- School of Resource and Environmental Management, Simon Fraser University, Burnaby, BC, Canada
Food loss is a systemic problem contributing to negative environmental, social, and economic impacts. However, despite food loss quantification being identified as critical for food loss prevention and reduction, there is a dearth of studies exploring opportunities to digitize or simplify food loss measurement. Moreover, post-harvest food loss estimates can be difficult to obtain as farmers grow different types of crops and have diverse technical skills as well as resources. Digital agriculture technologies such as farm management apps that can help farmers accurately record their yield and sales may provide a useful method for quantifying food loss. Accurate food loss quantification may also help provide better baseline measurement for policymakers. To assess the potential role of digital agricultural tools for food loss quantification, this exploratory study recruited seven farmers in southwest British Columbia to test an open access farm management app called LiteFarm for 2 months and digitally recorded their harvest logs. Drawing upon semi-structured key informant interviews, this study found that time scarcity and crop diversity were barriers to using the app. An unexpected benefit to the app is that it can better inform land use decisions when utilized for pre-harvest planning and therefore may help with loss prevention. Findings from this study highlight farmers' struggles to focus on sustainability and reducing food loss, especially when balancing their economic interests. Inclusive digital technologies and deeper engagement with farmers are needed to develop food loss quantification methods that fit diverse farming contexts.
Introduction
Supply chain issues, economic hardships, the COVID-19 pandemic, and disruptive climate events have shocked our global food system and demonstrates its precarity and systemic injustices (Wheeler and Von Braun, 2013; Béné, 2020; Clapp and Moseley, 2020). While the pandemic has resulted in increasing food insecurity (Devereux et al., 2020), farmers globally were faced with disruptions that resulted in massive wastage (Aldaco et al., 2020; FAO, 2020; Fei et al., 2020). For example, in the midst of the pandemic, the dairy sector saw 3.7 million gallons of milk (5% of the USA's milk supply) dumped each day and roughly 750,000 unhatched eggs smashed every week (Yaffe-Bellany and Corkery, 2020). With the loss of restaurant, hotel and school food demands, farmers have lost half their customer base, resulting in food being tilled under due to canceled orders (Hobbs, 2020; Yaffe-Bellany and Corkery, 2020). In Canada, avoidable food loss amounts to 6.1 million tons, excluding food waste from the retail and consumer sectors (Nikkel et al., 2019). This equates to roughly 17 percent of Canada's total food loss and waste (FLW), and is valued at 26.3 billion dollars annually (Nikkel et al., 2019).
While definitions may differ, “food loss” generally describes food that is wasted throughout the production, post-harvest, and processing stages, up to but not including the retail level, meanwhile, “food waste” is food that is wasted from the retail stage to the consumer level (FAO, 2019). Many scholars have identified inconsistencies and potential confusion with FLW definitions (Chaboud and Daviron, 2017). However, for the purpose of this study, we will only focus on food loss occurring at the farm (post-harvest) that was intended for human consumption, and not food that is wasted at the processing stage, or food that has left the farm but is then rejected by the retailer. Measuring food loss and providing standardized estimations across the globe remains a glaring gap in food systems research. Roe (2020) argues that there are still numerous challenges around food loss measurement methodology, with few quantitative studies measuring food loss on farms, in comparison to numerous studies quantifying consumer or household food waste. The studies that do attempt to measure food loss (Delgado et al., 2017; Sheahan and Barrett, 2017; Johnson et al., 2018) utilize varying methods, thus limiting the ability for data comparison. Divergent methods for measuring food loss have produced conflicting results and scholars have called for more standardization in food loss quantification methodology. Furthermore, even when farmers are given the opportunity to measure food loss, encouraging participation is difficult (Hartikainen et al., 2018). For example, Hartikainen et al.'s study (Hartikainen et al., 2018) did not report their quantitative food loss data as the sample sizes were too low to be conclusive. The potential causes for minimal food loss data reported in the study included lack of time for collection and extenuating circumstances such as weather events or shifts in retail demand (Hartikainen et al., 2018). Addressing inconsistent food loss measurement methodologies can provide the foundation for developing evidence-based interventions to increase economic, environmental, and social benefits to farmers and subsequent stakeholders in the food supply chain.
There are numerous causes for food loss. These includes human error, farm equipment malfunction and weather/climate-related factors (Johnson et al., 2018), as well as demand side reasons including food aesthetics, mismatched prices, and retail/consumer choice (Soma et al., 2021). Food loss remains a pressing issue globally although past studies have disproportionately framed this issue as predominant to the global South. However, FLW in Canada alone totals roughly 35.5 million metric tons of every year which equates to 56.5 million megatons of CO2 and roughly 49.5 billion dollar's worth of food wasted annually (Nikkel et al., 2019). Canada's FLW management policy lags behind many European Union nations such as Italy and France which have comprehensive reuse and recovery laws compared to Canada's sporadic landfill regulations and municipal bans (Giordano et al., 2020).
One potential solution proposed to facilitate the ease of quantifying food loss is digital agriculture. In the realm of FLW, Benyam et al. (2021) explores the potential applications of Digital Agriculture Technology (DAT) on FLW prevention and reduction. DAT tools include GPS simulations for optimal distribution routes, digital marketing campaigns that improve consumer awareness, nanotechnology that supports precision agriculture and more. Therefore, digital agriculture can potentially support novel techniques for FLW prevention and reduction. DAT has been touted by proponents as potentially positioning the Canadian agricultural industry as a global leader in safe, nutritious and sustainable food in the 21st century (Advisory Council on Economic Growth, 2017). DAT includes technology that can be used to track farm data efficiently (Klerkx and Rose, 2020). However, FLW data tracking and data management remains understudied in this digital revolution. Investing in technology that can accurately and efficiently track food waste may result in further advancement in DAT. However, as noted by critical social science research on this matter, it is important to ensure that concerns around the potential implication of DAT use and who has control of the data should also be addressed (Bronson and Knezevic, 2016).
Recognizing the growing investments by governments and financial institutions in digital or “smart” technologies, this study seeks to understand the perception, adoption, and engagement, of DATs by farmers in British Columbia (B.C) for the purpose of better recording of yield and sales data and therefore potentially better food loss quantification. This study analyzes farmers' use and their feedback of an open-source farm management app called LiteFarm (see litefarm.org). LiteFarm is a free, open source sustainable farm management software and designed by researchers at the University of British Columbia. The app was developed to measure the environmental impact (water use, energy use, inputs) of diverse types of farm management practices. LiteFarm can also be used to measure food loss by facilitating manual data entry in a harvest log feature. In this study, we train farmers to measure their post-harvest food loss and farm yield with this app. The purpose of this study is to better understand if digital technologies such as apps can help facilitate ease in the measurement of post-harvest waste. This is an exploratory study of (n = 7) farmers in two differing communities (conventional and organic) in southwestern British Columbia and their use of the LiteFarm app for a period of 2 months. The following are the primary research questions and sub questions for this study:
1. What is the potential role of digital agriculture tools (in this study: the use of LiteFarm app and software) for facilitating food loss measurement on farms?
2. What barriers and opportunities exist within the adoption of digital agriculture technologies that could better support food loss quantification, prevention, and reduction at the farm-level?
This study will contribute to the discussion around the growing use of DAT and its current role in our global food systems. In learning more from the farmers about their experience with using app-based DAT to measure food loss, this study can contribute to the literature addressing the potential social, environmental, and economic implications of DAT. There is currently a gap in the literature on empirical studies demonstrating the impact of DAT on FLW prevention and reduction, with most studies focusing only on estimated potential (Shepherd et al., 2020; Benyam et al., 2021). The following section (literature review) will briefly highlight the drivers of food loss at the farm level, challenges with quantification, and highlight the potential role of DAT. Following the literature review, the paper will identify the methodology applied to test the app with farmers and introduce new findings that may provide insights for academics, policymakers, and technology developers on the potential use of DAT in food loss quantification.
Literature review
Drivers of food loss at the farm level
Definitions for “food loss” are generally associated with pre-harvest, post-harvest, and processing stages in the FSC (Lipinski et al., 2013; Chaboud and Daviron, 2017), as well as harvest, slaughter, catch, and unintentional equipment or infrastructure issues impacting edible food meant for human consumption (FAO, 2019; Feedback, 2021). Producers may be motivated to reduce their losses, yet there may be conditions and drivers that are out of a producer's control such as quality loss during distribution, environmental circumstances, or aesthetic standards at retail outlets, that can overburden the producers and result in ineffective interventions (Chaboud and Daviron, 2017; Soma et al., 2021).
Food loss at a farm level is also driven by misguided subsidies, market power imbalances, and a precarious labor system that relies on migrant workers (Soma et al., 2021). There is also power imbalance within the consumer and retail food market (Feedback, 2021). Canada has only five grocery stores commanding nearly 80% of the food retail market (Soma et al., 2021) and within those retail markets, consumer choice dominates market value, highlighting strict aesthetic standards and social food norms in North America (Janousek et al., 2018). Food loss at the farm level is also associated with the instability in agricultural labor forces. Given the labor instability and market volatility, some farmers have acknowledged that they overproduce in an attempt to minimize their financial risks (Gunders and Bloom, 2017). However, other food loss studies refute this point as many farmers also rely on historical observations and tested experience to minimize financial hardship (Johnson et al., 2019).
