- School of Environmental Sciences, Jawaharlal Nehru University, New Delhi, India
Warmer global climate and urban heat islands (UHIs) interact, by exacerbating heatwaves and increasing the extreme heat days in cities. The implications of added heat stress in urban environments due to intensifying surface UHIs (SUHIs) is of utmost concern. Seasonal, annual and decadal nighttime SUHI intensities (SUHIIs), from 2001 to 2020, for nine major populated cities of India are analyzed. This includes five megacities- Delhi, Mumbai, Kolkata, Bangalore, and Chennai, and four incipient megacities- Hyderabad, Ahmedabad, Surat, and Pune. The key role of increasing urbanization (pre- and post-2010) in expansion and intensification of nighttime SUHIs in India is highlighted. For all cities either pre-monsoon (MAM) or winter (December-February; DJF) seasons show the strongest SUHII development. During the 2001–2010, and the 2011–2020 decade, a nighttime SUHII maxima of respectively (i) 2.1°C and 2.5°C for Delhi, (ii) 1.3°C and 1.5°C for Mumbai, (iii) 1.3°C and 1.5°C for Kolkata, (iv) 0.6°C and 1.0°C Bangalore, (v) 1.7°C and 1.9°C for Chennai, (vi) 1.8°C and 2.3°C for Hyderabad, (vii) 2.8°C and 3.1°C for Ahmedabad, (viii) 1.9°C and 2.4°C for Surat, and (ix) 0.8°C and 1.3°C for Pune is noted. Further, all incipient megacities showed a mean annual growth rate of nighttime SUHII of over 0.007°C/year, substantially greater than in the megacities. High SUHII magnitudes, greater growth rates of SUHII, and huge populations, severely compounds the vulnerability of Indian cities to excessive heat exposure risk, especially during MAM heatwaves. Lastly, the implications of nighttime SUHII findings from the present study, on the increase in heat stress, the loss of labor productivity and the rise in heat-related mortality rate is emphasized. The study recommends implementation of city-specific action plans to mitigate the heat stressed urban environment. Targeted use of cooling strategies in localized hotspots within the urban areas where high intensity SUHIs are likely to form is also suggested.
1. Introduction
The global population crossed 8 billion in the end of the year 2022 and according to the latest United Nations (UN) publication on the “World Population Prospects,” the global population is expected to increase by another half a billion by 2030 (United Nations (UN), 2022). In 2022, just two countries- India and China accounted for over 2.8 billion people, and in 2023, India with a projected population of over 1.6 billion, is expected to overthrow China to become the most populous country in the world (United Nations (UN), 2022). At present, India has five megacities (i.e., cities with population >10 million) viz., Delhi (28.5 million), Mumbai (19.9 million), Kolkata (14.6 million), Bangalore (11.4 million), and Chennai (10.4 million) (United Nations (UN), 2018a). In addition to these five megacities, India also has four rapidly growing incipient megacities viz., Hyderabad (9.4 million), Ahmedabad (7.6 million), Surat (6.5 million), and Pune (6.2 million). Studies point that recently turned megacities- Bangalore and Chennai, followed the urbanization patterns of older Indian megacities, such as, Delhi and Mumbai remarkably well (Taubenböck et al., 2009, 2012; Jain et al., 2016; Ghosh and Das, 2017). Similar trends in urban growth are also expected to be followed by the other incipient megacities of India, throughout the next decade (Taubenböck et al., 2010; United Nations (UN), 2018a).
The sheer enormous magnitude of population in just these nine Indian cities- Delhi, Mumbai, Kolkata, Bangalore, Chennai, Hyderabad, Ahmedabad, Surat, and Pune, can be judged from the fact that they inhabited a total of 1.1 billion people in 2018. This roughly amounted to one-seventh of the world's entire population, and further these nine Indian cities are projected to inhabit over 1.5 billion people by the year 2030 (United Nations (UN), 2018a). From a climate risk and disaster mitigation point of view, cities of such humongous populations are inherently vulnerable to huge losses of life and property in the events of natural calamities, extreme event occurrences, or during any climate change related disasters (Intergovernmental Panel on Climate Change (IPCC), 2012; Tippett, 2018; United Nations (UN), 2018b; Eckstein et al., 2019; National Institute of Disaster Management (NIDM), 2019; Jain, 2022). In the two decades from 1999 to 2018, Eckstein et al. (2019) found records of over 12,000 extreme event occurrences globally, which resulted in the direct loss of lives of about 500,000 people, and financial damages amounting to approximately USD 3.5 trillion.
Studies have found a significant increase in frequency, severity and intensity of climate extremes e.g., droughts, heatwaves, wildfires, hurricanes, and floods; which likely will exacerbate life and property losses in the future, along with causing food shortages and an increase in disease causing pathogens (Robinson, 2001; Black et al., 2004; Li et al., 2009; Dai, 2011; Intergovernmental Panel on Climate Change (IPCC), 2012, 2014; Mishra et al., 2017; Hawcroft et al., 2018; Bisht et al., 2019; Hasegawa et al., 2021; Jain, 2021a, 2022; Jain et al., 2021; Climate Transparency, 2022). Unlike the other climate extreme events, heatwave caused fatalities are not immediately obvious, but lead the weather-related cause of deaths globally (Hajat and Kosatky, 2010; Peterson et al., 2013; World Health Organization (WHO), 2020). Heatwave occurrences are of particular interest in cities, as the urbanized (or built-up) areas in cities are, typically, warmer by 1–4°C than their nearby rural surroundings, a phenomenon extensively documented as the urban heat island (UHI) effect (Oke, 1973; Voogt and Oke, 2003; Parker, 2010; Peng et al., 2012; Nuruzzaman, 2015; Shastri et al., 2017; Zhou and Chen, 2018; Anjos et al., 2020). In 2015, an excess of 175 million people, globally, suffered from heatwave exposure compared to the average number of people exposed to heatwaves during previous years (World Health Organization (WHO), 2020). Moreover, the 95th percentile global heatwave exposure is projected (RCP8.5-SSP3 scenario) to be 18–37 times more during 2071–2100 than it was century ago (1971–2000) (Liu et al., 2017). Both, inland Indian cities, such as, Delhi, Ahmedabad, and Hyderabad, and coastal Indian cities, such as, Mumbai, Kolkata, and Chennai are severely impacted by heatwaves. The infamous 2015 Indian subcontinent heatwave killed over 2,500 and 7,00 people in India and Pakistan, respectively (Wehner et al., 2016). Moreover, Mishra et al. (2017) stresses that a 2°C increase in global temperatures will cause a tremendous increase in occurrence of severe heatwaves, such as the 2015 Indian subcontinent heatwave, by almost 15 times by the next 30 years and by an astounding 92 times by the end of this century. Against the backdrop of continuously rising global temperatures and increasing extreme heat events, the heat stress in cities is further compounded by the spatial expansion and increasing intensity of UHIs, especially during the night (Intergovernmental Panel on Climate Change (IPCC), 2012, 2014; Jain, 2021b, 2022; Shreevastava et al., 2021).
Traditionally, the UHI intensity (UHII) is computed as the difference in the 2m air temperature, measured at two separate weather stations, each located at a fair distance from each other, such that they can distinctly be characterized as “urban” or “rural,” and yet fall within the same geographical and climatic set-up (Oke, 1973, 1997; Arnfield, 2003; Voogt and Oke, 2003). However, with the availability of freely accessible remote sensing and satellite data, it has become easier for urban meteorologists and urban climate scientists to study both, the daytime and the nighttime UHIs across the world (Peng et al., 2012; Zhou et al., 2014; Shastri et al., 2017; Liu et al., 2021; Siddiqui et al., 2021). Since satellite based UHI estimations use land surface temperature (LST) instead of 2 m air temperature, the term surface UHI (or SUHI) is used instead of UHI. A major benefit of SUHI over UHI is that satellite based LST data is two-dimensional (gridded data) while weather station based 2 m air temperature is one-dimensional (point data). Thus, SUHI assessments come in handy when questions overlooking- (1) mapping of SUHI footprint and spatial expansion of SUHI over time in a city, (2) identification of microclimate SUHI hotspots within the urban area, (3) seasonal, annual, and decadal changes in the intensity of SUHI, and (4) comparative SUHI analysis (devoid of instrument and methodology biases) with other cities arise. In most studies, it has been found that the SUHII is strongest during clear nights, during days of calm or no wind, and in the summer season (Oke, 1981, 1997; Arnfield, 2003; Voogt and Oke, 2003; Parker, 2010; Peng et al., 2012; Nuruzzaman, 2015; Santamouris, 2016; Anjos et al., 2020). Nighttime LST is more sensitive toward urbanization led thermal heating than daytime LST and is preferred for SUHI estimations (Siddiqui et al., 2021).
A fairly long-term record of over two decades of Moderate Resolution Imaging Spectroradiometer (MODIS) nighttime LST data has come into fruition recently. From a climate science perspective, a long-term LST record is crucial for analyzing changing trends in SUHII for a city, along with its larger impact on urban climate, heat stress and heat-related mortality. At a global scale Peng et al. (2012) used 5-year MODIS LST data to assess seasonal and diurnal SUHII for 419 big cities, while Chakraborty and Lee (2019) used 15 years of MODIS LST to calculate diurnal, seasonal, and annual SUHII for more than 9500 urban clusters. Through satellite LST observations (2002–2021), Liu et al. (2022) found mean surface warming trend per decade for over 2000 city clusters in the world, of which the highest was noted in Asian megacities, heavily contributed due to rapid urbanization. Variability in seasonal and diurnal UHI, for 101 Asian and Australian cities, was explored by Santamouris (2015), and the relationship between city size and UHII was analyzed. Using a regional climate model and MODIS LST data on cities in the US, Sarangi et al. (2021) noted how the UHI effect dominates the diurnal variations in urban heat stress intensity, is at peak during the nighttime, and that the heat stress exposure during nighttime intensified by 3–5 hours per day. Raj et al. (2020) found increasing nighttime SUHIIs in 44 major cities of India using satellite temperature data from 2000 to 2017. Kumar et al. (2017) in their study over 89 Indian cities highlight that nighttime SUHI is strongly correlated to the percentage of imperviousness in the urban areas. Shastri et al. (2017) calculated daytime and nighttime SUHII during two seasons-pre-monsoon (MAM) and winter (DJF), for 84 urban locations in India, using MODIS LST data from 2003 to 2013. However, the study was based on city clustering algorithm, which relies on population estimates, rather than impervious area estimates, to delineate urban and rural boundaries; the latter being a better criterion, especially in a populous country like India. In this regard, the present paper is the first to assess 20 years (2001–2020) of nighttime SUHIs, during all four seasons- winter (December-February; DJF), pre-monsoon (March-May; MAM), monsoon (June-August; JJA), and post-monsoon (September-November; SON), over all the nine major populated cities of India i.e., Delhi, Mumbai, Kolkata, Bangalore, Chennai, Hyderabad, Ahmedabad, Surat, and Pune. Moreover, the study provides an insight into the SUHII variability and trends at a decadal, annual, and at a seasonal time scale for the nine Indian cities. The paper also discusses the far-reaching implications of nighttime SUHI expansion and the increasing severity of SUHII, in worsening the heat stress and discomfort of city dwellers of India. Ramifications of increasing SUHIIs during pre-monsoon (or summer; MAM season) in synergy with increasing global temperatures and increasing heatwaves on the human mortality rate are also discussed. Lastly, a call to action via targeted SUHI mitigation strategies and development of city-specific action plans focused to reduce heat stress are suggested.
