- 1Department of Psychiatry, University of Pennsylvania Perelman School of Medicine, Philadelphia, PA, United States
- 2National Institute on Alcohol Abuse and Alcoholism, Bethesda, MD, United States
- 3Department of Psychiatry, Cambridge Health Alliance, Harvard Medical School, Boston, MA, United States
Background: Excessive consumption of opioids is associated with impaired metabolic function including increased body mass index (BMI). Opioid antagonist naltrexone (NTX) is an effective treatment for opioid use disorder (OUD) that has the potential to mitigate such metabolic disturbances. Understanding the relationship between treatment adherence and BMI in NTX-treated OUD patients may provide valuable insights into optimizing clinical outcomes.
Methods: Patients with opioid dependence were offered up to three monthly injections of extended-release (XR) NTX. Treatment completers (n = 41) were defined as those who had received all three XR-NTX injections, and non-completers (n = 20) as those missing at least one injection. Logistic regression was performed to examine the association between pre-treatment BMI and treatment completion.
Results: BMI was positively associated with treatment completion. This association remained significant after adjusting for potentially confounding variables.
Conclusion: Our findings suggest that baseline BMI may serve as a potential predictor of XR-NTX treatment adherence in patients with OUD and could help healthcare providers and policy makers alike in developing strategies to improve retention and tailor interventions for specific patient subgroups.
1. Introduction
Opioid use disorder (OUD) is a serious global public health issue affecting over 16 million individuals worldwide (1). In the United States, OUD ranks as one of the leading causes of disability-adjusted life-years (2–5). OUD is associated with excessive body weight gain (BWG) leading to overweight and obesity (6–8). Exogenous opioids enhance μ-opioidergic neurotransmission (9, 10) that not only stimulates neuropeptides that promote appetite but also inhibits neuropeptides that suppress appetite (11). The enhanced μ-opioid neurotransmission further modulates eating behaviors by increasing the preference for pleasurable and indulgent foods high in sweetness and fat (12, 13). Furthermore, chronic exposure to opioids and substances of abuse produces neuroadaptations that promotes overeating during abstinence due to the lack of the reinforcing effects of the substances (14). As a result, these mechanisms (11) may play a role in the development of food cravings and even food addiction (15–18).
Besides negative psychosocial impacts (low self-esteem and societal stigmatization) of BWG (19), OUD patients are particularly susceptible to the detrimental medical sequelae of BWG such as the Metabolic Syndrome (20), which is a cluster of cardiovascular risk factors, including abdominal adiposity, insulin resistance, impaired glucose tolerance, dyslipidemia, and hypertension (21). These effects may contribute to the high rates (22, 23) of treatment non-adherence with the OUD medication-assisted treatment (MAT) involving opioid agonists such as buprenorphine and methadone (24, 25), which, despite their positive clinical outcomes in terms of reducing overdose mortality, infectious diseases, crime, and societal impact (26, 27), also elicit concerns about their potential negative effects on eating habits and consequent unwanted BWG (20, 28, 29). In contrast, the remaining MAT constituent (30), opioid antagonist naltrexone (NTX), has been associated with diminished sweet taste’s hedonic appeal (31), along with decrements in food intake, body fat mass (32) and BWG (33). Moreover, in combination with bupropion, NTX is commonly prescribed for weight management in individuals who are overweight or obese (34). Likewise, NTX analog, samidorphan (35), counteracts BWG arising in the context of antipsychotic therapy (36). Heightened BMI can be associated with negative affective states, body image concerns or weight-related stigmatization that may impact patients’ self-esteem or self-efficacy driving their engagement with treatment (19). The anticipated alleviation of opioid-induced metabolic effects through the opioid receptor blockade by NTX could potentially enhance adherence to NTX treatment through negative reinforcement (11, 37).
The assessment of adherence to medication often relies on self-reports and collateral information (38) as well as pill counts (39), electronic monitoring devices (40–42), biomarkers or blood tests (43), direct observation of intake (44), pharmacy records (45), mobile apps and digital tools (46). Assessing oral NTX adherence in OUD patients remains a daunting task (42) due to conscious and unconscious denial, cognitive deficits secondary to the use of mind-altering substances, fear of disclosure and lack of trust (47) in the healthcare system and in the clinical research establishment (25, 48, 49). The monthly injectable, extended-release NTX (XR-NTX) is associated with better adherence than oral NTX, and such adherence can be easily assessed objectively. Nevertheless, adherence to XR-NTX varies across individuals, and there is a particularly high rate of premature dropout during the first 3 months of treatment (50–52). It is challenging because relapse to opioids almost invariably follows non-adherence to XR-NTX, and repeat detoxification is required before resuming XR-NTX (53, 54). Relapse after discontinuing XR-NTX also poses a high risk of fatal overdose due to the loss of tolerance to opioids (55) and hypersensitivity of opioid receptors (56). Efforts to improve adherence have identified incentives for continual treatment, such as physicians and businessmen with professional and financial reasons to remain abstinent (57), and neurobiological markers (54, 58). However, the effectiveness of these approaches in improving treatment adherence remains unclear.
To that end, we operationalized adherence as receipt of all three XR-NTX injections offered on the present study (i.e., treatment completion), while non-completers were those who have missed at least one injection (37, 52, 58). While this is just one possible definition of treatment adherence (59), we have chosen such approach because it provides a clear, measurable, and unambiguous definition. In addition, it is not affected by other variables that may be subjected to missing data (e.g., urine toxicology screenings), ensuring a more accurate estimate of the variable of interest. Building on the above considerations, we hypothesized that higher BMI would be associated with more treatment adherence to XR-NTX in opioid dependent patients.
