- 1Department of Clinical Psychology, The Affiliated Brain Hospital of Guangzhou Medical University, Guangzhou, China
- 2Department of Psychiatry, The Affiliated Brain Hospital of Guangzhou Medical University, Guangzhou, China
- 3Department of Management, Hong Kong Baptist University, Hong Kong, China
Major Depressive Disorder (MDD) with childhood trauma is one of the functional subtypes of depression. Frequency-dependent changes in the amplitude of low-frequency fluctuations (ALFF) have been reported in MDD patients. However, there are few studies on ALFF about MDD with childhood trauma. Resting-state functional magnetic resonance imaging was used to measure the ALFF in 69 MDD patients with childhood trauma (28.7 ± 9.6 years) and 30 healthy subjects (28.12 ± 4.41 years). Two frequency bands (slow-5: 0.010–0.027 Hz; slow-4: 0.027–0.073 Hz) were analyzed. Compared with controls, the MDD with childhood trauma had decreased ALFF in left S1 (Primary somatosensory cortex), and increased ALFF in left insula. More importantly, significant group × frequency interactions were found in right dorsal anterior cingulate cortex (dACC). Our finding may provide insights into the pathophysiology of MDD with childhood trauma.
Introduction
Major depressive disorder (MDD) is described as long sadness and interest loss in generally pleasant activities, following with inability to perform daily activities, over a period of at least 2 weeks (1). At present, depending on its characteristics, MDD can be divided into different subtypes (2).
Childhood trauma is considered as a key factor affecting the occurrence of depression (3, 4). Some scholars believe that MDD with childhood trauma may be one of the functional subtypes of depression (5). To gain an insight of MDD and its heterogeneity, latest studies have been focusing on its clinically relevant depression hypotypes (6). Previous studies have found that nearly half of depression patients have experienced childhood trauma (7, 8), and their clinical characteristics are different from those of ordinary depression patients (9). For instance, symptoms of MDD are often more serious. The symptoms of MDD include higher suicide risk, serious cognitive impairment, and decreased response to antidepressants (10). In addition, there are some specific changes in brain imaging of depression patients with childhood trauma. Brain network studies reported that there are constant disruptions of resting-state networks (RSNs) in patients with MDD, including increased connection between the DMN and FPN, hyperconnectivity in the default mode network (DMN), and hyporconnectivity in the frontoparietal network (FPN) (11). Saleh et al. reported that in depressive subjects, greater early life stress exposure was linked to slower processing speeds and smaller orbitofrontal cortex volumes; however, in non-depressive subjects, it was associated with faster speeds and larger volumes (12). Some studies have found that, compared with the healthy control subjects, amplitude of low-frequency fluctuations (ALFF) in bilateral amygdala and left orbit/cerebellum is increased, while fALFF in the left inferior temporal gyrus and right middle frontal gyrus is decreased in children with MDD after trauma. On MDD, early life stress was positively correlated with regional activity in the left cerebellum and the right posterior central/infratemporal/superior frontal cingulate gyrus and inferior frontal gyrus (13).
Since typical task-based functional magnetic resonance imaging (fMRI) scans can filter out low-frequency physiological noise and keep the high-frequency component of the signal it may lead to confusion about performance differences or activity patterns of specific tasks between healthy subjects and patients. Resting-state fMRI (rs-FMRI), which is applied to evaluate interactions between brain regions when a subject is not performing a specific task (14), has been widely applied to identify the neural correlation to psychiatric disorders. Low frequency fluctuation amplitude analysis (ALFF) measures voxel-by-voxel fluctuations in the amplitude of a blood oxygen level related (BOLD) signal at very low frequencies (typically 0.010–0.080 Hz) (15), which are pointed out to reflect autonomous brain activity (16). Signals in different frequency bands may lead to distinguish brain oscillations with physiological functions and specific characteristics (17). Entire low-frequency spectrum is usually subdivided into four bands (slow-2, 0.198–0.250 Hz; slow-3, 0.073–0.198 Hz; slow-4, 0.027–0.073 Hz; slow-5, 0.010–0.027 Hz) (18). The slow-4 and slow-5 bands are the most associated to gray matter (GM) signals, which are helpful for identifying associations between the functional processing and disease (19). More recent studies have examined ALFF at slow-4 (0.027–0.073 Hz) and slow-5 (0.010–0.027 Hz).
Although ALFF analyses are widely used to study depression, few ALFF studies on MDD have specifically examined MDD with childhood trauma. Therefore, more research is needed to examine the pathophysiology and etiology of MDD with childhood trauma, to help with the early diagnosis and treatment of this subtype of depression, and further to enhance patients' quality of life. The search for biological markers of disease mechanism, treatment choices, diagnosis, or prognosis has become one of the principal goals of depression' neuroimaging (11). Our research aims to identify potential biomarkers to differentiate individuals with MDD with childhood trauma from individuals with ordinary depression.
Materials and Methods
Subjects
Ninety-nine subjects were recruited for this study. Among them, 69 were unmedicated MDD patients with childhood trauma and 30 were non-depressed comparison subjects (HC). Sixty-nine unmedicated subjects with MDD ranging from 18 to 45 were recruited from the outpatient and inpatient units The Affiliated Brain Hospital of Guangzhou Medical University. The absence or presence of MDD was evaluated by the structured clinical interview (SCID) for DSM-V diagnostic criteria. Patients with other neurological disorders, psychiatric axis-I or axis-II disorders, electroconvulsive therapy, medication used in the past 6 months, clinically relevant abnormalities in laboratory examinations or their medical history, and contraindication for magnetic resonance imaging (MRI) were excluded. Depression severity were evaluated by Zung's Self-Rating Depression Scale (SDS) and The 17-item Hamilton Depressive Rating Scale (HAMD) (20). The Childhood Truama Questinnaire (CTQ-SF) was used to measure childhood adversity. The CTQ-SF consist of five subscales: sexual abuse (SA), emotional abuse (EA), physical abuse (PA), emotional neglect (EN) and physical neglect (PN) (21). The cut off points for subscales are: SA grade ≥ 8, EA grade ≥ 13, PA grade ≥ 10, PN grade ≥ 10, and EN grade ≥ 15 (8, 22). In addition, 30 gender- age-, and education-matched healthy subjects were recruited from the community near the hospital. Before enrollment, all participants were fully gave notice of the information of the research, and written informed consent was formal obtained. Researches were executed under the Declaration of Helsinki and ratified by The Affiliated Brain Hospital of Guangzhou Medical University Ethics Committee.