2. Methods
2.1. Selection of cities
Past (2000), contemporary (2018), and future (2030) population estimates (United Nations (UN), 2018a) were used to identify nine major populated cities of India. In 2018, five cities, viz., Delhi (28.5 million), Mumbai (19.9 million), Kolkata (14.6 million), Bangalore (11.4 million), and Chennai (10.4 million) had a population of over 10 million, while four cities, viz., Hyderabad (9.4 million), Ahmedabad (7.6 million), Surat (6.5 million), and Pune (6.2 million) had a population of over 5 million. The population statistics, including the annual rate of population change between 2000–18 and 2018–2030 for these cities are provided in Table 1. It is estimated that by 2030, Hyderabad and Ahmedabad would likely grow to become megacities, while the population of two cities- Surat and Pune will likely increase to 9.7 million and 8.4 million, respectively, almost within reach of the megacity status (Table 1; United Nations (UN), 2018a). The geographical locations of each of the nine Indian cities, selected for the present study are shown in Figure 1. The figure also zooms in to each city (Figures 1A–I) and shows the extent of urbanization in each city. The percentage of impervious area for the year 2010 (Wang et al., 2017) has been used to map urban extents. Details on the dataset are elaborated in Section 2.2.
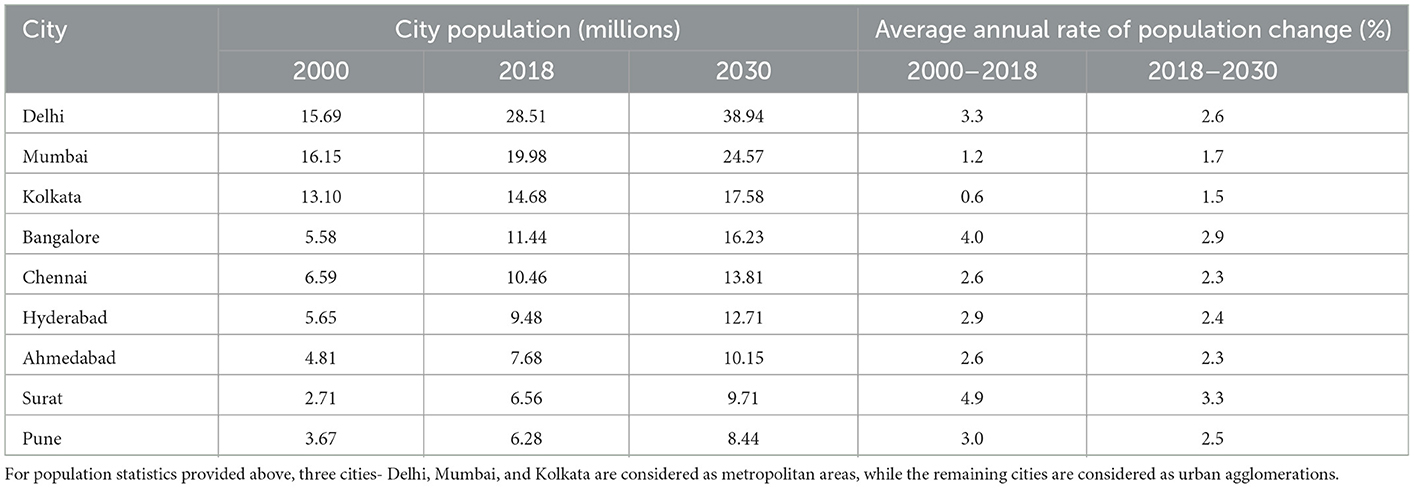
Table 1. Population statistics for the nine major populated Indian cities selected for the study. Past (2000), contemporary (2018), and future (2030) population (millions) are provided in the Table, along with the average annual rate of population change (%) from 2000 to 2018, and 2018 to 2030 [Source: United Nations (UN), 2018a].
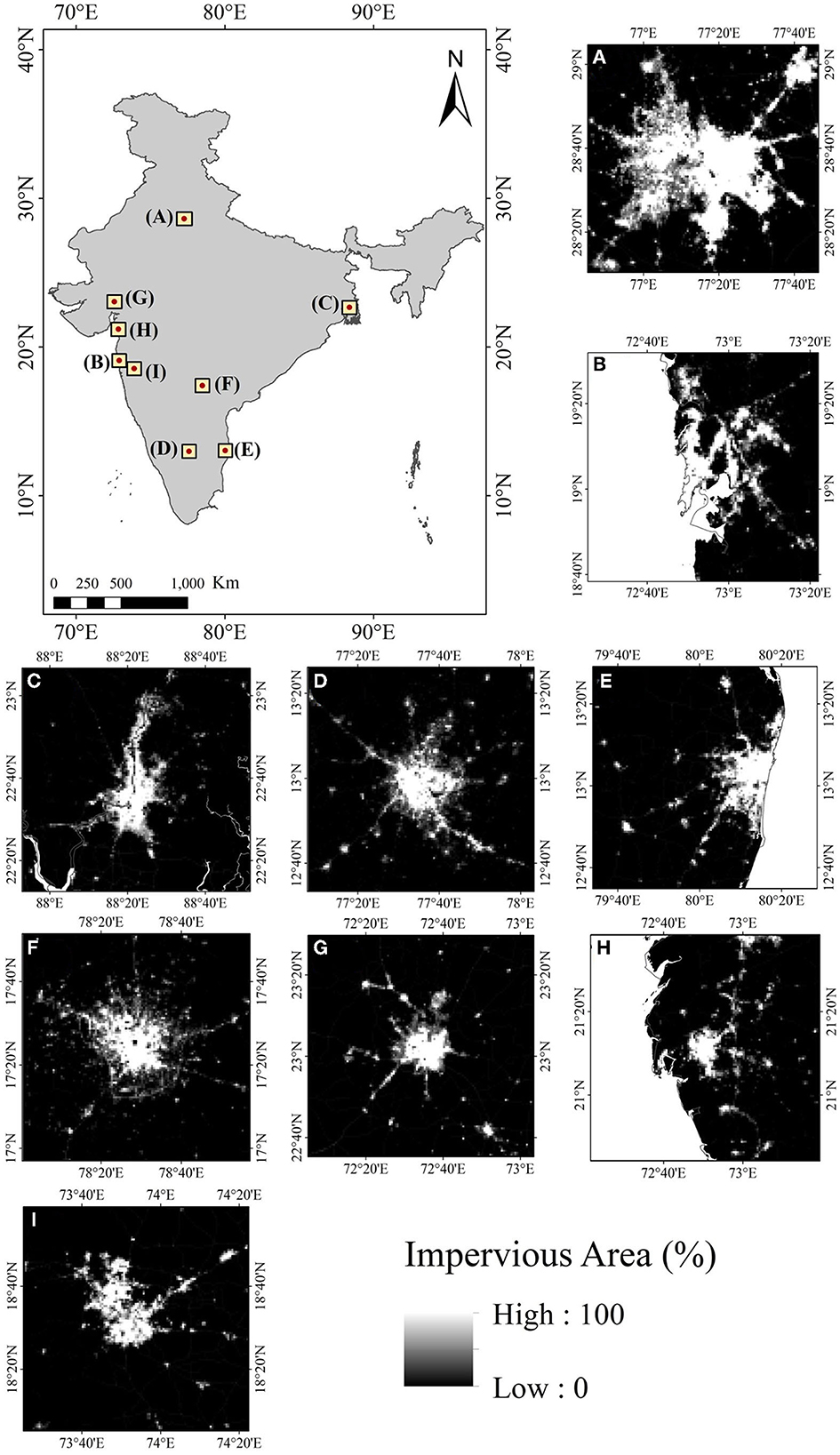
Figure 1. Study area showing geographical location of the nine major populated cities of India within its national boundary (shaded in gray). The cities are represented by a red dot within the 100 km surrounding boxes (shaded in yellow; box outlines in black) marking the region of interest. Impervious area (%) based on GMIS dataset for the year 2010 (Wang et al., 2017) for cities (A) Delhi, (B) Mumbai, (C) Kolkata, (D) Bangalore, (E) Chennai, (F) Hyderabad, (G) Ahmedabad, (H) Surat, and (I) Pune is provided alongside. The figures are generated with ArcMap ver. 10.6.
The two main reasons for choosing these particular nine Indian cities are- (1) their massive population size (>5 million), which inherently increases their vulnerability to loss of lives in the wake of extreme events or climate disasters, and (2) their status as centers of national, financial, technological, scientific, and cultural importance, which inherently increases their vulnerability to a greater loss of gross domestic product (GDP) in such events.
2.2. Delineation of urban-rural extents
Global Man-made Impervious Surface (GMIS) dataset available for the year 2010, at 1 km resolution (Wang et al., 2017), was accessed from the Socioeconomic Data and Applications Center (SEDAC) (https://sedac.ciesin.columbia.edu/data/set/ulandsat-gmis-v1). The GMIS 2010 dataset is derived from Global Land Survey (GLS) Landsat image archive and maps subpixel urban imperviousness using the “Human Built-up And Settlement Extent” (HBASE) approach. The GMIS dataset limitations include possibility of incorrect removal of small areas of urban pixels by HBASE, and some pixel misclassification to “NoData” category where cloud/ shadow areas and Landsat 7 ETM+ SLC off image data gaps exist (Brown de Colstoun et al., 2017). Even with these limitations, the GMIS and HBASE dataset for the year 2010 was found to have good (0.91) kappa coefficient over Europe (Zhang et al., 2020), and is considered a valuable global impervious area dataset for many urban based research studies (Yoo et al., 2019; Cho et al., 2020; Melancon et al., 2021; Shen and Zhao, 2021).
At the same time, Kim and Brown (2021) highlight that there still remains a general lack of protocol for classification criteria required when estimating SUHI. This is due to the fact that there is no clear international definition of what is considered as “urban,” and the administrative boundary of a city may not accurately represent the urban extent (especially due to creation of new satellite towns beyond the administrative limits of the city). However, many studies suggest that the use of impervious area percentage is a good delineator of urban extent and can be used to efficiently quantify SUHI (Li et al., 2018). Within the GMIS 2010 dataset, the class values range from 0 to 100, each representing the percentage of impervious area within a 1 km grid, and is used as a primary dataset in the present study for delineating the urban and rural extents.
