- 1Department of Psychiatry and Behavioral Sciences, Behavioral Research in Technology and Engineering (BRiTE) Center, University of Washington, Seattle, WA, United States
- 2Department of Computer Science, Dartmouth College, Hanover, NH, United States
- 3Department of Information Science, Cornell University, Ithaca, NY, United States
- 4The Zucker Hillside Hospital, Northwell Health, Glen Oaks, NY, United States
- 5Donald and Barbara Zucker School of Medicine at Hofstra/Northwell, Hempstead, NY, United States
Theoretical views and a growing body of empirical evidence suggest that psychiatric relapses in schizophrenia-spectrum disorders (SSDs) have measurable warning signs. However, because they are time- and resource-intensive, existing assessment approaches are not well-suited to detect these warning signs in a timely, scalable fashion. Mobile technologies deploying frequent measurements—i.e., ecological momentary assessment—could be leveraged to detect increases in symptoms that may precede relapses. The present study examined EMA measurements with growth curve models in the 100 days preceding and following 27 relapses (among n = 20 individuals with SSDs) to identify (1) what symptoms changed in the periods gradually preceding, following, and right as relapses occur, (2) how large were these changes, and (3) on what time scale did they occur. Results demonstrated that, on average, participants reported elevations in negative mood (d = 0.34), anxiety (d =0.49), persecutory ideation (d =0.35), and hallucinations (d =0.34) on relapse days relative to their average during the study. These increases emerged gradually on average from significant and steady increases (d = 0.05 per week) in persecutory ideation and hallucinations over the 100-day period preceding relapse. This suggests that brief (i.e., 1–2 item) assessments of psychotic symptoms may detect meaningful signals that precede psychiatric relapses long before they occur. These assessments could increase opportunities for relapse prevention as remote measurement-based care management platforms develop.
Introduction
Relapses in schizophrenia-spectrum disorders (SSDs) are devastating. In addition to disrupting the lives of individuals experiencing them, each relapse event cumulatively increases the likelihood of subsequent relapses (1), dysfunction (2), poor treatment response (3, 4), and suicide (5). Relapses in SSDs often culminate in psychiatric hospitalizations, which are among the costliest elements in healthcare (6). This is a key reason why SSDs are a leading cause of global disability and healthcare costs (7), costing more than $102 billion worldwide (8–10). Relapses are typically preceded by smaller elevations in symptoms (e.g., delusions, hallucinations, suspiciousness, anxiety) that are potentially detectable before full-blown relapse (11, 12). Intervening to address these symptoms rapidly has the potential to help mitigate the likelihood of relapse and associated sequelae.
Existing approaches used to detect relapse risk are limited by problems with feasibility. Symptoms are typically evaluated through clinical impressions, in-person interviews, or clinician-administered rating scales, which require direct contact with a trained provider. Depending on in-person interactions limits potential reach and scalability, as structured symptom assessments are time and resource intensive, and because the majority of individuals with serious mental illnesses are not regularly engaged in care (13). Further, rater-administered scales require respondents to summarize their experiences over long periods of time. This approach is susceptible to inaccuracies related to memory errors/recall bias (14), interpretive errors (15), or assessment demand characteristics (16).
Mobile devices can be used to deploy a series of brief, self-report measures administered during patients' day-to-day lives [i.e., ecological momentary assessment or EMA; (17)] using mobile technologies that many people with SSDs already have (18). These approaches not only have the potential to improve detection of relapse risk but also to characterize changes that occur in the post-relapse period as well, and to establish trajectory models. Assessment systems using EMA [(19) for a review] are feasible and acceptable to patients with chronic SSDs (20–22) as well as early psychosis (23, 24). The information provided by EMA could be useful in clinical settings aiming to detect symptom increases that signal impending relapse or to track recovery during high-risk periods (i.e., after hospitalizations). New approaches using weekly assessments of aggregate warning signs collected via mobile SMS (25, 26) have demonstrated that rather than occurring in a 2–4 week period as has been previously hypothesized, patients report gradual increases that begin 2 months before a relapse (27). Few studies have assessed changes in individual symptoms that precede and follow relapses with mobile devices. A more granular understanding of the symptom increases that precede relapses could help identify which individual symptoms change prior to relapse, what degree of increases might indicate impending relapse, and on what time scale these changes might be detected. This information could inform the further development of assessments aiming to better detect and prevent the onset of relapse and to inform attempts to understand relevant underlying biological/pharmacological processes.
Ben-Zeev and colleagues deployed a multi-modal mobile assessment system—CrossCheck—in a sample of individuals with schizophrenia for 12 months (28–30). This system administered EMA scales up to three times per week over this period. The present study aims to determine whether a brief report of individual symptoms assessed via EMA detects changes occurring before, during, and after psychiatric relapses. We operationalize this approach by examining (1) whether and to what degree EMA responses assessing symptoms change before relapse, (2) whether mean values predicted by those models on the day of relapse differ from participant averages throughout the study period, and (3) whether and to what degree responses change following relapse.
