- 1School of Electronics and Information, Xi’an Polytechnic University, Xi’an, China
- 2Intelligent Non-Invasive Neuromodulation Technology and Transformation Joint Laboratory, Xidian University, Xi’an, Shaanxi, China
- 3Engineering Research Center of Molecular and Neuro Imaging of the Ministry of Education, School of Life Sciences and Technology, Xidian University, Xi’an, Shaanxi, China
- 4Guangzhou Institute of Technology, Xidian University, Xi’an, Shaanxi, China
- 5South China Research Center for Acupuncture and Moxibustion, Medical College of Acu-Moxi and Rehabilitation, Guangzhou University of Chinese Medicine, Guangzhou, China
Background: Circadian rhythm was involved in the pathogenesis of depression. The detection of circadian genes and white matter (WM) integrity achieved increasing focus for early prediction and diagnosis of major depressive disorder (MDD). This study aimed to explore the effects of PER1 gene polymorphisms (rs7221412), one of the key circadian genes, on the association between depressive level and WM microstructural integrity.
Materials and methods: Diffusion tensor imaging scanning and depression assessment (Beck Depression Inventory, BDI) were performed in 77 healthy college students. Participants also underwent PER1 polymorphism detection and were divided into the AG group and AA group. The effects of PER1 genotypes on the association between the WM characteristics and BDI were analyzed using tract-based spatial statistics method.
Results: Compared with homozygous form of PER1 gene (AA), more individuals with risk allele G of PER1 gene (AG) were in depression state with BDI cutoff of 14 (χ2 = 7.37, uncorrected p = 0.007). At the level of brain imaging, the WM integrity in corpus callosum, internal capsule, corona radiata and fornix was poorer in AG group compared with AA group. Furthermore, significant interaction effects of genotype × BDI on WM characteristics were observed in several emotion-related WM tracts. To be specific, the significant relationships between BDI and WM characteristics in corpus callosum, internal capsule, corona radiata, fornix, external capsule and sagittal stratum were only found in AG group, but not in AA group.
Conclusion: Our findings suggested that the PER1 genotypes and emotion-related WM microstructure may provide more effective measures of depression risk at an early phase.
Introduction
Major depressive disorder (MDD) is one of the most common psychiatric disorders (1–3). According to the World Health Organization, MDD ranks among the top causes of worldwide disease burden and disability (4, 5), with an estimated lifetime prevalence of 20.6% in the general population (6) and with the peak ages of diagnosis from mid-adolescence to mid-40s (7, 8). To reduce that burden, earlier diagnosis, and interventions, as well as identification of those young people who are at high risk of MDD has been prioritized (9–11).
Recently, many lines of evidence in humans or animal models clearly demonstrated a close relationship between MDD and the disturbances of sleep-wake and circadian systems (12–17). The hypothesis that dysregulations of circadian rhythm may play a critical role in the pathophysiology of MDD, is supported by recent findings in the field of molecular biology and genetics of the complex machinery regulating biological clocks (13, 18–21). Therefore, disturbed circadian function have been suggested to be a major risk factor in the development of MDD (14, 18, 22–25). Focused on the people who are vulnerable to the disturbances of circadian rhythm will provide pivotal evidence in the prevention of MDD.
At the gene level, accumulated evidence have implicated that altered circadian gene expression might represent a vulnerability factor for depression (19, 26–28). Based on the chronic mild stress (CMS) animal model of depression, previous studies have indicated that the diurnal oscillation of the expression of several circadian rhythm genes (such as CLOCK, CRY2, PER1, and PER3, etc.) were disturbed and its degree directly was correlated with mood-related behavior (29–31). However, the animal model of depression did not model the full syndrome of depression. Several studies have explored the relationship between depression and circadian rhythm genes in human. Previous studies have found disruptions of the rhythmic expression of PER1, PER2, CRY1, BMAL1, NPAS2, and GSK-3b in MDD, which might influence the susceptibility to recurrence after antidepressant treatment (32). Moreover, a study of postmortem human brains have reported that daily rhythmic patterns of circadian gene expression in the brain were seriously disrupted and/or desynchronized in MDD subjects, further confirming a key connection between circadian gene variation and MDD (19). On the other hand, a number of studies have tested links between circadian gene polymorphisms and the susceptibility to MDD, and several specific single-nucleotide polymorphisms (SNPs) were involved (33, 34). These studies suggested that clock gene alleles, which caused a modification of the circadian clock mechanism, might directly contribute to the onset of mood disorders, or might elicit a misalignment/disruption of the circadian system under the detrimental environment (35).
Due to the genetic architecture of depression is complex and genetic effects are not always saliently expressed at a behavioral level (36), the integration of genomics and neuroimaging techniques (imaging genetics) on MDD might help to identify biomarkers of genetic susceptibility and highlight brain changes mediated by underlying genetic factors (37–41). Recently, using diffusion tensor imaging (DTI) technique and tract-based spatial statistics (TBSS) method, many researches have focused on the interaction effect between risk genes and abnormalities of white matter (WM) integrity or fiber tracts in MDD, such as 5-HTTLPR heterozygotes (42, 43), BDNF-met carriers (44), SLC6A15-A carriers (45), NTRK2 homozygous A (46), FKBP5-T carriers (47), COMT homozygous G (48, 49), VMAT1 Thr136Ile-A carriers (50), THP2 homozygous G (51), RERE homozygous T (52). In addition, significant correlations have been observed between the MDD polygene risk score and fractional anisotropy (FA) values, as well as between SLC6A4, COMT or BDNF promoter region DNA methylation and WM microstructure (53–56). Furthermore, in terms of circadian gene, Bollettini et al. have found that CLOCK C carriers showed widespread increased mean diffusivity (MD) in several WM tracts compared to T homozygotes, and that PER34/4 homozygotes showed significant increased radial diffusivity (RD) and reduced FA in several brain WM tracts compared with PER35/5 homozygotes in depressed bipolar patients, but no significant interaction effect of the two clock genes on WM microstructure (57). Among the clock genes involved in the control system of circadian rhythms, PER1 gene aroused our interest, because its rhythmic expression was disrupted in MDD subjects (19, 30, 32), as well as polymorphisms of rs7221412 was associated with the timing of human behavioral rhythms (mean activity timing was delayed by 67 min in rs7221412GG versus rs7221412AA homozygotes) and time of death (58). However, to our knowledge, the interaction effects between PER1 gene polymorphisms and the WM microstructural abnormalities in MDD patients or susceptible population have not been depicted.
Considering that the dysregulated expression of circadian genes in MDD are mainly selectively altered in emotion-related brain regions (19), we aimed to explore the interaction effects of polymorphisms of PER1 gene (rs7221412) and depression level on the WM microstructural integrity in a group of university students. We hypothesize that the PER1 gene polymorphisms will modulate the relationship between depression level and WM microstructural integrity in emotion-related brain regions.
Materials and methods
Participants
100 healthy college students (all right-handed) were originally recruited from Xidian University in China via advertisements. The subjects would be excluded if they met the following conditions: (1) incomplete information or data (nine subjects failed to acquire the genotype and five subjects did not collect image data); (2) current or chronic illness (excluded four subjects); (3) traces of drugs (including excessive alcohol, nicotine, and caffeine use); (4) personal or family history of neurological or psychiatric disease (3 participants were removed); and (5) sleep disorders (such as sleep apnea, and nocturnal myoclonus, 2 subjects were discarded). At last, 77 subjects (50 men and 27 women; mean age: 20.94 ± 2.09 years, range from 18 to 27 years) were included in this study. Recruitment and screening took place between August 2018 and June 2019 and all data collection took place between March 2019 and January 2020. Each subject was required to finish the venous blood collection, behavioral questionnaires and Magnetic Resonance Imaging (MRI) scanning. In order to make sure subjects are in the same state during the experiment, we performed the behavioral data collection and MRI scanning within a day, and administered the venous blood collection in 3 days. The study protocol and all experimental procedures were reviewed and approved by the Institutional Research Ethics Committee of the Xijing Hospital of the Fourth Military Medical University for research on human subjects and performed in accordance with the Declaration of Helsinki. Written informed consent was obtained from all subjects prior to the study.
PER1 polymorphism identification and genotyping
Two milliliter venous blood was prepared for genomic DNA extraction by using Blood Genomic DNA Kit (TIANGEN BIOTECH CO., LTD., Beijing, China) according to the manufacturer’s instructions. Polymorphism in period homolog 1 (PER1) gene were sequenced. Primers used for the polymerase chain reaction were as follows. PER1 (rs7221412): 5’-ATGTGGGCAATAATACATAAGCA-3’ (forward), 5’-CTATTGACCATTACTTCGTGGA-3’ (reverse). The gene sequencing was performed in the laboratories of Nanjing JINSIRUI Bio-tech Co., Ltd. (Nanjing, China). In our study cohort, the distribution of polymorphism was consistent with the Hardy-Weinberg Equilibrium (χ2 = 0.941, P = 0.332).
Behavioral data acquisition
The Beck Depression Inventory (BDI) (59) has been employed to measure the depressive level (60–64). Two depressive states were identified with the most commonly used cutoff BDI = 14 (BDI < 14, without depression; BDI ≥ 14, with depression) (65). The Munich ChronoType Questionnaire (MCTQ) were employed to quantify the phenotype of circadian rhythm (66). In this study, four index of MCTQ were used, including outdoor time a week, average sleep duration, MSFsc (corrected mid-sleep phase on free days), and Social Jetlag (different between mid-sleep phase on free days and work days) (67, 68). The Pittsburgh Sleep Quality Index (PSQI) were applied for general sleep quality (69). We also applied Insomnia Severity Index (ISI) to evaluate the degree of insomnia and divided subjects into two categories with cutoff 8 (ISI < 8, without insomnia; ISI ≥ 8, with insomnia) (70, 71). All of these questionnaires were collected by trained interviewers.
Image acquisition
The DTI data were acquired on a 3-Tesla MRI system (General Electric Medical Solutions) at MR Research Center of the Xijing Hospital of the Fourth Military Medical University, Xi’an, in China. Subjects’ heads were positioned carefully with restraining foam pads to reduce head motion, and ear plugs were used to reduce the scanner noise discomfort. Prior to the DTI acquisition, high-resolution T1- and T2-weighted images were acquired for each subject by two expert radiologists to exclude the possibility of clinically silent lesions. Diffusion-weighted sequences with single-shot echo planar imaging in alignment with the anterior-posterior commissural plane were acquired with the following parameters: field of view = 240 × 240 mm2, repetition time (TR)/echo time (TE) = 10,000/82.4 ms, matrix = 256 × 256, slice thickness = 2 mm, and 70 continuous axial slices with no gap. The diffusion sensitizing gradients were applied along 64 non-parallel directions (b = 1,000 s/mm2) and one without diffusion weighting (b = 0).