MRI Data Acquisition
Philips 3T MR systems (Philips, Best, The Netherlands) were used to acquire imaging data. Subjects were asked to wear earplugs and positioned in the coil for support. Participants were requested to lie still with their eyes closed but to remain awake, and all participants reported that they did not fall asleep during the whole experiment. A total of 180 volumes of echo planar images were obtained axially (repetition time, 3,000 ms; echo time, 30 ms; slices, 36; thickness, 4 mm, no slice gap; field of view, 240 × 240 mm2; resolution, 64 × 64; flip angle, 90°).
Image Pre-processing
The first 10 volumes of each functional time series for the instability of the initial MRI signal and the adaptation of participants to the circumstance were discarded. The fMRI images were sliced acquisition corrected, head-motion corrected, normalized to the standard SPM5 Montreal Neurological Institute (MNI) template, and then re-sampled to 3-mm cubic voxels. Data were filtered using typical temporal bandpass (0.010–0.080 Hz), slow-4 bandpass (0.027–0.073 Hz), and slow-5 bandpass (0.010–0.027 Hz) separately after linear detrending. REST software was used to calculate ALFF (by Dr. Yong He, http://resting-fmri.sourceforge.net). The filtered time series was transformed into a frequency domain with a fast Fourier transform (FFT) (parameters: taper percent = 0, FFT length = shortest), and the power spectrum was then obtained. Since the power of a given frequency is proportional to the square of the amplitude of this frequency component of the original time series in the time domain, the square root was calculated at each frequency of the power spectrum and the averaged square root was obtained across 0.010–0.080 Hz at each voxel. This averaged square root was taken as the ALFF. For standardization purposes, the ALFF of each voxel was divided by the global mean ALFF value.
A corrected threshold of family-wise error (FWE) of p < 0.05 at cluster level was set. Coordinates were reported in MNI coordinates, as used by SPM.
Statistical Analysis
SPSS 17.0 software was used to analysis the general data. Mean ± SD was used to describe the continuous data that conformed the normal distribution. Between the data analysis of MDD with childhood trauma group and health control group, independent sample t-test was performed. When calculating the group differences within the ALFF measurement and the correlation between the clinical measurements and ALFF, age, gender, and education level were included as covariates. In depressed subjects, relationship between depression severity and ALFF values were analyzed by Pearson's correlations. Respectively, post-hoc test and simple effect analyses were conducted with the mALFF values averaged in order to further investigate the differences in frequency bands and groups. The above results are statistically significant with P < 0.05.
Results
Demographics
Clinical data and demographic of healthy and depressed subjects are showed in Table 1. The mean age of the 69 patients is 28.7 ± 9.6 years, and the average year of education is 13.27 ± 3.32 years. The illness duration is 21.32 ± 12.08 months. Thirty controls are recruited, of which the average age is 28.12 ± 4.41 years, and the mean years of education is 14.12 ± 3.24. There is no significant difference in gender, education state, age between two groups.
Correlations Between Clinical Data and the ALFF
In insula, a highly positive correlation between HAMD total score and the ALFF (p < 0.01) was found. On the contrary, a strong negative correlation between depressive symptom severity and ALFF was detected in the S1 and dACC (p < 0.01) (Table 2).
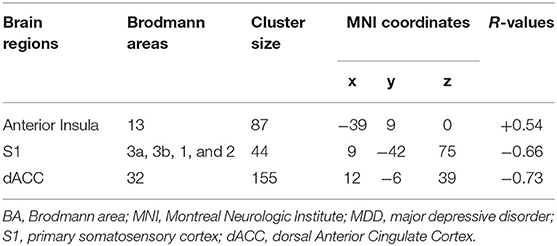
Table 2. Brain regions showing statistically significant correlation between ALFF and depression severity in terms of HAMD scores in subjects with MDD with CT.
ALFF Frequency Main Effect
Main effects from the two-way repeated measure ANOVA are shown in Figure 1. In midbrain, basal ganglia, cingulate cortex and fusiform gyrus, ALFF of slow 4 is obviously larger than that of slow 5, while ALFF of slow 5 is larger in lingual gyrus, middle temporal gyrus, inferior frontal gyrus and medial frontal ventral gyrus (see Figure 1).