Materials and Methods
Participants
Participants were recruited from a large psychiatric hospital in New York via on-site flyers as well as study staff review of hospital electronic medical records. The clinicians of potentially eligible individuals were contacted by a member of the research team and were asked to provide these individuals with a study description. A member of the study team reached out to participants who gave their clinician authorization to pass along their names and contact information. Prospective participants received a detailed description of the study before being evaluated for eligibility. Inclusion criteria were (1) being 18 years or older; (2) having a diagnosis of schizophrenia, schizoaffective disorder, or psychosis not otherwise specified; and (3) the occurrence of an inpatient psychiatric hospitalization, daytime psychiatric hospitalization, outpatient crisis management visit, or short-term psychiatric hospital emergency room visit within the last 12 months. Individuals were excluded if they (1) had hearing, vision, or motor impairments that would interfere with the use of a smartphone (determined using a demonstration smartphone during screening); (2) had a reading level below 6th grade [determined using the reading section of the Wide Range Achievement Test; (31)]; or (3) a lack of competency to consent.
Procedure
Participants were randomized to one of two conditions: (1) the intervention condition (i.e., access to the CrossCheck system and as needed follow-up for 12 months), or (2) treatment as usual (i.e., no change to participants usual care over the same period). Participants in both conditions were asked to attend in-person data collection visits every 3 months. At these visits, participants were administered the Brief Psychiatric Rating Scale [BPRS; (32)], a 24-item interview-rated assessment of an array of symptoms of schizophrenia-spectrum disorders. Baseline characteristics of participants—including those who experienced a relapse and those who did not during the study period (33)—are reported elsewhere.
Data for the present report are drawn from participants (n = 61) randomized to the CrossCheck condition. All participants in this condition were asked to carry a study device with them for 12 months. These devices were Samsung Galaxy S5 Android smartphones with unlimited data plans. Participants were instructed to charge the device each night while sleeping to reduce instances of missing data. This device had an integrated mobile monitoring assessment system called CrossCheck pre-installed. CrossCheck deployed EMA self-report scales and collected data from sensors that are embedded in most contemporary smartphones including accelerometers and Global Position System (GPS). A full description of the study software (28–30) as well as previous results involving passive sensing are reported elsewhere (33, 34).
Ecological Momentary Assessment (EMA)
CrossCheck prompted participants to complete a 10-item EMA self-report survey three times per week (i.e., all Mondays, Wednesdays, and Fridays), with the prompt, “Just checking in to see how you've been doing over the last few days.” A full list of EMA items can be found in Table 1; response options to each question ranged from 0 (not at all) to 3 (extremely). In addition to symptoms of psychosis, EMA items also assessed overall mental health (e.g., stress, depression, hopefulness, calmness, clarity of thought), and functioning (e.g., socialization, sleep). For the present study, we identified five variables based on research (11, 12, 27) suggesting that negative mood, anxiety, hallucinations, and delusions are elevated in assessments that precede relapses. Two of those items—persecutory ideation, and self-reported sleep quality—were assessed with a single item, while three—negative mood (average of the “depression” item and reverse-coded “hopefulness” item), anxiety (“stress” item and reverse-coded “calm” item), and hallucinations (average of “seeing things” and “voices” items)—were assessed as composite scores. These items are listed in Table 1.
Assessment of Relapse
Hospital electronic health record data were made available to the research team for tracking psychiatric relapses during the study period. Trained study staff identified potential relapses from this record with final confirmation by the study PI (DBZ). The following events, reported during study assessments or recorded in the medical record, were designated as relapses: psychiatric hospitalization, significant increase in the level of psychiatric care (i.e., frequency and intensity of services, dosage increase or additional medicines prescribed) together with either an increase of 25% from baseline on BPRS total score, suicidal or homicidal ideation that was clinically significant in the investigators' judgment, deliberate self-injury, or violent behavior resulting in damage to another person or property (35). When documentation of the relapse date was unavailable (e.g., instances of self-reported suicidal ideation that did not lead to hospitalization or suicide attempt) assessors gathered data about the specific relapse event date directly from study participants.
Data Analytic Plan
We deployed latent growth curve analysis within a multilevel modeling framework to model gradual changes in symptoms over 100-day periods before and after relapse, as well as sudden changes in symptoms before vs. after relapse. Specifically, we modeled discontinuous growth curves to characterize separate trajectories of changes in symptoms over time before and after relapse and sudden changes in symptoms on the day of relapse. Similar analytic approaches have been used to model gradual and sudden changes in symptoms for other disorders (e.g., substance use disorders) with respect to other significant events [e.g., initiating abstinence from alcohol (36) or tobacco (37)]. Growth curve models included fixed effects for (a) linear change over time in the 100 days before relapses, (b) linear change for the 100 days following the relapse, and (c) sudden changes in expected values from the end of the pre-relapse period to the beginning of the post-relapse period. Random subject-level terms for all three of these effects were also entered into this model, as well as a random intercept, to account for between-subject heterogeneity in symptom trajectories. Growth curve models were analyzed within a multilevel modeling framework (repeated measures nested within persons) and a separate model was fit for each symptom measure. Models were fit using maximum likelihood estimation, which reduces bias and improves precision when data are missing at random or missing completely at random (38).
