Decision Framework to Balance Environmental, Technical, Logistical, and Economic Criteria When Designing Structures With Reused Components
- 1Structural Xploration Lab, Swiss Federal Institute of Technology, Fribourg, Switzerland
- 2Laboratory for Maintenance and Safety of Structures, Swiss Federal Institute of Technology, Lausanne, Switzerland
The reuse of structural components in new buildings has great potential to reduce the environmental impacts of the construction sector but remains uncommon practice. An obstacle to its wider implementation is the lack of robust assessment methods and decision-making tools that consider the full spectrum of benefits and drawbacks. This paper proposes a multi-criteria decision framework that builds on a simulated set of design alternatives with varying ratios of reused and new structural components. A set of performance criteria is presented, addressing procurement risks, construction technique complexity, environmental impacts, and project costs. As the independent criterion evaluations often deliver conflicting results, a multi-criteria decision analysis helps identify the most appropriate solution. The design of a steel Pratt truss is used as a case study to demonstrate the applicability of the framework. Different alternatives with reuse rates above 65% are recommended for each preference scenario, reducing between 35 and 45% of adverse environmental impacts in comparison to an equivalent design made of new elements only. The study underpins the principle that there is no trivial and unique best option when designing with reused components. Multi-criteria decision analyses applied to structures with varying reuse rates constitute a promising tool to support decision-makers.
Introduction
The construction industry is currently the largest raw-material consumer worldwide (World Economic Forum, 2016). In Europe, the building sector is responsible for 36% of solid waste (Eurostat, 2020) and 40% of energy demand (European Commission, 2019). Construction and demolition activities worldwide are estimated to account for 11% of the overall energy- and process- related CO2 emissions (IEA, 2019), to which load-bearing systems contribute substantially (Kaethner and Burridge, 2012; Hoxha et al., 2017; Röck et al., 2020). Faced with natural-resources depletion, waste accumulation and climate change, efficient strategies need to be implemented in the building sector to lower its environmental impacts.
Today, conventional practice concerning obsolete structures in industrialized countries leads to their demolition and the recycling or the landfilling of their materials (Addis, 2006). In the Delft Ladder, which prioritizes resource management strategies according to their environmental impacts, one strategy that precedes recycling and landfilling is the reuse of building components beyond their first use and without reprocessing them (Braungart and McDonough, 2002; Baker-Brown, 2017). Reuse extends the use of building components for multiple service cycles, maintaining their formal and mechanical characteristics as much as possible (Ghyoot et al., 2018). Component reuse was for a long time a common practice in construction until it faded away following the industrial revolution and the decrease in production costs (Choppin et al., 2014). Today reuse is recognized by the International Energy Agency (IEA, 2019) as a key action and research axis to enable greater material efficiency and by the European Union (Bourguignon, 2018) as a necessary strategy toward a circular economy. Indeed, major environmental and urban challenges call for a circular economy and a design practice that includes reuse on a larger scale (Kirchherr et al., 2017; Charter, 2018).
As structural components contribute a significant share of the embodied carbon of buildings (Cole and Kernan, 1996; Kaethner and Burridge, 2012; Häkkinen et al., 2015; De Wolf et al., 2020b), this work focuses on the reuse of structural components which avoids new component production, energy-intensive recycling processes, possible short-term landfilling and hence usually lowers environmental impacts (Pongiglione and Calderini, 2014; Cooper and Gutowski, 2017; Gorgolewski, 2017; Brütting et al., 2020b). Different to conventional structural design, designing structures with reused components implies that the design is strongly influenced by a limited stock of reclaimed structural components (Gorgolewski, 2008) alongside additional challenges specific to the “reuse” supply chain: reconditioning; testing; selective deconstruction (Figure 1). As designing and building with reclaimed components involves significant differences from using new or recycled components, design processes, costs, environmental impacts and project management are affected (Addis, 2006). Consequently, specific design and decision support tools become necessary to understand and assess the impacts of reuse.
Regarding design tools, structural optimization is commonly employed in research and practice to obtain optimal designs subject to typical boundary conditions and loads (Ohsaki, 2016; Lagaros, 2018). Brütting et al. (2019, 2020b) presented a discrete structural optimization method to design reticular structures (e.g., trusses and frames) with reused and new components and subject to ultimate and serviceability limit state constraints. The optimization method considers the availability and mechanical properties of reclaimed structural components. The output is the optimal combination of reused and new members in the structure, identifying the reuse rate (RR) that minimizes the structure environmental impact. The formulation is based on Mixed-Integer Linear Programming (MILP) and thus provides globally optimal solutions (Nemhauser and Wolsey, 1999).
Regarding impacts of reuse on construction projects, the associated consequences have been described extensively and are of economic, technical, environmental, organizational and design nature (Gorgolewski and Morettin, 2009; Gorgolewski, 2011; Rakhshan et al., 2020). Today, partial and fragmented knowledge about the impacts of structural components does exist but no study has addressed all of these multiple and diverse factors when designing structures with reused elements. To achieve a wider implementation of reuse, efficient tools are needed to qualify and quantify the full spectrum of impacts related to reuse and to understand when reuse is the most beneficial (Densley Tingley et al., 2017). Holistic and transparent assessment methods are thus required to support multi-criteria decision-making for integrating reclaimed components in new construction projects.
Regarding environmental implications of reuse, Life Cycle Assessment (LCA) is a widely recognized method to assess environmental impacts and it is broadly used in the construction field. LCA accounts for all substances exchanged with the environment during production, use, and end-of-life phases of products (International Organisation for Standardisation, 2006; European Committee for Standardization, 2011). Product environmental impacts are not only related to the type and the amount of material it is made of, but also to the impacts, positive or negative, that such material has on the environment during service. As a result, two similar products that are obtained through different processes, here the “reuse” and “new” routes, have different environmental impacts. LCA has been for example applied to steel reuse by Yeung et al. (2017) and combined with structural optimization for steel structures in works by Brütting et al. (2018, 2019, 2020b).
Regarding economic impacts, costs analysis is frequently used to compare project-cost estimations of design alternatives (Islam et al., 2015), yet it has only been sporadically applied to reuse. A cost analysis of steel reuse case studies in the UK has been conducted by Dunant et al. (2018). Kim and Kim (2020) proposed a design tool for steel reuse that provides a life-cycle cost analysis parallelly to an LCA. An underlying result of both works is the generally conflicting nature of economic costs and environmental impacts of reuse. Decision-making based on conflicting criterion results are challenging and require adapted decision-supporting tools.
Besides existing tools to assess environmental and economic impacts of reuse, complementary metrics and quantification methods are lacking, especially to evaluate technical, social and organizational impacts of reusing components in new designs. Also, decision-making on reuse depends on multiple criteria but existing methodologies are limited to an independent evaluation of impacts and do not provide support in case of conflicting criteria, a situation where the best design option must be identified according to decision-makers preference. For these reasons, there is a need for a multi-criteria decision analysis (MCDA) framework taking into account the full range of benefits and drawbacks associated with reuse to recommend the best rate of reuse components according to decision-maker preferences.
MCDA is a robust tool to support decision-making in problems presenting multiple conflicting criteria that create complex tradeoffs. MCDA is appropriate to support the reuse of components in new projects as it offers rational support to decision-makers when comparing alternatives based on multiple, often contradictory, criteria (Greco et al., 2016). Assets of MCDA are the explicit selection of essential criteria, the incorporation of several decision-makers preferences and an informed assessment of the alternatives in the form of a ranking, a score or classification (Cinelli, 2017). Different MCDA methods have been developed over the past decades, including outranking approaches such as the Preference Ranking Organization Method for Enrichment Evaluations (PROMETHEE) (Brans and De Smet, 2016), the Elimination and Choice Expressing the Reality method (ELECTRE) (Figueira et al., 2013) and the Multi-Attribute Utility Theory (MAUT) (Dyer, 2005). The potential of MCDA in construction has been shown by Jato-Espino et al. (2014). Regarding reuse, MCDA frameworks have been developed for on-site adaptive reuse of heritage buildings (Wang and Zeng, 2010; Ferretti and Comino, 2015), refurbishment of non-heritage buildings (Silva and Almeida, 2013), and design-for-disassembly internal partitioning walls (Rajagopalan et al., 2021). Despite, today no study has adapted MCDA for the reuse of individual reclaimed structural components in new designs for load-bearing systems. This paper proposes a multi-criteria decision-making framework for the reuse of components in new structural projects. By combining several databases and methods, this research provides a comprehensive methodology useful to researchers, engineers and the construction industry when designing with reused components. The process of design-option generation, from either reused or new components or from a combination of both, is described using an existing structural optimization method. Then objectives are two-fold. First, a comprehensive set of performance criteria is developed, introducing new metrics for the procurement risks and construction complexity in addition to environmental impacts and project costs. Then, to recommend the best structural design option according to decision-maker preferences, an MCDA approach is proposed. The applicability of the framework is demonstrated through a steel truss case study and options are recommended for several decision-maker preference scenarios. The findings of this study broaden the understanding of component reuse as a sustainable approach toward material efficiency in line with international sustainable development goals (United Nations, 2020).
The study is organized as follows. Section Multi-Criteria Decision-Making Framework for the Reuse of Components in New Structural Projects describes the framework of supporting decision-making for new structural designs when reusable components are available. Section Case Study includes the application of the framework on a case study of a steel truss. Discussion of the work, its extension to other component reuse types and future work are presented in section Discussion. Section Conclusions concludes the study.
Multi-Criteria Decision-Making Framework for the Reuse of Components in New Structural Projects
Overview
This work proposes a framework to recommend the best design alternatives for the new construction of a steel load-bearing system (structure) from either reused or new structural linear components or from a combination of both. An underlying assumption of this work is that a stock of suitable reclaimed components has been identified a priori. The known stock characteristics include the component material properties, lengths, cross-sections and quantities. New structural components are alternatively produced in unlimited quantity and length and with typical standard cross-sections. Once the stock has been characterized, the selection of the best design alternative with the most suitable ratio of reused components is desired. In the following, this ratio is termed reuse rate (RR) and defined as the ratio between the mass of the new construction project made from reused components (Mreuse) over its total mass (Mstructure) (Brütting et al., 2020b), i.e., as RR = Mreuse/Mstructure.
The identification of the best design alternatives, i.e., solutions with the most suitable RR, is challenging due to the conflicting nature of multiple evaluation criteria, such as economic costs and environmental benefits. Therefore, a holistic framework for consideration of the implications of designing a new construction project partially or entirely with reused components may reduce difficulties in making an informed decision on the respective advantages and drawbacks. This work proposes such a framework built upon four phases that are shown in the flow chart in Figure 2 and detailed in the following sections.
Phase 1: Design-Alternative Generation
Phase 1 aims to generate design alternatives subject to the stock of available structural components and within the constraints of the new construction project. This phase is divided into three stages:
First, the construction and design constraints of the new project are identified. The constraints will generally include overall dimensions, minimum normative performance requirements (e.g., structural performance) and expected visual and spatial characteristics (e.g., typology, joint location, etc.).