2. Methods and materials
2.1. Participants
The study is a retrospective, secondary analysis of data collected by two intervention studies in 61 detoxified OUD patients (37, 58, 60). Participants of both studies were offered free, medically supervised, 3-month treatment with XR-NTX for OUD. One study stipulated intravenous heroin as the drug of choice (37), whereas the other included both individuals who use heroin and prescription pill (58, 60). Inclusion criteria were (1) DSM-IV-TR diagnosis of opioid dependence, established using the best estimate format based on all available information (including history and physical examination and the Mini-International Neuropsychiatric Interview) (2), active opioid use for more than 2 weeks in the 3 months prior to detoxification, and (3) good physical health, as evidenced by clinical examination. Participants were excluded if they demonstrated (1) any current major psychiatric disorder (i.e., psychosis, dementia, and schizophrenia) (2), current major medical problems, head trauma, or neurological disorder (3), current use of potentially confounding medications, including anti-dopaminergic agents, anticonvulsants, and beta-blockers, or (4) being pregnant or breastfeeding. Detailed information on the studies was reported elsewhere (37, 58, 60).
2.2. Study procedures
Participants’ weight and height and demographics were assessed at screening prior to treatment. Weight was measured using a digital electronic scale and height with a Harpenden stadiometer, calibrated on a weekly basis. Opioid craving was scored subjectively using a 10-point scale (0 = none; 9 = extremely) (60), for which data were unavailable for three participants.
Following complete opioid detoxification, as confirmed with a negative naloxone challenge test, subjects were offered up to three monthly intramuscular injections of XR-NTX (380 mg extended-release naltrexone-HCl, Vivitrol®, Alkermes Inc., Cambridge, MA). Sixty-one individuals having received at least one XR-NTX injection and for which baseline BMI measurements were available were included in the analysis. Treatment completers were categorized as individuals who have received all three monthly injections of XR-NTX, whereas non-completers only received one or two of the injections.
2.3. Data analysis
All statistical analyzes were performed with R.1 The association between treatment completion (0 = non-completion, 1 = completion) and BMI was examined by logistic regression. Due to the observational design of the study and the lack of randomization, we investigated the effects of controlling for other baseline variables (see Table 1) using the purposeful variable selection procedure (61). Specifically, each variable was tested individually for its association with treatment completion using a simple logistic regression. Variables that reached p < 0.25 were selected as candidates and entered in a multivariable logistic regression that tested their joint association with treatment completion. If the variable that had the largest value of p in the multivariable model reached p < 0.10, or if removing it from the model resulted in a change in any remaining parameter estimate greater than 15%, then the variable was retained in the model, and the variable that had the second largest value of p was subjected to the same examination. Otherwise, the variable with the largest value of p was removed, and a reduced multivariable logistic regression model was examined. The process was iterated until all remaining variables in the model were determined eligible to be retained. Lastly, the non-candidate variables that were not selected for the initial multivariable model were included one at a time, starting with the one that had the smallest value of p in the simple logistic regression analysis. The non-candidates that reached p < 0.15 in the multivariable model or caused a change in any remaining parameter estimate greater than 15% were retained in the model. Given that BMI was the variable of interest, and that data were obtained from two different studies, we included BMI and study (Study 1 vs. Study 2) in all multivariable models regardless of their p-values and effects on the coefficient estimates of other variables. The variable race was dichotomized to Caucasian vs. non-Caucasian because of the small number of individuals of other races. Due to the limitations of the Wald test in small and unbalanced samples (62, 63), we used the likelihood-ratio test to evaluate coefficient significance. Group data were summarized as mean ± standard deviation (SD). All analyzes were two-tailed, and p < 0.05 defined statistical significance.
The following two considerations guided our choice of a binary outcome (i.e., completion vs. non-completion). The first consideration was clinical relevance, as completion reflects the extent to which individuals have followed through with the entire a priori recommended treatment protocol. The second consideration was pragmatism, as binary categorization is easier to analyze and communicate in clinical settings compared to the varying number of injections.
3. Results
A total of 61 participants received at least one dose of XR-NTX, of whom 41 were completers and 20 were non-completers (see Methods). Baseline characteristics of included participants are presented in Table 1. BMI ranged from 18.9–36.2, consisting of normal weight (n = 40), overweight (BMI > 25; n = 12) and obese (BMI > 30; n = 9). Remarkably, 91% (n = 19 out of 21) of participants in the overweight/obese category vs. 55% (n = 22 out of 40) of participants with a normal weight completed the study treatment with three monthly XR-NTX injections (p < 0.01, Fisher Exact Test).
Logistic regression model showed that higher BMI was significantly associated with higher likelihood of treatment completion (B = 0.28, SE = 0.10, p = 0.002; exp.(B) = 1.32, 95% confidence interval = [1.10, 1.67]; χ2(1) = 10.01, Nagelkerke R2 = 0.21; see Figure 1). The association between treatment completion and each of the other baseline variables is summarized in Table 1.
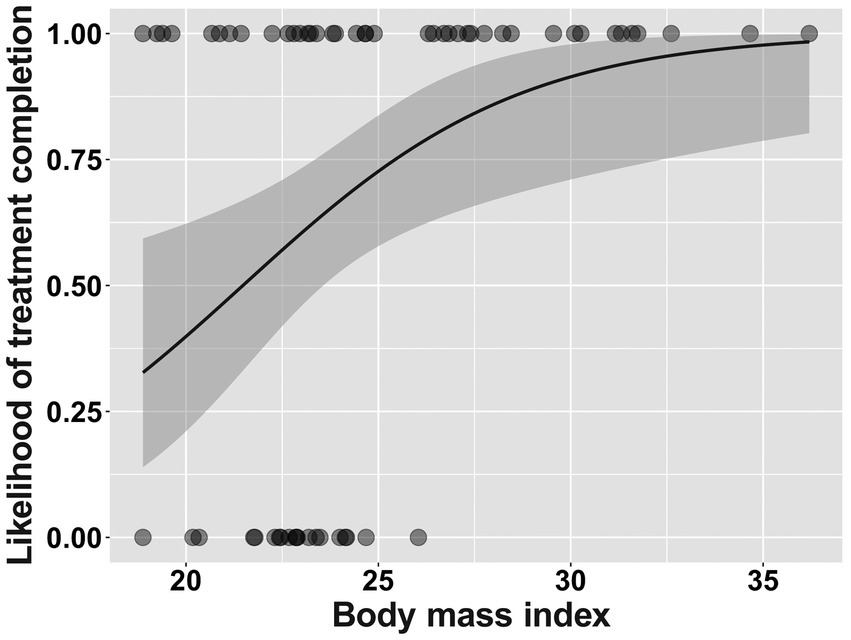
Figure 1. Simple logistic regression model of treatment completion predicted by body mass index. The shaded area represents 95% confidence interval.