Until recently, there has been a perception that developing countries have issues more commonly associated with food loss such as lack of infrastructure, while developed countries are associated with consumer food waste issues (Parfitt et al., 2010; Gustavsson et al., 2011). Only recently did the United Nations Environment Programme (2021) acknowledge the problem of putting the emphasis of food loss studies in developing countries. Not only does this overlook similar food loss problems occurring in developed nations, but it also results in solutions that are not aligned with farmer's true needs (Soma et al., 2021). Food loss reduction and prevention solutions should consider the complexities and the root causes of the losses to avoid exacerbating problems. Food loss management has two distinct paths, reduction, or prevention. There are significant challenges for food loss prevention and reduction including structural issues such as unfair trading practices (Piras et al., 2018). Unfair trading practices result from a power imbalance in a food supply chain between major retailers and producers which results in costs and risks shifted unfairly to one party (Piras et al., 2018). Examples include canceling orders without advanced notice for producers, and therefore putting farmers under significant financial pressure (Piras et al., 2018). Messner et al. (2020) draws attention to the plethora of studies looking at food loss reduction measures (Lipinski et al., 2013; Shafiee-Jood and Cai, 2016; Verma et al., 2019) that neglect the potential for a proactive approach to tackle the root causes of food loss (i.e., prevention).
Food loss quantification and challenges
Food loss has been calculated with inconsistent measurements for years (Chaboud and Daviron, 2017). Estimates made by the FAO (2019) place global food loss at roughly 14% before reaching the retail stages. Fruits/vegetables and tubers/root vegetables see the highest food loss numbers with the global average being roughly 22% of fruits and vegetables lost per year and 25% of tubers and root vegetables lost per year (FAO, 2019). It has been documented that current food loss statistics suffer from highly extrapolated data and outdated average values to generalize regional food loss situations (Johnson et al., 2018). Gustavsson et al. (2011) reports a global average food loss of 20%, however, Johnson et al. (2018) reported estimated food losses in North Carolina that are much larger. Johnson et al. (2018) acknowledges that their estimates include both marketable and edible food which would require additional measures including consumer acceptance and food supply chain capacity. Food loss metrics from Johnson et al. (2018) study include a 12% marketable yield loss for one of the squash fields, a 143% loss for one of the cucumber fields and an average marketable yield loss of 57% for all fruit and vegetable fields in the study. Neff et al. (2018) studied food loss on farms in Vermont and found that 16% of food loss was considered salvageable and is comparable to Gustavsson et al. (2011). However, Neff et al. (2018) combined unharvested metrics which follow Johnson et al. (2018) method, found 30% loss on the field as their average marketable food loss metric. Food loss studies can report results under many different labels, including terms such as marketable, saleable, and edible which incorporate similar characteristics, but are not always interchangeable. Therefore, clear food loss definitions are important when interpreting results.
Quantifying food loss remains a complex challenge that involves time intensive measuring techniques with little incentive for busy farmers. Kitinoja et al. (2018) explored post-harvest loss assessments for plant-based crops and found the range of losses between different crop types was substantial. For example, loss of legumes and grains range from 0–40 percent while other non-perishable food losses range from 0–80 percent in some cases. This discrepancy is due to the lack of quantitative food loss data collected from farm sites as studies more frequently collect qualitative data to estimate food loss (Kitinoja et al., 2018). Lack of clarity around the definition of food loss (Chaboud and Daviron, 2017), data gaps for FLW in specific regions (Gustavsson et al., 2011) and a lack of standardization of methodologies across studies (Kitinoja et al., 2018) were identified as key challenges for collecting accurate food loss quantification data.
A standard for food loss quantification is a four-step process outlined by the FAO in a 2016 methodology document. The steps include screening, survey, sampling, and synthesis to collect both qualitative and quantitative food loss data at the farm level (FAO, 2016). The concerns with this method arise within the biases for sampling and surveying; since the document instructs researchers to use “highly knowledgeable actors in the FSC to create their specific method for surveying and sampling” (FAO, 2016, pg. 4). The FAO's methodology provides a comprehensive framework for identifying specific food loss events; however, it would be difficult to extrapolate with a broad scale approach. While a roadmap document such as what was published by the FAO (2016) is important for starting the process of standardizing food loss quantification, it does not address the inconsistencies outlined by food loss literature. These inconsistencies include the lack of clarity in the definition of food loss (Chaboud and Daviron, 2017), issues with defining specific areas on the farm that will be subject to measurement (Chaboud and Daviron, 2017), and communication among several FSC actors that will be involved in a given food loss measurement (Gustavsson et al., 2011). A study by Johnson et al. (2018) measured food loss at nine farms in North Carolina and presented their results in a kilogram per hectare measure of food loss (Johnson et al., 2018). Their study utilized a physical sampling method and looked at quantity, quality, and condition to categorize food into marketable, edible, or inedible standards (Johnson et al., 2018). The results displayed an average of 5,114 kilograms per hectare of food was available after the primary harvest, equating to an average harvest loss of 57 percent (Johnson et al., 2018). It was acknowledged that these results are high and given the current information gap regarding food loss measurement there is a need for reevaluation (Johnson et al., 2018). Johnson et al. (2018) concluded that the information gap is not from the lack of ability by food researchers to measure losses, but rather a lack of human capital and financial resources to undertake accurate quantitative food loss measurements. The food loss measurement methods utilized by Johnson et al. (2018) focused on mass recovery and detailed post-harvest sorting which is time intensive. Other scholars suggest alternative methods with pre-harvest organization or on field estimates to lower time commitments (Kitinoja et al., 2018).
To address the complexity and resource intensive nature of food loss quantification, Roe (2020) suggests modeling as a solution to addressing the gap in food loss data. Modeling provides a method that can be applied with significantly less human capital than a traditional physical data collection study and allows for creative flexibility when it comes to including alternative variables such as quality and end use value (Delgado et al., 2017). Roe (2020) envisions food loss modeling as a framework toward filling the literature gap given its lower time commitment and replicability. Furthermore, an additional benefit from modeling is testing specific food loss quantification in different sectors of the FSC. The end goal with modeling is to find accurate food loss quantification methods that can be replicated in physical data studies (Roe, 2020).
Role of digital agriculture technology
Digital Agriculture Technology (DAT) has the potential to significantly influence methods for food loss quantification. However, its contribution to the field of FLW studies is currently understudied, and the potential benefits of DAT for food loss prevention, reduction, and quantification lack empirical evidence. DAT can be defined as any agricultural technology that assists in collecting, storing, analyzing, or sharing data, and can include sensors, data storage, telecommunications, and analytics (Klerkx and Rose, 2020; Shepherd et al., 2020). Measuring food loss quantification can benefit from several common DATs including food traceability technology (Bosona and Gebresenbet, 2013; Astill et al., 2019), predictive planning technology (Sarker et al., 2020) and precise input applications (Finger et al., 2019). Two recent studies that have addressed the connection between DAT and food loss are Benyam et al. (2021) and Ciccullo et al. (2021). Ciccullo et al. (2021) quantitatively estimates the effectiveness of DAT in the agri-food sector and partnered technology sectors. Benyam et al. (2021) explores the current literature related to food loss and waste quantification and DAT, drawing connections between current technologies and their potential application to prevent or reduce food loss globally.
Ciccullo et al. (2021) studies FLW prevention in the context of a circular economy and is the only study that has recently explored the use of DAT specifically for FLW prevention. This paper looked at technology providers and agri-food supply chain actors to assess the available technology and objectives for preventing FLW. The study found that there are many types of technologies that achieve different results in the food supply chain. These technologies can be broken down into two categories: 1) off the shelf or 2) customizable. Ciccullo et al. (2021) emphasizes that technologies work better for FLW prevention if they are customized and suggests a stronger and more collaborative relationship between technology developers and agri-food actors. Benyam et al. (2021) conducted a literature review summarizing several important considerations for future research around DAT and food loss quantification. The authors caution that the spillover effects, both positive and negative must be considered as farmers choosing to adopt DATs will not necessarily do so with the primary purpose of food loss prevention or reduction (Benyam et al., 2021). Thus, while food loss prevention or reduction may be beneficial, many farmers will not choose to adopt the technologies if it does not directly contribute to their economic output (Benyam et al., 2021). It is also important to note that adopting DAT for food loss prevention or reduction may provide potential benefits but will also include associated costs including upgraded infrastructure (Finger et al., 2019) and training for the labor force (Rotz et al., 2019), which may create initial barriers to DAT adoption (Klerkx and Rose, 2020; Benyam et al., 2021). Other barriers for DAT adoption are related to poor infrastructure (Finger et al., 2019), and technology gaps for older traditional farmers among others. However, certain barriers could be avoided by switching the emphasis to pre-harvest planning and taking a proactive approach (Chaboud and Daviron, 2017). Many of the benefits seen from DAT related to food loss quantification have been identified as potentially occurring in the pre-harvest stages (Ciccullo et al., 2021).
However, the use of DAT is still contentious among experts, with concerns around digitization itself and who controls/owns the data (Carolan, 2017). Farmers may see benefits from DAT but are unwilling to surrender any decision-making power or have their private operations monitored by large influential corporations (Carolan, 2017). Magruder (2018) and Shepherd et al. (2020) both agree that filling the information gap and providing producers with adequate training will help adoption.
