- 1Department of Psychiatry, Seoul National University Health Service Center, Seoul, South Korea
- 2Department of Psychology, Sogang University, Seoul, South Korea
- 3Institute for Hope Research, Sogang University, Seoul, South Korea
- 4Seoul National University Hospital, Seoul, South Korea
- 5Yeongeon Student Support Center, Seoul National University College of Medicine, Seoul, South Korea
- 6Department of Psychiatry, Seoul National University College of Medicine, Seoul, South Korea
- 7Department of Human Systems Medicine, Seoul National University College of Medicine, Seoul, South Korea
Objectives: Sleep disturbances are associated with both the onset and progression of depressive disorders. It is important to capture day-to-day variability in sleep patterns; irregular sleep is associated with depressive symptoms. We used sleep efficiency, measured with wearable devices, as an objective indicator of daily sleep variability.
Materials and methods: The total sample consists of 100 undergraduate and graduate students, 60% of whom were female. All were divided into three groups (with major depressive disorder, mild depressive symptoms, and controls). Self-report questionnaires were completed at the beginning of the experiment, and sleep efficiency data were collected daily for 2 weeks using wearable devices. We explored whether the mean value of sleep efficiency, and its variability, predicted the severity of depression using dynamic structural equation modeling.
Results: More marked daily variability in sleep efficiency significantly predicted levels of depression and anxiety, as did the average person-level covariates (longer time in bed, poorer quality of life, lower extraversion, and higher neuroticism).
Conclusion: Large swings in day-to-day sleep efficiency and certain clinical characteristics might be associated with depression severity in young adults.
Introduction
Young adulthood features psychological and environmental changes sometimes associated with the onset of depression and/or insomnia (1). A longitudinal epidemiological study of young adults found that those with lifetime histories of insomnia and/or hypersomnia evidenced higher rates of major depression and other psychiatric disorders (2). Insomnia or hypersomnia almost every day is one of the features of depressive symptoms (3), and existing literature on the link between depression and sleep disturbance indicates that depression and sleep disturbance are bidirectionally related (4–6). Sleep disturbance may be prodromal in terms of depression development (6, 7); sleep problems often precede depressive episodes (8). Sleep disturbances must be carefully evaluated when diagnosing depression.
Night-to-night sleep variability is an important second dimension of the sleep domain; individual-level sleep mean is the first dimension (9–11). Although sleep may vary from day-to-day, most research has focused on an individual average sleep (9). However, sleep variability is more predictive of various medical conditions than is the average sleep level (9, 12). Greater daily sleep variability may be associated with adverse health outcomes such as maladaptive health behaviors and impaired physiological processes (13). It may be considered to quantify the variability in daily sleep quality as a method of measuring sleep disturbance when diagnosing depressive symptoms.
Sleep quality is an important predictor of depression onset or recurrence (14, 15). Sleep quality is measured by assessing daily sleep variability either subjectively or objectively (16). Subjective tests include self-report questionnaires such as the Pittsburgh Sleep Quality Index (PSQI) (17). The global PSQI score is a well-established index of subjective sleep quality that reliably estimates the risk for depressive symptoms in general adult populations (18). The test is widely used because it is inexpensive and easy to administer, but it does not yield objective sleep data. Such data can be obtained via polysomnography (PSG), this is a typical test that can systematically examine objective sleep quality, but PSG requires a lot of resources for testing (19, 20). There is a need for alternatives that measure sleep quality in a cost-effective manner.
Wearable devices (sometimes termed fitness trackers) monitor health signals and track behaviors such as sleep patterns when assessing long-term disease progression or treatment responses (21, 22). Such devices yield data on individual sleep macrostructures (20), but sensor accuracy and reliability, and the algorithm used, must be considered (21). Such devices do not estimate objective sleep quality as accurately as PSG (20, 22, 23). The devices are worn on the wrist and measure natural sleep patterns; the data quality is inevitably inferior to that of PSG performed in a sophisticated setting. However, the devices enable longitudinal tracking of individual sleep patterns, thus capturing day-to-day sleep variability that PSG does not measure.
To the best of our knowledge, few studies have used wearable devices to examine the association between the severity of depression/anxiety, and daily sleep efficiency, particularly in terms of intra-individual variability of sleep efficiency over a relatively short period. This is our topic here. Although young adulthood is the peak period of new-onset major depression (2), young adults rarely seek treatment because most are unaware of their symptoms (24). Young adults are thus the optimal population for an intensive longitudinal study of the association between daily sleep variability and depression.
Materials and methods
Participants
Data were collected from June 2018 to October 2020 from 104 undergraduate and graduate students (42 males and 62 females). All completed mental health screening during regular on-campus checkups. We emailed those at risk for depression (to ask them to volunteer for the study); the control (CON) group was enrolled via leaflet advertising. The inclusion criteria were age 18–35 years, no prior diagnosis of a psychotic or substance use disorder, no prior loss of consciousness caused by a severe head injury, and no psychotropic drug use within 8 weeks prior to enrollment. Diagnoses of major depressive disorder (MDD) or mild depressive symptoms (MDS) were made by licensed psychiatrists based on semi-structured MINI-International Neuropsychiatric Interviews. The classification criteria of the MDD and MDS participants have been reported as in previously published manuscripts (24, 25). The MDD and MDS participants met at least one of the following criteria: Patient Health Questionnaire-9 ≥ 10 points; General Anxiety Disease-7 ≥ 10 points; State-Trait Anxiety Inventory-State ≥ 61 points (for males) or ≥65 points (for females); a history of at least 1 suicidal thought/attempt/plan within the past 6 months. Furthermore, the MDD/MDS participants experienced one or both of the following symptoms: (1) a depressive mood or (2) loss of interest or pleasure in daily life over the last 2 weeks. The CON group lacked any prior or current psychiatric disorder. All participants provided written informed consent prior to participation. This study was approved by the Institutional Review Board of the Seoul National University College of Medicine and Hospital (Seoul, Republic of Korea; approval no. 1608–079–785) and conducted to the ethical standards of the 1964 Declaration of Helsinki and its later modifications.
