- 1Joint Centre for Disaster Research, Massey University, Wellington, New Zealand
- 2Department of Civil and Environmental Engineering, The University of Auckland, Auckland, New Zealand
Earthquake early warning system (EEWS) plays an important role in detecting ground shaking during an earthquake and alerting the public and authorities to take appropriate safety measures, reducing possible damages to lives and property. However, the cost of high-end ground motion sensors makes most earthquake-prone countries unable to afford an EEWS. Low-cost Microelectromechanical systems (MEMS)-based ground motion sensors are becoming a promising solution for constructing an affordable yet reliable and robust EEWS. This paper contributes to advancing Earthquake early warning (EEW) research by conducting a literature review investigating different methods and approaches to building a low-cost EEWS using MEMS-based sensors in different territories. The review of 59 articles found that low-cost MEMS-based EEWSs can become a feasible solution for generating reliable and accurate EEW, especially for developing countries and can serve as a support system for high-end EEWS in terms of increasing the density of the sensors. Also, this paper proposes a classification for EEWSs based on the warning type and the EEW algorithm adopted. Further, with the support of the proposed EEWS classification, it summarises the different approaches researchers attempted in developing an EEWS. Following that, this paper discusses the challenges and complexities in implementing and maintaining a low-cost MEMS-based EEWS and proposes future research areas to improve the performance of EEWSs mainly in 1) exploring node-level processing, 2) introducing multi-sensor support capability, and 3) adopting ground motion-based EEW algorithms for generating EEW.
1 Introduction
Disasters have historically had severe effects on people and infrastructure (Coppola, 2007). Earthquakes are one of them, and they pose a substantial risk to areas near major active faults on land or in offshore subduction zones (Adhikari et al., 2018). Unlike other natural dangers such as cyclones and tsunamis, earthquakes cannot be predicted hours in advance, and when they are identified, earthquake detection and alerts occur in seconds. As a result of the short time between detecting an earthquake event and its imminent impact, developing an earthquake early warning system (EEWS) is difficult (Fischer et al., 2012).
Despite having a short warning window, EEWS can be a valuable tool in increasing safety in regions prone to significant ground shaking (Allen and Melgar, 2019). The warning period may be long enough for pre-programmed systems to implement emergency actions, such as stopping trains to lessen the likelihood of a derailment, turning off heavy machinery to reduce the possibility of losses, and closing gas distribution valves (Strauss and Allen, 2016). In addition, a brief public alert could also enable individuals to perform basic safety precautions like drop-cover-hold and mentally prepare for an impending earthquake (Nakayachi et al., 2019; Becker et al., 2020a; Becker et al., 2020b).
EEWS involves complex earthquake-related processing, which makes generating reliable alerts challenging (Kanamori et al., 1997). Technological advances in seismic instrumentation, digital communication, algorithms, and processing permit the implementation of a robust EEWS (Kanamori et al., 1997). Moreover, to identify earthquakes and send alerts in real-time, EEWSs require a network of geographically dispersed ground motion sensors to create alerts. As such, EEWS can be expensive to implement and maintain. In recent years multiple innovations, such as low-cost sensors (Wu and Mittal, 2021) and new networking architecture (Prasanna et al., 2022), have made it possible to have more affordable systems.
According to their strong-motion data acquisition system (DAS) class, the four types of ground motion sensors employed in EEWSs are A, B, C, and D (Myers, 2008). Class A type sensors are high-performance near state-of-the-art sensors that can record ground motion in a high DAS resolution and DAS dynamic range. In contrast, for classes B, C and D, the DAS resolution and DAS dynamic range tend to decrease accordingly, and the cost related to each type of sensor will increase with the performance (with class A being the most expensive) (Myers, 2008). For example, implementing EEWS with class A sensors will cost millions of dollars, while the EEWS with class C and D sensors need thousands of dollars for implementation (Brooks et al., 2021). Therefore, EEWSs can be classified into two groups according to their implementation cost: conventional high-end EEWSs and low-cost EEWSs (Myers, 2008). The conventional high-end EEWSs are high-cost networks constructed using high-performing class A seismic sensors. The low-cost EEWSs are constructed using lower-classed sensors, mainly class C MEMS-based seismic sensors.
Low-cost alternative technology solutions are emerging to create cost-effective EEWSs instead of expensive high-end EEWSs. Internet of Things (IoT) technologies powered by microelectromechanical systems (MEMS)-based sensors are a part of low-cost solutions (Allen and Melgar, 2019). Past research has been conducted in developing EEWSs using low-cost Micro-electromechanical systems (MEMS) based sensors. Examples of systems that use low-cost MEMS sensors include those in Taiwan (Wu et al., 2013a), California (Clayton et al., 2015), Iceland (earthquake-turnkey, 2020), China (Peng et al., 2019), and New Zealand (Prasanna et al., 2022). These affordable EEWS deployments have shown the practicality and capacity of MEMS-based sensor networks to deliver EEW. They could become a solution for earthquake-prone countries that may not have the sufficient economic capability to afford high-end EEWSs (Prasanna et al., 2022).
In addition, low-cost MEMS-based networks can be helpful, as complementary systems, for territories that have already implemented conventional EEWSs. There are several examples (e.g., Taiwan, the West Coast of the United States, and parts of mainland China) where a low-cost EEWS work as a secondary system supporting their main high-end EEWS (Wu et al., 2017), (Peng et al., 2020). Having a dense low-cost sensor network as a complementary system to the conventional EEWS could create a more robust solution to generate alerts with an acceptable level of warning time for the areas near the epicentre (Wu et al., 2013b).
Low-cost EEWSs have started showing promising results in detecting and providing warnings for ground shaking. In parallel, the affordability of low-cost ground motion sensors has created an opportunity to build crowdsourced EEWSs supported by community participation and engagement (Faulkner et al., 2011; Minson et al., 2015). Along with the inexpensive MEMS-based ground motion sensors that are easily accessible on the market, smartphones and laptops with MEMS-based accelerometers built into their hardware have also emerged as possible sensing tools that could serve as detection tools in an EEWS. Such ubiquity in consumer devices raises the possibility that these devices could be used to achieve operational EEWS via crowdsourcing (Minson et al., 2015). Therefore, there is a potential for low-cost MEMS-based sensors to open new avenues and opportunities supported by crowdsourced open EEWSs.
Recently, a few papers have reviewed EEW research (Cremen and Galasso, 12020; Tan et al., 2022; Velazquez et al., 2020), they cover the seismological, engineering, social, and organisational aspects and general state-of-the-art approaches to EEW. None of them is a comprehensive review of literature on existing low-cost EEWSs implemented worldwide focusing on the technical aspects. Such a technical review can be considered timely as it is invaluable to identify the strengths and weaknesses of the different approaches to inform opportunities and directions for future research in the area of low-cost EEW. In such a context, this article systematically reviews the existing low-cost EEWSs worldwide.
The remainder of this article is organised as follows. Section 2 outlines the methodology used in this study, followed by the findings from the systematic literature review (SLR) presented in Section 3. Discussion and future research areas are presented in Section 4. Finally, Section 5 concludes the paper by summarising the answers to the research questions raised.
2 Methodology
The steps outlined by Algiriyage et al. (2022) are adopted in this SLR to help with the reviewing process, including establishing research questions, finding relevant articles, applying exclusion criteria, extracting relevant data, and analysing the literature.
2.1 Research questions
This review asks three research questions to ensure that the essential components related to low-cost EEWSs are covered:
1. How to classify low-cost MEMS-based EEWSs?
2. What are the existing low-cost MEMS-based EEWSs implemented globally?
3. What are the limitations, challenges, and future research areas in the low-cost MEMS-based EEWSs domain?
By searching the literature to answer Question 1, a classification structure can be drawn for classifying existing low-cost EEWSs. A classification structure will help the researchers have a consistent understanding of different types of EEWS, thus will help in the analysis and comparisons of implemented EEWSs. With Question 2, the SLR explores and finds details about existing low-cost MEMS-based EEWSs, their processing, warning type, and EEW algorithm used. However, details on how EEWSs deliver early warning is not discussed in this study since most articles do not discuss their alert distribution method. The third question explores the limitations and challenges of the current low-cost MEMS-based EEWSs. Answers to the question provide future research areas which will enable researchers to design low-cost EEWS that could overcome the identified challenges and difficulties.
2.2 Search for relevant articles
The search for the relevant articles in the literature was conducted using a keyword search in the Scopus and Web of Science databases on 30 March 2022. A review of the body of literature revealed little relevant material about low-cost EEWSs before September 2009; hence only articles published after that date were considered. To answer the research questions, “earthquake early warning” and “low-cost” were the primary keywords in the search. In addition, “earthquake warning” and “earthquake detection” were used as alternatives to “earthquake early warning.” Alternatives to the phrase “low-cost” include “low cost,” “MEMS,” “cost,” “mobile,” “phone,” “smartphone,” “community-engaged,” “economical,” and “crowdsourced.” Peer-reviewed academic papers accessible online in full-text format and pertinent to the research aims are the only sources included in the initial literature search. Publications written in languages other than English, grey literature like government or corporate reports and non-academic research were all excluded. The initial keyword search produced 354 relevant articles. Duplicates were removed, resulting in 218 unique papers.