Image data processing and analyses
All DTI analysis was performed using analysis tools from FSL (FMRIB Software Library).1 The DTI data were corrected for eddy distortions and motion artifacts via affine registration on the first no-diffusion weighted volume of each subject by means of the FDT v2.0 (FMRIB’s Diffusion Toolbox) (72), part of FSL. The FA, MD, axial diffusivity (AD) and RD images was created by fitting the diffusion tensor to raw diffusion data after brain extraction using Brain Extraction Tool (73). Then, voxel-wise statistical analyses of the FA data were carried out using the TBSS v1.2 of FSL (74). Briefly, the FA images from all participants were non-linearly warped to the FMRIB58_FA template by FNIRT (75). Next, the mean FA image was created and thinned to create a mean FA skeleton (threshold of 0.2) representing the centers of all tracts common to the group. Each subject’s aligned FA data was then projected back onto this skeleton, and the resulting data were fed into voxel-wise permutation-based cross-subject statistics. The MD, AD and RD were performed the same analysis and projected onto the above mean FA skeleton.
Statistical analysis
For the age, scan time, body mass index (BMI), four index of MCTQ, PSQI, insomnia and BDI score, permutation-test-based Student’s two sample t-tests with 1,00,000 random sampling were carried out between the AG group and AA group. For the gender, insomnia state and depressive state, χ2 tests were performed between the two groups.
Then, voxel-wise DTI statistics analyses were performed for FA using FSL’s permutation-based non-parametric testing (Randomise v2.1, 10,000 permutations) with a general linear model. We applied a ‘‘two groups with continuous covariate interaction’’ model2 to explore the difference of the linear relationship between the FA and BDI values between the two groups. The design matrix included two genotypes (AG = 1 and AA = 0), two genotypes multiply by BDI value interaction (the interaction effects), as well as columns of sex, age, body mass index (BMI) and six circadian/sleep related parameters (outdoor time a week, average sleep duration, MSFsc, Social Jetlag, PSQI and ISI index) as covariates. Previous studies had found altered apparent diffusion coefficient of water during sleep (76), and diurnal fluctuations in white matter microstructural characteristics (77) and in brain volume (78). Therefore, in order to control the effect of time of day on our results, we also added the scan time of MRI as covariates. The MD, AD and RD underwent the similar analysis for the interaction effects genotypes × BDI. Furthermore, we compared the FA between the two PER1 genotypes with the above ten parameters as covariates. Multiple comparisons across voxels were corrected using the TFCE (threshold-free cluster enhancement) method (p < 0.05) (79). The JHU ICBM-DTI-81 white-matter label atlas (80) was used to label significant tracts.
For the convenience of showing results, we extracted and averaged the adjusted BDI, FA, MD and RD among the voxels in each WM tract which showed significant interaction effects of genotypes × BDI. The adjusted BDI, FA, MD and RD were the measures after removing the above ten covariates. Then, we performed Pearson correlation analysis between the adjusted WM characteristics and BDI for the two groups, respectively. Significant correlation was identified when p < 0.05, significant correlation trend was recognized when the p value was between 0.05 and 0.1.
Results
Demographic, behavioral, and genotype characteristics
The demographic characteristics of the participants according to the PER1 genotype were presented in Table 1. The distribution of PER1 genotype in the sample was: A/A 52 (67.53%), A/G 25 (32.47%), G/G 0, consistent with the Hardy-Weinberg Equilibrium (χ2 = 0.941, p = 0.332). Allelic frequencies were A = 83.77%, and G = 16.23%. Age, gender, BMI and scan time did not reach statistical significance between these two groups.
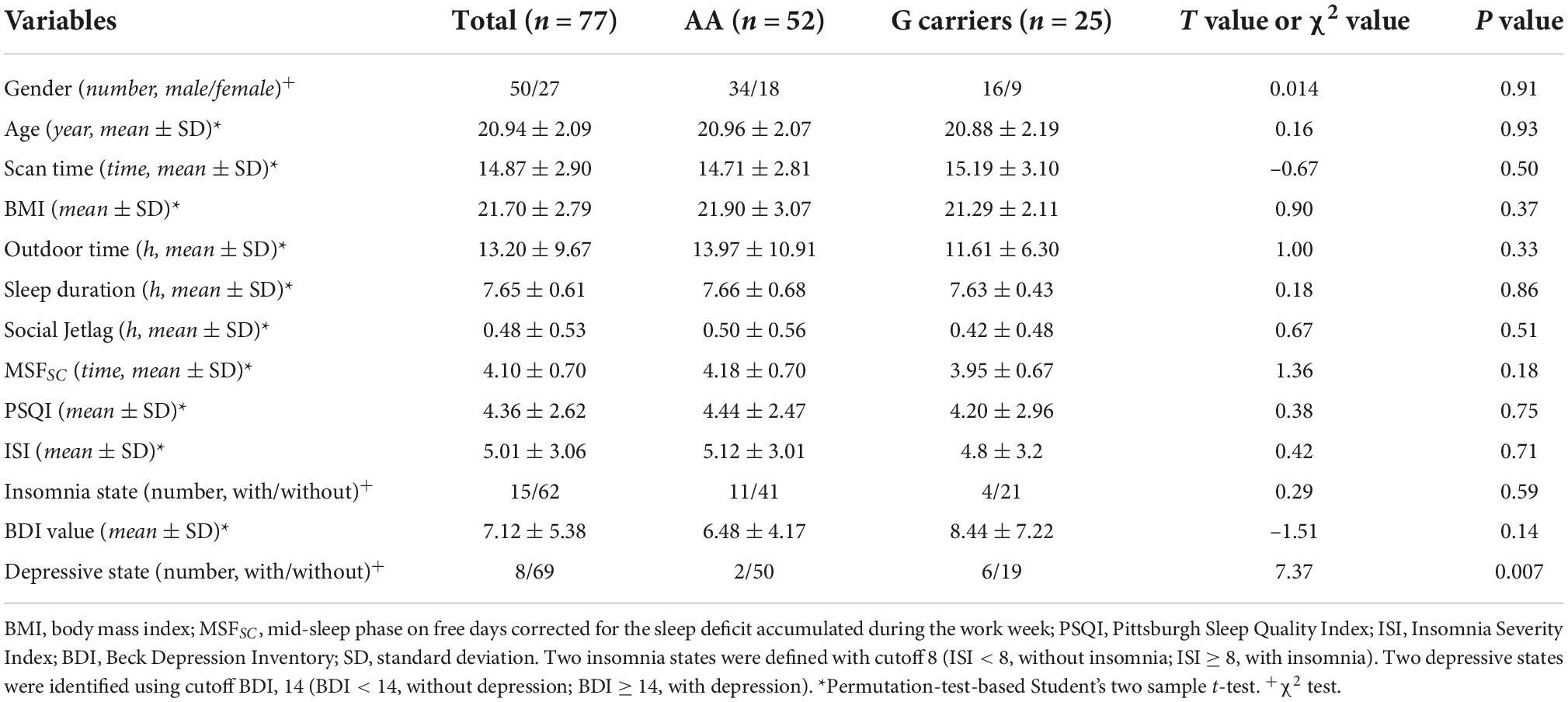
Table 1. Demographic characteristics and circadian rhythms associated phenotypes according to the polymorphism of PER1 gene (rs7221412).
Behavioral data measured by several scales were compared. The feature of circadian rhythm indicated by outdoor time, sleep duration, MSFSC and social jetlag, did not exhibit significant differences between AA and AG groups (p = 0.33, 0.86, 0.18, and 0.51, respectively, Table 1). Furthermore, the general sleep quality indicated by the score of PSQI, insomnia evaluated by ISI, and BDI values in the AA group did not markedly different from that in the AG group (p = 0.75, 0.71, and 0.14, respectively, Table 1).
11 subjects (11/52 = 21.15%) were in the insomnia state in the AG group, and 4 subjects (4/25 = 16%) in the AA group with the ISI threshold value of 8. The insomnia state did not show significant different between these two groups (p = 0.59). However, according to the BDI value with cutoff of 14, 6 subjects (6/25 = 24%) were in the depressive state in the AG group (25/77 ≈ 1/3), and 2 subjects (2/52 = 3.85%) in the AA group (52/77 ≈ 2/3). The AG group exhibited higher proportion of the with-depressive state than that of the AA group (χ2 = 7.37, p = 0.007) based on the χ2 test. In other words, 24% (6/25) subjects with the PER1 heterozygote were in depression state among the 1/3 population and 6/8 of people with depression were the AG genotype. However, only 4% (2/52) of subjects were in depression state among the PER1 A homozygote population and 2/8 of people with depression were in the AA group.
The alterations of fractional anisotropy between PER1 genotypes
Compared to AA homozygotes group, the AG group showed decreased FA in several WM tracts with TFCE correction (p < 0.05), including body of corpus callosum (CC), splenium of CC, left anterior limb of internal capsule (ALIC), left retrolenticular part of internal capsule (RLIC), right superior corona radiata (SCR), left posterior corona radiata (PCR), and left fornix(cres)/strial terminalis (Table 2 and Figure 1).
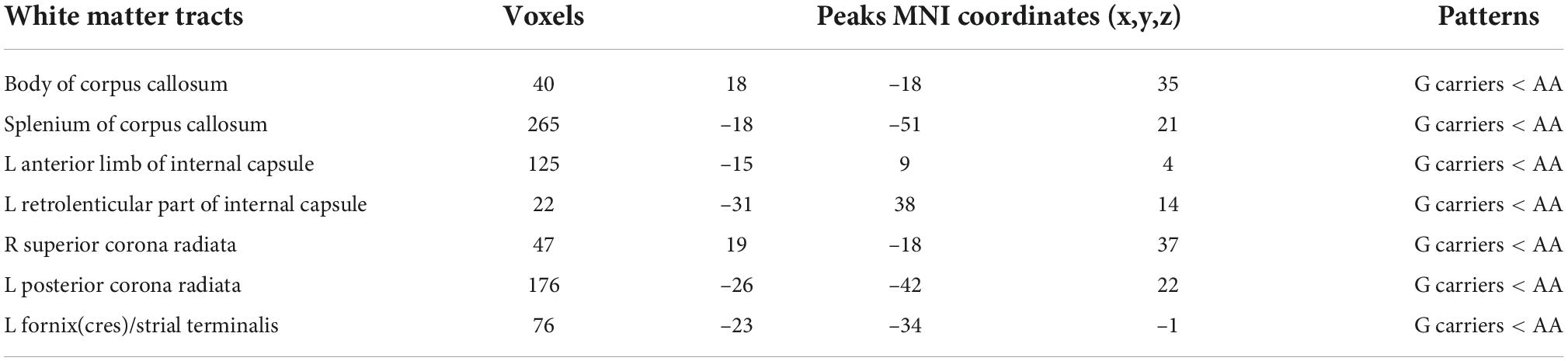
Table 2. White matter regions where Per1 Gene (rs7221412) variants between A/A and G carriers significantly differ in fractional anisotropy.