Time variables for modeling gradual change were scaled such that a one-unit increase in time reflected a 1-week period for the pre or post relapse period, and the time variable reflecting sudden change was dummy coded as 0 during the pre-relapse period and 1 in the post-relapse period. Thus, model coefficients indicated the rate of change in symptoms per week during the pre or post relapse period, and the amount of sudden change in symptoms when transitioning from pre relapse to post relapse (beyond what was attributable to the gradual change over time trajectories). Because our analyses aimed to examine gradual changes over periods consistent with previous research (27), our models included EMA data within 100 days before or after relapse.
For individuals with more than one psychiatric relapse (n = 4), each relapse was included in the analysis and time variables reflecting “weeks to relapse” and “weeks from relapse” were split at the halfway point between the two relapses. In a sensitivity analysis, we conducted analyses a second time with second relapses excluded for these four participants and an identical pattern of results were found. EMA variables were person-mean centered such that the zero point in all models represented the participant's average EMA response during the full study period. This allowed for interpretation of intercepts as the model-inferred difference between participants' expected symptom ratings on the day of relapse compared to their overall average response to that EMA item during the study period.
Results
Relapses
Twenty-seven relapse events occurred during the study period across 20 participants; models predicting relapse examine this subset of individuals. Of those events, they were characterized (non-mutually exclusively) as follows: psychiatric hospitalization (n = 22, 81.48%), increased frequency of services (n = 7, 25.93%), increased medication and BPRS increase (n = 6, 22.22%), suicidal ideation (n = 4, 14.81%) homicidal ideation (n = 1, 3.70%), self-injury (n = 2, 7.41%), violence (n = 1, 3.70%). At baseline, participants who went on to relapse did not significantly differ from those who did not relapse with regard to age, gender, race, ethnicity, diagnosis, or number of past psychiatric hospitalizations (past year or lifetime).
All model estimates are provided in Tables 2, 3. Parameter estimates in each model represent (1) the average participant-centered EMA value on the relapse day (“Intercept”), (2) immediate changes that occur from the end of the pre-relapse period to the beginning of the post-relapse period (“Immediate change”), (3) rates of change in the EMA values per week before relapse (“Gradual pre-relapse change”), and (4) rates of change in the EMA values after relapse (“Gradual post-relapse change”). All model-implied growth curves are displayed in Figures 1, 2.
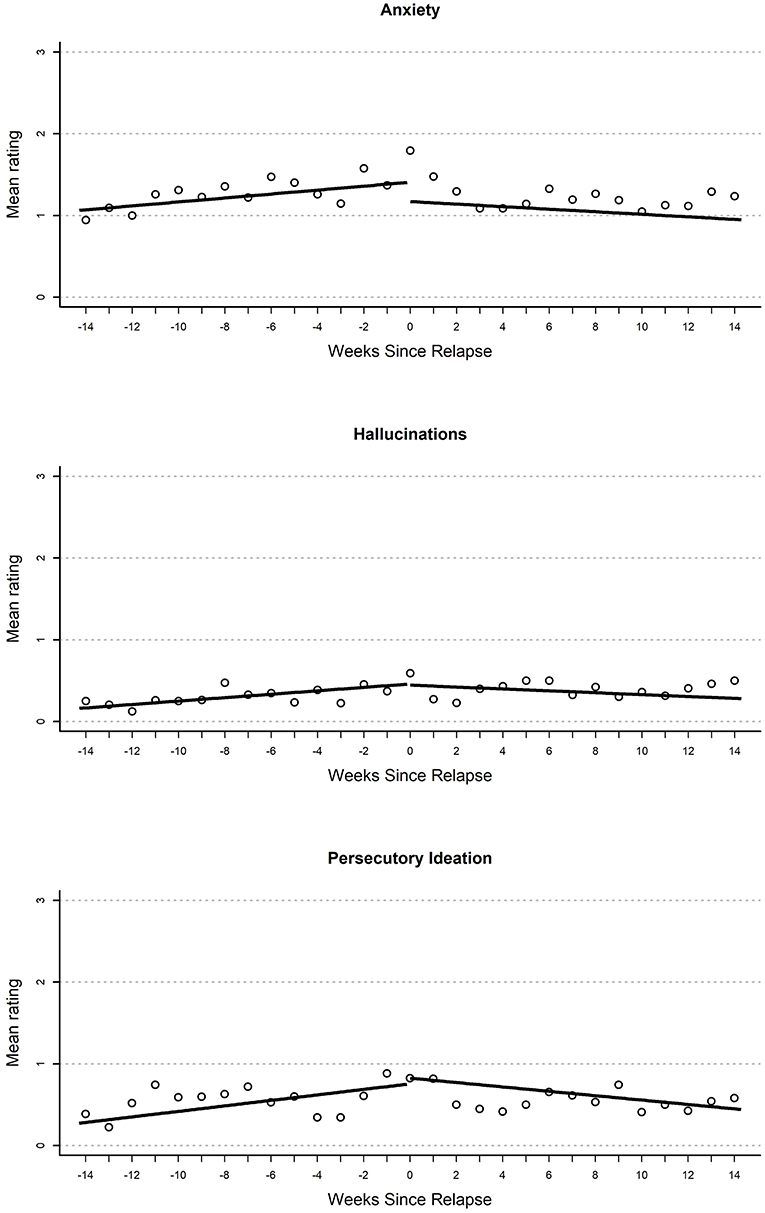
Figure 1. Plot of mean EMA rating for anxiety (top), hallucinations (middle), and persecutory ideation (bottom) variables by weeks until or since the relapse event.