During the purposeful model selection procedure, craving and age were selected as candidates for multivariable logistic regression, in addition to BMI and study (Study 1 vs. Study 2). Age was subsequently eliminated from the multivariable model, and education was added back. BMI remained a significant predictor of treatment completion. The final multivariable model is summarized in Table 2.
4. Discussion
The present study examined the association between BMI and treatment completion in opioid dependent patients that were offered up to three monthly injections of XR-NTX. The results indicate that higher BMI was associated with a higher likelihood of treatment completion, suggesting that individuals with higher BMI may demonstrate better adherence to XR-NTX therapy. It is reasonable to assume that improved BMI and related metabolic outcomes would strongly motivate continued adherence to XR-NTX.
Nonetheless, a comprehensive range of emotional, motivational, and cognitive processes are involved in planning a recovery path, assessing the risk of relapse or deterioration in opioid consumption, and evaluating the outcomes of ongoing XR-NTX therapy. Changes in any of these mechanisms can determine whether a patient gains control over opioid addiction or whether the addiction gains control over that patient. According to Prospect Theory (64), individuals subjectively evaluate outcomes through elaborate mental processes. These processes involve adjusting their expectations (i.e., prospects) regarding the likelihood of continued overweight/obesity or successful weight loss based on an acceptable level of recovery-related discomfort. This adjustment impacts the coping strategies that patients employ, which in turn influences their expectations and satisfaction with their body weight outcomes. It is possible that protracted withdrawal and/or food craving up to a new neutral state may not be perceived as aversive (65) in such reformulated contextual framing (66, 67), prompting adherence and eventual recovery (37).
On the other hand, the subjective valuation process leads to distinct slopes in the emotional functions of weight gain and loss. The weight gain may typically exhibit a steeper slope than the loss domain (68, 69), contributing to maladaptive coping strategies such as emotional eating, using food for comfort, self-blame, negative self-talk about body image, and engaging in disordered eating behaviors (70, 71). Moreover, since neural circuitries underlying the motivational effects of opioids and palatable food overlap (14, 72, 73) and may sensitize over time, cross-sensitization might occur as well (11, 74). This means that exposure to opioids could increase the consumption of unhealthy food, and vice versa. As such, preclinical evidence suggests that amphetamine-sensitive rats demonstrate cross-sensitization to sugar (75), and that exposure to high-fat diets in utero increases later sensitivity to drugs of abuse (14). In short, realization that opioid and food addictions share a common neurobiological foundation (11, 76) has important theoretical and therapeutic implications underscoring the need for biopsychosocial interventions by a multidisciplinary team comprised healthcare professionals, addiction specialists, nutritionists, and mental health providers delivering holistic care and support for individuals navigating weight management concurrently with the treatment of opioid addiction (77).
It is worth noting that therapeutic adherence is a complex entity affected by multiple factors, and BMI alone is unlikely to be a robust predictor in isolation. Individualized assessments and a comprehensive understanding of a patient’s circumstances are crucial for effective treatment planning and adherence support. From the biochemical perspective, higher BMI is associated with greater plasma leptin concentration and food consumption (78, 79). Blood glucose elevations, such as after a meal, trigger the release of insulin along with incretin hormones (e.g., glucagon-like peptide-1 and glucose-dependent insulinotropic polypeptide) that restrain the hedonic/motivational neural pathways otherwise driving the consumption of both palatable food (80, 81) and opioid drugs (11, 20). Moreover, if a patient has a higher BMI and low ratio of lean body mass to fat mass, the pharmacokinetics of naltrexone may be affected, potentially altering the drug’s side effects due to changes in the medication’s volume of distribution, clearance, and metabolism (82).
From social and environmental perspectives, BMI can be linked to many factors (83) that may indirectly influence adherence. For instance, socioeconomic status, access to healthcare, social support networks, and cultural norms related to body weight and medication use can vary among individuals with different BMIs. In fact, some opioid-dependent individuals may even experience weight loss (84, 85) potentially attributable to stress, lifestyle disruptions, such as neglecting nutritional needs or engaging in risky behaviors, which can further contribute to emaciation. Weight loss in opioid dependent patients may indicate malnutrition, poor overall health, and increased vulnerability to complications (84). These factors may impact adherence by affecting medication availability, support systems, or adherence-promoting messages by healthcare professionals. Whatever the case may be, encouraging healthy lifestyle, sleep hygiene, proper access to healthcare, regular exercise and physical activity can not only support weight management but also contribute to improved mood, overall well-being, stress reduction, and therapeutic adherence, which are essential aspects of addiction recovery.
Additionally, our results are consistent with previous findings that craving is associated with poor treatment outcomes in OUD (86–88). The predictive value of craving on drugs seeking behavior extends to other drugs of abuse, including cocaine (89) and methamphetamine (90). Here, we demonstrated a trending effect of baseline craving scores in predicting XR-NTX treatment completion, which became significant after controlling for BMI in the multivariable regression model. Nevertheless, this finding should be interpreted with caution due to the small sample size and utilization of a single-item 10-point subjective craving scale, as opposed to validated questionnaires (91).