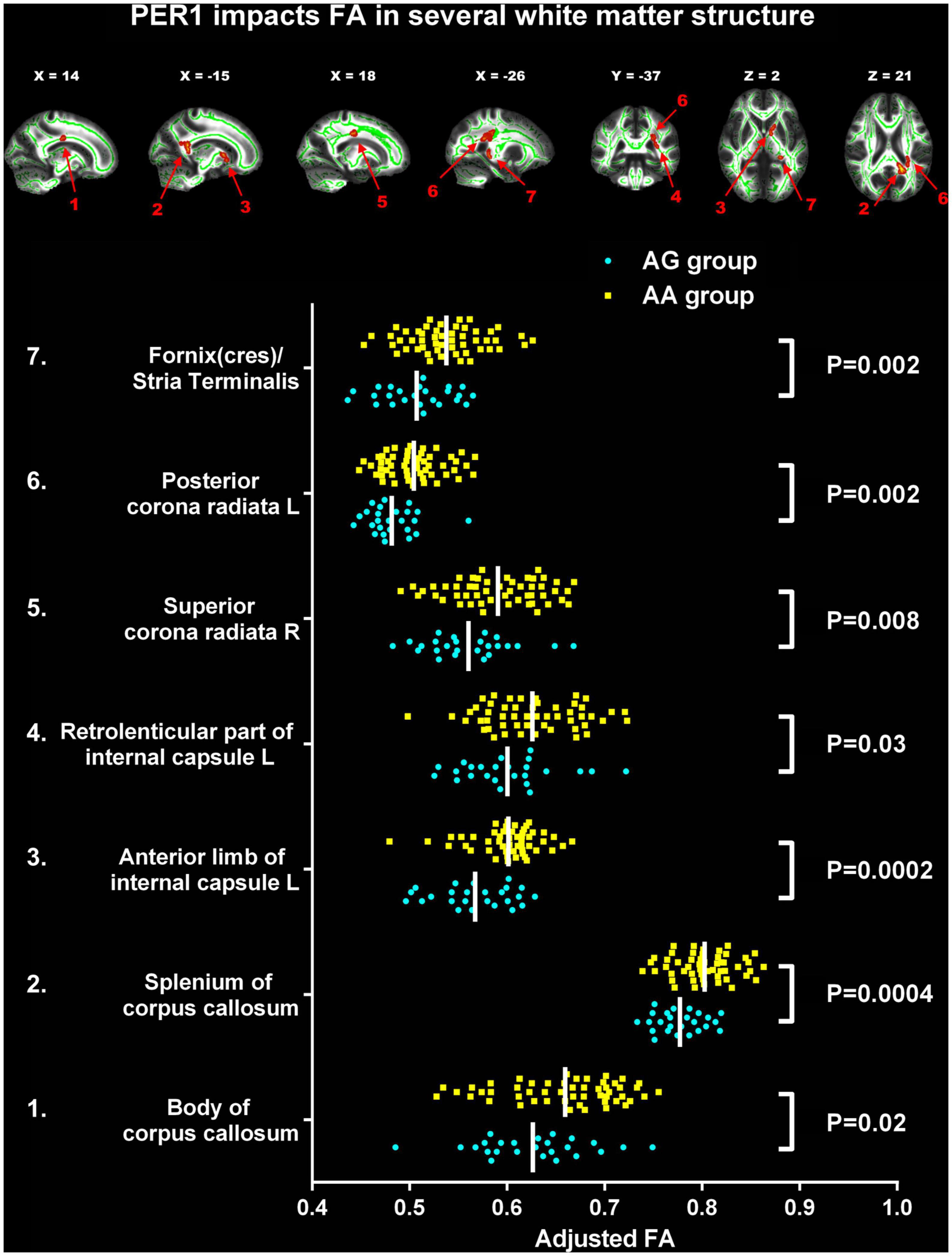
Figure 1. Significant changes of fractional anisotropy (FA) between two PER1 genotypes. The significant difference was observed in the following seven white matter tracts between G carrier group and AA group: 1. Body of corpus callosum (CC); 2. Splenium of CC; 3. The left anterior limb of internal capsule; 4. The left retrolenticular part of internal capsule; 5. The right superior corona radiata; 6. The left posterior corona radiata; 7. Left fornix(cres)/strial terminalis. FA, fractional anisotropy; L, left; R, right. The yellow box represents AA group; the blue dot represents the AG group.
The interaction effect of PER1 genotype on the association between white matter structure and Beck Depression Inventory values
The associations between BDI values and FA, AD, MD and RD were explored in the two PER1 genotype groups and the significant interaction effects between genotype and BDI were present in Table 3. Significant genotype × BDI interaction effects on FA were observed in the genu and body of CC, left anterior corona radiata (ACR), and left SCR. In detail, significant negative correlation was found between the FA in genu of CC and the BDI in AG group (R2 = 0.21, p = 0.02), however, this correlation was not found in AA group (R2 = 0.01, p = 0.44). Similar results were found in body of CC. The FA in the left ACR was not related with BDI in AG group (R2 = 0.07, p = 0.20), but showed positive correlated trend in AA group (R2 = 0.07, p = 0.07). Conversely, negative correlated trend between the FA in left SCR and BDI was found in AG group (R2 = 0.14, p = 0.07), no relationship between them in AA group (R2 = 0.04, p = 0.15, Figure 2).
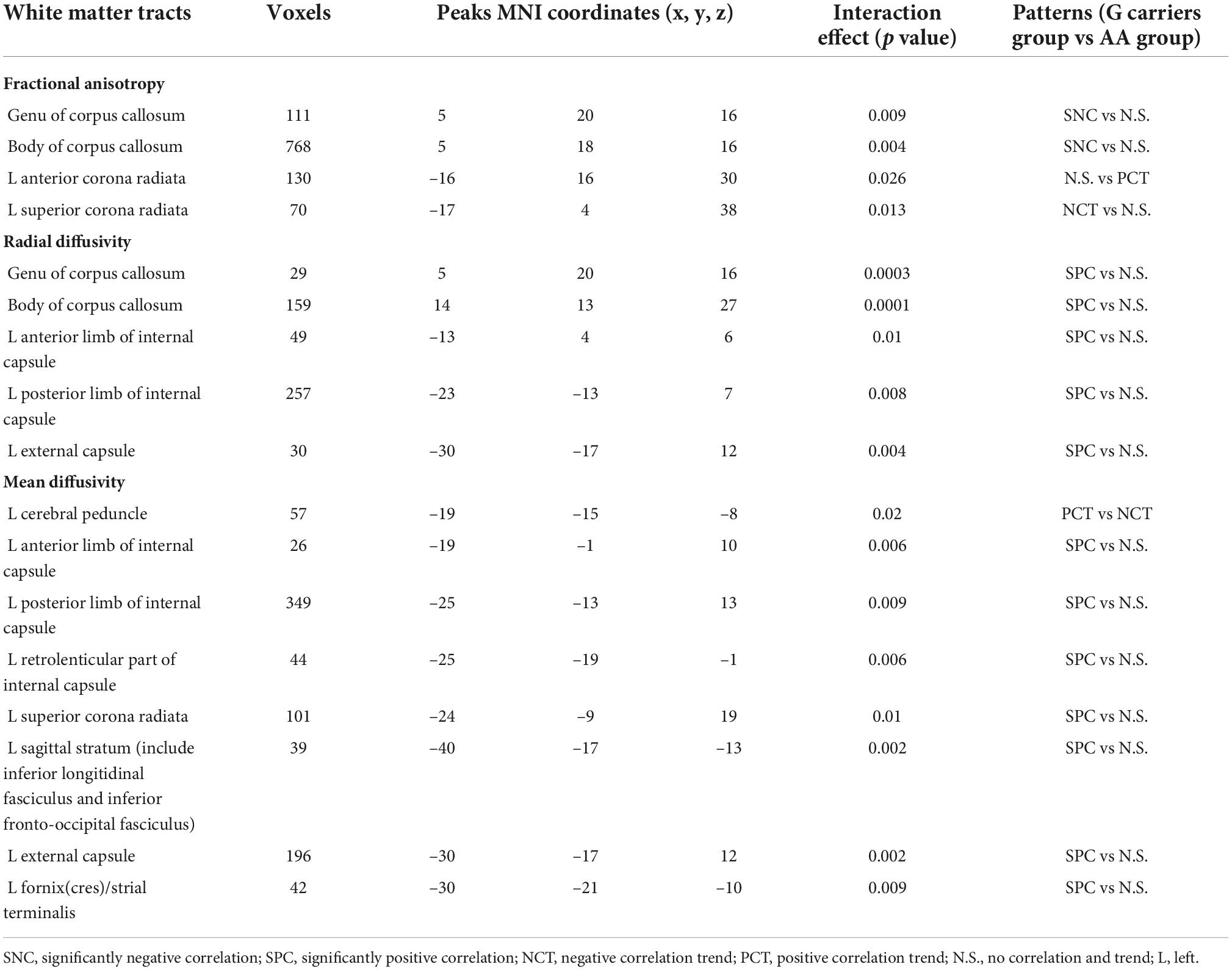
Table 3. Significant interaction effects between Per1 gene variants and BDI values in white matter microstructure.