Second, the design variables of the new construction project are defined. The variables describe the features that can be altered to generate design alternatives while respecting the project constraints. In this work, the central design variable is the reuse rate (RR), which ranges between 0% (all new) and 100% (all reuse).
Third, the generation of design alternatives resulting from the variation of one or several design variables begins. In this work, the main variable is the RR of the structure. Since structures with the same RR may have very different features (e.g., different material mass or utilization rates), design alternatives are here structures that minimize total material mass for prescribed ranges of RR.
Ranges of RR are defined by sets of 22 upper (UB) and lower (LB) bounds in 5-% steps: RRLB = [0; 0; 5; 10; …; 95; 100%] and RRUB = [0; 5; 10; 15; …; 100; 100%]. The actual RR resulting from each optimization is a specific value within each of the 5% domain steps. When lower and upper bounds are set to the identical value (cf. RRLB = RRUB = 0 or 100%), the optimization is constrained to output a solution with exactly the specified RR. Because of the discrete nature of the problem, i.e., in which both the truss structure and stock are defined by discrete quantities of components, explicitly prescribing an RR is impossible except for the two extremes all-new (RR = 0%) and all-reuse (RR = 100%).
The total material mass Mtotal being minimized for each range of RR is defined as:
where Mreuse is the mass of reused components eventually used in the structure, Mwaste is the mass of the cut-off waste produced when calibrating stock components, and Mnew is the total mass of new components used in the structure. The sum of Mreuse and Mnew gives the total mass of the structure Mstructure (cf. section Overview) and the sum of Mreuse and Mwaste equals the mass of reused stock components Mstock before cutting. Note that in this work neither environmental impacts nor costs are minimized by the structural optimization method as it is expected that this would interfere with the strategy to eventually process these criteria through MCDA.
Minimization of total material mass for each range of RR provides a global solution thanks to a state-of-the-art discrete structural optimization method based on Mixed-Integer Linear Programming (MILP) (Brütting et al., 2020b). Conceptually, the MILP optimization is formulated as:
The assignment of a part of a stock or a new element j at member position i is represented via a binary design variable tij which is an entry of the assignment matrix T ϵ {0;1}m×s, where s is the size of the stock of reclaimed elements plus the size of the set of elements available from new production. Another set of binary design variables y ϵ {0;1}s is defined to model the state when structural members are cut from a stock element (yj = 1) or not (yj = 0). Equation (2b) ensures that for each of the i = 1… m truss members exactly one stock or new element is assigned. Equation (2c) ensures that the use of stock element j for one or more members is constrained by the available length, where l'i and lj denote the member and element length, respectively. Note that new elements have an “unlimited” length. Equation (2d) constrains the solution to the a-priori set RR bounds.
The optimization formulation is complemented with continuous state variables (member forces, nodal displacements) as well as structural constraints such as equilibrium of forces, geometric compatibility, deflection limits (serviceability limit state), stress and member buckling constraints (ultimate limit state). As shown in Brütting et al. (2018, 2019) these conditions can be equally formulated as linear constraints. The resulting MILP model is solved with the branch-and-cut solver of the commercial software Gurobi (Gurobi Optimization LCC, 2019).
Phase 2: Performance-Criterion Independent Assessment
To recommend a design solution to decision-makers, the set of design alternatives generated during Phase 1 must be evaluated according to defined project-specific criteria. As the construction industry is responsible for a large part of current environmental damages, the reduction of environmental impacts is a driver for structural component reuse (Addis, 2006). Consequently, the structure environmental impact is employed as a first evaluation criterion. The economic viability of the project is another point of consideration when making decisions on the reuse of components (Rakhshan et al., 2020). Project costs are therefore employed as a second evaluation criterion. In practice the risk related to the supply-chain of reclaimed components can have major consequences on the project feasibility (Dunant et al., 2017). Unavailability of components and their procurement from a large number of different suppliers might result in project delays and require more complex project management. Consequently, the number of different sources for reclaimed components is considered as a third evaluation criterion. Eventually, the reuse of reclaimed components involves working with pre-existing stock features, which may require additional work to calibrate and integrate the components into a new construction project. The fourth evaluation criterion therefore compares the level of construction complexity of the design alternatives. All four criteria and their respective quantification methods are detailed in the following subsections (Figure 3).
Environmental Impacts
Environmental impacts of each design alternative are quantified through Life Cycle Assessment (LCA). While building environmental impacts are often evaluated exclusively regarding climate change, construction activities imply broader environmental damages such as the use of natural resources or the degradation of ecosystems. In this paper, environmental impacts are expressed in terms of a single environmental point score, calculated with the “ReCiPe” Endpoint (H) V1.12 impact method (Goedkoop et al., 2013; Huijbregts et al., 2017), which includes an extended spectrum of environmental damage indicators such as climate change, human health, and fossil resource depletion.
For LCA that includes reuse or recycling, the environmental impacts of the associated products must be allocated over multiple uses (Allacker et al., 2017; De Wolf et al., 2020a). In the present study, the cut-off approach, also known as the recycled content approach, is used (Ekvall and Tillman, 1997; Schrijvers et al., 2016). In this approach, the impacts related to recycling or reuse are attributed to the product that uses recycled or reused content. Conversely, no impact is attributed to the previous product that provides the materials to be recycled or reused and no assumption is made for future recyclability or reusability of the employed reclaimed component.
The LCA methodology, system boundary, material flows and process impacts to assess the environmental impacts of design alternatives with different RRs are those presented in Brütting et al. (2020b). The total environmental impacts of a design alternative, i.e., a structural system, are the sum of all process environmental impacts within the defined system boundary (Figure 3) from sourcing over manufacturing to assembling the structure components, including transports (cradle-to-gate). Starting from an obsolete building that serves as a material bank, two routes “reuse” and “new” are distinguished. The “reuse” route involves that a building structure is deconstructed, components are refurbished and eventually reused in the new construction project. The “new” route considers that a demolished building structure is turned into steel scrap and then new structural components are produced from a mix of primary and secondary (recycled) steel. The use and maintenance phases as well as the end-of-life phase of the new construction project are excluded from the system boundary because the exchanges with the environment during these phases are expected to be identical for all design alternatives and both routes, independent of RR. Environmental impacts related to material testing and certification of reclaimed components as well as structural design work are neglected in the LCA.
The numeric values for all process environmental impacts EIPro are adapted from Brütting et al. (2020b) and given in Table 1. They are computed from Ecoinvent 3.1 (Wernet et al., 2016), Gabi (Gordon, 1997; Millman and Giancaspro, 2012; Thinkstep, 2021). Process environmental impacts are multiplied with the respective system metric or material quantity (e.g., structure mass or surface area) to obtain the total environmental impacts. For example, the environmental impacts associated with deconstruction to reclaim a structural component is the product of its mass with the process impact factor for deconstruction EIDC. Equivalently, the environmental impacts associated with the new production of recycling steel components are computed from the component mass and EIP. Based on previous findings (Brütting et al., 2020b), the total environmental impacts typically decrease with an increasing RR. A lower environmental-impact point score (ReCiPe) is preferable and relates to an environmentally less detrimental design alternative.
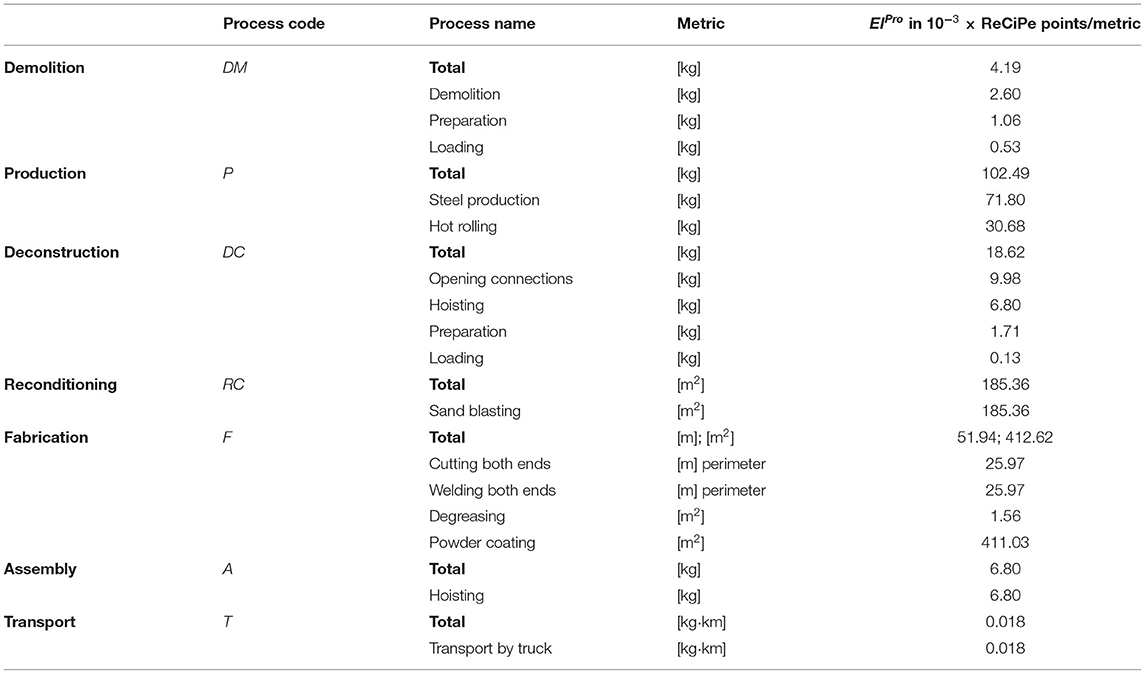
Table 1. Environmental impacts of “new” and “reuse” route processes (Brütting et al., 2020b).
Project Costs
The second evaluation criterion is the total project costs. As shown in Figure 3, the assessment of the total project costs collects all process costs from sourcing of materials until on-site assembly. Average indices for each process are taken from Dunant et al. (2018) and reported in detail in Table 2. The relative cost difference between “reuse” and “new” is assumed to be valid for the European context. These cost indices are related to system metrics such as structure mass and surface area and equally separated for both routes, “reuse” and “new.” In both routes, the cost indices include costs associated with the design process, off-site prefabrication stages and on-site assembly activities, but they differ in the early stages where materials are sourced and prepared. Equivalent to the LCA system boundary (section Environmental Impacts), for the “reuse” route, costs are associated with the selective deconstruction of buildings as well as the reconditioning of reclaimed components and additionally their testing. In the “new” route, costs relate to the production of new components with recycled content and hence include the costs for raw material extraction and demolition to obtain scrap material. Based on the provided cost indices and results presented by Dunant et al. (2018), costs commonly increase with an increasing reuse rate. To recommend a viable design alternative in the current economic model, construction costs should be minimized.