The GMIS 2010 dataset was qualitatively cross-referenced for urban area extents with the Bhuvan state-wise land use land cover dataset (2011–12; https://bhuvan-app1.nrsc.gov.in/thematic/thematic/index.php) and with the Google Earth imageries for the nine Indian cities for the year 2010. A review of ancillary information such as city maps, master plans and available town planning documents were also performed so that a good reference for urban and rural extents could be established. Based on these careful qualitative evaluations on the urbanization patterns in India, the GMIS data over the entire Indian landmass was classified into four categories- (1) all grids having impervious area < 10% as “rural,” (2) grids having impervious area between 10 and 24% as “peri-urban,” (3) grids having impervious area between 25 and 49% as “low-density urban,” and (4) grids having impervious area > 50% as “high-density urban.” Furthermore, it was established that for all the selected nine Indian cities, a 100 km bounding box (region of interest) was sufficiently (1) large enough to contain the entirety of the “high-density urban,” “low-density urban,” and “peri-urban” areas, (2) large enough to sufficiently include the surrounding “rural” areas for SUHII estimation, and at the same time, and (3) small enough to limit the topography related local weather changes as well as a temperature bias in SUHII. The centroid of each of the 100 km bounding box was superimposed over the city center points. The city center point vector data was prepared in ArcGIS ver.10.6, from the existing geographical coordinates (latitude and longitude) information for each city (Figure 1). The location of the bounding box for each city was determined by fulfilling the above conditions, whilst also ensuring that they do not overlap with nearby cities. In case of coastal cities, the area beyond the Indian landmass i.e., the Arabian Sea or the Bay of Bengal, was not considered in the SUHII estimations.
2.3. Estimation of SUHII
Quality checked monthly night-time MODIS LST product at 0.05° spatial resolution (MOD11C3v006; https://giovanni.gsfc.nasa.gov/giovanni/) was downloaded for the years 2001–2020 over the Indian landmass. For each year, the data was averaged seasonally for DJF, MAM, JJA, and SON. Seasonal night LST for each year was subset (clipped) for the nine city domains (100 km bounding boxes) and further for the four urban-rural classes viz., “rural,” “peri-urban,” “low-density urban,” and “high-density urban” within each city as elaborated in Section 2.2.
In order to estimate SUHII, the LST of pixels assigned class as “high-density urban” were subtracted from the mean LST in pixels assigned class as “rural.” Classes “peri-urban” and “low-density urban” were disregarded in their entirety for the computation of SUHII. Both these classes have a mixed proportion of urban-rural elements in varying degrees; an urban bias in the “low-density urban” class and a rural bias in the “peri-urban” class, and therefore the inclusion of these two “buffer zone” classes would have corrupted the true SUHII estimates (Voogt and Oke, 2003).
3. Results and discussion
3.1. Spatio-temporal nighttime SUHI footprint
Land-air interactions play a major role in urban climate, especially in governing urban heating (Jain et al., 2017; Jain, 2021b, 2022). Further, nighttime LST is found to be a better indicator of SUHII than daytime LST due to its higher sensitivity toward urbanization (Siddiqui et al., 2021). Figures 2–4 shows the seasonal nighttime LST for the 2001–2010 and 2011–2020 decades, over the nine major populated cities of India. The formation of a distinct nighttime SUHI in regions of greater impervious area percentage is clearly discernable from this figure. Formation of nighttime SUHI is found to relate remarkably well to the city size, and is primarily evident over the “high density urban” class i.e., pixels having over 50% of impervious area.
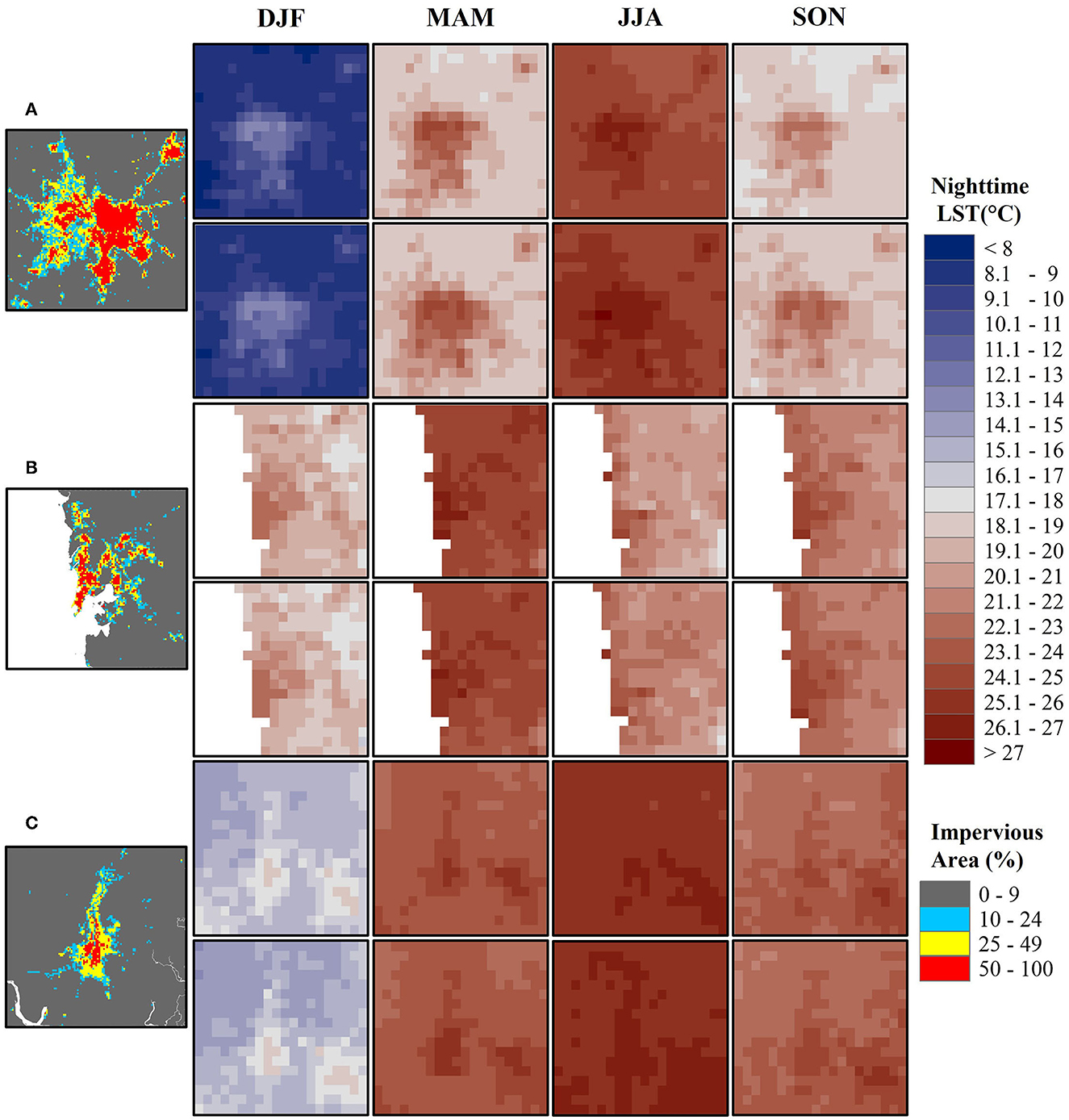
Figure 2. Nighttime LST [°C; blue to red (shaded)] computed seasonally for December-February (DJF), March-May (MAM), June-August (JJA), and September-November (SON) for cities (A) Delhi, (B) Mumbai, and (C) Kolkata. For each city shown, the upper panels represent nighttime LST for the 2001–2010 decade, and the lower panels represent nighttime LST for the 2011–2020 decade. SUHI formation during the night in the cities is clearly discernable in regions of greater impervious area percentage. Impervious area for the year 2010 (Source: Wang et al., 2017) is classified into four categories- (1) 0–9 % as “rural”, (2) 10–24 % as “peri-urban,” (3) 25–49 % as “low-density urban,” and (4) 50–100 % as “high-density urban” in the present study. The figures are generated with ArcMap ver. 10.6.
Of all the nine Indian cities, Delhi, which is both- the largest populated city of India, and the largest urbanized city (area under “high density urban” class), shows the largest nighttime SUHI footprint (Figure 2A). Other megacities, namely, Mumbai (Figure 2B), Kolkata (Figure 2C), Bangalore (Figure 3A), and Chennai (Figure 3B) also show a significant year-round nighttime SUHI, during both the 2001–2010, and 2011–2020 decades. On the other hand, incipient megacities with a greater city size, such as, Hyderabad (Figure 3C) and Ahmedabad (Figure 4A), show a substantially larger nighttime SUHI footprint, than a small-sized incipient megacity, such as, Surat (Figure 4B). SUHI is found to weaken over the “peri-urban” and “low-density urban” classes. This is most prominently observed in Pune (Figure 4C), an incipient megacity with a city size large enough to be comparable to some of the megacities, and yet most of its area falling in either the “peri-urban” or the “low-density urban” class. The most reasonable explanation for the observed SUHI weakening over these mixed urban-rural classes is the greater abundance of thermal cooling classes e.g., forests and waterbodies than in the “high density urban” areas (Arnfield, 2003; Anjos et al., 2020; Jain, 2021b). It should be noted that certain “rural” class land use land covers, such as, barren lands, rocky terrain, and uncultivated agricultural lands can, at times, emit higher a proportion of thermal radiation during the night, and as a result show an even higher LST than the city (Jain et al., 2017). Few isolated rural areas nearby to the cities of Delhi, Kolkata, and Hyderabad do show higher nighttime LST in the present study (Figures 2, 3). Such scenarios are heavily dependent on the intrinsic thermal properties e.g., specific heat capacity, thermal conductivity, and emissivity (Chen et al., 2006; Xian and Crane, 2006; Bowler et al., 2010; Hua et al., 2017) of the materials found in such areas, which lead to a higher nighttime LST.
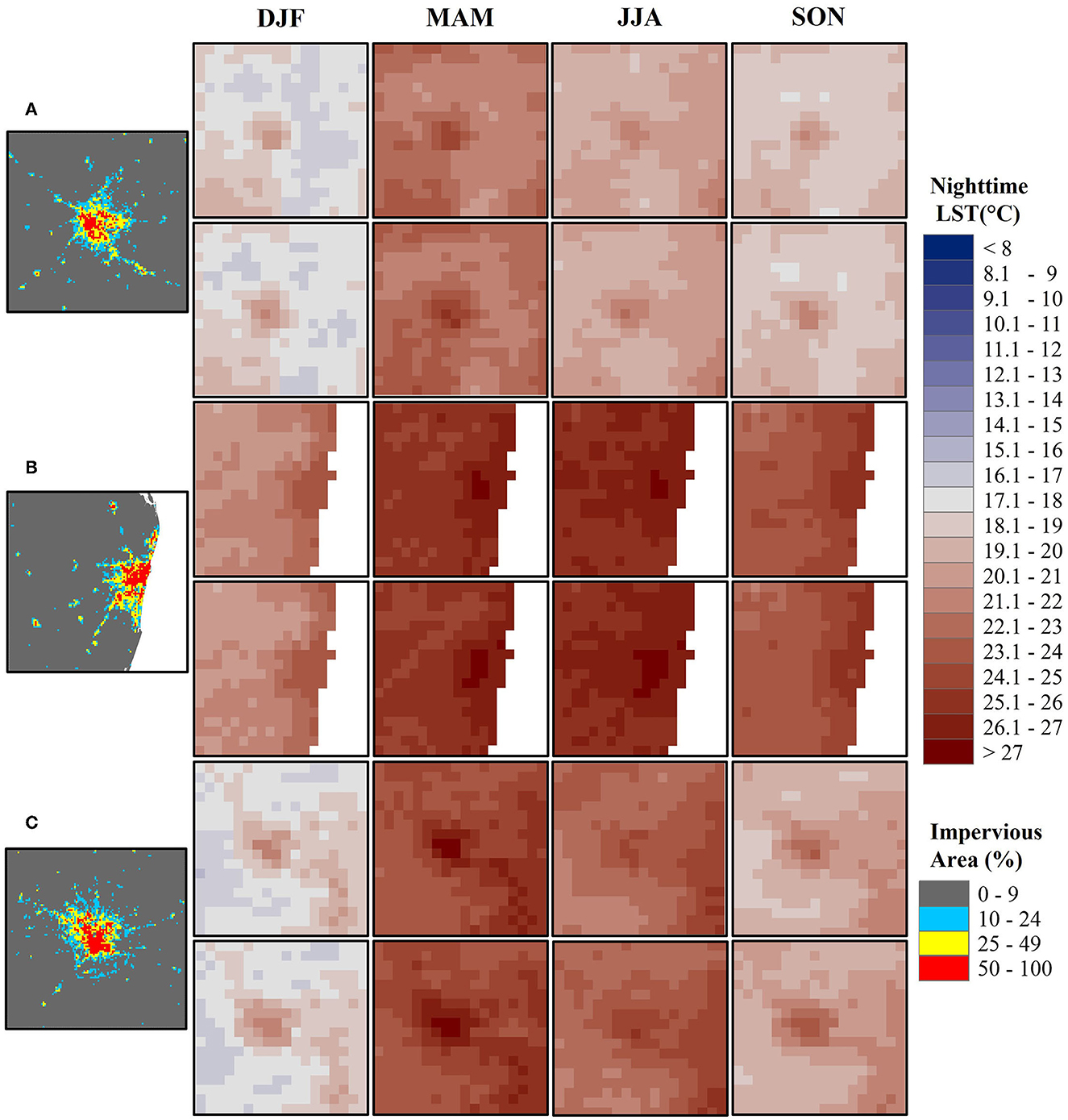
Figure 3. Same as Figure 2, but for cities (A) Bangalore, (B) Chennai, and (C) Hyderabad.
