- Department of Forensic Psychiatry, University Hospital of Psychiatry, University of Zurich, Zurich, Switzerland
The importance of “social capital” in offender rehabilitation has been well established: Stable family and community relationships offer practical assistance in the resettlement process after being released from custody and can serve as motivation for building a new sense of self off the criminal past, thus reducing the risk of re-offending. This also applies to offenders with severe mental disorders. The aim of this study was to identify factors that promote or hinder the establishment or maintenance of social relationships upon release from a court-ordered inpatient treatment using a modern statistical method—machine learning (ML)—on a dataset of 369 offenders with schizophrenia spectrum disorder (SSD). With an AUC of 0.73, support vector machines (SVM) outperformed all the other ML algorithms. The following factors were identified as most important for the outcome in respect of a successful re-integration into society: Social integration and living situation prior to the hospitalization, a low risk of re-offending at time of discharge from the institution, insight in the wrongfulness of the offense as well as into the underlying psychiatric illness and need for treatment, addressing future perspectives in psychotherapy, the improvement of antisocial behavior during treatment as well as a detention period of less than 1 year emerged as the most predictive out of over 500 variables in distinguishing patients who had a social network after discharge from those who did not. Surprisingly, neither severity and type of offense nor severity of the psychiatric illness proved to affect whether the patient had social contacts upon discharge or not. The fact that the majority of determinants which promote the maintenance of social contacts can be influenced by therapeutic interventions emphasizes the importance of the rehabilitative approach in forensic-psychiatric therapy.
Introduction
Stable family relationships and community ties are known to have great potential in the prevention of re-offending among former inmates (1–3). In criminology, this potentially protective factor is also referred to as “social capital” (2–4). Broadly speaking, social capital can be defined as a person’s individual capacity to call upon personal ties and social networks in order to advance some personal interest and can provide former inmates with resources beneficial to their reintegration (e.g., employment, financial and emotional support, housing) (3, 5). Apart from the benefits of a social network regarding the individual quality of life, friends and family members may also serve as some sort of non-professional social control beyond the professional network, thus shaping behavior: A sufficient, high-quality social network improves treatment adherence and may reduce the risk of violating probation (6). In turn, involvement in a criminogenic peer group as well as social isolation elevate the risk for probation failures and violent behavior (7–9). Naturally, these considerations are not only important concerning the reintegration of offenders being discharged from the penal system but also for offender patients with psychiatric illnesses being discharged from institutional court mandated therapy. In fact, connectedness with others has been indicated as an important factor in personal recovery in various international forensic psychiatric populations as well as in a recent Swiss explorative study (10–12). With risk management being the core competence of the forensic psychiatrist, maintaining and promoting resources beneficial to the prevention of re-offending and to mental stabilization need to be targeted during the therapeutic process. However, offenders with mental illnesses often struggle to maintain social contacts through their inpatient treatment, or even to build one prior to the offense in the first place (10). The reasons for this are manifold: Relatives and other social contacts have to deal with both mental health issues and juridical problems and the consecutive stigma, they may be or have been the target of the patients’ aggressive behavior and may be subjected to ambiguous feelings regarding the patient (e.g., grief, disbelief, anger, guilt, shame) (13–15). Since their ability to establish and maintain social contacts is oftentimes critically impaired as a result of their illness, offender patients with mental disorders are all the more in need of therapeutic support to activate these resources during their treatment. Recently, several interventions to promote recovery in the sense of establishing a social network have been discussed and applied. An example for such a strength- and resource-based approach in forensic psychiatric therapy is the Good Lives Model, which focuses on building interpersonal skills and social networks in order to facilitate change in criminal behavior (16–18). However, due to the heterogeneity of forensic psychiatric patients, therapists need to be aware not only of the general factors that may impede social rehabilitation, but also of the individual parameters.
The present study aims to determine the most predictive factors of social capital upon discharge from court mandated inpatient treatment, based on a unique group of forensic offenders with schizophrenia spectrum disorder (SSD). To the authors’ knowledge, this is the first scientific evaluation of social capital in such a large homogenous population of offender patients with SSD.
Materials and methods
The files of 370 delinquent patients diagnosed with SSD according to ICD-9 (295.x) (19) and ICD-10 (F20–29.x) (20), who were admitted to the Center for Inpatient Forensic Therapies of the University Hospital of Psychiatry Zurich for court mandated therapy, were assessed retrospectively. The case files consisted of professionally documented anamneses, psychiatric/psychologic inpatient and outpatient reports, police reports, testimonies, court proceedings, reports from social workers, and biannual extensive reports from clinicians as well as the nursing and care staff. Due to extensivity of the files and the high medical and legal importance assigned to cases of forensic patients in Switzerland, it can be assumed that the files contained all relevant information on the health and biography of a patient. A trained independent physician with + 5 years of forensic psychiatric experience systematically reviewed all case files and conducted a directed qualitative content analysis (21). A second trained independent rater encoded a random subsample of 10% of cases to assess inter-rater reliability. Cohen’s Kappa was 0.78, which can be regarded as substantial (22). The content analysis was performed according to a previously carefully designed questionnaire and rating protocol for coding based on a set of criteria originally proposed by Seifert (23–25). Before being put to use, questionnaire and extraction protocol design were repeatedly discussed in inter- and supervisions with senior researchers in forensic psychiatry from the researchers’ institution as well as other, international forensic psychiatric institutions.
The comprehensive dataset included items from the following domains: social-demographic data, childhood/youth experiences, psychiatric history, past criminal history, social/sexual functioning, details on the offense leading to forensic hospitalization, prison data, and particularities of the current hospitalization and psycho-pathological symptoms. The latter was defined by an adapted positive and negative syndrome scale (PANSS), whereby symptoms were divided into the usual 30 sub-categories and then rated on a three-tier scale instead of a seven-tier one (completely absent, discretely present, or substantially present). Social capital was defined as having at least one of the following social contacts: family ties, spousal relationships, a circle of friends of more than one person, and membership in a club. The dataset has already been evaluated in other studies as part of a larger, ongoing project with the goal of gaining further knowledge about the complex field of offender patients with SSD (7, 9, 26–35). Although the same database provides the basis for several analyses covering a wide range of objectives in this research area, and although there are a few overlapping parameters, it still contains a substantial number of unique variables, thus resulting in different theoretical and practical conclusions and implications. An overview of the basic characteristics of the population is provided in Table 1. Further details on data collection regarding our population can be found in Lau et al. (32).