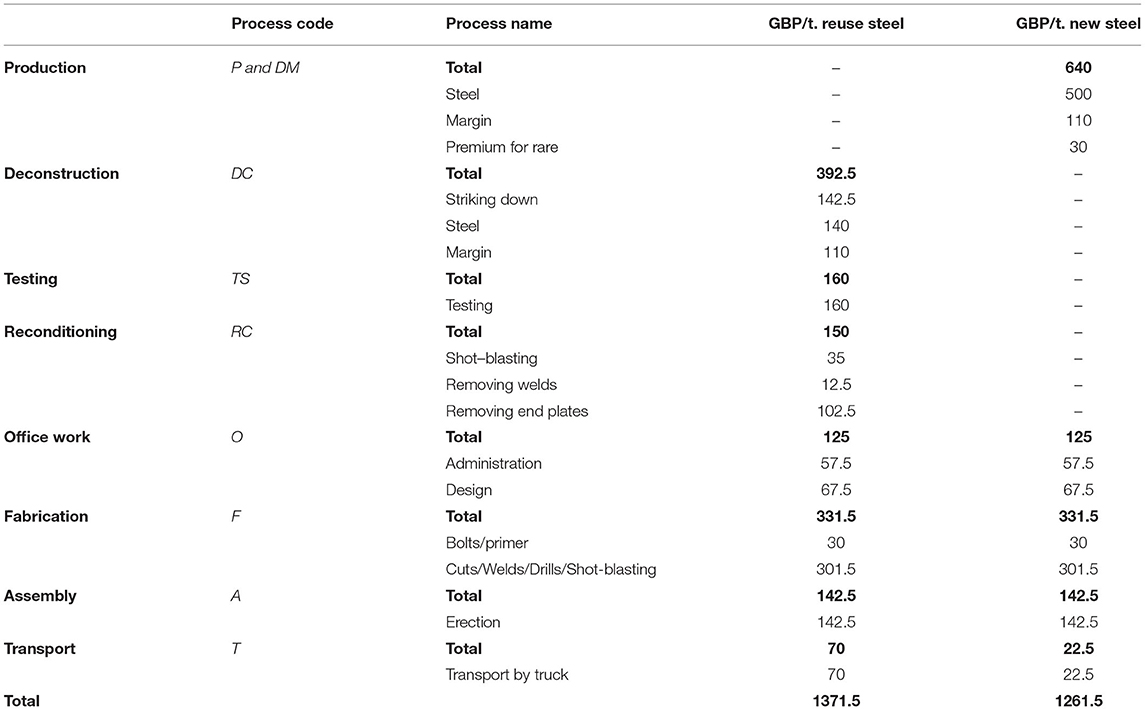
Table 2. Costs of “new” and “reuse” route processes, adapted from Dunant et al. (2018).
Procurement Risks
The procurement of reclaimed components differs strongly from that of new components. The supply chain of reclaimed components starts at the deconstruction sites, upon which it depends directly, and continues through reconditioning stages. Then, components are transported to off-site fabrication and eventually transported to the final site of the new construction project. Since today only a few specialized stockists exist, the supply chain for reclaimed components operates on a just-in-time basis, between deconstruction sites and new project sites. Construction and deconstruction projects are regularly subject to delays for a variety of reasons, including administrative delays. Keeping the number of deconstruction sites small not only reduces the complexity of the supply chain and therefore of project management but also reduces the risk of delays. Therefore, the third performance criterion is the total number of different deconstruction sites on which the design alternative depends. The number of deconstruction sites should be minimized to lower procurement risks.
Construction Complexity
The fourth evaluation criterion assesses the construction complexity due to structural component reuse. Design alternatives that combine large variations of reused components must be adapted to the specificities of component dimensions. This may require additional work to join and assemble all parts. For example, in conventional practice, when designing a steel truss, the aim is to reduce the number of colinear joints to facilitate easy assembly and reduce fabrication costs. When a limited stock of reclaimed components is used, the available components may be shorter than typical new ones and hence more colinear joints are required. The number of joints should be kept to a minimum to limit design complexity and extra assembly work. In this work, the construction complexity evaluation criterion is the number of colinear joints in the truss top and bottom chords.
Design-Alternative Independent Evaluation
Once all criteria are defined, design alternatives generated in Phase 1 are individually evaluated for each criterion independently. An illustrative example of such an evaluation is depicted in Figure 4, where each graph shows the performance of the four design alternatives a – d for each of the four criteria.
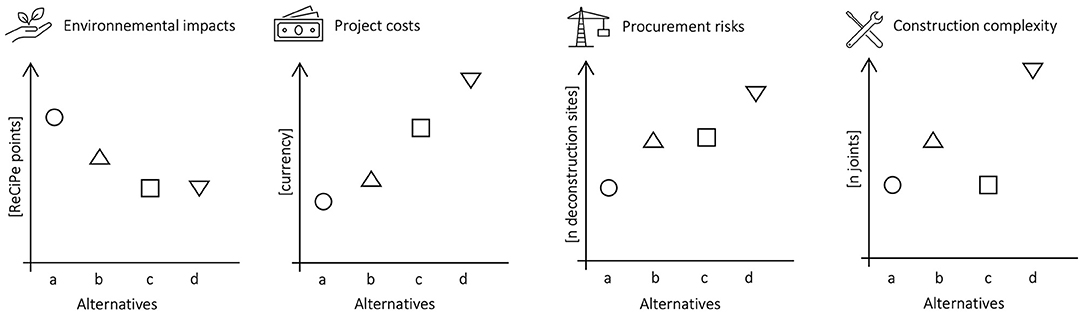
Figure 4. Evaluation of design alternatives for each criterion: Illustrative example of four design alternatives ordered from left by increasing reuse rate.
Phase 3: Multi-Criteria Decision Analysis
As evaluations of performance criteria such as the environmental impact and the project costs today are conflicting, recommending a design alternative is not trivial. The problem of depicting the best reuse rate (RR) in a new construction project is particularly suitable for Multi-Criteria Decision Analysis (MCDA). Additionally, creating a ranking of possible design alternatives based on their overall performance is useful to recommend the best option to decision-makers. Using an MCDA method is thus justified. The following defines the desired capabilities of the MCDA method and justifies the choice of PROMETHEE as the MCDA method recommended in the framework.
In this work, the principal requirement for the MCDA is to rank design alternatives. However, faced with conflicting criteria, finding the best solution depends on decision-maker preferences. This preference is typically formulated as a weight for each criterion (Cinelli, 2017). The selected MCDA method must thus be able to include this preference in the ranking of design alternatives.
Second, design alternatives are typically compared by decision-makers rather than independently evaluated. To reflect this decision-making process, the appropriate MCDA method must also be capable to rank alternatives based on pairwise comparisons.
Third, when two alternatives have similar performance-metric evaluations, decision-makers usually consider them as equal. Additionally, when the difference between options reaches a certain value, decision-makers may strictly prefer alternative a over alternative b. In MCDA, such situations are accounted for using thresholds of performance (Raphael, 2011). This situation is modeled using indifference and preference thresholds. The appropriate MCDA method must thus account for thresholds of performance in alternative comparisons.
Both the Preference Ranking Organization Method for Enrichment Evaluations (PROMETHEE) (Brans and Vincke, 1985; Brans and De Smet, 2016) and the Elimination and Choice Expressing the Reality method (ELECTRE) (Roy, 1991; Figueira et al., 2013) are frequently used methods that are aligned with the desired capabilities. These outranking methodologies are based on pairwise comparisons and provide a ranking of alternatives based on preference-weight scenarios and thresholds of performance for each criterion (Cinelli et al., 2020). In ELECTRE, veto thresholds can be used if the MCDA problem requires it. In this study, the choice of PROMETHEE for the selection of the best RR in new construction projects is supported by the fact that this MCDA method is described as easier to use and understand for decision-makers than ELECTRE (Salminen et al., 1998; Polatidis et al., 2006), whose exploitation procedures are sometimes considered more obscure (Cinelli et al., 2014).
PROMETHEE ranking is obtained using the following procedure. First, several MCDA parameters must be defined. Let be c = [ck=1, …, cn] the criterion vector of n criteria (here n = 4). For each criterion ck a weight wk is selected corresponding to the importance of this criterion ck with . For each criterion ck, a partial preference function Prefk(a, b) is selected, which represents the preference degree of alternative a over alternative b. A large value of function Prefk(a, b) shows that the alternative a is highly preferred over alternative b on criterion ck. Several preference functions have been defined. For instance, the V-shape function is defined following Equation (3), where indifference qk and preference pk thresholds satisfy qk < pk and Δk(a, b) = ck(a) − ck(b). Illustrated in Figure 5, the V-shape preference function is the most frequently used and is applied to all criteria in the proposed methodology.
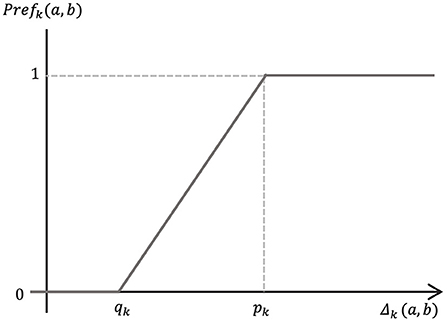
Figure 5. V-shape preference function. Adapted from Brans and Vincke (1985).
The indifference threshold qk corresponds to the greatest difference of performance evaluations between two options on criterion ck compatible with the decision-maker indifference. The preference threshold pk corresponds to the lowest metric-value difference between two alternatives on criterion ck, meaning that one is strictly preferred over the other on this criterion. These thresholds are selected using engineering judgment, specificities of criteria quantification methods and practical constraints. Discussions with engineers and architects may provide additional practical input.
For each pair of alternatives (a, b) ∈ A × A, the preference index π(a, b) is evaluated following Equation (4), where A denotes the set of all alternatives. π(a, b) is an evaluation of the degree of preference of alternative a over alternative b, taking into account all criteria:
In a second step, the positive ϕ+(a), negative ϕ−(a) and net ϕ(a) flows are calculated for each option a ∈ A based on Equations (5–7). The positive flow ϕ+(a) measures quantitatively when alternative a is preferred to the other alternatives (Equation 5), while the negative flow ϕ−(a) quantifies when other alternatives are preferred to alternative a (Equation 6). The net flow ϕ(a) (Equation 7) is the difference between positive and negative flows and expresses the strength of alternative a when compared with the set of all possible alternatives. Option a is preferred over alternative b if ϕ(a) > ϕ(b). Net-flow values are eventually ordered to provide the ranking of alternatives.
Phase 4: Design-Alternative Recommendation
The recommendation of the best design alternative is based on the ranking of the net-flow values. The highest net-flow values correspond to the best-ranked alternatives. Although the recommendations are not based on the absolute value of the net flows, a notable difference between the net-flow value of an alternative a over that of an alternative b is necessary to consider a significantly better than b. In some situations, several alternatives may have similar net flows, showing the incapacity of the method to select an optimal solution. In such cases, a more refined analysis could be made using SMAA-PROMETHEE (Corrente et al., 2014; Bertola et al., 2019), which is an extension of PROMETHEE to include additional performance metrics in the search for the best alternative.
Case Study
The following case study is aimed at evaluating the applicability of the method presented in section Multi-Criteria Decision-Making Framework for the Reuse of Components in New Structural Projects to a typical design project. The design of a pitched Pratt truss is chosen because it epitomizes a large number of common design tasks in practice. The goal of the MCDA is to find the most appropriate combination of reused and new components in the truss, according to preference scenarios that model stakeholders most likely choices.