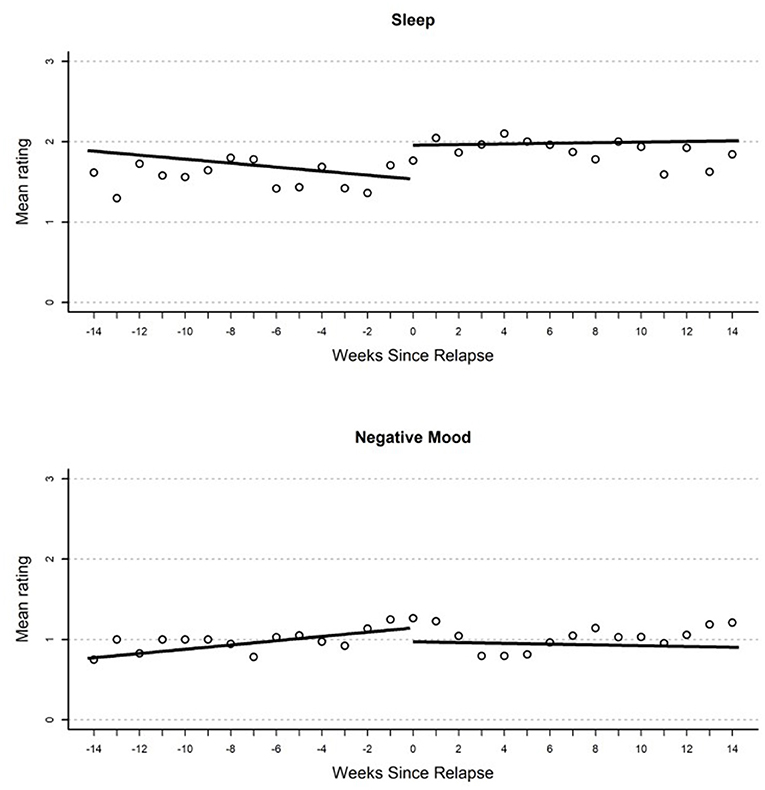
Figure 2. Plot of mean EMA rating for sleep (top) and negative mood (bottom) by weeks until or since the relapse event.
Negative Mood
Results from growth curve models examining change in EMA report of negative mood over time are reported in the first heading of Table 2. On the day of relapse, participants had significantly more negative mood compared to their average in the study (d = 0.34). Overall, this suggests a significantly elevated negative mood when relapse occurred (see Figure 1). There were no other significant effects in the model; however, there was a non-significant (p = 0.054) gradual increase in negative mood (d = 0.04 per week) that over the 100-day pre-relapse period.
Anxiety
Results from growth curves examining anxiety are reported in the second heading of Table 2. On the day of relapse, participants had model-implied ratings that indicated a statistically significant elevation in anxiety (d = 0.49). There were no other significant terms in the model. Overall, this provides support for elevations when relapse occurred (see Figure 1). There was a nominal gradual increase that occurred during the 100-day pre-relapse period (d = 0.04 per week, p = 0.075), as well as an immediate decrease when the relapse occurred (d = −0.45, p = 0.06), but both of these effects were non-significant.
Sleep
Parameter estimates from growth curves related to sleep are reported in the third heading of Table 2. There were no significant effects in the model. There were two non-significant effects of similar magnitude including a reduction in reported quality of sleep on the day of relapse relative to average in the study period (d = 0.41), as well as an immediate increase in sleep quality (d = 0.64) when the relapse period began.
Persecutory Ideation
Growth curve models examining persecutory ideation are reported in the first heading of Table 3. On the day of relapse, models inferred an elevated persecutory ideation score relative to participants' mean response (d = 0.35). This resulted from a significant linear increase during the 100-day pre-relapse period (d = 0.05 per week). Overall, this curve seems to suggest a steady but small increase in persecutory ideation over time before relapse and an elevation as the relapse occurred. There were no other significant effects in this model; however, there was a non-significant (p = 0.08) negative slope in the post-relapse period (d = 0.04).
Hallucinations
Parameter estimates from growth curves related to hallucinations are reported in the second heading of Table 3. On the day of relapse, models inferred an elevated rating of hallucinations relative to the participant mean (d = 0.34). This resulted from a significant positive slope in the 100-day pre-relapse period (d = 0.05 per week). No significant immediate change was found from the pre-relapse to post-relapse period or during the post-relapse period. Overall, this model suggests a steady increase in hallucinations in pre-relapse period, as well as an elevation relative to average when relapse occurs (see Figure 2).