Parts of the following section were published beforehand in a study by Kirchebner et al. (30) and, as the same methodology was applied, are partly replicated here. For further information regarding data collection and processing, please refer to previous publications (30, 36). Due to the explorative nature of this study, supervised ML appeared to be the most suitable approach in identifying the most relevant predictive factors out of a large number of parameters and to determine the model providing the best predictive power. An overview of the statistical steps is shown in Figure 1 and is further described in detail below. All the steps were performed using R version 3.6.3. (R Project, Vienna, Austria) and the MLR package v2.171 (Bischl, Munich, Germany). CI calculations of the balanced accuracy were conducted using MATLAB R2019a (MATLAB and Statistics Toolbox Release 2012, The MathWorks, Inc., Natick, Massachusetts, United States) with the add-on “computing the posterior balanced accuracy” v1.0. All raw data were first processed for machine learning (see Figure 1, Step 1): Several categorical variables were converted to binary code, while continuous and ordinal variables were not adjusted. Due to the retrospective nature of the study and the large number of variables included, there were missing values among variables. This especially applied to information on the broader biographical history of patients, although forensic records were comprehensive. Variables with more than 33% missing values were eliminated, leaving a set of 508 variables. The outcome variable “social network upon discharge from the institution” was dichotomized into (a) “present” and (b) “not present.” Having social network referred to either having family or spouses, having more than one friend or being member of a social club. As there was missing data regarding their social network upon discharge in one case, leading to exclusion, a total of 369 patients remained. Out of all 369 patients, only 140 (37.9%) had some kind of social network upon their discharge, while 229 (62.1%) did not (see Table 1).
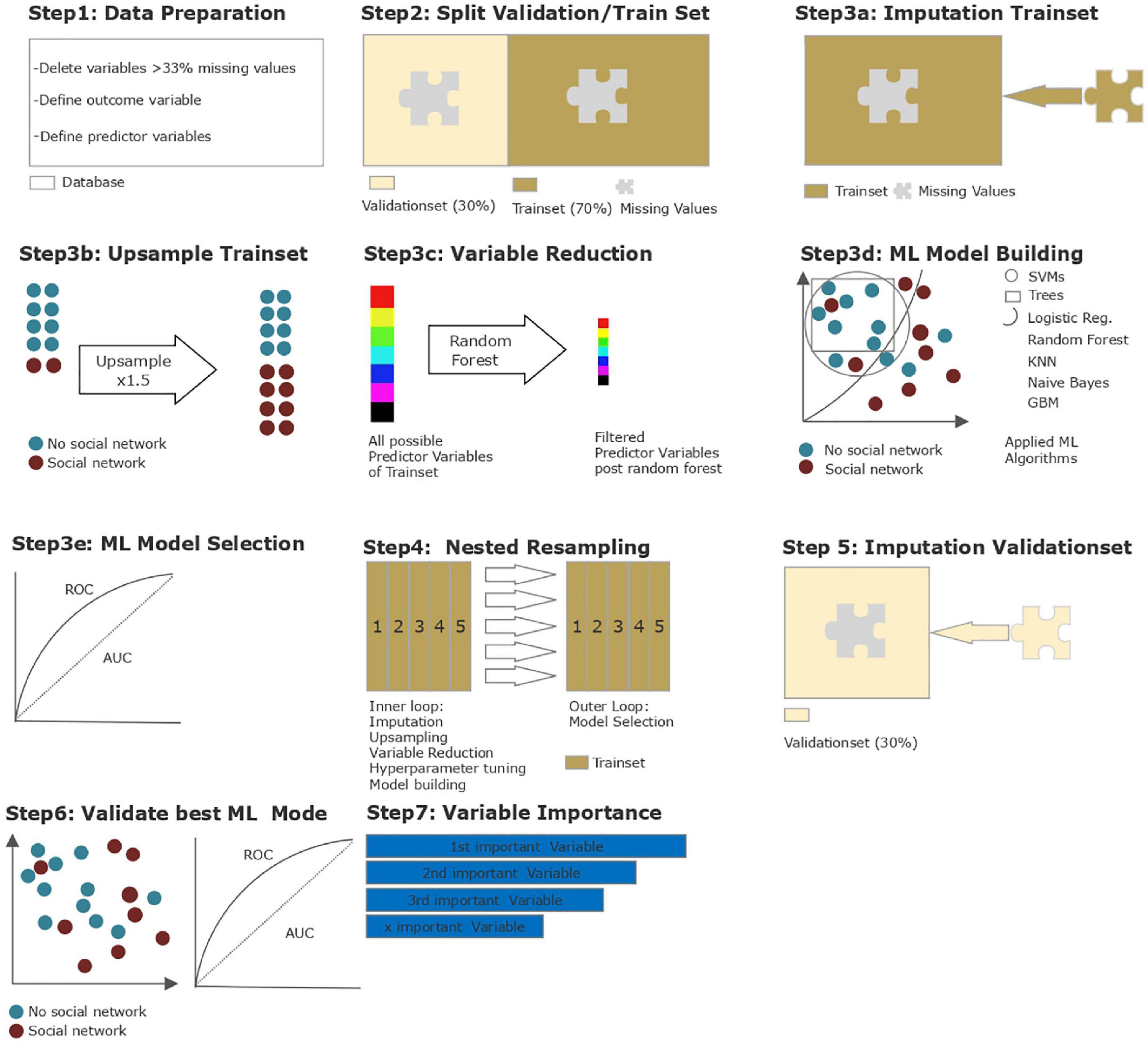
Figure 1. Step 1—Data preparation: Multiple categorical variables were converted to binary code. Continuous and ordinal variables were not manipulated. Outcome variable violent behavior/no violent behavior and 507 predictor variables were defined. Step 2—Datasplitting: Split into 70% training dataset and 30% validation dataset. Step 3 a, b, c, d, e–Model building and testing on training data I: Imputation by mean/mode; upsampling of outcome “social network” x1.5; variable reduction via random forest; model building via ML algorithms—logistic regression, trees, random forest, gradient boosting, KNN (k-nearest neighbor), support vector machines (SVM), and naive bayes; testing (selection) of best ML algorithm via ROC parameters. Step 4—Model building and testing on training data II: Nested resampling with imputation, upsampling, variable reduction and model building in inner loop and model testing on outer loop. Step 5–Model building and testing on validation data I: Imputation with stored weights from Step 3a and upsampling of outcome “social network” x2. Step6—Model building and testing on validation data II: Best model identified in Step 3e applied on imputed and balanced validation dataset and evaluated via ROC parameters. Step 7—Test for multicollinearity and ranking of variables by indicative power.