Measures
Objective sleep-related variables were acquired daily through wearable devices, and demographic and clinical information were gathered using one-off self-report questionnaires. Thus, the objective sleep variables exhibit day-level data structures, and the demographic and clinical scores have person-level data structures.
Objective sleep-related indices
Objective sleep data were collected using wearable devices, Fitbit Charge 2 (Fitbit Inc.); these are wearable wristbands that track daily activity levels and sleep patterns (20). All participants installed the Fitbit app on their mobile phones and synced the app to Fitbits provided by the researchers. All participants were instructed to wear the Fitbits for 2 weeks and then return them. We extracted sleep efficiencies and times in bed.
Sleep efficiency (%)
This measure of sleep quality is calculated using a combination of participant movements and heart-rate patterns and is not the same as the sleep score of the mobile application (16, 26). Scores range from 0 to 100%; a score of at least 85% reflects good sleep quality (27).
Time in bed (min)
This included bed times when both asleep and awake (16, 26).
Clinical assessment
Patient Health Questionnaire-9
The Patient Health Questionnaire-9 (PHQ) (28, 29) screens for depression and its severity. A score of at least 10 generally indicates severe depression. Cronbach’s α for the 9 items was 0.85.
Generalized Anxiety Disorder-7
The Generalized Anxiety Disorder-7 (GAD) (30, 31) is a self-report instrument assessing anxiety; higher scores indicate greater anxiety. Cronbach’s α for the 7 items was 0.90.
World Health Organization quality of life abbreviated version
The World Health Organization Quality of Life Abbreviated Version (QOL) (32, 33) measures social relationships and physical and psychological health in the context of a cultural environment. A higher score indicates a better quality of life. The QOL features 26 items (α = 0.91); we used the total QOL score.
Barratt Impulsiveness Scale
The Barratt Impulsiveness Scale (BIS) (34, 35) assesses attentional, motor, and non-planning impulsiveness; greater scores indicate more impulsive behaviors and preferences. We used the BIS total 30-item score (α = 0.86).
NEO Five Factor Inventory
The NEO Five Factor Inventory (NEO) (36, 37) examines the Big Five personality traits (agreeableness, conscientiousness, extraversion, neuroticism, and openness to experience); we used the 60-item (shorter) inventory. There were 12 items in each of the agreeableness subscale (α = 0.68), the conscientiousness subscale (α = 0.81), the extraversion subscale (α = 0.81), the neuroticism subscale (α = 0.86), and the openness to experience subscale (α = 0.72).
Pittsburgh sleep quality index
The PSQI (17, 38) evaluates sleep quality over the past month; a global score of at least six indicates poor sleep quality (7 items; α = 0.61). We used the global PSQI score.
Covariates
We adjusted for sex, age, time in bed, the QOL, the NEO personality traits, and the BIS and PSQI scores; all are associated with depression or anxiety (39–44). Note that the “Time in Bed” index (“BED” in Table 1) served as a person-level variable during analysis, although it was measured daily. We calculated the individual mean scores because all other covariates and outcome variables were person-level variables.
Data analyses
Before analysis, we extracted day-level sleep data from json files and created the “day” variable as follows. It was a sequential number according to the “date of sleep” and “start time (of sleep)” log recorded for each sleep event per participant. Then, we merged the sleep data with the person-level demographic and clinical data. Note that the “day” variable does not have an equal time interval for each individual as well as across different individuals. However, unequal time intervals were not an issue for our analysis since we did not examine lagged effects such as the association between yesterday’s sleep efficiency on today’s. Also, we obtained descriptive statistics for all participants and the three groups. To examine the construct validity of the measures, random and one-way ANOVA, the chi-squared test, and correlation analysis were performed.
It is of our main interest that the association between intra-individual variability in sleep efficiency and depression/anxiety is investigated. For this purpose, a variability measure should be obtained for sleep efficiency. The majority of research on intra-individual variability in sleep patterns have calculated intra-individual standard deviation (iSD) as a variability measure, which is simply the standard deviation of the observed data for each person. Although simple and intuitively appealing, using iSD is not optimal for unbalanced data as ours, in which individuals have differing numbers of observations. The obtained iSD is less reliable for individuals with a smaller number of observations (45). To address this issue, we used dynamic structural equation modeling (DSEM) (46). DSEM can treat individual mean and individual variance (specifically, log-transformed individual variance) of daily measures as latent variables. This allows researchers to include these latent variables as a predictor or an outcome in a model with other variables, and thus to examine the relationship between the variables more reliably. In addition, unlike the two-step iSD approach (the iSD is first calculated and then the relationship between iSD and other variables is examined), DSEM proceeds in a single step.