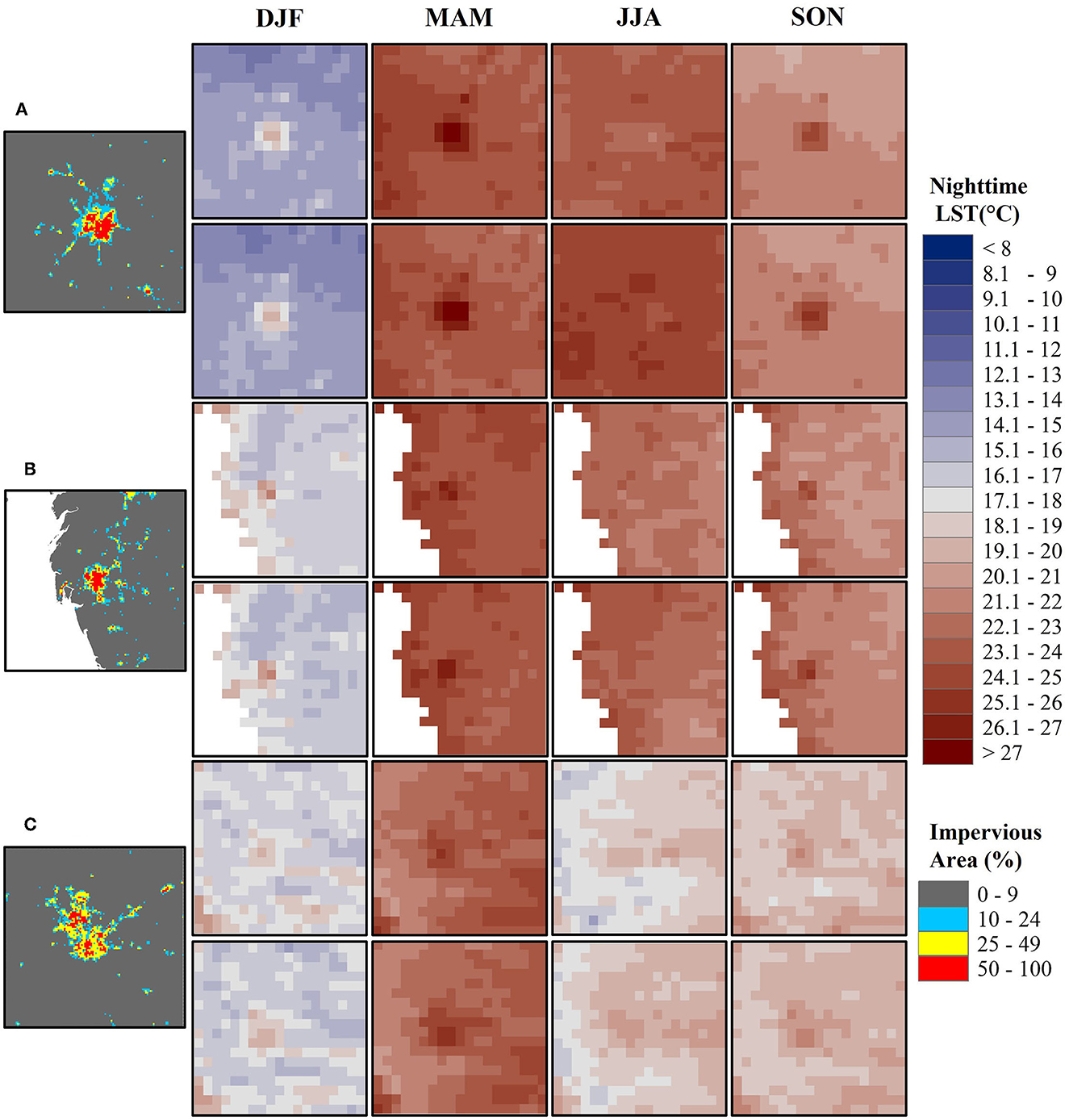
Figure 4. Same as Figure 2, but for cities (A) Ahmedabad, (B) Surat, and (C) Pune.
For all the cities in the present study a seasonal variation in the footprint of nighttime SUHI is noted. From Figures 2–4, the most intense formation of nighttime SUHI is noted during pre-monsoon (MAM) and winter (DJF) season. Similar results of a seasonal maxima during MAM and DJF have been highlighted in a previous nighttime SUHII study over India by Shastri et al. (2017). For most cites, nighttime SUHI during the post-monsoon (SON) season is not observed to be as intense as either during DJF or during MAM season. Further, all cities show that the nighttime SUHI formation is weakest during the monsoon season i.e., JJA. The monsoon season is when the cities of India experience maximum precipitation, along with strong convective winds and lush vegetation; all factors that mitigate SUHI formation. Based on the mean seasonal SUHI for the two decades, Ahmedabad is observed as the only city to show the existence of an urban cool island, evident during the JJA season of 2001–2010 (Figure 4A). The significant impact of increasing urbanization (pre- and post-2010 scenarios) on the nighttime SUHI is also evident from Figures 2–4. Compared to the 2001–2010 decade, the nighttime SUHI size during the 2011–2020 decade is found to have significantly grown for all the nine major cities of India. This nighttime SUHI expansion is observed during all the seasons, however, most strongly evident during MAM. The pattern of radial outward growth of nighttime SUHI is consistent with the patterns of urban sprawl witnessed over these Indian cities (Taubenböck et al., 2009, 2012; Jain et al., 2016; Ghosh and Das, 2017).
3.2. Seasonal, annual, and decadal nighttime SUHII trends
The characterization of SUHII variability and trends is of foremost importance in order to understand the processes related to heat stress in humans (Anjos et al., 2020). The season of the highest intensity of nighttime SUHI, for each city, in each decade, is highlighted in the present study. For all the nine Indian cities, either DJF or MAM season shows the prevalence of the strongest nighttime SUHII. During the 2001–2010 and the 2011–2020 decades, a nighttime SUHII magnitude of respectively (i) 2.1°C and 2.5°C (in MAM) for Delhi, (ii) 1.3°C and 1.5°C (in DJF) for Mumbai, (iii) 1.3°C and 1.5°C (in DJF) for Kolkata, (iv) 0.6°C and 1.0°C (for both DJF and MAM) for Bangalore, (v) 1.7°C and 1.9°C (in DJF) for Chennai, (vi) 1.8°C and 2.3°C (in DJF) for Hyderabad, (vii) 2.8°C and 3.1°C (in DJF) for Ahmedabad, (viii) 1.9°C and 2.4°C (in DJF) for Surat, and (ix) 0.8°C and 1.3°C (in MAM) for Pune (Table 2) is found to be the strongest.
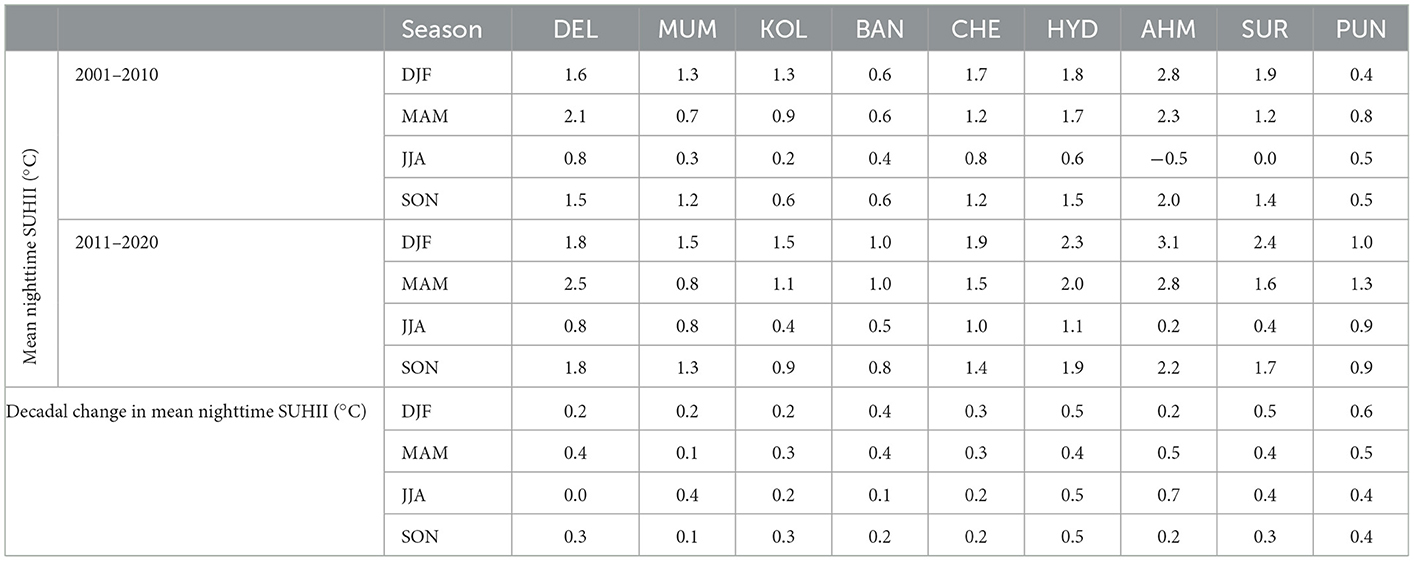
Table 2. Mean nighttime SUHII (°C) for seasons DJF, MAM, JJA, and SON during the decades 2001–2010, and 2011–2020 along with its decadal change for the nine major populated cities of India. In the Table these cities are represented as- Delhi (DEL), Mumbai (MUM), Kolkata (KOL), Bangalore (BAN), Chennai (CHE), Hyderabad (HYD), Ahmedabad (AHM), Surat (SUR), and Pune (PUN).