2.3 Exclusion criteria
The scope was narrowed to papers that only discussed low-cost MEMS-based implementations related to earthquake detection. The abstracts were manually examined, and those that contained the following information were discarded:
• low-cost EEW that uses different types of sensors other than MEMS-based accelerometers (e.g., optical fibre cables, satellite-based, resonant switches, etc.),
• low-cost implementations related to different disaster detections or structural monitoring,
• surveys or perceptions related to implementing low-cost EEWSs,
• EEWSs that use smartphones solely to notify the stakeholders (as notification tools rather than detecting sensors),
• articles lacking sufficient details related to the EEWS implementation, and
• articles that only broadly discuss earthquake detection algorithms without focusing on EEWS implementation were excluded.
After filtering and applying the inclusion-exclusion criteria, 59 articles were selected for this study. The methodology for the literature search, the selection criteria, and the number of papers returned at each stage are shown in Figure 1.
2.4 Extract relevant data and analyse the literature
After identifying relevant articles, the next step of the methodology is to extract relevant information and analyse them to find answers to the research questions. The main activity to analyse qualitative data is to compare them through naming and classifying (Flick, 2007). As a result of this procedure, a structure for the data is created using Microsoft Excel, paving the way for a thorough comprehension of the problem, the field, and the data themselves. Constant comparison is conducted to analyse the articles to understand the different approaches implemented toward EEW and to develop a classification structure (Hernon, 2004). After extracting the relevant data using the literature, this SLR process is presented to answer the research questions.
3 Findings
This section shows the findings from the SLR of 59 articles. It shows the classifications for EEWS, which can help navigate the varying combinations of different characteristics of EEWSs that exist worldwide. Discussion follows on low-cost EEWSs that have already been implemented worldwide and the contexts and technologies used in the different systems.
3.1 Classification of earthquake early warning systems
The filtered articles revealed that there are different approaches to implementing EEWSs. However, to the authors’ understanding, no existing framework classifies such approaches to implementing EEWSs according to their characteristics. EEWSs are complex systems, and a comprehensive classification of such systems can help better organise the study of EEWS. This will particularly be helpful for those researching, designing, or implementing EEWSs.
3.1.1 Classification of the earthquake early warning systems based on the warning type
Findings show that EEWSs can be classified based on the number of sensors used to detect an earthquake (i.e., on-site networks use a single sensor and regional-based networks use an array of sensors) (Chen et al., 2015; Bindi et al., 2015; Picozzi et al., 2015).
On-site-based EEWS use only one sensor to detect an earthquake. To be more precise, it takes information from a sensor at a location to detect earthquakes and generate alerts at the same location using a single sensor, with all algorithm processing taking place at that station (Allen and Melgar, 2019; Bindi et al., 2015; Picozzi et al., 2015). In general, on-site EEWS serves a significant role in bridging the gap of the blind zone, which frequently experiences the worst ground shaking and where an EEWS cannot issue an alarm close to the epicentre (Chen et al., 2015; Wang et al., 2022). The basic form of an on-site EEWS can be a ground motion threshold-based method that sounds an alarm or warning as soon as unusual or harmful ground motion is discovered. However, few more advanced systems can identify P-waves and send out an alarm when the matching S-wave’s intensity is expected to be damaging (Hoshiba and Aoki, 2015).
On the other hand, regional EEWS use a network of sensors deployed in several parts of a particular earthquake-prone geographical area. Earthquake detection happens by processing the earthquake data collected by the network of sensors. The number of earthquake detection sensors can vary according to the sensor distribution and considered geographical area (Bindi et al., 2015; Picozzi et al., 2015). Generally, regional EEWSs can benefit areas far from the epicentre (Chen et al., 2015).
3.1.2 Classification of regional earthquake early warning systems based on the implemented earthquake early warning algorithm
Regional EEWSs can be further classified according to the type of algorithm used. Regional EEWSs are primarily implemented using two different kinds of EEW algorithms, namely: source-based and ground motion-based algorithms (Allen and Melgar, 2019). Figure 2 illustrates the classification based on EEW algorithms.
3.1.2.1 Source-based methods
Source-based approaches detect the earthquake and disseminate the alert to EEW stakeholders with detailed information about the detected earthquake. These methods typically identify an earthquake and characterise the position, origin time, and magnitude by using a suitable ground motion prediction equation plus a few seconds of P-wave data (0.5–4 s) from two to six sensors around the epicentre (GMPE) (Peng et al., 2021a).
Generally, source-based approaches could be implemented using two different types of algorithms: point source-based and finite fault-based (Figure 2). Even though both types of algorithms detect the earthquake characteristics using the P-waves, point source algorithms typically saturate for earthquake ∼ M > 6. The main causes of saturation are two: 1) it is difficult to distinguish earthquakes with a magnitude greater than six from those that occur in the first few seconds of the P-wave window, and 2) the lateral extent of the fault rupture is necessary for large earthquakes to determine the earthquake’s magnitude (Won et al., 2020). On the other hand, finite fault algorithms tend to estimate the earthquake’s magnitude without any saturation limitation by determining the fault rupture’s finite extent.
3.1.2.2 Ground motion or wavefield-based methods
Ground motion or wavefield-based algorithms became popular due to their robustness and fast processing time. They do not estimate the source parameters of an earthquake (Hoshiba, 2013; Hoshiba and Aoki, 2015; Kodera, 2018). Thus, they avoid vulnerabilities of the source-based algorithms (Hoshiba, 2021). The main idea behind these algorithms is to forecast the likely evolution of ground motion intensity in the future using the current state of ground shaking and knowledge of propagation physics in a short period of time. These algorithms assume that the observation sites are close to the hypocentre than the prediction sites, meaning that the seismic sensor network plays a major role (Hoshiba, 2021). Table 1 summarises the number of EEWSs implemented according to the proposed classification.

TABLE 1. Number of approaches presented in the reviewed articles based on the proposed classification.
3.2 Low-cost earthquake early warning systems implemented around the world
MEMS-based technologies have inspired researchers to implement low-cost EEWS in several countries worldwide over the last 10 years, demonstrating promising results and robustness during an earthquake event. Even though many countries are prone to seismic hazards, only a few have successfully implemented any form of EEWS (Bindi et al., 2015). However, several countries are currently in the process of implementing more affordable low-cost EEWSs. This section summarises existing low-cost MEMS-based EEWSs into types: the experimental or initial stage and the public alert generation stage according to the classification proposed in Section 3.1. In general, experimental or initial stage EEWSs deliver EEWs only to a selected set of people primarily for testing, whereas EEWSs in the public alert generation stage, deliver EEWs to the general public in a seismic-prone area that can be a region or an entire nation. Table 2 summarises the low-cost MEMS-based EEWSs identified from the selected articles.
3.2.1 Regional earthquake early warning systems
As identified in the classification section above, regional EEWSs are implemented using two different algorithms for earthquake detection, namely source-based and ground motion or wavefield algorithms. Here, the source-based (point source and finite fault) and ground motion-based approaches are analysed separately.
3.2.1.1 Source-based regional earthquake early warning systems
Source-based algorithms can be divided into two different algorithms: point-source-based and finite-fault-based. However, minimal information is available on the low-cost EEWSs that use a finite-fault algorithm for earthquake detection. Therefore, unless otherwise stated, all the implementations discussed here use point-source-based algorithms. Here, EEWSs were reviewed according to the type of sensor used.
3.2.1.1.1 Systems implemented using dedicated microelectromechanical systems-based sensors
The first ever low-cost EEWS was developed by Fleming et al. (2009) from Germany. They used their in-house built low-cost sensors SOSEWIN 1 and SOSEWIN 2 to implement an experimental self-organizing seismic early warning network in Istanbul and Berlin (Fischer et al., 2012). The system consisted of a decentralised, self-organizing wireless mesh sensor network, with sensors connected via WLAN (Wireless Local Area Network 2.4 GHz or 5 GHz). This system detects the P-waves using the short-term-average (STA)/long-term-average (LTA) method and captures the details of the P-waves to determine the source characteristics of the earthquake.
In contrast to the above approach, most regional low-cost EEWSs are implemented using a centralised processing unit. A low-cost EEWS implemented by Wu et al. (2013a) in Taiwan is a prime example of that approach. They designed a low-cost ground motion sensor known as P-alert, which uses MEMS-based accelerometers where the processing of the EEW algorithm takes place at a centralised server. The P-alert system uses the STA/LTA ratio (Allen, 1978) to detect the P-waves and continuously monitors the peak ground acceleration (PGA) using MEMS-based accelerometers. Following the detection of P-wave characteristics for the first 3 s of P-waves, they are sent to the central station via TCP/IP connections. During the alert generation, an event is declared once eight P-alert stations are triggered, and an alert will be disseminated to the public. A traditional earthquake location algorithm with a half-space linear increasing-velocity model is used to determine the hypocentre location. Peak displacement (Pd) and peak velocity attenuation (MPv) or peak displacement attenuation (MPd), and hypocentre distance are used to calculate magnitude. The system takes from 9 s to 28 s to generate a warning and an average of less than <20 s compared to the conventional high-end EEW in Taiwan, which generates warnings in 20 s.