After data preparation, the database was divided into one training and one validation subset (see Figure 1, Step 2). The training subset, including 70% of all cases (n = 258), was used for variable reduction and model building/selection. To enable the flexible application of all ML algorithms, imputation of missing values was carried out and imputation weights saved for later were reused on the validation subset (see Figure 1, Step 3a). As the outcome variable was unevenly distributed, a random up-sampling at a rate of 1.5 was conducted, leading to a more balanced outcome (see Figure 1, Step 3b). A major objective of the present study was to identify the most important predictor variables from 508 possible variables. A decrease in variables can also counteract overfitting while maintaining computing times in initial model building at an acceptable level. Thus, we performed a variable reduction through random-ForestSRC down to the point where the AUC did improve by no more than 5% through adding another item (see Figure 1, Step 3c). This led to a variable reduction down to the 8 most important predictors. As the database was relatively small for ML purposes and our focus lay on variable extraction and prediction, we applied discriminative model building with logistic regression, trees, random forest, gradient boosting, KNN (k-nearest neighbor), support vector machines (SVM), and as an easily applicable generative model building, naive Bayes (see Figure 1, Step 3d). No hyperparameters were optimized. For each model, performance was calculated and assessed in terms of its balanced accuracy (the average of true positive and true negative rate, better suited for model evaluation and calculation of confidence intervals in imbalanced data) and goodness of fit (measured with the receiver operating characteristic, balanced curve area under the curve method, ROC balanced AUC). Specificity, sensitivity, positive predictive value (PPV), and negative predictive value (NPV) were also evaluated. As our training dataset was artificially balanced, the model with the highest AUC was chosen for final model validation with the validation subset (see Figure 1, Step 3e). To avoid dependencies between the variables, we tested the set of identified variables for multicollinearity. Finally, to prevent overfitting, a nested resampling approach was employed. For this purpose, we used a nested resampling model with the inner loop performing imputation, oversampling, variable filtration, and model building within 5-fold cross-validation, and the outer loop for performance evaluation also embedded in 5-fold cross-validation—a technique for artificially creating different subsamples of a dataset (see Figure 1, Step 4). To evaluate the model selected before, the validation subset with 30% of all cases (n = 111) was applied (see Figure 1, Steps 5–7). As briefly mentioned above, the previously stored imputation weights were reused on the validation subset (see Figure 1, Step 5). Then the selected model was applied for validation (see Figure 1, Step 6). The identified variables were finally tested for multicollinearity and ranked according to their indicative power (see Figure 1, Step 7).
Results
Model calculation
An overview of the performance parameters of the different calculated algorithms during the nested resampling procedure can be found in Table 2. With a balanced accuracy of 70% and an AUC of 0.77, the support vector machines algorithm (SVM) outperformed all the other ML algorithms. The absolute and relative distribution of the 8 most predictive variables identified during nested resampling and used for the model buildings are shown in Table 3. As described above, the model did not improve by adding another item. The quality of the final model in the validation step is provided in Table 4. As expected, the balanced accuracy of 64.7 and the AUC of 0.73 were lower than the results of the initial training model, but they were still meaningful. With a sensitivity of 50.9% and a specificity of 72.5%, patients holding some form of social capital upon discharge were identified correctly in half the cases, while three-fourths of cases were identified correctly as having no social capital (see Table 4).
Predictors of a social network upon discharge
The distribution of the importance of variables of the final validation model is presented in Figure 2 as a one-sided tornado graph. Social isolation at time of the offense committed, a time spent in prison for more than a year and a doubtful or unfavorable legal prognosis emerged as most indicative factors for having no social network upon discharge, while insight into the illness and treatment, developing insight into the wrongfulness of the committed offense during treatment, a sufficient or favorable legal prognosis, an improvement of antisocial behavior, and living together with parents/mother/father at the time of the offense were most indicative for having a social network upon discharge. Another predictive factor that proved beneficial in the presence of a social network upon discharge was a psychotherapeutic focus on future perspectives.