Discussion
Results demonstrated that a brief mobile EMA system detected changes in self-reported mood and psychotic symptoms that occurred in the period preceding relapse, both gradually over time before the relapse and immediately as psychiatric relapses occur. A series of growth curve models suggested that there were elevations in negative mood (d = 0.34), anxiety (d = 0.49), persecutory ideation (d = 0.35), and hallucinations (d = 0.34) on relapse days relative to average, and steady increases in persecutory ideation and hallucinations were evident in the 100-day period preceding relapse. These increases represented a small effect (d = 0.05 for both hallucinations and persecutory ideation) each week for the duration of the pre-relapse period. This suggests that brief assessments (i.e., 1–2 items) may register changes in each of these domains that occur in the 100 days that precede psychiatric relapses. Brief assessments of persecutory ideation and hallucinations, administered multiple times per week, appear to detect a signal that could indicate risk.
In general, the present study provides preliminary support for the validity of EMA in providing information relevant to consequential clinical events. A brief EMA questionnaire appeared to detect changes in relation to meaningful and impactful clinical events. Because the financial and resource cost to administer EMA questionnaires is so low, the growing evidence supporting the utility of this information makes a compelling case for the use of remote monitoring in routine clinical care. The questionnaire deployed in the present study is brief (i.e., <2 min to complete), technologically simple, and does not require clinical staff to administer or calculate total scores. Indications of risk from EMA could trigger clinician outreach, indicate an increase in in-person sessions, or be used to encourage deployment of evidence-based mHealth self-management interventions directly to patients (39, 40). Our results suggested that low-level symptoms appeared to build up in the period leading up to relapse. Such subtle changes that may not be noticeable to individuals could potentially be detected with routine monitoring systems. Clinical interventions aiming to prevent or reduce the impact of psychiatric relapses may benefit from targeting individuals who sustain increases in these domains over time. Interventions that provide support and self-management strategies, even in response to small symptom increases, could provide benefits that prevent a costly and debilitating full relapse.
One limitation of the present study pertains to the size of the sample, as only 20 participants in the study relapsed during the study period, this limits statistical power to identify population-level trends in self-reported symptoms. While the lack of significance of these effects precludes further interpretation, there were several effects that fell just below (i.e., p <0.10) the threshold for statistical significance: gradual increases in the pre-relapse period in negative mood and anxiety, reductions in reported quality of sleep at the time of relapse, immediate post-relapse reductions in anxiety and sleep problems, and gradual post-relapse reductions in persecutory ideation. The study's lack of power may have reduced likelihood of finding significance. Future studies with larger samples, longer EMA periods, and more frequent assessments have the potential to clarify these areas. Second, as our EMA was administered on Monday, Wednesday, and Friday each week for the study period, there are many days during which participants did not provide any data. It is possible periods of missing data are aligned with precisely the periods during which participants are at greatest risk for relapse, as it may be difficult to complete EMA questionnaires when experiencing severe symptoms. Future longitudinal studies would be strengthened with a greater frequency of assessments, which could be feasible in light of the fact that participants with SMI in EMA studies report that they do not find these assessments to be burdensome (22, 24). While the questionnaire deployed assessed a range of symptoms, it was not designed for the purpose of assessment of early warning signs. Other systems examining EMAs have developed questionnaires expressly for this purpose (27). Importantly, during the CrossCheck study, members of the study team reached out to several participants who appeared at risk of relapse. Though clinically and ethically indicated in the study protocol, it is possible that taking these steps reduced the number of relapses during the study period and thus attenuated the strength of the relationships between EMA self-reported symptoms and relapse. Last, research examining emerging technologies using EMA along with passive sensors in this population has noted that the application of these data may be most useful ideographically and not only a population scale (41, 42). While the present study took such a population-based approach, this does not rule out idiosyncratic patterns (i.e., “relapse signatures”) (28) that characterize specific individualized risk. As clinical EMAs continue to expand into research and routine clinical care, future studies should continue to characterize trajectories of symptom change that precede and follow relapses in larger samples and with greater granularity.
The present study characterizes in greater depth the temporal changes in symptoms that characterize schizophrenia-spectrum disorders. In addition to reporting elevations in anxiety, negative mood, hallucinations and persecutory ideation right as relapses occur, participants also reported significant gradual increases, week-by-week, in persecutory ideation and hallucination in the lead up to relapses. Though brief and easy to administer, self-reported symptoms could provide critical information for remote measurement-based care management potentially aiding in detecting elevated risk and monitoring response to interventions (43). Applying this approach in psychiatry would mirror preventive interventions for physical health, for example, the treatment of risk factors for cardiovascular disease to prevent heart attacks rather than initiating treatment after they occur. If deployed proactively, psychiatric interventions could similarly reduce the significant impact of SSDs on healthcare systems and the lives of those affected.
Data Availability Statement
The datasets presented in this study can be found in online repositories. The names of the repository/repositories and accession number(s) can be found below: The data analyzed for this study can be found at: https://www.mh4mh.org/eureka-data.