Methods and material
Research context
This study is based in Metro Vancouver, a regional district located in southwestern British Columbia (BC), Canada. Metro Vancouver is on the unceded traditional territories of the Coast Salish People including the Squamish, Musqueam, Tsleil-Waututh, Stó:lo, Semiahmoo, Tsawwassen, Kwikwetlem, Kwantlen, Matsqui, Qayqayt, Hwlitsum, and Katzie nations. According to a 2016 Canada Census, Metro Vancouver has a population of 2.57 million people (Metro Vancouver, 2011). Metro Vancouver was chosen as the study location for its food production diversity, commitment to local food system resiliency and strong stance on supporting DAT (BC Food Security Task Force, 2019). This study focusses on fruit and vegetable crops which make up a small amount of growing opportunity in southwestern BC. There is roughly 2.8% of Canada's field vegetable crops produced in BC, however, 68% of those field vegetables are grown in southwestern BC, as well as almost 55% of fruits and berries (BC Ministry of Agriculture, Food and Fisheries, 2016). Given the relatively small land base in southwestern BC and disproportionate population density, it becomes increasingly important to study how food production can continue under this increased pressure. To avoid continued reliance on a globalized, imported food supply, the provincial government has put in place measures to support local producers with grocery store marketing programs such as Buy BC and land protections such as the Agricultural Land Reserve (BC Ministry of Agriculture, Food and Fisheries, 2022).
This study utilizes an app called LiteFarm (Wittman et al., 2020; Wohlers et al., 2021) which was developed at the University of British Columbia (UBC). LiteFarm (see https://www.litefarm.org) was developed to support farmers in their pursuit of environmentally friendly practices, however, the app is also a farm management tool and is useful for record keeping purposes. LiteFarm is not the only app-based farm management, COG Pro is an organic certified record keeping app that was developed in Oregon and allows for organized notes designed for smaller scale organic farmers. Similarly, Oregon State University has recently developed an app for Community Supported Agriculture (CSA) which connects consumers directly to farmers on a digital interface. CSA programs are popular in southwestern BC and this app has the potential to bridge communication gaps currently found in the local food system. App-based management may provide small scale farmers with a competitive advantage.
Methodology
This study secured research ethics approval from Simon Fraser University. There were two components to this study, the first part of the study consisted of training and providing participants with the opportunity to use LiteFarm for a period of approximately 2 months. There were seven farms included in this study represented by farmers and administrative farm operators of said farms. No quantitative data was collected by the researchers; however, food loss records could be utilized by farmers and support for collecting quantitative data was offered. The study's second component was semi-structured key informant interviews with each participant to assess their experience using LiteFarm. This study echoes another digital agriculture study which integrated the findings of seven dairy farms that trialed a new recording scheme, and then applied semi-structured interviews to assess the value of big data on farm decision making (Newton et al., 2020). The questions asked in the interview pertain to LiteFarm's effectiveness as a farm management app, namely when used to better track yield and sales, and as a food loss quantification estimation method. In terms of inclusion/exclusion criteria, this study contacted participants in the Metro Vancouver area that were accessible by motor vehicle and had fruit and vegetable crops that grew during the spring and summer seasons. Fruits and vegetables were chosen as LiteFarm was not capable of recording livestock records at the time of the study and food loss related to livestock has different causes and effects compared to fruits and vegetables.
The LiteFarm trial period started in April 2021 with online and in-person training sessions. The trial groups were divided into two timeframes to allow for adequate training and site visits. The first group started using the app in May 2021 and ended their trial in July 2021. The second group started in July 2021 and ended their trial in September 2021. Training sessions were led by the researcher utilizing a PowerPoint presentation and practicing with features on the app. Site visits were used to address problems related to the LiteFarm app or for general record keeping. This study looked at food loss quantification and utilized the harvest logs feature (see Figures 1–3) in the LiteFarm app. Harvest logs allow for a farmer to input anything they harvest off the field as well as anything eaten, gifted, or sold.
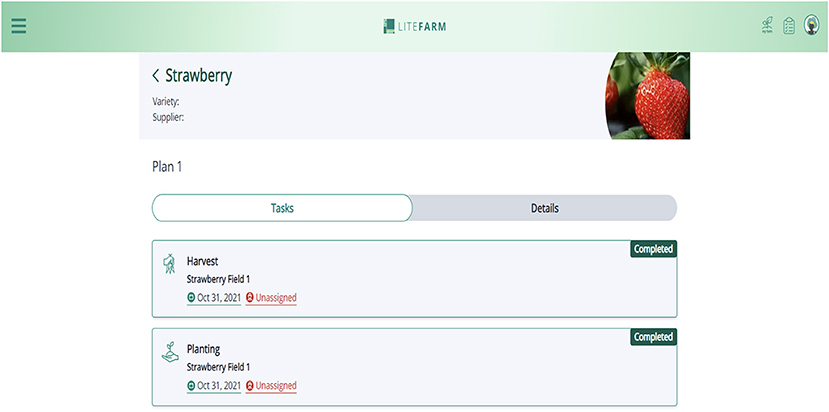
Figure 1. This LiteFarm page depicts the planning page where farmers would add harvest logs or other actions from the fields in the farm map. This page is where the harvest logs were recorded and harvested vs. sold calculations would be manually recorded.
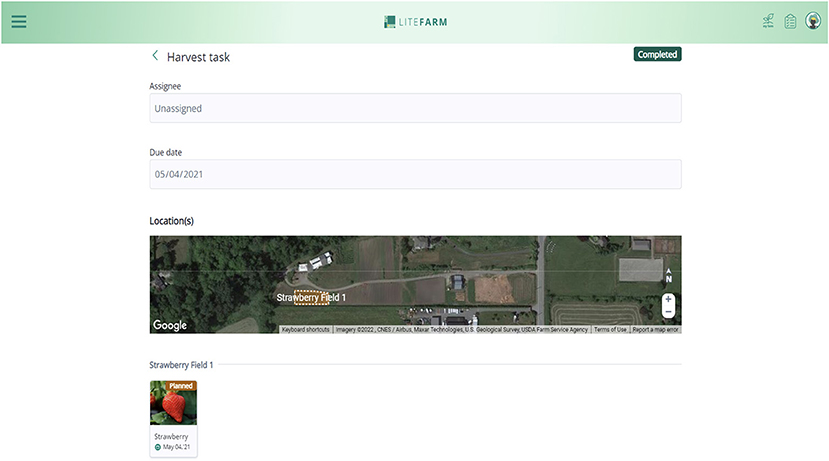
Figure 2. This page shows an overview for a given harvest log. Each log would be associated with a field and crop, allowing farmers to add a harvest use and quantity measurements.
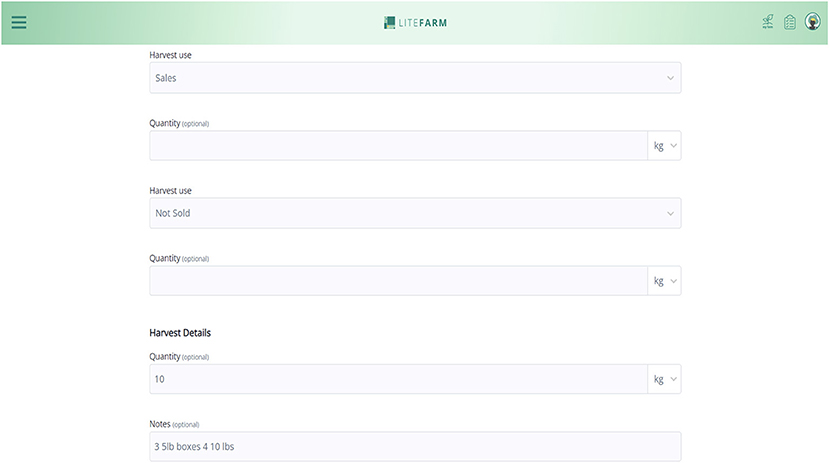
Figure 3. This page is related to Figure 2 as it is the bottom half of the harvest logs. This is where the use, quantity and other details are recorded.
Our method for estimating food loss saw farmers tracking their initial harvest and then inputting their sale records from that harvest. Once they had both numbers, they would take the difference between their harvest and their sales, and we quantified that number as the food loss. The harvest logs were the main feature used in the study; however, farmers were trained on other features in the app that may enhance the overall experience. Creating fields using the map feature (see Figure 4) and understanding overall environmental conditions with nutrient logs (Figure 5) were also used by farmers and could give the researchers insight into the overall effectiveness of LiteFarm as a management app. Creating mapped fields was required to input harvest logs, however, any other features were optional to the farmers.
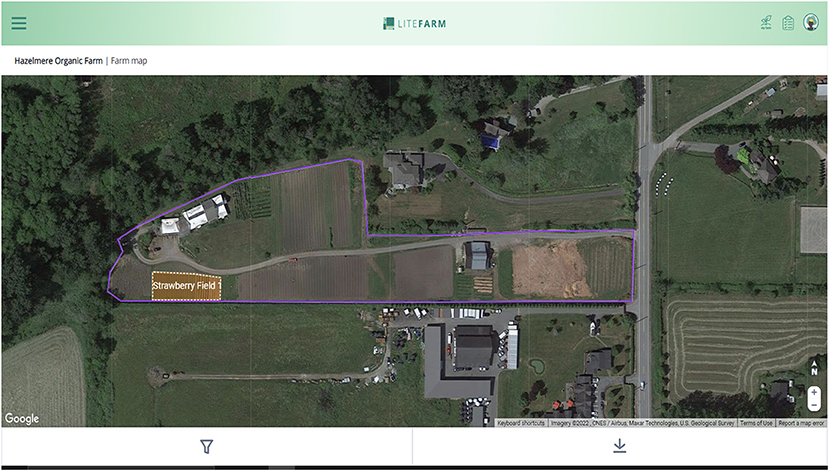
Figure 4. This LiteFarm page depicts the farm map, a feature where farmers add specific fields, draw property boundaries, and can judge spatial features.