Also, the P-alert EEWS has been used to detect the earthquake’s rupture directivity, building a real-time shake map for ongoing earthquakes and damage localisation in buildings after an earthquake (Jan et al., 2018; Yin et al., 2016) The P-alert system’s performance was evaluated using Taiwan’s 2018 Mw 6.4 Hualien earthquake (Hsu et al., 2018b). Using the same P-alert sensor network, Yang et al. (2018) implemented a regional EEWS called ShakeAlarm. ShakeAlarm can provide a wider lead time than their national-level regional EEWS operated by Central Weather Bureau (CWB) by bypassing the location estimation by employing the relationship between the shake contour area and earthquake magnitude (Yang et al., 2018). Similarly, a team of researchers from India implemented an EEWS at the Himalayan Belt using (Franchi et al., 2019) P-alert sensors (Mittal et al., 2019; Chamoli et al., 2021).
Several researchers from China have also implemented EEWS in seismically active regions using low-cost MEMS-based sensors. As an initial step, Peng et al. (2017) constructed a sensor called GL-P2B using MEMS-based accelerometers (Peng et al., 2017). They demonstrated that their sensor could detect smaller earthquakes M < 1.5 and a high signal-to-noise ratio (SNR) at distances greater than 50 km for earthquakes of M > 3. To detect the earthquake, they used the same detection algorithms adopted by Wu and Zhao (2006), Wu et al. (2007) and tested their network by deploying approximately 170 stations in Sichuan, China (Peng et al., 2019; Peng et al., 2017). Following that, another group of researchers implemented a low-cost EEWS by building a new low-cost MEMS-based ground motion sensor known as the SIT-S Model in addition to the GL-P2B sensors in the Zhejiang province (Zou et al., 2019; Finazzi, 2020). Afterwards, a hybrid EEWS was introduced in the Sichuan region by integrating the GL-P2B sensors with P-alert sensors and local broadband seismic stations. There are around 270 MEMS-based sensors (170 GL-P2B sensors and 100 P-alert sensors) and 13 local broadband seismic stations where the hybrid EEWS processing takes place at a centralised server (Peng et al., 2020). Currently, around 1631 MEMS-based stations contribute to the regional EEWS in the Sichuan Area operated by the Sichuan Earthquake Administration (Peng et al., 2021b). Processing of the EEW algorithm takes place at a centralised server adopting the peak displacement (Pd) scaling approach proposed by Kuyuk and Allen (2013) for estimating the earthquake magnitude and calculating seismic intensities at various target sites, a point source algorithm based on the conventional ground motion prediction equation (GMPE) is used (Kong et al., 2016a).
The United States also plays a significant role in contributing to low-cost EEW. Areas near the San Andreas fault in California, United States, are prone to frequent seismic events. Such a context has motivated US researchers to investigate implementing EEWSs in this region. Two primary regional networks deliver alerts to the public in the United States using dedicated MEMS-based sensors: Quake Catcher Network (QCN). Clayton et al. (2015) from California constructed the CSN by building their in-house sensor. In their system, event detection is carried out at the centralised server (Google App Engine). In contrast, the sensor systems themselves generate measurements of characteristics like pick times, maximum amplitude, apparent frequency, and signal-to-noise levels in close to real-time (Clayton et al., 2015). The CSN’s primary functions include monitoring the health and safety of structures, producing zonation maps of populated regions, and giving maps of maximum shaking soon after a significant earthquake to assist first responders (Hong et al., 2021).
On the other hand, the QCN distributed/volunteer computing initiative enables any internet-connected computer with an internal or external MEMS accelerometer to function as a strong-motion seismic station (Cochran et al., 2009). The QCN offers a dense, wide-scale seismic network by utilising the primary benefit of distributed computing, which is the ability to use a large number of processors at a cheap infrastructure cost. Data is analysed on an individual’s laptop or desktop during idle computer cycles, and only a little amount of data is forwarded to a central server for additional analysis. The QCN servers go through the data, including detection time, signal amplitudes, Internet protocol (IP) addresses, and other relevant information, to ascertain which data corresponds to earthquakes. This system can detect moderate to large earthquakes (magnitude > 5). Over 2,000 volunteers had signed up by 2014, mainly from California and Mexico (Hong et al., 2021). Currently, sensors called “Joy-Warrior” and “O-NAVI” are used as external MEMS accelerometers in computers. Following that, another team from the University of California Berkeley and the Humboldt State University implemented a small experimental MEMS-based EEWS called ElarmS to support the conventional high-end EEWS (Nof et al., 2019). They proved that a MEMS-based sensor array with a finite-fault model could estimate earthquake magnitude and shaking intensity distribution faster and more reliable compared to using Global Navigation Satellite Systems (GNSS) (Nof et al., 2019).
In addition to the above efforts, researchers in several other countries attempted to implement low-cost EEWSs. A group of researchers from Italy implemented an experimental MEMS-based low-cost EEWS using Adel ASX1000 type accelerometers to detect small local magnitude earthquakes between 2.0 < ML < 3.0 in two seismically active areas of Italy: the inner part of the Umbria Valley and the southern-easy Alpne Front (Cascone et al., 2021). They used 15 ASX1000 prototype sensors and a centralised server where communication between the server and the sensors occurred via LAN connection (Kong et al., 2020a). In addition, several approaches were attempted by researchers in Italy toward low-cost EEWS (D’Alessandro et al., 2018; D’Alessandro et al., 2014). Also, Taale et al. (2021) from Canada explored the feasibility of implementing an experimental regional EEWS using the MEMS-based accelerometers embedded in the smart meters (SM). They have tested SM’s capability in detecting an earthquake by doing different tests such as statics tests, noise floor tests, dynamic tests, etc. and declared that SM infrastructure as an EEWS is technically feasible (Taale et al., 2021). Table A1 provides a table summarising the EEWSs implemented using dedicated MEMS-based sensors.
3.2.1.1.2 Systems implemented using smartphones
Off-the-shelf sensors like those found in smartphones are also offered as scalable opportunistic sensor nodes as an alternative to in-house-built low-cost MEMS-based sensors (Brooks et al., 2021). The nearly universal availability of MEMS-based class C accelerometers in cell phones makes phone-based sensing possible. These motion sensors can be configured to function as seismometers, identifying the shaking caused by earthquakes. Several countries have tried implementing low-cost EEWS using smartphones. Almost all the smartphone-based EEWSs are regional networks, and the processing of the earthquake detection algorithm takes place partially or entirely at a centralised location. Wang et al. (2018) found that for an EEW generation, it is necessary to have at least four stationary smartphones to mitigate the effect of unwanted accelerations.
According to the EEW literature, Japan has implemented a low-cost EEWS using smartphones (Uga et al., 2012). This system is one of the earliest EEWS in the literature that uses smartphones as ground motion sensors. This EEWS has been integrated as a support system to Japan’s conventional JMA EEWS. Like Japan’s approach, Zambrano et al. (2015) and Zambrano et al. (2014) developed an EEWS using smartphones, demonstrating robust results compared to Japan’s approach. They constructed their EEW network using three different layers: smartphones, intermediate servers, and a control centre, where different tasks are assigned to these layers. They have also analysed different wave propagation techniques according to the geographical areas. At the time of the implementation, their method detected an earthquake 12 s before the peak acceleration, which was a notable achievement compared to other smartphone implementations.
Similar to Japan, a team of researchers from the United States (Minson et al., 2015) implemented an EEWS with consumer smartphones via crowdsourcing. In their approach, in addition to MEMS-based accelerometer data, they incorporated data from GNSS (Global Navigation Satellite Systems) and GPS (Global Positioning System) to overcome the saturation issue for predicting large earthquakes (Mw > 7) (Hsu et al., 2018a). They tested their EEWS using simulated data from the Mw 7.0 Hayward fault earthquake in California and actual data from the Mw 9.1 Tohoku-Oki earthquake, and the results were promising. Similar to their approach, researchers from the University of California implemented a smartphone-based EEWS called “MyShake” (Allen et al., 2020; Kong et al., 2015) where they proved that a robust EEWS could be implemented using a smartphone-based network with artificial intelligence to detect an earthquake from human activities. The network delivers alerts to the phones if the estimated magnitude of an earthquake is 4.5 or greater or Modified Mercalli Intensity (MMI) III or greater zone (Strauss et al., 2020). MyShake can detect an earthquake and its characteristics within ∼5–7 s after the origin time, and the alert can be disseminated to smartphones within ∼1–5 s (Kong et al., 2019a; Allen et al., 2020).
