- 1Faculty of Education, Western University, London, ON, Canada
- 2Faculty of Applied Health Sciences, University of Waterloo, Waterloo, ON, Canada
Youth violence is considered one of the most preventable causes of morbidity and premature mortality. Various risk factors have previously been identified, however, there is presently a crucial need to develop effective decision-support tools in order to identify children and youth at increased risk for violence. The current study utilised data collected from the interRAI Child and Youth Mental Health Screener (ChYMH-S), within the province of Ontario, to develop and validate a methodology for the purpose of identifying young persons who were at greater risk of harm to others. Additional data from 59 mental health agencies validated the algorithm, and it was found to be a strong predictor of harmful behaviour toward others. The RIO algorithm provides a valuable decision-support tool with strong psychometric properties that may be used to identify young persons who exhibit signs or symptoms associated with increased likelihood of harm toward others, in order to provide early intervention efforts for these vulnerable youth, thereby reducing the likelihood of future aggressive behaviours.
Introduction
Childhood physical aggression is an important public health concern, as it has the potential to lead to more serious, violent behaviours, resulting in a plethora of adverse consequences (1, 2). Violence among youth is considered one of the most preventable causes of morbidity and premature mortality, with homicide continuing to be one of the leading causes of death for young people between the ages of 10–24 (3, 4). Notably, the World Health Organisation has made a dedicated effort to focus on delineating risk factors of youth violence over the recent decades, moving toward a prevention model for violence (5, 6). Through the identification of modifiable risk factors of youth violence, preventative strategies could be implemented to reduce risk of aggression toward others.
Physical Aggression in Youth
While the age of onset for serious injury toward others typically does not occur before the age of 12, studies have found that the majority of children demonstrate physical aggression toward others by 17 months, although it is rare for such young children to cause serious harm (7–9). Most children will learn over time how to regulate their physical aggression; however, those who do not are at highest risk of engaging in serious violent behaviour later in life (2). From a developmental perspective, the precursors of chronic physical aggression are present before the child begins school, suggesting that the spontaneous onset of aggressive behaviours in school-aged children is quite atypical (2, 10, 11). Finally, as these precursors are present at such an early stage of life, it falls in line that a number of the most well-established risk factors can be found within the family context and environment [e.g., (12)].
Potential Risk Factors Regarding Injury Toward Others
A number of family factors have been implicated in the development of physical aggression and subsequent violent behaviour in children and youth. Tremblay and colleagues (12) reported that at 5 months old, the best predictors of a high physical aggression trajectory were coercive parenting and family dysfunction. Moreover, children who have been physically abused within their home are more likely to exhibit aggressive behaviour at school, engage in serious violent acts during their teenage years, and commit violent crimes as adults (13–15). Studies have also found that poor family management practises, such as low parental supervision and monitoring, severe and inconsistent discipline, and unclear expectations predict delinquency later on (16, 17).
Certain individual characteristics and behaviours have also been linked to harm toward others. Poor impulse control and emotion regulation have been associated with violent behaviour from childhood through to early adulthood (18, 19). Research has also found that antisocial behaviour presenting early in life can predict future violence, with disruptive behaviour in childhood being one of the best predictors of violent offending during adolescence and adulthood, particularly for boys (20–22). Further, early-onset conduct problems, such as engaging in destructive behaviours, have repeatedly been identified as important predictors of future violent and criminal acts (23, 24).
Finally, the literature has found that injury toward others is an enduring and robust predictor of future violent acts (25). Children who exhibit chronic physical aggression during their elementary school years are more likely to continue engaging in physical violence during adolescence (2). Importantly, the link between early onset of aggression to more serious and chronic violent behaviour is evidenced in numerous studies; for example, the Denver Youth Study reported that 62% of children who engaged in violent behaviours at 9 years of age or younger became chronic violent offenders during adolescence (26–28). Another study reported that two-thirds of boys who were highly aggressive at 10–13 years of age had criminal records of violent offences by the age of 26. This represented a 6-fold increase compared to those who had low aggression (29). In addition to a history of aggressive behaviour, pro-violence attitudes are also linked to the onset and perpetration of violence among youth (23, 30).
The current study's objective was to develop and validate a methodology for identifying young persons who are at heightened risk of harming others utilising a comprehensive instrument used as standard of care in many mental health agencies within the province of Ontario, Canada. A validated methodological approach to identify adults who are at risk of harm to others (RHO) has previously been developed by interRAI (31). interRAI is an international non-profit collaborative committed to improving the lives of vulnerable persons across the lifespan. In particular, the interRAI child and youth suite of instruments was designed to facilitate a standardised, comprehensive, and coordinated approach to the delivery of mental health services for infants, toddlers, children and youth. An initiative was undertaken to develop a new decision-support algorithm for identifying youth at greatest risk of harm to others by harnessing the power of the existing interRAI assessment system, given that no system for such identification currently exists. A similar methodology utilised in the RHO was applied, creating the Risk of Injury to Others (RIO) algorithm, to assist service providers in determining whether a child or youth was at high risk of harming other individuals. This article describes the development and validation efforts of the RIO algorithm.
Methods
Sample
The method used in the development and validation of the RIO algorithm parallels that of an algorithm that we have previously developed, the Risk of Suicide and Self-Harm (RiSsK) (32). The following is an abbreviated version of the methodology. For a more detailed and comprehensive account of our Methods, please refer to our previous work (32).
The participants of this study were children and youth who received mental health services from Ontario health agencies. Notably, the study drew from four sample populations for the different stages of the methodology: (1) derivation, (2) validation, (3) descriptive analyses, and (4) longitudinal analyses. Data from the Child and Youth Mental Health Screener (ChYMH-S) (33) were used in both the derivation and validation stages. The RIO algorithm was derived using 60,414 records from 54,280 unique individuals, collected between September 1, 2015 and January 31, 2019. The participants had a mean age of 11.8 years with males comprising 49.8% of the sample (SD 3.74, range 4–18 years). Following the derivation stage, secondary data analyses were completed to validate the algorithm using 2,117 records from 2,098 unique individuals that were completed between February 1, 2019 and March 5, 2019. The participants had a mean age of 11.7 years with males comprising 49.0% of the sample (SD 3.67, range 4–18 years). Fifty-nine mental health organisations were included in the original development efforts of the RIO algorithm. There were no differences in the methods or sources between the derivation and validation samples.
In the post-scale development stage, two additional related sources of data were used to conduct (1) additional descriptive analyses related to diagnoses and (2) longitudinal analyses related to predictive validity. These data sources included the Child and Youth Mental Health (ChYMH) (34) and the Child and Youth Mental Health and Developmental Disability (ChYMH-DD) (35). To conduct the analyses related to diagnoses, a sample of 25,104 ChYMH and ChYMH-DD assessments on 13,899 unique individuals was used, completed between September 1, 2015 and January 31, 2019. The participants had a mean age of 12.1 years and males made up 57.0% of the sample (SD 3.51, range 4–18 years). To conduct the longitudinal analyses, a sample of 6,608 ChYMH-S, ChYMH, and ChYMH-DD assessments on 5,542 unique individuals was used, completed between November 4, 2015 and January 31, 2019. The participants had a mean age of 11.5 years and males made up 58.3% of the sample (SD 3.53, range 4–18 years).