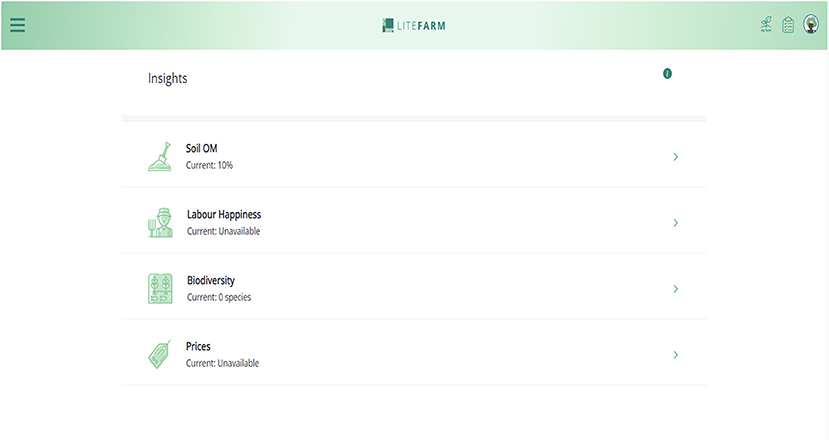
Figure 5. This page is the insights page, and it depicts features such as soil composition, labor happiness and financial records. This was an optional feature for participants.
Following the two trial periods of using LiteFarm, each participant was interviewed individually to collect in-depth qualitative data from their experience. The semi-structured interview questions are included as an appendix. The interviews were either in-person or online according to public health restrictions and participant preference. On average, participants took approximately 30–60 min to complete the post-trial interview. Interview participants were offered a $200 honorarium in exchange for their 2 months of testing the app. Each interview was transcribed using a software called Otter.ai and coded using the data analysis program NVivo.
The data analysis was done through NVivo using an inductive coding method. Inductive coding refers to qualitative data coding that first uses observations, in this case interviews, and developed themes from those observations (Chandra and Shang, 2019). Quotes associated with certain themes were counted and visuals created to emphasize aspects of food loss quantification and general app-based management on farms. For confidentiality, a pseudonym will be used for any direct quotes in the findings chapter. Figure 6 represents the scope of the study focusing on farm-based post-harvest loss:
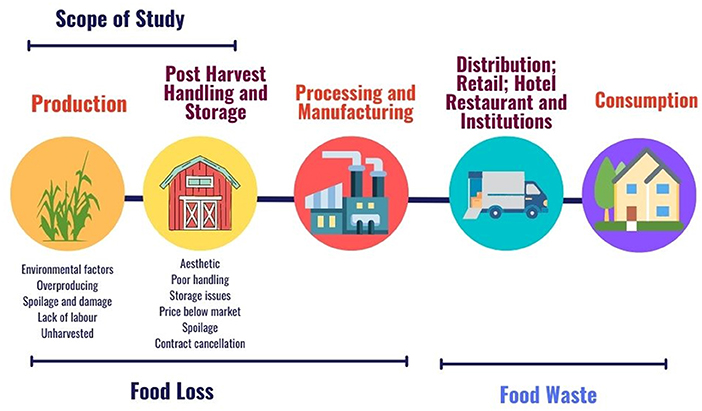
Figure 6. Scope of the study food loss and waste diagram [adapted from Nikkel et al. (2019)].
Limitations
The COVID-19 pandemic brought unprecedented changes to the agricultural industry in itself, even more so when it came to implementing this research. Pandemic restrictions were not conducive to in-depth learning and created barriers in recruiting and establishing relationships with the participants. The COVID-19 pandemic also forced many farmers into financial crisis and under undue stress. Four out of the seven farmers who participated had never used a digital agriculture app and had sporadic internet access. In the farm areas where the internet was sporadic, this made online training and general app usage difficult. Participants in north Surrey had better internet access and had more exposure to technology making their experience more streamlined. Under the COVID-19 pandemic conditions, the gaps in internet connectivity create inequitable results for using the app.
Due to the resource constraints as noted above, the small sample size of this study (n = 7) was justified (Lakens, 2022). This small sample size was helpful as the study required the researchers to be on stand-by to address the specific concerns of each participant and troubleshoot for a period of 2 months. While the sample size can be considered relatively small. As noted by Davies and Dodd (2002) applying “quantitative notions of rigor to qualitative research provides a poor instrument for evaluating qualitative research”. In a study on appropriate sample size for qualitative research, according to Boddy (2016), small sample size can be justified when it helps show direction for a future research field and it can be justified if it focuses on in depth-qualitative research. Moreover, other acceptable reasons for small sample size include the nature of the topic (Morse, 2000), and the amount of time spent with the participant (Marshall et al., 2013), which in the case of this study involved a novel use of an app and 2 months of trial. Finally, qualitative studies investigating theme saturation found that data saturation became evident at six individuals in studies that conduct qualitative in-depth interviews (Guest et al., 2006). As such, while the sample may seem small, the purpose and scope of this study justifies a sample of seven. Most importantly, another study on digital agriculture and the trial of a novel recording scheme also engaged with seven farms (Newton et al., 2020).
It is important to note that LiteFarm was not designed by UBC as an app for food loss quantification and this study looked to experimentally use an app for this unintended purpose. LiteFarm was designed by UBC as an environmental focused farm management app. The idea to use this app for food loss quantification was not initially seen by the developers and several customized settings had to be used for this study. There is no explicit “loss” category in the app, the food loss was calculated through a difference in harvest log inputs which are not tracked in the app but had to be manually recorded. This unintended use of LiteFarm may provide feedback for future updates of LiteFarm or new app developments.
Findings
The interview results that were compiled and analyzed provide a producer level perspective on food loss management with a digital agriculture app. The findings for this study have been categorized into themes resulting from the farmer's feedback and the qualitative coding in NVivo. Information about the participants can be found in Table 1. The average farm size in Metro Vancouver is 50 acres, which is relatively small as the region is surrounded by mountain ranges and also includes numerous urban centers (Metro Vancouver, 2014). The average farm size for our study is 17.4 acres (with the highest being 100 acres and the smallest being 1) and as such is relatively on the smaller side of the regional average. In Canada, the average age of farmers in 2021 is 56 years old (Statistics Canada, 2021). Meanwhile the average age of farmers in this study is 41 years old, with the oldest being 59 and the youngest being 22 years old.
Overall potential for utilizing DAT as a food loss quantification tool
The main research question for this study sought to understand whether the app LiteFarm could be utilized to support better food loss quantification for farmers (see Table 2 for Summary Findings). Most farmers perceive their unharvested yield as small, however Johnson et al. (2018) highlights that these could be underestimates of the true loss, as their study estimates 5.9 billion kilograms of food is left unharvested in the US each year. After seven farmers tested LiteFarm, the general consensus was that LiteFarm's method to log their yield and sales and therefore measured loss was not more accurate than previous measurement techniques (e.g., paper documentation). However, it is important to note that all of the farmers specified that they never formally and methodically measured food loss prior to this study. Although some farmers may find manual entry on digital interface challenging, other farmers were excited about using the app on their computer or phones and the potential of measuring loss:
The idea of an app that can allow you to just enter activities on your phone is a great idea for tracking food loss especially in the organic sector, I think in general any technology that can help people save time is good (Robert).
I think this technology on your phone can assist people with food loss measurement, it will be easy since we are on our phones a lot (Jack).
It was clear in hearing the initial feedback from farmers that they were open to measuring and tracking food loss with apps or digital tools if it is convenient and saves their time.
Barriers for food loss quantification using digital agriculture technology
Challenges with the definition of food loss and informal approach
The farmers in this study identified food loss as produce that is unusable in their agricultural operation, which is very different from common definitions used in the FLW and agricultural literature (Chaboud and Daviron, 2017). The farmers in the study saw food loss as a rare occurrence, because if produce was not sold it could be stored in freezers, tilled back into the soil, processed into a different product, eaten by livestock, consumed by the farmer themselves or even gifted to alternative food programs. Their perspective echoed the findings by Soma et al. (2021) which found that academic definitions of “food loss” did not always mesh with farmers' view. Because the outlets to absorb “food loss” (including tilling under the soil) are deemed to be numerous, many participants saw minimal utility in measuring “food loss.” Identifying a clear definition for food loss and educating farmers on the added benefits of accurate food loss quantification could provide a better contrast between informal food loss quantification and a more organized approach via LiteFarm. During the interviews, several farmers noted their informal approach to estimating losses prior to using the app:
We [Farmers] know there are statistics out there and this [LiteFarm app] could be quite practical and provide a proactive approach, right now it just happens in our brains (Jack).
Right now, it's all chicken scratch on my notes, this [LiteFarm app] could help out with a lot of that record keeping (Robert).
Even though several farmers acknowledged the potential benefits to formalizing food loss quantification through a digital tool like LiteFarm, using the app for food loss quantification did not resonate for most of the farmers given this discrepancy around food loss definitions.
Time and resource scarcity
All seven participating farmers in this study quoted time scarcity as a strong barrier to using LiteFarm for food loss quantification. While each farmer managed their operation with a different style or personal philosophy, the reality is that farming is a demanding career. Farmers must grow their produce while also managing their relationships with processors, distributors, and retailers. When asked about measuring losses at the initial stage, several farmers had the same response, “No time”. There are barely enough hours in a day for farmers to accomplish their daily tasks and asking them to carve out additional time for record keeping that is “theoretically beneficial” is difficult. Farmer such as Robert mentioned the time required to input the data:
The manual entry was cumbersome, if the software had an automated feature or predictive features it might help incentivize farmers to use it (Robert).