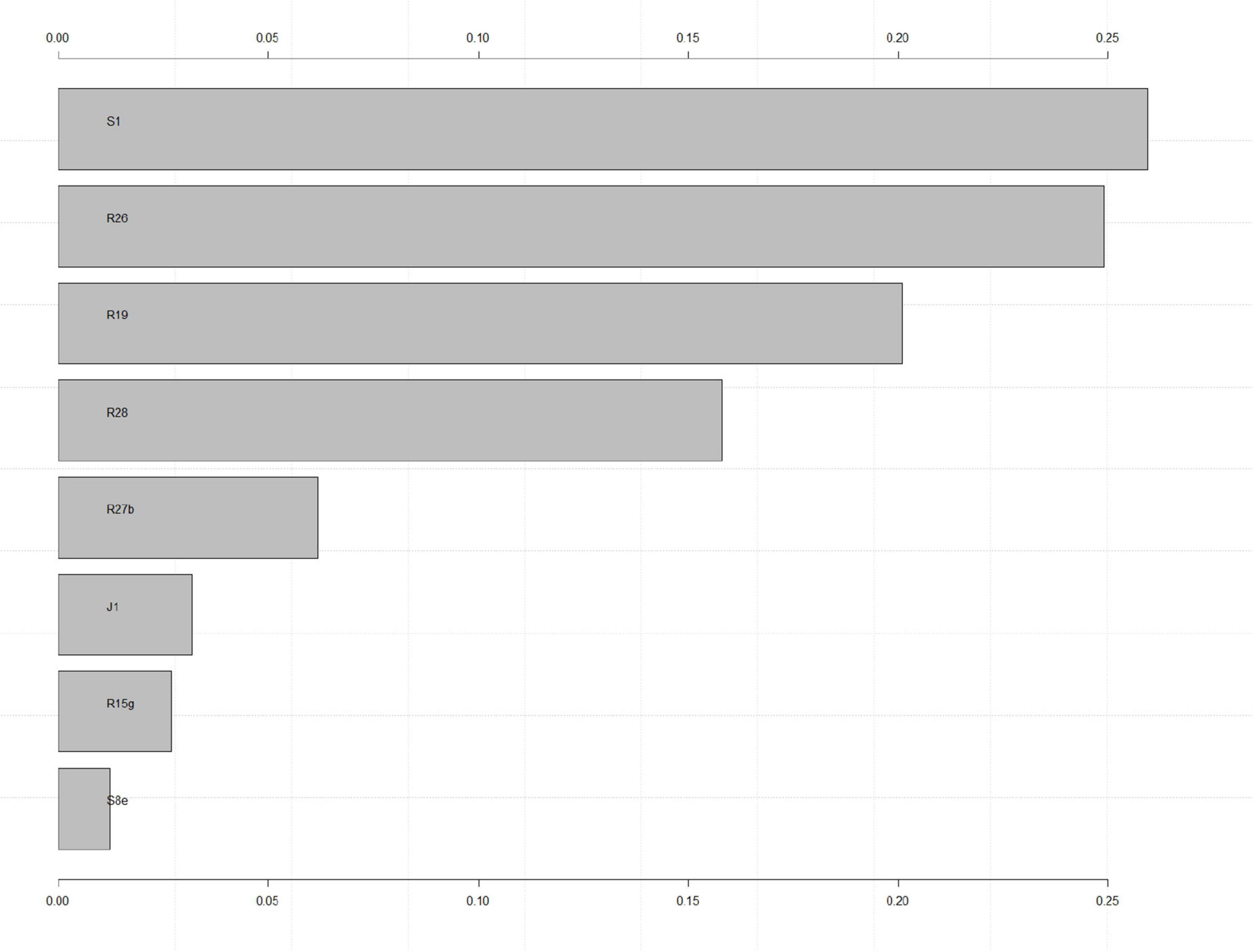
Figure 2. S1, social isolation at time of offense; R26, insight into illness and its treatment; R19, insight in wrongfulness of offense during current hospitalization; R28, assigned legal prognosis at discharge; R27b, improvement of antisocial behavior during treatment; R15g, main content of psychotherapy: future perspectives; J1, time spent in prison >1 year; S8e, Living situation at time of offense: at parents/mother/father.
Discussion
The aim of this study was to determine the factors that distinguish between offender patients with SSD who had some form of social capital upon discharge from court mandated inpatient treatment from those who did not. The goal was to use an explorative approach to identify the most predictive factors in social contacts and community ties upon discharge. In order to do so, we applied ML algorithms to a large database consisting of 369 patients and were thus able to create an appropriate model. With a balanced accuracy of 65% and an AUC of 0.73, the SVM model could correctly identify patients without social capital upon their discharge in three-quarters the cases. Variables related mostly to life conditions before the patients’ detention, the decline in socially intolerant behavior due to either illness or antisocial behavior as well as to the estimated risk of re-offending after discharge. A longer duration of imprisonment (>1 year) was also identified as negative predictor of social ties upon discharge. This seems obvious: Establishing a social network is already difficult for patients with SSD due to their symptomatology, and is further exacerbated by institutionalization, which is usually accompanied, at least initially, by strict regulation of access to the outside world (including digital communication such as via social media). It seems therefore understandable, that the formation of community ties is severely impaired due to their often year-long hospitalization. This is in line with findings regarding offenders without mental disorders who have been subjected to long imprisonment (37–39). It appears that despite forensic-psychiatric treatment focusing on rehabilitation, it failed to establish a social network in those cases. This fact raises even more concern as it has been suggested that social contacts and support may in turn contribute to a shortened duration of the institutionalization in forensic psychiatric populations (40). However, the findings on that matter are yet contradictory and, in a recent Swiss study, lack of social contacts has not been confirmed as predictor for a longer duration of stay in the specific group of offender patients with SSD (29, 41). Two overlapping static predictors of social capital were general social isolation at the time of the offense, which was associated with a lack of social contacts upon discharge in three-fourths of cases, and living situation at the time of the offense: Patients who had been living together with both or one parent prior to their institutionalization could rely on some form of social network upon discharge at least in around one-third of cases. As the social network upon discharge comprised mostly of family members rather than friends, spouses or communal ties (see Table 1), it seems understandable that a previously existing family relationship made it more likely for patients to reintegrate into after their discharge from the institution. This emphasizes the importance of integrating and involving relatives in the therapeutic process: In doing so, clinicians and therapists can help reduce the double stigma of patients and their families (mental illness and criminal offense/internment measure), empower family systems through active participation and improved psychoeducational knowledge transfer, and create transparency in the therapeutic process. Research on the need and burdens of relatives of mentally ill offenders is still limited, but the few existing findings clearly indicate family members’ need for participation (13, 42). In line with the scarcity of research in this area, there are hardly any established, operationalized interventions for the involvement of relatives in forensic psychiatric therapy. Nevertheless, a turnaround has become apparent in the last few years, which is mainly driven by family members’ initiatives and projects (43, 44).