The three aforementioned assessment tools (i.e., ChYMH, ChYMH-DD, and ChYMH-S) are used routinely as the standard of care in Ontarian mental health agencies. Thus, the inclusion criteria for this study consisted of children and adolescents between the ages of 4–18 years who presented at mental health facilities utilising the interRAI child/youth suite of instruments as standard of care.
Measures
The ChYMH-S is a relatively new assessment instrument developed by interRAI, a non-profit collaborative that is composed of researchers and clinicians from over 35 countries. It is a brief assessment tool utilised to assess, triage, and prioritise children and adolescents seeking mental health services.
Nearly 100 items comprise the ChYMH-S. The items are generally selected from the larger comprehensive Child and Youth Mental Health assessment (34), with some additional items specific to screening purposes. The full interRAI ChYMH and ChYMH-DD assess mental health needs more extensively. These comprehensive tools consist of ~400 items that are used to assess psychiatric, social, environmental, and medical issues for children and youth. The ChYMH, ChYMH-DD, and ChYMH-S are divided into various subsections, such as mental state indicators, education, and behaviour. Further, the tool is supported by a detailed training manual containing coding rules for all items. The result is a reliable and valid assessment that can be used for a number of different purposes (e.g., case documentation and program planning) (36).
Procedure
The ChYMH, ChYMH-S, and ChYMH-DD were routinely administered as part of the standard of care for young persons seeking mental health services in 59 agencies across the Province of Ontario. Assessors gathered information face-to-face or via telephone using a semi-structured interview format, from all available sources (e.g., conversations with parents/guardians, the child, and teachers; medical and education records; and clinical observations).
Secure web-based software was implemented to record assessment information. Before making the data available for analysis, personal identifiers were removed. Ethics approval was obtained from Western University's ethics review board to conduct secondary analyses on data collected in various Ontarian mental health agencies (REB #106415).
Analysis
The intended use of the algorithm is to predict those at highest risk of injury to others based on an ordinal summary score in order to help facilitate early intervention efforts for these vulnerable youth. Assessors were asked to record perceived risk of “danger to others” using a single ordinal item that ranges in value from 0 (minimal) to 4 (very severe or imminent), based on all evidence available to the assessor at that time. We used this estimate as the dependent variable to be predicted by a variety of items from the ChYMH-S. As such, the dependent variable is a subjective professional opinion, as opposed to an objective behavioural measure of aggression. Because this scale is intended to be used with the comprehensive ChYMH assessment, all of the independent variables included in the algorithm must be available on both the ChYMH-S and full ChYMH. Notably, the single item for risk of danger to others is not recorded in the full ChYMH instrument, as it is in the ChYMH Screener. All of the screening records were used for scale development in order to properly represent the population of the sample. For example, if a young person has been screened twice, such as within an inpatient and outpatient setting, both of their records would be included.
While various modelling options were explored, it was ultimately decided to use the simple unweighted mean clinician rating of risk as a starting point in these analyses. Modelling was done using an interactive decision tree tool, which is supported by the SAS Enterprise Miner package (37). The software employs both Chi-Square Automated Interaction Detection (CHAID) and Classification and Regression Trees (CART) to create decision trees for categorical or continuous dependent variables. While it is possible to use a fully automated process to generate decision trees, our approach was iterative in nature using both clinical judgement and statistical criteria to identify potential splitting rules in developing the final decision tree. SAS defaults to propose binary splits for suggested independent variables, but we consistently checked to determine whether trichotomous or more granular splits were warranted. Enterprise Miner identifies candidate variables for splits in decision trees based on statistical criteria such as variance reduction (for continuous variables), Gini Impurity (for nominal variables), or chi-squared tests of significance (for binary variables). The software orders candidate variables based on the strength of their statistical performance for each split, but it also allows the analyst to specify other splits based on substantive reasons. In some cases, the decision related to a specific split in the tree may be based on expected performance across multiple nodes rather than a single node. This allows the user to interactively control which variable is selected and explore alternative trees before proceeding.
A key strength of decision trees, as opposed to conventional regression models, is that it can naturally handle complex interactions that can identify important subgroups that would be difficult to identify with simple two-way multiplicative interaction terms. The end result after the analyst sequentially divides all cases into their respective nodes is a tree with mutually exclusive and exhaustive classifications. Attention was paid to not “overfit” the model with unreasonably small terminal nodes in the decision tree. In addition, in the derivation process, Enterprise Miner provides real time feedback on performance of each split in a virtual hold-back sample, which allows the analyst to avoid specifying splits that will be unstable across samples.
In decision tree modelling, the initial splits are particularly important. Forced splits were considered as initial splits, such as age and sex, in addition to top-ranking variables; however, the forced splits were not selected in our decision tree model because they failed to offer any additional explanatory power. Moreover, they resulted in some fragmentation and small cell sizes in some of the tree's branches. The final tree model was subsequently tested among both age and sex groups.
An important goal within the design of our RIO algorithm was for the final ordinal scale to have a compact range: 7 groups (labels of 0–6). Due to the large sample and numerous explanatory variables, decision trees could have 30 or more terminal nodes. As a result, some of the nodes needed to be combined after modelling, which was achieved using weighted k-means clustering. The end product was a parsimonious tree for which the final nodes could be logically assigned to one of the 7 groups.
Multinomial logistic regression was then employed using the seven groups of the algorithm to test model fit of the dependent variable, as well as provide the c-statistic [area under the receiver operator characteristic (ROC) curve] and odds ratios. This was repeated using the validation sample, which consisted of new screener assessments that had accrued since the derivation data work had begun—approximately a 5-week period. The next steps used a sample of 25,104 ChYMH and ChYMH-DD assessments, in which the RIO scale was calculated, and additional descriptive analyses related to diagnoses were conducted. Further, using a sample of 6,608 ChYMH-S, ChYMH, and ChYMH-DD assessments, the RIO scale was calculated, and longitudinal analyses related to predictive validity were conducted. All available initial screener assessments were included at time point 1. These were linked to the next assessment (either a screener, ChYMH, or ChYMH-DD) at time point 2, which was between 31 and 120 days in the future. The association between the subjective assessor rating of “danger to others” at baseline and five measures of violence at follow-up was examined. Additionally, the association between the RIO score at baseline and five measures of violence at follow-up was examined. Analyses were performed using SAS 9.4 and SAS Enterprise Miner 14.1.
Results
A schematic representation of the final RIO algorithm is presented in Figure 1. The RIO algorithm categorises young persons into levels of risk that suggest the need for heightened concern that the individual may be a danger to others, based on criteria as identified from the ChYMH-S. The final tree that was selected comprised of 21 terminal nodes, and used nine items from the ChYMH-S. All of the items included in the end product can be found on both the full ChYMH assessment and screener.