It is important to acknowledge the limitations of the study, including its retrospective nature, potential confounders, selection bias, small sample size, and the inclusion of data from two different studies, which may limit the generalizability of the findings. The study also relied on subjective craving scores, which may introduce measurement errors and biases compared to more objective measures of craving and treatment adherence (37, 92, 93). Further research with larger prospective cohorts and other measures of treatment adherence is warranted to validate these findings and explore the underlying mechanisms.
In conclusion, improved BMI and related metabolic outcomes are likely to serve as strong motivators for continued adherence, though the findings need be interpreted with caution given the aforementioned limitations of the study. The association between high BMI and improved therapeutic outcome with XR-NTX, if confirmed by future research, would indicate that XR-NTX should be the default first step if MAT is considered in obese/overweight OUD patients. The findings underscore the importance of the biopsychosocial aspects of opioid addiction treatment and weight management. A multidisciplinary approach can deliver holistic care and support to individuals navigating weight management concurrently with the treatment of opioid addiction. Overall, this study adds to our understanding of the complex interplay between BMI, treatment completion, and adherence in the context of OUD and XR-NTX therapy. By considering the individualized needs and circumstances of patients, healthcare providers can enhance treatment planning and adherence support, leading to improved outcomes in both addiction recovery and optimal weight management.
Data availability statement
The original contributions presented in the study are included in the article/supplementary materials, further inquiries can be directed to the corresponding authors.
Ethics statement
The studies involving humans were approved by University of Pennsylvania Institutional Review Board. The studies were conducted in accordance with the local legislation and institutional requirements. The participants provided their written informed consent to participate in this study.
Author contributions
DL designed the study and collected the data. XL, KL, CW, and ZS analyzed the data. XL, DL, KL, G-JW, IE, CW, and ZS interpreted the results. XL, DL, IE, CW, and ZS wrote the manuscript. All authors provided critical revision of the manuscript for intellectual content and approved the final version of the manuscript.
Funding
This work was supported by the Commonwealth of Pennsylvania CURE grant SAP#4100055577 (Anna Rose Childress, University of Pennsylvania), the NARSAD Young Investigator Grant from the Brain & Behavior Research Foundation (#30780) (ZS), and the following National Institutes of Health grants: T32DA028874 (XL), R01DA024553 (Charles P. O’Brien, University of Pennsylvania), R00AA026892 (CW), and K01DA051709 (ZS). The funding sources had no role in the study design, collection, analysis, or interpretation of the data, writing the manuscript, or the decision to submit the paper for publication.
Conflict of interest
The authors declare that the research was conducted in the absence of any commercial or financial relationships that could be construed as a potential conflict of interest.
Publisher’s note
All claims expressed in this article are solely those of the authors and do not necessarily represent those of their affiliated organizations, or those of the publisher, the editors and the reviewers. Any product that may be evaluated in this article, or claim that may be made by its manufacturer, is not guaranteed or endorsed by the publisher.
Footnotes
References
1. Dydyk, AM, Jain, NK, and Gupta, M. Opioid Use Disorder. Treasure Island, FL: Stat Pearls Publishing (2023).
2. Mokdad, AH, Ballestros, K, Echko, M, Glenn, S, Olsen, HE, Mullany, E, et al. The state of US health, 1990-2016: burden of diseases, injuries, and risk factors among US states. JAMA. (2018) 319:1444–72. doi: 10.1001/jama.2018.0158
3. Rhee, TG, and Rosenheck, RA. Association of current and past opioid use disorders with health-related quality of life and employment among US adults. Drug Alcohol Depend. (2019) 199:122–8. doi: 10.1016/j.drugalcdep.2019.03.004
4. Hedegaard, H, Miniño, AM, Spencer, MR, and Warner, M. Drug overdose deaths in the United States, 1999-2020. NCHS Data Brief. (2021) 426:1–8.
5. Injuryfacts. US odds of dying (2021 data). (2023) Available at: https://injuryfacts.nsc.org/all-injuries/preventable-death-overview/odds-of-dying.
6. Stokes, A, Lundberg, DJ, Hempstead, K, Berry, KM, Baker, JF, and Preston, SH. Obesity and incident prescription opioid use in the U.S., 2000-2015. Am J Prev Med. (2020) 58:766–75. doi: 10.1016/j.amepre.2019.12.018
7. Sweeney, MM, Antoine, DG, Nanda, L, Geniaux, H, Lofwall, MR, Bigelow, GE, et al. Increases in body mass index and cardiovascular risk factors during methadone maintenance treatment. J Opioid Manag. (2019) 15:367–74. doi: 10.5055/jom.2018.0526
