- 1Centre for Environmental Policy, Imperial College London, London, United Kingdom
- 2The Sargent Centre for Process Systems Engineering, Imperial College London, London, United Kingdom
- 3Department of Chemical Engineering, Imperial College London, London, United Kingdom
Integration of alternative technological concepts such as switching to alternative fuels, advanced energy efficiency, and carbon capture and storage in existing industrial energy systems can prove highly effective at minimizing emissions; however, their adoption is low since solutions using these concepts raise costs considerably. The hypothesis of this work is a hierarchical combination of these concepts can reduce mitigation cost. To this end a mixed method approach is applied combining energy simulation with a novel Mixed Integer Linear Programming model developed to explore 48 alternative solutions to make industrial energy systems more sustainable. The method was applied to the most common industrial energy systems configurations. Results show that the added cost of integrating alternative technological concepts are lowered when energy efficiency via direct heat recovery is explored first in an optimization-based hierarchy of options. The hierarchy is advanced energy efficiency before fuel and technology switching or integrating carbon capture and storage. This means process integration can pay for steeper reductions in carbon emissions. Integrating alternative technological concepts optimally and hierarchically reduced emissions by 61%, and costs by 55.7% compared to a partial integration for a heat-only business-as-usual industrial energy systems. Even though switching to an alternative fuel (blue hydrogen) reduces carbon emissions by 72%, costs increase by at least 3% compared to a system using fuel gas and fuel oil. A hierarchical integration of blue hydrogen reduces cost by 47% and carbon emissions by 88.7%. Partial integration of carbon capture and storage reduces carbon emissions by 36% but costs increase by 89%, with full integration using optimization and the hierarchy costs only increase by 6.3%. Therefore, the cost-effectiveness of integrating alternative technological concepts is highly influenced by the hierarchy which seeks to minimize demand for energy from industrial processes first, then increase the supply efficiency of industrial energy systems, and before switching to alternative fuels and technologies.
Highlights
- A hierarchy of alternative technological concepts makes decarbonization cost effective.
- Direct heat recovery should be prioritized first.
- Cost savings of over 20% is possible from an optimal hierarchical ordering.
- Carbon emissions reduces further by at least 15%.
- An efficient industrial energy system can pay for steeper decarbonization measures.
1. Introduction
1.1. Background
The transition from an agrarian society to an industrial one requires large-scale manufacturing—raw materials are refined, processed, purified, and transported. These processes give birth to three components: energy, emissions, and product. Energy is also consumed ubiquitously as every product experience a life cycle, and every step along this cycle is energy exhausting and carbon emitting. In Europe, the average energy consumption per capita has increased from 2.467 MWh in 1970 to 6.022 MWh in 2016 (IEA, 2016), and in the same period, global temperatures increased at a rate of 0.2°C per decade, compared to a hundredth of the rate for the centuries prior (NOAA, 2016). This jump can be attributed to the rampant utilization of fossil-fuels, and the harsh expansion of urbanization in modern society. It is no surprise that the manufacturing sector is responsible for 37% of all energy demand (IEA, 2018). Industrial processes are energy intensive, and 60–70% of industrial emissions are caused by industrial energy systems (IES) (EIA, 2021). IES provide utilities to industrial processes. Two of the most common typologies of existing IES are heat-only systems, and combined heat-and-power systems (EIA, 2021).
Concerns of increased carbon emission from industrial processing have forced the consideration of alternative technological concepts, i.e., fuel and technologies that are less carbonaceous that support a sustainable future. However, transition to alternative fuels and technologies remains stagnant, due to the high capital investment involved (Stančin et al., 2020). Alternative technological concepts for IES can be grouped into three broad concepts: advanced energy efficiency (through the reduction of energy demand from industrial processes, and maximizing the IES supply efficiency), fuel switching (to electricity, biogas, and hydrogen), and carbon capture and storage (CCS). Previous research in this area focused on evaluating the potential of these concepts singly in detailed models and integrated assessment models to show how each of these concepts can contribute toward a clean and sustainable process industry. The consensus is that these concepts are capital intensive, and policies are required to support uptake. However, any industrial site would require multiple concepts to have zero carbon emissions. Therefore, is there a hierarchy to how these concepts can be combined, and can this hierarchy further reduce their mitigation cost without the need for policies? This forms the hypothesis of the research in this paper. The focus of this paper is on decarbonizing existing IES and exploring how a hierarchical ordering of alternative technological concepts can further reduce carbon emissions and costs.
1.2. Literature review
In industrial energy systems (IES), combustion of fossil fuels to provide heat for steam generation, expansion, distribution, utilization, and recovery occurs to provide heat and power to several process on an industrial site. Two typical and common configurations within the energy intensive sector are: heat-only (Oluleye and Smith, 2016), and combined heat-and-power (CHP) systems (Sun et al., 2016) designed to provide high, medium, and low-pressure steam to several industrial processes.
Traditionally, IES have been designed with the sole purpose of minimizing cost (Chou and Shih, 1987). Nishio et al. (1980) developed a multi-objective optimization problem to maximize energy efficiency while also minimizing investment costs. In Papoulias and Grossmann (1983) an MILP model is developed and applied to design grassroot industrial energy system by building a superstructure. A robust mixed integer non-linear programming model is developed in Zhao and You (2019) and Tang et al. (2022) formulated an MINLP framework for grassroot design of IES and process unit. The optimization framework developed in Tang et al. (2022) reduced the system's total annualized cost by 11%. These models have been extensively adopted and enhanced for retrofitting IES for higher energy efficiency (Zhang et al., 2001; Martelli et al., 2017). Most existing IES are sub-optimal (Oluleye and Smith, 2016; Sun et al., 2016) therefore operational optimization techniques that minimize cost by adjusting the flow of heat and power without capital expenditure exist (Zhang et al., 2001). Oluleye and Smith (2016) applied operational optimization principles to adjust heat and power distribution in an industrial energy system. Halmschlager et al. (2022) observed a cost-effective switch to renewables made possible by a new combined MILP optimization approach. Operational optimization has also been proven to be effective in energy reductions, as employed by Chen et al. (2013) in the steel industry, and Sun et al. (2016, 2017) for industrial energy system producing heat and power. However, reduction in carbon dioxide emissions from operational optimization alone is not sufficient to transition to a sustainable and clean chemical process industry.
Energy efficiency has been explored as a cost-effective approach to minimize carbon dioxide emissions. Within the process industry energy efficiency is achieved by minimizing process demand for energy and maximizing the supply efficiency of the IES designed to meet process energy demand. Minimum demand for energy is possible through process integration techniques such as Pinch Analysis and Total Site Analysis, as shown by Zhang et al. (2001), and maximizing supply efficiency of IES is possible through waste heat recovery technologies, shown by Vescovo (2009) and Oluleye and Smith (2016). Process integration is a systematic method of analyzing energy usage in a plant following thermodynamic principles (Smith, 2017). Pinch Analysis and Total Site Analysis have been developed and applied to yield minimum energy demand from industrial processes (Linnhoff et al., 1982). In this technique, a process is split into cold and hot streams, where a pinch between these streams represents maximum heat recovery. The potential of minimizing energy demand by these approaches has been examined by Worrell and Galitsky (2004) and Tock et al. (2010). Minimizing energy demand has proven cost-effective by Zhang et al. (2001) through heat integration in a petroleum refinery, resulting in 16% energy demand reduction. However, once a process in pinched there is still residual heat sources below that are rejected to cooling water. Furthermore, existing IES still satisfy the minimum demand for energy using supply systems that are both inefficient and powered by fossil fuels. Given that these techniques are cost effective, it is necessary to examine whether including them in the integration of steeper carbon dioxide mitigation methods results in minimum mitigation cost, more specifically can the benefits from minimizing process demand for energy pay for steeper decarbonization measures?
