, NY: Guilford Press (2012). p. 43–61.
62. Molenaar PCM. A manifesto on psychology as idiographic science: Bringing the person back into scientific psychology, this time forever. Measurement. (2004) 2:201–18. doi: 10.1207/s15366359mea0204_1
63. Cattell RB. Patterns of change: measurement in relation to state-dimension, trait change, lability, and process concepts. In: Handbook of Multivariate Experimental Psychology. Chicago: Rand McNally (1966). p. 355–402.
64. Nesselroade JR. Interindividual differences in intraindividual change. In Collins LM, Horns JL, editors. Best Methods for the Analysis of Change: Recent Advances, Unanswered Questions, Future Directions. Washington DC: American Psychological Association (1991). p. 92–105.
65. Kievit RA, Frankenhuis WE, Waldorp LJ, Borsboom D. Simpson's paradox in psychological science: a practical guide. Front Psychol. (2013) 4:513. doi: 10.3389/fpsyg.2013.00513
66. Vandenbroucke JP. De N = 1 trial, de meest ideale onderzoeksopzet, die te weinig wordt gebruikt. Ned Tijdschr Geneeskd. (2006) 150:2794–5.
67. Kent MA, Camfield CS, Camfield PR. Doube-blind methylphenidate trials – practical, useful, and highly endorsed by families. Arch Pediatr Adolesc Med. (1999) 153:1292–6. doi: 10.1001/archpedi.153.12.1292
68. Nikles JC, Mitchell GK, DelMar CB, Clavarino A, McNairn N. An n-of-1 trial service in clinical practice: testing the effectiveness of stimulants for attention-deficit/ hyperactivity disorder. Pediatrics. (2006) 117:2040–6. doi: 10.1542/peds,.2005-1328
69. Saint-Mont U. Randomization does not help much, comparability does. PLoS ONE. (2015) 10:e0132102. doi: 10.1371/journal.pone.0132102
70. OCEBM Levels of Evidence Working Group. The Oxford 2011 Levels of Evidence. Oxford Centre for Evidence-Based Medicine. Available online at: https://www.cebm.net/wp-content/uploads/2014/06/CEBM-Levels-of-Evidence-2.1.pdf (assessed January 13, 2020).
71. Hill AB. The environment and disease: association or causation? Proc R Soc Med. (1965) 58:295–300. doi: 10.1177/003591576505800503
72. Kraemer HC, Kazdin AE, Offord DR, Kessler RC, Jensen PS, Kupfer DJ. Coming to terms with the terms of risk. Arch Gen Psychiatry. (1997) 54:337–43. doi: 10.1001/archpsyc.1997.01830160065009
73. Stavrakakis N, Booij SH, Roest AM, De Jonge P, Oldehinkel AJ, Bos EH. Temporal dynamics of physical activity and affect in depressed and nondepressed individuals. Health Psychol. (2015) 34S:1268–77. doi: 10.1037/hea0000303
74. Snippe E, Bos EH, Van der Ploeg K, Sanderman R, Fleer J, Schroevers MJ. Time-series analysis of daily changes in mindfulness, repetitive thinking, and depressive symptoms during a mindfulness-based treatment. Mindfulness. (2015) 6:1053–62. doi: 10.1007/s12671-014-0354-7
75. Hoenders HR, Bos EH, De Jong JT, De Jonge P. Temporal dynamics of symptom and treatment variables in a lifestyle-oriented approach to anxiety disorder: a single-subject time-series analysis. Psychother Psychosom. (2012) 81:253–5. doi: 10.1159/000335928
76. Pearl J, Glymour M, Jewell NP. Causal Inference in Statistics: A Primer. Chichester: John Wiley & Sons (2016).
77. Dablander F. An Introduction to Causal Inference. (2019). Available online at: https://fabiandablander.com/r/Causal-Inference.html (accessed October 20, 2020).