8. Byanyima, JI, Li, X, Vesslee, SA, Kranzler, HR, Shi, Z, and Wiers, CE. Metabolic profiles associated with opioid use and opioid use disorder: a narrative review of the literature. Curr Addict Rep. (2023) 2023:493. doi: 10.1007/s40429-023-00493-4
9. Nogueiras, R, Romero-Picó, A, Vazquez, MJ, Novelle, MG, López, M, and Diéguez, C. The opioid system and food intake: homeostatic and hedonic mechanisms. Obes Facts. (2012) 5:196–207. doi: 10.1159/000338163
10. Guyenet, SJ, and Schwartz, MW. Clinical review: regulation of food intake, energy balance, and body fat mass: implications for the pathogenesis and treatment of obesity. J Clin Endocrinol Metab. (2012) 97:745–55. doi: 10.1210/jc.2011-2525
11. Elman, I, Borsook, D, and Lukas, SE. Food intake and reward mechanisms in patients with schizophrenia: implications for metabolic disturbances and treatment with second-generation antipsychotic agents. Neuropsychopharmacology. (2006) 31:2091–120. doi: 10.1038/sj.npp.1301051
12. Berridge, KC, and Robinson, TE. Liking, wanting, and the incentive-sensitization theory of addiction. Am Psychol. (2016) 71:670–9. doi: 10.1037/amp0000059
13. Nolan, LJ, and Scagnelli, LM. Preference for sweet foods and higher body mass index in patients being treated in long-term methadone maintenance. Subst Use Misuse. (2007) 42:1555–66. doi: 10.1080/10826080701517727
14. Bocarsly, ME, Barson, JR, Hauca, JM, Hoebel, BG, Leibowitz, SF, and Avena, NM. Effects of perinatal exposure to palatable diets on body weight and sensitivity to drugs of abuse in rats. Physiol Behav. (2012) 107:568–75. doi: 10.1016/j.physbeh.2012.04.024
15. Grigson, PS. Like drugs for chocolate: separate rewards modulated by common mechanisms? Physiol Behav. (2002) 76:389–95. doi: 10.1016/S0031-9384(02)00758-8
16. Gordon, EL, Ariel-Donges, AH, Bauman, V, and Merlo, LJ. What is the evidence for food addiction? A systematic review. Nutrients. (2018) 10:477. doi: 10.3390/nu10040477
17. Canan, F, Karaca, S, Sogucak, S, Gecici, O, and Kuloglu, M. Eating disorders and food addiction in men with heroin use disorder: a controlled study. Eat Weight Disord. (2017) 22:249–57. doi: 10.1007/s40519-017-0378-9
18. Neale, J, Nettleton, S, Pickering, L, and Fischer, J. Eating patterns among heroin users: a qualitative study with implications for nutritional interventions. Addiction. (2012) 107:635–41. doi: 10.1111/j.1360-0443.2011.03660.x
19. Puhl, RM, and Heuer, CA. Obesity stigma: important considerations for public health. Am J Public Health. (2010) 100:1019–28. doi: 10.2105/AJPH.2009.159491
20. Elman, I, Howard, M, Borodovsky, JT, Mysels, D, Rott, D, Borsook, D, et al. Metabolic and addiction indices in patients on opioid agonist medication-assisted treatment: a comparison of buprenorphine and methadone. Sci Rep. (2020) 10:5617. doi: 10.1038/s41598-020-62556-0
21. Cornier, MA, Dabelea, D, Hernandez, TL, Lindstrom, RC, Steig, AJ, Stob, NR, et al. The metabolic syndrome. Endocr Rev. (2008) 29:777–822. doi: 10.1210/er.2008-0024
22. Semahegn, A, Torpey, K, Manu, A, Assefa, N, Tesfaye, G, and Ankomah, A. Psychotropic medication non-adherence and its associated factors among patients with major psychiatric disorders: a systematic review and meta-analysis. Syst Rev. (2020) 9:17. doi: 10.1186/s13643-018-0676-y
23. Dibonaventura, M, Gabriel, S, Dupclay, L, Gupta, S, and Kim, E. A patient perspective of the impact of medication side effects on adherence: results of a cross-sectional nationwide survey of patients with schizophrenia. BMC Psychiatry. (2012) 12:20. doi: 10.1186/1471-244X-12-20
24. Kinsky, S, Houck, PR, Mayes, K, Loveland, D, Daley, D, and Schuster, JM. A comparison of adherence, outcomes, and costs among opioid use disorder Medicaid patients treated with buprenorphine and methadone: a view from the payer perspective. J Subst Abus Treat. (2019) 104:15–21. doi: 10.1016/j.jsat.2019.05.015
25. Roux, P, Lions, C, Michel, L, Cohen, J, Mora, M, Marcellin, F, et al. Predictors of non-adherence to methadone maintenance treatment in opioid-dependent individuals: implications for clinicians. Curr Pharm Des. (2014) 20:4097–105. doi: 10.2174/13816128113199990623
26. Strang, J, Volkow, ND, Degenhardt, L, Hickman, M, Johnson, K, Koob, GF, et al. Opioid use disorder. Nat Rev Dis Primers. (2020) 6:3. doi: 10.1038/s41572-019-0137-5
27. Volkow, ND, and Blanco, C. Medications for opioid use disorders: clinical and pharmacological considerations. J Clin Invest. (2020) 130:10–3. doi: 10.1172/JCI134708
28. Vallecillo, G, Robles, MJ, Torrens, M, Samos, P, Roquer, A, Martires, PK, et al. Metabolic syndrome among individuals with heroin use disorders on methadone therapy: prevalence, characteristics, and related factors. Subst Abus. (2018) 39:46–51. doi: 10.1080/08897077.2017.1363122
29. Manza, P, Kroll, D, McPherson, KL, Johnson, A, Dennis, E, Hu, L, et al. Sex differences in weight gain during medication-based treatment for opioid use disorder: a meta-analysis and retrospective analysis of clinical trial data. Drug Alcohol Depend. (2022) 238:109575. doi: 10.1016/j.drugalcdep.2022.109575
30. National Academies of Sciences Engineering and Medicine In: M Mancher and AI Leshner, editors. Medications for opioid use disorder save lives. Washington, DC: National Academies Press (2019).