Energy efficiency can also be achieved by maximizing the supply efficiency of IES. Supply efficiency refers to the performance of the industrial energy system, designed to satisfy the process demand for heat and power (Ivanov et al., 2021). In the EU, 70% of energy is employed in industrial processes, while 33% of this energy is wasted (Oluleye and Smith, 2016; Jouhara et al., 2018). This energy can be utilized to reduce energy cost and emissions. Even though demand may be minimized, there remains an ample amount of waste heat, resulting in < 65% efficiency (US Department of Energy, 2015). The energy supply system is made more efficient by recovering and utilizing waste heat. Vescovo (2009) examines the potential of waste heat recovery technologies in a cement manufacturing plant, satisfying ~20% of the site power demand. Johansson and Söderström (2013) assessed multiple waste heat utilization technologies and found that organic Rankine cycles (ORCs) achieved the highest efficiencies. Dunkelberg et al. (2018) and Philipp et al. (2018) examine the emissions savings potential of energy supply efficiency technologies. Improving supply efficiency has great cost-saving potential (Vescovo, 2009), but, like energy demand reduction, it does not necessarily ensure steep reduction in carbon emissions since the system may still rely on fossil-fuels.
The carbon emissions reduction potential of advanced energy efficiency measures is not sufficient to transition to a sustainable process industry if reliance is still on fossil-based energy. Hence the need to integrate alternative technologies and fuels. Fuel switching is a seemingly simple and effective alternative to carbonaceous fuels. Natural gas produces ~230 g of CO2 for every kWh generated, while biogas produces 24 g of CO2 for every kWh, nearly 10% the amount (EESI, 2017). The potential of emissions reductions with fuel switching is extraordinary; however, the cost of switching to an alternative fuel is not only dependent on the change in fuel price, but also a change in technology. An alternative fuel may require a different boiler with higher capital costs. This is also highly variable with location. Han et al. (2017) analyzed fuel switching potential for coal plants in China, concluding that electric boilers would be economically infeasible, costing five times as much. Kim (2022) compared the characteristics of IES under a switch to electrification with those under fossil-fuels and discovered heat-integrated design methods are effective for transforming to electricity, and noted that cost-effectiveness of electrified energy systems is highly influenced by heat recovery.
The long-term potential of industrial fuel switching in the UK was enviro-economically studied by Element Energy (2018). Hydrogen was found to have the highest potential in terms of reduced emissions by 2030; however, hydrogen as fuel faces immense economic challenges since infrastructure is still being put in place for a reliable hydrogen distribution network. Investments in Hydrogen are forecasted to surpass over $300 billion by 2030 to drive its price down and make it a feasible alternative (Mackey and Herbst, 2021). Biomass is expected to have huge potential, achieving ample emissions potential at a feasible and low cost. However, the fuel faces challenges with supply and applicability of the fuel depending on the industrial application involved (Element Energy, 2018). Amiri et al. (2021) devised a biomass-based cogeneration system to work in total site context but the economics is not considered. Rehfeldt et al. (2020) studied the potential of fuel switching in Germany to reach GHG targets in industry. González Palencia et al. (2013) analyzed the abatement potential of fuel substitution in the non-ferrous metal industry. The study concludes that reaching emissions reductions of ~50% is possible with fuel switching, but would result in a 20% increase in cost, an impractical jump compared to traditional fuels. Fuel switching may prove more economically feasible if a hierarchy of decarbonization concepts is applied to ensure that energy efficiency is maximized first, and fuel switching occur via optimization. The economic gains from process integration (energy demand reduction), and waste heat recovery (supply efficiency increase) may reduce the cost of switching to alternative fuels to achieve steeper reduction in carbon emissions.
Besides switching to alternative fuels, CCS plays a vital role in the transition to zero carbon emissions, especially in industries such as steel, where carbon emissions are unavoidable. It's expected that the technology will contribute ~17% of the reductions in emissions until 2050 (UNECE, 2021). Technological adoption remains a barrier due to its substantially high operating and capital costs, and energy loss of ~30% (Armstrong and Styring, 2015). Since CCS is retrofitted onto an existing energy system, post-combustion is the most suitable method. This strategy does, however, alter the operating parameters since CCS requires additional energy to operate. CCS potential and emissions abatement is highlighted by Khalilpour and Abbas (2011) by retrofitting post-combustion CCS on a coal-fired power plant with the purpose of CO2 abatement while mitigating energy expense. 90% of the carbon dioxide emissions was captured, with a 19.4% increase in energy demand. Khalilpour and Abbas (2011) examined the combination of heat integration with CCS. This reduced the 19.4% energy increase attributed to carbon capture, down to 15.9% through advanced energy efficiency.
There is little research on how combining these alternative technological concepts hierarchically can minimize cost and reduce carbon emissions further. Research up to date has focused on selecting between concepts or comparing concepts. For example, a study by An et al. (2018) evaluates different strategies in the Chinese iron and steel industry to assess the potential for energy and carbon emissions savings. To assess the potential of a strategy, a cost optimization model is built to select the most beneficial technological development path. The selected development path considers the emission reduction potential of a technology and the costs associated. Other aspects considered include the technology readiness level, political targets, and the forecasted evolution of the iron and steel sector, etc. The model aims first and foremost at minimizing the cost per year of the pathway chosen. This research will be the first to evaluate the combinatorial benefits of multiple options for achieving low-to-zero carbon IES. Previous research is burdened with comparing these options, neglecting the possible value from a hierarchical combination of these options. The alternative technological concepts for clean IES are minimizing demand for energy, maximizing IES supply efficiency, fuel switching and integrating CCS. They will be required in any process site or cluster to achieve low-to-zero carbon emission. In a case where minimizing energy demand and maximizing supply efficiency are both applied, in that order, the system first reaches its thermodynamic limit for heat recovery, followed by an assessment of waste heat available when the processes and site are pinched. If there remains a surplus, this is recovered, and used in the IES. Hierarchically combining decarbonization strategies can result in lower costs, yet research is directed toward systematic analysis of individual strategies. No other work has proposed an optimization model which considers the cost mitigation of a hierarchy of integrated decarbonization strategies for an IES. The scope is to support the transition of existing IES.
1.3. Novelty and contributions
To our knowledge, this paper is the first to offer a systematic analysis and propose a hierarchical combination of alternative technological concepts for decarbonizing industrial energy systems. The unique methodology combines detailed modeling of two typical existing industrial energy systems in a software and a novel MILP mathematical optimization framework designed to build a superstructure of 48 combinations of alternative concepts which is reduced subject to cost. The differentiated approach of this work allows the identification of unique integration methods to reduce cost of carbon abatement strategies in existing industrial energy systems. Additionally, this study at a micro scale provides a concrete example of abatement strategy implementation for industry decisions makers. There exists a lack of models showing how decarbonization concepts can be combined to achieve reductions in CO2e emissions and mitigation cost. There is also a gap in research for comparing cost mitigation of different decarbonization strategies for the two typical typologies of IES. This study develops and applies a systematic novel mixed integer linear problem (MILP) model which serves to evaluate these decarbonization strategies and presents a hierarchy which achieves lower mitigation cost. Asides the novel methodology, this outcome can provide novel insights on how to integrate new technologies and fuel in process sites.