The structure overall dimensions and member layout are presented in Figure 6A. For the structural optimization, all members are assumed to be pin-jointed (truss formulation). Loads are applied at top chord nodes and consist of superimposed dead load (roof) and snow load. A stock with nine different reclaimed component types with individual cross-section, length and number of available elements is manually simulated (Figure 6B). In absence of real-life data on stocks, it is assumed that such stock is representative of typical ones. The stock components are square hollow sections (SHS) of standard sizes from 40 × 3.2 to 90 × 8 (width and wall thickness in mm) as given in EN 10210 (European Committee for Standardization, 2019). The material is steel of grade S235 with a density of 7,850 kg/m3. Each of the nine stock element types is assumed to originate from an individual deconstruction site (cf. evaluation criterion “procurement risks,” section Procurement risks). New elements are all standard SHS cross-sections assumed to be manufactured with bespoke lengths. For more details on structure, stock and new element properties, the reader is referred to Brütting et al. (2020b).
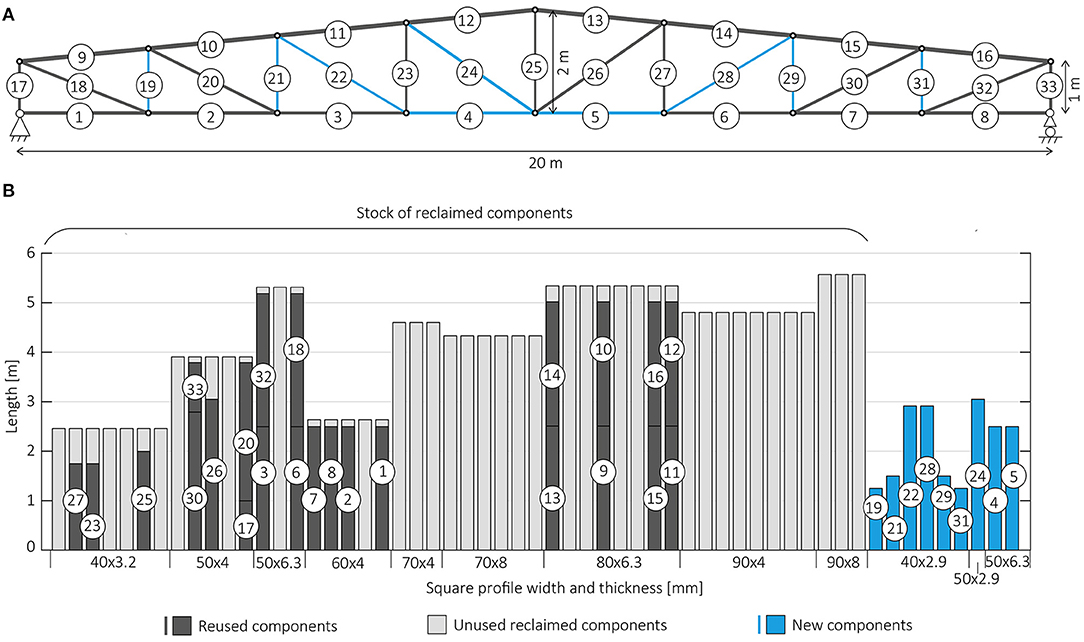
Figure 6. Alternative with a reuse rate of 85.0% and construction constraints. (A) Location of reused and new components; (B) list of components used in the alternative, mixing segments from the reclaimed stock and new components.
Generation of Design Alternatives Through Structural Optimization
The set of optimal truss design alternatives is generated by applying the MILP optimization procedure described in section Phase 1: Design-Alternative Generation. Figure 6 illustrates an optimized truss corresponding to the design alternative generated for a RR between or equal to RRLB = 85.0% and RRUB = 90.0%. Unused reclaimed components are represented as light-gray bars. Reclaimed component segments that are assigned to the optimized structure are indicated by dark-gray bars. In the optimization process, reclaimed components can be cut into one or more segments, but they cannot be extended. The cut-off lengths of stock components are represented as light-gray bars above the dark-gray ones. Components available from new production are represented as blue bars. New components are standard steel sections. They can be produced with any length and are directly adequately dimensioned. The number marked on each dark-gray or blue segment in Figure 6B corresponds to its member position in the structure in Figure 6A.
In practice, truss chords are often made of a single profile, whereas the structural optimization formulation would allow for different profiles for each chord member. From a structural analysis, it is evident that the normal forces are of very different magnitude in each chord member (larger forces at mid-span and lower ones near the supports). Therefore, the conventional practical rationalization to a single profile for the entire chord might result in very low capacity utilization. To mitigate between practicality and good material utilization, in this work, two design alternatives are generated via the optimization and for each 5% RR step: one with “fabrication constraints” and the other one without. These extra fabrication constraints explicitly limit the number of discontinuities among adjacent chord members (Brütting et al., 2020b) and hence contribute to reducing the number of colinear joints, whereas omission of fabrication constraints results in more assembly complexity (cf. section Construction Complexity). In detail, fabrication constraints ensure that: (1) the number of different profiles is limited to four in the top and bottom chords, and (2) cross-section width variation of adjacent members is limited to ±10 mm to ease joint fabrication (Brütting et al., 2020b).
As two alternatives are generated for each RR step, the set of design alternatives is composed of 44 options shown in Figure 7. Generally, the optimal RR obtained through the structural optimization method tends toward the set lower bound of each 5% step. This is explained by the fact that the optimization objective is the minimization of the total mass which is more easily achieved by integrating well-dimensioned new elements rather than potentially oversized reclaimed elements. This also explains the two overlapping solutions in Figure 8 as well as in Figure 10 with RR = 0% for the case with RRLB and RRUB explicitly set to 0% and with the range defined by RRLB = 0% and RRUB = 5%.
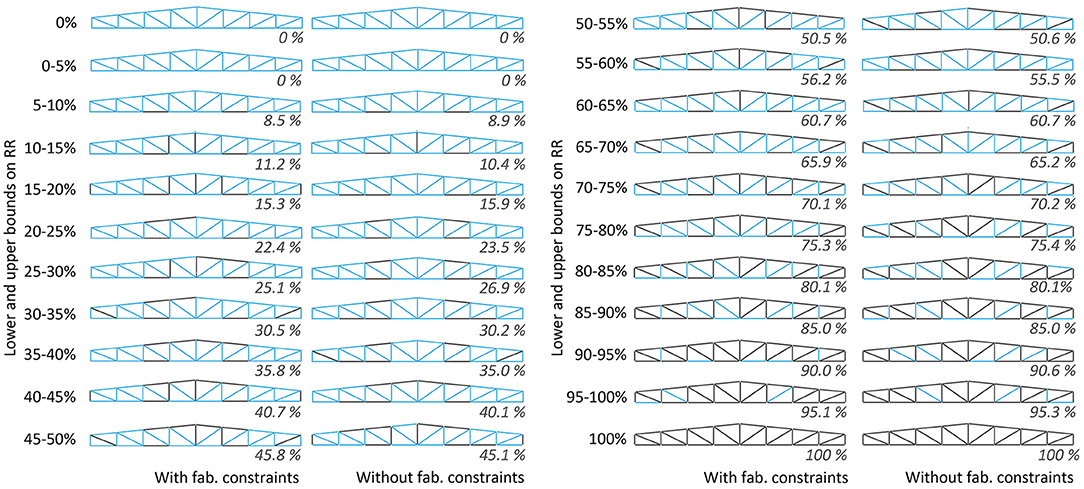
Figure 7. The 44 alternatives generated through structural optimization. New components are represented in blue and reused ones in dark gray. Black numbers indicate the bounds on RR and dark-gray italic numbers indicate the actual RR obtained through optimization.
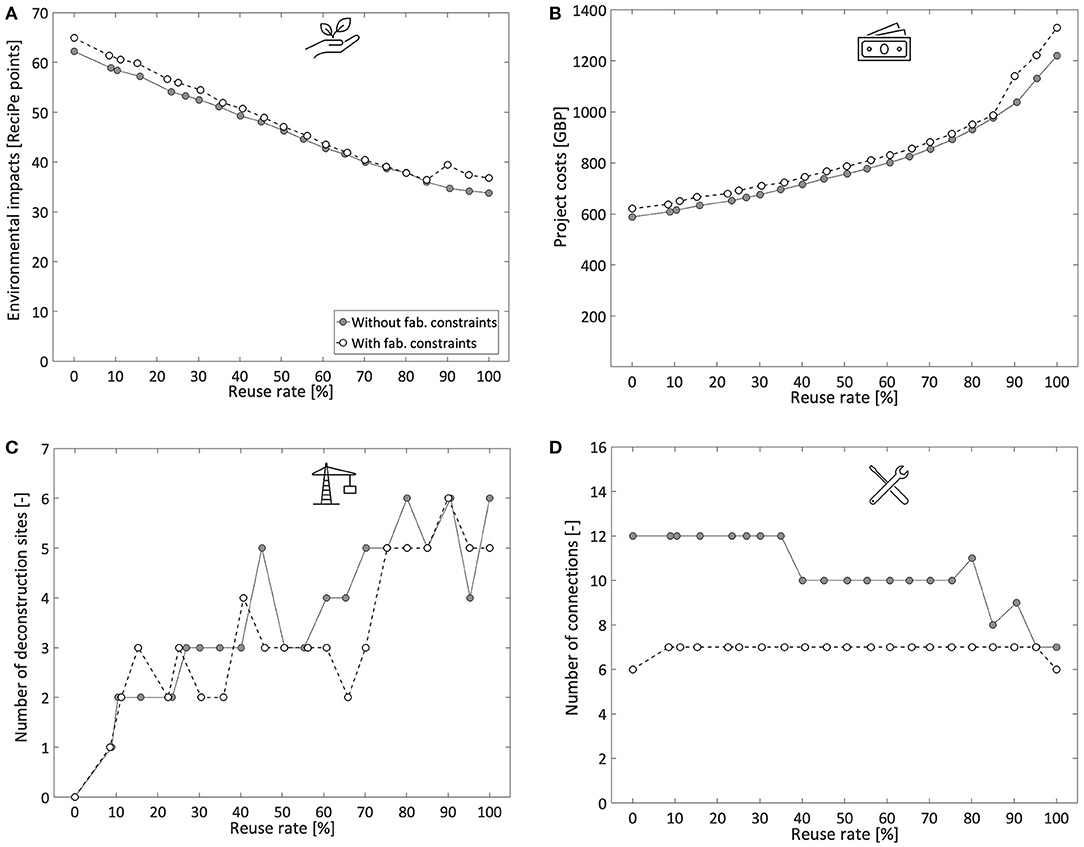
Figure 8. Evaluation of the set of alternatives for each criterion as a function of the reuse rate. (A) Environmental impacts; (B) project costs; (C) procurement risks; (D) construction complexity.
All generated design alternatives (i.e., MILP solutions) are global optima with respect to the set RR bounds. The computation time to obtain the globally optimal solution for one of the design alternatives ranges between 2 s and 247 s (mean 38 s), depending on RR bounds and inclusion of the fabrication constraints.