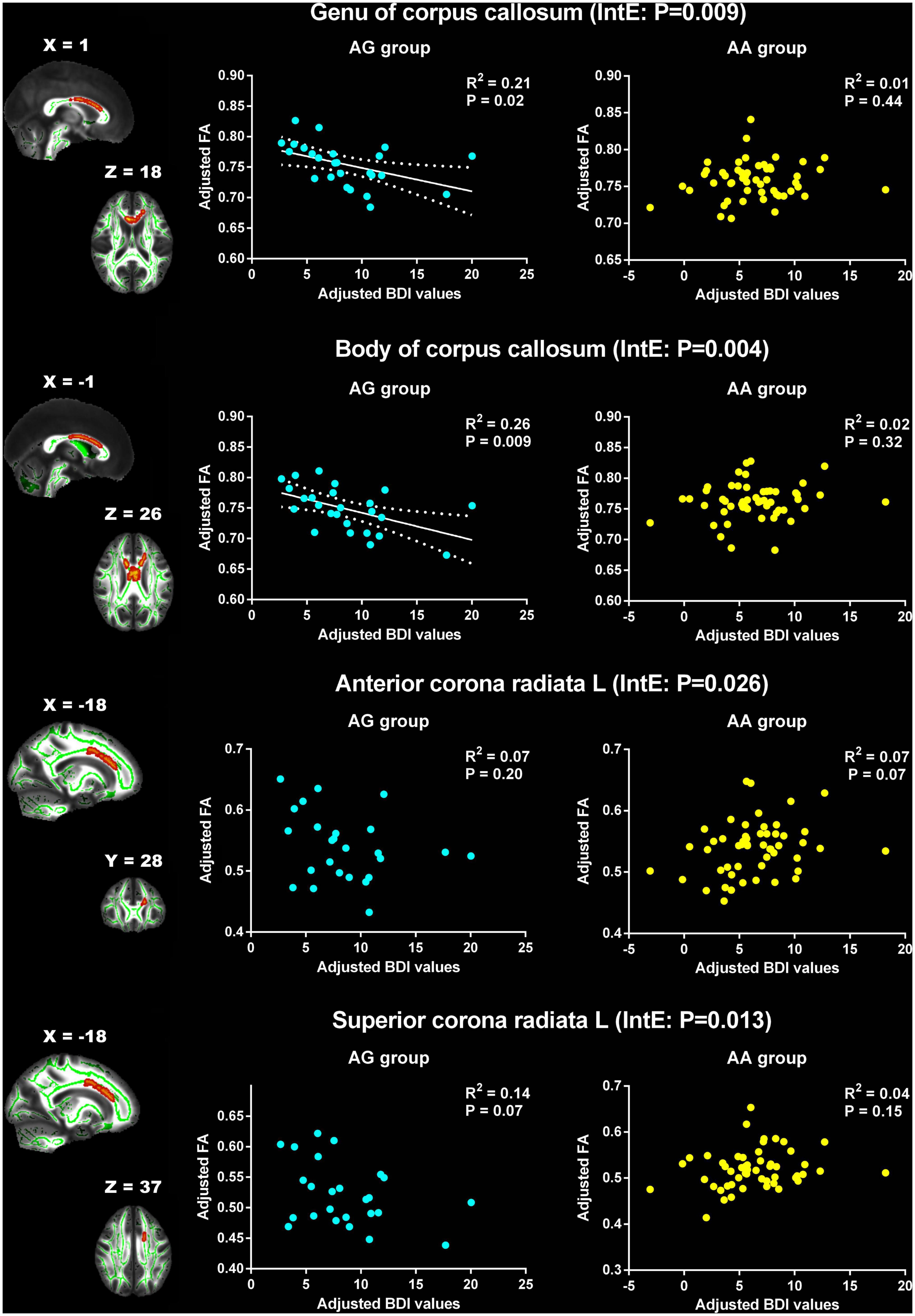
Figure 2. Significant effects of PER1 genotypes on the associations between BDI values and FA. The significant interaction effects of genotypes × BDI were observed in the white matter tracts of genu of corpus callosum, body of corpus callosum, the left anterior corona radiata and the left superior corona radiata. The adjusted BDI and FA were the measures after removing the ten covariates. For the convenience of showing interaction effect, we averaged the adjusted BDI and FA among the voxels in the above WM tracts, and performed the correlation analysis between the adjusted BDI value and FA of WM tracts in each group. BDI the Beck Depression Inventory; FA fractional anisotropy; L left. The blue dot represents the AG group; the yellow dot represents the AA group. InE interaction effects. The white solid line represents the linear regression of the correlation.
For RD, the significant interaction effects were observed in genu and body of CC, left ALIC, left posterior limb of internal capsule (PLIC) and left external capsule (Table 3). The RD of these WM tracts showed significant positive correlation with BDI in AG group, but no association in AA group (Figure 3).
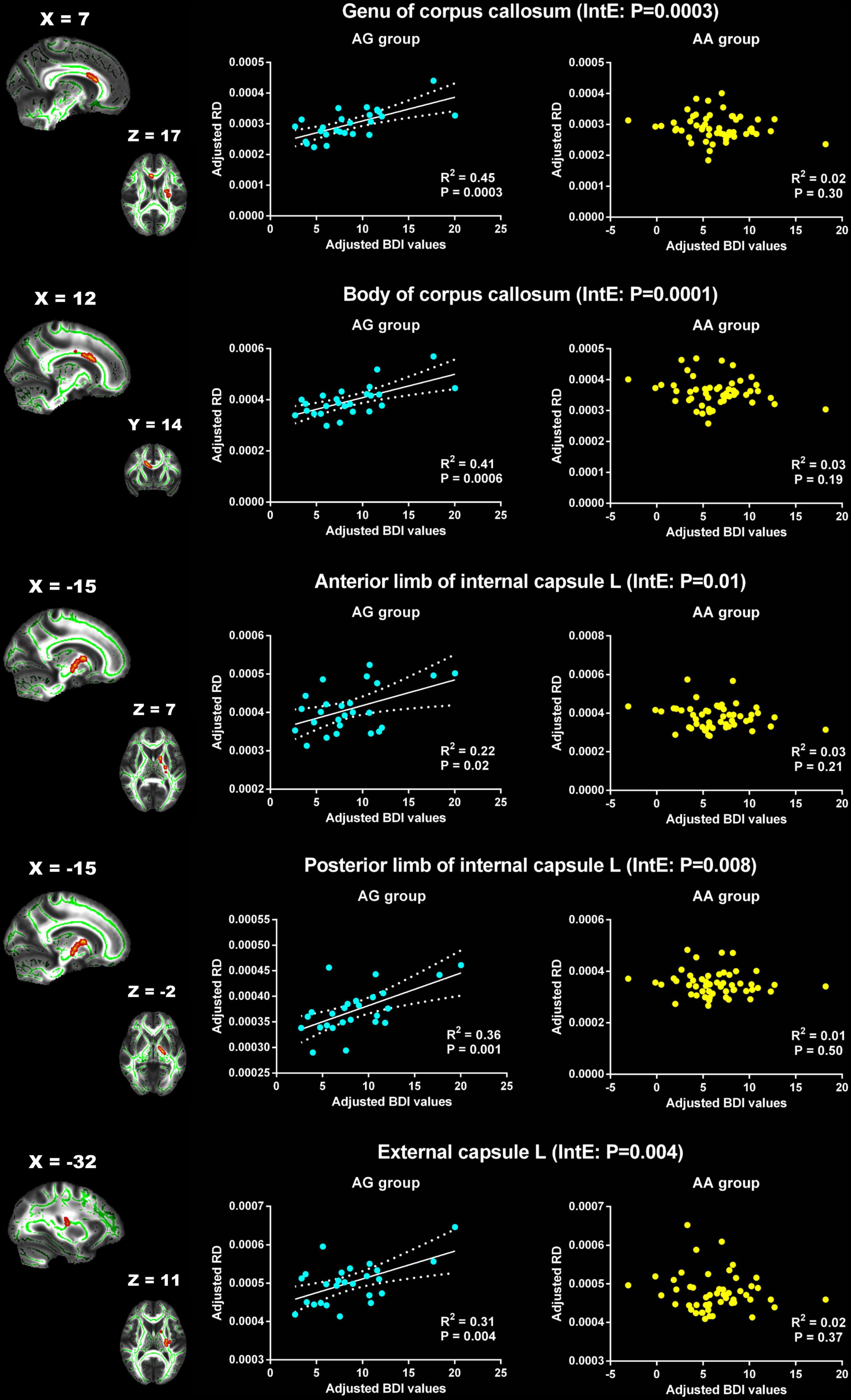
Figure 3. Significant effects of PER1 genotypes on the associations between BDI values and RD. The significant interaction effects of genotypes × BDI were observed in the white matter tracts of genu of corpus callosum, body of corpus callosum, the left anterior limb of internal capsule, the left posterior limb of internal capsule and the left external capsule. The adjusted BDI and RD were the measures after removing the ten covariates. For the convenience of showing interaction effect, we averaged the adjusted BDI and RD among the voxels in the above WM tracts, and performed the correlation analysis between the adjusted BDI value and RD of WM tracts in each group. BDI, the Beck Depression Inventory; RD, radial diffusivity; L, left. The blue dot represents the AG group; the yellow dot represents the AA group. InE, interaction effects. The white solid line represents the linear regression of the correlation.
For MD, the significant interaction effects were found in cerebral peduncle, ALIC, PLIC, RLIC, SCR, sagittal stratum, external capsule and fornix(cres)/strial terminalis on the left cerebral hemisphere (Table 3). The MD in the left cerebral peduncle exhibited positive correlation trend with BDI in AG group (R2 = 0.11, p = 0.10), but showed negative correlation trend in AA group (R2 = 0.05, p = 0.10). The changes of the relationship between BDI and the MD of the remaining WM tracts from the AG group to the AA group were similar. In the AG group, the BDI was significant positive correlated with MD. However, in the AA group, no significant relationship was found between them (Figure 4). However, for AD, no significant interaction effects were found.
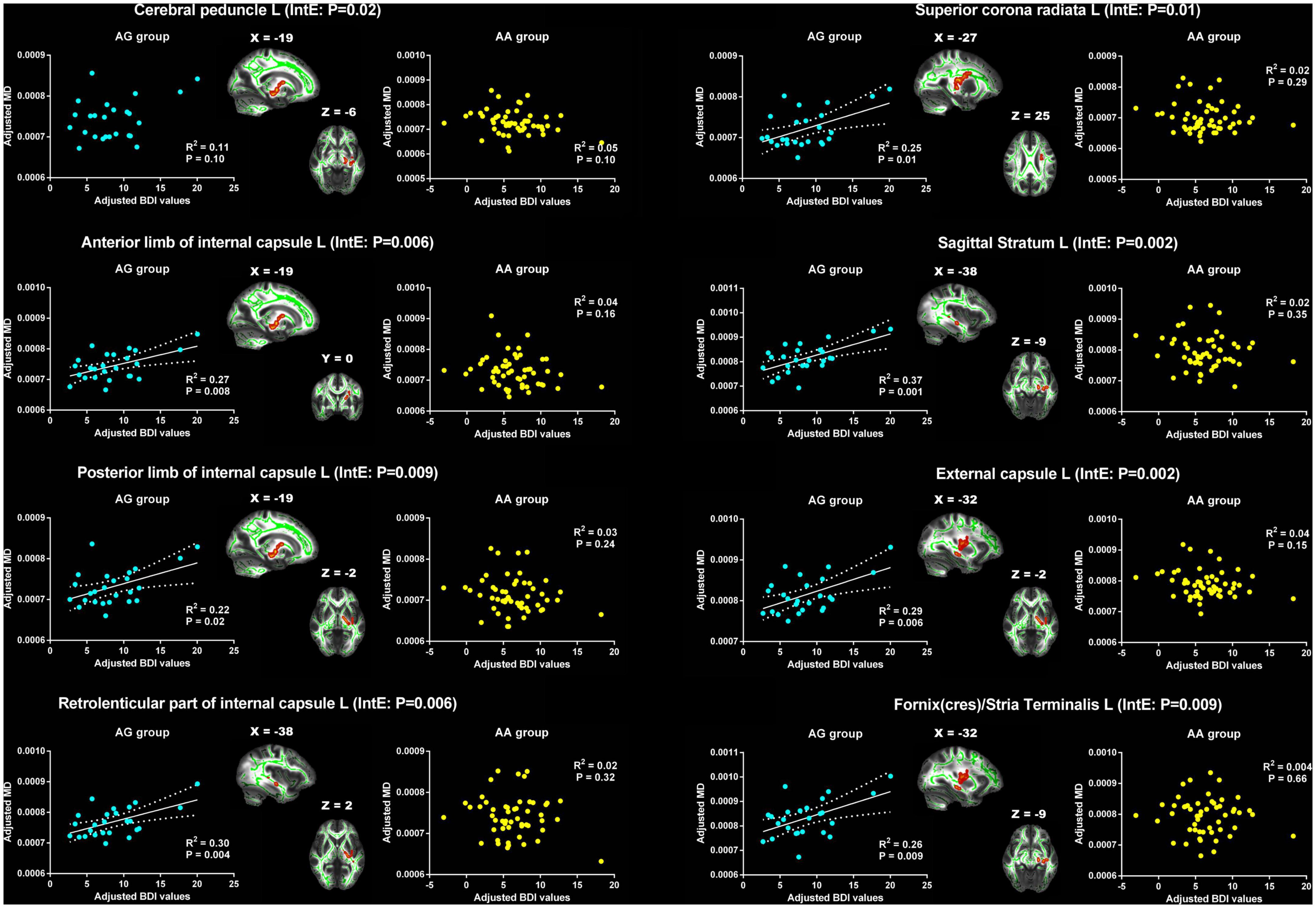
Figure 4. Significant effects of PER1 genotypes on the associations between BDI values and MD. The significant interaction effects of genotypes × BDI were observed in the white matter tracts of cerebral peduncle, anterior limb, posterior limb and retrolenticular part of internal capsule, superior corona radiata, sagittal stratum, external capsule and fornix/stria terminalis on the left cerebral hemisphere. The adjusted BDI and MD were the measures after removing the ten covariates. For the convenience of showing interaction effect, we averaged the adjusted BDI and MD among the voxels in the above WM tracts, and performed the correlation analysis between the adjusted BDI value and MD of WM tracts in each group. BDI, Beck Depression Inventory; MD, mean diffusivity; L, left. The blue dot represents the AG group; the yellow dot represents the AA group. InE, interaction effects. The white solid line represents the linear regression of the correlation.
