- 1Global Health Institute, Xi’an Jiaotong University, Xi’an, China
- 2Department of Social Medicine, School of Public Health, Guangxi Medical University, Nanning, China
- 3Guilin People’s Hospital, Guilin, China
- 4Department of Epidemiology, Universtiy of Florida, Gaineville, FL, United States
- 5Department of Biostatistics and Epidemiology, School of Public Health, Wuhan University, Wuhan, China
- 6People’s Hospital of Guangxi Zhuang Autonomous Region, Nanning, China
- 7Department of Epidemiology, School of Public Health, Cheeloo Medical College, Shandong University, Jinan, China
- 8The Affiliated Brain Hospital of Guangzhou Medical University, Guangzhou, China
Objectives: To identify mechanisms underpinning the complex relationships between influential factors and suicide risk with psychological autopsy data and machine learning method.
Design: A case-control study with suicide deaths selected using two-stage stratified cluster sampling method; and 1:1 age-and-gender matched live controls in the same geographic area.
Setting: Disproportionately high risk of suicide among rural elderly in China.
Participants: A total of 242 subjects died from suicide and 242 matched live controls, 60 years of age and older.
Measurements: Suicide death was determined based on the ICD-10 codes. Influential factors were measured using validated instruments and commonly accepted variables.
Results: Of the total sample, 270 (55.8%) were male with mean age = 74.2 (SD = 8.2) years old. Four CART models were used to select influential factors using the criteria: areas under the curve (AUC) ≥ 0.8, sensitivity ≥ 0.8, and specificity ≥ 0.8. Each model included a lead predictor plus 8–10 hierarchically nested factors. Depression was the first to be selected in Model 1 as the lead predictor; After depression was excluded, quality of life (QOL) was selected in Model 2; After depression and QOL were excluded, social support was selected in Model 3. Finally, after all 3 lead factors were excluded, marital status was selected in Model 4. In addition, CART demonstrated the significance of several influential factors that would not be associated with suicide if the data were analyzed using the conventional logistic regression.
Conclusion: Associations between the key factors and suicide death for Chinese rural elderly are not linear and parallel but hierarchically nested that could not be effectively detected using conventional statistical methods. Findings of this study provide new and compelling evidence supporting tailored suicide prevention interventions at the familial, clinical and community levels.
Introduction
Increasing suicide among senior residents in rural China
Increased rate risk of suicide among seniors has been reported in some developed countries (1–4). The increasing suicide among senior residents in rural China has becoming a significant issue. The unevenly paced development has resulted in growing financial inequality, leading to extra suicide risk for vulnerable subgroups of population (5). Senior residents in rural China represent one of such subgroups. These seniors are vulnerable due to many life-threatening events, including family separation, poor health in general, physical illness and mental health issues in particular (6–9). Economic growth in China has attracted many young and educated farmers from rural areas to urban areas, leaving the elderly with young children at homes (6). Family separation may exert significant pressure on these left-behind seniors, leading to depression and suicide (6). Furthermore, people in old ages often suffer from chronic diseases with poor health status, exacerbating the risk for suicide (5, 8).
In addition to factors related to QOL, family separation, mental health and diseases, old adults who remain in rural homes may experience progressive deterioration in income (6, 8). Despite that most farmer workers in urban areas can find a job, make money and send money back home, not all rural migrants are successful; and for those who are successful, not all of them can have extra money to send home to support their parents and children. Furthermore, it is not uncommon that many farmer workers will not get paid till the end of a year, which also prevents them from sending money back home timely to support their parents and children.
In addition to shortage of income, many old adults experience difficulties in obtaining social support for problem solving, such as access to healthcare, and labor shortage for farming and childcaring during special seasons (6, 8). Furthermore, it is not uncommon for one spouse of a married couple who remains in rural home to deal with unstable marriage and divorce after the other one moved to urban areas to make money. For these old adults, unstable marriage may become the deepest and most significant risk for suicide (6).
The need for new analytics to advance suicide research
Much has been documented regarding influential factors of suicide for old adults in rural China. Typical examples include depression and other mental health issues (6), poor QOL (9, 10), lack of social support (11, 12), lack of education, low income (13, 14), unstable marital status (15, 16) and gender (17, 18). However, when these factors are analyzed together in a multivariate model, many are no longer statistically significant, suggesting the lack of independent effect of individual factors. Taking depression and poor QOL as an example, when analyzed separately, these two factors are significantly associated with suicide in various populations (19–21), including seniors in rural China (22, 23). However, when these two factors are analyzed in one model, QOL is no longer significant (24). This finding does not indicate the lack of influence of QOL but a more complex relation between QOL, depression and suicide that cannot be detected using the conventional methods.
The need for different analytical approaches is further supported by recent system reviews and meta-analyses of suicide studies conducted in different countries and regions in the past 50 + years. Multivariate analytical approaches such as logistic regression, mediation and moderation analysis, and structural equation modeling have been widely used in published studies, but the established models often performed poorly in predicting suicide with estimated AUC (area under the curve) rarely greater than 0.60 and sensitivity and specificity rarely greater than 70% (25, 26). The relationship between multiple risk factors and suicide is much more complex than independent and linear. To extract convincing evidence from data supporting suicide prevention and control, new and more innovative analytical approaches are needed.