Manual entry apps do not save time when quantifying food loss and can be a huge barrier for farmers thinking to adopt this type of farm management app. Whether LiteFarm was more accurate or not was not the issue as record keeping could not be prioritized over essential farming activities. Automation for data inputs becomes a very important incentive for farmers as it could solve this barrier for manual entry software. The idea around automation is that it only requires an initial set up and then it accomplishes tasks without continuous farmer input. There was a consensus among all seven farmers that an automated software would provide more value than LiteFarm in its current state, however, the specific features and what an automated software/app can measure would require further study. Farmers also highlighted the complex timeframes of when agricultural planning takes place. A farmer's busiest time is during the spring and summer when there are physical field work tasks, LiteFarm is an app that requires constant inputs to remain accurate and valuable. Jack explains:
Most planning happens in November, December, and January, this is when farmers would have time to sit down with a computer and plan or sift through records. If LiteFarm had an automated feature or a way to import sales records or harvest log notes it may become more valuable in those planning months (Jack).
If LiteFarm did not require constant action in the busy summer months, it may garner more interest from farmers that would like to study their stats in their quieter seasons. While our study anticipated the best results being in the summer months with real time data, Jack suggests that there would be more time to evaluate the data from LiteFarm during off-season time when farmers have the ability to reflect and plan.
Not a “one size fits all” solution
Farming in Metro Vancouver is diverse and requires specific operational conditions to maintain a successful agricultural operation. This study looked at seven fruit and vegetable farmers, however, each farmer grew, organized, and sold their products differently. Given this diverse farming context, a broad approach app for food loss can be challenging to successfully implement. Two farmers in this study, Robert, and Susan, are both organic farmers in Metro Vancouver. Robert grows fruits and vegetables on a four-acre property, some of these vegetables are exposed in open fields while others are protected in greenhouses. Susan grows her fruits and vegetables on a two-acre property with all of her fields being completely exposed. Even though both farmers are organic certified and mostly grow on open fields, their operational styles require different management techniques. Susan prefers to have her crops matched to purchase orders as soon as possible while Robert likes to assess his fields and make pre planting estimates. LiteFarm offers a generalized method for farm management, however, each farmer experienced the app differently, even when seemingly attempting to input similar information. Robert noted the issue with a one size fits all approach in digital agriculture:
The app is one size fits all…changing the app would make it helpful for me but probably only me (Robert).
John, a medium scale, conventional/mixed use winery farmer, provides a similar view on the need to be context specific:
The app is useful but it comes down to the individual grower, some growers are strictly focused on increasing yields […] others may see value in the protecting their land and the environment, it depends on what values and style they use and maybe LiteFarm needs to have customizable options (John).
One of the most frequent suggestions for the LiteFarm app was including customizable features like adding preferences or additional categories for record keeping. Those customizable features speak to the fact that farmers need specific help with their operations based on specific crops, activities, or the scale of the farm. This study found that the specific techniques used on a farm are not necessarily supported through generalized, broad scale software tools. Feedback from 3 out of the 7 participants expressed that they wanted an option to include qualitative reasons for food loss so they can follow up. Jack specifically asked:
A huge piece is the ‘why', why does this food loss happen, it's easy enough to record it but farmers need more information to address it. There needs to be an increased incentive besides for our own personal curiosity (Jack).
Jack brings up that LiteFarm's food loss quantification method did not include both quantitative and qualitative data to allow for a full picture of the agricultural operation. Without the why, they felt it did not allow actions to prevent and reduce loss. If a farmer is not previously quantifying their food loss, it can be an added burden to measure and add a new daily activity. George is a blueberry farmer who sends all of their produce directly to a cannery following a harvest. The only method for food loss quantification would be picking up berries that have fallen off the bush and not retrieved for shipping to the cannery. The LiteFarm method for food loss quantification using manual inputs was not feasible as George estimates they ship roughly 5,000 pounds of blueberries to the cannery per day. The only feasible method for food loss quantification would be an automated system (e.g., remote sensing) as you cannot hire people to pick thousands of blueberries off the ground. In contrast to George's case, Robert can use the LiteFarm method for food loss quantification as he sells his product to grocery stores and restaurants. This means that if he over produces a crop and cannot sell the whole harvest, he can manually track the loss easier.
Educational and technological gaps
The use of digital apps generally benefits certain demographic, age ranges, and those with a certain level of technological experience. These gaps were identified by the participants and are seen as barriers to DAT adoption. Many farms may refuse to adopt DAT because they are family owned and have been operating for many generations without technological assistance. These farms may utilize specific growing techniques that hold unique value for the farmers:
The number one barrier to digital agriculture adoption is because of succession related reasons, farmers learned from their father and grandfathers and may not so easily change with electronics and technology. They want to keep the farm running successfully while sticking with the family techniques. It will be the younger generation that changes (Susan).
Farms with these values may not adopt new technology that supports different agricultural techniques. After DAT adoption, there were barriers that several participants including Robert, George, Jack, and Susan mentioned around “user friendliness”. While customizing is key for some, others found too many options in the app to be overwhelming:
There were times that it had too many features, it just became overwhelming and not user friendly with lengthy drop-down menus (Jack).
While many of the participants were initially willing to attempt to use LiteFarm for food loss quantification, it became evident in their interviews that without in-depth training, more time and more human resources, app-based management to measure losses would not be a top priority for their agricultural operations. There were also additional barriers related to language. George explained that his farm hires migrant workers who are mostly Asian or east Asian. Since they generally communicate in their native language, this restricts the type of on-farm activities they can do. LiteFarm has only three language options English, Spanish or Portuguese. This could pose issues for internal farm communication if workers who do not speak the three languages were expected to help with app inputs. Furthermore, George explains that many farm workers are older, as younger generations are pursuing jobs in other fields, and no longer working at the farm. The older generations of workers have an additional technological gap even if they are English, Spanish, or Portuguese speakers.
Competing with economic priorities
Sustainability focused efforts to measure loss do not show tangible results within short time frames. The tension between economic and environmental priorities also relates to time and resource scarcity as farmers may elect to use their time differently for which tangible economic returns can be realized immediately. Although food loss costs Canadian farmers 2.88 billion dollars annually which means that there is an economic incentive for reduction (Nikkel et al., 2019), the participants in this study regularly pointed out that when losses occur, it was often due to factors beyond their control, such as competing with cheaper imports:
…food may be described as lost because it was not sold. The reason could be because it was priced out by imported food that can sell at half the price of local food… (John).
John's perspective also reflects the feeling of helplessness and the futility of measuring losses when there seems to be no alternative. This sentiment confirms the perspectives of other farmers around factors that they feel are beyond their control.
Opportunities for food loss quantification using digital agriculture technology
Pre-harvest planning for food loss prevention
One unexpected result that came from using LiteFarm was how some of the farmers used the app for pre-harvest planning which in theory could potentially help with preventing food loss. Although pre-harvest planning does not address the gap for quantifying food loss, farmers identified LiteFarm as a useful tool to better plan future harvests using their experience and the app's field mapping software.
I would not use LiteFarm to track my food loss however I would more so use LiteFarm to maximize and more efficiently utilize land use which could indirectly prevent future food loss (Jack).
An additional pre harvest planning aspect that was suggested by Susan pertains to facilitating communication between food supply chain actors. LiteFarm has the potential to host a type of communication network that would support pre harvest planning with transparent, streamlined food supply chain actions. Susan describes this example here:
I spend a lot of time on managing orders, talking on the phone with grocery stores, distributors, restaurants, and they all have different contacts and different orders on different days. It becomes a lot of switching from different communication platforms, if you had something that put those together or at least reminded me who I need to talk to and why, that would be helpful. (Susan).
Susan identifies this opportunity for food supply chain actors to communicate in an organized manner.
Increasing awareness of environmental issues
Climate change will lead to increased land degradation and create significant economic losses, amplifying the need to protect arable land and reduce food loss (IPCC, 2022). However, conventional farming practices that anchor many of Metro Vancouver's large scale food producers may not easily shift toward more environmentally friendly practices. DAT that highlights these environmental concerns may provide a mechanism to raise environmental awareness even with conventional operations. For example, as one the larger-scale farmer in this study, George described his emerging awareness about the issue of food loss and its environmental impact through this app:
I never really considered climate change as a factor in our personal operation and knowing the connection [referring to food loss] to our harvest will be important moving forward (George).
While George had no intention of neglecting environmental considerations with his operation, it is apparent that the economic aspect weighs heavily on his mind. When George realized the connection between climate change and food loss, he noted how this information will impact how he moves forward.
Discussion
Our results on the use of the LiteFarm app for food loss quantification were mixed, with some farmers enjoying their experience and others feeling overwhelmed. It became clear that LiteFarm is a great tool for planning, but in this study, we found that it was challenging for farmers to use the app during the busy growing season. The barriers for using LiteFarm as a food loss quantification DAT and the opportunities are highlighted and compared to previously published studies.
Barriers to food loss quantification using digital agriculture technology
This study found several barriers for food loss quantification using DAT including time and resource scarcity, generalized technology designs, educational gaps, technological gaps and competing economic priorities. One initial barrier to participation in food loss quantification is that farmers' approach and perspectives around food loss differs from typical academic definitions. The FAO (2019) defines food loss as food that is lost in the production, post-harvest, and processing stages up to but not including retail. However, this study found that farmers were hesitant to define unsold foods as “food loss” since alternative options exist such as tilling food back into the soil, processing them into non-perishables or donating food to alternative markets. There is also an expectation by the farmers that there will always be losses, which added to the hesitancy of quantifying the inevitable. However, Johnson et al. (2018) found that many food loss statistics were underestimated, potentially due to inconsistent definitions for food loss or confusion related to alternative uses. This study and other food loss quantification studies (Delgado et al., 2017; Sheahan and Barrett, 2017; Hartikainen et al., 2018; Johnson et al., 2018) stress the importance of measuring food loss even though farmers may express concerns and not see immediate benefits.