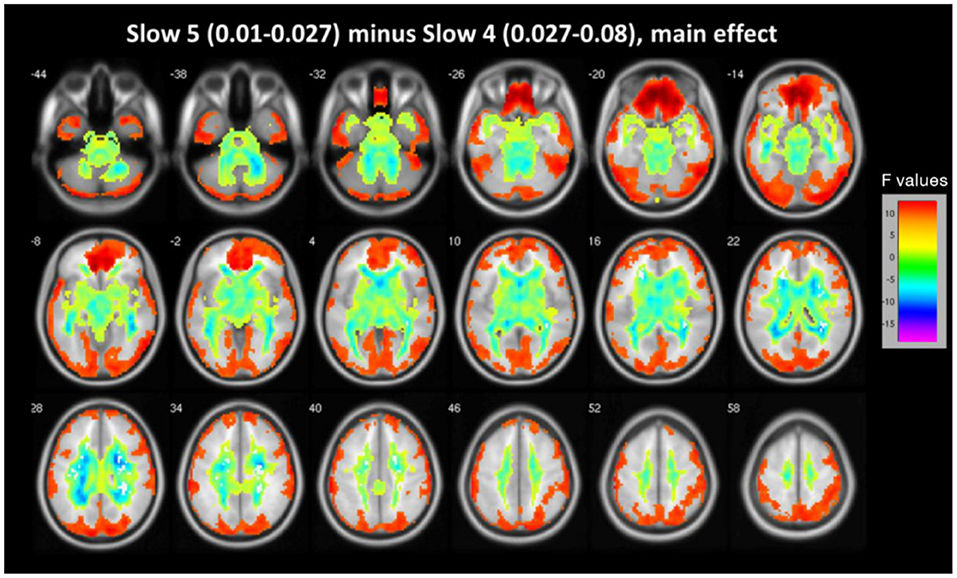
Figure 1. The main effect for frequency band on ALFF. Most of the brain showed marked differences in ALFF between the two frequency bands (slow-4 vs. slow-5). Warm color represents greater ALFF in the slow-5 band than in the slow-4 band, while cool color represents lower ALFF. A two-way repeated measures ANOVA obtained the results.
ALFF Group Main Effect
Differences between groups are demonstrated in Table 3 and Figure 2. Compared to controls, patients exhibited decreased ALFF in a cluster in the postcentral gyrus (sensorimotor cortex), [x = 9, −42, 75], Z = 5.72, voxels = 154, PFWE < 0.05, see Figure 2A. Patients had higher ALFF in the left anterior insula, [−39, 9, 0], Z = 3.29, voxels = 22, PFWE < 0.05 (Figure 2B).

Table 3. The brain region showing marked group main effect and interaction effect of group and frequency (slow−4 and slow−5) on ALFF.
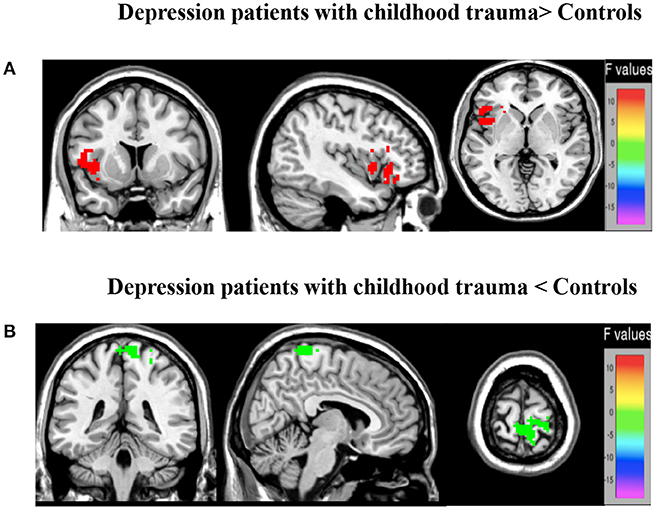
Figure 2. Brain regions showing marked group main effect (A,B) on ALFF. (A) Warm colors represent reduced ALFF in the MDD with CT when compared with HC; (B) cool colors represent the decreased ALFF in the young depression group when compared with HC.
Group X Frequency Interaction
We found the significant group by frequency interaction in dACC, [12, −6, 39], Z = 5.20, voxels = 56, PFWE < 0.05, corrected at cluster-level see Figure 3A. The interaction is plotted in Figure 3.
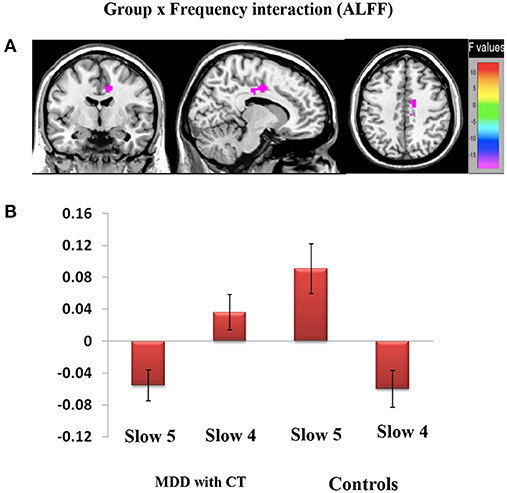
Figure 3. Brain regions showing significant group and frequency (slow-4 and slow-5) interaction (A,B) effects on ALFF. (A) The interaction between frequency band and group on ALFF. A two-way repeated-measure ANOVA and post-hoc test obtained the results. The beta values for dACC were plotted (B) to show the directions of the interaction.
Discussion
Functional MRI has been broadly used to explore the brain systems's dysfunction in patients with psychiatric disorders. Our study found that the frequency-dependent changes in the ALFF occur in MDD patients with childhood trauma. ALFF analysis is applied to examine the amplitude of low-frequency oscillations (LFOs) in MDD with childhood trauma in different frequency bands, namely slow-5 and slow-4.
Our study is the first systematic investigation to explore frequency-dependent changes in LFO amplitudes (as indexed by the ALFF) from resting-state fMRI signals in MDD patients with childhood trauma. In different brain regions, the main frequency-dependent effects varies according to the different frequency bands: slow-4 oscillations were greater than slow-5 oscillations primarily in subcortical regions, such as midbrain, basal ganglia, cingulate cortex, and fusiform gyrus; and slow-5 oscillations were greater than slow-4 oscillations in several cortical areas, such as the inferior frontal gyrus, ventromedial frontal gyrus and middle temporal gyrus. Compared with controls, major depressive patients with childhood trauma indicated decreased ALFF values in S1 and increased ALFF values in insula. Moreover, a remarkable interaction was identified between frequency bands and groups in dACC, which suggests that the observed dynamic changes in low-frequency fluctuations may be related to frequency-dependent. Due to this reason, we decided to analyze group differences in the ALFF across the slow-5 and slow-4 ranges.