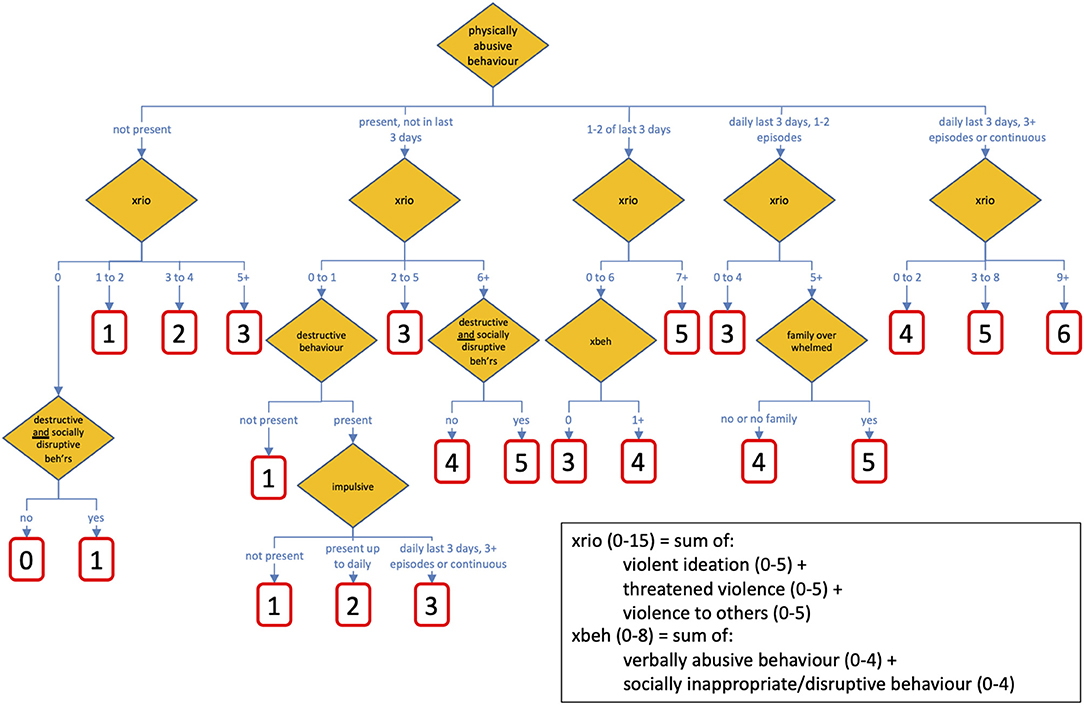
Figure 1. Risk of Injury to Others (RIO) decision tree diagram. Socially disruptive beh'rs, socially inappropriate/disruptive behaviours.
Groups were assigned a score from 0 (lowest) to 6 (highest) with higher scores indicating heightened risk of harm to others, as depicted in Table 1. The young person may fall into a given level via a number of different pathways that represent various combinations of the predictors. Highest risk was found in a small minority of young persons (~0.6% scored 6, the highest value on the RIO), in which 41.3% of these were rated as having a risk of harm to others that was severe, very severe, or imminent. Conversely, over half of those assessed were classified in the lowest risk group, in which only 0.08% were rated at these levels of risk. Table 1 shows the odds ratios of higher RIO levels, compared to the lowest group. The validation results for the 2,117 screening assessments are shown in Table 2. The C-statistic was 0.860 for the derivation sample and 0.853 for the validation sample.
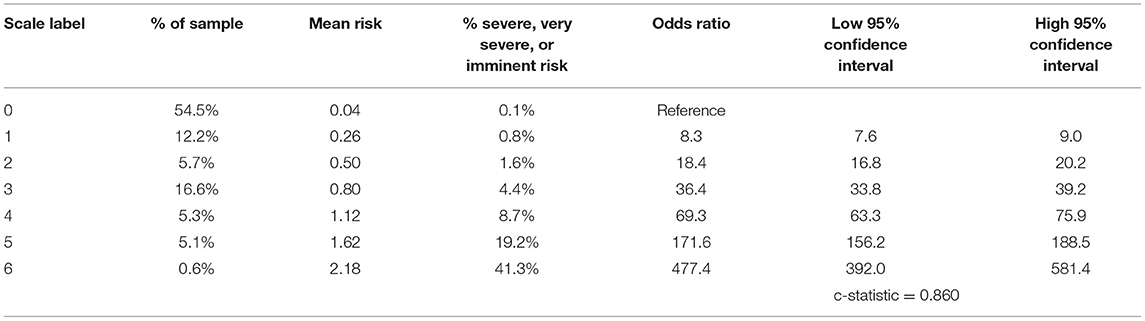
Table 1. Derivation results of Risk of Injury to Others (RIO) algorithm (N = 60,414 screener assessments).
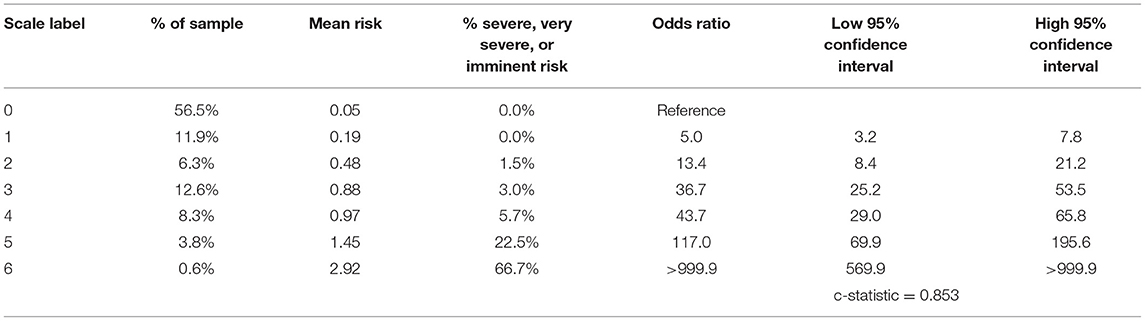
Table 2. Validation results of Risk of Injury to Others (RIO) algorithm (N = 2,117 screener assessments).
The derivation sample by age group and sex are presented in Tables 3 and 4, respectively. As shown in Table 3, younger children scored higher on the RIO algorithm than older children, indicating that they were judged to be at higher risk of harm to others. Specifically, for children 12 and older, only 3.2% were classified as a 5 or 6 on the RIO algorithm compared to children aged 8–11 years (8.1%) and those 7 and under (10.5%). As shown in Table 4, males scored higher on the RIO algorithm than females, with 8.4% of males classified as a 5 or 6 compared to only 3.2% of females.
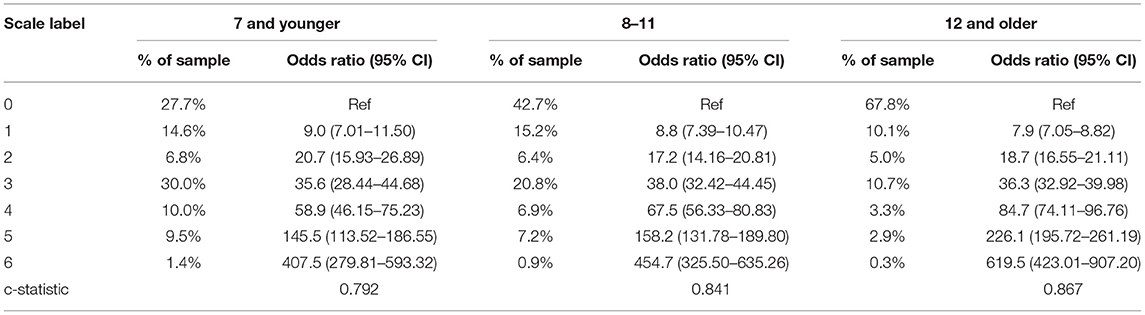
Table 3. Risk of Injury to Others (RIO) algorithm by age, derivation sample (N = 60,414 screener assessments).