Inconsistent food loss definitions also bring up concerns related to cognitive dissonance. Markowitz et al. (2014) describes the challenges with climate change communication when certain mitigation measures may impact an individual's livelihood. In the case of food loss, most farmers understand the environmental impacts of postharvest loss (Soma et al., 2021). However their personal livelihoods and financial situations make addressing food loss difficult. As such, farmers may elect to ignore food loss quantification all together to avoid internal tension and guilt. Accurate food loss quantification will require consistency in its method and considerations for the complexity of behaviors and different contexts seen in the agricultural field.
DAT adoption also has different challenges as many farm technologies have been designed as a “one size fits all” solution not acknowledging the educational and technological gaps in diverse farming contexts. For example, rural farmers may face barriers such as gaps in internet infrastructure and financial constraints that are not present in larger-scale, urban farming operations (Weersink et al., 2021). In this study, farmers experienced internet connectivity issues in some of the rural areas of Metro Vancouver. This hindered both online training opportunities as well as daily inputs for LiteFarm. Farmers in these rural areas generally had a small living establishment or lived in housing away from their agricultural operation resulting in a lack of established internet infrastructure for themselves. Bronson (2019) found that when rural, small-scale farmers were asked about benefits from DAT their responses expressed historic concerns that DAT would only benefit industrialized operations and already powerful food systems actors. Without guaranteed returns for individual returns, farmers would be less willing to support building new infrastructure for data-based DAT (Bronson, 2019). DAT is programmed to provide large data sets on individual crop which could be more influential for monoculture or commodity farmers that focus on a few desirable crops (Bronson and Knezevic, 2019). Polyculture farmers rely on several different crops for one order, maybe in the form of salad boxes or CSA type programs. As seen in this study, farmers were able to use LiteFarm's flexibility to their benefit, however, most DAT focusses on monoculture and commodity crops with large data sets which may not be useful for a general group of polyculture farmers (Bronson and Knezevic, 2019). Furthermore, if farmers become dependent on DAT it may expose them to financial volatility related to equipment maintenance (Rotz et al., 2019). Once farmers are accustomed to technology or have incurred debt to acquire the technology, it can financially lock in those farmers (Rotz et al., 2019). Fortunately, LiteFarm is open source and free which could pave the way for other open-source technology that allows for creativity and technological advancement without incurring debt for high end equipment. However, LiteFarm exposed language inclusivity issues that may arise with DAT adoption. Many farms may employ multicultural labor or operate under agricultural standards from around the world. As Soma and Nuckchady (2021) identified, equity for DAT is important for broad adoption and features such as different languages, universal visuals and flexible qualitative inputs are important in the agricultural field.
There are barriers to LiteFarm's adoption that were inherent to certain farms given their specific farming routines. For farmers with different types of crops, committing several hours of data input for every harvest is difficult. Alexander et al. (2017) and Johnson et al. (2018) both agree that time scarcity is a major barrier for measurement and adopting new DAT. Training, inputs and equipment maintenance all contribute to labor costs and time spent on daily operations which, without guaranteed returns, creates a tension against economic priorities (Benyam et al., 2021). These economic priorities will often take precedent over food loss quantification and associated environmental or social factors (Benyam et al., 2021). This study confirmed Benyam et al. (2021) findings that economic concerns weigh heavily on a farmer's decisions and that without a guaranteed return from quantifying food loss, it would be difficult to justify the time commitment toward training and accomplishing this task. Benyam et al. (2021) also describes how farmers may see benefits from DAT adoption for the purpose of FLW prevention or reduction, however, the risk of failure and the time needed to use new technology may disincentivize new adopters. Farmers may also struggle with “temporal discounting” (Critchfield and Kollins, 2001) as time scales for individual economic priorities do not align with broad environmental priorities.
Another concern related to DAT adoption relates to the social aspects and concerns around data ownership and concerns around intergovernmental data sharing which Soma and Nuckchady (2021) found to worry farmers because they would not know who might be utilizing their data and why. These concerns were echoed in this study's recruitment phase. Several prospective farmers were unsure about participating in this study, and a few were willing to share that they did not want their food loss data shared with the government, the technology company, or any farmers in their community. These concerns stemmed from social ridicule they might face if their food loss data was not considered appropriate and this relates to the previously identified cognitive dissonance barrier discussed in this paper (Markowitz et al., 2014).
Opportunities to address food loss using digital agriculture technology
As noted by Messner et al. (2020), food loss prevention is more important in the food loss hierarchy, however, reduction is more commonly researched and practiced in our global food system. The literature on DAT identified how automated sensors can provide predictive land management tasks from current and past conditions (Sarker et al., 2020) or utilizing farmer experience with technological support to maximize land use (Finger et al., 2019). LiteFarm has taken both predictive land management and input mapping to create a flexible crop planning experience for farmers. When LiteFarm was proposed to our participants as a way for food loss quantification, it was initially met with skepticism. However, when training for LiteFarm included mapping fields and tracking potential harvests, many farmers described this as a high value planning method. Mapping allowed farmers to dictate their own boundaries, use their knowledge to create a map of their farm, and draw practical conclusions from their experience.
While better land use may benefit society by slowing agricultural land expansion and mitigating land degradation (UNCCD, 2021), it may not support issues related to market volatility and weather risks that lead to overproduction. Soma et al. (2021) highlights that farmers overproduce as a form of insurance related to market volatility, weather risks and contractual obligations. This overproduction may lead to increased land degradation and increased costs for the farm. Golan et al. (2020) proposes an idea of optimal food loss where overproduced food is necessary to ease risks. LiteFarm and other DAT apps may allow farmers to utilize their current land base better and increase yields. However, without adequate markets or alternatives, increased food production may further exacerbate the situation.
Social factors may push farmers toward DAT adoption following initial uncertainty. George, a large-scale blueberry farm in this study initially saw no benefit from the LiteFarm app for his operation. However, he agreed to test the app after a reference from one of his colleagues. Farmers can be persuaded to adopt new techniques or technologies if there is a social precedent or reference from the community. Le Coent et al. (2021) supports the point that farmers are influenced by social norms especially if their peers are voluntarily protecting an environmental or economic public good such as air quality, ground water quality or farmer's market infrastructure. In the case of DAT, farmers may choose to support new technology testing if other farmers agree on broad scale benefits such as efficient land use planning or other environmental benefits. Other potential benefits that were raised was the facilitation of communications between sectors. For example, through the sharing of the app, certain sectors such as retailers and restaurants could better identify upcoming harvests from their producers and make changes to their consumer offerings. These options to facilitate communications and sales could indirectly support food loss prevention.
Conclusions
The use of DAT has the potential to create positive contributions to food loss measurement, prevention, and reduction. This study highlighted some of the potential opportunities from the use of digital agriculture through the use of the farm management app LiteFarm. This study started with two main objectives, 1) to assess the DAT's potential as a tool for food loss quantification and 2) to identify the opportunities and barriers for farmers adopting and using DAT as a tool for food loss quantification. The findings demonstrate that although DAT has the potential as a tool for better pre-harvest farm planning, it did not resonate with most of the farmers as a tool for food loss quantification. This is primarily because farms operate differently and utilize diverse agricultural techniques which were not captured by a “one size fits all” technology such as LiteFarm. As food loss often occurs due to factors beyond the farmers' control (Soma et al., 2021), several farmers felt that measuring losses felt futile. This study found that economic priorities will outweigh environmental priorities, agreeing with Benyam et al. (2021) who also found this as a barrier to DAT adoption because farmers were not guaranteed economic returns for the given time investment. DAT was also shown to affect farmers differently depending on agricultural worldview and crop type; this is echoed by Bronson and Knezevic (2019) who discuss DAT bias toward large commodity farms vs. polyculture farms. Furthermore, Roe (2020) confirms that a successful measurement method or technology must recognize the complexity and nuances around the diversity of different farms. There will rarely be a standardized method or program for food loss quantification and DAT may just be a tool that is shaped with a given farmer's experience.
One unexpected aspect of the study is the potential for the app to improve land use management which may lead to decreasing land degradation. This is important considering the United Nations report that roughly 25 percent of the global land base is degraded by over usage and climate related impacts (UNCCD, 2021). While these outcomes do not directly address food loss quantification, it can potentially support efforts toward food loss prevention. Unintended findings about the potential for pre-harvest planning means that future follow up studies should consider exploring the food loss prevention and pre-harvest planning potential of DAT apps. To conclude, while this study found that the technology may offer opportunities for farmers, it is important to support deeper collaboration with farmers when designing new DAT to support food loss quantification. Initiatives such as co-creation and collaboration will help to improve user friendliness and prioritizing language inclusivity will better support the ability for diverse farmers/farm laborers to use the tools. Moreover, integrating communication tools to allow for streamlined collaboration with buyers may help with better planning and facilitating purchases with buyers. Digital agricultural tools like LiteFarm can help support the broader goals of food loss prevention and potentially reduction, but the tool itself will not address the root cause of the food loss problem such as deeper issues around time scarcity, lack of resources, and factors beyond the farmers control (international trade, market pricing). Solving food loss will require more equitable relationships between all actors in the global food system.
Data availability statement
The raw data supporting the conclusions of this article will be made available by the authors, without undue reservation.