Using DSEM we constructed a model in which the PHQ and GAD were outcomes, and each outcome was regressed on individual mean and (log-transformed) individual variance of daily sleep efficiency. The individual mean sleep efficiency was included as a predictor as well as the individual variance considering that individual mean and individual variance tend to be correlated although not completely overlapping (9). To control for the effects of covariates including sex, age, time in bed, the QOL, NEO personality traits, BIS, and PSQI, these variables were also included as predictors in the model.
Sleep data extraction, descriptive statistics, random and one-way ANOVA, chi-squared test, correlation analyses, and graphical representations were performed using R version 4.1.2 (47), and DSEM analysis employed Mplus version 8.7 (48). In ANOVA, chi-squared test, and correlation analyses, P-values < 0.05 were considered to indicate statistical significance. In DSEM, Bayesian estimation with MCMC was used and a posterior distribution was provided for each parameter. Therefore, we considered it statistically significant if the 95% credible interval for a parameter does not contain 0.
Results
Data from 100 participants were analyzed; we excluded data from two of the MDS and two of the MDD groups who considered issues with sensor wearing (recorded sleep efficiency less than 3 days) or were outliers (deviating three standard deviations from the mean sleep efficiency of all participants). The descriptive statistics, the random and one-way ANOVA, and chi-square test results are presented in Table 1.
One-way ANOVA was used to compare the MDD, MDS, and CON groups; the depression [F(2, 97) = 23.26, P < 0.001], anxiety [F(2, 97) = 15.36, P < 0.001], the QOL [F(2, 97) = 18.66, P < 0.001], conscientiousness [F(2, 97) = 3.17, P = 0.046], extraversion [F(2, 97) = 3.96, P = 0.022], and neuroticism [F(2, 97) = 13.59, P < 0.001] parameters differed significantly, as reported previously (25) (for the one-way ANOVA results only). Box plots of variables exhibiting significant group differences are shown in Figure 1.
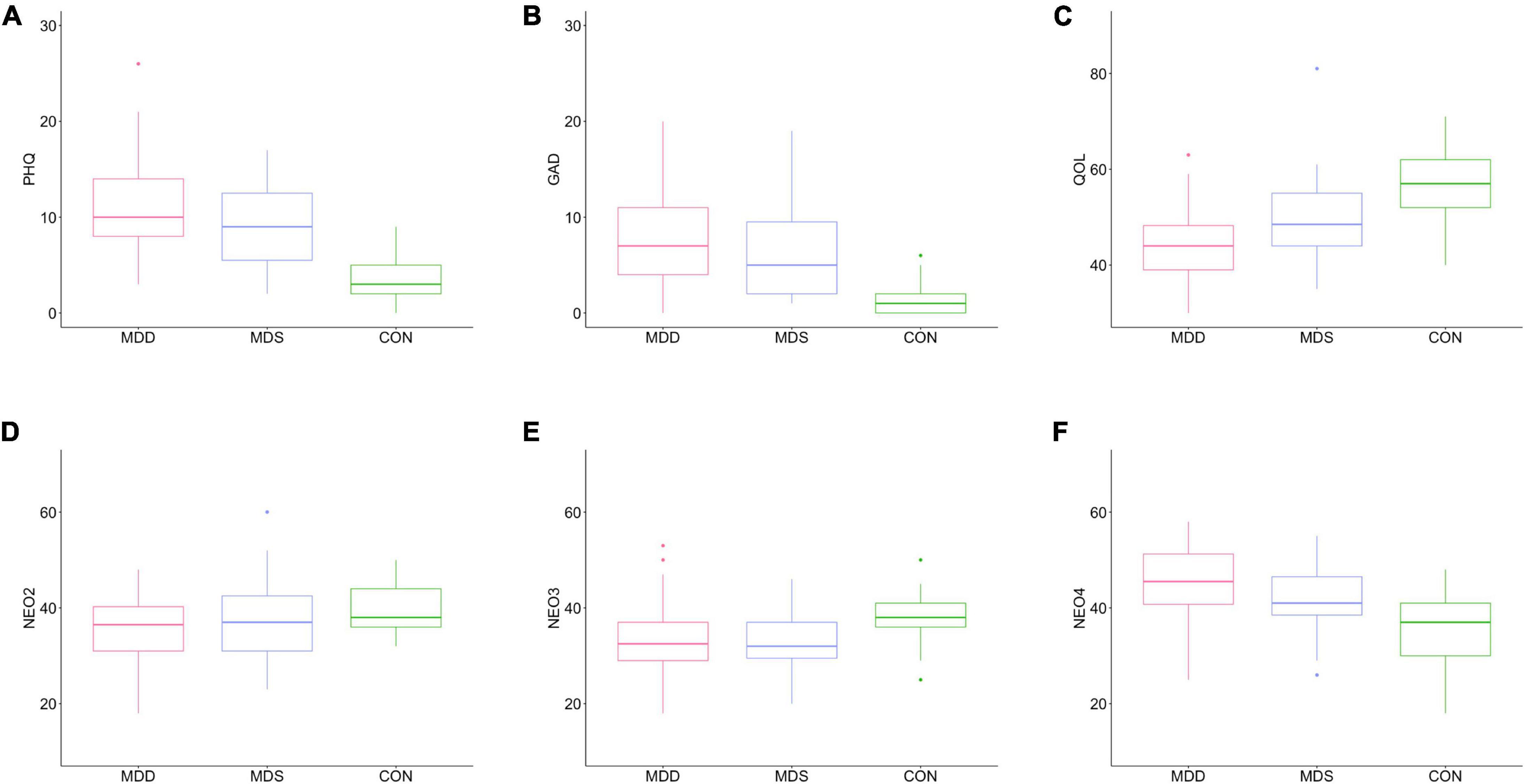
Figure 1. Box plots of significant among-group differences. (A) PHQ, (B) GAD, (C) QOL, (D) NEO2, (E) NEO3, and (F) NEO4. MDD, major depressive disorder; MDS, mild depressive symptoms; CON, control; PHQ, Patient Health Questionnaire-9; GAD, General Anxiety Disease-7; QOL, WHO quality of life; NEO2, NEO conscientiousness; NEO3, NEO extraversion; NEO4, NEO neuroticism.