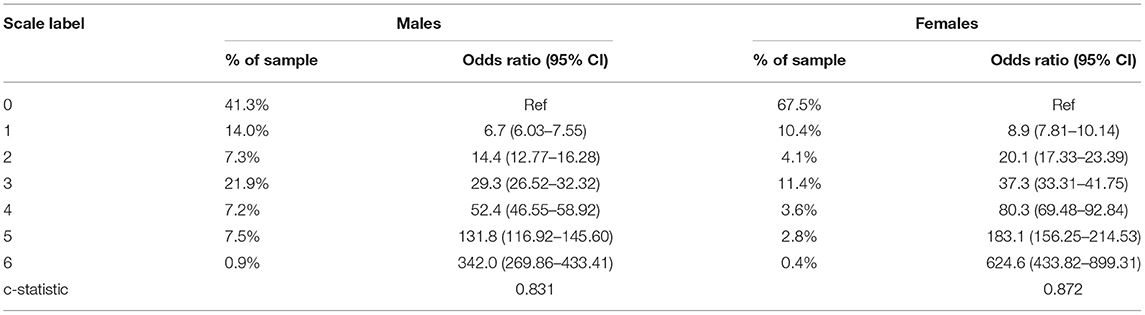
Table 4. Risk of Injury to Others (RIO) algorithm by sex, derivation sample (N = 60,414 screener assessments).
Further collapsing the RIO score into dichotomous groups, various cut-points of the scale were tested for their explanatory power of various levels of actual risk of injury toward others. Such cut-points would be employed to identify cases for specific services or referral related to harm to others. These results are summarised in Table 5. For flagging mild or moderate risk, a RIO cut-point of 2 or greater may be optimal, while for flagging severe risk, a RIO score of 3 or greater was found to perform best.
Using the ChYMH and ChYMH-DD assessment data, which include the nine items necessary to assign the RIO scale, diagnoses associated with higher RIO scores were investigated. As can be seen from Table 6, the most prevalent diagnoses associated with higher risk of harm to others were Disruptive Behaviour, Reactive Attachment, Substance-Related, and Attention Deficit/Hyperactivity disorders. Diagnoses associated with lower RIO scores were Eating, Mood, and Anxiety disorders.
Using the ChYMH, ChYMH-S, and ChYMH-DD assessment data, the predictive validity of the subjective assessor rating of “danger to others” and the RIO score were investigated. As can be seen from Figure 2, the “danger to others” rating is predictive of 5 future violent behaviour items (C-statistics of 0.66–0.72). Further, as shown in Figure 3, RIO itself is highly predictive of these 5 behaviour items (C-statistics of 0.70–0.83). Of note is that 8 of these 10 C-statistics are 0.7 or greater, suggesting a good model (38).
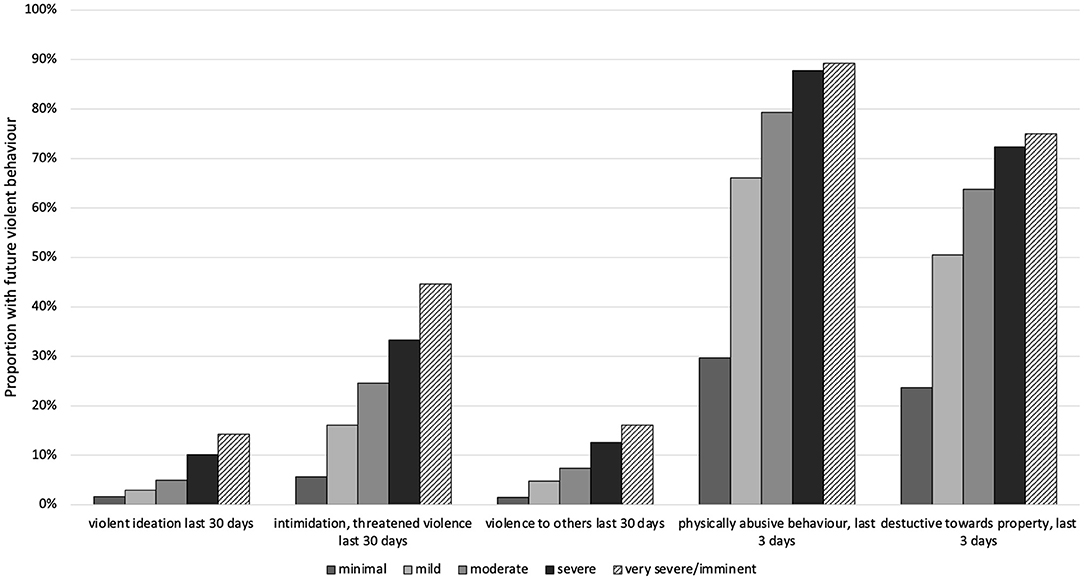
Figure 2. Longitudinal analysis: High risk for future violent behaviour by subjective assessor rating of “danger to others” at baseline (N = 6,608).
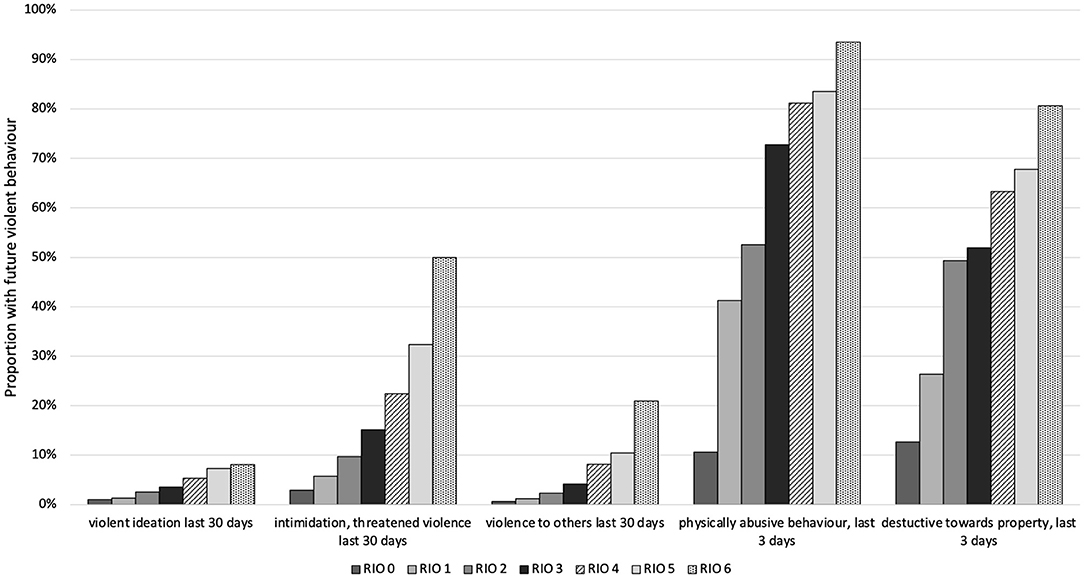
Figure 3. Longitudinal analysis: High risk for future violent behaviour by Risk of Injury to Others (RIO) score at baseline (N = 6,608).