Criterion Independent Evaluation
Once generated, each design alternative is evaluated for each performance criterion individually, according to the four metrics presented in section Phase 2: Performance-Criterion Independent Assessment. Figure 8A plots the environmental impacts of the 44 alternatives against their reuse rate. Except for alternatives with fabrication constraints and a RR above 85%, the environmental impacts steadily decrease with increasing RR. The lowest environmental impacts are obtained for the alternative without fabrication constraints and RR = 100%. The difference between the three alternatives without fabrication constraints and RR ≥ 85% remains small. Figure 8B plots the project costs against RR. The project costs increase as RR increases. Costs non-linearly increase for alternatives with RR ≥ 85%. The all-new alternative (RR = 0%) without fabrication constraints has the lowest project costs, while all-reuse alternatives (RR = 100%) are the most expensive. Figure 8C plots the number of deconstruction sites against RR. With a few exceptions, the number of deconstruction sites increases in stages as RR increases. Four alternatives with RR ≥ 80% require the largest number of deconstruction sites (6) and thus have the highest procurement risks. Conversely, the risks are avoided by the absence of reclaimed components (RR = 0%). Figure 8D plots the number of joints against RR. The increase of joints here means an increase in construction complexity. The number of joints decreases with increasing RR for the alternatives without fabrication constraints. For the 22 alternatives with fabrication constraints, the number is lower and nearly constant. The alternatives with the lowest construction complexity are the alternatives with fabrication constraints and with a RR of 0 or 100%.
The evaluation of the 44 design alternatives for all performance criteria shows that there is no clear dominance of one alternative over all criteria. The evaluation confirms that criteria are conflicting: environmental impacts conflict with project costs and procurement risks, while the function related to construction complexity is optimal for two alternatives with extremes and opposite RR.
Multi-Criteria Decision Analysis
The evaluation of all alternatives for each construction criterion (section Criterion Independent Evaluation) is used as an input for the multi-criteria comparison. The multi-criteria comparison is performed with the PROMETHEE method (section Phase 3: Multi-Criteria Decision Analysis) using Visual PROMETHEE v. 1.4 Academic Edition (Mareschal, 2021).
V-shape functions are used for all criteria, requiring the setting of indifference q and preference p thresholds for each performance criterion (Table 3). Concerning the procurement risks and construction-complexity criteria, preference and indifference thresholds are fixed based on the highest and lowest metric-value difference obtained between two alternatives in the criterion independent evaluation. Regarding the environmental impacts, the indifference threshold is set to neglect the margin of error related to the ReCiPe method. Based on discussions with practitioners, the preference threshold is set to account for a strict preference of option a over option b when option a is 30% less detrimentally impactful than option b. The indifference q threshold of the project costs is set to neglect project-cost difference lower than 5%. The difference p threshold corresponds to the highest project-cost difference obtained between two options during the project-cost criterion evaluation.
The ranking of the alternatives obtained with PROMETHEE depends on the weighting attributed to each performance criterion. Preferences of decision-makers are case-study dependent. In this study, four preference scenarios are modeled to illustrate stakeholders' potential mindsets.
• Scenario 1 “equal weight”: the decision-makers attribute the same weight to all criteria;
• Scenario 2 “stockist”: a specialized actor organizes the supply chain, thus the procurement criterion is omitted;
• Scenario 3 “construction-complexity indifference”: the construction-complexity criterion has a smaller weight;
• Scenario 4 “high-environmental preference”: a larger weight is attributed to the environmental impact criterion.
The weighting factors of the four preference scenarios are detailed in Figure 9.
Comparisons of alternatives are performed to ultimately recommend the best one according to each preference scenario. The first comparison is performed for Scenario 1, where the four performance criteria are weighed equally (Figure 9). Figure 10 presents the net-flow value ϕ obtained for each alternative of the set against RR for this scenario at the top left. As the best alternative is the option with the highest net-flow value, the alternative with fabrication constraints and RR = 65.9% is thus clearly recommended as the best design alternative for Scenario 1. Note that the eight top-ranked alternatives are those with fabrication constraints. Generally, for RR ≤ 85%, alternatives with fabrication constraints perform better than alternatives with comparable RR but without fabrication constraints.
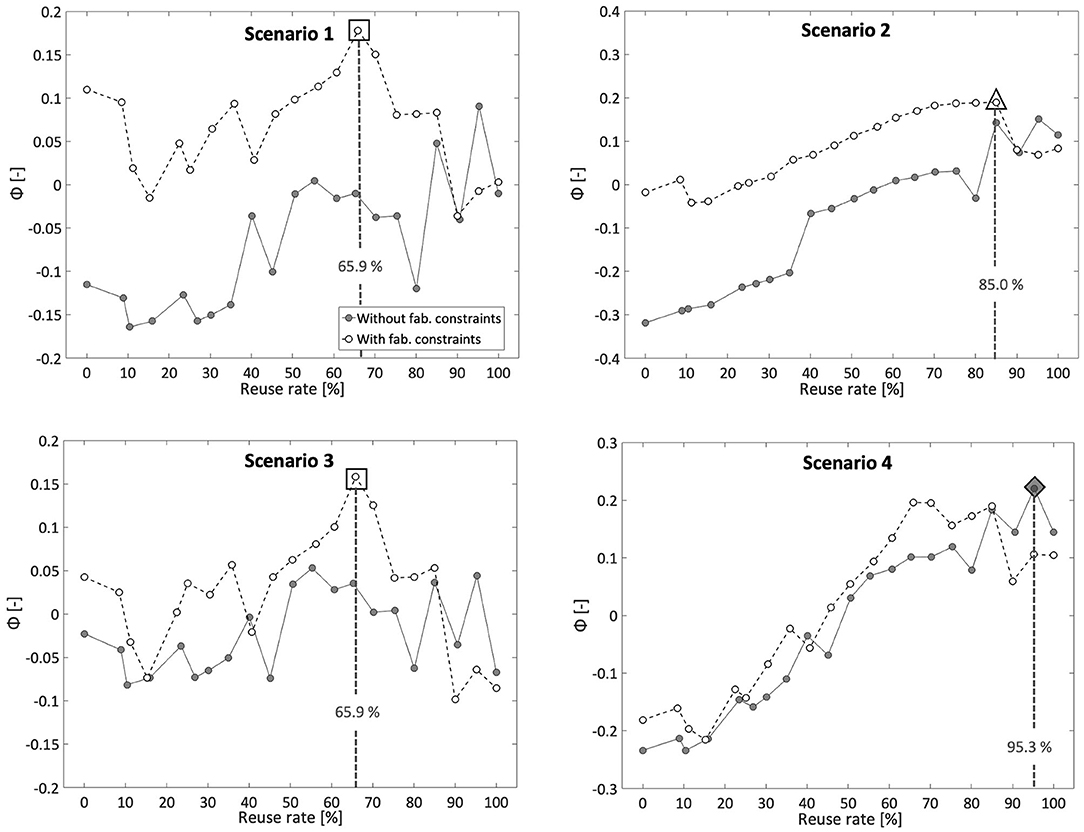
Figure 10. PROMETHEE net flow (Φ) of design alternatives as a function of the reuse rate for the four scenarios.
In Scenario 2, the existence of a specialized stockist is presumed. The presence of a specialized stockist on the market reduces the risks associated with the reclaimed component supply chain as it does no longer operate on a just-in-time basis. Hence, in the weighting preferences, the weight of procurement-risks criteria is omitted, and remaining preference weights are split equally between environmental impacts, project costs and construction complexity (Figure 9). Figure 10 plots the corresponding net-flow values at the top right. The alternative with fabrication constraints and RR = 85.0% is recommended as the best alternative although other alternatives with slightly lower RR present very close net-flow values ϕ. The recommended RR is thus about 20% higher than in Scenario 1. The existence of specialized stockists appears conducive to allowing the integration of more reused components in the new construction project and thus design decisions with less adverse environmental impacts.
In Scenario 3, the importance given by decision-makers to the construction complexity is low compared to the one given to the three other performance criteria: a weight of 10% is attributed to the construction complexity whereas a weight of 30% is attributed to each of the three other criteria (Figure 9). Figure 10 plots the net-flow values ϕ against RR for Scenario 3 at the bottom left. As in Scenario 1, the alternative with fabrication constraints and RR = 65.9% is recommended as the best alternative. The distribution of net-flow values ϕ across reuse rates actually presents similarities in both scenarios 1 and 3. This is related to the fact that, in this case study, the design-complexity criterion has similar values for all the alternatives generated with fabrication constraints, except the alternatives with RR = 0% and RR = 100%.
In Scenario 4, decision-makers give higher importance to environmental issues than in previous scenarios. The weight attributed to the environmental-impact criterion is 50%, whereas this criterion is weighted with only 25–33% in the first three scenarios (Figure 9). As shown at the bottom right in Figure 10, the alternative without fabrication constraints and RR = 95.3% is recommended as the best alternative in this scenario. In comparison with Scenarios 1 and 3, a “high environmental preference” favors an increase of RR by 30%. Generally, greater valuation of environmental impacts leads to the selection of alternatives with higher RR. Still, the alternative that was identified as the best option in scenarios 1 and 3 (RR = 65.9%) also performs very well in scenario 4.
Figure 11A summarizes the design alternatives that are recommended for the four preference scenarios. These results are discussed using the alternative with construction constraints and no reused components (RR = 0%) as a representative benchmark of today's conventional practice. In this case study, this benchmark also corresponds to the alternative that would be preferred by decision-makers who would attribute no or very little weight to the environmental benefits related to reuse in their decision-making. Figure 11B plots the respective values of the recommended alternatives in addition to the conventional benchmark option for the four evaluation criteria.
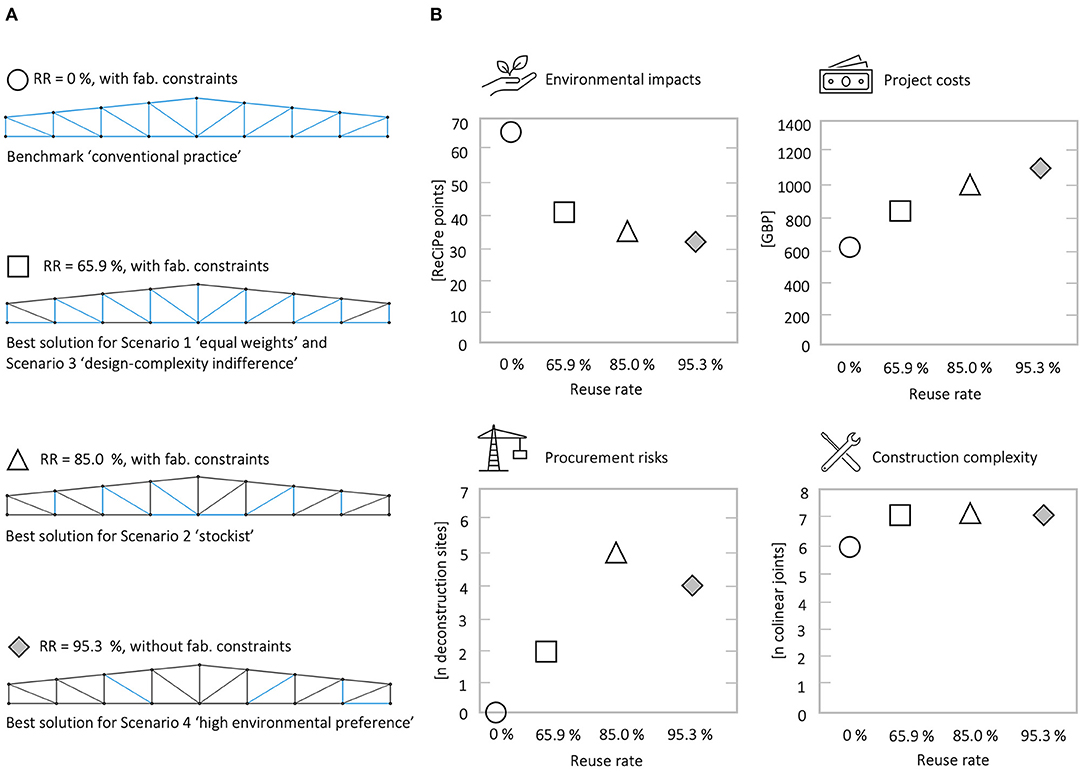
Figure 11. Comparison of the benchmark and the recommended alternatives. (A) Summary of the design options. Dark-gray bars correspond to reused components and blue bars to new components; (B) evaluation of the four options for each performance criterion.