The correlations between person-level variables are shown in Table 2. The PSQI revealed significant relationship with depression (r = 0.34, P < 0.001), anxiety (r = 0.33, P < 0.001), QOL (r = −0.33, P < 0.001), and neuroticism (r = 0.22, P = 0.025) scales. However, person-level time in bed and sleep efficiency indicators did not show a significant correlation with the aforementioned variables. Moreover, the association between PSQI, time in bed, and sleep efficiency was not significant.
Figure 2 is the trace plots of daily sleep efficiencies of three participants. These participants manifest slightly different levels of mean sleep efficiency. However, they show substantial difference in variability in sleep efficiency. The participant in the Figure 2A, shows greater “ups and downs” of sleep efficiency (around the mean) compared to those in the Figure 2B, which in turn shows more fluctuation in sleep efficiency than those shown in the Figure 2C.
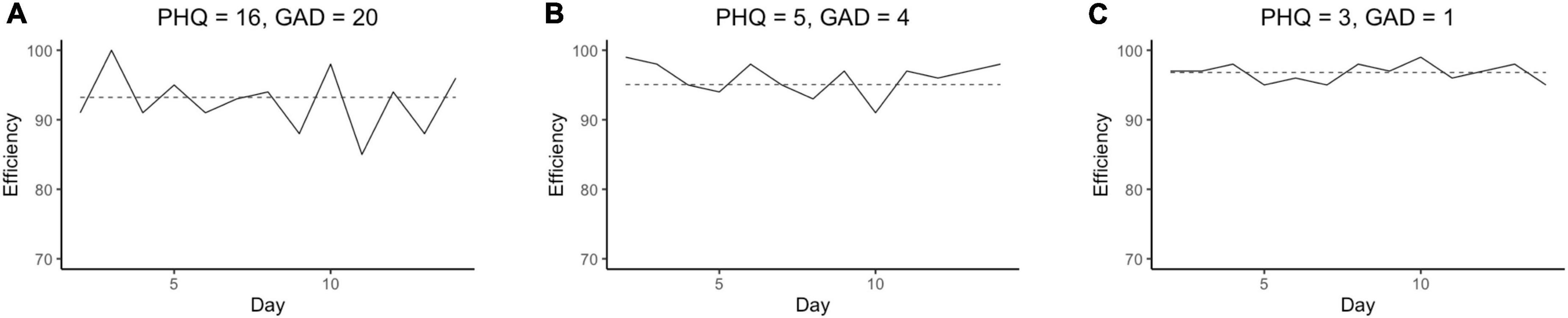
Figure 2. The trace plots for sleep efficiency of participant 23 in MDD group (A), participant 31 in MDS group (B), and participant 3 in CON group (C). Solid line represents each participant’s daily sleep efficiency and dashed line indicates average sleep efficiency for each participant. PHQ, Patient Health Questionnaire-9; GAD, General Anxiety Disease-7; MDD, major depressive disorder; MDS, mild depressive symptoms; CON, control.
Table 3 summarizes the results of the DSEM analysis. Greater intra-individual variability in daily sleep efficiency was associated with higher levels of depression (b = 2.526, 95% CI = [0.221, 5.084]) and anxiety (b = 3.696, 95% CI = [1.313, 6.292]). However, the mean daily sleep efficiency did not show a significant relationship with depression (b = 0.292, 95% CI = [−0.387, 1.061]) and anxiety (b = 0.618, 95% CI = [−0.072, 1.412]). These results suggest that fluctuation in night-to-night sleep efficiency may be more important in terms of the development of depression and anxiety, rather than the average level of sleep efficiency. Longer bedtime, lower QOL, less extraversion, and more neuroticism were significantly associated with higher levels of depression (for bedtime, b = 0.024, 95% CI = [0.008, 0.04]; for QOL, b = −0.114, 95% CI = [−0.224, −0.003]; for extraversion, b = −0.14, 95% CI = [−0.27, −0.008]; for neuroticism, b = 0.223, 95% CI = [0.11, 0.341]). Lower QOL and greater neuroticism were associated with higher anxiety (for QOL, b = −0.112, 95% CI = [−0.221, −0.002]; for neuroticism b = 0.314, 95% CI = [0.203, 0.423]).
Discussion
We explored whether variability in terms of daily sleep efficiency measured using wearable devices predicted depression and anxiety levels; we controlled for demographic and clinical characteristics. DSEM revealed that greater variability of daily sleep efficiency was associated with higher depression and anxiety levels than was average daily sleep efficiency, consistent with the findings of previous studies; night-to-night sleep fluctuations affect disease risk (9, 12, 13).