Further predictors related primarily to the patients’ development during the therapeutic process: Insight into the illness and its treatment and the improvement of antisocial behavior during treatment emerged as highly relevant predictors for having social capital upon discharge. This could be well expected, as both symptomatology of SSD as well as antisocial behavior and attitudes impair social compatibility. If it is assumed that a certain degree of antisociality is also an expression of SSD in offender patients in the sense of a loss of the set of values and norms, there is also a high degree of interdependence between the two items and by targeting the former through therapeutic interventions, the latter is positively influenced as well. On the other hand, the possibility of comorbid antisocial behavior patterns in patients with SSD, especially those with involvement in the judicial system, has been discussed as well (45). Either way, these findings highlight the importance of targeting both the underlying SSD through psychoeducational measures as well as antisocial attitudes and behavior, e.g., through group therapy programs on social skills as well as standardized treatment programs such as the cognitive-behavioral Reasoning and Rehabilitation Programme, which was originally developed for use in the correctional system, but has been further developed for the rehabilitation of offenders with mental illness (46). Furthermore, insight into the illness and its treatment forms the basis for many other factors that positively influence the further course: insight into the need for treatment correlates with treatment adherence, which in turn positively influences clinical outcome regarding current symptomatology as well as psychotic relapses and chronification, hospitalization rates and re-offending rates (47–51).
Another dynamic factor predicting the presence of social capital upon discharge was the patients’ insight into wrongfulness of the offense. This result could be interpreted in two ways: It may be possible that patients who are socially connected are more often confronted by their relatives and network regarding the offense leading to the institutionalization. This may be especially the case for patients whose victims belong to their social network, e.g., family members. Another plausible explanation for this finding is that patients who have the capacity to develop and maintain social capital are in general healthier and less impaired by their underlying mental illness than their socially isolated counterparts, and that those patients are therefore also more capable of actually reflecting on their behavior and past actions. It is also possible that, while having actually little meaningfulness when it comes to re-delinquency, a previously existing social network may be more willing to keep in contact with offender patients if they critically examine and reflect upon their actions, as it is often demanded by the public, the media and society in general (52).
The legal prognosis assigned at release, i.e., the risk of re-offending, also played a role in the development and/or maintenance of social contacts: Patients with a rather unfavorable or doubtful legal prognosis could rely on a social network upon discharge less often than patients with a lower risk of re-offending. It could be hypothesized that the risk of re-offending is not so much causal for the existence of a social network at the time of discharge, but rather that it is the other way around: Clinicians and forensic psychiatric experts evaluate the risk of re-offending constantly during the course of the institutionalization as well as prior to the patients’ release, based on various established risk assessment tools, which often include the presence of social contacts as a protective factor (e.g., SAPROF) (53, 54). In fact, successful cooperation with relatives or other social contacts during the therapeutic process is associated with lower rates of criminal recidivism (55). Just as with insight into wrongfulness of the offense, another explanation for this finding may very well be that healthier patients are labeled as less likely to re-offend and are also more capable of maintaining social contacts.
Finally, patients were more likely to have some sort of social capital if the main psychotherapeutic content was future perspectives. Yet, this item did not exclusively refer to the current or future social network, but could include ideas on housing, occupational and financial perspectives as well. This fits well with findings from non-offending patients with SSD highlighting the importance of an encouraging psychotherapy as part of the recovery process (56). However, to the knowledge of the authors, the content of psychotherapeutic sessions regarding future perspectives has not specifically been explored in research yet.
Looking at the predictors identified above, a considerable overlap between many factors is striking: Naturally, forensic psychiatric experts assign a more favorable legal prognosis to patients with more insight into their illness and the wrongfulness of their index offense, and with a more stable treatment adherence, which often stems from insight into the necessity of treatment. Patients with such insight are also more likely to actively engage in constructive psychotherapy and may be more open toward the development of realistic future perspectives. Accordingly, the predictors of the presence of a social network at discharge cannot be viewed as singular factors acting independently of one another. Rather, they must be regarded as a mutually dependent structure. Interestingly, these predictors even dominated factors related to the severity of the disorder upon discharge (e.g., positive and negative symptoms according to the PANSS) or the severity of the offense previously committed. Insight into the illness and the consecutive need for treatment, reflected in treatment adherence, has also been associated with recovery in non-offender patients with SSD (56, 57). However, it has to be noted that insight into one’s own illness and need for treatment can be a double-edged sword: Studies in non-forensic populations of patients with SSD have shown that those who accept they are suffering from a mental illness often feel disempowered and stigmatized, are more likely to adopt a “disabled role” and to socially withdraw (58–60). This can of course hinder the recovery process. Similar observations have not yet been made in forensic psychiatry, and should be explored further in the future, especially as offenders with SSD may be subjected to the double stigmatization of being mentally ill and having a history of delinquency.