The highest RR is recommended in Scenario 4, where preferences are modified to suit strong attention to environmental impacts. The recommended alternative in Scenario 4 has 45% lower environmental impacts in comparison to the benchmark alternative and is 54% more expensive. The second-highest RR is recommended for Scenario 2, where the existence of a specialized stockist levels the recommended RR at 85.0%. In both the equal-weight scenario and the design-complexity-indifference scenario, a RR of 65.9% is recommended, reducing by 35% the environmental impacts of the structural design in comparison with conventional practice. Despite being about a third less environmentally detrimental, this alternative is also 37% more expensive than the benchmark structure and involves two deconstruction sites as well as one more colinear joint. Although the cost data for both reuse and new components are subject to change over time, the price difference quantified in this study illustrates one of the key challenges for reuse to be widely adopted by the construction industry. In future, it may happen that new-material costs may rise or that taxes must be paid for demolition, recycling, or landfilling, thus favoring the reuse case. Through the proposed workflow it is possible to study such scenarios and to recommend the preferred solution even based on future projected market developments.
Apart from one scenario, all the recommended alternatives include fabrication constraints. This shows that fabrication constraints generally help to generate better design alternatives. Relatively high RRs (≥65%) are recommended for all 4 scenarios. This shows that reusing components in new structures helps obtaining better design solutions, irrespective of the preference scenario, i.e., irrespective of stakeholder's priorities.
Overall, the solution with fabrication constraints and a RR of 65.9% is well-ranked in all four preference scenarios (Figure 10). When compared according to individual criteria of project costs, procurement risks or construction complexity (Figure 8), it performs equally or better than the best options selected in all 4 preference scenarios. Despite being the cheapest option among the several recommended ones, this alternative is today still 37% more expensive than the benchmark scenario. When compared according to environmental impacts, it performs 22% worse than the best option corresponding to scenario 4, but still 35% better than the traditional construction with no reused components. This means that a RR of ~60–70% would represent a good consensus across all scenarios, and hence across stakeholders having different interests. Note that cheaper options would be recommended in case decision-makers attribute even more weight to the cost criterion.
The identification of this particular RR and the other two RRs corresponding to the best solutions for scenarios 2 and 4 is nearly impossible when looking only at single-criterion analyses (Figure 8), in which no trivial or unique solution exists. This conclusion highlights why the identification of preference weights and their integration in an MCDA is a promising approach to identify a decision-makers overall preference about rates of component reuse in new projects.
Comparison of MCDA Methods
In this section, the results obtained with PROMETHEE are compared with those of the other well-known outranking MCDA method: ELECTRE. This comparison aims to evaluate whether recommended alternatives are similar between the two methods.
ELECTRE stands for Elimination and Choice Expressing the Reality and is another outranking methodology (Roy, 1991) where recommended solutions are obtained using pairwise comparison. To account for the performance thresholds of Table 3, ELECTRE III (Figueira et al., 2013) has been used to recommend the best RR. Results have been computed using the J-ELECTRE v3.0 software (Pereira and de Oliveira Nepomuceno, 2021) where alternatives are ranked in a dominance matrix based on ascending and descending rankings.
Figure 12 presents the rank of alternatives obtained with ELECTRE, where alternatives ranked first are recommended. When compared with the results obtained with PROMETHEE (Figure 10), similar trends in the recommendation of design alternatives are observed, showing that both methods provide similar results in most scenarios. This confirms results obtained in other applications where PROMETHEE and ELECTRE have been compared, see for example (Kangas et al., 2001).
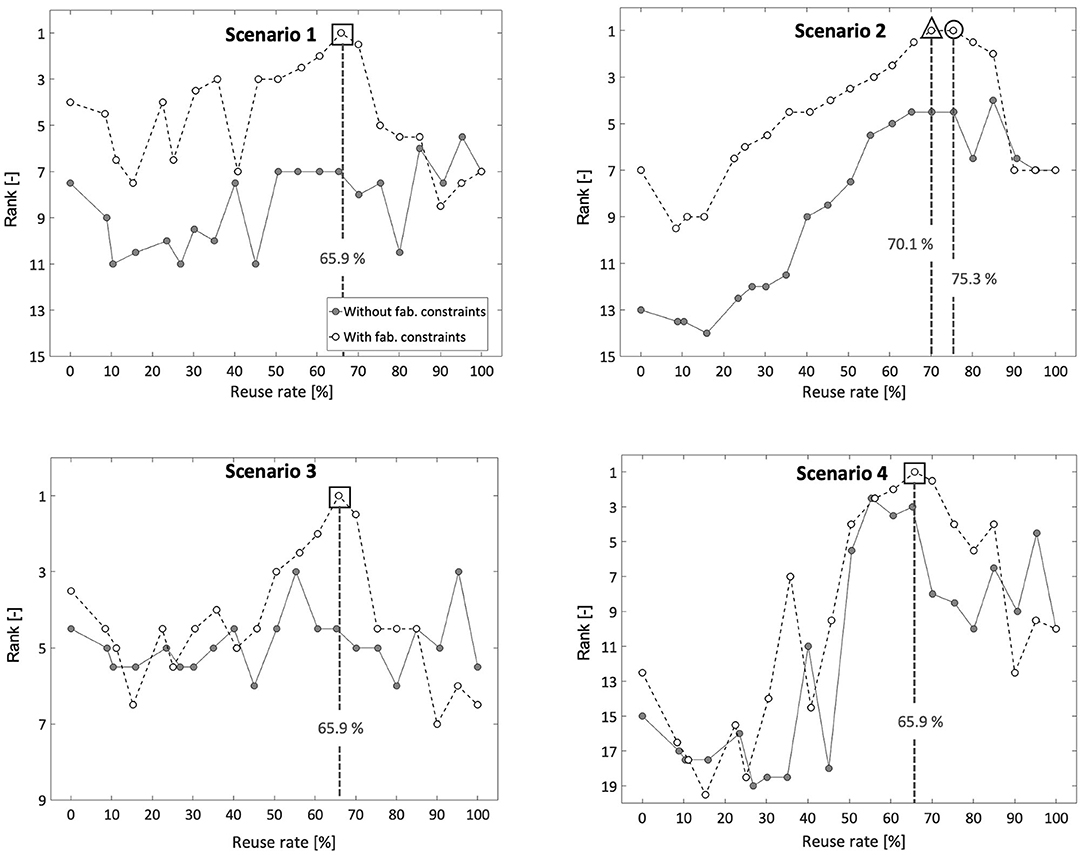
Figure 12. ELECTRE rank of design alternatives as a function of the reuse rate for the four scenarios.
For Scenarios 1 and 3, the same design alternative among the 42 possibilities is recommended by both MCDA methods (i.e., RR of 65.9% with fabrication constraints). For Scenario 2, two alternatives (RR of 70.1 and 75.3% with fabrication constraints) are equally recommended by ELECTRE while a RR of 85.0% with fabrication constraints is ranked first by PROMETHEE. However, PROMETHEE net-flow values ϕ of design alternatives with a RR between 70.1 and 85.0% and fabrication constraints are almost equal (Figure 10). That means that these alternatives are almost equally rated by PROMETHEE, and they include the alternatives recommended by ELECTRE.
Only in Scenario 4 do recommended alternatives significantly differ between the two MCDA methods. While a RR of 95.3% is recommended by PROMETHEE, ELECTRE suggests a RR of 65.9%. ELECTRE surprisingly recommends the same alternative as for Scenarios 1 and 3 although voting powers are distributed significantly differently (Figure 9). As scenario 4 is characterized by a large weight allocation to one criterion (i.e., 50% for environmental impacts) and small weight allocation to the other criteria, the comparison of results suggests that, in this application, the recommendation of design alternative by ELECTRE is less influenced by the asymmetrical weight allocation than PROMETHEE. In other words, in this case study, the poor performance for a criterion with a low weight seams less compensated by the good performance for a criterion with a large weight.
In summary, both methods provide similar results except when weight is distributed asymmetrically between performance criteria. The choice made in this study to use PROMETHEE is justified as this method is easier to use (Salminen et al., 1998; Polatidis et al., 2006). ELECTRE could be recommended in another reuse application if veto thresholds between performance criteria assessments are required.
Discussion
This section begins with a discussion of the proposed framework and its limitations. Then, future extension of the framework to other types of reuse problems is examined.
Discussion on the Method
This work provides a general workflow to solve a design problem while dealing with conflicting criteria. The proposed methodology combines MILP to generate alternatives with PROMETHEE as an MCDA approach to rank them. Regarding the analysis approach, the present study shows that decisions related to the reuse of components in construction depend not only on economic costs and environmental benefits but also on parameters specific to the reuse field, i.e., the procurement risks and the complexity of the construction. As the transfer of these parameters into costs is not trivial and would imply additional assumptions and complexity to the framework, an MCDA analysis seems preferable to a cost-benefit analysis.
With regard to the MILP process, it is possible to perform the optimization with other objective functions than weight minimization and to generate other alternatives. Weight minimization is usually directly related to reducing the costs and environmental impacts of a structural design (cf. Tables 1, 2). In addition, weight minimization may have other positive aspects not considered in the study, such as material efficiency and easier handling of parts. Thus, it is likely that the best alternative is one that is weight-optimized, but future research could explore and compare other optimization objectives.
The present framework does not include the identification nor the characterization of the reclaimed component stock. These steps are part of a preliminary study while this framework focuses on the project development stage. The environmental impacts of the preliminary study are expected to be negligible. Additionally, extra costs and complexity due to material identification and characterization are fixed values for all design alternatives generated from the same stock of reclaimed components. Consequently, impacts related to the preliminary study are excluded from the design alternative comparison.