Ethics statement
The studies involving human participants were reviewed and approved by Simon Fraser University. The patients/participants provided their written informed consent to participate in this study.
Author contributions
TS: study conception and design. AH: data collection. AH and TS: analysis and interpretation of results and draft manuscript preparation. Both authors reviewed the results and approved the final version of the manuscript.
Funding
This study was funded by the Social Sciences and Humanities Research Council Insight Grant No. 435–2019-0155.
Conflict of interest
The authors declare that the research was conducted in the absence of any commercial or financial relationships that could be construed as a potential conflict of interest.
Publisher's note
All claims expressed in this article are solely those of the authors and do not necessarily represent those of their affiliated organizations, or those of the publisher, the editors and the reviewers. Any product that may be evaluated in this article, or claim that may be made by its manufacturer, is not guaranteed or endorsed by the publisher.
References
Advisory Council on Economic Growth. (2017). Unleashing the growth potential of key sectors. Available online at: https://www.budget.gc.ca/aceg-ccce/pdf/key-sectors-secteurs-cles-eng.pdf (accessed October 19, 2022).
Aldaco, R., Hoehn, D., Laso, J., Margallo, M., Ruiz-Salmón, J., Cristobal, J., et al. (2020). Food waste management during the COVID-19 outbreak: a holistic climate, economic and nutritional approach. Sci. Total Environ. 742, 140524. doi: 10.1016/j.scitotenv.2020.140524
Alexander, P., Brown, C., Arneth, A., Finnigan, J., Moran, D., and Rounsevell, M. D. (2017). Losses, inefficiencies, and waste in the global food system. Agric. Syst. 153, 190–200. doi: 10.1016/j.agsy.2017.01.014
Astill, J., Dara, R. A., Campbell, M., Farber, J. M., Fraser, E. D., Sharif, S., et al. (2019). Transparency in food supply chains: A review of enabling technology solutions. Trends Food Sci. Technol. 91, 240–247. doi: 10.1016/j.tifs.2019.07.024
BC Food Security Task Force (2019). The Future of BC's Food System. Available online at: https://engage.gov.bc.ca/app/uploads/sites/121/2020/01/FSTF-Report-2020-The-Future-of-Food.pdf (accessed October 19, 2022).
BC Ministry of Agriculture Food Fisheries. (2016). Agriculture Census. Available online at: https://www2.gov.bc.ca/assets/gov/farming-natural-resources-and-industry/agriculture-and-seafood/statistics/census/census-2016/aginbrief_2016_all_province_region_regional_districts.pdf (accessed October 19, 2022).
BC Ministry of Agriculture Food Fisheries. (2022). Featured Services. Available online at: https://www2.gov.bc.ca/gov/content/governments/organizational-structure/ministries-organizations/ministries/agriculture (accessed October 19, 2022).
Béné, C. (2020). Resilience of local food systems and links to food security – A review of some important concepts in the context of COVID-19 and other shocks. Food Secur. 12, 805–822. doi: 10.1007/s12571-020-01076-1
Benyam, A., Soma, T., and Fraser, E. (2021). Digital agricultural technologies for food loss and waste prevention and reduction: Global trends, adoption opportunities and barriers. J. Cleaner Product. 323, 129099. doi: 10.1016/j.jclepro.2021.129099
Boddy, C. R. (2016). Sample size for qualitative research. Qualit. Market Res. 19, 426–432. doi: 10.1108/QMR-06-2016-0053
Bosona, T., and Gebresenbet, G. (2013). Food traceability as an integral part of logistics management in food and agricultural supply chain. Food Contr. 33, 32–48. doi: 10.1016/j.foodcont.2013.02.004
Bronson, K. (2019). Looking through a responsible innovation lens at uneven engagements with digital farming. NJAS - Wageningen J. Life Sci. 90, 100294. doi: 10.1016/j.njas.2019.03.001
Bronson, K., and Knezevic, I. (2016). Big Data in food and agriculture. Big Data Soc. 3, 205395171664817. doi: 10.1177/2053951716648174
Bronson, K., and Knezevic, I. (2019). The digital divide and how it matters for Canadian food system equity. Canadian J. Commun. 44, 63–68. doi: 10.22230/cjc.2019v44n2a3489
Carolan, M. (2017). Agro-digital governance and life itself: food politics at the intersection of code and affect. Sociol. Ruralis 57, 816–835. doi: 10.1111/soru.12153
Chaboud, G., and Daviron, B. (2017). Food losses and waste: Navigating the inconsistencies. Global Food Secur. 12, 1–7. doi: 10.1016/j.gfs.2016.11.004
Chandra, Y., and Shang, L. (2019). “Inductive coding,” in Qualitative Research Using R: A Systematic Approach. (Springer, Singapore), 91–106. doi: 10.1007/978-981-13-3170-1_8
Ciccullo, F., Cagliano, R., Bartezzaghi, G., and Perego, A. (2021). Implementing the circular economy paradigm in the agri-food supply chain: The role of food waste prevention technologies. Resour. Conserv. Recycl. 164, 105114. doi: 10.1016/j.resconrec.2020.105114
Clapp, J., and Moseley, W. G. (2020). This food crisis is different: COVID-19 and the fragility of the neoliberal food security order. J. Peasant Stud. 47, 1393–1417. doi: 10.1080/03066150.2020.1823838
Critchfield, T. S., and Kollins, S. H. (2001). Temporal Discounting: Basic Research and the Analysis of Socially Important Behaviour. J. Appl. Behav. Analy. 34, 101–122. doi: 10.1901/jaba.2001.34-101
Davies, D., and Dodd, J. (2002). Qualitative research and the question of rigor. Qualit. Health Res. 12, 279–289. doi: 10.1177/104973230201200211
Delgado, L., Schuster, M., and Torero, M. (2017). Reality of food losses: a new measurement methodology. Munich Personal RePEc Archive
Devereux, S., Béné, C., and Hoddinott, J. (2020). Conceptualising COVID-19's impacts on household food security. Food Secur. 12, 769–772. doi: 10.1007/s12571-020-01085-0
FAO (2016). Food Loss Analysis: Causes and Solutions Case studies in the Small-scale Agriculture and Fisheries Subsectors. Available online at: https://www.fao.org/3/az568e/az568e.pdf (accessed October 19, 2022).
FAO (2019). The State of Food and Agriculture 2019: Moving forward on Food Loss and Waste Reduction. RomeQ20. Available online at: https://www.fao.org/3/ca6030en/ca6030en.pdf (accessed October 19, 2022).
FAO (2020). QandA: COVID-19 pandemic – impact on food and agriculture | FAO| Food and Agriculture Organization of the United Nations. Available online at: http://www.fao.org/2019-ncov/q-and-a/impact-on-food-and-agriculture/en/ (accessed October 19, 2022).
Feedback. (2021). Causes of Food Waste in International Supply Chains. Available online at: https://feedbackglobal.org/wp-content/uploads/2017/02/Causes-of-food-waste-in-international-supply-chains.pdf (accessed October 19, 2022).
Fei, S., Ni, J., and Santini, G. (2020). Local food systems and COVID-19: an insight from China. Resour. Conserv. Recycl. 162, 105022. doi: 10.1016/j.resconrec.2020.105022
Finger, R., Swinton, S. M., El Benni, N., and Walter, A. (2019). Precision farming at the nexus of agricultural production and the environment. Ann. Rev. Resour. Econ. 11, 313–335. doi: 10.1146/annurev-resource-100518-093929
Giordano, C., Falasconi, L., Cicatiello, C., and Pancino, B. (2020). The role of food waste hierarchy in addressing policy and research: A comparative analysis. J. Cleaner Prod. 252, 119617. doi: 10.1016/j.jclepro.2019.119617
Golan, E., Minor, T., and Thornsbury, S. (2020). Existing efforts in modelling early value chain food losses. Econ. Food Loss Prod. Ind. 2, 14–24. doi: 10.4324/9780429264139-2
Guest, G., Bunce, A., and Johnson, L. (2006). How many interviews are enough? An experiment with data saturation and variability. Field Method. 18, 59–82. doi: 10.1177/1525822X05279903
Gunders, D., and Bloom, J. (2017). Wasted: How America is Losing up to 40% of its Food from Farm to Fork to Landfill 2nd Edition. Natural Resources Defense Council. Available online at: https://www.nrdc.org/resources/wasted-how-america-losing-40-percent-its-food-farm-fork-landfill (accessed October 19, 2022).
Gustavsson, J., Cederberg, C., and Sonesson, U. (2011). Global Food Losses and Food Waste. Available online at: https://www.fao.org/3/mb060e/mb060e00.pdf (accessed October 19, 2022).
Hartikainen, H., Mogensen, L., Svanes, E., and Franke, U. (2018). Food waste quantification in primary production – The Nordic countries as a case study. Waste Manage. 71, 502–511. doi: 10.1016/j.wasman.2017.10.026
Hobbs, J. E. (2020). Food supply chains during the COVID-19 pandemic. Canad. J. Agric. Econ. 68, 171–176. doi: 10.1111/cjag.12237
IPCC (2022). Intergovernmental Panel on Climate Change. AR6 Summary for Policymakers. Available online at: https://report.ipcc.ch/ar6wg2/
Janousek, A., Markey, S., and Roseland, M. (2018). “We see a real opportunity around food waste”: exploring the relationship between on-farm food waste and farm characteristics. Agroecol. Sustain. Food Syst. 42, 933–960. doi: 10.1080/21683565.2018.1468381
Johnson, L. K., Bloom, J. D., Dunning, R. D., Gunter, C. C., Boyette, M. D., and Creamer, N. G. (2019). Farmer harvest decisions and vegetable loss in primary production. Agric. Syst. 176, 102672. doi: 10.1016/j.agsy.2019.102672
Johnson, L. K., Dunning, R. D., Bloom, J. D., Gunter, C. C., Boyette, M. D., and Creamer, N. G. (2018). Estimating on-farm food loss at the field level: A methodology and applied case study on a North Carolina farm. Resour. Conserv. Recycl. 137, 243–250. doi: 10.1016/j.resconrec.2018.05.017
Kitinoja, L., Tokala, V. Y., and Brondy, A. (2018). Challenges and opportunities for improved postharvest loss measurements in plant-based food crops. J. Postharvest Technol. 6, 16–34.