Considering limitations of the present study, the retrospective approach is of course notable. Accordingly, the parameters studied were not collected continuously in a standardized manner comparable to a prospective study design. This is not so much a problem for clearly defined variables, such as the length of the prison sentence, but poses difficulty for variables with a certain degree of latitude in their definition (e.g., antisocial behavior or insight in illness), as they may be subjected to a higher interrater bias. Even though interrater reliability was sustainable, as described in the methodology section, one has to be careful as to draw conclusions from especially the dynamic predictors and their clinical implications. While for instance the focus on future perspectives in psychotherapy was found to be of influence regarding social contacts, it was not clearly defined as to how exactly future perspectives were targeted (e.g., whether they related to occupational, financial or social aspects). The authors therefore recommend a replication of the current findings in a prospective setting to evaluate the robustness of causal inferences drawn from them. Furthermore, while populations in forensic psychiatric research are often relatively small compared to general psychiatry and other medical specialties, it has to be acknowledged that our sample of 369 patients, all collected from one single institution, can merely serve an exploratory purpose. Further application of the model to larger populations and other institutions is therefore recommended. As our population consisted only of offender patients with SSD, it has to be noted that the results presented may not be applied to other offender populations (e.g., with personality disorders, offenders with no psychiatric disorder). Also, as it would be expected in an offender population, there were only few women in our sample. This may limit the generalizability to female offender populations. Another notable aspect is that the focus in this study is exclusively on the presence or absence of a social network after discharge. Of course, social contacts are merely one facet of the complex concept of personal recovery. While it is well established how social capital benefits reintegration in society of mentally ill offenders, it is not possible to infer the actual importance of the social network at discharge in the overall construct of personal recovery from the present results. Future research on that matter is therefore needed. Finally, the study design allowed only an explorative variable reduction—although it was possible to break down over 500 variables to the 8 most influential, the findings do not allow a prospective model building regarding social reintegration. Lastly, when critically evaluating the process of model building, it has to be noted that the uneven distribution of the outcome variable would have had negative impact on the model building process and had to be counteracted through up-sampling. This however was performed merely on the training set during the model building process with the goal of creating optimal “lab conditions.” As the validation set did not undergo up-sampling, this procedure does not interfere with the validity of the results. The lack of up-sampling and the resulting uneven distribution of the outcome variable in the validation set also explains why the SVM performed with better performance parameters in the training set under artificially created ideal conditions.
In summation, the present findings contribute to a better understanding of social rehabilitation of offender patients with SSD after court mandated inpatient therapy. Through the use of ML as modern statistic instrument, we were able to identify the 8 factors most related with social capital upon discharge as well as their complex interplay out of a large dataset with over 500 different parameters. Clinical implications here are most likely to arise from the dynamic parameters that can be influenced by therapeutic interventions during hospitalization, whereas the static parameters that cannot be influenced by the course of treatment (e.g., social isolation at the time of the offense) might highlight special needs of affected patients. Of course, these findings do not allow other factors known to influence social reintegration to be dismissed as unimportant. As stated in the discussion of the limitations, social reintegration and recovery are complex processes which cannot be comprehensively evaluated with one single exploratory analysis. However, the findings highlight the value and importance of focusing on social resources and factors promoting them (e.g., decrease of antisocial behavior). Further research on the subject is needed, ideally in prospective trials considering various aspects of social re-integration and the maintenance of the social capital gained.
Data availability statement
The raw data supporting the conclusions of this article will be made available by the authors, without undue reservation.
Ethics statement
The studies involving human participants were reviewed and approved by Ethics Committee of the Canton of Zurich. Written informed consent for participation was not required for this study in accordance with the national legislation and the institutional requirements.
Author contributions
JK: conceptualization, methodology, software, and data curation. JK and LH: validation, formal analysis, and investigation. LH, JK, and SL: resources and writing—review and editing. LH: writing—original draft preparation and visualization. SL and JK: supervision. SL: project administration. All authors have read and agreed to the published version of the manuscript.
Conflict of interest
The authors declare that the research was conducted in the absence of any commercial or financial relationships that could be construed as a potential conflict of interest.
Publisher’s note
All claims expressed in this article are solely those of the authors and do not necessarily represent those of their affiliated organizations, or those of the publisher, the editors and the reviewers. Any product that may be evaluated in this article, or claim that may be made by its manufacturer, is not guaranteed or endorsed by the publisher.
References
1. Mills A, Codd H. Prisoners’ families and offender management: mobilizing social capital. Probat J. (2008) 55:9–24.
2. Farrall S. Social capital and offender reintegration: making probation desistance focused. In: Immarigeon R editor. After Crime and Punishment: Pathways to Offender Reintegration. Portland, OR: Willan Publishing (2004). p. 57–82.
3. Wolff N, Draine J. Dynamics of social capital of prisoners and community reentry: ties that bind? J Correct Health Care. (2004) 10:457–90.
4. Koetzle D, Matthews B. Social capital: the forgotten responsivity factor. Eur J Probat. (2020) 12:219–37.
5. Burch T. Imprisoning communities: how mass incarceration makes disadvantaged neighborhoods worse. By todd clear. Law Soc Rev. (2009) 43:716–8.
6. Skeem J, Eno Louden J, Manchak S, Vidal S, Haddad E. Social networks and social control of probationers with co-occurring mental and substance abuse problems. Law Hum Behav. (2009) 33:122–35. doi: 10.1007/s10979-008-9140-1
7. Sonnweber M, Lau S, Kirchebner J. Violent and non-violent offending in patients with schizophrenia: exploring influences and differences via machine learning. Compr Psychiatry. (2021) 107:152238. doi: 10.1016/j.comppsych.2021.152238
8. Gendreau P, Little T, Goggin C. A meta−analysis of the predictors of adult offender recidivism: what works! Criminology. (1996) 34:575–608.
9. Kirchebner J, Sonnweber M, Nater UM, Günther M, Lau S. Stress, schizophrenia, and violence: a machine learning approach. J Interpers Viol. (2022) 37:602–22.
10. Schoppmann S, Balensiefen J, Lau S, Graf M, Hachtel H. Patients’ views with regard to personal recovery in forensic psychiatry in German-speaking Switzerland-an explorative study. Front Psychiatry. (2021) 12:695096. doi: 10.3389/fpsyt.2021.695096
11. Shepherd A, Doyle M, Sanders C, Shaw J. Personal recovery within forensic settings–systematic review and meta-synthesis of qualitative methods studies. Crim Behav Ment Health. (2016) 26:59–75. doi: 10.1002/cbm.1966
12. Clarke C, Lumbard D, Sambrook S, Kerr K. What does recovery mean to a forensic mental health patient? A systematic review and narrative synthesis of the qualitative literature. J Forensic Psychiatry Psychol. (2016) 27:38–54.