The mean annual nighttime SUHII from 2003 to 2008 over majority (95%) of the 419 big (population > 1 million) cities of the world was found to range between 0 and 2°C (Peng et al., 2012). In a separate study over India, the MAM and DJF nighttime SUHII, from 2003 to 2013, for 84 urban locations was noted to range between 0–1°C and 0–1.5°C, respectively (Shastri et al., 2017). Figure 5 depicts the 20-year annual trend of the nighttime SUHII for all the nine Indian cities. For each year, the mean nighttime SUHII for the seasons DJF, MAM, JJA and SON are shown. The present study finds that the mean decadal nighttime SUHII from 2001 to 2020 in most of the nine (population > 5 million) cities of India ranged between 0–2.5°C (Table 2), which is slightly higher than the global average. Moreover, between 2001–2020, the mean decadal nighttime SUHII increased by 0.2–0.4°C in DJF, by 0.1–0.4°C in MAM, by 0.0–0.4°C in JJA, and by 0.1–0.3°C in SON, in the megacities of India (Table 2). Interestingly, a substantially higher decadal increase of 0.2–0.6°C in DJF, 0.4–0.5°C in MAM, 0.4–0.7°C in JJA, and 0.2–0.5°C in SON, is noted in the four incipient megacities of India.
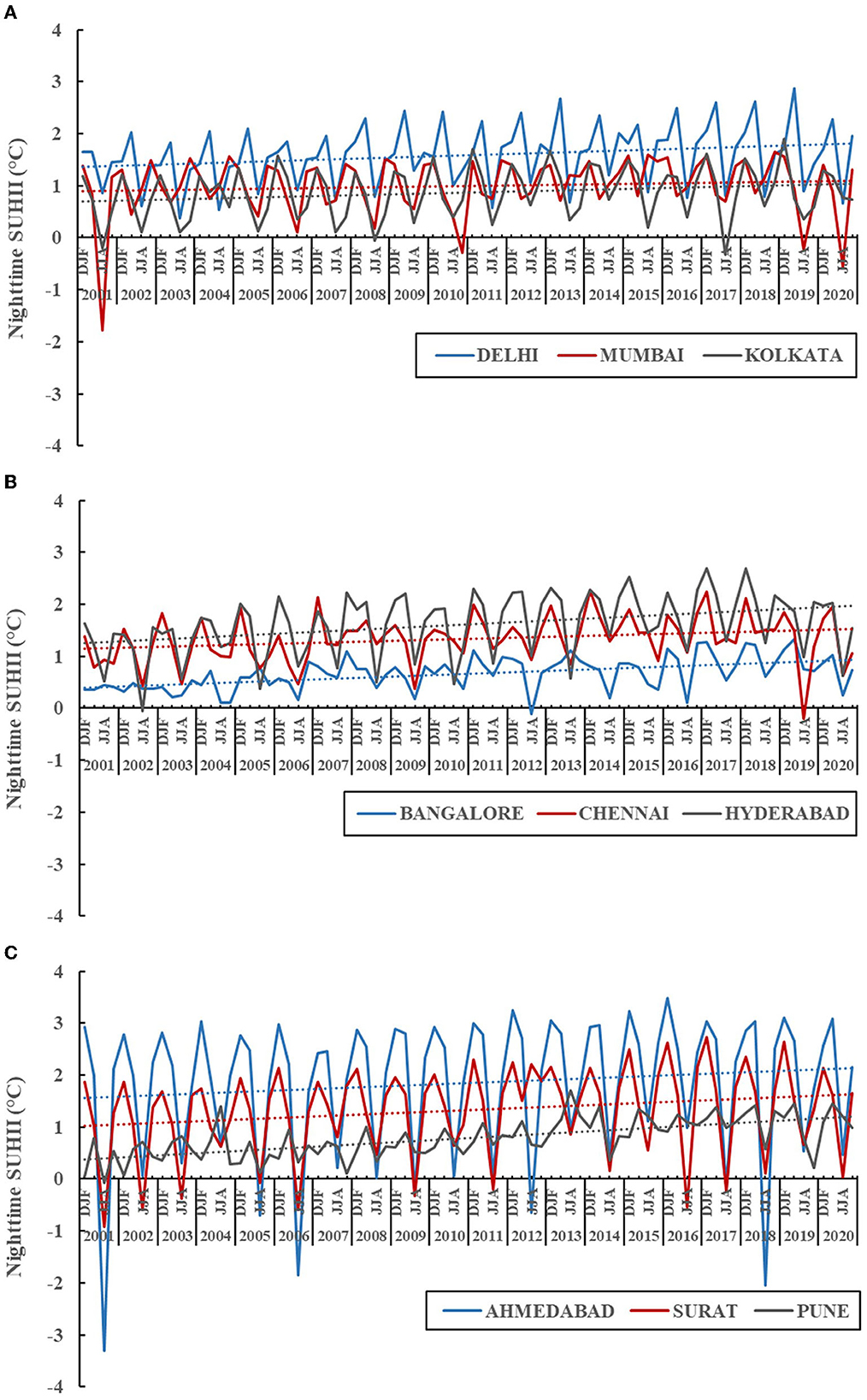
Figure 5. A 20-year (2001–2020) mean annual nighttime surface urban heat island intensity (SUHII; °C; solid lines) trend for the nine major populated cities of India (A) Delhi, Mumbai, and Kolkata, (B) Bangalore, Chennai, and Hyderabad, and (C) Ahmedabad, Surat, and Pune. Within each year the mean nighttime SUHI Intensity is estimated for DJF, MAM, JJA, and SON season. From 2001 to 2020, all cities show an increasing linear trend (dashed lines) in the mean annual nighttime SUHII.
The mean annual growth rate of nighttime SUHII from 2001 to 2020 was found to be (i) 0.0057°C/year and for Delhi, (ii) 0.0026°C/year for Mumbai, (iii) 0.0042°C/year for Kolkata, (iv) 0.0069°C/year for Bangalore, (v) 0.0047°C/year for Chennai, (vi) 0.0093°C/year for Hyderabad, (vii) 0.0073°C/year for Ahmedabad, (viii) 0.0076°C/year for Surat, and (ix) 0.0105°C/year for Pune (Figure 5). It is interesting to note that every incipient megacity had a mean annual growth rate of nighttime SUHII of over 0.007°C/year, while all megacities remained below this threshold rate. One reason for this larger SUHII growth rate in the incipient megacities could be due to the faster urban growth rate reported in these cities (Taubenböck et al., 2009). This could lead to a significantly higher urban heating rate, a higher urban LST, a stronger SUHII, and significant increase in the heat stress.
3.3. Implications for heat stress in Indian cities
A warmer global climate, and UHIs in the cities, interact by exacerbating heatwaves and increasing extreme heat days during the summer season (Kim and Brown, 2021; Jain, 2022). In cities, the extremely high day or night air temperature due to this synergistic effect can directly cause an increase in the heat-related morbidity and mortality rates (Santamouris, 2016; Kumar et al., 2017; Kim and Brown, 2021). The average MAM temperature experienced by Indians during 2017–2021 was 0.4°C warmer than the mean temperature averaged globally during 1986–2005 (Climate Transparency, 2022). Moreover, 12 of the 15 warmest recorded years in India, in the last 120 years, occurred between 2006 and 2020; of these 2016 (+0.71°C), 2009 (+0.55°C), 2017 (+0.54°C), 2010 (+0.54°C), and 2015 (+0.42°C) were the warmest 5 years (India Meteorological Department (IMD), 2021a).
Indian cities in particular have been found to be more affected by heatwaves, than the rural areas due to the UHI (or SUHI) development, and in addition, the heatwaves during MAM have also increased significantly in India (Shastri et al., 2017). Some of the most notable severe heatwave events in India have occurred in 2002 (April-May), 2015 (May-June), 2016 (April-May), 2019 (May-June), and 2022 (March-May), where the maximum recorded temperature during each heatwave event ranged from 49 to 51°C. Delhi and Ahmedabad are two prominent cities in northern India that face severely harsh MAM season, with daytime (maximum) and nighttime (minimum) air temperatures often crossing 40°C and 25°C, respectively. In such a case, a 2.1–2.8°C and a 2.5–3.1°C mean nighttime SUHII during MAM, observed in the last two decades, in Delhi and Ahmedabad, respectively (Table 2) is extremely concerning; more so during the period of severe heat events in summer. Similarly concerning is the nighttime SUHII maxima of over 1.5°C witnessed in other Indian cities, such as, Chennai, Hyderabad, and Surat. This compounds the heat stress faced in cities, especially during episodes of severe heatwaves during summers, and leads to higher incidences of heat-related fatalities, such as, deaths due to heat strokes.
As per a recent report by Climate Transparency (2022), at a warming of 1.5°C, about 142 million more Indians, each year, are projected to be exposed to heatwaves than the average exposure in 1986–2006, and about 2.8 times greater exposure at a warming of 3°C. Using 1980–2010 as the base period, the annual minimum and maximum air temperature anomalies (°C; solid lines; primary y-axis) in the last 50 years (1971–2020) on the Indian landmass surface (India Meteorological Department (IMD), 2021b) are plotted (Figure 6A). Along with the air temperature anomalies, the reported (annual) number of heat stroke deaths (solid bars; secondary y-axis; Figure 6A) in India in these 50 years are also shown. The earlier 25-year period from 1971 to 1995, mostly shows the prevalence of a negative air temperature anomaly for both, the minimum, and the maximum air temperature. However, the latter period from 1996–2020, generally consistent with the onset of rapid urbanization in Indian cities (Taubenböck et al., 2009, 2012) shows notably higher minimum and maximum air temperature anomalies (+0.5 to +1.0°C; Figure 6A). Moreover, in the figure, black arrows are used to mark the occurrence of severe heatwave events (e.g., 1995, 1998, 2003, 2010, 2012, 2015, 2016, and 2019) in India in the recent years.