Discussion
In the present study, we investigated the effects of PER1 (rs7221412) gene polymorphisms on the associations between WM integrity and depression level (measured by BDI) using TBSS analysis. We found that more subjects with PER1 heterozygotes were in depression state (BDI ≥ 14) than AA group, and showed poorer WM integrity in CC, internal capsule, corona radiata and fornix. Furthermore, significant relationships were found between BDI and WM microstructure characteristics in the above WM tracts, external capsule and sagittal stratum in AG group, but not in AA group. These results suggested that the poorer WM integrity in several WM tracts may be associated with the increased depression risk especially in subjects carrying variants of PER1 gene.
In our present study, no significant difference was found in circadian rhythm, sleep quality and insomnia between AG and AA groups. Lim et al. have explored the associations between polymorphisms of PER1 and the timing of behavioral rhythms measured by actigraphy in 537 Europeans, and found that mean activity timing was delayed by 67 min in GG group versus AA group (58). 184 subjects (34%) were AA, 263 subjects (49%) were AG and 90 subjects (17%) were GG among the 537 Europeans, which suggested that the distribution of PER1 was AA:AG:GG ≈ 2:3:1 in Europeans. However, we found that 67.53% were PER1 homozygous A and 32.47% were PER1 heterozygotes among 77 subjects, but no subjects were the PER1 homozygous variant G, which suggested that the distribution of PER1 was AA:AG:GG ≈ 2:1:0 in Chinese Han population. The lower allelic frequencies of G may be one of reasons why there were no significant changes of circadian rhythm/sleep-related behavioral data between AA and AG group. Furthermore, previous studies have found that several gene polymorphism frequency varies depending on both region and ethnicity in healthy and clinical diseases populations (81–84). In addition, the genotype distribution of PER1 in the present study was identified in a small sample of 77. Therefore, further studies could explore the effect of PER1 polymorphism on these behavioral data and verify these inconsistent results whether were due to ethnicity in a large population.
One interesting observation in our present study was that the AG group exhibited higher proportion of depression state with BDI cutoff of 14 than that of the AA group. These results indicated that subjects with PER1 heterozygote showed higher depression risk and more depression symptom compared with other persons. Previous research have found that several gene variations were associated with depression vulnerability, such as PER2 (34), TOMM40 rs2075650 SNP (85), 5-HTTLPR/BDNF Val66Met (86–88), CRHR1 (89) and COMT (90). Our findings were the complement for the association of the gene polymorphism with depression risk. Furthermore, Li et al. have found blunted diurnal rhythms in the expression of PER1 in MDD patients and these disruptions persisted 8 weeks after treatment (32). Therefore, combined our results, these findings suggested that PER1 gene may play a role in depression risk. Further studies were needed to verify our results with large sample.
Another interesting observation in our present study was that besides the higher depression proportion, the AG group exhibited poorer WM microstructure integrity than the AA group, mainly in CC, internal capsule, corona radiata and fornix. These WM tracts have been reported to have decreased FA in MDD (91–94). Vulser et al. have investigated the association of subthreshold depression with WM microstructure alterations in adolescents and found that decreased FA in the anterior body and genu of CC in adolescents with subthreshold depression (95). Our findings were consistent with these researches and suggested that subjects with PER1-G heterozygote with lower FA may probably develop to the subthreshold depression or MDD. In order to explore the effect of PER1 on the relationship between WM characteristics and depression level, we analyzed the interaction effects of genotype × BDI on WM characteristics. Significant correlations were found between BDI and WM characteristics in CC, internal capsule, corona radiata, fornix, external capsule and sagittal stratum in AG group, but not in AA group. The CC plays an important role in the inter-hemisphere information communication, and is critical for emotional regulation and associated with cognitive functioning (96). The corona radiata is part of the limbic-thalamo-cortical circuitry and is composed of ascending and descending fibers that relay information to and from the cerebral cortex. Several researches have indicated that the corona radiata is implicated in emotional and executive processing, major affected functions in MDD (97, 98). Other WM tracts also have been reported involving the mood and cognitive function (99–102). These results suggested that PER1-G heterozygote, 1/3 of Chinese college students, may be more prone to depression if they exhibited lower FA and higher RD and MD in emotion-related WM tracts. However, in AA group, we did not find these relationships. Therefore, PER1 gene polymorphisms and WM microstructure may be promising indictors for early identification of depression risk.
Emerging studies have showed that more than 90% of depressed patients reported disruptions in sleep including insomnia and early morning awakening. These patients tend to have more severe forms of MDD and may be at an increased risk for suicidal ideation and suicidal behaviors (20, 103, 104), which supports a circadian hypothesis of depression that based, in part, on data showing that a subgroup of depressed patients has dysregulated 24 h rhythms including sleep, hormonal secretions, core body temperature and mood (17, 24, 105, 106). Perhaps the strongest and most direct evidence for a circadian defect in depression comes from a study of postmortem 24 h sinusoidal gene expression rhythms across six regions of human brain showing a dramatic dysregulation of circadian genes in MDD compared to controls (19). This article has further revealed that the expression of core clock genes, including Period genes (PER1-3) was different between controls and MDD patients. PER genes regulate circadian rhythm by repressing the transcriptional activity driven by upstream rhythm genes, and inhibiting their own expression through a negative autoregulatory feedback loop that cycle in about 24 h (107, 108). Besides, PER genes show a staggered phase relationship, with PRE1 peaking soon after sunrise, PER3 peaking during midday, and PER2 peaking in the afternoon (19, 109, 110) while this circadian pattern was weak in MDD patients (19). A genome-wide study had found a strong relationship between sleep deprivation and the expression of PER genes in MDD and bipolar disorder patients (111). Moreover, the role of the circadian clock in the homeostasis of stem cells and in the regulation of cellular development including differentiation across tissue subtypes is supported by numerous studies (112–114). And the circadian rhythm disorder may disrupt normal sleep rhythm, which promotes myelination and oligodendrocyte precursor cells proliferation and is associated with higher expression of genes coding for phospholipid synthesis and myelination in oligodendrocytes (115–117). These brain alterations caused by circadian clock genes have been related to neurogenesis, and would further associated with psychiatric disease including depression (118, 119).
The present study has several limitations that should be considered. First, large samples of future researches were needed to validate our present study. Second, the current study was cross-sectional but not longitudinal. Whether subjects in the AG group with lower FA and higher MD and RD in specific emotion-related WM tracts really develop to depression should be investigated in the future longitudinal studies. Third, we measured the depression state by BDI in healthy college students. Further studies should verify our results in depressed people. Forth, we found that several emotion-related WM tracts were associated with BDI in AG genotype. However, our present study did not investigate the performance of emotion-related tasks. Future research should explore the effect of PER1 polymorphism on the association of WM microstructure integrity with specific emotion-related task.
Conclusion
In conclusion, decreased FA in several WM tracts were shown in PER1 (rs7221412) AG group compared with the AA group, and PER1 genotype had an interaction effect on the associations between the WM microstructural integrity in emotion-related WM tracts and depression level. These findings suggested that the PER1 gene polymorphisms and WM characteristics in several emotion-related regions may provide effective measures of prediction for depressive risk at an early phase. Future studies should validate these results in large sample.
Data availability statement
The raw data supporting the conclusions of this article will be made available by the authors, without undue reservation.
Ethics statement
The studies involving human participants were reviewed and approved by the Institutional Research Ethics Committee of the Xijing Hospital of the Fourth Military Medical University. The patients/participants provided their written informed consent to participate in this study.
Author contributions
RZ, J-BS, HD, and X-JY were guarantors of integrity of the entire study. RZ, J-BS, L-ML, and WQ contributed to study concepts/study design. RZ, XL, F-MW, Z-YH, and M-YC contributed to data acquisition. RZ, J-BS, HD, L-ML, C-ZT, and N-GX contributed to data analysis/interpretation. RZ, J-BS, HD, and CC contributed to manuscript drafting or manuscript revision. All authors contributed to manuscript revision and read and approved the submitted version.
Funding
This study was funded by the Innovation Team and Talents Cultivation Program of National Administration of Traditional Chinese Medicine (Grant No. ZYYCXTD-C-202004), the National Key R&D Program of China (Grant No. 2021YFF0306500), the Key Research and Development Program of Shaanxi (Grant No. 2020ZDLSF04-05), the National Science Foundation of China (Grant No. 81901827), Natural Science Basic Research Program of Shaanxi (Grant Nos. 2021JQ-211 and 2022JQ-649) and the Ph.D. start-up fund of Xi’an Polytechnic University (Grant No. BS201914).
Acknowledgments
We thank the subjects whose participation enabled this work.
Conflict of interest
The authors declare that the research was conducted in the absence of any commercial or financial relationships that could be construed as a potential conflict of interest.
Publisher’s note
All claims expressed in this article are solely those of the authors and do not necessarily represent those of their affiliated organizations, or those of the publisher, the editors and the reviewers. Any product that may be evaluated in this article, or claim that may be made by its manufacturer, is not guaranteed or endorsed by the publisher.