Group Differences in ALFF Between Frequency Bands
Previous studies of the ALFF have also detected the differences between brain areas in the slow-4 and slow-5 bands (18, 23, 24). Although, from different frequency bands, the significance and source of signals are not clear, it has been suggested that the different low-frequency bands relate to different neural processes and physiological functions (17, 25). Lower frequency oscillations have been linked with long-range connections and the integration of large neuronal systems, while signals from higher frequency bands have been linked to more local neuronal assemblies and short-range connections (25). Therefore, regions of the cerebral cortex such as the inferior frontal gyrus, ventromedial frontal gyrusand, and middle temporal gyrus, may facilitate long-distance connectivity in many large networks. Conversely, subcortical regions such as fusiform gyrus, cingulate cortex, and basal ganglia may mainly contribute to fast local events, which are regulated by extensive slow oscillations (25, 26). Additionally, compared with the slow-4 band, we found higher ALFF values in the slow-5 band in most of the main subregions of the default mode network (DMN) (27), including inferior frontal gyrus, middle temporal gyrus and ventromedial frontal gyrus. Our results are in accord with the previous studies on MDD patients which suggested that the slow-5 band shows stronger LFOs and higher functional activity in the majority of DMN subregions (18, 28, 29). It seems that differences in ALFF between frequency bands in MDD with childhood trauma are similar to MDD patients. Interestingly, in the cingulate cortex, lower ALFF were found in the slow-5 band, which differ from the results of ALFF studies into other diseases (28, 29). The cingulate cortex, a key DMN region shows a strong connectivity in primates with the parahippocampal gyrus and entorhinal cortex, and thus with the hippocampal memory system (30). It has also been reported that the functional connectivity of the cingulate cortex is related to rumination in depression (31). We assume that MDD patients with childhood trauma suffer mainly from a dysfunction of the cingulate cortex.
Increased ALFF Activity in Insular in MDD With CT Patients
The insula is regarded as a hub for external and internal information exchange (32). Accumulating evidence are based on studying changes in insula function in MDD patients, abnormalities in the insula caused by MDD, and dysregulation of the interaction between the insula and other important functional brain networks (such as DMN and central executive network) show can be explored (33–35). The insula plays an important role in generating and processing emotional information, including feelings of anxiety and disgust (36, 37). In short, previous findings demonstrate that the insula plays an important role in the pathogenesis of MDD patients with childhood trauma. Previous neuroimaging studies have found that the abnormal function of the insula is tightly related to the symptoms of depression, including reports that resting-state brain functional connectivity in insula can more accurately distinguish healthy controls from patients with depression (38). Numerous studies have shown a close relationship between changes in insula function and depression, but the results of these studies are inconsistent. Su et al. found that the ALFF decreases in the insula of depressive patients (39, 40), but some scholars found that the ALFF was increased in the insula of depressive patients (41). Whether MDD patients experience childhood trauma may be one of the reasons leading to the inconsistent. In our study, we found that the ALFF in the insula of the depressive group is observably higher than that in the HC group, indicating the dysfunction of insular in MDD patients with childhood trauma. This dysfunction may be the key factor that severely impairs the ability of MDD patients with childhood trauma to regulate their emotions. Our results show that compared to HC, MDD patients have an increased ALFF in the insula, which indicates abnormal insula activities, and it may further lead to a declined in the ability of regulating the negative emotions.
Decreased ALFF Activity in S1 in MDD With CT Patients
S1, parallel to the central sulcus, is located in the postcentral gyrus (42). S1 is responsible for integrating pain-related information. In animal models, researchers have made a solid conclusion that S1 is involved in sensory-discriminative pain processing; that is, it encodes the location and intensity of pain stimulation (43–45). A number of studies have found that empathizing with others' pain can also activate S1 (46). Moreover, research has shown that the cognitive processing of pain usually begins with activation in prefrontal brain regions, which then further regulates activity in pain-related regions of S1 (47–49). It indicates that the painful somatic symptoms of MDD may relate to functional changes in S1. Several studies have found that when subjects experience different emotions, the activity in S1 also changes and it is highly activated when the subjects focus on their own emotions (50). The result has indicated that S1 takes part in the processing of emotional experience, primarily in the generation of somatosensory emotions. More and more research is supporting the conclusion that S1 is involved in modulating and controlling associatively learned behaviors (51, 52). Schaefer et al. even suggested that S1 functions as an “embodied mind,” which supports the existence of the unconscious self in concert with the embodied aspect of the self (53). In short, these findings suggest that S1 is likely to be an important structure closely related to cognitive function. MDD patients with childhood trauma typically have many symptoms related to cognitive impairment, which may be associated with S1 dysfunction. Previous studies have found an increased GM volume in right S1 in patients with comorbid anxiety and MDD (54), while the total area of the somatosensory cortex is reduced in adolescent patients with MDD and in patients with bipolar disorder (55, 56). Kang et al. reported MDD patient's abnormalities in S1–thalamic functional connectivity related to the typical clinical symptoms of MDD, which indicates that such changes are neurobiological characteristics and potential biomarkers of MDD with childhood trauma (57). However, there has still been no research to report the relationship between changes in functional signals in S1 and the development of MDD. In our study, we found that compared to the healthy controls, MDD with CT patients had a lower ALFF in S1, indicating a lower level of spontaneous neural activity. This implies that the dysfunction in left S1 seems to be related to the development of MDD and lead to a possible decline or loss of sensation. We assume that a lower ALFF in S1 may be a specific signal of MDD with the subtype of childhood trauma.