78. Ramsey AT, Wetherell JL, Depp C, Dixon D, Lenze E. Feasibility and acceptability of smartphone assessment in older adults with cognitive and emotional difficulties. J Technol Hum Serv. (2016) 34:209–23. doi: 10.1080/15228835.2016.1170649
79. Maher JP, Rebar AL, Dunton GF. Ecological momentary assessment is a feasible and valid methodological tool to measure older adults' physical activity and sedentary behavior. Front Psychol. (2018) 9:1485. doi: 10.3389/fpsyg.2018.01485
80. Aan Het Rot M, Hogenelst K, Schoevers RA. Mood disorders in everyday life: a systematic review of experience sampling and ecological momentary assessment studies. Clin Psychol Rev. (2012) 32:510–23. doi: 10.1016/j.cpr.2012.05.007
81. Niendam TA, Tully LM, Iosif AM, Kumar D, Nye KE, Denton JC, et al. Enhancing early psychosis treatment using smartphone technology: a longitudinal feasibility and validity study. J Psychiatr Res. (2018) 96:239–46. doi: 10.1016/j.jpsychires.2017.10.017
82. Haedt-Matt AA, Keel PK. Revisiting the affect regulation model of binge eating: a meta-analysis of studies using ecological momentary assessment. Psychol Bull. (2011) 137:660–81. doi: 10.1037/a0023660
83. Miguelez-Fernandez C, de Leon SJ, Baltasar-Tello I, Penuelas-Calvo I, Barrignon ML, Capdevila AS, et al. Evaluating attention-deficit/hyperactivity disorder using ecological momentary assessment: a systematic review. Atten. Defic. Hyperact. Disord. (2018) 10:247–65. doi: 10.1007/s12402-018-0261-1
84. Van der Krieke L, Emerencia AC, Bos EH, Rosmalen JGM, Riese H, Aiello M, et al. Ecological momentary assessments and automated time series analysis to promote tailored health care: a proof-of-principle study. JMIR Res Protoc. (2015) 4:e100. doi: 10.2196/resprot.4000
85. Blaauw FJ, Schenk HM, Jeronimus BF, Van der Krieke L, De Jonge P, Aiello M, et al. Let's get Physiqual – An intuitive and generic method to combine sensor technology with ecological momentary assessments. J of Biomed Inform. (2016) 63:141–9. doi: 10.1016/j.jbi.2016.08.001
86. Wichers M, Snippe E, Riese H, Bos FM. De netwerkbenadering bij depressie: veel noten op de zang of heilige graal? Gedragstherapie. (2019) 52:43−68.
87. Curran PJ, Bauer DJ. The disaggregation of within-person and between-person effects in longitudinal models of change. Annu Rev Psychol. (2011) 62:583–619. doi: 10.1146/annurev.psych.093008.100356
88. Houtveen JH, Hamaker EL, Van Doornen LJ. Using multilevel path analysis in analyzing 24-h ambulatory physiological recordings applied to medically unexplained symptoms. Psychophysiology. (2010) 47:570–8. doi: 10.1111/j.1469-8986.2009.00951.x
89. Pe ML, Kircanski K, Thompson RJ, Bringmann LF, Tuerlinckx F, Mestdagh M, et al. Emotion network density in major depressive disorder. Clin Psychol Sci. (2015) 3:292–300. doi: 10.1177/2167702614540645
90. Nezlek JB, Holas P, Rusanowska M, Krejtz I. Being present in the moment: event-level relationships between mindfulness and stress, positivity, and importance. Pers Individ Dif . (2016) 93:1–5. doi: 10.1016/j.paid.2015.11.031
91. Bos FM, Snippe E, de Vos S, Hartmann JA, Simons CJP, van der Krieke L, et al. Can we jump from cross-sectional to dynamic interpretations of networks? Implications for the network perspective in psychiatry. Psychother Psychosom. (2017) 86:175–7. doi: 10.1159/000453583
92. Schenk HM, Bos EH, Slaets JP, De Jonge P, Rosmalen JG. Differential association between affect and somatic symptoms at the between- and within-individual level. Br J Health Psychol. (2017) 22:270–80. doi: 10.1111/bjhp.12229
93. Bouwmans MEJ, Oude Oosterik NAM, Bos EH, de Groot IW, Oldehinkel AJ, de Jonge P. The temporal order of changes in physical activity and subjective sleep in depressed versus nondepressed individuals: findings from the MOOVD study. Behav Sleep Med. (2018) 16:154–68. doi: 10.1080/15402002.2016.1180521
94. Klippel A, Viechtbauer W, Reininghaus U, Wigman J, van Borkulo C, Myin-Germeys I, et al. The cascade of stress: a network approach to explore differential dynamics in populations varying in risk for psychosis. Schizophr. Bull. (2018) 44:328–37. doi: 10.1093/schbul/sbx037
95. Liu S. Person-specific versus multilevel autoregressive models: accuracy in parameter estimates at the population and individual levels. Br J Math Stat Psychol. (2017) 70:480–98. doi: 10.1111/bmsp.12096
96. Krone T, Albers CJ, Kuppens P, Timmerman ME. A multivariate statistical model for emotion dynamics. Emotion. (2018) 18:739–54. doi: 10.1037/emo0000384
97. Verbeke G, Molenberghs G. Estimation of the marginal model. In Verbeke G, Molenberghs G, editors. Linear mixed models for longitudinal data. New York, NY: Springer (2000). p. 41–54.