Footnotes
- ^ http://www.fmrib.ox.ac.uk/fsl
- ^ https://fsl.fmrib.ox.ac.uk/fsl/fslwiki/GLM#Two_Groups_with_continuous_covariate_interaction
References
1. Kessler RC, Bromet EJ. The epidemiology of depression across cultures. Annu Rev Public Health. (2013) 34:119. doi: 10.1146/annurev-publhealth-031912-114409
2. Kupfer DJ, Frank E, Phillips ML. Major depressive disorder: new clinical, neurobiological, and treatment perspectives. Lancet. (2012) 379:1045–55. doi: 10.1016/S0140-6736(11)60602-8
3. GBD 2019 Mental Disorders Collaborators. Global, regional, and national burden of 12 mental disorders in 204 countries and territories, 1990–2019: a systematic analysis for the Global Burden of Disease Study 2019. Lancet Psychiatry. (2022) 9:137–50. doi: 10.1016/S2215-0366(21)00395-3
4. World Health Organization,. Depression. (2021). Available online at: https://www.who.int/news-room/fact-sheets/detail/depression (accessed September 13, 2021).
5. GBD 2019 Diseases and Injuries Collaborators. Global burden of 369 diseases and injuries in 204 countries and territories, 1990–2019: a systematic analysis for the Global Burden of Disease Study 2019. Lancet. (2020) 396:1204–22.
6. Hasin DS, Sarvet AL, Meyers JL, Saha TD, Ruan WJ, Stohl M, et al. Epidemiology of adult DSM-5 major depressive disorder and its specifiers in the United States. JAMA Psychiatry. (2018) 75:336–46. doi: 10.1001/jamapsychiatry.2017.4602
7. Otte C, Gold SM, Penninx BW, Pariante CM, Etkin A, Fava M, et al. Major depressive disorder. Nat Rev Dis Primers. (2016) 2:16065. doi: 10.1038/nrdp.2016.65
9. Lopez AD, Mathers CD, Ezzati M, Jamison DT, Murray CJ. Global and regional burden of disease and risk factors, 2001: systematic analysis of population health data. Lancet. (2006) 367:1747–57. doi: 10.1016/S0140-6736(06)68770-9
10. Hickie IB, Scott EM, Hermens DF, Naismith SL, Guastella AJ, Kaur M, et al. Applying clinical staging to young people who present for mental health care. Early Interv Psychiatry. (2013) 7:31–43. doi: 10.1111/j.1751-7893.2012.00366.x
11. Scott EM, Hermens DF, Glozier N, Naismith SL, Guastella AJ, Hickie IB, et al. Targeted primary care-based mental health services for young Australians. Med J Aust. (2012) 196:136–40. doi: 10.5694/mja11.10481
12. Kronfeld-Schor N, Einat H. Circadian rhythms and depression: human psychopathology and animal models. Neuropharmacology. (2012) 62:101–14. doi: 10.1016/j.neuropharm.2011.08.020
13. Monteleone P, Maj M. The circadian basis of mood disorders: recent developments and treatment implications. Eur Neuropsychopharmacol. (2008) 18:701–11. doi: 10.1016/j.euroneuro.2008.06.007
14. McClung CA. Circadian genes, rhythms and the biology of mood disorders. Pharmacol. Ther. (2007) 114:222–32. doi: 10.1016/j.pharmthera.2007.02.003
15. Germain A, Kupfer DJ. Circadian rhythm disturbances in depression. Hum Psychopharmacol. (2008) 23:571–85. doi: 10.1002/hup.964
16. Emens J, Lewy A, Kinzie JM, Arntz D, Rough J. Circadian misalignment in major depressive disorder. Psychiatry Res. (2009) 168:259–61. doi: 10.1016/j.psychres.2009.04.009
17. Lyall LM, Wyse CA, Graham N, Ferguson A, Lyall DM, Cullen B, et al. Association of disrupted circadian rhythmicity with mood disorders, subjective wellbeing, and cognitive function: a cross-sectional study of 91 105 participants from the UK Biobank. Lancet Psychiatry. (2018) 5:507–14. doi: 10.1016/S2215-0366(18)30139-1
18. Hickie IB, Naismith SL, Robillard R, Scott EM, Hermens DF. Manipulating the sleep-wake cycle and circadian rhythms to improve clinical management of major depression. BMC Med. (2013) 11:79. doi: 10.1186/1741-7015-11-79
19. Li JZ, Bunney BG, Meng F, Hagenauer MH, Walsh DM, Vawter MP, et al. Circadian patterns of gene expression in the human brain and disruption in major depressive disorder. Proc Natl Acad Sci U.S.A. (2013) 110:9950–5. doi: 10.1073/pnas.1305814110
20. Sato S, Bunney B, Mendoza-Viveros L, Bunney W, Borrelli E, Sassone-Corsi P, et al. Rapid-acting antidepressants and the circadian clock. Neuropsychopharmacology. (2022) 47:805–16. doi: 10.1038/s41386-021-01241-w
21. Bunney BG, Li JZ, Walsh DM, Stein R, Vawter MP, Cartagena P, et al. Circadian dysregulation of clock genes: clues to rapid treatments in major depressive disorder. Mol Psychiatry. (2015) 20: 48–55. doi: 10.1038/mp.2014.138
22. Franzen PL, Buysse DJ. Sleep disturbances and depression: risk relationships for subsequent depression and therapeutic implications. Dialogues Clin Neurosci. (2008) 10:473. doi: 10.31887/DCNS.2008.10.4/plfranzen
23. Hickie IB, Rogers NL. Novel melatonin-based therapies: potential advances in the treatment of major depression. Lancet. (2011) 378:621–31. doi: 10.1016/S0140-6736(11)60095-0
24. Logan RW, McClung CA. Rhythms of life: circadian disruption and brain disorders across the lifespan. Nat Rev Neurosci. (2019) 20:49–65. doi: 10.1038/s41583-018-0088-y
25. Chellappa S, Morris C, Scheer F. Circadian misalignment increases mood vulnerability in simulated shift work. Sci Rep. (2020) 10:18614. doi: 10.1038/s41598-020-75245-9
26. Turek FW. From circadian rhythms to clock genes in depression. Int Clin Psychopharmacol. (2007) 22:S1–8. doi: 10.1097/01.yic.0000277956.93777.6a
27. Charrier A, Olliac B, Roubertoux P, Tordjman S. Clock genes and altered sleep-wake rhythms: their role in the development of psychiatric disorders. Int J Mol Sci. (2017) 18:938. doi: 10.3390/ijms18050938
28. Wray NR, Ripke S, Mattheisen M, Trzaskowski M, Byrne EM, Abdellaoui A, et al. Genome-wide association analyses identify 44 risk variants and refine the genetic architecture of major depression. Nat Genet. (2018) 50:668–81.
29. Christiansen S, Bouzinova E, Fahrenkrug J, Wiborg O. Altered expression pattern of clock genes in a rat model of depression. Int J Neuropsychopharmacol. (2016) 19:yw061. doi: 10.1093/ijnp/pyw061
30. Savalli G, Diao W, Schulz S, Todtova K, Pollak DD. Diurnal oscillation of amygdala clock gene expression and loss of synchrony in a mouse model of depression. Int J Neuropsychopharmacol. (2014) 18:yu095. doi: 10.1093/ijnp/pyu095
31. Logan RW, Edgar N, Gillman AG, Hoffman D, Zhu X, McClung CA. Chronic stress induces brain region-specific alterations of molecular rhythms that correlate with depression-like behavior in mice. Biol Psychiatry. (2015) 78:249–58. doi: 10.1016/j.biopsych.2015.01.011
32. Li S-X, Liu L-J, Xu L-Z, Gao L, Wang XF, Zhang JT, et al. Diurnal alterations in circadian genes and peptides in major depressive disorder before and after escitalopram treatment. Psychoneuroendocrinology. (2013) 38:2789–99. doi: 10.1016/j.psyneuen.2013.07.009
33. Shi S, White M, Borsetti H, Pendergast JS, Hida A, Ciarleglio CM, et al. Molecular analyses of circadian gene variants reveal sex-dependent links between depression and clocks. Transl Psychiatry. (2016) 6:e748. doi: 10.1038/tp.2016.9
34. Lavebratt C, Sjöholm L, Partonen T, Schalling M, Forsell Y. PER2 variantion is associated with depression vulnerability. Am J Med Genet B Neuropsychiatr Genet. (2010) 153:570–81. doi: 10.1002/ajmg.b.31021
35. Schnell A, Albrecht U, Sandrelli F. Rhythm and mood: relationships between the circadian clock and mood-related behavior. Behav Neurosci. (2014) 128:326. doi: 10.1037/a0035883
36. Meyer-Lindenberg A, Weinberger DR. Intermediate phenotypes and genetic mechanisms of psychiatric disorders. Nat Rev Neurosci. (2006) 7:818–27. doi: 10.1038/nrn1993
37. Won E, Ham B-J. Imaging genetics studies on monoaminergic genes in major depressive disorder. Prog Neuropsychopharmacol Biol Psychiatry. (2016) 64:311–9. doi: 10.1016/j.pnpbp.2015.03.014
38. Han KM, Won E, Sim Y, Kang J, Han C, Kim YK, et al. Influence of FKBP5 polymorphism and DNA methylation on structural changes of the brain in major depressive disorder. Sci Rep. (2017) 7:42621. doi: 10.1038/srep42621
39. Ancelin ML, Carriere I, Artero S, Artero S, Maller J, Meslin C, et al. Lifetime major depression and grey-matter volume. J Psychiatry Neurosci. (2019) 44:45–53. doi: 10.1503/jpn.180026
40. Zhang HF, Mellor D, Peng DH. Neuroimaging genomic studies in major depressive disorder: a systematic review. CNS Neurosci Ther. (2018) 24:1020–36. doi: 10.1111/cns.12829
41. Gong L, He C, Yin Y, Ye Q, Bai F, Yuan Y, et al. Nonlinear modulation of interacting between COMT and depression on brain function. Eur Psychiatry. (2017) 45:6–13. doi: 10.1016/j.eurpsy.2017.05.024
42. Tatham EL, Hall GB, Clark D, Foster J, Ramasubbu R. The 5-HTTLPR and BDNF polymorphisms moderate the association between uncinate fasciculus connectivity and antidepressants treatment response in major depression. Eur Arch Psychiatry Clin Neurosci. (2016) 267:135–47. doi: 10.1007/s00406-016-0702-9
43. Tatham EL, Ramasubbu R, Gaxiola-Valdez I, Cortese F, Clark D, Goodyear B, et al. White matter integrity in major depressive disorder: implications of childhood trauma, 5-HTTLPR and BDNF polymorphisms. Psychiatry Res Neuroimaging. (2016) 253:15–25. doi: 10.1016/j.pscychresns.2016.04.014
44. Carballedo A, Amico F, Ugwu I, Fagan AJ, Fahey C, Morris D, et al. Reduced fractional anisotropy in the uncinate fasciculus in patients with major depression carrying the met-allele of the Val66Met brain-derived neurotrophic factor genotype. Am J Med Genet B Neuropsychiatr Genet. (2012) 159:537–48. doi: 10.1002/ajmg.b.32060
45. Choi S, Han K-M, Kang J, Won E, Chang HS, Tae WS, et al. Effects of a polymorphism of the neuronal amino acid transporter SLC6A15 gene on structural integrity of white matter tracts in major depressive disorder. PLoS One. (2016) 11:e0164301. doi: 10.1371/journal.pone.0164301
46. Murphy ML, Carballedo A, Fagan AJ, Morris D, Fahey C, Meaney J, et al. Neurotrophic tyrosine kinase polymorphism impacts white matter connections in patients with major depressive disorder. Biol Psychiatry. (2012) 72:663–70. doi: 10.1016/j.biopsych.2012.04.015
47. Tozzi L, Carballedo A, Wetterling F, McCarthy H, O’Keane V, Gill M, et al. Single-nucleotide polymorphism of the FKBP5 gene and childhood maltreatment as predictors of structural changes in brain areas involved in emotional processing in depression. Neuropsychopharmacology. (2016) 41:487–97. doi: 10.1038/npp.2015.170
48. Hayashi K, Yoshimura R, Kakeda S, Kishi T, Abe O, Umene-Nakano W, et al. COMT Val158Met, but not BDNF Val66Met, is associated with white matter abnormalities of the temporal lobe in patients with first-episode, treatment-naive major depressive disorder: a diffusion tensor imaging study. Neuropsychiatr Dis Treat. (2014) 10:1183. doi: 10.2147/NDT.S61275