Group × Frequency Interaction in dACC
In this study, a significant group × frequency interaction effect on the ALFF value in dACC is observed. This result indicates that the difference in resting brain function may result from an interaction between frequency bands and disease states. Moreover, it suggests that pathological conditions may increase the influence of some particular frequency bands in the brain, especially in dACC, and that each frequency band may have a specific pathological significance. The dACC is connected with areas of the central executive network and the salience network, which are involved in target maintenance and the integration of sensorimotor and visuomotor inputs, and therefore, guides the output of corresponding behaviors (58). Many studies have shown that functional changes in dACC are involved in the pathophysiological process of MDD (59, 60). In addition, some studies have shown that the components of emotional experience related to pain are processed by the anterior insula and dACC (61), and dACC mainly encodes the psychological aspect of pain sensation (62). These conclusions demonstrate that dACC plays a key role in dealing with social pain and functions as a negative information processing system. A number of animal studies have showed that the dACC was activated when infant mammals were separated from their mothers; however, after the damage to dACC, animal infants reduced their whining during separation. This result has indicated that the brain activities that take part in processing pain-related emotional components are closely related to the degree of painful feelings caused by negative social events.
Researchers also investigated whether different forms of negative social events will have the same effect on activating the brain areas that involved in processing painful components of emotion. In conclusion, in the tasks of social evaluation and social loss, brain areas involved in processing painful emotional components were activated (63). The tasks of negative emotional experiences (such as fear, anxiety, anger, disgust, and sadness) and physiological pain both can lead to the increased activity in insula and dACC. Based on that, a conclusion made is that the damage to the function of dACC may lead to an imbalance in negative information processing in MDD with childhood trauma, activating brain areas related to painful emotional components and producing various negative emotions.
We assume that the disease state and spontaneous brain activity may inhibit individuals' functions to each other, thus causing dysfunction in dACC. The remarkable correlation observed between ALFF in the right dACC within slow-5 suggests that the LFO amplitude in the region may be applied to monitor the disease progress of MDD with childhood trauma.
Limitations
Our study used a cross-sectional design with a relatively small sample. Other confounding differences between the two groups, such as social economic status and mood, may also explain our findings. Future studies may further investigate how ALFF changes as the depression progresses. Importantly, it would be interesting to directly compare MDD with childhood trauma with MDD without such trauma to isolate the effect of childhood trauma on brain networks in MDD. The exact origins and mechanisms underlying ALFF remain elusive. More studies are needed to comprehend the functional significance of ALFF changes in MDD.
Conclusions
In conclusion, our results show that changes in the ALFF occur in various brain regions in patients suffering MDD with CT, which indicates that the subtypes of MDD involve the dysfunction of multiple areas of the brain. The CT results of MDD patients demonstrate abnormal LFO amplitudes in many brain regions, consist of subcortical regions, cortical areas, insula, and S1, which highlight abnormalities in LFO amplitudes in MDD with CT and provide insights into understanding the pathophysiology of major depressive disorder.
Data Availability Statement
The raw data supporting the conclusions of this article will be made available by the authors, without undue reservation.
Ethics Statement
The studies involving human participants were reviewed and approved by Ethics Committee of Affiliated Brain Hospital of Guangzhou Medical University. Written informed consent to participate in this study was provided by the participants' legal guardian/next of kin.
Author Contributions
ZW and QL designed the study and drafted the primary manuscript. HW, ZW, and YZ supervised the recruitment and made statistical analyses. YY, JH, and YD took part in recruitment and data management. RY and HP made further revisions of the manuscript. All the authors had read and approved the final manuscript.
Funding
The Guangdong Natural Science Foundation, China (2015A030313800 to HP) supported the design of the study. The Guangzhou municipal key discipline in medicine for Guangzhou Brain Hospital (GBH2014-ZD04 to HP) supported the data collection of the study. The Science and Technology Plan Project of Guangdong Province, China (2019B030316001 to Yuping Ning) and the Guangzhou Municipal Psychiatric Disease Clinical Transformation Laboratory (201805010009 to Yuping Ning) supported the interpretation of data of this study.
Conflict of Interest
The authors declare that the research was conducted in the absence of any commercial or financial relationships that could be construed as a potential conflict of interest.
Acknowledgments
We would like to thank our subjects for their readiness to engage in this study.
Abbreviations
ALFF, amplitude of low-frequency fluctuations; BOLD, blood oxygenation level dependent; DMN, Default-mode network; CTQ-SF, Childhood Truama Questinnaire; dACC, dorsal anterior cingulate cortex; FC, functional connectivity; FWE, family-wise error; FFT, fast Fourier transforms; fMRI, functional magnetic resonance imaging; fALFF, fractional amplitude of low frequency fluctuations; GM, gray matter; HC, healthy controls; HAMD, Hamilton Depressive Rating Scale; LFO, low-frequency oscillations; MDD, Major Depressive Disorder; MRI, magnetic resonance imaging; MPFC, medial prefrontal cortex; MNI, Montreal Neurological Institute; MTG, middle temporal gyrus; S1, primary somatosensory cortex; SCID, structured clinical interview; STD, subthreshold depression; SDS, Zung's Self-Rating Depression Scale; TRD, treatment-resistant depression.