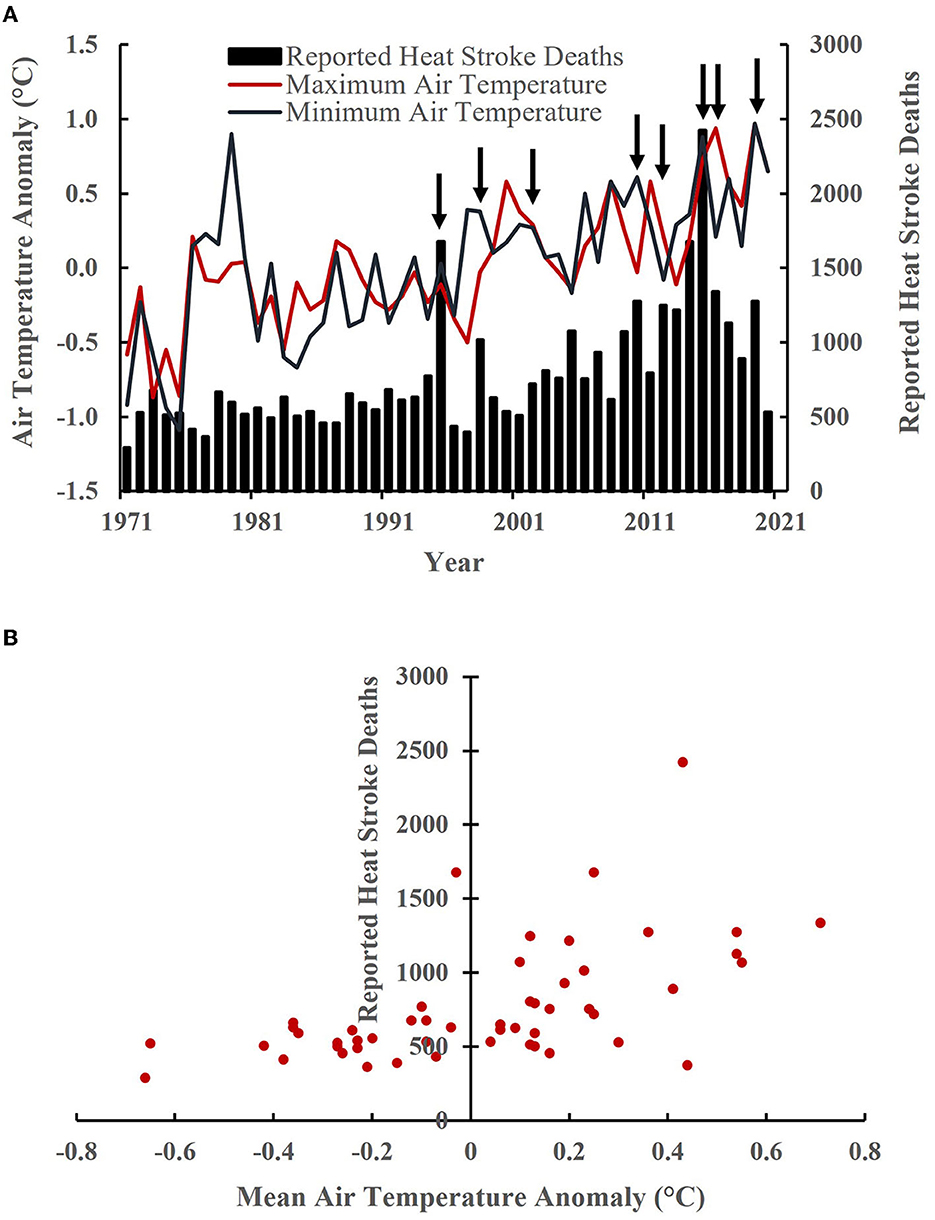
Figure 6. (A) Annual minimum and maximum air temperature anomalies (°C; solid lines; primary y-axis) on Indian landmass (India Meteorological Department (IMD), 2021b) and reported (annual) number of heat stroke deaths (solid bars; secondary y-axis) in India in the last 50 years (1971–2020). Base period from 1981 to 2010 is used to compute the annual air temperature anomalies. National Crime Records Bureau (National Crime Records Bureau (NCRB), 2022) Accidental Deaths and Suicides in India (ADSI) individual reports from 1971 to 2020 were used to extract heat stroke deaths data. Black arrows mark the occurrence of severe heatwave events (e.g., 1995, 1998, 2003, 2010, 2012, 2015, 2016, and 2019) in India in the recent years. (B) Scatter-plot showing correlation of annual mean air temperature anomalies (°C) over Indian landmass and the reported yearly heat stroke deaths in India in 50 years (1971–2020). A significant sharp increase in the reported heat stroke deaths is observed with the annual mean air temperature anomaly of +0.2°C and higher.
A congruence of increasingly positive air temperature (minimum and maximum) anomalies, with the increasing occurrences of severe heatwave events and increased heat stroke deaths (reported) in India is highlighted. As a caution, is important to note, that the actual number of heat stroke deaths, and other heat-related morbidities in India, is difficult to estimate, even for individual cities. Moreover, is widely accepted that the heat stroke deaths are much (even many times) higher than the official numbers reported in the National Crime Records Bureau (National Crime Records Bureau (NCRB), 2022) Accidental Deaths & Suicides in India (ADSI) yearly reports, and the official heat-related morbidities data is entirely unavailable (Hajat and Kosatky, 2010; Tran et al., 2013; Azhar et al., 2014; The Times of India, 2015; Swain et al., 2019). Even still, a significant sharp increase in the heat stroke deaths (annually reported) in India is observed, when the annual mean air temperature anomaly (with respect to 1980–2010 baseline) of +0.2°C and above, occurs over the Indian landmass (Figure 6B). The impact of heat-stress, including, excess deaths due to heat strokes, would be compounded in the Indian megacities and incipient megacities, wherein, the present study shows the existence of high mean nighttime SUHIIs (up to 3.1°C), high increases in mean decadal nighttime SUHIIs (up to 0.4°C), and high mean annual growth rates of nighttime SUHIIs (up to 0.0105°C/year) in the period from 2001 to 2020.
Furthermore, studies show that for every 1°C increase in ambient temperature, the mortality rate has been observed to increase by 1–3%, and above a critical heat threshold the mortality rates rise exponentially (Hajat and Kosatky, 2010). The mortality rate in the Mediterranean increased by 3.12% per 1°C as the ambient temperature increased above the heat threshold (Santamouris, 2016). In case of Delhi (India), the mortality rate during a 1991–1994 study increased by 3.94% per 1°C increase in ambient temperature above 29°C (Hajat and Kosatky, 2010). In 2018 and 2019, India observed the largest increase in heat related mortalities in the world (Romanello et al., 2021). Moreover, in 2021 an estimated loss of 167 billion potential labor hours and USD 159 billion in GDP due to extreme heat caused labor capacity reduction was reported (Climate Transparency, 2022). Increasingly warmer decades, increasing mean annual growth rate of SUHIIs, and increasing frequency of prolonged severe heatwaves, will continue to enlarge the gap between the ambient air temperature and the heat threshold temperature megacities like Delhi and incipient megacities like Ahmedabad. This puts tens of millions of city-dwellers in megacities and incipient megacities of India at a grave risk of heat-related morbidity and mortality in the near future.
4. Conclusion and recommendations
From a heat stress perspective, the triple whammy of unabated globally rising temperatures, increasing UHIIs (or SUHIIs), and increasingly frequent prolonged heatwaves of extreme severity during pre-monsoon (MAM) season is of utmost concern in cities of India (World Health Organization (WHO), 2020; India Meteorological Department (IMD), 2021a; Jain, 2021b, 2022; Jain et al., 2021). A number of factors such as urban area (e.g., impervious surface area, built-up density, building heights, etc.), city morphology (e.g., sky view factor, canyon width, building material properties, presence of thermal cooling classes, etc.), meteorological conditions (e.g., wind speed, wind direction, boundary layer height, precipitation, etc.), anthropogenic heat fluxes (vehicular emissions, air-conditioning heat output, industrial emissions, human metabolism, etc.) affect the ambient temperature, SUHI formation, and ultimately heat stress in cities. In the nine highest populated cities of India, the key role of increasing urbanization (pre- and post-2010) in nighttime SUHI expansion and intensification is highlighted in the present study. Two seasons, MAM and DJF show the strongest prevalence of nighttime SUHII in Indian cities, a period coinciding with increasing number of severe heatwaves in India.
The study also highlights that the incipient megacities of India showed a substantially greater mean annual growth rate of nighttime SUHII than the megacities where this rate remained below 0.007°C/year. In the last two decades, a mean nighttime SUHII of 2.1–2.8°C was witnessed during MAM in Delhi, a megacity with 28.5 million people (and a projected population of 39 million in 2030) and a mean nighttime SUHII of 2.5–3.1°C was witnessed during MAM in Ahmedabad, an incipient megacity showing the highest SUHII out of all Indian cities. Such situation is extremely concerning in the context of increasing heat stress, heat-related morbidity and mortality. An example is the 2010 (May) Ahmedabad heatwave which led to over 1,340 excess deaths (Azhar et al., 2014). A nighttime SUHII maxima of over 1.5°C, witnessed in other Indian cities, such as, Chennai, Hyderabad, and Surat, is also similarly concerning. In general, the intensities of nighttime SUHIs witnessed around the year in all nine cities of India are substantially high to cause significantly lowered thermal comfort and quality of life of the people.
In order to reduce the heat-related mortality in cities of India, key high intensity SUHI hotspots need to be identified. These hotpots of excessive heating within the cities should be of utmost focus for implementing targeted strategies aiming to reduce heat stress. In a previous local-scale line transect study over Delhi, the presence of thermal cooling classes along densely built-up urban areas was found to significantly reduce LST (Jain et al., 2017). Studies show that a strategic increase in inclusion of blue-green infrastructure (i.e., “blue” infrastructure e.g., rivers, ponds, watersheds, tanks, other waterbodies, etc. and “green” infrastructure e.g., forests, parks, gardens, etc.) significantly reduces ambient temperature, lowers UHII (or SUHII), and mitigates heat stress (Nuruzzaman, 2015; Hua et al., 2017; Jain, 2021a; Sanusi and Jalil, 2021). Urban greening strategies such as vertical gardens, rooftop and terrace gardens, community forests, urban agriculture are aesthetically pleasant and also provide thermal comfort in heat-stressed urban environments (Anjos et al., 2020; Jain, 2021b). In addition, inclusion of blue-green infrastructure also leads to greater energy savings due to lowered electricity power (e.g., air-conditioning) demands, while simultaneously decreasing the anthropogenic heat flux (Akbari et al., 1997). Other strategies such as cool roofs, cool pavements, green buildings, and water spray systems have also shown great potential in significantly reducing heat stress during summer extreme heat events (Fahed et al., 2020; Khare et al., 2021; Sanusi and Jalil, 2021).
It is recommended that all the major populated cities of India should formulate city-specific action plans incorporating the mitigation of UHIs (or SUHIs) and extreme heat events under a warmer future climate. Currently, Ahmedabad is the only city of India with a sophisticated and strategic action plan, called the “Ahmedabad Heat Action Plan 2019,” formulated in response to high mortality rates witnessed during frequently occurring extreme heat events. Targeted city-specific mitigation and action plans have a key role in combating the increasing heat stress faced in Indian cities. Policy focus should be on identification of localized hotspots of strongest UHI (or SUHI) formation within the cities and where the high intensity UHIIs (or SUHIIs) are likely to develop (Kim and Brown, 2021; Sanusi and Jalil, 2021). By taking urgent action through implementation of targeted cooling strategies and heat action plans, the cities of India would be a step closer to achieving the sustainable development goal (SDG 13) by the year 2030.
Data availability statement
The original contributions presented in the study are included in the article/supplementary material, further inquiries can be directed to the corresponding author.
Author contributions
MJ conceptualized the framework of the study, designed the methodology, accessed and processed the data, conducted formal analysis including data visualization, and wrote the manuscript.
Acknowledgments
The author acknowledges National Aeronautics and Space Administration (NASA) Giovanni (https://giovanni.gsfc.nasa.gov/giovanni/) for the access of nighttime (Moderate Resolution Imaging Spectroradiometer (MODIS) Land Surface Temperature (LST) product (MOD11C3v006) and Socioeconomic Data and Applications Center (SEDAC) (https://sedac.ciesin.columbia.edu/data/set/ulandsat-gmis-v1) for the access of Global Man-made Impervious Surface (GMIS) dataset. Both these datasets were crucial in the present study. The author acknowledges the efforts made by the reviewers and the editors in providing their insights, which aided in the improvement of this manuscript.