2. Methodology
2.1. Overview
A mixed method approach combining software simulation and mathematical optimization is applied in this work. First detailed simulation of the system is done in STAR software (University of Manchester, 2021) to confirm the material and energy and obtain the resultant emission and cost for the business-as-usual system, which is then used as input into the optimization framework. The use of simulation software is common (Ren et al., 2018). STAR modeling allows the easy design of energy systems involving steam and power generation. Once the initial system configuration is specified, and the input data (mainly for technologies and fuels) and overall operating conditions provided, the software calculates optimal flowrates, costs and emissions. A novel mixed integer linear model is developed for the system under consideration, resulting in the creation of a superstructure for each alternative concept. The methodology is applied to two case studies of existing industrial energy systems. The first case study of a heat only IES from Oluleye and Smith (2016), and the second case study for a combined heat and power system from Sun et al. (2016) thereby extending their work to integrate steeper carbon emissions reduction technologies.
2.2. Description of alternative technological concepts
The concepts considered as: operational optimization, Fuel Switching (natural gas, blue hydrogen, biogas, and electricity), Advanced Energy Efficiency (both reducing demand for energy and increasing IES supply efficiency), and Carbon Capture and Storage. In operational optimization the total cost is minimized by changing the flow of heat and power within the business as usual non optimal system to reduce fuel consumption. All other parameters are kept constant, and reduction in fuel utilization would result in a reduction in CO2 emissions. Steam flows are optimized to minimize operating cost while process heat recovery and steam flows to process are fixed. Since most existing industrial energy systems are suboptimal, operational optimization is required. The concept behind reducing energy demand is based on Process integration. Supply efficiency is increased by exploiting waste heat from the site processes and energy system using Organic Rankine Cycles (ORC). ORCs utilize waste heat by pumping an organic fluid in an evaporator, allowing the vapor to expand, and utilizing it as power. Details of this for the heat only system are provided in Oluleye and Smith (2016).
The steeper measures for reducing emissions are fuel switching (to natural gas as some systems are still not using natural gas, blue hydrogen, biogas, and electrification of heat) and integrating CCS. By extending the work of Oluleye and Smith (2016) and Sun et al. (2016) steeper measures are included in a bottom-up detailed model of industrial energy systems, and the benefits of hierarchical ordering of these alternative concepts can be explored. The forecasted prices and life cycle CO2 equivalent emissions for each fuel is given in Appendix Table B1 in Supplementary material. All the technologies considered in the system are also provided in Appendix Tables B2–B8 in Supplementary material. Emissions are obtained through calculations given in Section 2.3 while prices were obtained from BEIS (2020) and Henbest (2021). These serve as input into the optimization model. Integrating CCS raises two requirements: heat input and power input. Excess power required for CCS is calculated using Eq. (1), where excess power is assumed to keep flows and operating parameters constant. This is dependent on a capture rate “CR” obtained from TNO (2020) and a power input factor “PIF” which is the power needed per kilogram of emitted CO2e.
The heat input for CCS is also calculated as an increase in heat requirement would translate to fuel demand, thereby further increasing CO2e emissions, and requiring CCS to capture more CO2e. This is solved using a convergence loop:
Calculate annual CO2e emissions:
Calculate captured CO2e emissions using the capture rate “CR”:
Calculate the required heat input to capture CO2 using the heat input factor “HIF”:
The heat load of the CCS is then adjusted to the value obtained for “Hinput” which is typically LP steam (TNO, 2020). This process is repeated until convergence is reached. This feeds into the optimization model described in Section 2.3.
2.3. The MILP optimization framework
The optimization framework was developed to minimize the total cost of integrating the alternative concepts singly and in several pre-described hierarchies. The alternative concepts and pre-defined hierarchies create a superstructure which is reduced subject to the objective. The superstructure is made up of 48 solutions. In any industrial energy system 7 broad solutions outside the business-as-usual system can be explored to reduce emissions. They include performing operational optimization, reducing energy demand from the connected site processes, focusing on increasing efficiency of the industrial energy system without changing process demand for heating or a combination of these. The 7 broad solutions plus the business as usual system are: (1) BAU-NonOpt: The business-as-usual (BAU) non-optimal energy system, (2) S-Opt: Solution created from operational optimization of the BAU system, (3) S-EnDr: Solution created from only energy demand reduction from site processes, (4) S-SupEf: Solution created from IES supply efficiency increase, (5) S-Opt+ S-EnDr: Solution created from energy demand reduction and simultaneous optimization of the IES, (6) S-Opt+ S-SupEf: Solution created from supply efficiency increase and simultaneous optimization of the IES, (7) S-Opt+ S-EnDr+ S-SupEf: Solution created from first minimizing energy demand reduction via direct heat recovery before maximizing supply efficiency increase and simultaneous optimization of the IES, (8) S-Opt+ S-SupEf+ S-EnDr: Solution created from first maximizing supply efficiency increase before minimizing energy demand reduction via direct heat recovery and simultaneous optimization of the IES. The fuel used in each of these 8 cases can be changed for a lower carbon solution. Based on this, the 48 solutions in the superstructure are: 8 solutions around the business as usual energy system using the incumbent fuel building on the 8 options, 8 solutions for switching to Natural gas (an alternative fuel), 8 solutions for switching to blue hydrogen, 8 solutions for switching to biogas, 8 solutions for electrification of heat, and 8 solutions for integrating CCS all building on options. All 48 solutions are provided in Appendix Table A1 in Supplementary material.
The model can select any of these solutions to satisfy the objective function in Eq. (5).
The total annualized cost is the sum of the capital cost of all technologies in each solution multiplied by a binary variable and operating cost (both fixed and variable):
Where CCAPEX is to the total capital cost, and COPEX is the total operating cost for solution s, AF is the annualization factor, and captech the unit capital cost for each technology
A binary variable Y, is included for selection [Eq. (7)], and the annualization factor is calculated in Eq. (8):
Both the fixed [Eq. (10)] and variable [Eq. (11)] operating costs are accounted for:
The cost of fuel (Cfuel), electricity import (Cimport), and export (Cexport) are calculated using Eqs. (12)–(14), respectively:
Where Prfuel, Primp and Prexport are the unit prices of fuel, electricity import and export, top is the total operating hours in a year, Qf is the quantity of fuel consumed, Pimp and Pexport the quantity of power imported and exported.
The carbon dioxide emission calculations are provided below: fuel data is necessary to calculate each fuel's energy cost and carbon emissions. Yearly emissions were expressed in tons of CO2eq emitted per year. To do so, emission factors for scopes 1 and 3, given in kgCO2eq/kWh by the BEIS (2020), were added up. Fuel and power emissions are calculated using Eqs. (15)–(21):
First the electric power weight is calculated: Power weight refers to the proportion of power generated, to total heat and power generated.
Where W is the power weight in Eq. (5), Pgen is the power generated on site, and Hgen is the heat generated.
The power emissions factor (εpower)refers to the emissions because of combustion, per kWh of heat and power generated (over the whole operation), and Emcomb is the carbon dioxide emissions from fuel consumption.
Surplus emissions (Emsur) are dependent on the factor obtained above, as well as the total power generated and power weight %.
Finally, the emissions avoided (Emavo) from selling to the grid is merely the difference between imported power emissions and surplus emissions (Emsur). Where εgrid is the grid emission factor.
The emissions from importing power from the grid (Emimp) depend on the grid's efficiency and emissions factor. This is variable depending on infrastructure and location.
Fuel emissions are caused by direct combustion, as well as indirect causes from the life cycle of the fuel.