Similar to MyShake, researchers from Italy implemented an EEWS called the EQN (Earthquake Network) using an android application installed on smartphones from the community. Like the other mobile phone-based approaches, this system uses a centralised server to process the EEW algorithm to detect an earthquake and its source parameters (Finazzi, 2020; Finazzi, 2016; Finazzi and Fasso, 2017). A statistical approach has been used to define the threshold based on the number of active smartphones using a fixed and dynamic sub-network-based detection algorithm to detect an earthquake with minimal false alerts (1 false alert per year). Also, during this approach, two ways for detecting earthquake epicentre were introduced, which are suitable for smartphone-based EEWSs (Finazzi, 2020; Finazzi, 2016; Finazzi and Fasso, 2017). Currently, they have selected only phones stationed for charging as sensors. An earthquake is detected when concurrent triggers within 30 kms of each other surpass a dynamic threshold determined by the number of active smartphones and the intended false alarm probability, which is currently set at one per year per country (Bossu et al., 2021; Yang et al., 2021; Peng et al., 2017).
Following that, researchers from South Korea have also implemented a low-cost EEWS known as CrowdQuake that uses around eight thousand smartphones (Samsung Galaxy S7) securely embedded into enclosures and deployed at different locations (Kwon et al., 2020; Wu et al., 2021). They have used a deep convolutional-recurrent neural network (CRNN) model to detect earthquakes using a centralised server (Kwon et al., 2020). They demonstrated that their EEWS could detect earthquakes with MMI > 3 using two examples of detected earthquakes (Huang et al., 2020). Similarly, a team of researchers from Costa Rica built a smartphone-based EEWS using a mobile app (Brooks et al., 2021). They fixed the mobile phones using enclosures in different areas in the country where station spacing is ∼30 km and increases to 30–50 km away from the Middle America Trench. Also, due to the noisy nature of smartphone accelerometers, they use a quadrilateral mesh configuration of adjoining stations to trigger an alert (Becker et al., 2020a).
In 2020, Google launched the Android Earthquake Alerts system by forming a public-private partnership with the USGS (United States Geological Survey) using the already constructed MyShake EEW model (Allen and Stogaitis, 2022). Initially, it delivered ShakeAlert (Kohler et al., 2018) messages to all Android phones in California. Then in 2021, Google’s EEWS was constructed using the already existing MyShake EEW model and started delivering alerts to New Zealand and Greece using the android phones in those regions (Allen and Stogaitis, 2022; Kong et al., 2016b). Following that, Turkey, the Philippines, and some central Asia countries were added to Google’s android EEWS (Allen and Stogaitis, 2022). Since Google’s android Earthquake Alerts system is relatively new, there is not enough published literature to evaluate its performance.
The following figure (Figure 3) illustrates the timeline of smartphone-based EEWSs according to the literature.
3.2.1.2 Ground motion or wavefield-based Earthquake Early Warning Systems
There is no information on any systems that use smartphones as their ground motion sensors for ground motion or wave field-based algorithms. Thus, only implementations that use MEMS-based sensors are discussed here.
3.2.1.2.1 Systems implemented using dedicated microelectromechanical systems-based sensors
The usage of ground motion or wavefield algorithms in low-cost MEMS-based sensors is difficult to find in the literature. To the authors’ knowledge, there is only one implementation from researchers at Massey University, New Zealand, which discusses a ground motion-based detection algorithm in a low-cost environment (Prasanna et al., 2022). In particular, they implemented an EEWS using Raspberry Shake 4D, a MEMS-based low-cost ground motion sensor manufactured by Raspberry Shake. Unlike other EEWSs, the researchers processed their EEW algorithm at the node level rather than centrally. They employed PLUM as their EEW algorithm—a robust and well-known ground motion-driven method in EEWS (Kodera et al., 2018). Their results claim that node-level processing can outperform traditional centralised processing in terms of system latency, redundancy, and implementation cost.
3.2.2 On-site-based earthquake early warning systems implemented globally
There is minimal information in the literature on any systems that use smartphones as their ground motion sensors for on-site approaches except the one implemented by Hsu and Nieh (2020). Thus, we have mainly discussed implementations that use MEMS-based sensors.
3.2.2.1 Systems implemented using dedicated microelectromechanical systems-based sensors
On-site techniques, as described in Section 4.1, are primarily used to detect earthquakes and provide alerts at the sensor level. Even though it can detect an earthquake as soon as the seismic waves initially reach the sensor, there is a high probability of false alerts since the detection and generation of an alert depend only on one sensor. However, several countries have implemented the on-site approach as one of their EEWS due to its important benefit of minimal detection time, which can be useful in alerting the areas near the epicentre and rupturing fault during an earthquake (Chen et al., 2015). Even though the basic idea of the on-site approach is to generate an alert by using just a ground motion threshold, a few sophisticated approaches went beyond that scope by predicting the destructing S-wave’s intensity using the detected P-waves—these will be discussed below.
Initial efforts to build a sophisticated on-site EEWS were conducted by Wu et al. (2013b) from Taiwan. They constructed an on-site EEWS using P-alert seismic sensors, the same sensor used for the regional EEWS. The P-alert sensor has a display showing the severity of the shaking and a sound warning mechanism with a touch screen. The earthquake’s severity is determined using the first 3-s window of the P-wave, and an integrated alarm system is used to generate the alert. The time taken to detect the earthquake using the on-site approach is around 8 s. The P-alert system can provide 2–8 s warning time for sites in the Central Weather Bureau regional warning system’s blind zone. Following that, by adopting the same detection mechanism and algorithms by Wu et al. (2013b) and Zambrano et al. (2017), researchers from China implemented an on-site approach in the Zhaotong region by building their own low-cost sensor with three different alarms such as sound, text and screen flickering. Their approach took around 3.2 s to generate the warning; however, the system registered many false alarms that cannot be neglected (Peng et al., 2013). Likewise, another research team from China has implemented an on-site approach by building their own sensor called REMOS SIT (Ding et al., 2017).
Further, Hu et al. (2021) proposed another advanced low-cost seismic station for an on-site EEWS by embedding eight MEMS-based accelerometers into one system known as parallel acquisition. They proved that parallel acquisition would reduce the Root Mean Square value of the MEMS-based sensor’s noise compared to using a single sensor. Also, they implemented their network in the Sichuan region, China using 45 proposed seismic stations and demonstrated that the proposed on-site EEWS could detect earthquakes accurately (Hu et al., 2021). Likewise, a multi-parameter wireless sensing system called MPwise was introduced by Boxberger et al. (2017) to detect an earthquake which can be used as an on-site or regional EEWS. However, they tested their EEWS using the on-site approach in Kyrgystan with the SeisComP seismic software for earthquake-related processing in terms of detecting earthquake source parameters. Unlike other approaches, this device is embedded with multi-sensor capability, which can be connected with different sensors for various applications (Boxberger et al., 2017).
In addition to the above approaches, using SOESWIN sensors, Bindi et al. (2015) constructed an on-site EEWS for one of the highest earthquake hazard regions in Kyrgyzstan (Bindi et al., 2012) which works as a support system for their regional warning network. Similarly, a group of South Korean researchers built a standalone earthquake detection and alerting device for residences that can send notifications to nearby devices. In their approach, they tried to disseminate the earthquake intensity by calculating the PGA (peak ground acceleration) values from the ground motion data (Lee et al., 2019a; Lee et al., 2019b). Further, there are also different on-site approaches that have been implemented by Won et al. (2020) and D’Alessandro et al. (2020) in detecting earthquakes.
4 Discussion
Complications towards developing high-end conventional EEWSs have motivated researchers to pursue other feasible options in implementing an EEWS, and low-cost MEMS-based EEWSs have come to the fore as one of the solutions. Low-cost EEWSs have become a solution for earthquake-prone countries which are not economically able to afford high-end EEWS. Also, low-cost EEWSs serve as a support system for countries with high-end conventional EEWSs. As discussed previously, low-cost EEWSs can be divided into two types: on-site approaches and regional approaches. Regional-based low-cost EEWSs are more robust and accurate in disseminating alerts, and on-site approaches generate rapid alerts. Still, it uses a single station to detect an earthquake which can compromise the reliability and accuracy of generating alerts (Allen and Melgar, 2019). However, this SLR process identified several limitations and challenges related to these low-cost regional EEWSs. This section discusses the future research opportunities for regional EEWSs arising from the limitations and challenges identified during the SLR process.
4.1 Limitations
According to the literature review, the first limitation identified is that most regional EEWSs process their earthquake-related data centrally. A central data processing site offers several benefits, including improved control for locating, gathering, and processing data both during and shortly after a disaster (Aizu, 2011). Centralised data processing does, however, bring up several technological constraints and bottlenecks. One of the critical restrictions is the potential for data collecting to be disrupted at a central site because of effects on telecommunications infrastructures after a significant earthquake (Kobayashi, 2014). After an earthquake, facilities for data processing, intermediate data gathering, and data transportation may be significantly disrupted or destroyed (Prasanna et al., 2022). Giving time-sensitive warnings to end users may be difficult if the central processing capability is unavailable due to a loss of connectivity.