However, a study examined the relationship between intra-individual variability in daily subjective sleep quality and positive affect was found that people with higher fluctuations in daily sleep quality had more variability and higher mean positive affect than those who did not (49). These results are consistent with our study in terms of variability but are contrary to in terms of average. Therefore, in future studies, it is necessary to closely examine the associations between the objective and subjective aspects of the sleep quality and the level of depression and anxiety. Specifically, it is important to collect longitudinal sleep data in order to comprehensively consider the variability and mean values.
Wearable devices yield objective sleep measurements via real-time recording at minimal expense, and do not burden users (12); such devices facilitate the personalized and interactive healthcare (50). In this study, we used Fitbit Charge 2 as the wearable device and a previous study showed that sleep parameters measured with Fitbit devices were not statistically different from those measured with actigraphy (51); actigraphy has been used in chronobiology and sleep medicine for the past 20 years. However, some authors have questioned the validity of the raw data. In a previous experiment, sleep efficiency was high and varied only slightly (16); it was concluded that raw sleep efficiency data were not helpful. However, we found that raw sleep efficiency (daily variability) data predicted levels of depression and anxiety, although the sleep efficiencies of all groups were high. The daily (longitudinal) fluctuations in sleep efficiency revealed by wearable devices are valuable. Our research method can be easily utilized for sleep tracking to understand the temporal relationship between sleep efficiency routines and depression severity. Further, other sleep parameters such as sleep duration and times to fall asleep may be used to speculate associations with sleep routines and related health outcomes (52).
Furthermore, ANOVA revealed significant group differences in depression, anxiety, the subjective QOL, and personality traits; neither objective nor subjective sleep quality differed among the groups. Notably, the PSQI score exceeded 7 regardless of the severity of depression, indicating poor sleep quality. One study found that most American college students have poor sleep quality, explained principally by perceived stress (53); similar results were obtained in work on Portuguese college students in whom relationships were apparent among perceived stress, sleep difficulties, affect, and rumination (54). Thus, the high PSQI scores of our participants may reflect high stress rather than depression. Interestingly, PSQI was significantly correlated with PHQ, which looks incompatible with the non-significant group difference found in ANOVA. A further study is needed to investigate this issue, but we conjecture that classifying the MDD/MDS/CON groups yielded loss of information about individual difference in the severity of depression symptoms within groups, and it may have reduced the statistical power to detect the association between PSQI and depression (55).
Additionally, the objective sleep efficiency exceeded 90% for most participants, and it means sleep quality was good (27). The difference between subjective and objective sleep quality was apparent in correlation analysis. The PSQI score significantly correlated with depression, anxiety, the subjective QOL, and neuroticism but not sleep efficiency. Besides, we found a low correlation between PSQI and sleep efficiency. A non-significant association between subjective and objective sleep quality was found in previous studies (56, 57) in which psychological and physical discrepancies were also evident. Subjective sleep quality seems to be affected by psychological health (56); our DSEM finding that PSQI did not predict the PHQ or GAD score could be viewed as an extension of such results.
The significance of our findings is that depression and anxiety levels can be predicted by variability of daily sleep efficiency measured with wearable devices. This implies that the variability in sleep efficiency is an effective measure of individual’s mental health. Therefore, monitoring mental health using wearable devices in a cost-effective and interactive manner with focusing on variability in sleep quality across multiple days could help detect depression and anxiety in young adults.
Limitations
Our work had certain limitations. First, we explored whether variability in daily sleep efficiency and clinical characteristics predicted depression or anxiety but the depression, anxiety, and clinical scores were measured prior to assessment of daily sleep efficiency. Thus, we cannot infer a true temporal precedence between irregular daily sleep quality and depressive symptoms. A study on the temporal relationship between day-to-day variability in sleep efficiency and depression severity is required. Second, we collected only objective sleep data on a daily basis. A future study should collect both day-to-day clinical characteristics (such as mood state and subjective sleep quality) and objective sleep quality information. Third, all of our participants were undergraduate or graduate students, thus not representative sample of all young adults. A future study on young adults engaged in various occupations is necessary. Finally, some demographic factors were not collected. Residential status, body mass index, and physical condition may affect sleep quality.
Conclusion
In conclusion, young adults exhibiting greater daily variability in objective sleep efficiency may be at high risk for depression; longitudinal monitoring is required. Interventions should consider the magnitudes of day-to-day fluctuations in objective sleep quality and the self-reported psychological profiles.
Data availability statement
The original contributions presented in this study are included in the article/supplementary material, further inquiries can be directed to the corresponding authors.
Ethics statement
The studies involving human participants were reviewed and approved by Institutional Review Board of the Seoul National University College of Medicine and Hospital (Seoul, South Korea; approval no. 1608–079–785). The patients/participants provided their written informed consent to participate in this study.
Author contributions
J-AL: methodology, data analysis, and writing—original draft. J-YY and S-HC: recruit subjects, acquisition of data, and revision. SP: acquisition of data. HS: methodology, revision, editing, and supervision. JJ: project administration, conceptualization, funding acquisition, recruited subjects, and revision. All authors contributed to the article and approved the submitted version.
Funding
This research was supported by the Brain Research Program through the National Research Foundation of Korea (NRF) funded by the Ministry of Science and ICT (NRF-2016M3C7A1914449); and by the Institute for Hope Research at Sogang University.