When a fuel is combusted, it releases energy but also carbon emissions. This is dependent on the carbon content of the fuel (CC), as well as the molecular weight. These values are obtained from literature and emissions are calculated as shown below:
Fuel emissions (Emf) over a life cycle consider indirect upstream and direct emissions. These are classified in three scopes by the GHG (2011) protocol. Scope 1 consists of direct emissions, Scope 2 is a result of purchased utilities, and Scope 3 is all other indirect emissions such as transport and distribution (University of Manchester, 2021). Emissions factors “ε” for Scopes 1 and 3 for each fuel are obtained from McKinsey and Company (2022) and summed. The equation for calculating annual life-cycle emissions is shown as Eq. (21).
The model was built in spreadsheet software and solved using Lindo's system What's Best! (2021). Software. The problem is solved by first providing a continuous approximation of the model, and then applying the branch-and-bound algorithm in What's Best! to identify all possible integer solutions and find an optimal integer solution.
3. Case study
3.1. Industrial energy system under study
Two typical and common configurations of Industrial Energy Systems (IES) are used for case study in this work. A heat only systems in Figure 1A and a Combined Heat and Power (CHP) system in Figure 2. In the heat only system, heat recovery within and between processes is not maximized, whilst in the combined heat and power system heat recovery within and between processes is maximized. This allows to test the hypothesis on a highly efficient system to make the insights more useful. The Total Site Profile for the heat only system is also provided in Figure 1B (Oluleye and Smith, 2016). This work extends past work on process integration plus waste heat utilization, and utility system optimization to integrate steeper decarbonization measures and show whether benefits from process integration, i.e., energy efficiency first can reduce the cost/pay for these steeper measures.
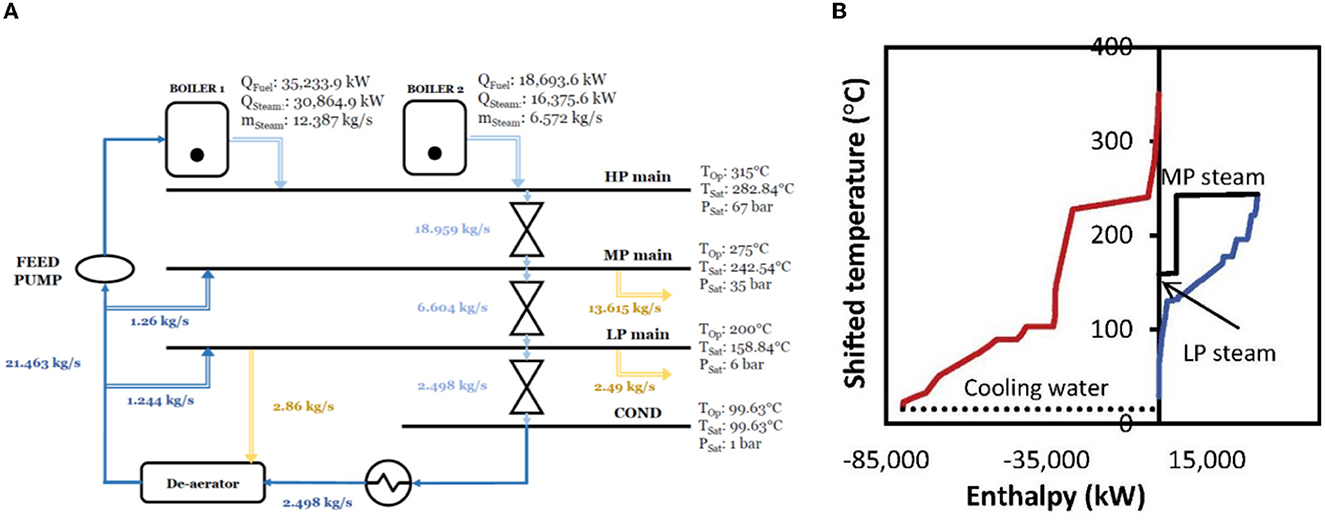
Figure 1. (A) Schematic of “Heat only” IES case study—BAU-NonOpt and (B) process energy demand depicted by the existing Total Site Profile (Oluleye and Smith, 2016).
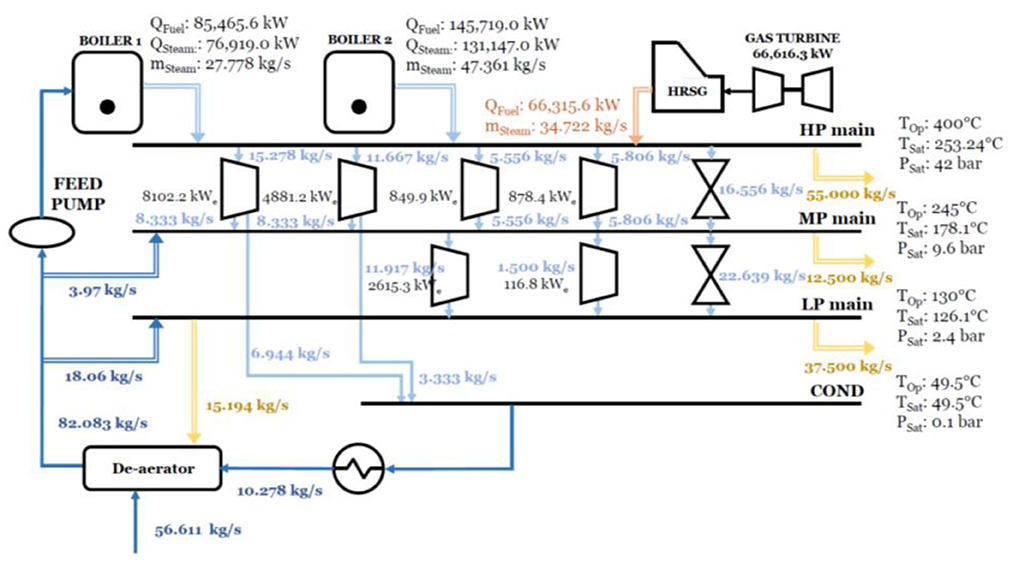
Figure 2. Schematic of “Combined heat and power” IES system for case study—BAU-NonOpt (Sun et al., 2016).
The heat only system consists of a fuel gas and fuel oil boiler, the system imports 10 MW of electrical power and operates for 8,600 h a year. The CHP systems consists of two natural gas fired boilers, a gas turbine, and a heat recovery steam generator. The site requires 30 MW of electrical power and operates for 8,600 h a year.
3.2. Case study results and discussion
The systems in Figures 1A, 2 were modeled in STAR software (University of Manchester, 2021) to determine the energy balance which is also shown in the Figures, input for alternative fuels and technologies for the 48 solutions are provided in the Appendix Tables B1–B8 in Supplementary material. After modeling these systems in STAR software to check the energy balances, the optimization framework in Section 2.3 is applied to create a superstructure of the 48 solutions formed by integrating the alternative technological concepts singly or in combined mode. Results are divided into two categories for the heat only system in Section 3.2.1, and the CHP system in Section 3.2.2.