Further, the SLR process indicated that almost all low-cost EEWSs are limited to a single type of ground motion sensor. For example, the EEWS developed by Wu et al. (2013b) uses only the P-alert sensors (Taiwan), and Peng et al. (2020) from China uses its in-house-built sensors for EEWS. Even though the MPwise device implemented by Boxberger et al. (2017) can support multiple MEMS-based sensors, they did not discuss the implementation of their system using multiple MEMS-based sensors. EEWSs without multi-sensor capability can be identified as one of the major limitations in almost all the low-cost MEMS-based EEWSs worldwide.
Another limitation is that most regional EEWSs globally are constructed using point source or finite fault source-based algorithms. Even though source-based algorithms generate reliable warnings, a significantly longer processing time is required for estimating earthquake source characteristics, making them less beneficial for the areas near the epicentre and effective only for areas far from the epicentre (Wu et al., 2013a). Areas affected during a large earthquake can be geographically large. On the other hand, significant earthquake damage will almost always occur at shorter epicentral distances and near the rupturing fault. Thus, it is crucial for any EEWS to generate an initial alert to the areas near the epicentre within a few seconds. To overcome this limitation, several countries have implemented on-site approaches that are fast but not reliable in generating alerts (Kwon et al., 2020).
4.2 Challenges
Network security is identified as a significant challenge for community-engaged EEWSs. Community engagement is becoming one of the emerging factors in constructing a low-cost EEWS where the public contributes to the network by installing or maintaining a sensor (Prasanna et al., 2022; Minson et al., 2015; Kong et al., 2016b). For example, installing P-alert sensors at houses or installing an EEW application on their smartphone sends data to the data processing centre. Although community participation is a promising alternative, network security becomes an issue that needs to be addressed and researched (Prasanna et al., 2022). Because sensors are spread over several geographic regions and are connected to various home-based private networks, security is essential to prevent unintentional or purposeful attacks. One specific component of system security is connected to spoof earthquake triggers, such as how simple it is to spoof earthquake triggers and how resistant the system is to spoof earthquake triggers from individual phones, ad hoc or coordinated groups of phones in a certain location and time period (Kong et al., 2019a; Kong et al., 2019b).
Following that, the sensor density of MEMS-based sensors in an EEWS is picked out as another significant challenge. Even though implementing a regional EEWS ensures a robust system compared to an on-site-based EEWS, a significant amount of time such systems in detecting earthquakes needs to be addressed. Regional low-cost EEWSs generate an alert for ground shaking by analysing the ground motion data from more than one sensor. Generally, regional EEWS disseminate an alert if and only if at least more than one station is triggered, which is also called multi-station triggering (Wu and Mittal, 2021; Prasanna et al., 2022; Brooks et al., 2021; Bossu et al., 2021; Cochran et al., 2019). This feature ensures a minimal number of false alerts generated by an EEWS. However, the multi-station triggering mechanism will cost a significant amount of time since it needs to wait for the data from multiple sensors. Therefore, to reduce the detection time of a low-cost regional EEWS, the sensor density should be significantly increased compared to conventional high-end EEWSs, where the high-end sensors are less prone to false alerts (Prasanna et al., 2022).
In addition to the challenges discussed above, detecting ground motions in smartphones while they are in motion is recognised as another challenge for smartphone-based EEWSs. When it comes to smartphone-based EEWSs, most EEWSs either work with smartphones that are fixed and charging (Brooks et al., 2021; Allen and Stogaitis, 2022). This reduces the number of active sensors in the network. The number of sensors in the network tends to vary according to the time of the day (peak hours and non-peak hours), limiting the sensing capability of the EEWS. Even though few initiatives were carried out on machine learning techniques to detect an earthquake and other daily activities when smartphones are in mobility, they are still not integrated into most smartphone-based EEWSs.
4.3 Future research
According to the identified limitations and challenges, the following are the future research areas proposed by this study.
4.3.1 Usage of decentralised or node-level processing
The ability to use sensors to decrease the amount of central processing and raise the level of processing at the node level has been made possible by advancements in sensor technology (edge computing) (Fischer et al., 2012; Prasanna et al., 2022). This will make it possible to process ground motion data and create warnings that may be shared across the sensor nodes and other connected devices at the node level (Prasanna et al., 2022). Node-level processing has also been recognised as a new paradigm for real-time IoT tasks. It has become popular to handle issues with response time, requirement, saving bandwidth, costs, and data security and privacy (Zhao et al., 2021; Khan, 2020). Furthermore, it makes systems more resilient by allowing end users to be warned at the local and regional levels, even when infrastructure failures affect parts of the system. To extract maximum benefits associated with sensor nodes’ capabilities and capacities, only a few attempts have been made to implement EEWSs with decentralised processing (Fischer et al., 2012; Prasanna et al., 2022). However, EEWSs that process earthquake-related data at the node level are still in their infancy, creating more room for further investigations and exploration.
4.3.2 Earthquake early warning systems with multi-sensor capability
The SLR process demonstrates the need for additional research to evaluate EEWSs that can integrate multiple MEMS-type sensors. These systems can be a promising approach toward having a denser sensor distribution in an EEWS. They can also generate benefits by having a large community-engaged EEW network where people can plug and play with their available ground motion sensor rather than limiting it only to a single type of sensor. However, EEWSs with multi-sensor capability need to be explored further. Therefore, there is a need for future research to investigate the possibilities of constructing a low-cost EEWS that can support different types of MEMS-based ground motion detection sensors.
4.3.3 Adaptation of ground motion or wavefield-based earthquake early warning algorithms
Ground motion or wavefield-based algorithms have shown promising results in overcoming the issues related to the source-based regional EEWSs (Hoshiba, 2021). Therefore, adopting ground motion-based algorithms into a regional-based EEWS can generate more robust and reliable alerts in a short period, making them a better alternative to on-site-based approaches for the earthquake-prone areas near the epicentre while keeping the source-based algorithms to generate warnings for the areas far from the epicentre. However, low-cost MEMS-based EEWSs constructed using ground motion algorithms have not been a subject of significant research. Even though the EEWS implemented by Prasanna et al. (2022) adopted the PLUM-based ground motion approach, there is a significant opportunity for further research and improvement.
In addition to the above key research areas, network security of community-engaged EEWSs, and detecting ground motions using smartphones that are in motion are two areas that need further research.
Figure 4 illustrates the discussion flow, highlighting the limitations and challenges of regional low-cost EEWSs that attributes to the future research areas identified during this study.
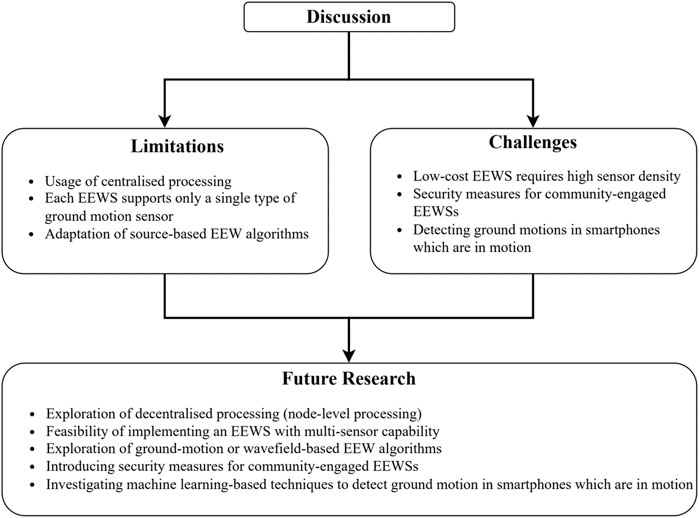
FIGURE 4. Discussion flowchart that summarises the limitations, challenges, and future research identified during this study.
5 Conclusion
EEWSs already exist worldwide, and more countries are exploring the feasibility of designing and deploying EEWS for earthquake resilience. EEWSs are shifting from conventional high-end EEWSs towards a new concept of low-cost MEMS-based EEWSs that integrates and engages with the community. The development made in modern-day’s IoT creates a path to implement an EEWS using low-cost MEMS-based ground motion detection sensors. Also, it has enabled the implementation of more sophisticated and robust low-cost MEMS-based EEWSs to generate reliable and accurate alerts.
This paper addresses the future research areas in the low-cost MEMS-based EEWSs by reviewing the literature and presenting an SLR on the low-cost EEWSs by generating three main systematic review questions. The findings from this review answered the following research questions:
1. How to classify low-cost MEMS-based EEWSs?
The reviewed articles revealed that networks can be classified into two categories according to the warning type and adopted EEW algorithm. Warning types can be on-site and regional, whereas the regional EEW algorithms can be classified as source-based and ground motion-based.