13. Rowaert S, Vandevelde S, Lemmens G, Vanderplasschen W, Vander Beken T, Vander Laenen F, et al. The role and experiences of family members during the rehabilitation of mentally ill offenders. Int J Rehabil Res. (2016) 39:11–9. doi: 10.1097/MRR.0000000000000152
14. Foldemo A, Gullberg M, Ek A-C, Bogren L. Quality of life and burden in parents of outpatients with schizophrenia. Soc Psychiatry Psychiatr Epidemiol. (2005) 40:133–8.
15. Ferriter M, Huband N. Experiences of parents with a son or daughter suffering from schizophrenia. J Psychiatr Ment Health Nurs. (2003) 10:552–60.
16. Ward T, Brown M. The good lives model and conceptual issues in offender rehabilitation. Psychol Crime Law. (2004) 10:243–57.
17. Ward T, Fortune C-A. The good lives model: aligning risk reduction with promoting offenders’ personal goals. Eur J Probat. (2013) 5:29–46.
18. Ward T, Mann RE, Gannon TA. The good lives model of offender rehabilitation: clinical implications. Aggress Violent Behav. (2007) 12:87–107.
19. World Health Organization. International Classification of Diseases Basic Tabulation List with Alphabetic Index. 9th revision ed. Geneva: World Health Organization (1978).
20. World Health Organization. ICD-10: International Statistical Classification of Diseases and Related Health Problems. 10th revision ed. Geneva: World Health Organization (2004).
21. Hsieh H-F, Shannon SE. Three approaches to qualitative content analysis. Qual Health Res. (2005) 15:1277–88.
22. Brennan PF, Hays BJ. Focus on psychometrics the kappa statistic for establishing interrater reliability in the secondary analysis of qualitative clinical data. Res Nurs Health. (1992) 15:153–8. doi: 10.1002/nur.4770150210
23. Seifert D. Die entwicklung des psychiatrischen massregelvollzzugs (§63StGB) in Nordrhein-Wesfalen. Psychiat Prax. (1997) 24:237–44.
24. Kutscher S, Schiffer B, Seifert D. Schizophrene patienten im psychiatrischen massregelvollzug (§63 StGB) Nordrhein-Westfalens. Fortschr Neurol Psychiatr. (2009) 77:91–6.
25. Habermeyer E, Wolff R, Gillner M, Strohm R, Kutscher S. Patienten mit schizophrenen Störungen im psychiatrischen Maßregelvollzug. Der Nervenarzt. (2010) 81:1117–24.
26. Günther MP, Kirchebner J, Lau S. Identifying direct coercion in a high risk subgroup of offender patients with schizophrenia via machine learning algorithms. Front Psychiatry. (2020) 11:415. doi: 10.3389/fpsyt.2020.00415
27. Hofmann LA, Lau S, Kirchebner J. Advantages of machine learning in forensic psychiatric research & mdash;uncovering the complexities of aggressive behavior in schizophrenia. Appl Sci. (2022) 12:819.
28. Kirchebner J, Günther MP, Lau S. Identifying influential factors distinguishing recidivists among offender patients with a diagnosis of schizophrenia via machine learning algorithms. Forensic Sci Int. (2020) 315:110435. doi: 10.1016/j.forsciint.2020.110435
29. Kirchebner J, Günther MP, Sonnweber M, King A, Lau S. Factors and predictors of length of stay in offenders diagnosed with schizophrenia - a machine-learning-based approach. BMC Psychiatry. (2020) 20:201. doi: 10.1186/s12888-020-02612-1
30. Kirchebner J, Lau S, Kling S, Sonnweber M, Günther MP. Individuals with schizophrenia who act violently towards others profit unequally from inpatient treatment—identifying subgroups by latent class analysis. Int J Methods Psychiatr Res. (2021) 30:e1856. doi: 10.1002/mpr.1856
31. Kirchebner J, Lau S, Sonnweber M. Escape and absconding among offenders with schizophrenia spectrum disorder–an explorative analysis of characteristics. BMC Psychiatry. (2021) 21:122. doi: 10.1186/s12888-021-03117-1
32. Lau S, Günther MP, Kling S, Kirchebner J. Latent class analysis identified phenotypes in individuals with schizophrenia spectrum disorder who engage in aggressive behaviour towards others. Eur Psychiatry. (2019) 60:86–96. doi: 10.1016/j.eurpsy.2019.05.005
33. Lau S, Kirchebner J, Kling S, Euler S, Günther MP. Childhood maltreatment, psychopathology, and offending behavior in patients with schizophrenia: a latent class analysis evidencing disparities in inpatient treatment outcome. Front Psychiatry. (2021) 12:612322. doi: 10.3389/fpsyt.2021.612322
34. Patterson A, Sonnweber M, Lau S, Günther MP, Seifritz E, Kirchebner J. Schizophrenia and substance use disorder: characteristics of coexisting issues in a forensic setting. Drug Alcohol Depend. (2021) 226:108850. doi: 10.1016/j.drugalcdep.2021.108850
35. Huber DA, Lau S, Sonnweber M, Günther MP, Kirchebner J. Exploring similarities and differences of non-European migrants among forensic patients with schizophrenia. Int J Environ Res Public Health. (2020) 17:7922. doi: 10.3390/ijerph17217922
36. Papadopoulos C, Ross J, Stewart D, Dack C, James K, Bowers L. The antecedents of violence and aggression within psychiatric in−patient settings. Acta Psychiatr Scand. (2012) 125:425–39.
37. Daly RJ. Effects of imprisonment and isolation. In: Pichot P, Berner P, Wolf R, Thau K editors. Psychiatry: The State of the Art Drug Dependence and Alcoholism, Forensic Psychiatry, Military Psychiatry. (Vol. 6), Boston, MA: Springer (1985). p. 249–54.