3.2.1. Heat only industrial energy system
Satisfying the process energy demand using the heat only business as usual system in Figure 1A with import of electricity releases 169,556 t/y of carbon emissions to the atmosphere. Therefore, making the system cleaner would require alternative technologies and fuels. A no regret way of reducing emissions is via operational optimization especially since the existing system is sub-optimal. Performing operational optimization on the system requires no new capital investment (Appendix Figure C1 in Supplementary material), and this reduces fuel consumption by 28.3%, resulting in a 26% reduction in carbon emissions, and a 24.7% reduction in total operating costs. The carbon emissions can be further reduced by minimizing process energy demand via heat recovery into the IES (Appendix Figure C2 in Supplementary material). This is possible by exploiting high quality heat rejected to cooling water in Figure 1B—about 14.378 kg/s of low-pressure steam is generated (Appendix Figure C2 in Supplementary material). By minimizing energy demand, fuel consumption reduces to 20.574 MW resulting in a 54% decrease in carbon emissions, the total annualized cost also reduces by 49%—this is possible since direct heat recovery is economically viable through the IES. If the IES is optimized simultaneously whilst reducing energy demand (Appendix Figure C3 in Supplementary material) a 4.4% additional reduction in carbon emissions is possible compared to the design without optimization.
There is potential to increase the efficiency of the IES by integrating ORC to generate electrical power from the condenser and process, a total of 9.2MW of electrical power is possible (Appendix Figure C4 in Supplementary material) (Oluleye and Smith, 2016). The resultant effect is a reduction in carbon emissions by 13.8% and total costs by 13.16% compared to the business as usual non-optimal system. A solution created by maximizing IES supply efficiency before energy demand reduction with optimization of the IES is done in Appendix Figure C5 in Supplementary material. In this case, electricity generation is prioritized over direct heat recovery. This reduces carbon emissions by 37.7% and cost by 37.2% with respect to the BAU non-optimal heat only system. The last hierarchy which encourages direct use of heat first before conversion to other forms of energy (Appendix Figure C6 in Supplementary material) reduces carbon emissions the most by 60.9% and cost by 56% with respect to the business as usual non-optimal system (Figure 1A).
Steeper emission reduction is possible with CCS integration (Appendix Figure C7 in Supplementary material). When CCS is fully integrated such that surplus LP steam from the IES is used further cost reduction of 37% is possible compared to a partially integrated CCS. The cost of CCS integration can be further reduced by a hierarchy that also allows energy demand from the site processes to be minimized via direct recovery into the IES (Appendix Figure C8 in Supplementary material). If the supply efficiency of an existing IES is optimized first, cost of CCS integration reduces by 43.8% (schematic shown in Appendix Figure C9 in Supplementary material). A hierarchy formed by using heat directly first, then converting waste heat to power (Appendix Figure C10 in Supplementary material) has potential to reduce CCS cost by 56% compared to partially integrating CCS. CCS integration alone costs 69.6 million £/y, this reduces to 30.7 million £/y when the technology is optimally and fully integrated with a hierarchy of advanced energy efficiency options first.
The annualized cost and total carbon emissions from the solutions created is shown in Figures 3–9. The business-as-usual system lowest reduction in cost (reduces by 55.7%) and carbon emissions (reduces by 61%) are from a hierarchy of concepts where process demand for energy is minimized via direct heat recovery before the IES supply efficiency in increased via energy conversion aligning with the fundamentals of thermodynamics (Figure 3). Switching to natural gas also observes the same pattern—lowest cost with 45.6% reduction, and carbon emissions reduction by 55.9% possible from the hierarchy (Figure 4). If fuel switching to blue hydrogen is considered, the hierarchy reduces cost by 48% and carbon emissions by 59.5% compared to no hierarchy (Figure 5). The same is observed for biogas in Figure 6—where cost reduction of 48.7% and carbon emissions of 62% is possible with the hierarchy. For a system using electric boilers (Figure 7) the hierarchy reduces cost by 48.7% and carbon emission by 55.9%. The benefits follow the same trend for CCS integration (Figure 8)—costs reduce by 55.9% and carbon emission by 72.8%.
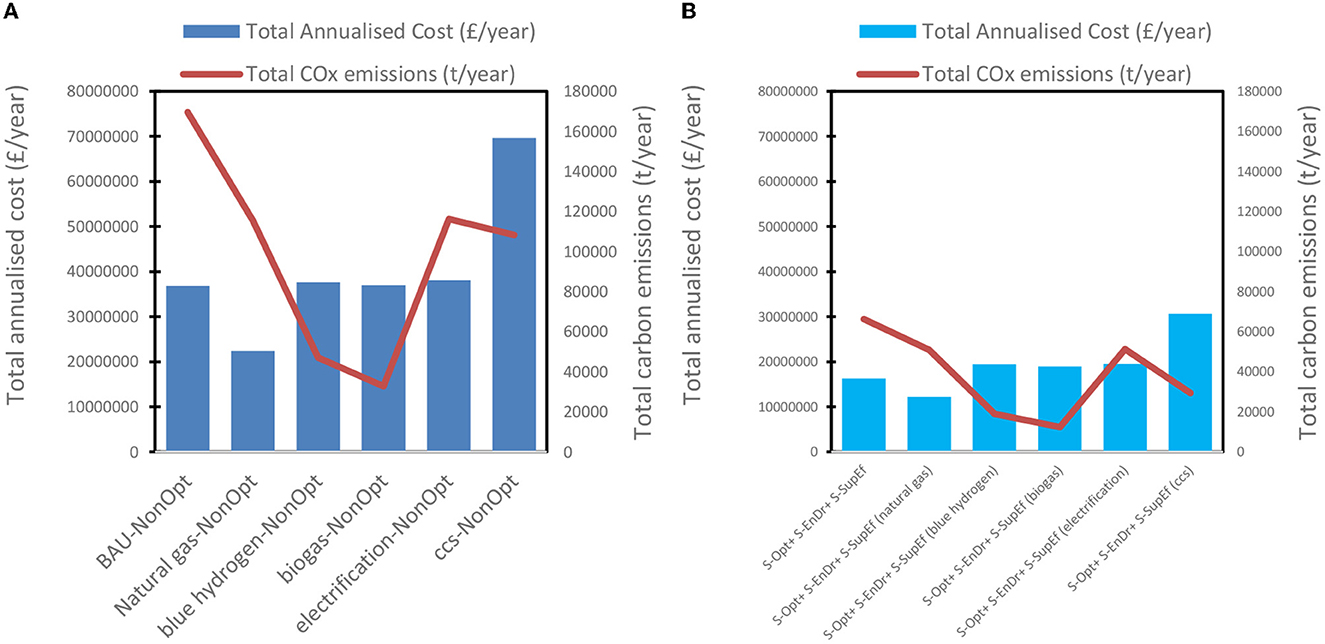
Figure 9. (A) “Heat only” concepts result without hierarchy. (B) “Heat only” concepts result for best hierarchy.
Overall, alternative technological concepts are more expensive than the business as usual non-optimal system (Figure 9A) except for a switch to natural gas. Reducing their mitigation cost is possible with the hierarchy, i.e., maximizing the performance of the existing system simultaneously with a switch to alternatives. Both cost and carbon emissions reduce (Figure 9B).
As most existing IES in process sites or clusters are still heat only systems it is necessary to transition to low carbon cost-effectively. The analysis in this work has determined a strategy that aligns with hierarchical ordering of the concepts to form solutions that reduce cost of steeper measures by at least 40% and further reduces carbon emissions. For example, CCS partial integration increases cost by 32.8 million £/y but reduces carbon emissions by 61,313 t/y. CCS fully integrated using the hierarchy yields −6.13 million £/y savings and further reduces carbon emissions by 140,188 t/y with respect to the business as usual non-optimal design.