1. What are the existing low-cost EEWSs implemented around the world?
Low-cost EEWSs were classified according to research question 1 and analysed in terms of the type of low-cost sensor used, whether smartphones or Dedicated ground motion sensors.
2. What are the limitations and challenges in implementing a low-cost EEWS?
It was identified that: 1) most of the low-cost EEWSs were centralised, 2) EEWSs support only a single type of low-cost ground motion detection sensor, and 3) most of the regional EEWSs adopted the source-based algorithms which consume a significant amount of time in detecting and estimating earthquake parameters. Also, the main challenges in implementing a low-cost EEWS were identified as: 1) security measure which needs to be analysed further in terms of constructing a community-engaged EEWS, and 2) detecting human activities related motions from the earthquake’s ground motion in smartphone-based EEWSs.
After answering these questions, opportunities, directions and research areas for further investigation were identified. Exploring the feasibility of node-level processing, introducing multi-sensor support capability, and adopting ground motion-based EEW algorithms for regional EEWSs are areas for future research. Investigation into these identified research areas and opportunities for low-cost EEWSs will be beneficial for building robust, low-cost MEMS-based EEWS, significantly benefiting regions of high seismicity.
Author contributions
CC presented the idea and conceptualized the article along with RP and MS. The methodology is constructed by CC, RP, and MT. CC carries out a formal analysis of the data and investigation. Writing the draft is done by CC and reviewing and editing the article is accomplished by RP, MS, and MT. RP, MS, and MT supervised the study.
Funding
This research is funded by the Resilience to Nature’s Challenges-Urban Theme 2020, and by the Toka Tū Ake EQC, New Zealand.
Conflict of interest
The authors declare that the research was conducted in the absence of any commercial or financial relationships that could be construed as a potential conflict of interest.
Publisher’s note
All claims expressed in this article are solely those of the authors and do not necessarily represent those of their affiliated organizations, or those of the publisher, the editors and the reviewers. Any product that may be evaluated in this article, or claim that may be made by its manufacturer, is not guaranteed or endorsed by the publisher.
References
Adhikari, M., Paton, D., Johnston, D., Prasanna, R., and McColl, S. T. (2018). Modelling predictors of earthquake hazard preparedness in Nepal. Procedia Eng. 212, 910–917. doi:10.1016/j.proeng.2018.01.117
Aizu, I. (2011). The role of ICT during the disaster – a story of how Internet and other information and communication services could or could not help relief operations at the Great East Japan Earthquake. Melville, South Africa: Global Information Society Watch Report, 1.2014.
Algiriyage, N., Prasanna, R., Stock, K., Doyle, E. E. H., and Johnston, D. (2022). Multi-source multimodal data and deep learning for disaster response: A systematic review. SN Comput. Sci. 3 (1), 92. doi:10.1007/s42979-021-00971-4
Allen, R. M., Kong, Q., and Martin-Short, R. (2020). The MyShake platform: A global vision for earthquake early warning. Pure Appl. Geophys. 177 (4), 1699–1712. doi:10.1007/s00024-019-02337-7
Allen, R. M., and Melgar, D. (2019). Earthquake early warning: Advances, scientific challenges, and societal needs. Annu. Rev. Earth Planet. Sci. 47, 361–388. doi:10.1146/annurev-earth-053018-060457
Allen, R. M., and Stogaitis, M. (2022). Global growth of earthquake early warning. Sci. Assoc. Adv. Sci. 375, 717–718. doi:10.1126/science.abl5435
Allen, R. v. (1978). Automatic earthquake recognition and timing from single traces. Bull. Seismol. Soc. Am. 68 (5), 1521–1532. doi:10.1785/bssa0680051521
Becker, J. S., Potter, S. H., Prasanna, R., Tan, M. L., Payne, B. A., Holden, C., et al. (2020). Scoping the potential for earthquake early warning in aotearoa New Zealand: A sectoral analysis of perceived benefits and challenges. Int. J. Disaster Risk Reduct. 51, 1–16. doi:10.1016/j.ijdrr.2020.101765
Becker, J. S., Potter, S. H., Vinnell, L. J., Nakayachi, K., McBride, S. K., and Johnston, D. M. (2020). Earthquake early warning in aotearoa New Zealand: A survey of public perspectives to guide warning system development. Humanit. Soc. Sci. Commun. 7 (1), 1–12. doi:10.1057/s41599-020-00613-9
Bindi, D., Abdrakhmatov, K., Parolai, S., Mucciarelli, M., Grunthal, G., Ischuk, A., et al. (2012). Seismic hazard assessment in Central Asia: Outcomes from a site approach. Soil Dyn. Earthq. Eng. 37, 84–91. doi:10.1016/j.soildyn.2012.01.016
Bindi, D., Boxberger, T., Orunbaev, S., Pilz, M., Stankiewicz, J., Pittore, M., et al. (2015). On-site early-warning system for Bishkek (Kyrgyzstan). Ann. Geophys. 58 (1). doi:10.4401/ag-6664
Bossu, R., Finazzi, F., Steed, R., Fallou, L., and Bondár, I. (2021). ‘ shaking in 5 seconds!’ A voluntary smartphone-based earthquake early warning system. arXiv preprint arXiv:2102.06739.
Boxberger, T., Fleming, K., Pittore, M., Parolai, S., Pilz, M., and Mikulla, S. (2017). The multi-parameter wireless sensing system (MPwise): Its description and application to earthquake risk mitigation. Sensors Switz. 17, 2400–2410. doi:10.3390/s17102400
Brooks, B. A., Protti, M., Ericksen, T., Bunn, J., Vega, F., Cochran, E. S., et al. (2021). Robust earthquake early warning at a fraction of the cost: ASTUTI Costa Rica. AGU Adv. 2 (3), e2021AV000407. doi:10.1029/2021av000407
Cascone, V., Boaga, J., and Cassiani, G. (2021). Small local earthquake detection using low‐cost MEMS accelerometers: Examples in northern and central Italy. Seismic Rec. 1 (1), 20–26. doi:10.1785/0320210007
Chamoli, B. P., Kumar, A., Chen, D. Y., Gairola, A., Jakka, R. S., Pandey, B., et al. (2021). A prototype earthquake early warning system for northern India. J. Earthq. Eng. 25 (12), 2455–2473. doi:10.1080/13632469.2019.1625828
Chen, D.-Y., Wu, Y.-M., and Chin, T.-L. (2015). Incorporating low-cost seismometers into the central weather Bureau seismic network for earthquake early warning in taiwan. Terr. Atmos. Ocean. Sci. 26 (5), 503. doi:10.3319/tao.2015.04.17.01(t)
Clayton, R. W., Heaton, T., Kohler, M., Chandy, M., Guy, R., and Bunn, J. (2015). Community seismic network: A dense array to sense earthquake strong motion. Seismol. Res. Lett. 86 (5), 1354–1363. doi:10.1785/0220150094
Cochran, E. S., Bunn, J., Minson, S. E., Baltay, A. S., Kilb, D. L., Kodera, Y., et al. (2019). Event detection performance of the PLUM earthquake early warning algorithm in southern California. Bull. Seismol. Soc. Am. 109 (4), 1524–1541. doi:10.1785/0120180326
Cochran, E. S., Lawrence, J. F., Christensen, C., and Jakka, R. S. (2009). The quake-catcher network: Citizen science expanding seismic horizons. Seismol. Res. Lett. 80 (1), 26–30. doi:10.1785/gssrl.80.1.26
Cremen, G., and Galasso, C. (0120). Earthquake early warning: Recent advances and perspectives Earth-Science Reviews, 205. Elsevier B.V. doi:10.1016/j.earscirev.2020.103184
D’Alessandro, A., D’Anna, R., Greco, L., Passafiume, G., Scudero, S., Speciale, S., et al. (2018). Monitoring earthquake through MEMS sensors (MEMS project) in the town of acireale (Italy) In 5th IEEE international Symposium on inertial Sensors and systems. Göttingen, Germany: INERTIAL 2018 - Proceedings, 1–4. doi:10.1109/ISISS.2018.8358143
D’Alessandro, A., Luzio, D., and D’Anna, G. (2014). Urban MEMS based seismic network for post-earthquakes rapid disaster assessment. Adv. Geosci. 40, 1–9. doi:10.5194/adgeo-40-1-2014
D’Alessandro, A., Scudero, S., Vitale, G., di Benedetto, A., and lo Bosco, G. (2020). “Optimization of low-cost monitoring systems for on-site earthquake early-warning of critical infrastructures,” in International conference on computational science and its applications, 963–975.
Ding, W., Liao, C., and Wang, H. (2017). MEMS-based seismic intensity instrument for earthquake early warning. Int. J. Comput. Sci. Eng. 15 (1–2), 41–48. doi:10.1504/IJCSE.2017.085978
earthquake-turnkey (2020). TurnKey earthquake early warning. Available at: https://earthquake-turnkey.eu/the-project/.