Discussion
A variety of factors predicted high risk of injury to others. Several of the contributors were related to a prior history of abusive behaviours and violent thoughts/actions, certain individual traits and behaviours, and family factors. Young persons who displayed violent ideation, threatened violence, or engaged in violent actions toward others received higher scores on the RIO algorithm. This strong relationship between prior ideas, threats, and acts of violence, and future risk of injury toward others is well-supported by the literature. In a comprehensive multivariate analysis, one of the most salient predictors of harm toward others was prior violent behaviour, among both boys and girls (39). Research has also shown that the frequency of violent threats is positively associated with engagement in violent acts (40). Finally, in a comprehensive review of the literature, Hawkins and colleagues (41) reported that youth who have favourable attitudes toward violence were more likely to commit violent acts in the future.
We found that children and youth who exhibited physically abusive behaviour were at higher risk of injury toward others, and this was, in fact, the first predictor included within the RIO algorithm. This is in line with prior research that has found physical aggression to be the most consistent predictor of future violent offending (2, 42). In addition to physical abuse, verbally abusive behaviour was also associated with increased risk of harm toward others, in our model. This is consistent with extant literature that reported adolescents in the sixth grade who engaged in bullying, which encompassed both physical and verbal abuse (e.g., picking on another kid, slapping, or hitting), were more likely to be perpetrators of dating violence by the eighth grade (43). Children and youth who were more impulsive also received higher scores on the RIO algorithm, which is similar to previous work that has found a strong correlation between impulsivity and aggressive behaviours toward others (41, 44).
Socially inappropriate/disruptive behaviours, as well as destructive behaviours, also significantly contributed to higher scores on the RIO. This finding is in accordance with prior research, which has reported that a range of anti-social behaviours, including under-age smoking, stealing, and destruction of property, are linked to greater risk of violence among males (45, 46). Studies have also found that deficits in social and cognitive ability in childhood are associated with future aggressive behaviour (47, 48). Children who struggle with social and cognitive functioning may not be able to fully comprehend social norms, thereby acting in socially inappropriate and disruptive ways. Interestingly, belief and commitment to a social/moral order is suggested to decrease risk of engaging in violent behaviour (49). Therefore, children and youth who act in ways that would be viewed as contradictory to social norms may be at increased risk of harm toward others, which would support the current study's finding.
The last predictor variable of the RIO algorithm is the family being overwhelmed by the child or youth's condition, which could be due to a number of different reasons, including ineffective coping strategies when dealing with the young person's difficult presentation. Other family stressors may increase the distress level in an expediential manner, further taxing the situation. Research has shown that a number of family factors can increase a child's risk of engaging in injury toward others, such as low parental supervision and monitoring (17). Interestingly, a chaotic family life has been shown to increase risk of youth violence (50). Families may feel stressed because of the chaotic nature of their family environment, thus contributing to the child's increased risk of injuring others, a finding consistent with this study. Research has also found that harsh and inconsistent discipline is associated with aggression in children [e.g., (51)]. It can be postulated that when a family is overwhelmed by the child's situation, caregivers may not feel capable of providing the fair, consistent discipline the child requires for positive development, thereby resulting in a higher likelihood of the young person engaging in harmful behaviours.
Findings also indicated an association between age and scores on the RIO algorithm, whereby younger children were more likely to have a higher RIO score compared to older children. More specifically, younger children were at heightened risk of engaging in behaviours that would injure others compared to their older counterparts, a finding that is consistent with extant literature (52). However, it is important to note that although younger children tend to be more physically aggressive, older children and youth are larger in stature and size; therefore, their aggressive behaviours could lead to more serious injuries in others, despite the fact that it occurs less frequently. This relationship between age and severity of aggressive behaviour is well-documented in the literature, with the period of adolescence and young adulthood being known as a time of heightened risk behaviour, such as engaging in more violent acts. It has been reported that the age of onset for serious, violent offending typically does not occur before the age of 12, but this rate increases drastically from 12 to 16 years of age, doubling between 13 and 14 years old (7, 53). Therefore, it is critical to make the distinction that, although younger children are more likely to be physically aggressive, older children are more likely to engage in more serious, violent acts.
The current study also examined DSM-diagnoses related to the RIO algorithm, and identified disruptive behaviour, reactive attachment, substance-related, and attention deficit hyperactivity among the top diagnoses associated with higher RIO scores. The association between these diagnoses and higher risk of harm to others is well-supported by the literature. For example, one study that examined the most common psychiatric disorders among children and adolescents referred to mental health services for serious aggressive behaviour found that the most common diagnoses behind aggression were oppositional defiant disorder (93.02%), attention deficit hyperactivity disorder (88.37%) and conduct disorder (38.75%) (54). It has also been reported that aggression is a frequently co-occurring condition to reactive attachment disorder (RAD) (55). Several other studies have consistently found aggression to be one of the key risk factors associated with substance-use disorder (56, 57); furthermore, aggression has been found to be significantly related to early substance use initiation among youth (58).
Finally, the current study also investigated the predictive validity of the subjective assessor rating of “danger to others” as well as the RIO score itself. First, the findings showed that the assessors' rating of perceived risk of “danger to others,” subjective as it is, predicts future violent behaviour. More specifically, higher assessor ratings at time point 1 were associated with increased violent ideation, intimidation/threatened violence, violence to others, physically abusive behaviour, and destructive behaviour toward property at time point 2. This supports the use of this measure in the derivation of RIO. It may not be a “gold standard,” but it supports the validity of a measurement that predicts what is intended to be modelled. Second, the findings indicate that the RIO score itself at baseline is associated with increased violence in the future across the same five items previously described. This demonstrates the utility of the RIO algorithm in predicting future aggressive behaviour toward others among children and youth.
Use and Utility of RIO
Based on our results, RIO is an empirically based decision-support tool that may be used to identify young persons who have a higher likelihood of engaging in harmful behaviour toward others. Because it can validly and reliably predict high-risk physically aggressive behaviour, mental health professionals will be able to make more systematic evaluations in determining whether an individual is at heightened risk of committing violent or injurious acts. Ultimately, the algorithm was designed to help facilitate early intervention efforts to provide support for these vulnerable youth in order to decrease the likelihood of future aggression.
Importantly, the use and utility of the RIO algorithm falls in line with that of our previously published RiSsK algorithm (32). For example, the RIO score can similarly be obtained automatically when the ChYMH-S assessment is submitted from the assessor's computer, and these results are also intended to be used along with other information obtained during the screening process.
Subsequent care planning steps are informed by whether the young person's score falls within the lower or upper range. If a score falls within the lower range, the clinical team should discuss further to decide whether, based on all available information, the RIO level seems appropriate. If a score falls within the upper range, the clinical team should consider the individual to be at high risk of injuring others. Clinicians can use the Harm to Others collaborative action plan (CAP) developed by interRAI to assist with their care planning (59, 60). When the young person is at high risk of injuring others, immediate intervention for acute physical aggression is required, followed by debriefing discussions and assessment of the incident. Regardless of moderate or high risk, it is imperative the clinician performs an assessment of harmful behaviour (e.g., precipitating factors, targets, intensity, frequency, and duration of episodes); this information will be used in the selection of subsequent interventions.