3.2.2. Combined heat and power industrial energy system
The business-as-usual (BAU) CHP system is provided in Figure 2. The system consumes natural gas and produces electricity using a gas turbine and steam expansion via six steam turbines. The business-as-usual systems costs 76.86 million £/y to operate and emits 718,207 t/y of carbon emissions. Therefore, there is need to decarbonize the system. For this system, the processing units are already at maximum for heat recovery. Therefore, the options are operational optimization, fuel switching to blue hydrogen, biogas and electricity, and CCS integration. Operational optimization schematic is shown in Appendix Figure D1 in Supplementary material, natural gas consumption reduces to 147.855 MW fuel energy and no heat is wasted from the condenser—implying that the system maximizes the production of electricity from steam expansion. Operational optimization of the system reduces carbon emissions by 19.7% and costs by 17.8% with no capital investment. Integrating CCS partially reduces emissions by 76% and costs increase by 59.15 million £/y with respect to the BAU system. An optimized and fully integrated CCS (Appendix Figure D2 in Supplementary material) reduces emissions by 86% and cost increase by 8.63 million £/y with respect to the BAU system.
Optimizing the business as usual system reduces costs and emissions (Figure 10), the same is observed for fuel switch to blue hydrogen (Figure 11), fuel switch to biogas (Figure 12), and electrification via the use of electric boilers (Figure 13). CCS integration also follows the same trend (Figure 14). Alternative technological concepts integrated into the CHP systems increases cost and generally reduces emissions except for electrification, since the electricity isn't from a 100% renewable grid (Figure 15A), a way to reduce mitigation cost is to integrate these alternatives optimally and hierarchically (Figure 15B). For CCS integration cost reduces by 37.14% and emissions captured increases by 40.5% with these hierarchies. Therefore, a cost effective clean industrial energy system is possible with an optimized hierarchical ordering of alternative technological concepts.
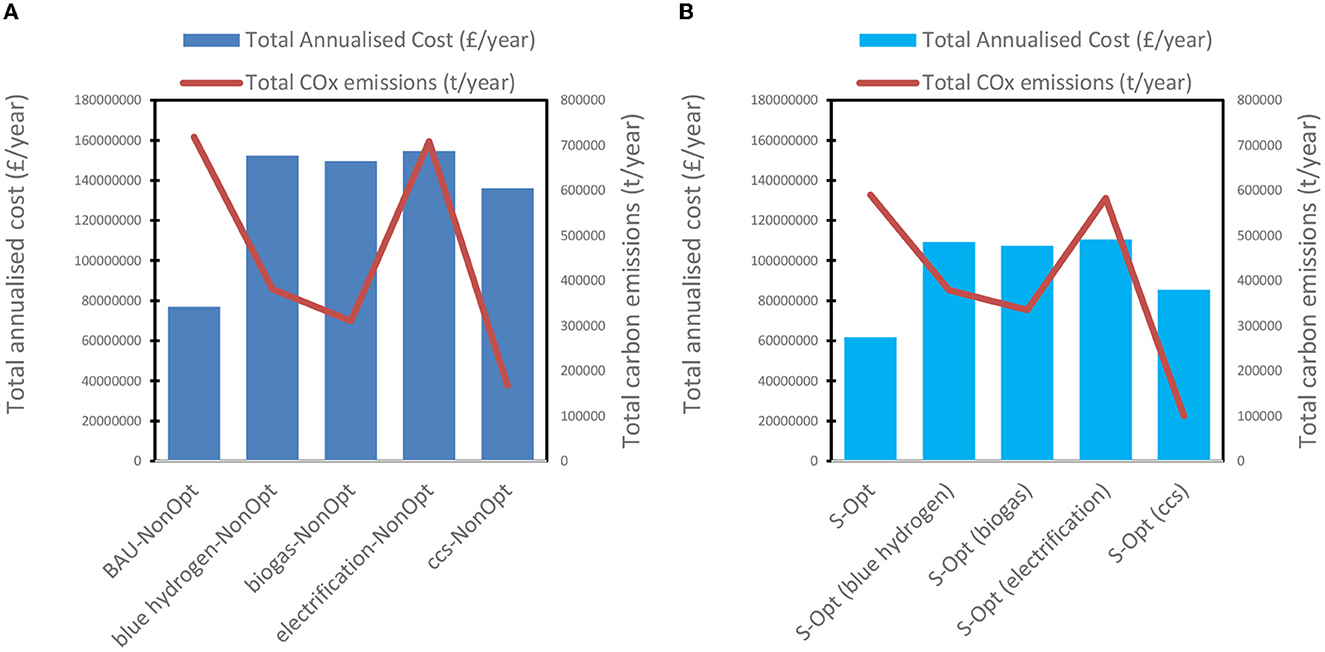
Figure 15. (A) “CHP system” concepts result without hierarchy. (B) “CHP system” concepts result for best hierarchy.
The main hypothesis of this work is cost effective clean industrial energy systems is possible with a hierarchical ordering of alternative technological concepts. The objective was to determine the hierarchy required to minimize mitigation cost associated with clean industrial energy systems. From the results and analysis, a hierarchy results in lower mitigation cost as shown in Sections 3.2.1 and 3.2.2 and summarized in the graphical abstract. The graphical abstract can be interpreted as: before the integration of alternative fuels, technologies and CCS into existing industrial energy systems there is need to reduce demand for heat from the site processes using Process Integration techniques (Pinch Analysis and Total Site Analysis), then ensure the supply system is efficiency by waste heat utilization. By following the hierarchy, the cost of steeper decarbonization measures can be reduced by at least 30%.
3.3. Wider implication of findings
The methodology and case study presented in this paper extends past work on process integration plus waste heat utilization, and utility system optimization to integrate steeper decarbonization measures and show whether benefits from process integration, i.e., energy efficiency first can reduce the cost/pay for these steeper measures. Results show that maximizing energy efficiency in existing industrial energy systems can reduce the cost of steeper decarbonization measures for heat only, and combined heat and power systems by at least 30%. A summary of the hierarchy of alternative technology concepts to minimize the cost of CO2 reduction in industrial energy systems in shown in the graphical abstract. Optimal solutions created from minimizing energy demand reduction via direct heat recovery, maximizing energy supply efficiency through waste heat recovery should be done before integrating alternative fuels and technologies to achieve steeper reduction in CO2 emissions cost effectively.
4. Conclusions
At least 45% of industrial carbon emissions are caused by combustion of fuels in industrial energy systems (IES). Satisfying the needs of the Paris agreement and transitioning to a sustainable process industry would require integration of alternative technological concepts. Such concepts can be grouped into advanced energy efficiency (to account for reducing demand from process, and increasing IES energy supply efficiency), switching to alternative fuels and CCS integration. Uptake of these concepts are low due to the high capital investment. Hence there is need to reduce cost. This paper is based on a hypothesis that hierarchical ordering of concepts that form solutions where heat recovery is maximized first can result in lower cost showing that the benefits of process integration can pay for steeper decarbonization concepts. To this end a mixed method approach is applied that combines detailed modeling of the IES in a software plus a novel mixed integer linear optimization framework that creates a superstructure of 48 solutions from single and combined concepts. The methodology is applied to two of the most common IES typologies—heat only, and combined heat and power systems. The case study also represents the IES of a total site that is pinched and one of a total site where heat recovery between and within process has not been maximized to add more value to the findings. The heat only system emits 169,556 t/y and the CHP system 718,207 t/y of carbon emissions into the atmosphere. Results show that for the heat only system a hierarchy of operational optimization, energy demand reduction, and supply efficiency increase yields the highest reduction in carbon emissions (by 60.9%) and the reduction in cost (55.7%). When an alternative fuel like blue hydrogen is integrated cost increases by 2.2% and emissions reduce by 72.23%. However, if the BAU system is optimized and energy efficiency maximized first, switching to blue hydrogen costs 47.2% less. Partially Integrating CCS in the CHP system increases cost by 77% and reduces emissions by 76% however, full integration with the hierarchy reduces CCS cost by 37%. The findings from this work shows how to decarbonize energy provision in the industrial sector at minimum cost. At least 30% reduction in mitigation cost is achieved using the hierarchy. Applying the knowledge of hierarchy to implementing steeper measures for reducing carbon emissions in industrial energy systems is a practical significance of the findings.