49. Seok J-H, Choi S, Lim HK, Lee SH, Kim I, Ham BJ, et al. Effect of the COMT val158met polymorphism on white matter connectivity in patients with major depressive disorder. Neurosci Lett. (2013) 545:35–9. doi: 10.1016/j.neulet.2013.04.012
50. Won E, Han K-M, Kang J, Kim A, Yoon HK, Chang HS, et al. Vesicular monoamine transporter 1 gene polymorphism and white matter integrity in major depressive disorder. Prog Neuropsychopharmacol Biol Psychiatry. (2017) 77:138–45. doi: 10.1016/j.pnpbp.2017.02.028
51. Ping L, Xu J, Zhou C, Lu J, Lu Y, Shen Z, et al. Tryptophan hydroxylase-2 polymorphism is associated with white matter integrity in first-episode, medication-naïve major depressive disorder patients. Psychiatry Res Neuroimaging. (2019) 286:4–10. doi: 10.1016/j.pscychresns.2019.02.002
52. Kakeda S, Watanabe K, Katsuki A, Sugimoto K, Ueda I, Igata N, et al. Genetic effects on white matter integrity in drug-naive patients with major depressive disorder: a diffusion tensor imaging study of 17 genetic loci associated with depressive symptoms. Neuropsychiatr Dis Treat. (2019) 15:375–83. doi: 10.2147/NDT.S190268
53. Whalley HC, Sprooten E, Hackett S, Hall L, Blackwood DH, Glahn DC, et al. Polygenic risk and white matter integrity in individuals at high risk of mood disorder. Biol Psychiatry. (2013) 74:280–6. doi: 10.1016/j.biopsych.2013.01.027
54. Won E, Choi S, Kang J, Kim A, Han KM, Chang HS, et al. Association between reduced white matter integrity in the corpus callosum and serotonin transporter gene DNA methylation in medication-naive patients with major depressive disorder. Transl Psychiatry. (2016) 6:e866. doi: 10.1038/tp.2016.137
55. Choi S, Han K-M, Won E, Yoon BJ, Lee MS, Ham BJ, et al. Association of brain-derived neurotrophic factor DNA methylation and reduced white matter integrity in the anterior corona radiata in major depression. J Affect Disord. (2015) 172:74–80. doi: 10.1016/j.jad.2014.09.042
56. Na KS, Won E, Kang J, Kim A, Choi S, Tae WS, et al. Differential effect of COMT gene methylation on the prefrontal connectivity in subjects with depression versus healthy subjects. Neuropharmacology. (2018) 137:59–70. doi: 10.1016/j.neuropharm.2018.04.030
57. Bollettini I, Melloni EMT, Aggio V, Poletti S, Lorenzi C, Pirovano A, et al. Clock genes associate with white matter integrity in depressed bipolar patients. Chronobiol Int. (2017) 34:212–24. doi: 10.1080/07420528.2016.1260026
58. Lim AS, Chang AM, Shulman JM, Raj T, Chibnik LB, Cain SW, et al. A common polymorphism near PER1 and the timing of human behavioral rhythms. Ann Neurol. (2012) 72:324–34. doi: 10.1002/ana.23636
59. Beck AT, Steer RA, Ball R, Ranieri W. Comparison of beck depression inventories-IA and-II in psychiatric outpatients. J Pers Assess. (1996) 67:588–97. doi: 10.1207/s15327752jpa6703_13
60. Levandovski R, Dantas G, Fernandes LC, Caumo W, Torres I, Roenneberg T, et al. Depression scores associate with chronotype and social jetlag in a rural population. Chronobiol Int. (2011) 28:771–8. doi: 10.3109/07420528.2011.602445
61. Peng X, Lau WKW, Wang C, Ning L, Zhang R. Impaired left amygdala resting state functional connectivity in subthreshold depression individuals. Sci Rep. (2020) 10:17207. doi: 10.1038/s41598-020-74166-x
62. Hu Y, Wu X, Chen X, Maguire P, Wang D. Can increased cognitive load help people with subthreshold depression to forget negative information? J Affect Disord. (2021) 283:384–94. doi: 10.1016/j.jad.2021.01.062
63. Li X, Mo X, Liu T, Shao R, Teopiz K, McIntyre RS, et al. Efficacy of Lycium barbarum polysaccharide in adolescents with subthreshold depression: interim analysis of a randomized controlled study. Neural Regen Res. (2022) 17:1582–7. doi: 10.4103/1673-5374.330618
64. Zhang S, Zhang Y, Ma W, Qi Z, Wang Y, Tao Q, et al. Neural correlates of negative emotion processing in subthreshold depression. Soc Cogn Affect Neurosci. (2022) 17:655–61. doi: 10.1093/scan/nsac003
65. Smarr KL, Keefer AL. Measures of depression and depressive symptoms. Arthritis Care Res. (2020) 72(Suppl. 10):608–29. doi: 10.1002/acr.24191
66. Roenneberg T, Wirz-Justice A, Merrow M. Life between clocks: daily temporal patterns of human chronotypes. J Biol Rhythms. (2003) 18:80–90. doi: 10.1177/0748730402239679
67. Roenneberg T, Kuehnle T, Pramstaller PP, Ricken J, Havel M, Guth A, et al. A marker for the end of adolescence. Curr Biol. (2004) 14:R1038–9. doi: 10.1016/j.cub.2004.11.039
68. Wittmann M, Dinich J, Merrow M, Roenneberg T. Social jetlag: misalignment of biological and social time. Chronobiol Int. (2006) 23:497–509. doi: 10.1080/07420520500545979
69. Buysse DJ, Reynolds CF, Monk TH, Monk TH, Berman SR, Kupfer DJ, et al. The Pittsburgh Sleep Quality Index: a new instrument for psychiatric practice and research. Psychiatry Res. (1989) 28:193–213. doi: 10.1016/0165-1781(89)90047-4
70. Morin CM, Belleville G, Bélanger L, Ivers H. The Insomnia Severity Index: psychometric indicators to detect insomnia cases and evaluate treatment response. Sleep. (2011) 34:601–8. doi: 10.1093/sleep/34.5.601
71. Manzar MD, Jahrami HA, Bahammam AS. Structural validity of the Insomnia Severity Index: a systematic review and meta-analysis. Sleep Med Rev. (2021) 60:101531. doi: 10.1016/j.smrv.2021.101531
72. Jenkinson M, Smith S. A global optimisation method for robust affine registration of brain images. Med Image Anal. (2001) 5:143–56. doi: 10.1016/S1361-8415(01)00036-6
73. Smith SM. Fast robust automated brain extraction. Hum Brain Mapp. (2002) 17:143–55. doi: 10.1002/hbm.10062
74. Smith SM, Jenkinson M, Johansen-Berg H, Rueckert D, Nichols TE, Mackay CE, et al. Tract-based spatial statistics: voxelwise analysis of multi-subject diffusion data. Neuroimage. (2006) 31:1487–505. doi: 10.1016/j.neuroimage.2006.02.024
75. Rueckert D, Sonoda LI, Hayes C, Hill DL, Leach MO, Hawkes DJ, et al. Nonrigid registration using free-form deformations: application to breast MR images. IEEE Trans Med Imaging. (1999) 18:712–21. doi: 10.1109/42.796284
76. Demiral SB, Tomasi D, Sarlls J, Lee H, Wiers CE, Zehra A, et al. Apparent diffusion coefficient changes in human brain during sleep – Does it inform on the existence of a glymphatic system? Neuroimage. (2019) 185:263–73. doi: 10.1016/j.neuroimage.2018.10.043
77. Jiang C, Zhang L, Zou C, Long X, Liu X, Zheng H, et al. Diurnal microstructural variations in healthy adult brain revealed by diffusion tensor imaging. PLoS One. (2014) 9:e84822. doi: 10.1371/journal.pone.0084822
78. Nakamura K, Brown RA, Narayanan S, Collins DL, Arnold DL. Diurnal fluctuations in brain volume: statistical analyses of MRI from large populations. Neuroimage. (2015) 118:126–32. doi: 10.1016/j.neuroimage.2015.05.077
79. Smith SM, Nichols TE. Threshold-free cluster enhancement: addressing problems of smoothing, threshold dependence and localisation in cluster inference. Neuroimage. (2009) 44:83–98. doi: 10.1016/j.neuroimage.2008.03.061
80. Mori S, Oishi K, Jiang H, Jiang L, Li X, Akhter K, et al. Stereotaxic white matter atlas based on diffusion tensor imaging in an ICBM template. Neuroimage. (2008) 40:570–82. doi: 10.1016/j.neuroimage.2007.12.035
81. Shen T, You Y, Joseph C, Mirzaei M, Klistorner A, Graham SL, et al. BDNF polymorphism: a review of its diagnostic and clinical relevance in neurodegenerative disorders. Aging Dis. (2018) 9:523–36. doi: 10.14336/AD.2017.0717
82. Dhana K, Barnes LL, Liu X, Agarwal P, Desai P, Krueger KR, et al. Genetic risk, adherence to a healthy lifestyle, and cognitive decline in African Americans and European Americans. Alzheimers Dement. (2022) 18:572–80. doi: 10.1002/alz.12435
83. Carmichael J, Hicks AJ, Spitz G, Gould KR, Ponsford J. Moderators of gene-outcome associations following traumatic brain injury. Neurosci Biobehav Rev. (2021) 130:107–24. doi: 10.1016/j.neubiorev.2021.08.015
84. Wang M, Ma Y, Yuan W, Su K, Li MD. Meta-analysis of the COMT Val158Met polymorphism in major depressive disorder: effect of ethnicity. J Neuroimmune Pharmacol. (2016) 11:434–45. doi: 10.1007/s11481-016-9651-3
85. McFarquhar M, Elliott R, McKie S, Thomas E, Downey D, Mekli K, et al. TOMM40 rs2075650 may represent a new candidate gene for vulnerability to major depressive disorder. Neuropsychopharmacology. (2014) 39:1743–53. doi: 10.1038/npp.2014.22
86. Gutiérrez B, Bellón JÁ, Rivera M, Molina E, King M, Marston L, et al. The risk for major depression conferred by childhood maltreatment is multiplied by BDNF and SERT genetic vulnerability: a replication study. J Psychiatry Neurosci. (2015) 40:187–96. doi: 10.1503/jpn.140097
87. Ancelin ML, Scali J, Norton J, Ritchie K, Dupuy AM, Chaudieu I, et al. Heterogeneity in HPA axis dysregulation and serotonergic vulnerability to depression. Psychoneuroendocrinology. (2017) 77:90–4. doi: 10.1016/j.psyneuen.2016.11.016
88. Delli Colli C, Borgi M, Poggini S, Chiarotti F, Cirulli F, Penninx BWJH, et al. Time moderates the interplay between 5-HTTLPR and stress on depression risk: gene x environment interaction as a dynamic process. Transl Psychiatry. (2022) 12:274. doi: 10.1038/s41398-022-02035-4
89. Davis EG, Keller J, Hallmayer J, Pankow HR, Murphy GM Jr, Gotlib IH, et al. Corticotropin-releasing factor 1 receptor haplotype and cognitive features of major depression. Transl Psychiatry. (2018) 8:5. doi: 10.1038/s41398-017-0051-0
90. Chao J-K, Yang M-C, Chen C-S, Wang IC, Kao WT, Shi MD. A gender-specific COMT haplotype contributes to risk modulation rather than disease severity of major depressive disorder in a Chinese population. J Affect Disord. (2019) 246:376–86. doi: 10.1016/j.jad.2018.12.088
91. He E, Liu M, Gong S, Fu X, Han Y, Deng F, et al. White matter alterations in depressive disorder. Front Immunol. (2022) 13:826812. doi: 10.3389/fimmu.2022.826812
92. Chen G, Hu X, Li L, Huang X, Lui S, Kuang W, et al. Disorganization of white matter architecture in major depressive disorder: a meta-analysis of diffusion tensor imaging with tract-based spatial statistics. Sci Rep. (2016) 6:21825. doi: 10.1038/srep21825
93. Lee J, Chi S, Ko M, Song M, Ham BJ, Ko YH, et al. Prospective study on microstructure in medication-naïve adolescents with first-episode major depressive disorder. J Affect Disord. (2021) 293:268–75. doi: 10.1016/j.jad.2021.06.048
94. van Velzen L, Kelly S, Isaev D, Aleman A, Aftanas LI, Bauer J, et al. White matter disturbances in major depressive disorder: a coordinated analysis across 20 international cohorts in the ENIGMA MDD working group. Mol Psychiatry. (2020) 25:1511–25.