Machine learning as an alternative
Machine learning (ML) provides a promising alternative to the conventional multivariate regression approach. One typical ML method is the classification and regression tree (CART) (27–29). Different from the conventional approaches of multivariate linear regression, multinomial logistic regression, multivariate logistic regression, multivariate ordinal logistic regression, ML is data-driving that can disentangle complex relationships between many predictors and an outcome based on the significance of individual predictors. Therefore, ML can also avoid variable mask effect as in multivariate regression in which a proximal variable (i.e., depression) can block the effect of a distal variable (i.e., QOL) on suicide. Additionally, variables detected through decision trees in CART are linked hierarchically, thus providing further information on potentially nested hierarchical relationships among key predictors. Lastly, ML makes it possible to test different risk scenarios by purposefully excluding some key variables. ML algorithms such as CART/decision tree, random forest, and deep learning have already shown its superiority to the conventional approaches in predicting suicidal behaviors (30–32) and death (33, 34) in other populations.
The purpose of this study
In this study, we examined factors related to suicide for old adults in rural China using CART, taking advantages of the psychological autopsy data collected by a case-control project. The goal is to provide new data beyond linear relationships to advance our understanding of suicide and better inform evidence-based precision interventions.
Materials and methods
Research design, participants and data
Data were from a suicide study project targeting rural residents 60 years of age and older. Procedures for sampling and data collection were detailed elsewhere (6). Briefly, three provinces in China were randomly selected based on the gross domestic production (GDP); and 12 counties were randomly selected from the three provinces using the level of economic development. Suicide cases were identified based on death certificates from the County Death Registries using the ICD-10 criteria. Subjects who died by suicide during the study period from February 2014 to September 2015 were included.
For each suicide case, a 1:1 match was used to select living controls. The criteria used to select controls were: living in the same neighborhood, the same gender, and within a similar age range (± 3 years old).
Data were collected using classic psychological autopsy technique (35, 36). Interviewers were faculty members and graduate students from three universities in the sampled provinces that co-hosted the project. All interviewers received a 10-day training on psychological autopsy method. Data for cases were collected from informants using questionnaire. Two informants per subject were recruited, including family members, neighbors, friends, and other important persons of the participants.
Institutional Review Board (IRB)‘s approval for data collection was obtained from the Shandong University, the Central South University and Guangxi Medical University in China; and IRB approval for data analysis was obtained from University of Florida in the US.
Variables and measurement
A total of 15 predictor variables were included for this study based on the suicide literature.
Demographic and socioeconomic factors (6 variables)
Age (in year), gender (male/female), marital status (stable/unstable), educational attainment (less than primary, primary, more than primary), employment (employed/not), and personal income (100 RMB Yuan/year).
Depression
This variable was assessed using the Geriatric Depression Scale (GDS) (37). GDS is a 30-item scale that measures depressive symptoms in the week prior to suicide (for cases) or prior to the interview (for controls). Cronbach alpha was 0.94 from our data, total scores (range, 0–30) were computed such that higher scores indicated more depressive symptoms.
Quality of life
This variable was measured using the Quality of Life Scale (38), a 6-item scale tapping the information regarding physical, mental, financial and social wellbeing. A typical item was “How do you rate your physical health status in the past month?,” varying from 1 (very poor) to 5(excellent). Cronbach alpha = 0.78 from correlation analysis and GFI = 0.97 from confirmative factor analysis. Total scores were computed (range: 6–30) with higher scores indicated better QOL.
Social support
This variable was measured using the Duke Social Support Index (DSSI) (39). DSSI uses 23 items to evaluate social interaction, subjective and instrumental social support. DSSI scores significantly predicted suicide in a previous study (40). The Cronbach alpha = 0.89 in the current study.
Variables for living arrangement
Two variables were: (1) If left behind (yes/no): Subjects were coded as “yes” if all adult children left rural homes for urban areas for at least 10 months during the past year and did not visit home more than twice; otherwise, “no.” (2) Living alone (yes/no): This variable was measured using the question: “With whom you are currently living?” Subjects responded “No one” were coded as “yes”; otherwise, “no.”
Perceived burdens to family
Two variables were: (1) Economic burden (yes/no) was measured using the question: “Have you ever thought that your diseases added an economic burden to your family?” (2) Physical and mental burden (yes/no) was measured using the question: “Have you ever thought that your diseases brought a physical and/or mental burden to your family?” The two questions were measured using the Likert scale varying from 1 (not at all) to 4 (very much). Subjects with response “4” were coded as “yes”; otherwise, “no.”
Health status in general (poor/not poor)
This variable was assessed using the question: “How do you rate your own health in general?” Answer option varied from 1 (very bad) to 5 (very good). Subjects with response of 4 or less were coded as “poor”; otherwise, “not poor.” (2) Having a chronic disease (yes/no): This variable was assessed using the question: “Have you ever been diagnosed with a severe or chronic disease?” with answer options of 1 (yes) and 0 (no).
Family suicide history (yes/no)
This variable was assessed using the question: “Has any member in your family ever attempted suicide or committed suicide?” with answer options of 1 (yes) and 0 (no).