This research shows the economic benefits of energy efficiency first and optimal hierarchical integration of alternatives. Future work will include other system configurations like trigeneration and polygeneration systems, and extend the framework to account for uncertainties in inputs by using a robust optimization framework. A promising area of future work is to apply the methodology on a macro-level to several industrial energy systems in a country or region to show how industrial decarbonization can be achieved cost-effectively. This result can feed into policy creation for transitioning existing industrial energy systems into cleaner ones by first maximizing energy efficiency first.
Data availability statement
The original contributions presented in the study are included in the article/Supplementary material, further inquiries can be directed to the corresponding author.
Author contributions
GO: conceptualization, data curation, investigation, methodology, formal analysis, resources, software, visualization, validation, writing, and supervision—original draft. DB: formal analysis, software, case study development, validation, and writing—review and editing. BK: software analysis, case study development, conceptualization, resources, validation, and writing—review and editing. All authors contributed to the article and approved the submitted version.
Acknowledgments
The authors would like to appreciate the reviewers for the value added.
Conflict of interest
The authors declare that the research was conducted in the absence of any commercial or financial relationships that could be construed as a potential conflict of interest.
Publisher's note
All claims expressed in this article are solely those of the authors and do not necessarily represent those of their affiliated organizations, or those of the publisher, the editors and the reviewers. Any product that may be evaluated in this article, or claim that may be made by its manufacturer, is not guaranteed or endorsed by the publisher.
Supplementary material
The Supplementary Material for this article can be found online at: https://www.frontiersin.org/articles/10.3389/frsus.2023.1057064/full#supplementary-material
References
Amiri, H., Sotoodeh, A., and Amidpour, M. (2021). A new combined heating and power system driven by biomass for total-site utility applications. Renew. Energy 163, 1138–1152. doi: 10.1016/j.renene.2020.09.039
An, R., Yu, B., Li, R., and Wei, Y.-M. (2018). Potential of energy savings and CO2 emission reduction in China's iron and steel industry. Appl. Energy 226, 862–880. doi: 10.1016/j.apenergy.2018.06.044
Armstrong, K., and Styring, P. (2015). Assessing the potential of utilization and storage strategies for post-combustion CO2 emissions reduction. Front. Energy Res. 3, e8. doi: 10.3389/fenrg.2015.00008
BEIS (2020). Final UK Greenhouse Gas Emissions National Statistics: 1990 to 2017. Available online at: https://www.gov.uk/government/statistics/final-uk-greenhouse-gas-emissions-nationalstatistics-1990-to-2018 (accessed February 3, 2022).
Chen, C., Lin, C., and Lee, J. (2013). Retrofit of steam power plants in a petroleum refinery. Appl. Thermal Eng. 61, 7–16. doi: 10.1016/j.applthermaleng.2013.04.001
Chou, Y., and Shih, X. (1987). Thermodynamic approach to the design and synthesis of plant utility system. Ind. Eng. Chem. Res. 26, 1100–1108. doi: 10.1021/ie00066a009
Dunkelberg, H., Wagner, J., Hannen, C., Schlüter, B. A., Phan, L., Hesselbach, J., et al. (2018). Optimization of the energy supply in the plastics industry to reduce the primary energy demand. J. Clean. Prod. 192, 790–800. doi: 10.1016/j.jclepro.2018.04.254
EIA (2021). Use of Energy Explained—Energy Use in Industry. Available online at: at: https://www.eia.gov/energyexplained/use-of-energy/industry.php (accessed March 5, 2022).
Element Energy (2018). Industrial Fuel Switching Market Engagement Study. Cambridge: Element Energy Limited.
GHG (2011). Corporate Value Chain (Scope 3) Accounting and Reporting Standard. World Resources Institute. Available online at: https://ghgprotocol.org/sites/default/files/standards/Corporate-Value-Chain-Accounting-Reporing-Standard_041613_2.pdf (accessed March 4, 2022).
González Palencia, J. C., Furubayashi, T., and Nakata, T. (2013). Analysis of CO2 emissions reduction potential in secondary production and semi-fabrication of non-ferrous metals. Energy Policy 52, 328–341. doi: 10.1016/j.enpol.2012.09.038
Halmschlager, D., Beck, A., Knöttner, S., Koller, M., and Hofmann, R. (2022). Combined optimization for retrofitting of heat recovery and thermal energy supply in industrial systems. Appl. Energy 305, 117820. doi: 10.1016/j.apenergy.2021.117820
Han, Y., Shen, B., and Zhang, T. (2017). A techno-economic assessment of fuel switching options of addressing environmental challenges of coal-fired industrial boilers: an analytical work for China. Energy Proc. 142, 3083–3087. doi: 10.1016/j.egypro.2017.12.448
Henbest (2021). New Energy Outlook 2021. BloombergNEF. Available online at: https://about.bnef.com/new-energy-outlook/ (accessed February 13, 2022).
IEA (2016). European Union Electric Power Capacity. Available online at: https://data.worldbank.org/indicator/EG.USE.ELEC.KH.PC?locations=EU (accessed March 14, 2022).
IEA (2018). Clean and Efficient Heat for Industries. Available online at: https://www.iea.org/commentaries/clean-and-efficient-heat-for-industry (accessed April 16, 2022).
Ivanov, K., Georgieva, N., Tasheva, S., and Gandova, V. (2021). Analysis of energy efficiency of an industrial system. IOP Conf. Ser. Mater. Sci. Eng. 1031, 012080. doi: 10.1088/1757-899X/1031/1/012080
Johansson, M., and Söderström, M. (2013). Electricity generation from low-temperature industrial excess heat-an opportunity for the steel industry. Energ. Eff. 7, 203–215. doi: 10.1007/s12053-013-9218-6
Jouhara, H., Khordehgah, N., Almahmoud, S., Delpech, B., Chauhan, A., and Tassou, S. (2018). Waste heat recovery technologies and applications. Therm. Sci. Eng. Prog. 6, 268–289. doi: 10.1016/j.tsep.2018.04.017
Khalilpour, K., and Abbas, A. (2011). HEN optimization for efficient retrofitting of coal-fired power plants with post-combustion carbon capture. Int. J. Greenh. Gas Conf. 5, 189–199. doi: 10.1016/j.ijggc.2010.10.006
Kim, J. (2022). Studies on the conceptual design of energy recovery and utility systems for electrified chemical processes. Renew. Sustain. Energy Rev. 167, 112718. doi: 10.1016/j.rser.2022.112718
Linnhoff, B., Townsend, D. W., Boland, D., Hewitt, G. F., Thomas, B. E. A., Guy, A. R., et al. (1982). User Guide on Process Integration for the Efficient Use of Energy, 1st edition. Rugby: IChemE. Revised 1st edition 1994
Mackey, P., and Herbst, S. (2021). Time to Accelerate the >$300bn Hydrogen Project Pipeline. H2 View. Available online at: https://www.h2-view.com/story/time-to-accelerate-the-300bn-hydrogen-project-pipeline/ (accessed June 5, 2022).