Similar to the RiSsK algorithm, the RIO also has broader applications beyond individualised care planning. For example, it can provide high-quality standardised data across large catchment areas, which would enable the identification of risk of injury to others across the system (e.g., examining different jurisdictional patterns); it can also be used to provide justification for specific services and expenditures, as well as for benchmarking purposes (61, 62). For a more detailed discussion of these broader applications, please refer to our previous work (32).
The major advantage of implementing the RIO algorithm would be that young persons with higher levels of risk should be receiving more emergent services and extensive resources (e.g., inpatient services) than those with lower-level risk. Nevertheless, this does not prohibit the likelihood of receiving appropriate services for young persons scoring at the lowest level of risk. Notably, research has shown that early identification and intervention can lead to reduced likelihood of future aggresssive behaviours [e.g., (63, 64)].
While there are a number of strengths in the current study, including internationally-used comprehensive assessment tools and the relatively large sample size, it also has limitations. For example, because all of the children and adolescents assessed were accessing inpatient or outpatient mental health services (i.e., entering the formal system), the results may not be generalizable to a community-based, non-clinical sample. As such, future research could examine whether the present study's findings are consistent when the sample population is from the community.
Additionally, assessors completed the items used to derive the RIO algorithm at the same time the overall risk score was determined utilising the interRAI ChYMH Screener. The algorithm was modelled on this overall risk score and, as a result, utilised concurrent measurement. Notably, while it may not have the ideal characteristics of an independent gold-standard measure on which to derive the RIO score, the validation efforts also utilised other instruments within the suite (e.g., ChYMH, ChYMH-DD) that did not incorporate the overall risk score, providing additional evidence of its utility. This approach was viewed as reasonable given the goals of the algorithm and its use across numerous instruments within the interRAI suite of child and youth assessments. Further concurrent validity measures were also examined within the ChYMH and ChYMH-DD that were not in the interRAI ChYMH Screener at the time the overall risk index was obtained by assessors; this included the items known to use/carry weapons and serious injury to another in the last 90 days. Furthermore, the present study investigated concurrent validity cross-sectionally among first assessments of individuals as well as predictively (using RIO at baseline and its association with these two items at a follow-up assessment between 31 and 182 days). Findings indicated strong concurrent validity.
Conclusion
The adverse consequences of injury toward others are wide-ranging, including psychopathology, substance use, reduced psychosocial functioning, and the most severe and tragic consequence being youth homicide (65–68). In light of the negative sequelae of youth violence, identifying risk factors associated with harmful behaviour is crucial for the development of strategic prevention and intervention programs. This underscores the critical utility of the RIO algorithm, as it provides a psychometrically sound decision-support tool that can assist clinicians in identifying children and adolescents at heightened risk of injuring others, thus facilitating earlier intervention.
Data Availability Statement
The datasets presented in this article are not readily available because of privacy restrictions and regulations. Requests to access the datasets should be directed to the corresponding author.
Ethics Statement
The studies involving human participants were reviewed and approved by Western University's Ethics Review Board (REB #106415).
Author Contributions
SS contributed to the conceptual basis of the study and its methodology. SS and JP developed the analytical strategy. JP performed the statistical analysis. All authors contributed to the formulation of the ideas presented in the study, provided critical feedback to the manuscript, were involved in the writing, and reviewing of the final manuscript.
Funding
Public Health Agency of Canada (#1617-HQ-000050).
Conflict of Interest
The authors declare that the research was conducted in the absence of any commercial or financial relationships that could be construed as a potential conflict of interest.
Publisher's Note
All claims expressed in this article are solely those of the authors and do not necessarily represent those of their affiliated organizations, or those of the publisher, the editors and the reviewers. Any product that may be evaluated in this article, or claim that may be made by its manufacturer, is not guaranteed or endorsed by the publisher.
Acknowledgments
The authors would like to sincerely thank the children, youth, and their families, along with the service providers within the agencies for their participation in the research.
References
1. National Research Council and Institute of Medicine. Juvenile Crime, Juvenile Justice. Washington, DC: The National Academies Press (2001).
2. Broidy LM, Nagin DS, Tremblay RE, Bates JE, Brame B, Dodge KA, et al. Developmental trajectories of childhood disruptive behaviors and adolescent delinquency: a six-site, cross-national study. Dev Psychol. (2003) 39:222–45. doi: 10.1037/0012-1649.39.2.222
3. Miniño A. Mortality among teenagers aged 12-19 years: United states, 1999-2006. NCHS Data Brief. (2010) 37:1–8. doi: 10.1037/e665432010-001
4. Wang H, Naghavi M, Allen C, Barber RM, Bhutta ZA, Carter A, et al. Global, regional, and national life expectancy, all-cause mortality, and cause-specific mortality for 249 causes of death, 1980-2015: a systematic analysis for the global burden of disease study 2015. Lancet. (2016) 388:1459–544. doi: 10.1016/S0140-6736(16)31012-1
5. Butchart A, Mikton C, Dahlberg LL, Krug EG. Global status report on violence prevention 2014. Inj Prev. (2015) 21:213. doi: 10.1136/injuryprev-2015-041640
6. Krug EG, Mercy JA, Dahlberg LL, Zwi AB. The world report on violence and health. Lancet. (2002) 360:1083–8. doi: 10.1016/S0140-6736(02)11133-0
7. Elliott DS. Serious violent offenders: onset, developmental course, and termination – the American society of criminology 1993 presidential address. Criminology. (1994) 32:1–21. doi: 10.1111/j.1745-9125.1994.tb01144.x
8. Hay DF, Castle J, Davies L. Toddlers' use of force against familiar peers: a precursor of serious aggression? Child Dev. (2000) 71:457–67. doi: 10.1111/1467-8624.00157
9. Tremblay RE, Japel C, Pérusse D, McDuff P, Boivin M, Zoccolillo M, et al. The search for the age of 'onset' of physical aggression: Rousseau and bandura revisited. Crim Behav Ment Health. (1999) 9:8–23. doi: 10.1002/cbm.288
10. Brame B, Nagin DS, Tremblay RE. Developmental trajectories of physical aggression from school entry to late adolescence. J Child Psychol Psychiatry. (2001) 42:503–12. doi: 10.1111/1469-7610.00744
11. Hay DF, Waters CS, Perra O, Swift N, Kairis V, Phillips R, et al. Precursors to aggression are evident by 6 months of age. Dev Sci. (2014) 17:471–80. doi: 10.1111/desc.12133
12. Tremblay RE, Nagin DS, Séguin JR, Zoccolillo M, Zelazo PD, Boivin M, et al. Physical aggression during early childhood: trajectories and predictors. Can Child Adolesc Psychiatr Rev. (2005) 14:3–9. doi: 10.1542/peds.114.1.e43
13. Al Odhayani A, Watson WJ, Watson L. Behavioural consequences of child abuse. Can Fam Physician. (2013) 59:831–6.
14. Fagan AA. The relationship between adolescent physical abuse and criminal offending: support for an enduring and generalized cycle of violence. J Fam Violence. (2005) 20:279–90. doi: 10.1007/s10896-005-6604-7
15. Lansford JE, Miller-Johnson S, Berlin LJ, Dodge KA, Bates JE, Pettit GS, et al. Early physical abuse and later violent delinquency: a prospective longitudinal study. Child Maltreat. (2007) 12:233–45. doi: 10.1177/1077559507301841
16. Capaldi DM, Patterson GR. Can violent offenders be distinguished from frequent offenders? Prediction from childhood to adolescence. J Res Crime Delinq. (1996) 33:206–31. doi: 10.1177/0022427896033002003
17. Pardini DA, Waller R, Hawes SW. Familial influences on the development of serious conduct problems and delinquency. In: Morizot J, Kazemian L, editors. The Development of Criminal and Antisocial Behavior: Theory, Research and Practical Applications. Cham: Springer (2015). p. 201–20.