References
1. Park LT, Zarate CA. Depression in the primary care setting. N Engl J Med. (2019) 380:559–68. doi: 10.1056/NEJMcp1712493
2. Alexopoulos GS. Depression in the elderly. Lancet. (2005) 365:1961–70. doi: 10.1016/S0140-6736(05)66665-2
3. Heim C, Newport DJ, Mletzko T, Miller AH, Nemeroff CB. The link between childhood trauma and depression: insights from HPA axis studies in humans. Psychoneuroendocrinology. (2008) 33:693–710. doi: 10.1016/j.psyneuen.2008.03.008
4. Scheuer S, Wiggert N, Brückl TM, Awaloff Y, Uhr M, Lucae S, et al. Childhood abuse and depression in adulthood: the mediating role of allostatic load. Psychoneuroendocrinology. (2018) 94:134–42. doi: 10.1016/j.psyneuen.2018.04.020
5. Heim C, Mletzko T, Purselle D, Musselman DL, Nemeroff CB. The dexamethasone/corticotropin-releasing factor test in men with major depression: role of childhood trauma. Biol Psychiatr. (2008) 63:398–405. doi: 10.1016/j.biopsych.2007.07.002
6. Ulbricht CM, Chrysanthopoulou SA, Levin L, Lapane KL. The use of latent class analysis for identifying subtypes of depression: a systematic review. Psychiatr Res. (2018) 266:228–46. doi: 10.1016/j.psychres.2018.03.003
7. Nelson J, Klumparendt A, Doebler P, Ehring T. Childhood maltreatment and characteristics of adult depression: meta-analysis. Br J Psychiatr. (2017) 210:96–104. doi: 10.1192/bjp.bp.115.180752
8. Xie P, Wu K, Zheng Y, Guo Y, Yang Y, He J, et al. Prevalence of childhood trauma and correlations between childhood trauma, suicidal ideation, and social support in patients with depression, bipolar disorder, and schizophrenia in southern China. J Affect Disord. (2018) 228:241–8. doi: 10.1016/j.jad.2017.11.011
9. Wiersma JE, Hovens JG, van Oppen P, Giltay EJ, van Schaik DJ, Beekman AT, et al. The importance of childhood trauma and childhood life events for chronicity of depression in adults. J Clin Psychiatr. (2009) 70:983–9. doi: 10.4088/jcp.08m04521
10. Gamble SA, Talbot NL, Duberstein PR, Conner KR, Franus N, Beckman AM, et al. Childhood sexual abuse and depressive symptom severity: the role of neuroticism. J Nerv Ment Dis. (2006) 194:382–5. doi: 10.1097/01.nmd.0000218058.96252.ac
11. Yu M, Shinohara RT, Oathes DJ, Cook PA, Duprat R, Moore TM, et al. Childhood trauma history is linked to abnormal brain connectivity in major depression. Proc Natl Acad Sci USA. (2019) 116:8582–90. doi: 10.1073/pnas.1900801116
12. Saleh A, Potter GG, McQuoid DR, Boyd B, Turner R, MacFall JR, et al. Effects of early life stress on depression, cognitive performance and brain morphology. Psychol Med. (2017) 47:171–81. doi: 10.1017/S0033291716002403
13. Du L, Wang J, Meng B, Yong N, Yang X, Huang Q, et al. Early life stress affects limited regional brain activity in depression. Sci Rep. (2016) 6:25338. doi: 10.1038/srep25338
14. Arnone D, McIntosh AM, Ebmeier KP, Munafò MR, Anderson IM. Magnetic resonance imaging studies in unipolar depression: systematic review and meta-regression analyses. Eur Neuropsychopharmacol. (2012) 22:1–16. doi: 10.1016/j.euroneuro.2011.05.003
15. Zhou Y, Wang K, Liu Y, Song M, Song SW, Jiang T. Spontaneous brain activity observed with functional magnetic resonance imaging as a potential biomarker in neuropsychiatric disorders. Cognitive Neurodyn. (2010) 4:275–94. doi: 10.1007/s11571-010-9126-9
16. Fox MD, Raichle ME. Spontaneous fluctuations in brain activity observed with functional magnetic resonance imaging. Nat Rev Neurosci. (2007) 8:700–11. doi: 10.1038/nrn2201
17. Knyazev GG. Motivation, emotion, and their inhibitory control mirrored in brain oscillations. Neurosci Biobehav Rev. (2007) 31:377–95. doi: 10.1016/j.neubiorev.2006.10.004
18. Wang L, Kong Q, Li K, Su Y, Zeng Y, Zhang Q, et al. Frequency-dependent changes in amplitude of low-frequency oscillations in depression: a resting-state fMRI study. Neurosci. Lett. (2016) 614:105–11. doi: 10.1016/j.neulet.2016.01.012
19. Zuo XN, Di Martino A, Kelly C, Shehzad ZE, Gee DG, Klein DF, et al. The oscillating brain: complex and reliable. Neuroimage. (2010) 49:1432–45. doi: 10.1016/j.neuroimage.2009.09.037
20. Helmreich I, Wagner S, Mergl R, Allgaier A-K, Hautzinger M, Henkel V, et al. Sensitivity to changes during antidepressant treatment: a comparison of unidimensional subscales of the Inventory of Depressive Symptomatology (IDS-C) and the Hamilton Depression Rating Scale (HAMD) in patients with mild major, minor or subsyndromal depression. Eur Arch Psychiatr Clin Neurosci. (2011) 262:291–304. doi: 10.1007/s00406-011-0263-x
21. Bernstein DP, Stein JA, Newcomb MD, Walker E, Pogge D, Ahluvalia T, et al. Development and validation of a brief screening version of the childhood trauma questionnaire. Child Abuse Negl. (2003) 27:169–90. doi: 10.1016/s0145-2134(02)00541-0