Ethics Statement
The studies involving human participants were reviewed and approved by IRBs of Dartmouth College (#24356) and Northwell Health/Long Island Jewish Medical Center (#14-100B) and registered as a clinical trial (#NCT01952041). The patients/participants provided their written informed consent to participate in this study.
Author Contributions
BB conducted the literature search, conducted data analysis, and wrote the first and subsequent revised drafts of the manuscript. KH helped devise the data analytic strategy and assisted in generation of figures. DB-Z, AC, TC, and JK oversaw the study resulting in this publication, including drafting the grant application, designing the study protocol, and supervising research staff managing study activities. AC and TC developed and refined mobile technologies employed. DB-Z supervised the drafting and revising of the manuscript. All authors contributed to and approved the final version of the manuscript.
Funding
This project was funded by the NIMH Exceptional Unconventional Research Enabling Knowledge Acceleration (EUREKA) Program grant number R01MH103148 (PI: DB-Z). Dr. Buck is supported by a Mentored Patient-Oriented Research Career Development Award from NIMH (K23MH122504). Dr. Hallgren is supported by a Mentored Research Scientist Development Award from NIAAA (K01AA024796).
Conflict of Interest
DB-Z has an intervention content licensing agreement with Pear Therapeutics and has financial interest in FOCUS technology. He has consulted for Trusst Health Inc., eQuility, and Otsuka Pharmaceuticals Ltd. TC is a co- founder and holds equity stake at HealthRhythms Inc. JK has been a consultant for or received honoraria from Alkermes, Allergan, Dainippon Sumitomo, H. Lundbeck. Intracellular Therapies, Janssen Pharmaceutica, Johnson and Johnson, LB Pharmaceuticals, Merck, Minerva, Neurocrine, Otsuka, Reviva, Roche, Saladex, Sunovion, Takeda and Teva. He has received grant support from Otsuka, Lundbeck, and Janssen. JK is also a shareholder in Vanguard Research Group, LB Pharmaceuticals, Inc. and North Shore Therapuetics. KH has been a consultant for Pear Therapeutics.
The remaining authors declare that the research was conducted in the absence of any commercial or financial relationships that could be construed as a potential conflict of interest.
Acknowledgments
We are grateful for the participants' engagement in the project, as well as discussions at the BRiTE Center and with our collaborators elsewhere that enhanced the quality of this work.
References
1. Ascher-Svanum H, Zhu B, Faries DE, Salkever D, Slade EP, Peng X, Conley RR. The cost of relapse and the predictors of relapse in the treatment of schizophrenia. BMC Psychiatry. (2010) 10:2. doi: 10.1186/1471-244X-10-2
2. Almond S, Knapp M, Francois C, Toumi M, Brugha T. Relapse in schizophrenia: costs, clinical outcomes and quality of life. Br J Psychiatry. (2004) 184:346–51. doi: 10.1192/bjp.184.4.346
3. Emsley R, Chiliza B, Asmal L, Harvey BH. The nature of relapse in schizophrenia. BMC Psychiatry. (2013) 8:50. doi: 10.1186/1471-244X-13-50
4. Takeuchi H, Siu C, Remington G, Fervaha G, Zipursky RB, Foussias G, et al. Does relapse contribute to treatment resistance? Antipsychotic response in first- vs. second-episode schizophrenia. Neuropsychopharmacology. (2019) 44:1036–42. doi: 10.1038/s41386-018-0278-3
5. Chung DT, Ryan CJ, Hadzi-Pavlovic D, Singh SP, Stanton C, Large MM. Suicide rates after discharge from psychiatric facilities: a systematic review and meta-analysis. JAMA Psychiatry. (2017) 74:694–702. doi: 10.1001/jamapsychiatry.2017.1044
6. Weiden PJ, Olfson M. Cost of relapse in schizophrenia. Schizophr Bull. (1995) 21:419–29. doi: 10.1093/schbul/21.3.419
7. Vos T, Allen C, Arora M, Barber RM, Bhutta ZA, Brown A, et al. Global, regional, and national incidence, prevalence, and years lived with disability for 310 diseases and injuries, 1990-2015: a systematic analysis for the Global Burden of Disease Study 2015. Lancet. (2016) 388:1545–602. doi: 10.1016/S0140-6736(16)31678-6
8. Chong HY, Teoh SL, Wu DB-C, Kotirum S, Chiou C-F, Chaiyakunapruk N. Global economic burden of schizophrenia: a systematic review. Neuropsychiatr Dis Treat. (2016) 12:357–73. doi: 10.2147/NDT.S96649
9. Evensen S, Wisløff T, Lystad JU, Bull H, Ueland T, Falkum E. Prevalence, employment rate, and cost of schizophrenia in a high-income welfare society: a population-based study using comprehensive health and welfare registers. Schizophr Bull. (2016) 42:476–83. doi: 10.1093/schbul/sbv141
10. Knapp M, Mangalore R, Simon J. The global costs of schizophrenia. Schizophr Bull. (2004) 30:279–93. doi: 10.1093/oxfordjournals.schbul.a007078
11. Tarrier N, Barrowclough C, Bamrah JS. Prodromal signs of relapse in schizophrenia. Soc Psychiatry Psychiatr Epidemiol. (1991) 26:157–61. doi: 10.1007/BF00795207
12. Wang D, Gopal S, Baker S, Narayan VA. Trajectories and changes in individual items of positive and negative syndrome scale among schizophrenia patients prior to impending relapse. NPJ Schizophr. (2018) 4:10. doi: 10.1038/s41537-018-0056-6
13. Kessler RC, Berglund PA, Bruce ML, Koch JR, Laska EM, Leaf PJ, et al. The prevalence and correlates of untreated serious mental illness. Health Serv Res. (2001) 36:987–1007.
