Introduction
During the last century, the term “quantum engineering” has adopted very different meanings. In early appearances it often implied the construction quantum systems—e.g., engineering of optical properties through controlled quantum dynamics (Rosencher et al., 1996), atom-by-atom design of nanostructures (Fernández Rossier, 2013), or hybridization of existing quantum objects (Wallquist et al., 2009)—or to the preparation of specific quantum states—e.g., engineering of intrinsically quantum states in trapped ions (Poyatos et al., 1997) or cavity-QED setups (Haroche, 1999). More recently, the quantum engineering have begun to denote a field of reserach, covering either narrow scopes around quantum information tasks (Smith, 2018; Asfaw et al., 2022) or a very broad description that includes all quantum technologies (Dzurak et al., 2022).
In this work we refer to quantum engineering as the field devoted to the fabrication, control and characterization of quantum systems with an intrinsically quantum dynamics. In this sense, quantum engineering traverses all areas of quantum technologies, including communication, computing, simulation, metrology and sensing, and also impacts other areas of basic and applied science, where the control of the quantum dynamics and quantum systems brings out new phenomena. Quantum engineering uses the language of quantum information science as a toolbox to understand and design complex quantum states and quantum operations, but it also builds on the tools from quantum control, quantum optics and many-body physics.
Inspired by other areas of engineering, as in the work by Zagoskin (2017), one may structure quantum engineering in a bottom-up approach (c.f. Figure 1), according to the degree of complexity of the objects involved: 1) the design and operation of individual quantum units, 2) the engineering of interactions between such units, 3) the combination of those structures into operational devices for communication, computing or sensing, or new emerging structures, 4) and the creation of interfaces between quantum, classical or hybrid devices according to systems engineering. Alternatively, we can focus on the tasks at hand: 1) fabrication, 2) operation and control, and 3) characterization. In the following text we highlight different challenges in several of these possible subdivisions.
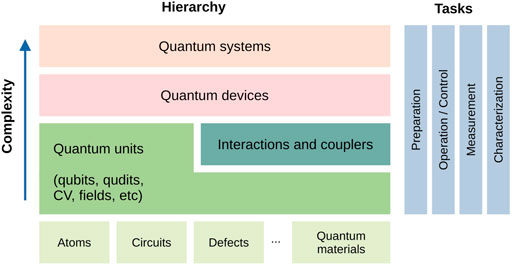
FIGURE 1. The field of Quantum Engineering, organized by the degree of complexity of the objects it deals with, and by the tasks it aims to perform.
Quantum units
The starting point of all quantum technologies is the “isolation” of elementary quantum degrees of freedom that can be prepared, controled and measured. Quantum information theory introduced the notions of qubits and continuous variables as paradigms that can be engineered and operated in systematic ways, and which may be reproduced in various physical systems. Indeed, the literature is now abundand in physical realizations of qubits using trapped ions, quantum dots, Rydberg atoms and other nonlinear quantum systems, and of continuous variables using photonic, phononic and optomechanic systems, to name some platforms.
The present challenges in this scale of complexity revolve around the optimization of existing qubits, improving their coherence properties and their operation speeds and fidelities. For instance, while superconducting circuits seem to be ahead of the quantum computing race in terms of scalability and reproducibility, they would still benefit from better readout fidelities (Sunada et al., 2022) and qubit coherence times (Place et al., 2021). However, we must accept that some platforms might face unsolvable obstacles, such as the impact of cosmic radiation in solid-state qubits (Cardani et al., 2021), that require still unforeseen paradigm shifts.
We must therefore continue searching for newer and better quantum units. A promising path for this is looking for emergent of robust quantum units arising from the combination of large numbers of imperfect components. Such is the case of topological systems, including the well-known Majorana modes and other anyonic fermionic systems, platforms that may sustain universal and intrinsically protected quantum information processing (Lahtinen and Pachos, 2017). Equally interesting is the engineering of higher-dimensional quantum systems, such as magnetic molecules (Gaita-Ariño et al., 2019) and the prospect for building self-assembled qudit arrays with them. However, this is an area of development that is held back by our limited understanding of qudit-based quantum information processing.