Conflict of interest
The authors declare that the research was conducted in the absence of any commercial or financial relationships that could be construed as a potential conflict of interest.
Publisher’s note
All claims expressed in this article are solely those of the authors and do not necessarily represent those of their affiliated organizations, or those of the publisher, the editors and the reviewers. Any product that may be evaluated in this article, or claim that may be made by its manufacturer, is not guaranteed or endorsed by the publisher.
Abbreviations
PSG, polysomnography; MDD, major depressive disorder; MDS, mild depressive symptoms; CON, control; BED, time in bed; EFFI, sleep efficiency; MEAN EFFI, individual mean of daily sleep efficiency; VAR EFFI, log-transformed individual variance of daily sleep efficiency; PHQ, Patient Health Questionnaire-9; GAD, General Anxiety Disease-7; QOL, WHO quality of life; NEO1, NEO agreeableness; NEO2, NEO conscientiousness; NEO3, NEO extraversion; NEO4, NEO neuroticism; NEO5, NEO openness to experience; BIS, Barratt Impulsiveness Scale-11; PSQI, Pittsburgh Sleep Quality Index; iSD, intra-individual standard deviation; DSEM, dynamic structural equation modeling.
References
1. Akram U, Akram A, Gardani M, Ypsilanti A, McCarty K, Allen S, et al. The relationship between depression and insomnia symptoms amongst a sample of UK university students. Sleep Med Res. (2019) 10:49–53. doi: 10.17241/smr.2019.00332
2. Breslau N, Roth T, Rosenthal L, Andreski P. Sleep disturbance and psychiatric disorders: a longitudinal epidemiological study of young adults. Biol Psychiatry. (1996) 39:411–8. doi: 10.1016/0006-3223(95)00188-3
3. American Psychiatric Association.Diagnostic and Statistical Manual of Mental Disorders. Washington, DC: American Psychiatric Association (2013).
4. Alvaro PK, Roberts RM, Harris JK. A systematic review assessing bidirectionality between sleep disturbances, anxiety, and depression. Sleep. (2013) 36:1059–68. doi: 10.5665/sleep.2810
5. Fang H, Tu S, Sheng J, Shao A. Depression in sleep disturbance: a review on a bidirectional relationship, mechanisms and treatment. J Cell Mol Med. (2019) 23:2324–32. doi: 10.1111/jcmm.14170
6. Franzen PL, Buysse DJ. Sleep disturbances and depression: risk relationships for subsequent depression and therapeutic implications. Dialog Clin Neurosci. (2008) 10:473–81. doi: 10.31887/DCNS.2008.10.4/plfranzen
7. Perlis ML, Giles DE, Buysse DJ, Tu X, Kupfer DJ. Self-reported sleep disturbance as a prodromal symptom in recurrent depression. J Affect Disord. (1997) 42:209–12. doi: 10.1016/S0165-0327(96)01411-5
8. Murphy MJ, Peterson MJ. Sleep disturbances in depression. Sleep Med Clin. (2015) 10:17–23. doi: 10.1016/j.jsmc.2014.11.009
9. Bei B, Wiley JF, Trinder J, Manber R. Beyond the mean: a systematic review on the correlates of daily intraindividual variability of sleep/wake patterns. Sleep Med Rev. (2016) 28:108–24. doi: 10.1016/j.smrv.2015.06.003
10. Bei B, Seeman TE, Carroll JE, Wiley JF. Sleep and physiological dysregulation: a closer look at sleep intraindividual variability. Sleep. (2017) 40:zsx109. doi: 10.1093/sleep/zsx109
11. Fischer D, McHill AW, Sano A, Picard RW, Barger LK, Czeisler CA, et al. Irregular sleep and event schedules are associated with poorer self-reported well-being in us college students. Sleep. (2019) 43:zsz300. doi: 10.1093/sleep/zsz300
12. Fang Y, Forger DB, Frank E, Sen S, Goldstein C. Day-to-day variability in sleep parameters and depression risk: a prospective cohort study of training physicians. NPJ Digit Med. (2021) 4:28. doi: 10.1038/s41746-021-00400-z
13. Slavish DC, Taylor DJ, Lichstein KL. Intraindividual variability in sleep and comorbid medical and mental health conditions. Sleep. (2019) 42:zsz052. doi: 10.1093/sleep/zsz052
14. Baglioni C, Battagliese G, Feige B, Spiegelhalder K, Nissen C, Voderholzer U, et al. Insomnia as a predictor of depression: a meta-analytic evaluation of longitudinal epidemiological studies. J Affect Disord. (2011) 135:10–9. doi: 10.1016/j.jad.2011.01.011
15. Joo HJ, Kwon KA, Shin J, Park S, Jang S-I. Association between sleep quality and depressive symptoms. J Affect Disord. (2022) 310:258–65. doi: 10.1016/j.jad.2022.05.004
16. de Arriba-Pérez F, Caeiro-Rodríguez M, Santos-Gago JM. How do you sleep? Using off the shelf wrist wearables to estimate sleep quality, sleepiness level, chronotype and sleep regularity indicators. J Ambient Intell Hum Comput. (2018) 9:897–917. doi: 10.1007/s12652-017-0477-5