Conflict of interest
The author declares that the research was conducted in the absence of any commercial or financial relationships that could be construed as a potential conflict of interest.
Publisher's note
All claims expressed in this article are solely those of the authors and do not necessarily represent those of their affiliated organizations, or those of the publisher, the editors and the reviewers. Any product that may be evaluated in this article, or claim that may be made by its manufacturer, is not guaranteed or endorsed by the publisher.
References
Akbari, H., Kurn, D. M., Bretz, S. E., and Hanford, J. W. (1997). Peak power and cooling energy savings of shade trees. Energy Build. 25, 139–148. doi: 10.1016/S0378-7788(96)01003-1
Anjos, M., Targino, A. C., Krecl, P., Oukawa, G. Y., and Braga, R. F. (2020). Analysis of the urban heat island under different synoptic patterns using local climate zones. Build. Environ. 185, 107268. doi: 10.1016/j.buildenv.2020.107268
Arnfield, A. J. (2003). Two decades of urban climate research: a review of turbulence, exchanges of energy and water, and the urban heat island. Int. J. Climatol. 23, 1–26. doi: 10.1002/joc.859
Azhar, G. S., Mavalankar, D., Nori-Sarma, A., Rajiva, A., Dutta, P., Jaiswal, A., et al. (2014). Heat-related mortality in India: excess all-cause mortality associated with the 2010 Ahmedabad heat wave. PLoS ONE 9, e91831. doi: 10.1371/journal.pone.0091831
Bisht, D. S., Sridhar, V., Mishra, A., Chatterjee, C., and Raghuwanshi, N. S. (2019). Drought characterization over India under projected climate scenario. Int. J. Climatol. 39, 1889–1911. doi: 10.1002/joc.5922
Black, E., Blackburn, M., Harrison, G., Hoskins, B., and Methven, J. (2004). Factors contributing to the summer 2003 European heatwave. Weather. 59, 217–223. doi: 10.1256/wea.74.04
Bowler, D. E., Buyung-Ali, L., Knight, T. M., and Pullin, A. S. (2010). Urban greening to cool towns and cities: a systematic review of the empirical evidence. Landsc. Urban Plan. 97, 147–155. doi: 10.1016/j.landurbplan.2010.05.006
Brown de Colstoun, E. C., Huang, C., Wang, P., Tilton, J. C., Tan, B., Phillips, J., et al. (2017). Global Man-made Impervious Surface (GMIS) Dataset from Landsat. Palisades, NY: NASA Socioeconomic Data and Applications Center (SEDAC). doi: 10.7927/H4P55KKF (accessed November 11, 2021).
Chakraborty, T., and Lee, X. (2019). A simplified urban-extent algorithm to characterize surface urban heat islands on a global scale and examine vegetation control on their spatiotemporal variability. Int. J. Appl. Earth Obs. Geoinf. 74, 269–280. doi: 10.1016/j.jag.2018.09.015
Chen, X. L., Zhao, H. M., Li, P. X., and Yin, Z. Y. (2006). Remote sensing image-based analysis of the relationship between urban heat island and land use/cover changes. Rem. Sens. Environ. 104, 133–146. doi: 10.1016/j.rse.2005.11.016
Cho, D., Yoo, C., Im, J., Lee, Y., and Lee, J. (2020). Improvement of spatial interpolation accuracy of daily maximum air temperature in urban areas using a stacking ensemble technique. GISci. Rem. Sens. 57, 633–649. doi: 10.1080/15481603.2020.1766768
Climate Transparency (2022). Climate Transparency Report- G20 Response to the Energy Crisis: Critical for 1.5°C. Available online at: https://www.climate-transparency.org/g20-climate-performance/g20report2022#1542188493496-385f4162-3ead (accessed October 25, 2022).
Dai, A. (2011). Drought under global warming: a review. Wiley Interdiscip. Rev. Clim. Change. 2, 45–65. doi: 10.1002/wcc.81
Eckstein, D., Hutfils, M. L., and Winges, M. (2019). Global Climate Risk Index 2020: Who Suffers Most from Extreme Weather Events? Germanwatch Nord-Süd Initiative eV. Available online at: https://www.germanwatch.org/en/16046 (accessed August 26, 2020).
Fahed, J., Kinab, E., Ginestet, S., and Adolphe, L. (2020). Impact of urban heat island mitigation measures on microclimate and pedestrian comfort in a dense urban district of Lebanon. Sustain. Cities Soc. 61, 102375. doi: 10.1016/j.scs.2020.102375
Ghosh, S., and Das, A. (2017). Exploring the lateral expansion dynamics of four metropolitan cities of India using DMSP/OLS night time image. Spat. Inf. Res. 25, 779–789. doi: 10.1007/s41324-017-0141-3
Hajat, S., and Kosatky, T. (2010). Heat-related mortality: a review and exploration of heterogeneity. J. Epidemiol. Community Health. 64, 753–760. doi: 10.1136/jech.2009.087999
Hasegawa, T., Sakurai, G., Fujimori, S., Takahashi, K., Hijioka, Y., and Masui, T. (2021). Extreme climate events increase risk of global food insecurity and adaptation needs. Nat. Food. 2, 587–595. doi: 10.1038/s43016-021-00335-4
Hawcroft, M., Walsh, E., Hodges, K., and Zappa, G. (2018). Significantly increased extreme precipitation expected in Europe and North America from extratropical cyclones. Environ. Res. Lett. 13:12,124006. doi: 10.1088/1748-9326/aaed59
Hua, W., Chen, H., Zhou, L., Xie, Z., Qin, M., Li, X., et al. (2017). Observational quantification of climatic and human influences on vegetation greening in China. Rem. Sens. 9, 425. doi: 10.3390/rs9050425
India Meteorological Department (IMD) (2021a). Statement on Climate of India During 2020. Press Release, Ministry of Earth Sciences (MoES), Government of India. Available online at: https://www.imdpune.gov.in/Statement%20of%20Climate%20of%20India-2020.pdf (accessed August 19, 2022).
India Meteorological Department (IMD) (2021b). Annual Report 2021. Ministry of Earth Sciences (MoES), Government of India. Available online at: https://mausam.imd.gov.in/imd_latest/contents/ar2021.pdf (accessed November 25, 2022).
Intergovernmental Panel on Climate Change (IPCC) (2012). “Managing the risks of extreme events and disasters to advance climate change adaptation,” in A Special Report of Working Groups I and II of the Intergovernmental Panel on Climate Change, eds. Field, C.B., Barros, V., Stocker, T.F., et al. (New York, UK: Cambridge University Press), 1–583.
Intergovernmental Panel on Climate Change (IPCC) (2014). “Climate Change 2014: Synthesis Report,” in Contribution of Working Groups I, II and III to the Fifth Assessment Report of the Intergovernmental Panel on Climate Change, eds. Pachauri, R.K., Meyer, L.A. (Geneva: IPCC), 1–151.
Jain, M. (2021a). “Potential impacts of gaseous air pollutants on global crop yields under climate change uncertainties and urbanization,” in Air Pollution and Its Complications, eds. Tiwari, S., Saxena, P. (Cham: Springer) 109–127.
Jain, M. (2021b). “Mitigation of urbanization ill-effects through urban agriculture inclusion in cities,” in New Forms of Urban Agriculture: An Urban Ecology Perspective, eds. Diehl, J., and Kaur, H. (Singapore: Springer) 39–56.
Jain, M. (2022). “Increasing atmospheric extreme events and role of disaster risk management: Dimensions and approaches,” in Extremes in Atmospheric Processes and Phenomenon: Assessment, Impacts and Mitigation, eds. Saxena, P., Shukla, A., and Gupta, A. K. (Singapore: Springer) 303–328.
Jain, M., Dimri, A. P., and Niyogi, D. (2016). Urban sprawl patterns and processes in Delhi from 1977 to 2014 based on remote sensing and spatial metrics approaches. Earth Int. 20, 1–29. doi: 10.1175/EI-D-15-0040.1
Jain, M., Dimri, A. P., and Niyogi, D. (2017). Land-air interactions over urban-rural transects using satellite observations: analysis over Delhi, India from 1991–2016. Rem. Sens. 9, 1283. doi: 10.3390/rs9121283
Jain, M., Saxena, P., Sharma, S., and Sonwani., S. (2021). Investigation of forest fire activity changes over the central India domain using satellite observations during 2001–2020. GeoHealth. 5, 12. doi: 10.1029/2021GH000528
Khare, V. R., Vajpai, A., and Gupta, D. (2021). A big picture of urban heat island mitigation strategies and recommendation for India. Urban Clim. 37, 100845. doi: 10.1016/j.uclim.2021.100845
Kim, S. W., and Brown, R. D. (2021). Urban heat island (UHI) intensity and magnitude estimations: a systematic literature review. Sci. Total Environ. 779, 146389. doi: 10.1016/j.scitotenv.2021.146389
Kumar, R., Mishra, V., Buzan, J., Kumar, R., Shindell, D., and Huber, M. (2017). Dominant control of agriculture and irrigation on urban heat island in India. Sci. Rep. 7, 14054. doi: 10.1038/s41598-017-14213-2
Li, H., Zhou, Y., Li, X., Meng, L., Wang, X., Wu, S., et al. (2018). A new method to quantify surface urban heat island intensity. Sci. Total Environ. 624, 262–272. doi: 10.1016/j.scitotenv.2017.11.360
Li, Y., Ye, W., Wang, M., and Yan, X. (2009). Climate change and drought: a risk assessment of crop-yield impacts. Clim. Res. 39, 31–46. doi: 10.3354/cr00797
Liu, H., Huang, B., Zhan, Q., Gao, S., Li, R., and Fan, Z. (2021). The influence of urban form on surface urban heat island and its planning implications: evidence from 1288 urban clusters in China. Sustain. Cities Soc. 71, 102987. doi: 10.1016/j.scs.2021.102987
Liu, Z., Anderson, B., Yan, K., Dong, W., Liao, H., and Shi, P. (2017). Global and regional changes in exposure to extreme heat and the relative contributions of climate and population change. Sci. Rep. 7, 43909. doi: 10.1038/srep43909
Liu, Z., Zhan, W., Bechtel, B., Voogt, J., Lai, J., Chakraborty, T., et al. (2022). Surface warming in global cities is substantially more rapid than in rural background areas. Commun. Earth Environ. 3, 219. doi: 10.1038/s43247-022-00539-x
Melancon, A. M., Molthan, A. L., Griffin, R. E., Mecikalski, J. R., Schultz, L. A., and Bell, J. R. (2021). Random forest classification of inundation following hurricane florence (2018) via L-band synthetic aperture radar and ancillary datasets. Rem. Sens. 13:24,5098. doi: 10.3390/rs13245098
Mishra, V., Mukherjee, S., Kumar, R., and Stone, D. A. (2017). Heat wave exposure in India in current, 1.5 C, and 2.0 C worlds. Environ. Res. Lett. 12, 124012. doi: 10.1088/1748-9326/aa9388
National Crime Records Bureau (NCRB) (2022). Accidental Deaths and Suicides in India (ADSI) Reports from 1971-2020. Ministry of Home Affairs (MHA), Government of India. Available online at: https://ncrb.gov.in/en/accidental-deaths-suicides-india-adsi (accessed December 2, 2022).