18. Krakowski M. Violence and serotonin: influence of impulse control, affect regulation, and social functioning. J Neuropsychiatry Clin Neurosci. (2003) 15:294–305. doi: 10.1176/jnp.15.3.294
19. Roberton T, Daffern M, Bucks RS. Emotion regulation and aggression. Aggress Violent Behav. (2012) 17:72–82. doi: 10.1016/j.avb.2011.09.006
20. Farrington DP. Early predictors of adolescent aggression and adult violence. Violence Vict. (1989) 4:79–100. doi: 10.1891/0886-6708.4.2.79
21. Farrington DP. Early developmental prevention of juvenile delinquency. Crim Behav Ment Health. (1994) 4:209–27. doi: 10.1002/cbm.1994.4.3.209
22. Miller C. Childhood animal cruelty and interpersonal violence. Clin Psychol Rev. (2001) 21:735–49. doi: 10.1016/S0272-7358(00)00066-0
23. Herrenkohl TI, Maguin E, Hill KG, Hawkins JD, Abbott RD, Catalano RF. Developmental risk factors for youth violence. J Adolesc Health. (2000) 26:176–86. doi: 10.1016/S1054-139X(99)00065-8
24. Jakobsen IS, Fergusson D, Horwood JL. Early conduct problems, school achievement and later crime: findings from a 30-year longitudinal study. N Z J Ed Stud. (2012) 47:123–35. doi: 10.1016/j.drugalcdep.2011.09.023
25. Sussman S, Skara S, Weiner MD, Dent CW. Prediction of violence perpetration among high-risk youth. Am J Health Behav. (2004) 28:134–44. doi: 10.5993/AJHB.28.2.4
26. Farrington DP, Ttofi MM, Coid JW. Development of adolescence-limited, late-onset, and persistent offenders from age 8 to age 48. Aggress Behav. (2009) 35:150–63. doi: 10.1002/ab.20296
27. Thornberry TP, Huizinga D, Loeber R. The prevention of serious delinquency and violence: implications from the program of research on the causes and correlates of delinquency. In: Howell JC, Krisberg B, Hawkins JD, Wilson JJ, editors. Sourcebook on Serious, Violent and Chronic Juvenile Offenders. Thousand Oaks, CA: Sage Publications (1995). p. 213–37.
28. Xie H, Drabick DAG, Chen D. Developmental trajectories of aggression from late childhood through adolescence: similarities and differences across gender. Aggress Behav. (2011) 37:387–404. doi: 10.1002/ab.20404
29. Stattin H, Magnusson D. The role of early aggressive behavior in the frequency, seriousness, and types of later crime. J Consult Clin Psychol. (1989) 57:710–8. doi: 10.1037/0022-006X.57.6.710
30. Allwood MA, Bell DJ. A preliminary examination of emotional and cognitive mediators in the relations between violence exposure and violent behaviors in youth. J Community Psychol. (2008) 36:989–1007. doi: 10.1002/jcop.20277
31. Hirdes JP. Mental Health and Addictions Across Care Settings: Use of interRAI Instruments to Support Recovery. Leuven: interRAI Network of Mental Health (2017).
32. Stewart SL, Celebre A, Hirdes JP, Poss JW. Risk of suicide and self-harm in kids: the development of an algorithm to identify high-risk individuals within the children's mental health system. Child Psychiatry Hum Dev. (2020) 51:913–24. doi: 10.1007/s10578-020-00968-9
33. Stewart SL, Hirdes JP, McKnight M, Curtin-Telegdi N, Perlman CM, MacLeod K, et al. interRAI Child and Youth Mental Health Screener (ChYMH-S) Assessment Form and User's Manual. Version 9.3. Washington, DC: interRAI (2017).
34. Stewart SL, Hirdes JP, Curtin-Telegdi N, Perlman C, MacLeod K, Ninan A, et al. interRAI Child and Youth Mental Health (ChYMH) Assessment Form and User's Manual. Version 9.3. Washington, DC: interRAI (2015).
35. Stewart SL, LaRose L, Gleason K, Nicolson R, McKnight M, Knott W, et al. interRAI Child and Youth Mental Health – Developmental Disabilities (ChYMH- DD) Assessment Form and User's Manual. Version 1. Washington, DC: interRAI (2015).
36. Stewart SL, Babcock SE. interRAI Child and Youth Mental Health-Screener (ChYMH-S): a psychometric evaluation and validation study. Child Psychiatry Hum Dev. (2020) 51:769–80. doi: 10.1007/s10578-020-01003-7
37. De Ville B, Neville P. Decision Trees for Analytics Using SAS Enterprise Miner. Cary NC: SAS Institute (2013).
38. Hosmer DW, Lemeshow S. Applied Logistic Regression. 2nd Ed. New York, NY: John Wiley & Sons (2000).
39. Resnick MD, Ireland M, Borowsky I. Youth violence perpetration: what protects? What predicts? Findings from the national longitudinal study of adolescent health. J Adolesc Health. (2004) 35:424.e1–10. doi: 10.1016/j.jadohealth.2004.01.011
40. Singer MI, Flannery DJ. The relationship between children's threats of violence and violent behaviors. Arch Pediatr Adolesc Med. (2000) 154:785–90. doi: 10.1001/archpedi.154.8.785
41. Hawkins JD, others, a. Predictors of Youth Violence. National Criminal Justice Reference Service [PDF document]. (2000). Available online at: https://www.ncjrs.gov/pdffiles1/ojjdp/179065.pdf (accessed February 5, 2021).
42. Piquero AR, Carriaga ML, Diamond B, Kazemian L, Farrington DP. Stability in aggression revisited. Aggress Violent Behav. (2012) 17:365–72. doi: 10.1016/j.avb.2012.04.001
43. Foshee VA, Reyes HLM, Vivolo-Kantor A, Basile KC, Chang L, Faris R, et al. Bullying as a longitudinal predictor of adolescent dating violence. J Adolesc Health. (2014) 55:439–44. doi: 10.1016/j.jadohealth.2014.03.004
44. Kerig PK, Stellwagen KK. Roles of callous-unemotional traits, narcissism, and machiavellianism in childhood aggression. J Psychopathol Behav Assess. (2010) 32:343–52. doi: 10.1007/s10862-009-9168-7
45. Lewis AS, Oberleitner LM, Morgan PT, Picciotto MR, McKee SA. Association of cigarette smoking with interpersonal and self-directed violence in a large community-based sample. Nicotine Tob Res. (2016) 18:1456–62. doi: 10.1093/ntr/ntv287
46. Mitchell S, Rosa P. Boyhood behaviour problems as precursors of criminality: a fifteen-year follow-up study. J Child Psychol Psychiatry. (1981) 22:19–33. doi: 10.1111/j.1469-7610.1981.tb00528.x
47. Dodge KA, Schwartz D. Social information processing mechanisms in aggressive behavior. In: Stoff DM, Breiling J, Masur J, editors. Handbook of Antisocial Behavior. Toronto, ON: Wiley (1997). p. 171–80.