Engineering of interactions and quantum materials
Most quantum technologies are based on combining multiple quantum units, through connections or interactions that augment their capabilities, with the help of an exponentially larger Hilbert space and the available entanglement.
In some cases, the challenge of this composition lays in the engineering of simple but selective interactions that are very accurately controlled in time and space. We achieve selectivity in time, for instance by developing tuneable couplers that enable isolated pairs of superconducting qubits to correlate with each other, cancelling other spurious crost-talk interactions (Arute et al., 2019). We achieve spatial control of interactions, for instance by using tweezers to bring ultracold atoms close together, to enable topologies that are consistent with the desired algorithms or error correction models (Bluvstein et al., 2022).
A notch up in complexity we find quantum simulations, a subfield centered around the difficult task of engineering more complex interactions to reproduce Hamiltonian that address important theoretical challenges: e.g., understanding many-body localization, the mechanisms for high-Tc superconductivity, dynamical lattice gauge theory models with complex many-body interactions, or topological order beyond topological insulators. The realization of such models has been long centered around AMO setups that can flexibily implement fermionic and bosonic, matter and field degrees of fredom (Zohar et al., 2015). Despite great progress in recent experiments (Schweizer et al., 2019), the quest for new models is always an open problem, as it is facing the practical challenges of preparing ground states, or combining interactions with Floquet engineering, as explained below.
However, while the search for complex models has strongly focused on the AMO platforms, the state-of-the-art in quantum fabrication and engineering justifies considering the development of new ad-hoc quantum materials that fulfill some of the above mentioned tasks—from emergent qubits, to quantum simulators and quantum sensors. This new field of research sports a variety of technologies such as the atom-by-atom design of structures—from implanting NV centers in diamond or phosphorous quantum dots in Si, to single-atom transistors—, functionalizing materials for quantum tasks, designing and exploring the physics of 2D quantum materials, or creating hybrid structures that combine many of above mentioned quantum units—quantum emitters, nanophotonic structures, superconducting metamaterials and resonators, etc—for specific purposes or for exploring emergent phenomena.
Quantum control of quantum devices
In early works, the notion of quantum engineering has been often associated to the systematic control of a quantum system’s dynamics. Indeed, it may be argued that control theory is the most relevant tool in quantum engineering, with applications that include the cancellation of noise in qubits, the systematic construction of quantum operations by combination of elementary gates or the physical implementation of said gates by controlling qubits with different external fields.
At the lowest level of complexity we find quasi-static and periodic controls, aiming at simulation of complex quantum interactions that are both useful and demonstrably hard. In this area, Floquet engineering has produced remarkable outcomes in the field of ultracold atoms (Weitenberg and Simonet, 2021), but as mentioned above, work is still required to increase the complexity of the models and handle the intrinsic challenges imposed by these control methods, such as interactions and cooling.
At a highest level of complexity we find the overwhelming family of quantum optimal control methods. This is a field that has grown in ambition, starting from original works that aimed at controlling isolated states with arbitrary pulses—e.g., those provided by Krotov methods (Tannor et al., 1992)—, evolving to work with open systems (Koch, 2016), and incorporate new technologies that aim for experimentally-friendly controls with limited bandwidths (Romero-Isart and García-Ripoll, 2007; Motzoi et al., 2011; Koch, 2016; Machnes et al., 2018; Müller et al., 2022). In this context one must highlight the development of new paradigms in the field of control theory, covered by the umbrella term of shortcuts to adiabaticity (Guéry-Odelin et al., 2019). Under this denomination we find a large family of techniques—fast quasi-adiabatic techniques, quantum invariants, counteradiabatic methods, etc—that create sometimes arbitrarily fast controls with intrinsic guarantees of robustness, and often resilient to experimental distortions (García-Ripoll et al., 2020).
Beyond the theoretical progress in the art of control theory, we must consider seriously how to integrate these methods with existing experiments, and whether machine learning methods may help in this connection. The bridge between theory and experiments may relay onunifying open and closed loop control methods with physics-informed machine learning (Weitenberg and Simonet, 2021) or eventually evolving towards black-box, computationally intensive methods such as reinforcement learning (Niu et al., 2019). The answers to these questions are deeply connected to the systems design issues discussed next.