31. Langleben, DD, Busch, EL, O'Brien, CP, and Elman, I. Depot naltrexone decreases rewarding properties of sugar in patients with opioid dependence. Psychopharmacology. (2012) 220:559–64. doi: 10.1007/s00213-011-2503-1
32. Taveira, TH, Wu, WC, Tschibelu, E, Borsook, D, Simonson, DC, Yamamoto, R, et al. The effect of naltrexone on body fat mass in olanzapine-treated schizophrenic or schizoaffective patients: a randomized double-blind placebo-controlled pilot study. J Psychopharmacol. (2014) 28:395–400. doi: 10.1177/0269881113509904
33. Kurbanov, DB, Currie, PJ, Simonson, DC, Borsook, D, and Elman, I. Effects of naltrexone on food intake and body weight gain in olanzapine-treated rats. J Psychopharmacol. (2012) 26:1244–51. doi: 10.1177/0269881112450783
34. Dahlberg, S, Chang, ET, Weiss, SR, Dopart, P, Gould, E, and Ritchey, ME. Use of Contrave, naltrexone with bupropion, bupropion, or naltrexone and major adverse cardiovascular events: a systematic literature review. Diabetes Metab Syndr Obes. (2022) 15:3049–67. doi: 10.2147/DMSO.S381652
35. Tan, LA, Gajipara, N, Sun, L, Bacolod, M, Zhou, Y, Namchuk, M, et al. In vivo characterization of the opioid receptor-binding profiles of Samidorphan and naltrexone in rats: comparisons at clinically relevant concentrations. Neuropsychiatr Dis Treat. (2022) 18:2497–506. doi: 10.2147/NDT.S373195
36. Citrome, L, Graham, C, Simmons, A, Jiang, Y, Todtenkopf, MS, Silverman, B, et al. An evidence-based review of OLZ/SAM for treatment of adults with schizophrenia or bipolar I disorder. Neuropsychiatr Dis Treat. (2021) 17:2885–904. doi: 10.2147/NDT.S313840
37. Wang, AL, Elman, I, Lowen, SB, Blady, SJ, Lynch, KG, Hyatt, JM, et al. Neural correlates of adherence to extended-release naltrexone pharmacotherapy in heroin dependence. Transl Psychiatry. (2015) 5:e531. doi: 10.1038/tp.2015.20
38. Vrijens, B, De Geest, S, Hughes, DA, Przemyslaw, K, Demonceau, J, Ruppar, T, et al. A new taxonomy for describing and defining adherence to medications. Br J Clin Pharmacol. (2012) 73:691–705. doi: 10.1111/j.1365-2125.2012.04167.x
39. Shi, L, Liu, J, Koleva, Y, Fonseca, V, Kalsekar, A, and Pawaskar, M. Concordance of adherence measurement using self-reported adherence questionnaires and medication monitoring devices. PharmacoEconomics. (2010) 28:1097–107. doi: 10.2165/11537400-000000000-00000
40. Shiga, T, Kimura, T, Fukushima, N, Yoshiyama, Y, Iwade, K, Mori, F, et al. Effects of a pharmacist-led educational interventional program on electronic monitoring-assessed adherence to direct Oral anticoagulants: a randomized, controlled trial in patients with Nonvalvular atrial fibrillation. Clin Ther. (2022) 44:1494–505. doi: 10.1016/j.clinthera.2022.09.011
41. Lee, JY, Kusek, JW, Greene, PG, Bernhard, S, Norris, K, Smith, D, et al. Assessing medication adherence by pill count and electronic monitoring in the African American study of kidney disease and hypertension (AASK) pilot study. Am J Hypertens. (1996) 9:719–25. doi: 10.1016/0895-7061(96)00056-8
42. Dermody, SS, Wardell, JD, Stoner, SA, and Hendershot, CS. Predictors of daily adherence to naltrexone for alcohol use disorder treatment during a Mobile health intervention. Ann Behav Med. (2018) 52:787–97. doi: 10.1093/abm/kax053
43. Zimmerman, RS, Mehrotra, P, Madden, T, and Paul, R. The value of assessing self-reported and biological indicators of outcomes in evaluating HIV programs. Curr HIV/AIDS Rep. (2021) 18:365–76. doi: 10.1007/s11904-021-00560-3
44. Berg, KM, Litwin, A, Li, X, Heo, M, and Arnsten, JH. Directly observed antiretroviral therapy improves adherence and viral load in drug users attending methadone maintenance clinics: a randomized controlled trial. Drug Alcohol Depend. (2011) 113:192–9. doi: 10.1016/j.drugalcdep.2010.07.025
45. Steiner, JF, and Prochazka, AV. The assessment of refill compliance using pharmacy records: methods, validity, and applications. J Clin Epidemiol. (1997) 50:105–16. doi: 10.1016/S0895-4356(96)00268-5
46. Demonceau, J, Ruppar, T, Kristanto, P, Hughes, DA, Fargher, E, Kardas, P, et al. Identification and assessment of adherence-enhancing interventions in studies assessing medication adherence through electronically compiled drug dosing histories: a systematic literature review and meta-analysis. Drugs. (2013) 73:545–62. doi: 10.1007/s40265-013-0041-3
47. Birkhauer, J, Gaab, J, Kossowsky, J, Hasler, S, Krummenacher, P, Werner, C, et al. Trust in the health care professional and health outcome: a meta-analysis. PLoS One. (2017) 12:e0170988. doi: 10.1371/journal.pone.0170988
48. Elman, I, Krause, S, Breiter, HC, Gollub, RL, Heintges, J, Baumgartner, WA, et al. The validity of self-reported drug use in non-treatment seeking individuals with cocaine dependence: correlation with biochemical assays. Am J Addict. (2000) 9:216–21. doi: 10.1080/10550490050148044
49. Elman, I, Krause, S, Karlsgodt, K, Schoenfeld, DA, Gollub, RL, Breiter, HC, et al. Clinical outcomes following cocaine infusion in nontreatment-seeking individuals with cocaine dependence. Biol Psychiatry. (2001) 49:553–5. doi: 10.1016/S0006-3223(00)01096-9
50. Jarvis, BP, Holtyn, AF, Subramaniam, S, Tompkins, DA, Oga, EA, Bigelow, GE, et al. Extended-release injectable naltrexone for opioid use disorder: a systematic review. Addiction. (2018) 113:1188–209. doi: 10.1111/add.14180
51. Nunes, EV, Bisaga, A, Krupitsky, E, Nangia, N, Silverman, BL, Akerman, SC, et al. Opioid use and dropout from extended-release naltrexone in a controlled trial: implications for mechanism. Addiction. (2020) 115:239–46. doi: 10.1111/add.14735
52. Tanum, L, Solli, KK, Latif, ZE, Benth, JS, Opheim, A, Sharma-Haase, K, et al. Effectiveness of injectable extended-release naltrexone vs daily buprenorphine-naloxone for opioid dependence: a randomized clinical noninferiority trial. JAMA Psychiat. (2017) 74:1197–205. doi: 10.1001/jamapsychiatry.2017.3206
53. Oesterle, TS, Thusius, NJ, Rummans, TA, and Gold, MS. Medication-assisted treatment for opioid-use disorder. Mayo Clin Proc. (2019) 94:2072–86. doi: 10.1016/j.mayocp.2019.03.029
54. Srivastava, AB, and Gold, MS. Naltrexone: a history and future directions. Cerebrum. (2018) 2018:13–8.
55. White, JM, and Irvine, RJ. Mechanisms of fatal opioid overdose. Addiction. (1999) 94:961–72. doi: 10.1046/j.1360-0443.1999.9479612.x
56. Tempel, A, Gardner, EL, and Zukin, RS. Neurochemical and functional correlates of naltrexone-induced opiate receptor up-regulation. J Pharmacol Exp Ther. (1985) 232:439–44.