17. Carpenter JS, Andrykowski MA. Psychometric evaluation of the Pittsburgh sleep quality index. J Psychosom Res. (1998) 45:5–13. doi: 10.1016/S0022-3999(97)00298-5
18. Huang Y, Zhu M. Increased global Psqi score is associated with depressive symptoms in an adult population from the United States. Nat Sci Sleep. (2020) 12:487–95. doi: 10.2147/nss.S256625
19. Cook JD, Prairie ML, Plante DT. Utility of the fitbit flex to evaluate sleep in major depressive disorder: a comparison against polysomnography and wrist-worn actigraphy. J Affect Disord. (2017) 217:299–305. doi: 10.1016/j.jad.2017.04.030
20. de Zambotti M, Goldstone A, Claudatos S, Colrain IM, Baker FC. A Validation study of fitbit charge 2™ compared with polysomnography in adults. Chronobiol Int. (2018) 35:465–76. doi: 10.1080/07420528.2017.1413578
21. de Zambotti M, Godino JG, Baker FC, Cheung J, Patrick K, Colrain IM. The boom in wearable technology: cause for alarm or just what is needed to better understand sleep? Sleep. (2016) 39:1761–2. doi: 10.5665/sleep.6108
22. Stucky B, Clark I, Azza Y, Karlen W, Achermann P, Kleim B, et al. Validation of fitbit charge 2 sleep and heart rate estimates against polysomnographic measures in shift workers: naturalistic study. J Med Internet Res. (2021) 23:e26476. doi: 10.2196/26476
23. Haghayegh S, Khoshnevis S, Smolensky MH, Diller KR, Castriotta RJ. Accuracy of wristband fitbit models in assessing sleep: systematic review and meta-analysis. J Med Int Res. (2019) 21:e16273. doi: 10.2196/16273
24. Lim J-A, Yun J-Y, Choi Y, Choi S-H, Kwon Y, Lee HY, et al. Sex-specific differences in severity of depressive symptoms, heart rate variability, and neurocognitive profiles of depressed young adults: exploring characteristics for mild depression. Front Psychiatry. (2020) 11:217. doi: 10.3389/fpsyt.2020.00217
25. Choi Y, Choi S-H, Yun J-Y, Lim J-A, Kwon Y, Lee HY, et al. The relationship between levels of self-esteem and the development of depression in young adults with mild depressive symptoms. Medicine (Baltimore). (2019) 98:e17518. doi: 10.1097/md.0000000000017518
26. Fitbit developer. GetSleep Log by Date. (2022). Available online at: https://dev.fitbit.com/ (accessed July 20, 2022).
27. National Sleep Foundation. What Is Sleep Quality? (2022). Available online at: https://www.thensf.org/what-is-sleep-quality/ (accessed July 20, 2022).
28. Kroenke K, Spitzer RL. The Phq-9: a new depression diagnostic and severity measure. US: SLACK. Psychiatr Ann. (2002) 32:509–15. doi: 10.3928/0048-5713-20020901-06
29. Park S-J, Choi H-R, Choi J-H, Kim K-W, Hong J-P. Reliability and validity of the korean version of the patient health questionnaire-9 (Phq-9). Anxiety Mood. (2010) 6:119–24.
30. Spitzer RL, Kroenke K, Williams JBW, Löwe BA. Brief measure for assessing generalized anxiety disorder: the Gad-7. Arch Intern Med. (2006) 166:1092–7. doi: 10.1001/archinte.166.10.1092
31. Seo J-G, Cho YW, Lee S-J, Lee J-J, Kim J-E, Moon H-J, et al. Validation of the generalized anxiety disorder-7 in people with epilepsy: a Mepsy study. Epilepsy Behav. (2014) 35:59–63. doi: 10.1016/j.yebeh.2014.04.005
32. The WHOQOL Group. Development of the world health organization WHOQOL-bref quality of life assessment. Psychol Med. (1998) 28:551–8. doi: 10.1017/S0033291798006667
33. Min SK, Kim KI, Lee CI, Jung YC, Suh SY, Kim DK. Development of the Korean versions of who quality of life scale and WHOQOL-bref. Qual Life Res. (2002) 11:593–600. doi: 10.1023/A:1016351406336
34. Patton JH, Stanford MS, Barratt ES. Factor structure of the barratt impulsiveness scale. J Clin Psychol. (1995) 51:768–74. doi: 10.1002/1097-4679(199511)51:63.0.CO;2-1
35. Lee S-R, Lee W-H, Park J-S, Kim S-M, Kim J-W, Shim J-H. The study on reliability and validity of Korean version of the barratt impulsiveness scale-11-revised in nonclinical adult subjects. J Korean Neuropsychiatr Assoc. (2012) 51:378–86. doi: 10.0000/jkna.2012.51.6.378
36. Costa PTJr, McCrae RR. Revised Neo Personality Inventory (Neo-Pi-R) and Neo Five-Factor (Neo-Ffi) Inventory Professional Manual. Odessa, FL: Psychological Assessment Resources (1992).
37. Ahn C-K, Chae J-H. Standardization of the Korean version of the revised neo personality inventory. Korean J Couns Psychother. (1997) 9:443–72.