22. Jansen K, Cardoso TA, Fries GR, Branco JC, Silva RA, Kauer-Sant'Anna M, et al. Childhood trauma, family history, and their association with mood disorders in early adulthood. Acta Psychiatr Scand. (2016) 134:281–136. doi: 10.1111/acps.12551
23. Yu R, Chien Y-L, Wang H-LS, Liu C-M, Liu C-C, Hwang T-J, et al. Frequency-specific alternations in the amplitude of low-frequency fluctuations in schizophrenia. Human Brain Mapp. (2014) 35:627–37. doi: 10.1002/hbm.22203
24. Yang L, Yan Y, Li Y, Hu X, Lu J, Chan P, et al. Frequency-dependent changes in fractional amplitude of low-frequency oscillations in Alzheimer's disease: a resting-state fMRI study. Brain Imag Behav. (2019) 14:2187–201. doi: 10.1007/s11682-019-00169-6
25. Buzs aki G, Draguhn A. Neuronal oscillations in cortical networks. Science. (2004) 304:1926–9. doi: 10.1126/science.1099745
26. Salvador R, Suckling J, Schwarzbauer C, Bullmore E. Undirected graphs of frequency-dependent functional connectivity in whole brain networks. Philos Trans R Soc B: Biol Sci. (2005) 360:937–46. doi: 10.1098/rstb.2005.1645
27. Raichle ME, MacLeod AM, Snyder AZ, Powers WJ. A default mode of brain function. PNAS. (2001) 98:676–82. doi: 10.1073/pnas.98.2.676
28. Liu X, Wang S, Zhang X, Wang Z, Tian X, He Y. Abnormal amplitude of low-frequency fluctuations of intrinsic brain activity in Alzheimer's disease. J Alzheimers Dis. (2014) 40:387–97. doi: 10.3233/JAD-131322
29. Wei L, Duan X, Zheng C, Wang S, Gao Q, Zhang Z, et al. Specific frequency bands of amplitude low-frequency oscillation encodes personality. Hum Brain Mapp. (2014) 35:331–9. doi: 10.1002/hbm.22176
30. Bubb EJ, Kinnavane L, Aggleton JP. Hippocampal–diencephalic–cingulate networks for memory and emotion: An anatomical guide. Brain Neurosci Adv. (2017) 1:2398212817723443. doi: 10.1177/2398212817723443
31. Berman MG, Peltier S, Nee DE, Kross E, Deldin PJ, Jonides J. Depression, rumination and the default network. Soc Cogn Affect Neurosci. (2011) 6:548–55. doi: 10.1093/scan/nsq080
32. Mufson EJ, Mesulam MM. Insula of the old world monkey. II: Afferent cortical input and comments on the claustrum. J Comp Neurol. (1982) 212:223–37. doi: 10.1002/cne.902120103
33. Menon V. Large-scale brain networks and psychopathology: a unifying triple network model. Trends Cogn Sci. (2011) 15:483–506. doi: 10.1016/j.tics.2011.08.003
34. Manoliu A, Meng C, Brandl F, Doll A, Tahmasian M, Scherr M, et al. Insular dysfunction within the salience network is associated with severity of symptoms and aberrant inter-network connectivity in major depressive disorder. Front Human Neurosci. (2014) 7:930. doi: 10.3389/fnhum.2013.00930
35. Kaiser RH, Andrews-Hanna JR, Wager TD, Pizzagalli DA. Large-scale network dysfunction in major depressive disorder: a meta-analysis of resting-state functional connectivity. JAMA Psychiatr. (2015) 72:603–11. doi: 10.1001/jamapsychiatry.2015.0071
36. Singer T, Critchley HD, Preuschoff K. A common role of insula in feelings, empathy and uncertainty. Trends Cogn Sci. (2009) 13:334–40. doi: 10.1016/j.tics.2009.05.001
37. Lamm C, Singer T. The role of anterior insular cortex in social emotions. Brain Struct Funct. (2010) 214:579–91. doi: 10.1007/s00429-010-0251-3
38. Ambrosi E, Arciniegas DB, Madan A, Curtis KN, Patriquin MA, Jorge RE, et al. Insula and amygdala resting-state functional connectivity differentiate bipolar from unipolar depression. Acta Psychiatr Scand. (2017) 136:129–39. doi: 10.1111/acps.12724
39. Fitzgerald PB, Laird AR, Maller J, Daskalakis ZJ. A meta-analytic study of changes in brain activation in depression. Human Brain Mapp. (2008) 29:683–95. doi: 10.1002/hbm.20426
40. Su L, Cai Y, Xu Y, Dutt A, Shi S, Bramon E. Cerebral metabolism in major depressive disorder: a voxel-based meta-analysis of positron emission tomography studies. BMC Psychiatr. (2014) 14:321. doi: 10.1186/s12888-014-0321-9
41. Liu CH, Ma X, Song LP, Fan J, Wang WD, Lv XY, et al. Abnormal spontaneous neural activity in the anterior insular and anterior cingulate cortices in anxious depression. Behav Brain Res. (2015) 281:339–47. doi: 10.1016/j.bbr.2014.11.047
42. Kaas JH, Nelson RJ, Sur M, Lin CS, Merzenich MM. Multiple representations of the body within the primary somatosensory cortex of primates. Science. (1979) 204:521–3. doi: 10.1126/science.107591
43. Guilbaud G, Benoist JM, Levante A, Gautron M, Willer JC. Primary somatosensory cortex in rats with pain-related behaviours due to a peripheral mononeuropathy after moderate ligation of one sciatic nerve: neuronal responsivity to somatic stimulation. Exp Brain Res. (1992) 92:227–45. doi: 10.1007/BF00227967
44. Follett KA, Dirks B. Characterization of responses of primary somatosensory cerebral cortex neurons to noxious visceral stimulation in the rat. Brain Res. (1994) 656:627–32. doi: 10.1016/0006-8993(94)91362-5
45. Canavero S, Bonicalzi V. Role of primary somatosensory cortex in the coding of pain. Pain. (2013) 154:1156–8. doi: 10.1016/j.pain.2013.02.032