57. Merlo, LJ, Greene, WM, and Pomm, R. Mandatory naltrexone treatment prevents relapse among opiate-dependent anesthesiologists returning to practice. J Addict Med. (2011) 5:279–83. doi: 10.1097/ADM.0b013e31821852a0
58. Shi, Z, Jagannathan, K, Wang, AL, Fairchild, VP, Lynch, KG, Suh, JJ, et al. Behavioral and Accumbal responses during an affective go/no-go task predict adherence to injectable naltrexone treatment in opioid use disorder. Int J Neuropsychopharmacol. (2019) 22:180–5. doi: 10.1093/ijnp/pyz002
59. McDonald, HP, Garg, AX, and Haynes, RB. Interventions to enhance patient adherence to medication prescriptions: scientific review. JAMA. (2002) 288:2868–79. doi: 10.1001/jama.288.22.2868
60. Shi, Z, Langleben, DD, O'Brien, CP, Childress, AR, and Wiers, CE. Multivariate pattern analysis links drug use severity to distributed cortical hypoactivity during emotional inhibitory control in opioid use disorder. Neuroimage Clin. (2021) 32:102806. doi: 10.1016/j.nicl.2021.102806
61. Bursac, Z, Gauss, CH, Williams, DK, and Hosmer, DW. Purposeful selection of variables in logistic regression. Source Code Biol Med. (2008) 3:17. doi: 10.1186/1751-0473-3-17
62. Hauck, WW, and Donner, A. Wald's test as applied to hypotheses in logit analysis. J Am Stat Assoc. (1977) 72:851–3. doi: 10.2307/2286473
63. Lafontaine, F, and White, KJ. Obtaining any Wald statistic you want. Econ Lett. (1986) 21:35–40. doi: 10.1016/0165-1765(86)90117-5
64. Kahneman, D, and Tversky, A. Prospect theory: an analysis of decision under risk. Econometrica. (1979) 47:263–91. doi: 10.2307/1914185
65. Kahneman, D, and Miller, DT. Norm theory: comparing reality to its alternatives. Psychol Rev. (1986) 93:136–53. doi: 10.1037/0033-295X.93.2.136
66. Elman, I, Ariely, D, Tsoy-Podosenin, M, Verbitskaya, E, Wahlgren, V, Wang, A-L, et al. Contextual processing and its alterations in patients with addictive disorders. Addict Neurosci. (2023) 7:100100. doi: 10.1016/j.addicn.2023.100100
67. Elman, I, Upadhyay, J, Lowen, S, Karunakaran, K, Albanese, M, and Borsook, D. Mechanisms underlying unconscious processing and their alterations in post-traumatic stress disorder: neuroimaging of zero monetary outcomes contextually framed as “no losses” vs. “no gains”. Front Neurosci. (2020) 14:604867. doi: 10.3389/fnins.2020.604867
68. Elman, I, Lowen, S, Frederick, BB, Chi, W, Becerra, L, and Pitman, RK. Functional neuroimaging of reward circuitry responsivity to monetary gains and losses in posttraumatic stress disorder. Biol Psychiatry. (2009) 66:1083–90. doi: 10.1016/j.biopsych.2009.06.006
69. Ariely, D, Huber, J, and Wertenbroch, K. When do losses loom larger than gains? J Mark Res. (2005) 42:134–8. doi: 10.1509/jmkr.42.2.134.62283
70. Hayward, LE, Vartanian, LR, and Pinkus, RT. Weight stigma predicts poorer psychological well-being through internalized weight Bias and maladaptive coping responses. Obesity (Silver Spring). (2018) 26:755–61. doi: 10.1002/oby.22126
71. Hemmingsson, E. A new model of the role of psychological and emotional distress in promoting obesity: conceptual review with implications for treatment and prevention. Obes Rev. (2014) 15:769–79. doi: 10.1111/obr.12197
72. Avena, NM, and Gold, MS. Food and addiction - sugars, fats and hedonic overeating. Addiction. (2011) 106:1214–5. doi: 10.1111/j.1360-0443.2011.03373.x
73. Gearhardt, AN, and DiFeliceantonio, AG. Highly processed foods can be considered addictive substances based on established scientific criteria. Addiction. (2023) 118:589–98. doi: 10.1111/add.16065
74. Avena, NM, Rada, P, and Hoebel, BG. Evidence for sugar addiction: behavioral and neurochemical effects of intermittent, excessive sugar intake. Neurosci Biobehav Rev. (2008) 32:20–39. doi: 10.1016/j.neubiorev.2007.04.019
75. Avena, NM, and Hoebel, BG. Amphetamine-sensitized rats show sugar-induced hyperactivity (cross-sensitization) and sugar hyperphagia. Pharmacol Biochem Behav. (2003) 74:635–9. doi: 10.1016/S0091-3057(02)01050-X
76. Blum, K, Kazmi, S, Modestino, EJ, Downs, BW, Bagchi, D, Baron, D, et al. A novel precision approach to overcome the "addiction pandemic" by incorporating genetic addiction risk severity (GARS) and dopamine homeostasis restoration. J Pers Med. (2021) 11:212. doi: 10.3390/jpm11030212
77. Vanbuskirk, KA, and Potenza, MN. The treatment of obesity and its co-occurrence with substance use disorders. J Addict Med. (2010) 4:1–10. doi: 10.1097/ADM.0b013e3181ce38e7
78. Mendoza-Herrera, K, Florio, AA, Moore, M, Marrero, A, Tamez, M, Bhupathiraju, SN, et al. The leptin system and diet: a Mini review of the current evidence. Front Endocrinol (Lausanne). (2021) 12:749050. doi: 10.3389/fendo.2021.749050