Statistical analysis
Descriptive (mean, standard deviation, proportion, and rate) and bivariate (student t-test and chi-square test) analyses were used to describe the study sample and to compare the difference in variables between the suicide cases and living controls. CART was used for machine learning to detect predictors using a new procedure we invented. The new procedure, termed as the stepwise exclusion CART modeling, started with the CART to construct the first prediction model by including all 15 predictors and from the 15 to detect the first lead influential factor.
The modeling procedure was repeated to detect the second lead factor among the remaining 14 by excluding the first leader factor. The exclusion CART modeling procedure repeated until a CART model could not be further improved according to the criteria. As a conventional rule (27–29), sensitivity (≥ 0.8), specificity (≥ 0.8 and area under the curve (AUC ≥ 0.8) were used as criteria for data-model fit assessment.
With our stepwise exclusion approach, a total of four lead factors were detected using four CART models. PROC HPSPLIT was used for CART modeling (27) and for each model, AUC, importance and rank of selected variables were estimated. In all modeling analyses, the sample was partitioned into 65% for training and 35% for validation (29).
Comparison with logistic regression. The same variables included in a CART model were analyzed using a conditional logistic regression for case-controlled data. Odds ratio (OR) and 95% confidence interval (CI) were estimated. Statistical inference was made at p < 0.05 level (95% CI excluding 1.00).
Data processing and statistical analyses were completed using the commercial software SAS V. 9.14 (SAS Institute, Cary, NC, USA).
Results
Study sample and comparability between suicide cases and living controls
Results in Table 1 indicate that the case and control groups were similar in most demographic and socioeconomic variables except two: marital status and employment. Student t-test indicated significant differences between cases and controls for GDS scores, DSSI scores, and QOL scores (p < 0.01 for all).
Classification and regression tree models and suicide predictors
Table 2 summarizes results of the four CART models with the stepwise exclusion procedure. Estimated AUCs for the training sample varied from 0.96 for Model 1 to 0.82 for Model 4, greater than 0.8, the criterion for this study. Model 1 used 10 of all 15 factors to predict suicide with GDS score (importance = 11.17), DSSI score (importance = 3.22), and QOL score (importance = 2.80) as the three most influential factors. After exclusion of GDS, Model 2 predicted suicide using another 10 factors from the remaining 14, and with QOL score, DSSI score and income as the three most influential factors. Results for all four models are detailed in Table 2.
Four typical binary trees
Figure 1 presents four binary trees corresponding to the four CART models in Table 2. Figure 1A presents the tree from Model 1, in which the total 484 subjects were first divided into three groups with GDS scores = 7.20 and 15.30 as cutoffs. The proportion of suicide cases, from high to low was 84% (OR = 16.80) for those with GDS ≥ 15.30, 29% (OR = 5.80) for those with 15.30 < GDS ≤ 7.20, and 5% (OR = 1.00) for those with GDS < 7.20. The 239 participants with GDS ≥ 15.30 were further divided into two groups with QOL score = 18.12 as the cutoff. The proportion of suicide cases was 88% (OR = 1.91) for those with lower QOL relative to 46% for those with higher QOL. Likewise, the 119 participants with 7.20 ≤ GDS < 15.30 were further divided into two groups by marital status with 42% (OR = 2.21) of suicide cases for those with unstable marriage relative to 19% for those with stable marriage.