46. Apkarian AV, Bushnell MC, Treede RD, Zubieta JK. Human brain mechanisms of pain perception and regulation in health and disease. Eur J Pain. (2005) 9:463–84. doi: 10.1016/j.ejpain.2004.11.001
47. Rainville P, Duncan GH, Price DD, Carrier B, Bushnell MC. Pain affect encoded in human anterior cingulate but not somatosensory cortex. Science. (1997) 277:5968–71. doi: 10.1126/science.277.5328.968
48. Peyron R, Laurent B, García-Larrea L. Functional imaging of brain responses to pain. A review and meta-analysis. Neurophysiol Clin. (2000) 30:263–88. doi: 10.1016/s0987-7053(00)00227-6
49. Rainville P. Brain mechanisms of pain affect and pain modulation. Curr Opin Neurobiol. (2002) 12:195–204. doi: 10.1016/s0959-4388(02)00313-6
50. Damasio AR, Grabowski TJ, Bechara A, Damasio H, Ponto LL, Parvizi J, et al. Subcortical and cortical brain activity during the feeling of self-generated emotions. Nat Neurosci. (2000) 3:1049–56. doi: 10.1038/79871
51. Galvez R, Weiss C, Weible AP, Disterhoft JF. Vibrissa-signaled eyeblink conditioning induces somatosensory cortical plasticity. J Neurosci. (2006) 26:6062–8. doi: 10.1523/JNEUROSCI.5582-05.20
52. Chau LS, Davis AS, Galvez R. Neocortical synaptic proliferation following forebrain-dependent trace associative learning. Behav Neurosci. (2013) 127:285–92. doi: 10.1037/a0031890
53. Schaefer M, Northoff G. Who am i: the conscious and the unconscious self. Front Hum Neurosci. (2017) 11:126. doi: 10.3389/fnhum.2017.00126
54. Qi H, Ning Y, Li J, Guo S, Chi M, Gao M, et al. Gray matter volume abnormalities in depressive patients with and without anxiety disorders. Medicine. (2014) 93:e345. doi: 10.1097/MD.0000000000000345
55. Koutsouleris N, Meisenzahl EM, Borgwardt S, Riecher-Rössler A, Frodl T, Kambeitz J, et al. Individualized differential diagnosis of schizophrenia and mood disorders using neuroanatomical biomarkers. Brain. (2015) 138:2059–73. doi: 10.1093/brain/awv111
56. Schmaal L, Hibar DP, Sämann PG, Hall GB, Baune BT, Jahanshad N, et al. Cortical abnormalities in adults and adolescents with major depression based on brain scans from 20 cohorts worldwide in the ENIGMA Major depressive disorder working group. Mol Psychiatr. (2017) 22:900–9. doi: 10.1038/mp.2016.60
57. Kang L, Zhang A, Sun N, Liu P, Yang C, Li G, et al. Functional connectivity between the thalamus and the primary somatosensory cortex in major depressive disorder: a resting-state fMRI study. BMC Psychiatr. (2018) 18:339. doi: 10.1186/s12888-018-1913-6
58. Seeley WW, Menon V, Schatzberg AF, Keller J, Glover GH, Kenna H, et al. Dissociable intrinsic connectivity networks for salience processing and executive control. J Neurosci. (2007) 27:2349–56. doi: 10.1523/JNEUROSCI.5587-06.2007
59. Wagner G, Köhler S, Bär KJ. Treatment associated changes of functional connectivity of midbrain/brainstem nuclei in major depressive disorder. Sci Rep. (2017) 7:8675. doi: 10.1038/s41598-017-09077-5
60. Schwartz J, Ordaz SJ, Kircanski K, Ho TC, Davis EG, Camacho MC, et al. Resting-state functional connectivity and inflexibility of daily emotions in major depression. J Affect Disord. (2019) 249:226–34. doi: 10.1016/j.jad.2019.01.040
61. Price DD. Psychological and neural mechanisms of the affective dimension of pain. Science. (2000) 288:1769–72. doi: 10.1126/science.288.5472.1769
62. Treedea R-D, Kenshalo DR, Gracelyb RH, Jones AK. The cortical representation of pain. Pain. (1999) 79:105–11. doi: 10.1016/s0304-3959(98)00184-5
Keywords: major depressive disorder, childhood trauma, low-frequency fluctuation, resting state, MRI, insula, primary somatosensory cortex, dorsal anterior cingulate cortex
Citation: Wu Z, Luo Q, Wu H, Wu Z, Zheng Y, Yang Y, He J, Ding Y, Yu R and Peng H (2021) Amplitude of Low-Frequency Oscillations in Major Depressive Disorder With Childhood Trauma. Front. Psychiatry 11:596337. doi: 10.3389/fpsyt.2020.596337
Received: 19 August 2020; Accepted: 29 December 2020;
Published: 22 January 2021.
Edited by:
Boldizsar Czeh, University of Pécs, HungaryReviewed by:
Jiajia Zhu, First Affiliated Hospital of Anhui Medical University, ChinaHong Yang, Zhejiang University, China
Copyright © 2021 Wu, Luo, Wu, Wu, Zheng, Yang, He, Ding, Yu and Peng. This is an open-access article distributed under the terms of the Creative Commons Attribution License (CC BY). The use, distribution or reproduction in other forums is permitted, provided the original author(s) and the copyright owner(s) are credited and that the original publication in this journal is cited, in accordance with accepted academic practice. No use, distribution or reproduction is permitted which does not comply with these terms.
*Correspondence: Hongjun Peng, cGVuZ2hqZ3pAMTYzLmNvbQ==; Rongjun Yu, eXUucm9uZ2p1bkBnbWFpbC5jb20=
†These authors have contributed equally to this work