79. Zhao, S, Zhu, Y, Schultz, RD, Li, N, He, Z, Zhang, Z, et al. Partial leptin reduction as an insulin sensitization and weight loss strategy. Cell Metab. (2019) 30:706–19.e6. doi: 10.1016/j.cmet.2019.08.005
80. Figlewicz, DP. Modulation of food reward by endocrine and environmental factors: update and perspective. Psychosom Med. (2015) 77:664–70. doi: 10.1097/PSY.0000000000000146
81. Stice, E, Figlewicz, DP, Gosnell, BA, Levine, AS, and Pratt, WE. The contribution of brain reward circuits to the obesity epidemic. Neurosci Biobehav Rev. (2013) 37:2047–58. doi: 10.1016/j.neubiorev.2012.12.001
82. Hanley, MJ, Abernethy, DR, and Greenblatt, DJ. Effect of obesity on the pharmacokinetics of drugs in humans. Clin Pharmacokinet. (2010) 49:71–87. doi: 10.2165/11318100-000000000-00000
83. Lee, A, Cardel, M, and Donahoo, WT. Social and environmental factors influencing obesity In: KR Feingold, B Anawalt, MR Blackman, A Boyce, G Chrousos, and E Corpas, editors. Endotext. South Dartmouth, MA: MDText.com, Inc. (2000).
84. Tang, AM, Forrester, JE, Spiegelman, D, Flanigan, T, Dobs, A, Skinner, S, et al. Heavy injection drug use is associated with lower percent body fat in a multi-ethnic cohort of HIV-positive and HIV-negative drug users from three U.S. cities. Am J Drug Alcohol Abuse. (2010) 36:78–86. doi: 10.3109/00952990903544851
85. Chavez, MN, and Rigg, KK. Nutritional implications of opioid use disorder: a guide for drug treatment providers. Psychol Addict Behav. (2020) 34:699–707. doi: 10.1037/adb0000575
86. McHugh, RK, Fitzmaurice, GM, Carroll, KM, Griffin, ML, Hill, KP, Wasan, AD, et al. Assessing craving and its relationship to subsequent prescription opioid use among treatment-seeking prescription opioid dependent patients. Drug Alcohol Depend. (2014) 145:121–6. doi: 10.1016/j.drugalcdep.2014.10.002
87. Tsui, JI, Anderson, BJ, Strong, DR, and Stein, MD. Craving predicts opioid use in opioid-dependent patients initiating buprenorphine treatment: a longitudinal study. Am J Drug Alcohol Abuse. (2014) 40:163–9. doi: 10.3109/00952990.2013.848875
88. Hulse, GK, Ngo, HT, and Tait, RJ. Risk factors for craving and relapse in heroin users treated with oral or implant naltrexone. Biol Psychiatry. (2010) 68:296–302. doi: 10.1016/j.biopsych.2010.04.003
89. Paliwal, P, Hyman, SM, and Sinha, R. Craving predicts time to cocaine relapse: further validation of the now and brief versions of the cocaine craving questionnaire. Drug Alcohol Depend. (2008) 93:252–9. doi: 10.1016/j.drugalcdep.2007.10.002
90. Hartz, DT, Frederick-Osborne, SL, and Galloway, GP. Craving predicts use during treatment for methamphetamine dependence: a prospective, repeated-measures, within-subject analysis. Drug Alcohol Depend. (2001) 63:269–76. doi: 10.1016/S0376-8716(00)00217-9
91. Kakko, J, Alho, H, Baldacchino, A, Molina, R, Nava, FA, and Shaya, G. Craving in opioid use disorder: from neurobiology to clinical practice. Front Psych. (2019) 10:592. doi: 10.3389/fpsyt.2019.00592
92. Elman, I, Lukas, SE, Karlsgodt, KH, Gasic, GP, and Breiter, HC. Acute cortisol administration triggers craving in individuals with cocaine dependence. Psychopharmacol Bull. (2003) 37:84–9.
Keywords: opioid use disorder, medication-assisted treatment, body weight gain, addiction, food, obesity, eating
Citation: Li X, Langleben DD, Lynch KG, Wang G-J, Elman I, Wiers CE and Shi Z (2023) Association between body mass index and treatment completion in extended-release naltrexone-treated patients with opioid dependence. Front. Psychiatry. 14:1247961. doi: 10.3389/fpsyt.2023.1247961
Edited by:
Anthony DeFulio, Western Michigan University, United StatesReviewed by:
Mark Gold, Washington University in St. Louis, United StatesAnil K. Bachu, Baptist Health Foundation, United States
Copyright © 2023 Li, Langleben, Lynch, Wang, Elman, Wiers and Shi. This is an open-access article distributed under the terms of the Creative Commons Attribution License (CC BY). The use, distribution or reproduction in other forums is permitted, provided the original author(s) and the copyright owner(s) are credited and that the original publication in this journal is cited, in accordance with accepted academic practice. No use, distribution or reproduction is permitted which does not comply with these terms.
*Correspondence: Corinde E. Wiers, Y29yaW5kZS53aWVyc0BwZW5ubWVkaWNpbmUudXBlbm4uZWR1; Zhenhao Shi, emhzaGlAcGVubm1lZGljaW5lLnVwZW5uLmVkdQ==
†These authors have contributed equally to this work and share last authorship