38. Sohn SI, Kim DH, Lee MY, Cho YW. The reliability and validity of the Korean version of the Pittsburgh sleep quality index. Sleep Breath. (2012) 16:803–12. doi: 10.1007/s11325-011-0579-9
39. Demyttenaere K. Quality of life in depression and anxiety: does it matter? Int J Psychiatry Clin Pract. (2006) 10:27–30. doi: 10.1080/13651500600552511
40. Karsten J, Penninx BWJH, Riese H, Ormel J, Nolen WA, Hartman CA. The state effect of depressive and anxiety disorders on big five personality traits. J Psychiatr Res. (2012) 46:644–50. doi: 10.1016/j.jpsychires.2012.01.024
41. Markarian SA, Pickett SM, Deveson DF, Kanona BB. A model of bis/bas sensitivity, emotion regulation difficulties, and depression, anxiety, and stress symptoms in relation to sleep quality. Psychiatry Res. (2013) 210:281–6. doi: 10.1016/j.psychres.2013.06.004
42. Moustafa AA, Tindle R, Frydecka D, Misiak B. Impulsivity and its relationship with anxiety, depression and stress. Compr Psychiatry. (2017) 74:173–9. doi: 10.1016/j.comppsych.2017.01.013
43. Sullivan K, Ordiah C. Association of mildly insufficient sleep with symptoms of anxiety and depression. Neurol Psychiatry Brain Res. (2018) 30:1–4. doi: 10.1016/j.npbr.2018.03.001
44. Thompson KN, Hübel C, Cheesman R, Adey BN, Armour C, Davies MR, et al. Age and sex-related variability in the presentation of generalized anxiety and depression symptoms. Depress Anxiety. (2021) 38:1054–65. doi: 10.1002/da.23213
45. Du H, Wang L. Reliabilities of intraindividual variability indicators with autocorrelated longitudinal data: implications for longitudinal study designs. Multivariate Behav Res. (2018) 53:502–20. doi: 10.1080/00273171.2018.1457939
46. Asparouhov T, Hamaker EL, Muthén B. Dynamic structural equation models. Struct Equ Model Multidiscip J. (2018) 25:359–88. doi: 10.1080/10705511.2017.1406803
47. R Core Team.R: A Language and Environment for Statistical Computing. Vienna: R Foundation for Statistical Computing (2021).
49. Ying F, Wen JH, Klaiber P, DeLongis A, Slavish DC, Sin NL. Associations between intraindividual variability in sleep and daily positive affect. Affect Sci. (2022) 3:330–40. doi: 10.1007/s42761-021-00082-6
50. Lee S, Kim H, Park MJ, Jeon HJ. Current advances in wearable devices and their sensors in patients with depression. Front Psychiatry. (2021) 12:672347. doi: 10.3389/fpsyt.2021.672347
51. Lee HA, Lee HJ, Moon JH, Lee T, Kim MG, In H, et al. Comparison of wearable activity tracker with actigraphy for sleep evaluation and circadian rest-activity rhythm measurement in healthy young adults. Psychiatry Investig. (2017) 14:179–85. doi: 10.4306/pi.2017.14.2.179
52. Robbins R, Affouf M, Seixas A, Beaugris L, Avirappattu G, Jean-Louis G. Four-year trends in sleep duration and quality: a longitudinal study using data from a commercially available sleep tracker. J Med Internet Res. (2020) 22:e14735.
53. Lund HG, Reider BD, Whiting AB, Prichard JR. Sleep patterns and predictors of disturbed sleep in a large population of college students. J Adolesc Health. (2010) 46:124–32. doi: 10.1016/j.jadohealth.2009.06.016
54. Amaral AP, Soares MJ, Pinto AM, Pereira AT, Madeira N, Bos SC, et al. Sleep difficulties in college students: the role of stress, affect and cognitive processes. Psychiatry Res. (2018) 260:331–7. doi: 10.1016/j.psychres.2017.11.072
55. Maxwell SE, Delaney HD. Bivariate median splits and spurious statistical significance. Psychol Bull. (1993) 113:181–90. doi: 10.1037/0033-2909.113.1.181
56. Allen SF, Elder GJ, Longstaff LF, Gotts ZM, Sharman R, Akram U, et al. Exploration of potential objective and subjective daily indicators of sleep health in normal sleepers. Nat Sci Sleep. (2018) 10:303–12. doi: 10.2147/nss.S168841
Keywords: sleep efficiency, variability, depression, wearable device, dynamic structural equation models
Citation: Lim J-A, Yun J-Y, Choi S-H, Park S, Suk HW and Jang JH (2022) Greater variability in daily sleep efficiency predicts depression and anxiety in young adults: Estimation of depression severity using the two-week sleep quality records of wearable devices. Front. Psychiatry 13:1041747. doi: 10.3389/fpsyt.2022.1041747
Received: 11 September 2022; Accepted: 21 October 2022;
Published: 07 November 2022.
Edited by:
Qingzhong Wang, Shanghai University of Traditional Chinese Medicine, ChinaReviewed by:
Seog Ju Kim, Sungkyunkwan University, South KoreaMyung Hun Jung, Hallym University Sacred Heart Hospital, South Korea
Copyright © 2022 Lim, Yun, Choi, Park, Suk and Jang. This is an open-access article distributed under the terms of the Creative Commons Attribution License (CC BY). The use, distribution or reproduction in other forums is permitted, provided the original author(s) and the copyright owner(s) are credited and that the original publication in this journal is cited, in accordance with accepted academic practice. No use, distribution or reproduction is permitted which does not comply with these terms.
*Correspondence: Joon Hwan Jang, amhqYW5nNjAyQHNudS5hYy5rcg==; Hye Won Suk, aHN1azJAc29nYW5nLmFjLmty