48. Werner RS, Cassidy KW, Juliano M. The role of social-cognitive abilities in preschoolers' aggressive behaviour. Br J Dev Psychol. (2006) 24:775–99. doi: 10.1348/026151005X78799
49. Catalano RF, Hawkins JD. The social development model: a theory of antisocial behavior. In: Hawkins JD, editor. Cambridge Criminology Series. Delinquency and Crime: Current Theories. New York, NY: Cambridge University Press (1996). p. 149–97.
50. Bushman BJ, Newman K, Calvert SL, Downey G, Dredze M, Gottfredson M, et al. Youth violence: what we know and what we need to know. Am Psychol. (2016) 71:17–39. doi: 10.1037/a0039687
51. Dodge KA, Greenberg MT, Malone PS, the Conduct Problems Prevention Research Group. Testing an idealized dynamic cascade model of the development of serious violence in adolescence. Child Dev. (2008) 79:1907–27. doi: 10.1111/j.1467-8624.2008.01233.x
52. Côté SM, Vaillancourt T, Barker ED, Nagin D, Tremblay RE. The joint development of physical and indirect aggression: predictors of continuity and change during childhood. Dev Psychopathol. (2007) 19:37–55. doi: 10.1017/S0954579407070034
53. Wallinius M, Delfin C, Billstedt E, Nilsson T, Anckarsäter H, Hofvander B. Offenders in emerging adulthood: school maladjustment, childhood adversities, and prediction of aggressive antisocial behaviors. Law Hum Behav. (2016) 40:551–63. doi: 10.1037/lhb0000202
54. Turgay A. Aggression and disruptive behavior disorders in children and adolescents. Expert Rev Neurother. (2004) 4:623–32. doi: 10.1586/14737175.4.4.623
55. Gleason MM, Zamfirescu A, Egger HL, Nelson CA, Fox NA, Zeanah CH. Epidemiology of psychiatric disorders in very young children in a Romanian pediatric setting. Eur Child Adolesc Psychiatry. (2011) 20:527–35. doi: 10.1007/s00787-011-0214-0
56. Allen TJ, Moeller FG, Rhoades HM, Cherek DR. Subjects with a history of drug dependence are more aggressive than subjects with no drug use history. Drug Alcohol Depend. (1997) 46:95–103. doi: 10.1016/S0376-8716(97)00061-6
57. Morie KP, Garavan H, Bell RP, De Sanctis P, Krakowski MI, Foxe JJ. Intact inhibitory control processes in abstinent drug abusers (II): a high-density electrical mapping study in former cocaine and heroin addicts. Neuropharmacology. (2014) 82:151–60. doi: 10.1016/j.neuropharm.2013.02.023
58. Jester JM, Nigg JT, Buu A, Puttler LI, Glass JM, Heitzeg MM, et al. Trajectories of childhood aggression and inattention/hyperactivity: differential effects on substance abuse in adolescence. J Am Acad Child Adolesc Psychiatry. (2008) 47:1158–65. doi: 10.1097/CHI.0b013e3181825a4e
59. Stewart SL, Kam CCS, Marshman ME, Leschied A, Perlman CM, Brown GP, et al. Harm to others. In: Stewart SL, Theall LA, Morris JN, Berg K, Björkgren M, Declercq A, et al. interRAI (CAPs) for Use With the interRAI (ChYMH) Assessment Instrument, Research Version 1 Standard Edition. Washington, DC: interRAI (2015).
60. Stewart SL, Theall LA, Morris JN, Berg K, Björkgren M, Declercq A, et al. interRAI Child and Youth Mental Health Collaborative Action Plans (CAPs) for use with the interRAI Child and Youth Mental Health (ChYMH) Assessment Instrument. Version 9.3, Standard Edition. Washington, DC: interRAI (2015).
61. Hirdes JP, Poss JW, Curtin-Telegdi N. The method for assigning priority levels (MAPLe): a new decision-support system for allocating home care resources. BMC Med. (2008) 6:9. doi: 10.1186/1741-7015-6-9
62. Stewart SL, Poss JW, Thornley E, Hirdes JP. Resource intensity for children and youth: the development of an algorithm to identify high service users in children's mental health. Health Serv Insights. (2019) 12. doi: 10.1177/1178632919827930
63. Golly AM, Stiller B, Walker HM. First step to success: replication and social validation of an early intervention program. J Emot Behav Disord. (1998) 6:243–50.
64. Sprague JR, Walker HM. Early identification and intervention for youth with antisocial and violent behavior. Except Child. (2000) 66:367–79.
65. Huesmann LR, Dubow EF, Boxer P. Continuity of aggression from childhood to early adulthood as a predictor of life outcomes: implications for the adolescent-limited and life-course-persistent models. Aggress Behav. (2009) 35:136–49. doi: 10.1002/ab.20300
66. Fite PJ, Colder CR, Lochman JE, Wells KC. Pathways from proactive and reactive aggression to substance use. Psychol Addict Behav. (2007) 21:355. doi: 10.1037/0893-164X.21.3.355
67. Haltigan JD, Vaillancourt T. Joint trajectories of bullying and peer victimization across elementary and middle school and associations with symptoms of psychopathology. Dev Psychol. (2014) 50:2426–36. doi: 10.1037/a0038030
68. Musu L, Zhang A, Wang K, Zhang J, Oudekerk B. Indictors of School Crime and Safety, 2018. Washington, DC: U.S. Departments of Education and Justice (2019).
Appendix
Keywords: children and youth, mental health, physical aggression, harm to others, interRAI
Citation: Stewart SL, Celebre A, Hirdes JP and Poss JW (2022) Risk of Injury to Others: The Development of an Algorithm to Identify Children and Youth at High-Risk of Aggressive Behaviours. Front. Psychiatry 12:750625. doi: 10.3389/fpsyt.2021.750625
Received: 30 July 2021; Accepted: 18 November 2021;
Published: 03 January 2022.
Edited by:
Philip Lindner, Karolinska Institutet (KI), SwedenReviewed by:
Aspasia Serdari, Democritus University of Thrace, GreecePhilip Baiden, University of Texas at Arlington, United States
Copyright © 2022 Stewart, Celebre, Hirdes and Poss. This is an open-access article distributed under the terms of the Creative Commons Attribution License (CC BY). The use, distribution or reproduction in other forums is permitted, provided the original author(s) and the copyright owner(s) are credited and that the original publication in this journal is cited, in accordance with accepted academic practice. No use, distribution or reproduction is permitted which does not comply with these terms.
*Correspondence: Angela Celebre, YWNlbGVickB1d28uY2E=