Martelli, E., Elsido, C., Mian, A., and Marechal, F. (2017). MINLP model and two-stage algorithm for the simultaneous synthesis of heat exchanger networks, utility systems and heat recovery cycles. Comput. Chem. Eng. 106, 663–689. doi: 10.1016/j.compchemeng.2017.01.043
McKinsey Company (2022). Global Energy Perspective 2022. Available online at: https://www.mckinsey.com/~/media/McKinsey/Industries/Oil%20and%20Gas/Our%20Insights/Global%20Energy%20Perspective%202022/Global-Energy-Perspective-2022-Executive-Summary.pdf (accessed July 16, 2022).
Nishio, J., Itoh, T., and Umeda, A. (1980). A thermodynamic approach to steam-power system design. Ind. Eng. Chem. Proc. DD 19, 306. doi: 10.1021/i260074a019
NOAA (2016). Global Climate Report 2022. Available online at: https://www.ncei.noaa.gov/access/monitoring/monthly-report/global/202201 (accessed August 1, 2022).
Oluleye, G., and Smith, R. (2016). A mixed integer linear programming model for integrating thermodynamic cycles for waste heat exploitation in process sites. Appl. Energy Issue 178, 434–453. doi: 10.1016/j.apenergy.2016.06.096
Papoulias, S. A., and Grossmann, I. E. (1983). A structural optimization approach in process synthesis-II heat recovery networks. Comput. Chem. Eng. 7, 707–721. doi: 10.1016/0098-1354(83)85023-6
Philipp, M., Schumm, G., Peesel, R. H., Walmsley, T. G., Atkins, M. J., Schlosser, F., et al. (2018). Optimal energy supply structures for industrial food processing sites in different countries considering energy transitions. Energy 46, 112–123. doi: 10.1016/j.energy.2017.05.062
Rehfeldt, M., Fleiter, T., Herbst, A., and Eidelloth, S. (2020). Fuel switching as an option for medium-term emission reduction—a model-based analysis of reactions to price signals and regulatory action in German industry. Energy Policy 2020, 111889. doi: 10.1016/j.enpol.2020.111889
Ren, X., Jia, X., Varbanov, P., Klemeš, J., and Liu, Z. (2018). Targeting the cogeneration potential for Total Site utility systems. J. Clean. Prod. 170, 625–635. doi: 10.1016/j.jclepro.2017.09.170
Smith, R. (2017). Process integration: current status and future challenges. Comput. Aided Chem. Eng. 40, 9780444639653. doi: 10.1016/B978-0-444-63965-3.50003-9
Stančin, H., Mikulcic, H., Wang, X., and Duic, N. (2020). A review on alternative fuels in future energy system. Renew. Sustain. Energy Rev. 128, 109927. doi: 10.1016/j.rser.2020.109927
Sun, L., Doyle, S., and Smith, R. (2016). Understanding steam costs for energy conservation projects. Appl. Energy 161, 647–655. doi: 10.1016/j.apenergy.2015.09.046
Sun, L., Gai, L., and Smith, R. (2017). Site utility system optimization with operation adjustment under uncertainty. Appl. Energy 186, 450–456. doi: 10.1016/j.apenergy.2016.05.036
Tang, Q., Zhang, W., Hu, J., He, C., Chen, Q., and Zhang, B. (2022). Design optimization of industrial energy systems with energy consumption relaxation models for coupling process units and utility streams. J. Clean. Prod. 344, 131072. doi: 10.1016/j.jclepro.2022.131072
TNO (2020). Post-Combustion CO2 Capture Add-on for Power Plants—Gaseous Fuels. Available online at: https://energy.nl/en/fact_sheet/post-combustion-co2-capture-add-on-for-power-plantsgaseous-fuels/ (accessed August 15, 2022).
Tock, L., Maréchal, F., Metzger, C., and Arpentinier, P. (2010). “Process integration analysis of an industrial hydrogen production process,” in 18th World Hydrogen Energy Conference 2010. Proceedings.
UNECE (2021). Technology Brief on Carbon Capture, Use and Storage (CCUS). Geneva: United Nations Economic Commission for Europe.
University of Manchester (2021). Centre for Process Integration STAR: Utility Systems. Available online at: http://epsassets.manchester.ac.uk/medialand/cpi/vol1/software/setup_star_v2_9.zip; https://www.ceas.manchester.ac.uk/cpi/research/resources/software/ (accessed August 14, 2022).
US Department of Energy (2015). “Innovating clean energy technologies in advanced manufacturing—process heating,” in Quadrennial Technology Review (Washington DC: US Department of Energy).
Vescovo, R. (2009). ORC recovering industrial heat: power generation from waste energy streams. Cogeneration On-site Power Prod. 10, 53–57.
Worrell, E., and Galitsky, C. (2004). Energy Efficiency Improvement Opportunities for Cement Making—An ENERGY STAR Guide for Energy and Plant Managers. Berkeley, CA: Lawrence Berkeley National Laboratory (LBNL54036).
Zhang, B., Wu, S., and Chen, Q. (2001). An optimization procedure for retrofitting process energy systems in refineries. Comput. Aided Chem. Eng. 2012, 1005–1009. doi: 10.1016/B978-0-444-59506-5.50032-8
Zhao, L., and You, F. (2019). A data-driven approach for industrial utility systems optimization under uncertainty. Energy 182, 559–569. doi: 10.1016/j.energy.2019.06.086
Glossary
Notation
pr, Price (£kWh−1)
TC, Total annualized cost (£ yr−1)
C, Annualized cost (£ yr−1)
Qfuel, Fuel enthalpy (kW)
top, Operation time (h yr−1)
Em, CO2e emissions (kgCO2e yr−1)
m, Mass flowrate (kg s−1)
CC, Carbon content (kgCO2e kgfuel−1)
M, Molar mass (kg.mol−1).
ε, Emission factor (kgCO2e kWh−1)
P, Power (kWh)
H, Site heat (kW)
W, Power weight (%)
cap, Capacity (kW)
AF, Annualization factor (unitless)
DR, Discount rate (unitless)
OPEX, Annual operating expenditure (£yr−1)
CR, Capture rate (%)
PIF, Power input factor (kWh kgCO2e−1)
HIF, Heat input factor (kWh kgCO2e−1).
Abbreviations
IES, Industrial Energy System
CHP, Combined Heat and Power
CCS, Carbon Capture and Storage
ORC, Organic Rankine Cycle.
Keywords: alternative technologies, alternative fuels, industrial energy systems, decarbonization, sustainable transition, mathematical optimization
Citation: Oluleye G, Bishay D and Kas B (2023) Can a hierarchical ordering of alternative technological concepts for decarbonizing industrial energy systems minimize mitigation costs? Front. Sustain. 4:1057064. doi: 10.3389/frsus.2023.1057064
Received: 29 September 2022; Accepted: 02 March 2023;
Published: 27 March 2023.
Edited by:
Fadwa Eljack, Qatar University, QatarReviewed by:
Viknesh Andiappan, Swinburne University of Technology Sarawak Campus, MalaysiaGalina Chebotareva, Ural Federal University, Russia
Tran Le Luu, Vietnamese-German University, Vietnam
Copyright © 2023 Oluleye, Bishay and Kas. This is an open-access article distributed under the terms of the Creative Commons Attribution License (CC BY). The use, distribution or reproduction in other forums is permitted, provided the original author(s) and the copyright owner(s) are credited and that the original publication in this journal is cited, in accordance with accepted academic practice. No use, distribution or reproduction is permitted which does not comply with these terms.
*Correspondence: Gbemi Oluleye, by5vbHVsZXllQGltcGVyaWFsLmFjLnVr