14. Blum LH, Vakhrusheva J, Saperstein A, Khan S, Chang RW, Hansen MC, et al. Depressed mood in individuals with schizophrenia: a comparison of retrospective and real-time measures. Psychiatry Res. (2015) 227:318–23. doi: 10.1016/j.psychres.2015.03.008
15. Khan A, Yavorsky WC, Liechti S, Diclemente G, Rothman B, Opler M, et al. Assessing the sources of unreliability (rater, subject, time-point) in a failed clinical trial using items of the positive and negative syndrome scale (PANSS). J Clin Psychopharmacol. (2013) 33:109–17. doi: 10.1097/JCP.0b013e3182776ebe
16. Ben-Zeev D, McHugo GJ, Xie H, Dobbins K, Young MA. Comparing retrospective reports to real-time/real-place mobile assessments in individuals with schizophrenia and a nonclinical comparison group. Schizophr Bull. (2012) 38:396–404. doi: 10.1093/schbul/sbr171
17. Shiffman S, Stone AA, Hufford MR. Ecological momentary assessment. Annu Rev Clin Psychol. (2008) 4:1–32. doi: 10.1146/annurev.clinpsy.3.022806.091415
18. Firth J, Cotter J, Torous J, Bucci S, Firth JA, Yung AR. Mobile phone ownership and endorsement of “mHealth” among people with psychosis: a meta-analysis of cross-sectional studies. Schizophr Bull. (2016) 42:448–55. doi: 10.1093/schbul/sbv132
19. Bell IH, Lim MH, Rossell SL, Thomas N. Ecological momentary assessment and intervention in the treatment of psychotic disorders: a systematic review. Psychiatr Serv. (2017) 68:1172–81. doi: 10.1176/appi.ps.201600523
20. Eisner E, Drake RJ, Berry N, Barrowclough C, Emsley R, Machin M, et al. Development and long-term acceptability of ExPRESS, a mobile phone app to monitor basic symptoms and early signs of psychosis relapse. J Med Internet Res. (2019) 7:e11568. doi: 10.2196/11568
21. Granholm E, Loh C, Swendsen J. Feasibility and validity of computerized ecological momentary assessment in schizophrenia. Schizophr Bull. (2008) 34:507–14. doi: 10.1093/schbul/sbm113
22. Palmier-Claus JE, Rogers A, Ainsworth J, Machin M, Barrowclough C, Laverty L, et al. Integrating mobile-phone based assessment for psychosis into people's everyday lives and clinical care: a qualitative study. BMC Psychiatry. (2013) 13:34. doi: 10.1186/1471-244X-13-34
23. Kumar D, Tully LM, Iosif A-M, Zakskorn LN, Nye KE, Zia A, et al. A mobile health platform for clinical monitoring in early psychosis: implementation in community-based outpatient early psychosis care. JMIR Ment Health. (2018) 5:e15. doi: 10.2196/mental.8551
24. Niendam TA, Tully LM, Iosif AM, Kumar D, Nye KE, Denton JC, et al. Enhancing early psychosis treatment using smartphone technology: a longitudinal feasibility and validity study. J Psychiatr Res. (2018) 96:239–46. doi: 10.1016/j.jpsychires.2017.10.017
25. Španiel F, Vohlídka P, Hrdlička J, KoŽený J, Novák T, Motlová L, et al. ITAREPS: Information Technology Aided Relapse Prevention Programme in Schizophrenia. Schizophr Res. (2008) 98:312–7. doi: 10.1016/j.schres.2007.09.005
26. Španiel F, Hrdlička J, Novák T, KoŽený J, Höschl C, Mohr P, et al. Effectiveness of the Information Technology-Aided Program of Relapse Prevention in Schizophrenia (ITAREPS). J Psychiatr Pract. (2012) 18:269–80. doi: 10.1097/01.pra.0000416017.45591.c1
27. Spaniel F, Bakstein E, Anyz J, Hlinka J, Sieger T, Hrdlicka J, et al. Relapse in schizophrenia: definitively not a bolt from the blue. Neurosci Lett. (2018) 669:68–74. doi: 10.1016/j.neulet.2016.04.044
28. Ben-Zeev D, Brian R, Wang R, Wang W, Campbell AT, Aung MSH, et al. CrossCheck: Integrating self-report, behavioral sensing, and smartphone use to identify digital indicators of psychotic relapse. Psychiatr Rehabil J. (2017) 40:266–75. doi: 10.1037/prj0000243
29. Wang R, Aung MSH, Abdullah S, Brian R, Campbell AT, Choudhury T, et al. CrossCheck: Towards passive sensing and detection of mental health changes in people with schizophrenia. Proc Int Conf Ubiquitous Comput. (2016) 886–97. doi: 10.1145/2971648.2971740
30. Wang R, Scherer EA, Walsh M, Wang W, Aung MSH, Ben-Zeev D, et al. Predicting symptom trajectories of schizophrenia using mobile sensing. Proc ACM Interact Mob Wearab Ubiquit Technol. (2017) 1:1–24. doi: 10.1145/3130976
31. Wilkinson G, Robertson G. Wide Range Achievement Test, 4th Edition (WRAT-4) Professional Manual. Lutz, FL: Psychological Assessment Resources (2004).