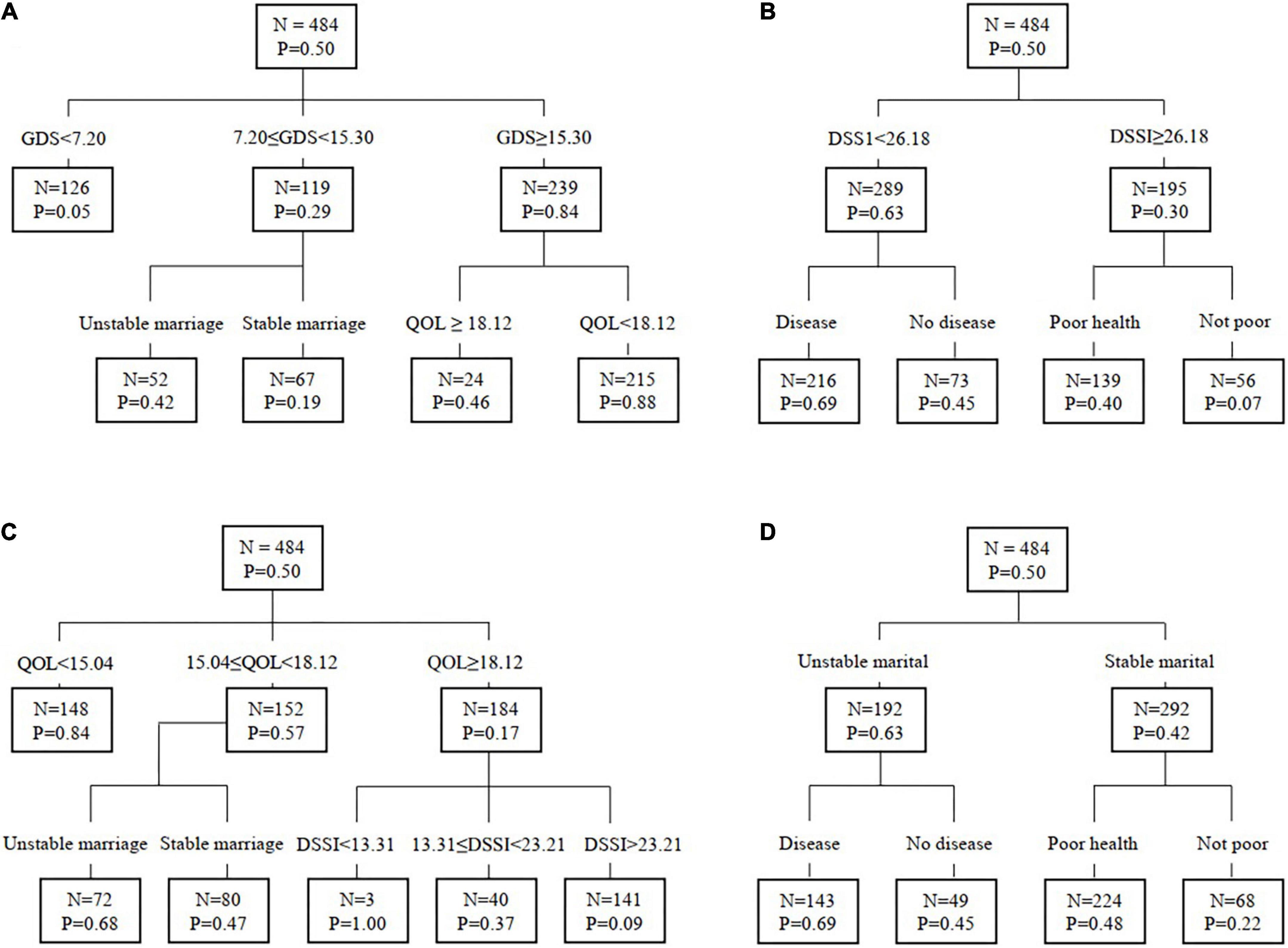
Figure 1. (A–D) Four samples of binary decision trees from CART modeling analysis. GDS, geriatric depression scale score; QOL, quality of life scale score; DSSI, duke social support index score; P, proportion of suicide cases.
Figure 1B shows a typical tree of Model 2 with GDS excluded. The total sample was first divided into three groups with QOL scores of 15.04 and 18.12 as cutoff points. The proportion of suicide cases from high to low was 84% (OR = 4.94) for those with QOL score < 15.04, 57% (OR = 3.35) for those with 15.04 ≤ QOL score < 18.12, and 17% (OR = 1.00) for those with QOL score ≥ 18.12. The 152 participants with 15.04 ≤ QOL score < 18.12 were further divided into two groups by marital status with 68% (OR = 1.45) of suicide cases for those with unstable marriage, and 47% for those with stable marriage.
The remaining 184 subjects with QOL score ≥ 18.12 were divided into three groups with DSSI scores of 13.31 and 23.21 as cutoff points. The proportion of suicide cases from high to low was 100% (OR = 11.11) for those with DSSI score < 13.31, 37% (OR = 4.11) for those with 13.31 ≤ DSSI score < 23.21, and 9% (OR = 1.00) for those with DSSI score ≥ 23.21.
Figure 1C depicts a typical tree of Model 3. In this tree without GDS and QOL, all 484 subjects were first divided into two groups with DSSI = 26.18 as the cutoff; 63% suicide cases were in the lower DSSI-score group and 30% in the higher DSSI-score group (OR = 2.10). The 289 subjects in the lower DSSI group were also divided into two groups by chronic diseases with 69% of suicide cases for those with a chronic disease and 45% for those without (OR = 1.53). The remaining 185 subjects in the higher DSSI group were also divided into two groups by general health status with higher proportion of suicide cases in the group with a poor health status (40 vs. 7%, OR = 5.71).
Figure 1D illustrates a typical tree of Model 4 after exclusion of GDS, QOL and DSSI. In this model, the 484 subjects were first divided into two group by marital status with higher proportion of suicide cases in the group with unstable marriage relative to the stable marriage group (63 vs. 42%, OR = 1.50). Subjects with an unstable marriage (n = 192) were divided again into two groups by chronic diseases with higher proportion of suicide cases in the group with chronic disease than the group without (69 vs. 44%, OR = 1.57). Subjects with a stable marriage (n = 292) were also divided into two groups by general health status with higher proportion of suicide cases in the group with poor general health (48 vs. 22%, OR = 2.18).
Results from conditional logistic regression
Results in Table 3 shows that Logistic Regression Model 1 found two from the 15 factors that were significantly associated with suicide: GDS scores with OR [95% CI] = 1.28 [1.17, 1.41] and employment OR = 3.04 [1.07, 8.63]. Logistic Regression Model 2 detected four significant factors: QOL scores with OR = 0.66 [0.57, 0.75], employment status with OR = 2.09 [1.02, 4.26], marital status with OR = 2.37 [1.05, 5.35], and DSSI score with OR = 0.91 [0.86, 0.96]. Results from Model 3 were similar to those from Model 2 with perceived physical/mental burden to family and having a chronic disease added. Four significant factors detected by Model 4 were marital status, perceived physical/mental burden, and having a chronic disease.