Quantum systems
The combination of quantum units, with their native and engineered interactions, and with the protocols to operate them, gives rise to new quantum devices that are arguably very useful, both in the lab and as standalone technologies. However, with the advent of these quantum computers, simulators, networks and sensors, it also arises the need for a new type of systems engineering that regards both the interaction between these devices with our classical world, as well as among quantum devices themselves.
On the quantum-classical front we already have witnessed very relevant achievements in the development of cloud-native quantum computing infrastructures. These highly scalable stacks integrate the quantum computers with various layers of software that control everything, from the design of individual optical or microwave pulses to implement gates, up to the high-level programming of jobs and user management (Bandic et al., 2022).
We must remark the intrinsic classical challenge already posed by piling up multiple quantum units in a single quantum device, where each of those units requires individual controls and measurements. Let us consider the operation of a Rydberg atom quantum computer. While existing machines may trap 100s of such qubits, implementing local gates in each of these qubits demands either long times—if the gates are to be made sequentially—or huge resources in laser and electronics—if each qubit is to have its own control. This is not unique to one platform: the same problems arises in superconducting qubits, semiconductor qubits, trapped ions, NV-centers, etc.
To address this challenge, we expect deeper levels of quantum-classical integration, with the advent of in-chip DACs and control logic (Johnson et al., 2010), that alleviate the impressive overhead that arises from the local control of qubits, and improve the scalability. This technology must be accompanied by further interdisciplinary work where quantum engineers interact with electronic and software engineers to systematically address the scalable and robust callibration of the quantum devices the compilation and implementation of quantum algorithms as elementary gates, and ultimately the development of complete quantum operating systems.
On the quantum-quantum front, the central question is the actual networking and interfacing of quantum systems. On the short term, we already need quantum links between quantum processors (Kurpiers et al., 2018; Chang et al., 2020) that help in scaling up our NISQ resources. On the long term the consensus vision aims at fully networked quantum systems, where quantum computers exchange quantum information among themselves, and also collect and process the information coming from quantum memories and quantum sensors. This vision poses relevant challenges in transducing information in and out of quantum computers, in routing that information according to quantum rules (not just classical paradigms), and in storing and retrieving information from the quantum equivalent of RAM’s—which may or may not end up looking like the formal standards (Giovannetti et al., 2008). How we scalably store, exchange and route quantum information is a physical, informational and an engineering problem, the surface of which have barely scratched.
Conclusion
As briefly discussed above, quantum engineering is an emergent interdisciplinary area of research that covers all levels of complexity and application of quantum technologies, from its fundamental components up to the development of fully integrated networks of devices. At each level of complexity and type of application, the field opens numerous challenges with a great potential impact in the maturity and scalability of quantum technologies. Simultaneously, we expect that the systematization of the tools and language of quantum engineer will provide a fertile ground for interdisciplinary collaboration between physicists, material scientists, electronics engineers, software engineers and other areas of development.
Author contributions
The author confirms being the sole contributor of this work and has approved it for publication.
Conflict of interest
The author declares that the research was conducted in the absence of any commercial or financial relationships that could be construed as a potential conflict of interest.
Publisher’s note
All claims expressed in this article are solely those of the authors and do not necessarily represent those of their affiliated organizations, or those of the publisher, the editors and the reviewers. Any product that may be evaluated in this article, or claim that may be made by its manufacturer, is not guaranteed or endorsed by the publisher.