The following limitations are also recognized. Data processing, rather than data collection, is the focus of the proposed framework. Due to the lack of data covering an identical system boundary (Figure 3) in terms of environmental impacts and cost, data from Brütting et al. (2020b) and Dunant et al. (2018) are combined in this work for the environmental and cost assessments. The work of Brütting et al. (2020b) uses data from existing databases and a literature survey based on a European context. Additionally, distances between deconstruction, storage and construction sites may influence the environmental-impact criterion in other case studies. Future work could assess and improve the data quality by comparing it to data measured with practitioner surveys and fieldworks. Besides, the presented framework uses the average value between the minimum and maximum total cost estimated by Dunant et al. (2018). Uncommon economic circumstances of a case study (e.g., testing of individual material not required, reuse of the existing connections, …) would drive the cost to the extremes of the price spectrum (Dunant et al., 2018). In general, the price ratio between new and reused components is influenced by several factors such as the price of new construction materials.
Finally, reuse rate recommendations could be further assessed through an in-depth sensitivity analysis of the criteria weights and difference and preferences thresholds. In this study, the preference scenarios illustrate potential mindsets of stakeholders. Future stakeholder survey would provide more detailed decision-makers profiles. The distinction in preference profiles could be made across stakeholder types: designers (architects or engineers), clients, builders (general contractors) and legislators. The scenarios would therefore be adjusted for these distinct profiles.
Discussion on the Framework Extension
The proposed framework has been developed for the reuse of linear steel components in reticular structures and applied to a Pratt truss case study. The framework application to other reuse problems (e.g., other materials, types of structure, building layers) is discussed in this section.
In this work, a MILP-based discrete structural optimization method has been employed for the design alternatives generation. The major benefit of MILP is that obtained solutions are global optima, which allows a rigorous comparison between design alternatives. In previous work, it has been shown that the employed optimization method is applicable to a wide range of structure typologies such as space trusses and frames and that it can be adapted to project-specific needs (Brütting et al., 2018, 2019, 2020a). It should be noted that solving MILPs to global optimality is at worst-case exponentially complex (Nemhauser and Wolsey, 1999), which might prevent the application of this technique to large-scale problems with many structural members and stock elements. In this case, alternative methods such as heuristics could be employed to generate locally optimal design alternatives.
Future work could extend the proposed MCDA workflow and its combination with structural optimization to structures made of other materials (e.g., timber, concrete) as well as to surface structures (e.g., slabs, walls). For problems that reuse products other than structural components, design-alternative generation tools need to be further investigated.
The system boundaries of the four performance criteria are expected to remain valid for other materials, types of structure and/or layers with minor adaptations. However, numerical values for the project costs and the environmental impacts of each process must be adapted to each reuse problem and project context. Data quality may vary depending on the type of reuse problem. Data availability may be another limitation that practitioner surveys and fieldworks may help overcome. Both tools can help collect, for example, economic data from practitioners and information on the processes influencing environmental impacts through field measurements (e.g., machine types and operation times). Regarding the construction-complexity performance criterion, its translation into a metric is strongly related to the design problem and the implications on the construction of reuse of reclaimed components over the use of new ones. In the presented case study, the construction complexity metric was a function of the number of colinear joints in the steel truss. The application of the framework to other types of reuse and structures would require this metric to be adapted to the corresponding project-specific implications on the construction.
Conclusions
Defining the optimal rate of reused components in a new construction project is a non-trivial task that depends on multiple performance-criterion evaluations as well as decision-maker preferences. The development and application of a multi-criteria decision analysis (MCDA) lead to the following conclusions:
• The inclusion of specific reuse criteria, such as procurement risks and construction complexity, in addition to economic costs and environmental impacts, enhances the quality of decision-making in design projects involving reclaimed components;
• The integration of decision-maker preferences and simulated design alternatives into an MCDA is shown particularly relevant when designing structures through reuse since there is no clear dominance among the set of compared reuse options. The applicability of the same method to other construction components or systems is yet to be validated.
• Solutions involving both reused and new components are often better than solutions composed exclusively of one type of component. In the present case study, a reuse rate of around 65% is often recommended and reduces the environmental impacts of the construction project by 35% compared to a solution that only involves new components.
Overall, the study confirms that a key decision when designing new circular building structures is not whether to reuse components or not, but at what rate should reused components be integrated into the new design.
Data Availability Statement
The raw data supporting the conclusions of this article will be made available by the authors, without undue reservation.
Author Contributions
CK and NB defined the research objectives and developed the methodology with the support of JB. JB computed the set of design alternatives through optimization. NB and CK computed the MCDA for the case-study. CK wrote the first draft of the manuscript, with NB, JB, and CF actively contributing to the final version. All authors approved the submitted version.
Funding
This research was funded by the Swiss National Science Foundation (SNSF) through the doc.CH program (grant number P0ELP1_192059).
Conflict of Interest
The authors declare that the research was conducted in the absence of any commercial or financial relationships that could be construed as a potential conflict of interest.
References
Addis, B. (2006). Building with Reclaimed Components and Materials: A Design Handbook for Reuse and Recycling. London: Earthscan.
Allacker, K., Mathieux, F., Pennington, D., and Pant, R. (2017). The search for an appropriate end-of-life formula for the purpose of the European Commission environmental footprint initiative. Int. J. Life Cycle Assess. 22, 1441–1458. doi: 10.1007/s11367-016-1244-0
Baker-Brown, D. (2017). The Re-Use Atlas: a Designer's Guide Towards a Circular Economy. London: RIBA Publishing.
Bertola, N. J., Cinelli, M., Casset, S., Corrente, S., and Smith, I. F. C. (2019). A multi-criteria decision framework to support measurement-system design for bridge load testing. Adv. Eng. Inform. 39, 186–202. doi: 10.1016/j.aei.2019.01.004
Bourguignon, D. (2018). Circular Economy Package: Four Legislative Proposals on Waste. European Parliamentary Research Service.
Brans, J.-P., and De Smet, Y. (2016). “PROMETHEE methods,” in Multiple Criteria Decision Analysis: State of the Art Surveys International Series in Operations Research and Management Science, eds S. Greco, M. Ehrgott, and J. R. Figueira (New York, NY: Springer), 187–219.
Brans, J. P., and Vincke, P. H. (1985). Note—a preference ranking organisation method. Manage Sci. 31, 647–656. doi: 10.1287/mnsc.31.6.647
Braungart, M., and McDonough, W. (2002). Cradle to Cradle: Remaking the Way We Make Things. New York, NY: North Point Press.
Brütting, J., Desruelle, J., Senatore, G., and Fivet, C. (2019). Design of truss structures through reuse. Structures 18, 128–137. doi: 10.1016/j.istruc.2018.11.006
Brütting, J., Senatore, G., and Fivet, C. (2018). “Optimization formulations for the design of low embodied energy structures made from reused elements,” in Advanced Computing Strategies for Engineering Lecture Notes in Computer Science, eds I. F. C. Smith, and B. Domer (Cham: Springer International Publishing), 139–163. doi: 10.1007/978-3-319-91635-4_8
Brütting, J., Senatore, G., Schevenels, M., and Fivet, C. (2020a). Optimum design of frame structures from a stock of reclaimed elements. Front. Built Environ. 6:57. doi: 10.3389/fbuil.2020.00057
Brütting, J., Vandervaeren, C., Senatore, G., De Temmerman, N., and Fivet, C. (2020b). Environmental impact minimization of reticular structures made of reused and new elements through life cycle assessment and mixed-integer linear programming. Energy Build. 215:109827. doi: 10.1016/j.enbuild.2020.109827
Charter, M. (2018). Designing for the Circular Economy. London: Routledge. doi: 10.4324/9781315113067
Choppin, J., Delon, N., and Encore, H. (2014). Matière Grise: Matériaux/Réemploi/Architecture. Paris: Pavillon de l'Arsenal.
Cinelli, M. (2017). The art of supporting decision-making. EIRJ 4, 298–308. doi: 10.31273/eirj.v4i2.166
Cinelli, M., Coles, S. R., and Kirwan, K. (2014). Analysis of the potentials of multi criteria decision analysis methods to conduct sustainability assessment. Ecol. Indic. 46, 138–148. doi: 10.1016/j.ecolind.2014.06.011
Cinelli, M., Kadziński, M., Gonzalez, M., and Słowiński, R. (2020). How to support the application of multiple criteria decision analysis? Let us start with a comprehensive taxonomy. Omega 96:102261. doi: 10.1016/j.omega.2020.102261
Cole, R. J., and Kernan, P. C. (1996). Life-cycle energy use in office buildings. Build Environ. 31, 307–317. doi: 10.1016/0360-1323(96)00017-0
Cooper, D. R., and Gutowski, T. G. (2017). The environmental impacts of reuse: a review. J. Ind. Ecol. 21, 38–56. doi: 10.1111/jiec.12388
Corrente, S., Figueira, J. R., and Greco, S. (2014). The SMAA-PROMETHEE method. Eur. J. Oper. Res. 239, 514–522. doi: 10.1016/j.ejor.2014.05.026
De Wolf, C., Hoxha, E., and Fivet, C. (2020a). Comparison of environmental assessment methods when reusing building components: a case study. Sustain. Cities Soc. 61:102322. doi: 10.1016/j.scs.2020.102322
De Wolf, C., Hoxha, E., Hollberg, A., Fivet, C., and Ochsendorf, J. (2020b). Database of embodied quantity outputs: lowering material impacts through engineering. J. Archit. Eng. 26:04020016. doi: 10.1061/(ASCE)AE.1943-5568.0000408
Densley Tingley, D., Cooper, S., and Cullen, J. (2017). Understanding and overcoming the barriers to structural steel reuse, a UK perspective. J. Clean. Prod. 148, 642–652. doi: 10.1016/j.jclepro.2017.02.006
Dunant, C., Drewniok, M., Sansom, M., Corbey, S., Allwood, J., and Cullen, J. (2017). Real and perceived barriers to steel reuse across the UK construction value chain. Resour. Conserv. Recycl. 126, 118–131. doi: 10.1016/j.resconrec.2017.07.036
Dunant, C., Drewniok, M. P., Sansom, M., Corbey, S., Cullen, J. M., and Allwood, J. M. (2018). Options to make steel reuse profitable: an analysis of cost and risk distribution across the UK construction value chain. J. Clean. Prod. 183, 102–111. doi: 10.1016/j.jclepro.2018.02.141
Dyer, J. S. (2005). “Maut—multiattribute utility theory,” in Multiple Criteria Decision Analysis: State of the Art Surveys International Series in Operations Research and Management Science, eds J. Figueira, S. Greco, and M. Ehrogott (New York, NY: Springer), 265–292. doi: 10.1007/0-387-23081-5_7
Ekvall, T., and Tillman, A.-M. (1997). Open-loop recycling: criteria for allocation procedures. Int. J. LCA 2:155. doi: 10.1007/BF02978810
European Commission (2019). Energy Performance of Buildings. Available online at: https://ec.europa.eu/energy/en/topics/energy-efficiency/energy-performance-of-buildings/overview (accessed September 6, 2019).
European Committee for Standardization (2011). EN 15978:2011 Sustainability of Construction Works - Assessment of Environmental Performance of Buildings - Calculation Method. European Committee for Standardization.