Discussion
In this article, we reported our research on suicide risk factors among old adults in rural China with a case-control design. Psychological autopsy data were analyzed using CART, an ML method with a stepwise exclusion procedure. Capitalizing on the case-control design with no misclassification in the outcome variable, our analysis has successfully demonstrated the first time that several key risk factors reported by different studies such as depression, QOL, social support and marital status do not affect suicide risk independently but through a hierarchically nested mechanism. This study also detected four different suicide risk scenarios each of which consisting of hierarchically nested risk factors. To our knowledge, this is the first ML suicide risk study of the elderly in rural China. Study findings add new data to the literature on suicidology and provide compelling evidence supporting tailored precision interventions. It is worth noting that the CART and logistic regression are two different analytical methods, each with its own advantages and limitations. The CART is used to enhance our analytical capability, not to replace logistic regression and other methods.
Depression as the lead predictor
According to results from CART modeling, depression represents the most proximal risk factor for suicide with high accuracy in predicting suicide. Of all 10 variables, depression ranks the first, 3.47 times stronger than the second one (social support), and 3.99 times stronger than the third (QOL). These findings confirm the importance of these factors in affecting suicide among old adults in rural China (5, 21).
CART modeling provides additional information regarding the influence of depression. First, as GDS scores increased, the OR for suicide increased from 1.00 with low GDS score, to 5.80 with moderate DGS score, and further to 16.80 with high GDS scores, suggesting a dose-response relation. Secondly, suicide risk for highly depressed subjects can be altered by QOL, suggesting an interaction. Likewise, marital status can alter suicide risk for moderately depressed subjects with an unstable marriage. Lastly, the hierarchical relationship from marital status and QOL to depression, further to suicide risk suggests a complex moderated mediation mechanism.
Importance of quality of life
Our stepwise CART modeling indicates that QOL represents a suicide risk above depression, forming a 2 = level risk factor structure. After depression was removed, QOL becomes the lead factor. In this scenario, the second and third most important factors are social support and personal income. In addition, in this setting employment, perceived burdens to family, and gender are included; but chronic disease excluded. Different from depression, QOL and suicide showed a reverse dose-response relationship. Furthermore, for subjects with moderate QOL, suicide risk is higher for those with an unstable marriage; while for subjects with high QOL, risk is higher for those lacking social support.
QOL has been associated with suicide among the elderly in China (9, 41) and other countries (42). The current study is the first to delineate a dose-response relationship between the two. Furthermore, although low QOL is a suicide risk, moderate and high QOL can also increase suicide risk for those with an unstable marriage or lack of social support. Likewise, the hierarchical relationship from marital status to social support, further to QOL suggests a potential moderated mediation mechanism underpinning the suicide risk.
Role of marital status and social support
Our stepwise exclusion CART analysis also found social support and marital status the level-3 influential factor after the removal of depression and QOL. In this hypothetic setting, lack of social support was the lead predictor. Consistent with other studies in China (6, 8, 21) and other countries (21, 43), this study finding confirms the influence of social support in suicidology; what new from our analysis include (1) for subjects with lower social support, their suicide risk can be further increased if also suffering from a chronic disease; (2) for subjects with higher social support, the suicide risk can increase for those with poor general health.
Likewise, in a suicide scenario with no impact of depression, QOL and social support, marital status would be a lead factor. In this condition, subjects with an unstable marriage would be at high suicide risk relative to those with a stable marriage (6, 16, 24). Furthermore, the risk could be exacerbated by chronic diseases for subjects with unstable marriage; and by poor general health for those with stable marriage. The relationship between marital status and suicide among young adults in rural China was reported (44); this study adds new data for the elderlies.
Implications for suicide prevention and control
Suicide prevention is a global challenge (45, 46). Findings of this study provide new and compelling data supporting suicide prevention interventions among the elderly in rural China. At the individual level, psychiatrists and psychologists can develop new and tailored existent therapies, counseling and other cares based on the hierarchically nested influential factors for different suicide scenarios. For example, for seniors with mental health problems, treating depression remains the first-line intervention. However, for those with moderate depression, unstable marriage should be considered in counseling while for those with more severe depression, QOL should be considered. In addition, attention should be paid to chronic disease and general health status for those with unstable marriage and/or lack of social support even they are not depressed and having good QOL.
The influence of QOL, social support and marital status suggests the importance of community participation and public health policies in suicide prevention for rural seniors with no obvious mental health problems. For those who are left behind and/or live alone, with poor health, and/or having a chronic disease, their suicide risk will increase if lack of QOL and social support or live with an unstable marriage. Therefore, emphasizing community participation to enhance QOL and social support would work for risk reduction. Suicide risk can be further reduced by promoting stable marriage and provision of care for poor health and chronic diseases.
Machine learning method to advance suicide research
Big data and ML open a new approach toward data analysis for research (47, 48). In this study, we demonstrate several advantages of CART, an ML method recently gaining popularity in suicide research (27–29). In addition to finding risk factors, this method can predict suicide with high accuracy, provide information about potential underlying relationships among predictors, including mediation and moderated mediation mechanisms. Therefore, application of ML in data analysis will allow researchers to systemize knowledge on risk factors, and generate new data better informing interventions.