Klerkx, L., and Rose, D. (2020). Dealing with the game-changing technologies of Agriculture 4.0: How do we manage diversity and responsibility in food system transition pathways? Global Food Secur. 24, 100347. doi: 10.1016/j.gfs.2019.100347
Lakens, D. (2022). Sample size justification. Collabra: Psychol. 8, 33267. doi: 10.1525/collabra.33267
Le Coent, P., Préget, R., and Thoyer, S. (2021). Farmers follow the herd: a theoretical model on social norms and payments for environmental services. Environ. Resour. Econ. 78, 287–306. doi: 10.1007/s10640-020-00532-y
Lipinski, B., Hanson, C., Waite, R., Searchinger, T., and Lomax, J. (2013). “Reducing Food Loss and Waste.” Working Paper, Installment 2 of Creating a Sustainable Food Future. Washington, DC: World Resources Institute
Magruder, J. R. (2018). An assessment of experimental evidence on agricultural technology adoption in developing countries. Ann. Rev. Resour. Econ. 10, 299–316. doi: 10.1146/annurev-resource-100517-023202
Markowitz, E., Hodge, C., Harp, G., St John, C., Marx, S. M., Speiser, M., et al. (2014). A Guide to Effective Climate Change Communication. Columbia: Centre for Research on Environmental Decisions, Earth Institute, Columbia University.
Marshall, B., Cardon, P., Poddar, A., and Fontenot, R. (2013). Does sample size matter in qualitative research?: a review of qualitative interviews in IS research. J. Comput. Inf. Syst.54, 11–22. doi: 10.1080/08874417.2013.11645667
Messner, R., Richards, C., and Johnson, H. (2020). The “Prevention Paradox”: food waste prevention and the quandary of systemic surplus production. Agric. Human Values 37, 805–817. doi: 10.1007/s10460-019-10014-7
Metro Vancouver. (2011). Regional Food System Strategy Sustainable Region Initiative Turning Ideas into Action. Available online at: www.metrovancouver.org (accessed October 19, 2022).
Metro Vancouver. (2014). Farming in Metro Vancouver: Policy Backgrounder. Retrieved June 9th from Available online at: http://www.metrovancouver.org/services/regional-planning/PlanningPublications/Metro2050-PolicySummary-Agriculture.pdf (accessed October 19, 2022).
Morse, J. M. (2000). Determining sample size. Qualit. Health Res. 10, 3–5. doi: 10.1177/104973200129118183
Neff, R. A., Dean, E. K., Spiker, M. L., and Snow, T. (2018). Salvageable food losses from Vermont farms. J. Agric. Food Syst. Commun. Develop. 8, 39–72. doi: 10.5304/jafscd.2018.082.006
Newton, J. E., Nettle, R., and Pryce, J. E. (2020). Farming smarter with big data: Insights from the case of Australia's national dairy herd milk recording scheme. Agric. Syst. 181, 102811. doi: 10.1016/j.agsy.2020.102811
Nikkel, L., Maguire, M., Gooch, M., Bucknell, D., LaPlain, D., Dent, B., et al. (2019). The Avoidable Crisis of Food Waste: The Roadmap. Ontario, Canada: Second Harvest and Value Chain Management International.
Parfitt, J., Barthel, M., and MacNaughton, S. (2010). Food waste within food supply chains: quantification and potential for change to 2050. Biol. Sci. 365, 3065–3081. doi: 10.1098/rstb.2010.0126
Piras, S., Garcia Herrero, L., Burgos, S., Colin, F., Gheoldus, M., Ledoux, C., et al. (2018). Unfair Trading Practice Regulation and Voluntary Agreements targeting food waste: A policy assessment in select EU Member States. REFRESH Deliverable 3.2. Retrieved from: https://eu-refresh.org/unfair-trading-practice-regulation-and-voluntary-agreements-targeting-food-waste.html (accessed October 20, 2022).
Roe, B. (2020). Existing efforts in modelling early value chain food losses. Econ. Food Loss Prod. Ind. 3, 25–39. doi: 10.4324/9780429264139-3
Rotz, S., Gravely, E., Mosby, I., Duncan, E., Finnis, E., Horgan, M., et al. (2019). Automated pastures and the digital divide: How agricultural technologies are shaping labour and rural communities. J. Rural Stud. 68, 112–122. doi: 10.1016/j.jrurstud.2019.01.023
Sarker, M. N. I., Islam, M. S., Murmu, H., and Rozario, E. (2020). Role of big data on digital farming. Int J Sci Technol Res. 9, 1222–5.
Shafiee-Jood, M., and Cai, X. (2016). Reducing food loss and waste to enhance food security and environmental sustainability. Environ. Sci. Technol. 50, 8432–8443. doi: 10.1021/acs.est.6b01993
Sheahan, M., and Barrett, C. B. (2017). Review: Food loss and waste in Sub-Saharan Africa. Food Policy 70, 1–12. doi: 10.1016/j.foodpol.2017.03.012
Shepherd, M., Turner, J. A., Small, B., and Wheeler, D. (2020). Priorities for science to overcome hurdles thwarting the full promise of the ‘digital agriculture' revolution. J. Sci. Food Agric. 100, 5083–5092. doi: 10.1002/jsfa.9346
Soma, T., Kozhikode, R., and Krishnan, R. (2021). Tilling food under: Barriers and opportunities to address the loss of edible food at the farm-level in British Columbia, Canada. Resour. Conserv. Recycl. 170, 105571. doi: 10.1016/j.resconrec.2021.105571
Soma, T., and Nuckchady, B. (2021). Communicating the benefits and risks of digital agriculture technologies: perspectives on the future of digital agricultural education and training. Front. Commun. 6, 259. doi: 10.3389/fcomm.2021.762201
Statistics Canada. (2021). Canada's 2021 Census of Agriculture: A story about the transformation of the agriculture industry and adaptiveness of Canadian farmers. Available online at: https://www150.statcan.gc.ca/n1/daily-quotidien/220511/dq220511a-eng.htm (accessed October 19, 2022).
UNCCD. (2021). Restoring Life to the Land. The Role of Sustainable Land Management in Ecosystem Restoration. Available online at: https://www.unccd.int/publications (accessed October 19, 2022).
United Nations Environment Programme. (2021). Food Waste Index Report 2021. Nairobi. Available online at: https://www.unep.org/resources/report/unep-food-waste-index-report-2021 (accessed October 19, 2022).
Verma, M., Plaisier, C., van Wagenberg, C. P., and Achterbosch, T. (2019). A systems approach to food loss and solutions: Understanding practices, causes, and indicators. Towards Sustain. Global Food Syst. 11, 102–120. doi: 10.3390/su11030579
Weersink, A., von Massow, M., Bannon, N., Ifft, J., Maples, J., McEwan, K., et al. (2021). COVID-19 and the agri-food system in the United States and Canada. Agric. Syst. 188, 103039. doi: 10.1016/j.agsy.2020.103039
Wheeler, T., and Von Braun, J. (2013). Climate change impacts on global food security. Science. 341, 508–513. doi: 10.1126/science.1239402
Wittman, H., James, D., and Mehrabi, Z. (2020). Advancing food sovereignty through farmer-driven digital agroecology. Ciencia e Investigación Agrarian. 47, 235–248. doi: 10.7764/ijanr.v47i3.2299
Wohlers, A., Le, E., Stewart, M., and Frame, M. (2021). LiteFarm sustainability assessment framework. Vancouver: University of British Columbia Library
Yaffe-Bellany, D., and Corkery, M. (2020). Dumped Milk, Smashed Eggs, Plowed Vegetables: Food Waste of the Pandemic - The New York Times. Available online at: https://www.nytimes.com/2020/04/11/business/coronavirus-destroying-food.html (accessed October 19, 2022).
Keywords: digital agriculture, food loss, technology, app-based management, quantification
Citation: Hook A and Soma T (2022) Sustainability potential of app-based food loss measurement: Farmers' perspectives in southwestern British Columbia, Canada. Front. Sustain. 3:1024100. doi: 10.3389/frsus.2022.1024100
Received: 21 August 2022; Accepted: 14 October 2022;
Published: 31 October 2022.
Edited by:
Antonino Marvuglia, Luxembourg Institute of Science and Technology (LIST), LuxembourgReviewed by:
Thomas Schaubroeck, Luxembourg Institute of Science and Technology (LIST), LuxembourgStephanie Zimmer, Institut fir Biologësch Landwirtschaft an Agrarkultur Luxemburg (IBLA), Luxembourg
Copyright © 2022 Hook and Soma. This is an open-access article distributed under the terms of the Creative Commons Attribution License (CC BY). The use, distribution or reproduction in other forums is permitted, provided the original author(s) and the copyright owner(s) are credited and that the original publication in this journal is cited, in accordance with accepted academic practice. No use, distribution or reproduction is permitted which does not comply with these terms.
*Correspondence: Tammara Soma, tammara_soma@sfu.ca