National Institute of Disaster Management (NIDM) (2019). Climate Risk Management (CRM) Framework for India: Addressing Loss and Damage (LandD). National Institute of Disaster Management, Government of India. https://nidm.gov.in/PDF/pubs/GIZ_NIDM_Climate%20RiskManagementFramework.pdf (accessed August 25, 2020).
Nuruzzaman, M. (2015). Urban heat island: causes, effects and mitigation measures-a review. Int. J. Environ. Monitor. Analysis. 3, 67–73. doi: 10.11648/j.ijema.20150302.15
Oke, T. R. (1973). City size and the urban heat island. Atmos. Environ. 7, 769–779. doi: 10.1016/0004-6981(73)90140-6
Oke, T. R. (1981). Canyon geometry and the nocturnal urban heat island: comparison of scale model and field observations. J. Climatol. 1:237–254. doi: 10.1002/joc.3370010304
Oke, T. R. (1997). “Urban climates and global environmental change,” in Applied Climatology: Principles and Practices, eds. Thompson, R. D. and Perry, A. (New York: Routledge) 273–287.
Parker, D. E. (2010). Urban heat island effects on estimates of observed climate change. Wiley Interdiscip. Rev. Clim. Change. 1, 123–133. doi: 10.1002/wcc.21
Peng, S., Piao, S., Ciais, P., Friedlingstein, P., Ottle, C., Bréon, F. M., et al. (2012). Surface urban heat island across 419 global big cities. Environ. Sci. Technol. 46, 696–703. doi: 10.1021/es2030438
Peterson, T. C., Heim, R. R., Hirsch, R., Kaiser, D. P., Brooks, H., Diffenbaugh, N. S., et al. (2013). Monitoring and understanding changes in heat waves, cold waves, floods, and droughts in the United States: state of knowledge. Bull. Amer. Meteorol. Soc. 94, 821–834. doi: 10.1175/BAMS-D-12-00066.1
Raj, S., Paul, S. K., Chakraborty, A., and Kuttippurath, J. (2020). Anthropogenic forcing exacerbating the urban heat islands in India. J. Environ. Manag. 257, 110006. doi: 10.1016/j.jenvman.2019.110006
Robinson, P. J. (2001). On the definition of a heat wave. J. Appl. Meteorol. 40, 762–775. doi: 10.1175/1520-0450(2001)040<0762:OTDOAH>2.0.CO;2
Romanello, M., McGushin, A., Di Napoli, C., Drummond, P., Hughes, N., Jamart, L., et al. (2021). The 2021 report of the lancet countdown on health and climate change: code red for a healthy future. Lancet. 398, 1619–1662. doi: 10.1016/S0140-6736(21)01787-6
Santamouris, M. (2015). Analyzing the heat island magnitude and characteristics in one hundred Asian and Australian cities and regions. Sci. Total Environ. 512, 582–598. doi: 10.1016/j.scitotenv.2015.01.060
Santamouris, M. (2016). Innovating to zero the building sector in Europe: minimising the energy consumption, eradication of the energy poverty and mitigating the local climate change. Sol. Ener. 128, 61–94. doi: 10.1016/j.solener.2016.01.021
Sanusi, R., and Jalil, M. (2021). Blue-Green infrastructure determines the microclimate mitigation potential targeted for urban cooling. IOP Conf. Ser. Earth Environ. 918, 012010. doi: 10.1088/1755-1315/918/1/012010
Sarangi, C., Qian, Y., Li, J., Leung, L. R., Chakraborty, T. C., and Liu, Y. (2021). Urbanization amplifies nighttime heat stress on warmer days over the US. Geophys. Res. Lett. 48, e2021GL095678. doi: 10.1029/2021GL095678
Shastri, H., Barik, B., Ghosh, S., Venkataraman, C., and Sadavarte, P. (2017). Flip flop of day-night and summer-winter surface urban heat island intensity in India. Sci. Rep. 7, 1–8. doi: 10.1038/srep40178
Shen, P., and Zhao, S. (2021). 1/4 to 1/3 of observed warming trends in China from 1980 to 2015 are attributed to land use changes. Clim. Change. 164, 1–19. doi: 10.1007/s10584-021-03045-9
Shreevastava, A., Prasanth, S., Ramamurthy, P., and Rao, P. S. C. (2021). Scale-dependent response of the urban heat island to the European heatwave of 2018. Environ. Res. Lett. 16, 104021. doi: 10.1088/1748-9326/ac25bb
Siddiqui, A., Kushwaha, G., Nikam, B., Srivastav, S. K., Shelar, A., and Kumar, P. (2021). Analysing the day/night seasonal and annual changes and trends in land surface temperature and surface urban heat island intensity (SUHII) for Indian cities. Sustain. Cities Soc. 75, 103374. doi: 10.1016/j.scs.2021.103374
Swain, S., Bhattacharya, S., Dutta, A., Pati, S., and Nanda, L. (2019). Vulnerability and adaptation to extreme heat in Odisha, India: a community based comparative study. Int. J. Environ. Res. Public Health 16:24,5065. doi: 10.3390/ijerph16245065
Taubenböck, H., Esch, T., Felbier, A., Wiesner, M., Roth, A., and Dech, S. (2012). Monitoring urbanization in mega cities from space. Rem. Sens. Environ. 117, 162–176. doi: 10.1016/j.rse.2011.09.015
Taubenböck, H., Wegmann, M., Roth, A., Mehl, H., and Dech, S. (2009). Urbanization in India–Spatiotemporal analysis using remote sensing data. Comput. Environ. Urban Syst. 33, 179–188. doi: 10.1016/j.compenvurbsys.2008.09.003
Taubenböck, H., Wegmann, M., Wurm, M., Ullmann, T., and Dech, S. (2010). The Global trend of urbanization: spatiotemporal analysis of megacities using multi-temporal remote sensing, Landscape metrics, and gradient analysis. SPIE Rem. Sens. 7831, 135–154. doi: 10.1117/12.864917
The Times of India (2015). You're Experiencing World's 5th Deadliest Heatwave Ever. Newspaper article. Available online at: http://timesofindia.indiatimes.com/articleshow/47485972.cms?utm_source=contentofinterestandutm_medium=textandutm_campaign=cppsthttps://timesofindia.indiatimes.com/india/youre-experiencing-worlds-5th-deadliest-heatwave-ever/articleshow/47485972.cms (accessed December 8, 2022).
Tippett, M. K. (2018). Extreme weather and climate. NPJ Clim. Atmos. Sci. 1, 1–2. doi: 10.1038/s41612-018-0057-1
Tran, K. V., Azhar, G. S., Nair, R., Knowlton, K., Jaiswal, A., Sheffield, P., et al. (2013). A cross-sectional, randomized cluster sample survey of household vulnerability to extreme heat among slum dwellers in Ahmedabad, India. Int. J. Environ. Res. Public Health. 10, 2515–2543. doi: 10.3390/ijerph10062515
United Nations (UN) (2018a). The World's Cities in 2018- Data Booklet. Department of Economic and Social Affairs, Population Division. Available online at: https://www.un.org/development/desa/pd/sites/www.un.org.development.desa.pd/files/files/documents/2020/Jan/un_2018_worldcities_databooklet.pdf (accessed June 19, 2022).
United Nations (UN) (2018b). Revision of World Urbanization Prospects. United Nations, New York. Available online at: https://population.un.org/wup/Publications/Files/WUP2018-Report.pdf (accessed August 8, 2020).
United Nations (UN) (2022). World Population Prospects 2022: Summary of Results. Department of Economic and Social Affairs, Population Division. Available online at: https://www.un.org/development/desa/pd/sites/www.un.org.development.desa.pd/files/wpp2022_summary_of_results.pdf (accessed July 17, 2022).
Voogt, J. A., and Oke, T. R. (2003). Thermal remote sensing of urban climates. Rem. Sens. Environ. 86, 370–384. doi: 10.1016/S0034-4257(03)00079-8
Wang, P., Huang, C., de Colstoun, E. C. B., Tilton, J. C., and Tan, B. (2017). Documentation for the Global Human Built-up and Settlement Extent (HBASE) Dataset from Landsat. Palisades, NY: NASA Socioeconomic Data and Applications Center (SEDAC). doi: 10.7927/H4DN434S (accessed April 23, 2021).
Wehner, M., Stone, D., Krishnan, H., AchutaRao, K., and Castillo, F. (2016). The deadly combination of heat and humidity in India and Pakistan in summer 2015. Bull. Amer. Meteorol. Soc. 97, S81–S86. doi: 10.1175/BAMS-D-16-0145.1
World Health Organization (WHO) (2020). Information and public health advice: heat and health. https://www.who.int/globalchange/publications/heat-and-health/en/ (accessed September 16, 2020).
Xian, G., and Crane, M. (2006). An analysis of urban thermal characteristics and associated land cover in Tampa Bay and Las Vegas using Landsat satellite data. Rem. Sens. Environ. 104, 147–156. doi: 10.1016/j.rse.2005.09.023
Yoo, C., Han, D., Im, J., and Bechtel, B. (2019). Comparison between convolutional neural networks and random forest for local climate zone classification in mega urban areas using Landsat images. ISPRS J. Photogramm. Rem. Sens. 157, 155–170. doi: 10.1016/j.isprsjprs.2019.09.009
Zhang, X., Liu, L., Wu, C., Chen, X., Gao, Y., Xie, S., et al. (2020). Development of a global 30 m impervious surface map using multisource and multitemporal remote sensing datasets with the Google Earth Engine platform. Earth Syst. Sci. Data. 12, 1625–1648. doi: 10.5194/essd-12-1625-2020
Zhou, D., Zhao, S., Liu, S., Zhang, L., and Zhu, C. (2014). Surface urban heat island in China's 32 major cities: spatial patterns and drivers. Rem. Sens. Environ. 152, 51–61. doi: 10.1016/j.rse.2014.05.017
Keywords: surface urban heat island intensity (SUHII), nighttime SUHII, urban climate, global warming, heat stress, heat-related mortality, remote sensing, Indian cities
Citation: Jain M (2023) Two decades of nighttime surface urban heat island intensity analysis over nine major populated cities of India and implications for heat stress. Front. Sustain. Cities 5:1084573. doi: 10.3389/frsc.2023.1084573
Received: 30 October 2022; Accepted: 27 January 2023;
Published: 16 February 2023.
Edited by:
Florent Louis, Université de Lille, FranceReviewed by:
Robert Balling, Arizona State University, United StatesTao Lin, Institute of Urban Environment (CAS), China
Copyright © 2023 Jain. This is an open-access article distributed under the terms of the Creative Commons Attribution License (CC BY). The use, distribution or reproduction in other forums is permitted, provided the original author(s) and the copyright owner(s) are credited and that the original publication in this journal is cited, in accordance with accepted academic practice. No use, distribution or reproduction is permitted which does not comply with these terms.
*Correspondence: Madhavi Jain, bWFkaGF2aWphaW4uOTBAZ21haWwuY29t