References
Arute, F., Arya, K., Babbush, R., Bacon, D., Bardin, J. C., Barends, R., et al. (2019). Quantum supremacy using a programmable superconducting processor. Nature 574, 505–510. doi:10.1038/s41586-019-1666-5
Asfaw, A., Blais, A., Brown, K. R., Candelaria, J., Cantwell, C., Carr, L. D., et al. (2022). Building a quantum engineering undergraduate program. IEEE Trans. Ed. 65, 220–242. doi:10.1109/TE.2022.3144943
Bandic, M., Feld, S., and Almudever, C. G. (2022). “Full-stack quantum computing systems in the NISQ era: Algorithm-driven and hardware-aware compilation techniques,” in 2022 Design, Automation & Test in Europe Conference & Exhibition (DATE), 14 - 23 March 2022. 1–6. doi:10.23919/DATE54114.2022.9774643
Bluvstein, D., Levine, H., Semeghini, G., Wang, T. T., Ebadi, S., Kalinowski, M., et al. (2022). A quantum processor based on coherent transport of entangled atom arrays. Nature 604, 451–456. doi:10.1038/s41586-022-04592-6
Cardani, L., Valenti, F., Casali, N., Catelani, G., Charpentier, T., Clemenza, M., et al. (2021). Reducing the impact of radioactivity on quantum circuits in a deep-underground facility. Nat. Commun. 12, 2733. doi:10.1038/s41467-021-23032-z
Chang, H.-S., Zhong, Y. P., Bienfait, A., Chou, M.-H., Conner, C. R., Dumur, É., et al. (2020). Remote entanglement via adiabatic passage using a tunably dissipative quantum communication system. Phys. Rev. Lett. 124, 240502. doi:10.1103/PhysRevLett.124.240502
Dzurak, A. S., Epps, J., Laucht, A., Malaney, R., Morello, A., Nurdin, H. I., et al. (2022). Development of an undergraduate quantum engineering degree. IEEE Trans. Quantum Eng. 3, 1–10. doi:10.1109/TQE.2022.3157338
Gaita-Ariño, A., Luis, F., Hill, S., and Coronado, E. (2019). Molecular spins for quantum computation. Nat. Chem. 11, 301–309. doi:10.1038/s41557-019-0232-y
García-Ripoll, J., Ruiz-Chamorro, A., and Torrontegui, E. (2020). Quantum control of frequency-tunable transmon superconducting qubits. Phys. Rev. Appl. 14, 044035. doi:10.1103/PhysRevApplied.14.044035
Giovannetti, V., Lloyd, S., and Maccone, L. (2008). Quantum random access memory. Phys. Rev. Lett. 100, 160501. doi:10.1103/PhysRevLett.100.160501
Guéry-Odelin, D., Ruschhaupt, A., Kiely, A., Torrontegui, E., Martínez-Garaot, S., and Muga, J. G. (2019). Shortcuts to adiabaticity: Concepts, methods, and applications. Rev. Mod. Phys. 91, 045001. doi:10.1103/RevModPhys.91.045001
Haroche, S. (1999). “Quantum engineering with atoms and photons in a cavity,” in Epistemological and experimental perspectives on quantum physics. Editors D. Greenberger, W. L. Reiter, and A. Zeilinger (Dordrecht: Springer Netherlands Vienna Circle Institute Yearbook), 155–166. doi:10.1007/978-94-017-1454-9_12
Johnson, M. W., Bunyk, P., Maibaum, F., Tolkacheva, E., Berkley, A. J., Chapple, E. M., et al. (2010). A scalable control system for a superconducting adiabatic quantum optimization processor. Supercond. Sci. Technol. 23, 065004. doi:10.1088/0953-2048/23/6/065004
Koch, C. P. (2016). Controlling open quantum systems: Tools, achievements, and limitations. J. Phys. Condens. Matter 28, 213001. doi:10.1088/0953-8984/28/21/213001
Kurpiers, P., Magnard, P., Walter, T., Royer, B., Pechal, M., Heinsoo, J., et al. (2018). Deterministic quantum state transfer and remote entanglement using microwave photons. Nature 558, 264–267. doi:10.1038/s41586-018-0195-y
Lahtinen, V., and Pachos, J. (2017). A short introduction to topological quantum computation. SciPost Phys. 3, 021. doi:10.21468/SciPostPhys.3.3.021
Machnes, S., Assémat, E., Tannor, D., and Wilhelm, F. K. (2018). Tunable, flexible, and efficient optimization of control pulses for practical qubits. Phys. Rev. Lett. 120, 150401. doi:10.1103/PhysRevLett.120.150401
Motzoi, F., Gambetta, J. M., Merkel, S. T., and Wilhelm, F. K. (2011). Optimal control methods for rapidly time-varying Hamiltonians. Phys. Rev. A . Coll. Park. 84, 022307. doi:10.1103/PhysRevA.84.022307