95. Vulser H, Paillere Martinot ML, Artiges E, Miranda R, Penttilä J, Grimmer Y, et al. Early variations in white matter microstructure and depression outcome in adolescents with subthreshold depression. Am J Psychiatry. (2018) 175:1255–64. doi: 10.1176/appi.ajp.2018.17070825
96. Lacerda AL, Brambilla P, Sassi RB, Nicoletti MA, Mallinger AG, Frank E, et al. Anatomical MRI study of corpus callosum in unipolar depression. J Psychiatr Res. (2005) 39:347–54. doi: 10.1016/j.jpsychires.2004.10.004
97. Stave EA, De Bellis MD, Hooper SR, Woolley DP, Chang SK, Chen SD, et al. Dimensions of attention associated with the microstructure of corona radiata white matter. J Child Neurol. (2017) 32:458–66. doi: 10.1177/0883073816685652
98. Wakana S, Jiang H, Nagae-Poetscher LM, van Zijl PC, Mori S. Fiber tract-based atlas of human white matter anatomy. Radiology. (2004) 230:77–87. doi: 10.1148/radiol.2301021640
99. Henderson SE, Johnson AR, Vallejo AI, Katz L, Wong E, Gabbay V, et al. A preliminary study of white matter in adolescent depression: relationships with illness severity, anhedonia, and irritability. Front Psychiatry. (2013) 4:152. doi: 10.3389/fpsyt.2013.00152
100. Xiao J, He Y, McWhinnie CM, Yao S. Altered white matter integrity in individuals with cognitive vulnerability to depression: a tract-based spatial statistics study. Sci Rep. (2015) 5:9738. doi: 10.1038/srep09738
101. Roos A, Wedderburn CJ, Fouche JP, Joshi SH, Narr KL, Woods RP, et al. Prenatal depression exposure alters white matter integrity and neurodevelopment in early childhood. Brain Imaging Behav. (2022) 16:1324–36. doi: 10.1007/s11682-021-00616-3
102. Hermens D, Jamieson D, Fitzpatrick L, Sacks DD, Iorfino F, Crouse JJ, et al. Sex differences in fronto-limbic white matter tracts in youth with mood disorders. Psychiatry Clin Neurosci. (2022) 76:481–9. doi: 10.1111/pcn.13440
103. Geoffroy PA, Palagini L. Biological rhythms and chronotherapeutics in depression. Prog Neuropsychopharmacol Biol Psychiatry. (2021) 106: 110158. doi: 10.1016/j.pnpbp.2020.110158
104. Bernert R, Kim J, Iwata N, Perlis ML. Sleep disturbances as an evidence-based suicide risk factor. Curr Psychiatry Rep. (2015) 17:554. doi: 10.1007/s11920-015-0554-4
105. Wulff K, Gatti S, Wettstein JG, Foster RG. Sleep and circadian rhythm disruption in psychiatric and neurodegenerative disease. Nat Rev Neurosci. (2010) 11:589–99. doi: 10.1038/nrn2868
106. Hasler BP, Buysse DJ, Kupfer DJ, Germain A. Phase relationships between core body temperature, melatonin, and sleep are associated with depression severity: further evidence for circadian misalignment in non-seasonal depression. Psychiatry Res. (2010) 178:205–7. doi: 10.1016/j.psychres.2010.04.027
107. Orozco-Solis R, Sassone-Corsi P. Epigenetic control and the circadian clock: linking metabolism to neuronal responses. Neuroscience. (2014) 264: 76–87. doi: 10.1016/j.neuroscience.2014.01.043
108. Aguilar-Arnal L, Sassone-Corsi P. The circadian epigenome: how metabolism talks to chromatin remodeling. Curr Opin Cell Biol. (2013) 25:170–6. doi: 10.1016/j.ceb.2013.01.003
109. Bellet MM, Sassone-Corsi P. Mammalian circadian clock and metabolism – the epigenetic link. J Cell Sci. (2010) 123:3837–48. doi: 10.1242/jcs.051649
110. Masri S, Sassone-Corsi P. Plasticity and specificity of the circadian epigenome. Nat Neurosci. (2010) 13:1324–9. doi: 10.1038/nn.2668
111. Foo J, Trautmann N, Sticht C, Treutlein J, Frank J, Streit F, et al. Longitudinal transcriptome-wide gene expression analysis of sleep deprivation treatment shows involvement of circadian genes and immune pathways. Transl Psychiatry. (2019) 9:343. doi: 10.1038/s41398-019-0671-7
112. Aguilar-Arnal L, Sassone-Corsi P. The clock within. Nature. (2011) 480:185–7. doi: 10.1038/480185a
113. Mogi A, Yomoda R, Kimura S, Tsushima C, Takouda J, Sawauchi M, et al. Entrainment of the circadian clock in neural stem cells by epidermal growth factor is closely associated with ERK1/2-mediated induction of multiple clock-related genes. Neuroscience. (2018) 379:45–66. doi: 10.1016/j.neuroscience.2018.02.045
114. Benitah SA, Welz PS. Circadian regulation of adult stem cell homeostasis and aging. Cell Stem Cell. (2020) 26:817–31. doi: 10.1016/j.stem.2020.05.002
115. Cirelli C, Gutierrez CM, Tononi G. Extensive and divergent effects of sleep and wakefulness on brain gene expression. Neuron. (2004) 41:35–43. doi: 10.1016/S0896-6273(03)00814-6
116. Bellesi M, Pfister-Genskow M, Maret S, Keles S, Tononi G, Cirelli C. Effects of sleep and wake on oligodendrocytes and their precursors. J Neurosci. (2013) 33:14288–300. doi: 10.1523/JNEUROSCI.5102-12.2013
117. Hinard V, Mikhail C, Pradervand S, Curie T, Houtkooper RH, Auwerx J, et al. Key electrophysiological, molecular, and metabolic signatures of sleep and wakefulness revealed in primary cortical cultures. J Neurosci. (2012) 32: 12506–17. doi: 10.1523/JNEUROSCI.2306-12.2012
118. Mirza FJ, Amber S, Sumera, Hassan D, Ahmed T, Zahid S, et al. Rosmarinic acid and ursolic acid alleviate deficits in cognition, synaptic regulation and adult hippocampal neurogenesis in an Abeta1-42-induced mouse model of Alzheimer’s disease. Phytomedicine. (2021) 83:153490. doi: 10.1016/j.phymed.2021.153490
Keywords: PER1 gene polymorphisms, diffusion tensor imaging, Beck Depression Inventory, depressive risk, tract-based spatial statistics
Citation: Zhao R, Sun J-B, Deng H, Cheng C, Li X, Wang F-M, He Z-Y, Chang M-Y, Lu L-M, Tang C-Z, Xu N-G, Yang X-J and Qin W (2022) Per1 gene polymorphisms influence the relationship between brain white matter microstructure and depression risk. Front. Psychiatry 13:1022442. doi: 10.3389/fpsyt.2022.1022442
Received: 18 August 2022; Accepted: 24 October 2022;
Published: 11 November 2022.
Edited by:
Lu Liu, Peking University Sixth Hospital, ChinaReviewed by:
Yuzhen Liu, Chinese Academy of Medical Sciences and Peking Union Medical College, ChinaCarlos Portugal-Nunes, University of Trás-os-Montes and Alto Douro, Portugal
Copyright © 2022 Zhao, Sun, Deng, Cheng, Li, Wang, He, Chang, Lu, Tang, Xu, Yang and Qin. This is an open-access article distributed under the terms of the Creative Commons Attribution License (CC BY). The use, distribution or reproduction in other forums is permitted, provided the original author(s) and the copyright owner(s) are credited and that the original publication in this journal is cited, in accordance with accepted academic practice. No use, distribution or reproduction is permitted which does not comply with these terms.
*Correspondence: Jin-Bo Sun, sunjb@xidian.edu.cn; Xue-Juan Yang, xjyang@xidian.edu.cn
†These authors have contributed equally to this work