98. Hoffman L. Multilevel models for examining individual differences in within-person variation and covariation over time. Multivariate Behav Res. (2007) 42:609–29. doi: 10.1080/00273170701710072
99. Asparouhov T, Hamaker EL, Muthén B. Dynamic structural equation models. Struct Eq Model. (2018) 25:359–88. doi: 10.1080/10705511.2017.1406803
100. Oravecz Z, Tuerlinckx F, Vandekerckhove J. Bayesian data analysis with the bivariate hierarchical Ornstein-Uhlenbeck process model. Multivariate Behav Res. (2016) 51:106–19. doi: 10.1080/00273171.2015.1110512
101. Driver CC, Voelkle MC. Hierarchical Bayesian continuous time dynamic modeling. Psychol Methods. (2018) 23:774–99. doi: 10.1037/met0000168
102. Persons JB, Silberschatz G. Are results of randomized controlled trials useful to psychotherapists? J Consult Clin Psychol. (1998) 66:126. doi: 10.1037/0022-006X.66.1.126
103. Lillie EO, Patay B, Diamant J, Issell B, Topol EJ, Schork NJ. The n-of-1 clinical trial: the ultimate strategy for individualizing medicine? Personalized Med. (2011) 8:161–73. doi: 10.2217/pme.11.7
104. Zhan S, Ottenbacher KJ. Single subject research designs for disability research. Disabil Rehabil. (2001) 23:1–8. doi: 10.1080/09638280150211202
105. Ter Kuile MM, Bulte I, Weijenborg PTM, Beekman A, Melles R, Onghena P. Therapist-aided exposure for women with lifelong vaginismus: a replicated single-case design. J Consul Clin Psychol. (2009) 77:149–59. doi: 10.1037/a0014273
106. Bos FM, Wichers M, Emerencia A, Veling W, Haarman B, Van der Veen DC, et al. Developing a flexible interface to generate personalized diaries in mental health care. Support Health Technol. (2019) 9:3.
107. Robinson OC, McAdams DP. Four functional roles for case studies in emerging adulthood research. Emerg Adulth. (2015) 3:413–21. doi: 10.1177/2167696815592727
108. Bishop S, Miller IW, Norman W, Buda M, Foulke M. Cognitive therapy of psychotic depression: a case report. Psychotherapy. (1986) 23:167–73. doi: 10.1037/h0085584
109. Burger J, van der Veen DC, Robinaugh DJ, Quax R, Riese H, Schoevers RA, et al. Bridging the gap between complexity science and clinical practice by formalizing idiographic theories: a computational model of functional analysis. BMC Med. (2020) 18:99. doi: 10.1186/s12916-020-01558-1
110. West SG, Hepworth JT. Statistical issues in the study of temporal data: daily experiences. J Pers. (1991) 59:609–62. doi: 10.1111/j.1467-6494.1991.tb00261.x
111. Bouwmans MEJ, Beltz AM, Bos EH, Oldehinkel AJ, de Jonge P, Molenaar PCM. The person-specific interplay of melatonin, affect, and fatigue in the context of sleep and depression. Pers Ind Diff . (2018) 123:163–70. doi: 10.1016/j.paid.2017.11.022
112. Yang X, Ram N, Gest SD, Lydon-Staley DM, Conroy DE, Pincus AL, et al. Socioemotional dynamics of emotion regulation and depressive symptoms: a person-specific network approach. Complexity. (2018) 2018:5094179. doi: 10.1155/2018/5094179
113. Simon GE, Perlis RH. Personalized medicine for depression: can we match patients with treatments? Am J Psychiatry. (2010) 167:1445–55. doi: 10.1176/appi.ajp.2010.09111680
114. Cuijpers P, Reynolds CF, Donker T, Li J, Andersson G, Beekman A. Personalized treatment of adult depression: medication, psychotherapy, or both? A systematic review. Depress Anxiety. (2012) 29:855–64. doi: 10.1002/da.21985
Keywords: psychiatry, single-subject, idiographic, nomothetic, N-of-1, intra-individual
Citation: Zuidersma M, Riese H, Snippe E, Booij SH, Wichers M and Bos EH (2020) Single-Subject Research in Psychiatry: Facts and Fictions. Front. Psychiatry 11:539777. doi: 10.3389/fpsyt.2020.539777
Received: 02 March 2020; Accepted: 08 October 2020;
Published: 13 November 2020.
Edited by:
Suraj Bahadur Thapa, University of Oslo, NorwayReviewed by:
Oliver Robinson, University of Greenwich, United KingdomDaniel R. Little, The University of Melbourne, Australia
Copyright © 2020 Zuidersma, Riese, Snippe, Booij, Wichers and Bos. This is an open-access article distributed under the terms of the Creative Commons Attribution License (CC BY). The use, distribution or reproduction in other forums is permitted, provided the original author(s) and the copyright owner(s) are credited and that the original publication in this journal is cited, in accordance with accepted academic practice. No use, distribution or reproduction is permitted which does not comply with these terms.
*Correspondence: Marij Zuidersma, bS56dWlkZXJzbWFAdW1jZy5ubA==