In addition, we have explored other advantages of ML by incorporating a stepwise exclusion procedure to assess different hypothetic suicide-risk scenarios. ML is a data-driving approach, adding the stepwise exclusion procedure further strengthens the method for researchers to test new theories.
Limitations and future research
Data used for this study were derived from informants of the subjects, caution is needed in interpreting the findings, despite strengths of the psychological autopsy technique. The interview was held 2–6 months after suicide death, recall bias was thus likely. In addition, sample size can be further increased for subgroup analysis, given the robust result from of our study. Lastly, even though we tested 15 variables, not all factors are included, such as personality trait (49), adverse childhood experience and trauma (50). We will continue our research to examine these other factors in future studies.
Data availability statement
The raw data supporting the conclusions of this article will be made available by the authors, without undue reservation.
Ethics statement
The study was approved by the institutional review boards of Shandong University, Central South University, and Guangxi Medical University. The patients/participants provided their written informed consent to participate in this study.
Author contributions
XC proposed the study, guided and participated in data analysis, and drafted and revised the manuscript. QM participated in conceiving the study, data analysis, and manuscript development. BY participated in data analysis and manuscript development. XB participated in revised the manuscript. ZM, CJ, and LZ conducted the psychological autopsy study and reviewed and commented the manuscript for improvement. All authors contributed to the article and approved the submitted version.
Funding
This work was supported by the American Foundation for Suicide Prevention (grant no. SRG-0-169-12), the Science and Technology Plan Project of Guangdong Province (grant no. 2019B030316001), and the Natural Science Foundation of Guangxi Zhuang Autonomous Region (grant no. 2014GXNSFBA118163).
Acknowledgments
We thank our research collaborators in Shandong, Hunan, and Guangxi provinces of China. We also thank all interviewees for their unique contribution to the study.
Conflict of interest
The authors declare that the research was conducted in the absence of any commercial or financial relationships that could be construed as a potential conflict of interest.
Publisher’s note
All claims expressed in this article are solely those of the authors and do not necessarily represent those of their affiliated organizations, or those of the publisher, the editors and the reviewers. Any product that may be evaluated in this article, or claim that may be made by its manufacturer, is not guaranteed or endorsed by the publisher.
References
1. Stone DM, Simon TR, Fowler KA, Kegler SR, Yuan K, Holland KM, et al. Vital Signs: Trends in State Suicide Rates — United States, 1999–2016 and Circumstances Contributing to Suicide — 27 States, 2015. MMWR Morb Mortal Wkly Rep. (2018) 67:617–24. doi: 10.15585/mmwr.mm6722a1
2. Hiyoshi A, Kondo N, Rostila M. Increasing Income-Based Inequality in Suicide Mortality among Working-Age Women and Men, Sweden, 1990–2007: Is There a Point of Trend Change? J Epidemiol Commun Health. (2018) 72:1009–15. doi: 10.1136/jech-2018-210696
3. Lorant V, Kunst AE, Huisman M, Costa G, Mackenbach J. Socio-Economic Inequalities in Suicide: A European Comparative Study. Br J Psychiatry. (2005) 187:49–54. doi: 10.1192/bjp.187.1.49
4. Beghi M, Butera E, Cerri CG, Cornaggia CM, Lozupone M. Suicidal behaviour in older age: a systematic review of risk factors associated to suicide attempts and completed suicides. Neurosci Biobehav Rev. (2021) 127:193–211. doi: 10.1016/j.neubiorev.2021.04.011
5. Li X, Xiao Z, Xiao S. Suicide among the Elderly in Mainland China. Psychogeriatrics. (2009) 9:62–6. doi: 10.1111/J.1479-8301.2009.00269.X
6. Zhou L, Wang G, Jia C, Ma Z. Being left-behind, mental disorder, and elderly suicide in rural china: a case–control psychological autopsy study. Psychol. Med. (2019) 49:458–64. doi: 10.1017/S003329171800106x
7. He G, Xie J, Zhou J, Zhong Z, Qin C, Ding S. Depression in left-behind elderly in rural china: prevalence and associated factors. Geriatr Gerontol Int. (2016) 16:638–43. doi: 10.1111/Ggi.12518
8. Zhang J, Li Z. The association between depression and suicide when hopelessness is controlled for. Compr Psychiatry. (2013) 54:790–6. doi: 10.1016/j.comppsych.2013.03.004
9. Yan F, Xiang YT, Hou YZ, Ungvari GS, Dixon LB, Chan SSM, et al. Suicide attempt and suicidal ideation and their associations with demographic and clinical correlates and quality of life in chinese schizophrenia patients. Soc Psych Psych Epid. (2013) 48:447–54. doi: 10.1007/S00127-012-0555-0