Faulkner, M., Olson, M., Chandy, R., Krause, J., Chandy, K. M., and Krause, A. (2011). Demo abstract, the next big one: Detecting earthquakes and other rare events from community-based sensors. Proc. 10th ACM/IEEE Int. Conf. Inf. Process. Sens. Netw. IPSN’11, 121–122.
Finazzi, F., and Fasso, A. (2017). A statistical approach to crowdsourced smartphone-based earthquake early warning systems. Stoch. Environ. Res. Risk Assess. 31 (7), 1649–1658. doi:10.1007/s00477-016-1240-8
Finazzi, F. (2020). The earthquake network project: A platform for earthquake early warning, rapid impact assessment, and search and rescue. Front. Earth Sci. (Lausanne). 8. doi:10.3389/feart.2020.00243
Finazzi, F. (2016). The earthquake network project: Toward a crowdsourced smartphone-based earthquake early warning system. Bull. Seismol. Soc. Am. 106 (3), 1088–1099. doi:10.1785/0120150354
Fischer, J., Redlich, J. P., Zschau, J., Milkereit, C., Picozzi, M., Fleming, K., et al. (2012). A wireless mesh sensing network for early warning. J. Netw. Comput. Appl. 35 (2), 538–547. doi:10.1016/j.jnca.2011.07.016
Fleming, K., Picozzi, M., Milkereit, C., Kuhnlenz, F., Lichtblau, B., Fischer, J., et al. (2009). The self-organizing seismic early warning information network (SOSEWIN). Seismol. Res. Lett. 80 (5), 755–771. doi:10.1785/gssrl.80.5.755
Flick, Uwe (2007). “Analyzing qualitative data,” in Designing qualitative research, 1 oliver’s yard, 55 city road (London England EC1Y 1SP United Kingdom: SAGE Publications, Ltd), 100–108. doi:10.4135/9781849208826.n10
Franchi, F., Marotta, A., Rinaldi, C., Graziosi, F., and D’Errico, L. (2019). IoT-based disaster management system on 5G uRLLC network. doi:10.1109/ICT-DM47966.2019.9032897
Hernon, P. (2004). The sage encyclopedia of social science research methods. Libr. Inf. Sci. Res. 26 (3), 404–406. doi:10.1016/j.lisr.2004.02.002
Hong, H., Ni, L., and Sun, H. (2021). Application and prospect of MEMS technology to geophysics Proceedings of the 16th Annual IEEE International Conference on Nano/Micro Engineered and Molecular Systems NEMS, 2021, 1863–1866. doi:10.1109/NEMS51815.2021.9451455
Hoshiba, M., and Aoki, S. (2015). Numerical shake prediction for earthquake early warning: Data assimilation, real-time shake mapping, and simulation of wave propagation. Bull. Seismol. Soc. Am. 105 (3), 1324–1338. doi:10.1785/0120140280
Hoshiba, M. (2013). Real-time prediction of ground motion by Kirchhoff-Fresnel boundary integral equation method: Extended front detection method for Earthquake Early Warning. J. Geophys. Res. Solid Earth 118, 1038–1050. doi:10.1002/jgrb.50119
Hoshiba, M. (2021). Real-time prediction of impending ground shaking: Review of wavefield-based (Ground-Motion-Based) method for earthquake early warning. Front. Earth Sci. LausanneFrontiers Media S.A., Sep. 922. doi:10.3389/feart.2021.722784
Hsu, T.-Y., and Nieh, C. P. (2020). On-site earthquake early warning using smartphones. Sensors Switz. 20 (10), 2928. doi:10.3390/s20102928
Hsu, T.-Y., Yin, R.-C., and Wu, Y.-M. (2018). Evaluating post-earthquake building safety using economical MEMS seismometers. Sensors Switz. 18 (5), 1437. doi:10.3390/s18051437
Hsu, T. Y., Wu, Y. M., and Chien, C. C. (2018). “How well can we evaluate post-earthquake building safety using low-cost MEMS seismometers,” in Proceedings of the 7th asia-pacific workshop on structural health monitoring, 866–877. [Online]. Available: https://www.scopus.com/inward/record.uri?eid=2-s2.0-85064651814&partnerID=40&md5=757b316b29165982ab11be41fe24f27d.APWSHM
Hu, X.-X., Wang, X. Z., Chen, B., Li, C. H., Tang, Y. X., Shen, X. Y., et al. (2021). Improved resolution and cost performance of low‐cost mems seismic sensor through parallel acquisition. Sensors 21, 1–18. doi:10.3390/s21237970
Huang, X., Lee, J., Kwon, Y.-W., and Lee, C.-H. (2020). “CrowdQuake: A networked system of low-cost sensors for earthquake detection via deep learning,” in Proceedings of the ACM SIGKDD international conference on knowledge discovery and data mining, 3261–3271. doi:10.1145/3394486.3403378
Jan, J. C., Huang, H.-H., Wu, Y.-M., Chen, C.-C., and Lin, C.-H. (75032018). Near-real-time estimates on earthquake rupture directivity using near-field ground motion data from a dense low-cost seismic network. Geophys. Res. Lett. 45 (15), 7496–7503. doi:10.1029/2018GL078262
Kanamori, H., Hauksson, E., and Heaton, T. (1997). Real-time seismology and earthquake hazard mitigation. Nature 390 (6659), 461–464. doi:10.1038/37280
Khan, I. (2020). “Collaborative earthquake detection and response using smart devices,” in Proceedings of the IEEE/ACM 7th international conference on mobile software engineering and systems, 36–37. doi:10.1145/3387905.3388602
Kobayashi, M. (2014). Experience of infrastructure damage caused by the Great East Japan Earthquake and countermeasures against future disasters. IEEE Commun. Mag. 52 (3), 23–29. doi:10.1109/MCOM.2014.6766080
Kodera, Y. (2018). Real-time detection of rupture development: Earthquake early warning using P waves from growing ruptures. Geophys. Res. Lett. 45 (1), 156–165. doi:10.1002/2017GL076118
Kodera, Y., Yamada, Y., Hirano, K., Tamaribuchi, K., Adachi, S., Hayashimoto, N., et al. (2018). The propagation of local undamped motion (PLUM) method: A simple and robust seismic wavefield estimation approach for earthquake early warning. Bull. Seismol. Soc. Am. 108 (2), 983–1003. doi:10.1785/0120170085
Kohler, M. D., Cochran, E. S., Given, D., Guiwits, S., Neuhauser, D., Henson, I., et al. (2018). Earthquake early warning shakealert system: West coast wide production prototype. Seismol. Res. Lett. 89 (1), 99–107. doi:10.1785/0220170140
Kong, Q., Allen, R. M., Schreier, L., and Kwon, Y. W. (2016). MyShake: A smartphone seismic network for earthquake early warning and beyond. Sci. Adv. 2 (2), e1501055–e1501059. doi:10.1126/sciadv.1501055
Kong, Q., Allen, R. M., and Schreier, L. (95942016). MyShake: Initial observations from a global smartphone seismic network. Geophys. Res. Lett. 43 (18), 9588–9594. doi:10.1002/2016GL070955
Kong, Q., Inbal, A., Allen, R. M., Lv, Q., and Puder, A. (2019). Machine learning aspects of the myshake global smartphone seismic network. Seismol. Res. Lett. 90 (2), 546–552. doi:10.1785/0220180309
Kong, Q., Kwon, Y.-W., Schreierz, L., Allen, S., Allen, R., and Strauss, J. (2015). 15th international conference on innovations for community services (I4CS), 1–8.
Kong, Q., Lv, Q., and Allen, R. M. (2019). “Earthquake early warning and beyond: Systems challenges in smartphone-based seismic network,” in Proceedings of the 20th international workshop on mobile computing systems and applications, 57–62.
Kong, Q., Martin-Short, R., and Allen, R. M. (2020). Toward global earthquake early warning with the myshake smartphone seismic network, part 1: Simulation platform and detection algorithm. Seismol. Res. Lett. 91 (4), 2206–2217. doi:10.1785/0220190177
Kong, Q., Martin‐Short, R., and Allen, R. M. (2020). Toward global earthquake early warning with the MyShake smartphone seismic network, Part 2: Understanding MyShake performance around the world. Seismol. Res. Lett. 91 (4), 2218–2233. doi:10.1785/0220190178
Kuyuk, H. S., and Allen, R. M. (2013). A global approach to provide magnitude estimates for earthquake early warning alerts. Geophys. Res. Lett. 40 (24), 6329–6333. doi:10.1002/2013GL058580
Kwon, Y. W., Ahn, J. K., Lee, J., and Lee, C. H. (2020). “Earthquake early warning using low-cost MEMS sensors,” in International geoscience and remote sensing symposium (Waikoloa, HI: IGARSS), 6635–6637. doi:10.1109/IGARSS39084.2020.9323438
Lee, J., Khan, I., Choi, S., and Kwon, Y.-W. (2019). A smart iot device for detecting and responding to earthquakes. Electron. Switz. 8, 1–19. doi:10.3390/electronics8121546
Lee, J., Kim, J.-S., Choi, S., and Kwon, Y.-W. (20190120). A smart device using low-cost sensors to detect earthquakes IEEE international conference on big data and smart computing (BigComp), big data and smart computing (BigComp), 2019 IEEE international conference on. IEEE, 1. –4. doi:10.1109/BIGCOMP.2019.8679190
Minson, S. E., Brooks, B. A., Glennie, C. L., Murray, J. R., Langbein, J. O., Owen, S. E., et al. (2015). Crowdsourced earthquake early warning. Sci. Adv. 1 (3), e1500036. doi:10.1126/sciadv.1500036
Mittal, H., Wu, Y.-M., Sharma, M. L., Yang, B. M., and Gupta, S. (2019). Testing the performance of earthquake early warning system in northern India. Acta Geophys. 67 (1), 59–75. doi:10.1007/s11600-018-0210-6
Myers M. D., (2008). Prepared for U.S. Geological Survey and the Advanced National Seismic System National Implementation Committee instrumentation guidelines for the advanced national seismic system open-file report 2008–1262.