Müller, M. M., Said, R. S., Jelezko, F., Calarco, T., and Montangero, S. (2022). One decade of quantum optimal control in the chopped random basis. Rep. Prog. Phys. 85, 076001. doi:10.1088/1361-6633/ac723c
Niu, M. Y., Boixo, S., Smelyanskiy, V. N., and Neven, H. (2019). Universal quantum control through deep reinforcement learning. npj Quantum Inf. 5, 33–38. doi:10.1038/s41534-019-0141-3
Place, A. P. M., Rodgers, L. V. H., Mundada, P., Smitham, B. M., Fitzpatrick, M., Leng, Z., et al. (2021). New material platform for superconducting transmon qubits with coherence times exceeding 0.3 milliseconds. Nat. Commun. 12, 1779. doi:10.1038/s41467-021-22030-5
Poyatos, J. F., Gardiner, S. A., Walser, R., Cirac, J. I., Zoller, P., and Blatt, R. (1997). “Quantum engineering with trapped ions,”in New developments on fundamental problems in quantum physics. Editors M. Ferrero, and A. van der Merwe (Dordrecht: Springer Netherlands Fundamental Theories of Physics), 317–323. doi:10.1007/978-94-011-5886-2_40
Romero-Isart, O., and García-Ripoll, J. J. (2007). Quantum ratchets for quantum communication with optical superlattices. Phys. Rev. A . Coll. Park. 76, 052304. doi:10.1103/PhysRevA.76.052304
Rosencher, E., Fiore, A., Vinter, B., Berger, V., Bois, P., and Nagle, J. (1996). Quantum engineering of optical nonlinearities. Science 271, 168–173. doi:10.1126/science.271.5246.168
Schweizer, C., Grusdt, F., Berngruber, M., Barbiero, L., Demler, E., Goldman, N., et al. (2019). Floquet approach to ℤ2 lattice gauge theories with ultracold atoms in optical lattices Z2 lattice gauge theories with ultracold atoms in optical lattices. Nat. Phys. 15, 1168–1173. doi:10.1038/s41567-019-0649-7
Smith, L. (2018). National quantum initiative act. Available at: http://www.congress.gov/
Sunada, Y., Kono, S., Ilves, J., Tamate, S., Sugiyama, T., Tabuchi, Y., et al. (2022). Fast readout and reset of a superconducting qubit coupled to a resonator with an intrinsic purcell filter. Phys. Rev. Appl. 17, 044016. doi:10.1103/PhysRevApplied.17.044016
Tannor, D. J., Kazakov, V., and Orlov, V. (1992). “Control of photochemical branching: Novel procedures for finding optimal pulses and global upper bounds,” in Time-dependent quantum molecular dynamics. Editors J. Broeckhove, and L. Lathouwers (Boston, MA: Springer US Nato ASI Series), 347–360. doi:10.1007/978-1-4899-2326-4_24
Wallquist, M., Hammerer, K., Rabl, P., Lukin, M., and Zoller, P. (2009). Hybrid quantum devices and quantum engineering. Phys. Scr. T137, 014001. doi:10.1088/0031-8949/2009/T137/014001
Weitenberg, C., and Simonet, J. (2021). Tailoring quantum gases by Floquet engineering. Nat. Phys. 17, 1342–1348. doi:10.1038/s41567-021-01316-x
Zagoskin, A. (2017). Quantum engineering of superconducting structures: Principles, promise and problems. Low. Temp. Phys. 43, 751–755. doi:10.1063/1.4995621
Keywords: quantum engineering, quantum computing, quantum simulation, quantum communication, quantum sensing
Citation: García-Ripoll JJ (2022) Specialty Grand Challenge: Quantum engineering. Front. Quantum. Sci. Technol. 1:1029525. doi: 10.3389/frqst.2022.1029525
Received: 27 August 2022; Accepted: 07 September 2022;
Published: 23 September 2022.
Edited and reviewed by:
Fedor Jelezko, University of Ulm, GermanyCopyright © 2022 García-Ripoll. This is an open-access article distributed under the terms of the Creative Commons Attribution License (CC BY). The use, distribution or reproduction in other forums is permitted, provided the original author(s) and the copyright owner(s) are credited and that the original publication in this journal is cited, in accordance with accepted academic practice. No use, distribution or reproduction is permitted which does not comply with these terms.
*Correspondence: Juan José García-Ripoll, jj.garcia.ripoll@csic.es