10. Judit B, Monika M, Jozsef H, Olga HL, Dóra S, Péter V. Suicidal risk, psychopathology, and quality of life in a clinical population of adolescents. Front Psychiatry. (2018) 9:17. doi: 10.3389/fpsyt.2018.00017
11. Zhang YK, Yeager VA, Hou ST. The impact of community-based supports and services on quality of life among the elderly in china: a longitudinal study. J. Appl. Gerontol. (2018) 37:1244–69. doi: 10.1177/0733464816661945
12. Zhang D, Yang Y, Wu ML, Zhao X, Sun YY, Xie H, et al. The moderating effect of social support on the relationship between physical health and suicidal thoughts among chinese rural elderly: a nursing home sample. Int J Ment Health Nurs. (2018) 27:1371–82. doi: 10.1111/inm.12436
13. Wei J, Zhang J, Deng Y, Sun H, Guo P. Suicidal ideation among the chinese elderly and its correlates: a comparison between the rural and urban populations. Int J Environ Res Public Health. (2018) 15:422–31. doi: 10.3390/ijerph15030422
14. Xu H, Qin L, Wang J, Zhou L, Luo D, Hu M, et al. A cross-sectional study on risk factors and their interactions with suicidal ideation among the elderly in rural communities of hunan, China. BMJ Open. (2016) 6:e010914. doi: 10.1136/bmjopen-2015-010914
15. Kyung-Sook W, Sangsoo S, Sangjjin S, Young-Jeon S. Marital status integration and suicide: a meta-analysis and meta-regression. Soc Sci Med. (2018) 197:116–26. doi: 10.1016/j.socscimed.2017.11.053
16. Park SK, Lee CK, Kim H. Suicide mortality and marital status for specific ages, genders, and education levels in south korea: using a virtually individualized dataset from national aggregate data. J Affect Disord. (2018) 237:87–93. doi: 10.1016/j.jad.2018.05.003
17. Lu L, Xu L, Luan X, Sun L, Li J, Qin W, et al. Gender difference in suicidal ideation and related factors among rural elderly: a cross-sectional study in shandong, China. Ann Gen Psychiatry. (2020) 19:2. doi: 10.1186/s12991-019-0256-0
18. Canetto SS, Cleary A. Men, masculinities and suicidal behaviour. Soc Sci Med. (2012) 74:461–5. doi: 10.1016/j.socscimed.2011.11.001
19. Fässberg M, Cheung G, Canetto S, Erlangsen A, Lapierre S, Lindner R, et al. A systematic review of physical illness, functional disability, and suicidal behaviour among older adults. Aging Mental Health. (2016) 20:166–94. doi: 10.1080/13607863.2015.1083945
20. Alves V, Francisco L, Belo F, Leão de-Melo-Neto V, Barros V, Nardi A. Evaluation of the quality of life and risk of suicide. Clinics. (2016) 71:135–9. doi: 10.6061/clinics/2016(03)03
21. Yoshimasu K, Kiyohara C, Miyashita K. Suicidal risk factors and completed suicide: meta-analyses based on psychological autopsy studies. Environ Health Prev Med. (2008) 13:243–56. doi: 10.1007/S12199-008-0037-X
22. Rong J, Chen G, Wang X, Ge Y, Meng N, Xie T, et al. Correlation between Depressive Symptoms and Quality of Life, and Associated Factors for Depressive Symptoms among Rural Elderly in Anhui, China. Clin Interv Aging. (2019) 14:1901–10. doi: 10.2147/CIA.S225141
23. Gong Y, Zhang L, Wang Z, Liang Y. Pathway analysis of risk factors for severe suicidal ideation: a survey in rural China. Canad J Public Health. (2011) 102:472–5. doi: 10.1007/BF03404203
24. Li XY, Phillips MR, Zhang YP, Xu D, Yang GH. Risk factors for suicide in china’s youth: a case-control study. Psychol Med. (2008) 38:397–406. doi: 10.1017/S0033291707001407
25. Franklin J, Ribeiro J, Fox K, Bentley K, Nock M. Risk factors for suicidal thoughts and behaviors: a meta - analysis of 50 years of research. Psychol Bull. (2016) 143:187–232. doi: 10.1037/bul0000084
26. Carter G, Milner A, Mcgill K, Pirkis J, Kapur N, Spittal M. Predicting suicidal behaviours using clinical instruments: systematic review and meta-analysis of positive predictive values for risk scales. Br J Psychiatry. (2017) 210:387–95. doi: 10.1192/bjp.bp.116.182717
27. Lewis Rl. An Introduction to Classification and Regression Tree (Cart) Analysis. In Proceedings of the 2000 Annual Meeting of the Society for Academic Emergency Medicine. San Francisco, CA: (2000). p. 1–14.
28. Fonarow GC, Adams KF Jr., Abraham WT, Yancy CW, Boscardin WJ Adhere Scientific Advisory Committee, Study Group, and Investigators Risk stratification for in-hospital mortality in acutely decompensated heart failure: classification and regression tree analysis. JAMA. (2005) 293:572–80. doi: 10.1001/Jama.293.5.572
29. Breiman L, Friedman JH, Olshen RA, Stone CJ. Classification and Regression Trees. New York, NY: Chapman & Hall (1984).
30. Oh J, Yun K, Hwang J, Chae J. Classification of suicide attempts through a machine learning algorithm based on multiple systemic psychiatric scales. Front Psychiatry. (2017) 8:192. doi: 10.3389/fpsyt.2017.00192
31. Ji S, Pan S, Li X, Cambria E, Long G, Huang Z. Suicidal ideation detection: a review of machine learning methods and applications. IEEE Trans Comput Soc Syst. (2019) 8:214–26.