32. Crippa J a, Sanches RF, Hallak JE, Loureiro SR, Zuardia W. A structured interview guide increases Brief Psychiatric Rating Scale reliability in raters with low clinical experience. Acta Psychiatr Scand. (2001) 103:465–70. doi: 10.1034/j.1600-0447.2001.00185.x
33. Buck B, Scherer E, Brian R, Wang R, Wang W, Campbell A, et al. Relationships between smartphone social behavior and relapse in schizophrenia: a preliminary report. Schizophr Res. (2019) 208:167–72. doi: 10.1016/j.schres.2019.03.014
34. Buck B, Hallgren K, Scherer EA, Brian R, Wang R, Wang W, et al. Capturing behavioral indicators of persecutory ideation using mobile technology. J Psychiatr Res. (2019) 116:112–7. doi: 10.1016/j.jpsychires.2019.06.002
35. Csernansky JG, Schuchart EK. Relapse and rehospitalisation rates in patients with schizophrenia: effects of second generation antipsychotics. CNS Drugs. (2002) 16:473–84. doi: 10.2165/00023210-200216070-00004
36. Hallgren KA, Epstein EE, McCrady BS. Changes in hypothesized mechanisms of change before and after initiating abstinence in cognitive-behavioral therapy for women with alcohol use disorder. Behav Ther. (2019) 50:1030–41. doi: 10.1016/j.beth.2019.01.009
37. Rubin LF, Haaga DAF, Pearson JL, Gunthert KC. Depression as a moderator of the prospective relationship between mood and smoking. Heal Psychol. (2019) 39:99–106. doi: 10.1037/hea0000816
38. Hallgren KA, Witkiewitz K. Missing data in alcohol clinical trials: a comparison of methods. Alcohol Clin Exp Res. (2013) 37:2152–60. doi: 10.1111/acer.12205
39. Ben-Zeev D, Brian RM, Jonathan G, Razzano L, Pashka N, Carpenter-Song E, et al. Mobile Health (mHealth) versus clinic-based group intervention for people with serious mental illness: a randomized controlled trial. Psychiatr Serv. (2018) 69:978–85. doi: 10.1176/appi.ps.201800063
40. Ben-Zeev D, Scherer EA, Gottlieb JD, Rotondi AJ, Brunette MF, Achtyes ED, et al. mHealth for schizophrenia: patient engagement with a mobile phone intervention following hospital discharge. JMIR Ment Health. (2016) 3:e34. doi: 10.2196/mental.6348
41. Wisniewski H, Henson P, Torous J. Using a smartphone app to identify clinically relevant behavior trends via symptom report, cognition scores, and exercise levels: a case series. Front Psychiatry. (2019) 10:652. doi: 10.3389/fpsyt.2019.00652
42. Barnett I, Torous J, Staples P, Sandoval L, Keshavan M, Onnela JP. Relapse prediction in schizophrenia through digital phenotyping: a pilot study. Neuropsychopharmacology. (2018) 43:1660–6. doi: 10.1038/s41386-018-0030-z
Keywords: technology, mobile health, schizophrenia, relapse, ecological momentary assessment
Citation: Buck B, Hallgren KA, Campbell AT, Choudhury T, Kane JM and Ben-Zeev D (2021) mHealth-Assisted Detection of Precursors to Relapse in Schizophrenia. Front. Psychiatry 12:642200. doi: 10.3389/fpsyt.2021.642200
Received: 15 December 2020; Accepted: 03 May 2021;
Published: 31 May 2021.
Edited by:
Lina Gega, University of York, United KingdomReviewed by:
Ana Alves Francisco, Albert Einstein College of Medicine, United StatesMassimo Tusconi, University of Cagliari, Italy
Copyright © 2021 Buck, Hallgren, Campbell, Choudhury, Kane and Ben-Zeev. This is an open-access article distributed under the terms of the Creative Commons Attribution License (CC BY). The use, distribution or reproduction in other forums is permitted, provided the original author(s) and the copyright owner(s) are credited and that the original publication in this journal is cited, in accordance with accepted academic practice. No use, distribution or reproduction is permitted which does not comply with these terms.
*Correspondence: Benjamin Buck, YnVja2JlQHV3LmVkdQ==