38. Santhosh R, Mathew E. Social reintegration of released prisoners: an empirical analysis from two Indian states. Int Ann Criminol. (2021) 59:200–22.
39. Nguyễn, KH, Yvon D. The social re-integration of offenders. VNU J Sci Legal Stud. (2013) 29:24–38.
40. Völlm B, Edworthy R, Holley J, Talbot E, Majid S, Duggan C, et al. A Mixed-Methods Study Exploring the Characteristics and Needs of Long-Stay Patients in High and Medium Secure Settings in England: Implications for Service Organisation. Southampton: Health Services and Delivery Research (2017).
41. Eckert M, Schel SHH, Kennedy HG, Bulten BH. Patient characteristics related to length of stay in Dutch forensic psychiatric care. J Forensic Psychiatry Psychol. (2017) 28:863–80.
42. Rowaert S, Vandevelde S, Lemmens G, Audenaert K. How family members of mentally ill offenders experience the internment measure and (forensic) psychiatric treatment in Belgium: a qualitative study. Int J Law Psychiatry. (2017) 54:76–82. doi: 10.1016/j.ijlp.2017.05.003
44. Walravens T, Bierbooms J, Ter Horst P. Recovery and strength-based practice in long-term forensic psychiatry. In: Völlm B, Braun P editors. Long-Term Forensic Psychiatric Care: Clinical, Ethical and Legal Challenges. Cham: Springer International Publishing (2019). p. 81–102.
45. Hodgins S, Toupin J, Côté G. Schizophrenia and antisocial personality disorder: a criminal combination. In: Schlesinger LB editor. Explorations in Criminal Psychopathology: Clinical Syndromes with Forensic Implications. Springfield, IL: Charles C Thomas Publisher (1996). p. 217–37.
46. Young S, Das M, Gudjonsson GH. Reasoning and rehabilitation cognitive skills programme for mentally disordered offenders: predictors of outcome. World J Psychiatry. (2016) 6:410–8. doi: 10.5498/wjp.v6.i4.410
47. Gray R, Bressington D, Lathlean J, Mills A. Relationship between adherence, symptoms, treatment attitudes, satisfaction, and side effects in prisoners taking antipsychotic medication. J Forensic Psychiatry Psychol. (2008) 19:335–51.
48. Cavezza C, Aurora M, Ogloff JRP. The effects of an adherence therapy approach in a secure forensic hospital: a randomised controlled trial. J Forensic Psychiatry Psychol. (2013) 24:458–78.
49. Rezansoff SN, Moniruzzaman A, Fazel S, McCandless L, Somers JM. Adherence to antipsychotic medication and criminal recidivism in a Canadian provincial offender population. Schizophr Bull. (2017) 43:1002–10. doi: 10.1093/schbul/sbx084
50. Buckley PF, Wirshing DA, Bhushan P, Pierre JM, Resnick SA, Wirshing WC. Lack of insight in schizophrenia. CNS Drugs. (2007) 21:129–41.
51. Higashi K, Medic G, Littlewood KJ, Diez T, Granström O, De Hert M. Medication adherence in schizophrenia: factors influencing adherence and consequences of nonadherence, a systematic literature review. Ther Adv Psychopharmacol. (2013) 3:200–18. doi: 10.1177/2045125312474019
52. Kröber H-L. Leugnen der tat und tatbearbeitung in der prognostischen begutachtung. Forensis Psychiatr Psychol Kriminol. (2010) 4:32–8.
53. de Vogel V, de Vries Robbé M, de Ruiter C, Bouman YHA. Assessing protective factors in forensic psychiatric practice: introducing the SAPROF. Int J Forensic Ment Health. (2011) 10:171–7.
54. de Vries Robbé M, de Vogel V, Koster K, Bogaerts S. Assessing protective factors for sexually violent offending with the SAPROF. Sex Abuse. (2014) 27:51–70.
55. Noland E, Strandh M. Historical, clinical and situational risk factors for post-discharge recidivism in forensic psychiatric patients – a Swedish registry study. Int J Law Psychiatry. (2021) 79:101749. doi: 10.1016/j.ijlp.2021.101749
56. Liberman RP, Kopelowicz A, Ventura J, Gutkind D. Operational criteria and factors related to recovery from schizophrenia. Int Rev Psychiatry. (2002) 14:256–72.
57. Lysaker P, Yanos PT, Roe D. The role of insight in the process of recovery from schizophrenia: a review of three views. Psychosis. (2009) 1:113–21.
58. Warner R. Recovery from schizophrenia and the recovery model. Curr Opin Psychiatry. (2009) 22:374–80.
59. Lysaker PH, Davis LW, Warman DM, Strasburger A, Beattie N. Stigma, social function and symptoms in schizophrenia and schizoaffective disorder: associations across 6 months. Psychiatry Res. (2007) 149:89–95.
Keywords: social capital, machine learning, schizophrenia, personal recovery, forensic psychiatry
Citation: Hofmann LA, Lau S and Kirchebner J (2022) Maintaining social capital in offenders with schizophrenia spectrum disorder—An explorative analysis of influential factors. Front. Psychiatry 13:945732. doi: 10.3389/fpsyt.2022.945732
Received: 16 May 2022; Accepted: 06 October 2022;
Published: 20 October 2022.
Edited by:
Birgit Angela Völlm, University of Rostock, GermanyReviewed by:
Christian Huber, University Psychiatric Clinic Basel, SwitzerlandWing Chung Chang, The University of Hong Kong, Hong Kong SAR, China
Copyright © 2022 Hofmann, Lau and Kirchebner. This is an open-access article distributed under the terms of the Creative Commons Attribution License (CC BY). The use, distribution or reproduction in other forums is permitted, provided the original author(s) and the copyright owner(s) are credited and that the original publication in this journal is cited, in accordance with accepted academic practice. No use, distribution or reproduction is permitted which does not comply with these terms.
*Correspondence: Lena A. Hofmann, bGVuYS5ob2ZtYW5uQHB1a3poLmNo