European Committee for Standardization (2019). EN 10210-2 Hot Finished Steel Structural Hollow Sections. Part 2: Tolerances, Dimensions and Sectional Properties. European Committee for Standardization.
Eurostat (2020). Waste Statistics - Statistics Explained. Available online at: https://ec.europa.eu/eurostat/statistics-explained/index.php/Waste_statistics#Total_waste_generation (Accessed March 1, 2021).
Ferretti, V., and Comino, E. (2015). An integrated framework to assess complex cultural and natural heritage systems with multi-attribute value theory. J. Cult. Herit. 16, 688–697. doi: 10.1016/j.culher.2015.01.007
Figueira, J. R., Greco, S., Roy, B., and Słowiński, R. (2013). An overview of ELECTRE methods and their recent extensions. JMCDA 20, 61–85. doi: 10.1002/mcda.1482
Ghyoot, M., Devlieger, L., Billiet, L., and Warnier, A. (2018). Déconstruction et Réemploi: Comment Faire Circuler les Éléments de Construction. Lausanne: Presses Polytechniques et Universitaires Romandes.
Goedkoop, M., Heijungs, R., and Huijbregts, M. (2013). ReCiPe 2008 a Life Cycle Impact Assessment Method Which Comprises Harmonised Category Indicators at the Midpoint and the Endpoint Level - First Edition (version 1.08) - Report I: Characterisation. The Hague: Ministerie van VROM. Available online at: https://scholar.google.com/scholar_lookup?title=ReCiPe%202008%20a%20life%20cycle%20impact%20assessment%20method%20which%20comprises%20harmonised%20category%20indicators%20at%20the%20midpoint%20and%20the%20endpoint%20level&author=M.%20Goedkoop&publication_year=May%202013
Gordon, M. (1997). Demolition Energy Analysis of Office Buildings Structural Systems. Ottawa, ON: ATHENA Sustainable Materials Institute.
Gorgolewski, M. (2008). Designing with reused building components: some challenges. Build. Res. Inf. 36, 175–188. doi: 10.1080/09613210701559499
Gorgolewski, M. (2011). The implications of reuse and recycling for the design of steel buildings. Can. J. Civ. Eng. 33, 489–496. doi: 10.1139/l06-006
Gorgolewski, M. (2017). Resource Salvation: The Architecture of Reuse. Hoboken: Wiley-Blackwell. doi: 10.1002/9781118928806
Gorgolewski, M., and Morettin, L. (2009). “The process of designing with reused building components,” in Lifecycle Design of Buildings, Systems and Materials, ed E. Durmisevic (Enschede: International Council for Building Research Studies and Documentation (CIB), Working Commission W115 and the University of Twente, The Netherlands), p. 105.
Greco, S., Figueira, J., and Ehrgott, M. (2016). Multiple Criteria Decision Analysis. New York, NY: Springer. doi: 10.1007/978-1-4939-3094-4
Gurobi Optimization LCC (2019). Gurobi Optimizer Reference Manual. Available online at: http://www.gurobi.com (accessed May 5, 2021).
Häkkinen, T., Kuittinen, M., Ruuska, A., and Jung, N. (2015). Reducing embodied carbon during the design process of buildings. J. Build. Eng. 4, 1–13. doi: 10.1016/j.jobe.2015.06.005
Hoxha, E., Habert, G., Lasvaux, S., Chevalier, J., and Le Roy, R. (2017). Influence of construction material uncertainties on residential building LCA reliability. J. Clean. Prod. 144, 33–47. doi: 10.1016/j.jclepro.2016.12.068
Huijbregts, M. A. J., Steinmann, Z. J. N., Elshout, P. M. F., Stam, G., Verones, F., Vieira, M., et al. (2017). ReCiPe2016: a harmonised life cycle impact assessment method at midpoint and endpoint level. Int. J. Life Cycle Assess. 22, 138–147. doi: 10.1007/s11367-016-1246-y
IEA (2019). Material Efficiency in Clean Energy Transitions. Paris: IEA. Available online at: www.iea.org/publications/reports/MaterialEfficiencyinCleanEnergyTransitions/ (accessed August 20, 2019).
International Organisation for Standardisation (2006). ISO 14040-0 Environmental Management - Life Cycle Assessment - Principles and Framework. International Organisation for Standardisation.
Islam, H., Jollands, M., and Setunge, S. (2015). Life cycle assessment and life cycle cost implication of residential buildings—a review. Renew. Sustain. Energy Rev. 42, 129–140. doi: 10.1016/j.rser.2014.10.006
Jato-Espino, D., Castillo-Lopez, E., Rodriguez-Hernandez, J., and Canteras-Jordana, J. C. (2014). A review of application of multi-criteria decision making methods in construction. Autom. Constr. 45, 151–162. doi: 10.1016/j.autcon.2014.05.013
Kaethner, S. C., and Burridge, J. A. (2012). Embodied CO2 of structural frames. Struct. Eng. 90, 33–40. Available online at: https://www.istructe.org/journal/volumes/volume-90-(2012)/issue-5/embodied-co2-of-structural-frames/
Kangas, A., Kangas, J., and Pykäläinen, J. (2001). Outranking methods as tools in strategic natural resources planning. Silva Fenn. 35, 215–227. doi: 10.14214/sf.597
Kim, S., and Kim, S.-A. (2020). Framework for designing sustainable structures through steel beam reuse. Sustainability 12:9494. doi: 10.3390/su12229494
Kirchherr, J., Reike, D., and Hekkert, M. (2017). Conceptualizing the circular economy: an analysis of 114 definitions. Resour. Conserv. Recycl. 127, 221–232. doi: 10.1016/j.resconrec.2017.09.005
Lagaros, N. D. (2018). The environmental and economic impact of structural optimization. Struct. Multidisc. Optim. 58, 1751–1768. doi: 10.1007/s00158-018-1998-z
Mareschal, B. (2021). PROMETHEE and GAIA Software. Available online at: http://www.promethee-gaia.net/software.html (accessed March 9, 2021).
Millman, L. R., and Giancaspro, J. W. (2012). Environmental evaluation of abrasive blasting with sand, water, and dry ice. IJAEC 1, 174–182. doi: 10.7492/IJAEC.2012.019
Nemhauser, G. L., and Wolsey, L. A. (1999). Integer and Combinatorial Optimization. New York, NY: John Wiley and Sons.
Ohsaki, M. (2016). Optimization of Finite Dimensional Structures. Boca Raton, FL: CRC Press. doi: 10.1201/EBK1439820032
Pereira, V., and de Oliveira Nepomuceno, L. D. (2021). J-Electre v3.0. Available online at: https://sourceforge.net/projects/j-electre/files/ (accessed June 1, 2021).
Polatidis, H., Haralambopoulos, D. A., Munda, G., and Vreeker, R. (2006). Selecting an appropriate multi-criteria decision analysis technique for renewable energy planning. Energy Sources B Econ. Plann. Policy 1, 181–193. doi: 10.1080/009083190881607
Pongiglione, M., and Calderini, C. (2014). Material savings through structural steel reuse: a case study in Genoa. Resour. Conserv. Recycl. 86, 87–92. doi: 10.1016/j.resconrec.2014.02.011
Rajagopalan, N., Brancart, S., De Regel, S., Paduart, A., Temmerman, N. D., and Debacker, W. (2021). Multi-criteria decision analysis using life cycle assessment and life cycle costing in circular building design: a case study for wall partitioning systems in the circular retrofit lab. Sustainability 13:5124. doi: 10.3390/su13095124
Rakhshan, K., Morel, J.-C., Alaka, H., and Charef, R. (2020). Components reuse in the building sector – a systematic review. Waste Manag. Res. 38, 347–370. doi: 10.1177/0734242X20910463
Raphael, B. (2011). Multi-criteria decision making for collaborative design optimization of buildings. Built Environ. Proj. Asset Manag. 1, 122–136. doi: 10.1108/20441241111180398
Röck, M., Saade, M. R. M., Balouktsi, M., Rasmussen, F. N., Birgisdottir, H., Frischknecht, R., et al. (2020). Embodied GHG emissions of buildings – the hidden challenge for effective climate change mitigation. Appl. Energy 258:114107. doi: 10.1016/j.apenergy.2019.114107
Roy, B. (1991). The outranking approach and the foundations of electre methods. Theor. Decis. 31, 49–73. doi: 10.1007/BF00134132
Salminen, P., Hokkanen, J., and Lahdelma, R. (1998). Comparing multicriteria methods in the context of environmental problems. Eur. J. Oper. Res. 104, 485–496. doi: 10.1016/S0377-2217(96)00370-0
Schrijvers, D. L., Loubet, P., and Sonnemann, G. (2016). Developing a systematic framework for consistent allocation in LCA. Int. J. Life Cycle Assess. 21, 976–993. doi: 10.1007/s11367-016-1063-3
Silva, S., and Almeida, M. (2013). “Using MCDA to select refurbishment solutions to improve buildings IEQ,” in Portugal SB13 - Contribution of Sustainable Building to Meet EU 20-20-20 Targets (Guimarães).
Thinkstep (2021). GaBi Databases. Available online at: http://www.gabi-software.com/switzerland/databases/gabi-databases/ (accessed March 30, 2021).
United Nations (2020). The Sustainable Development Goals Report 2020. Available online at: https://unstats.un.org/sdgs/report/2020/The-Sustainable-Development-Goals-Report-2020.pdf (accessed May 5, 2021).
Wang, H.-J., and Zeng, Z.-T. (2010). A multi-objective decision-making process for reuse selection of historic buildings. Expert Syst. Appl. 37, 1241–1249. doi: 10.1016/j.eswa.2009.06.034
Wernet, G., Bauer, C., Steubing, B., Reinhard, J., Moreno-Ruiz, E., and Weidema, B. (2016). The ecoinvent database version 3 (part I): overview and methodology. Int. J. Life Cycle. Assess. 21, 1218–1230. doi: 10.1007/s11367-016-1087-8
World Economic Forum (2016). Shaping the Future of Construction: A Breakthrough in Mindset and Technology. Cologny: World Economic Forum.
Keywords: component reuse, multi-criteria decision analysis, structural design, circular economy, construction project, building materials, PROMETHEE, life cycle assessment
Citation: Küpfer C, Bertola N, Brütting J and Fivet C (2021) Decision Framework to Balance Environmental, Technical, Logistical, and Economic Criteria When Designing Structures With Reused Components. Front. Sustain. 2:689877. doi: 10.3389/frsus.2021.689877
Received: 01 April 2021; Accepted: 16 June 2021;
Published: 15 July 2021.
Edited by:
Huijuan Xiao, Hong Kong Polytechnic University, Hong KongCopyright © 2021 Küpfer, Bertola, Brütting and Fivet. This is an open-access article distributed under the terms of the Creative Commons Attribution License (CC BY). The use, distribution or reproduction in other forums is permitted, provided the original author(s) and the copyright owner(s) are credited and that the original publication in this journal is cited, in accordance with accepted academic practice. No use, distribution or reproduction is permitted which does not comply with these terms.
*Correspondence: Célia Küpfer, celia.kupfer@epfl.ch