32. Walsh C, Ribeiro J, Franklin J. Predicting suicide attempts in adolescents with longitudinal clinical data and machine learning. J Child Psychol Psychiatry. (2018) 59:1261–70. doi: 10.1111/Jcpp.12916
33. Choi S, Lee W, Yoon J, Won J, Kim D. Ten-Year prediction of suicide death using cox regression and machine learning in a nationwide retrospective cohort study in South Korea. J Affect Disord. (2018) 231:8–14. doi: 10.1016/j.jad.2018.01.019
34. Linthicum K, Schafer K, Ribeiro J. Machine learning in suicide science: applications and ethics. Behav Sci Law. (2019) 37:214–22. doi: 10.1002/bsl.2392
35. Knoll J. The Psychological Autopsy, Part I: Applications and Methods. J Psychiatr Pract. (2008) 14:393–7. doi: 10.1097/01.pra.0000341894.35877.1b
36. Knoll J. The Psychological Autopsy, Part Ii: Toward a Standardized Protocol. J Psychiatr Pract. (2009) 15:52–9. doi: 10.1097/01.pra.0000344920.36157.95
37. Niu L, Jia C, Ma Z, Wang G, Yu Z, Zhou L. Validating the geriatric depression scale with proxy-based data: a case-control psychological autopsy study in rural China. J Affect Disord. (2018) 241:533–8. doi: 10.1016/j.jad.2018.08.066
38. Phillips M, Yang G, Zhang Y, Wang L, Ji H, Zhou M. Risk factors for suicide in china: a national case-control psychological autopsy study. Lancet. (2002) 360:1728–36. doi: 10.1016/s0140-6736(02)11681-3
39. Koenig H, Westlund R, George L, Hughes D, Blazer D, Hybels C. Abbreviating the Duke Social Support Index for Use in Chronically Ill Elderly Individuals. Psychosomatics. (1993) 34:61–9. doi: 10.1016/s0033-3182(93)71928-3
40. Pan Y, Ma Z, Zhou L, Jia C. Psychometric characteristics of duke social support index among elderly suicide in rural china. Omega (Westport). (2018) 82:105–19. doi: 10.1177/0030222818805356
41. Chen G, Mo Q, Chen X, Yu B, He H, Wang G, et al. Quality of life, hopelessness, impulsivity, and suicide in the rural elderly in china: a moderated mediation analysis of psychological autopsy data. Front Pub Health. (2022) 10:900156. doi: 10.3389/fpubh.2022.900156
42. Minayo M, Cavalcante F. Suicide Attempts among the Elderly: A Review of the Literature (2002/2013). Cien Saude Colet. (2015) 20:1751–62. doi: 10.1590/1413-81232015206.10962014
43. Ajilore OA, Kumar A. Suicide in Late Life. Curr Psychiatry Rep. (2012) 13:234–41. doi: 10.1007/s11920-011-0193-3
45. Word Health Organization. Preventing Suicide: a Community Engagement Toolkit. Geneva: Word Health Organization (2018).
47. Cheng Q, Li T, Kwok C, Zhu T, Yip P. Assessing suicide risk and emotional distress in chinese social media: a text mining and machine learning study. J Med Internet Res. (2017) 19:e243. doi: 10.2196/jmir.7276
48. Ngiam KY, Khor IW. Big data and machine learning algorithms for health-care delivery. Lancet Oncol. (2019) 20:e262–73. doi: 10.1016/S1470-2045(19)30149-4
49. Brezo J, Paris J, Turecki G. Personality traits as correlates of suicidal ideation, suicide attempts, and suicide completions: a systematic review. Acta Psychiatr Scand. (2006) 113:180–206. doi: 10.1111/j.1600-0447.2005.00702.x
Keywords: suicide, rural Chinese, machine learning, depression, quality of life, social support
Citation: Chen X, Mo Q, Yu B, Bai X, Jia C, Zhou L and Ma Z (2022) Hierarchical and nested associations of suicide with marriage, social support, quality of life, and depression among the elderly in rural China: Machine learning of psychological autopsy data. Front. Psychiatry 13:1000026. doi: 10.3389/fpsyt.2022.1000026
Received: 21 July 2022; Accepted: 06 September 2022;
Published: 26 September 2022.
Edited by:
Bao-Liang Zhong, Wuhan Mental Health Center, ChinaReviewed by:
Juntao Tan, Affiliated Banan Hospital of Chongqing Medical University, ChinaVolition Montshiwa, North West University, South Africa
Copyright © 2022 Chen, Mo, Yu, Bai, Jia, Zhou and Ma. This is an open-access article distributed under the terms of the Creative Commons Attribution License (CC BY). The use, distribution or reproduction in other forums is permitted, provided the original author(s) and the copyright owner(s) are credited and that the original publication in this journal is cited, in accordance with accepted academic practice. No use, distribution or reproduction is permitted which does not comply with these terms.
*Correspondence: Liang Zhou, TGlhbmd6aG91X2NzdUB2aXAuMTYzLmNvbQ==; Zhenyu Ma, bWFfemhlbnl1QGd4bXUuZWR1LmNu