Nakayachi, K., Becker, J. S., Potter, S. H., and Dixon, M. (2019). Residents’ reactions to earthquake early warnings in Japan. Risk Anal. 39 (8), 1723–1740. doi:10.1111/risa.13306
Nof, R. N., Chung, A. I., Rademacher, H., Dengler, L., and Allen, R. M. (2019). MEMS accelerometer mini-array (mama): A low-cost implementation for earthquake early warning enhancement. Earthq. Spectra 55 (1), 21–38. doi:10.1193/021218EQS036M
Peng, C., Chen, Y., Chen, Q., Yang, J., Wang, H., Zhu, X., et al. (2017). A new type of tri-axial accelerometers with high dynamic range MEMS for earthquake early warning. Comput. Geosci. 100, 179–187. doi:10.1016/j.cageo.2017.01.001
Peng, C., Jiang, P., Chen, Q., Ma, Q., and Yang, J. (2019). Performance evaluation of a dense MEMS-based seismic sensor array deployed in the Sichuan-Yunnan border region for earthquake early warning. Micromachines (Basel) 10 (11), 735. doi:10.3390/mi10110735
Peng, C., Jiang, P., Ma, Q., Wu, P., Su, J., Zheng, Y., et al. (2021). Performance evaluation of an earthquake early warning system in the 2019-2020 M6.0 changning, sichuan, China, seismic sequence. Front. Earth Sci. Lausanne 9, 1–13. doi:10.3389/feart.2021.699941
Peng, C., Ma, Q., Jiang, P., Huang, W., Yang, D., Peng, H., et al. (2020). Performance of a hybrid demonstration earthquake early warning system in the sichuan-yunnan border region. Seismol. Res. Lett. 91, 835–846. doi:10.1785/0220190101
Peng, C., Zhu, X., Yang, J., Xue, B., and Chen, Y. (2013). Development of an integrated onsite earthquake early warning system and test deployment in Zhaotong, China. Comput. Geosci. 56, 170–177. doi:10.1016/j.cageo.2013.03.018
Picozzi, M., Emolo, A., Martino, C., Zollo, A., Miranda, N., Verderame, G., et al. Earthquake early warning system for schools: A feasibility study in southern Italy, ” Seismol. Res. Lett., vol. 86, no. 2A, pp. 398–412. 2015, doi:10.1785/0220140194
Prasanna, R., Chandrakumar, C., Nandana, R., Holden, C., Punchihewa, A., Becker, J. S., et al. (2022). ‘Saving precious seconds’—a novel approach to implementing a low-cost earthquake early warning system with node-level detection and alert generation. InformaticsMar 9 (1), 25. doi:10.3390/informatics9010025
Strauss, J. A., and Allen, R. M. (2016). Benefits and costs of earthquake early warning. Seismol. Res. Lett. 87 (3), 765–772. doi:10.1785/0220150149
Strauss, J. A., Kong, Q., Pothan, S., Thompson, S., Mejia, R. F., Allen, S., et al. (2020). MyShake citizen seismologists help launch dual-use seismic network in California. Front. Commun. (Lausanne). 5. doi:10.3389/fcomm.2020.00032
Taale, A., Ventura, C. E., and Marti, J. (2021). On the feasibility of IoT-based smart meters for earthquake early warning. Earthq. Spectra 37 (3), 2066–2083. doi:10.1177/8755293020981964
Tan, M. L., Becker, J. S., Stock, K., Prasanna, R., Brown, A., Kenney, C., et al. (2022). Understanding the social aspects of earthquake early warning: A literature review. Front. Commun. Lausanne 7. doi:10.3389/fcomm.2022.939242
Uga, T., Nagaosa, T., and Kawashima, D. (2012). 12th international conference on ITS telecommunications, 837–842.An emergency earthquake warning system using mobile terminals with a built-in accelerometer
Velazquez, O., Pescaroli, G., Cremen, G., and Galasso, C. (2020). A review of the technical and socio-organizational components of earthquake early warning systems. Front. Earth Sci. Lausanne 8. doi:10.3389/feart.2020.533498
Wang, C. Y., Huang, T. C., and Wu, Y. M. (2022). Using LSTM neural networks for onsite earthquake early warning. Seismol. Res. Lett. 93, 814–826. doi:10.1785/0220210197
Wang, K.-S., Mittal, H., Wu, Y.-M., and Chao, W.-A. (2018). Building effects on the p-alert-based real-time shaking map determination. Seismol. Res. Lett. 89 (6), 2314–2321. doi:10.1785/0220170252
Won, J., Park, J., Park, J.-W., and Kim, I. (2020). Bleseis: Low-cost iot sensor for smart earthquake detection and notification. Sensors Switz. 20 (10), 2963. doi:10.3390/s20102963
Wu, A., Lee, J., Khan, I., and Kwon, Y. W. (2021). CrowdQuake+: Data-driven earthquake early warning via IoT and deep learning Proceedings - 2021 IEEE International Conference on Big Data, 2021, 2068–2075. doi:10.1109/BigData52589.2021.9671971Big Data
Wu, B.-R., Hsiao, N. C., Lin, P. Y., Hsu, T. Y., Chen, C. Y., Huang, S. K., et al. (2017). An integrated earthquake early warning system and its performance at schools in Taiwan. J. Seismol. 21 (1), 165–180. doi:10.1007/s10950-016-9595-3
Wu, Y.-M., and Mittal, H. (2021). A review on the development of earthquake warning system using low-cost sensors in taiwan. Sensors (Basel) 21 (22), 7649. doi:10.3390/s21227649
Wu, Y., Chen, D. Y., Lin, T. L., Hsieh, C. Y., Chin, T. L., Chang, W. Y., et al. (2013). A high‐density seismic network for earthquake early warning in Taiwan based on low cost sensors. Seismol. Res. Lett. 84 (6), 1048–1054. doi:10.1785/0220130085
Wu, Y. M., Chen, D. Y., Lin, T. L., Hsieh, C. Y., Chin, T. L., Chang, W. Y., et al. (2013). A high-density seismic network for earthquake early warning in Taiwan based on low cost sensors. Seismol. Res. Lett. 84 (6), 1048–1054. doi:10.1785/0220130085
Wu, Y. M., Kanamori, H., Allen, R. M., and Hauksson, E. (2007). Determination of earthquake early warning parameters, τcandPd, for southern California. Geophys. J. Int. 170 (2), 711–717. doi:10.1111/j.1365-246X.2007.03430.x
Wu, Y. M., and Zhao, L. (2006). Magnitude estimation using the first three seconds P-wave amplitude in earthquake early warning. Geophys. Res. Lett. 33 (16), L16312–L16317. doi:10.1029/2006GL026871
Yang, B. M., Huang, T.-C., and Wu, Y.-M. (2018). Shakingalarm: A nontraditional regional earthquake early warning system based on time-dependent anisotropic peak ground-motion attenuation relationships. Bull. Seismol. Soc. Am. 108 (3), 1219–1230. doi:10.1785/0120170105
Yang, B. M., Mittal, H., and Wu, Y.-M. (2021). Real-time production of PGA, PGV, intensity, and sa shakemaps using dense MEMS-based sensors in taiwan. Sensors 21 (3), 943. doi:10.3390/s21030943
Yin, R.-C., Wu, Y.-M., and Hsu, T.-Y. (2016). Application of the low-cost MEMS-type seismometer for structural health monitoring: A pre-study Conference Record - IEEE Instrumentation and Measurement Technology Conference, 2016. doi:10.1109/I2MTC.2016.7520389
Zambrano, A. M., Perez, I., Palau, C., and Esteve, M. (2015). Distributed sensor system for earthquake early warning based on the massive use of low cost accelerometers. IEEE Lat. Am. Trans. 13 (1), 291–298. doi:10.1109/TLA.2015.7040661
Zambrano, A. M., Perez, I., Palau, C., and Esteve, M. (2017). Technologies of internet of Things applied to an earthquake early warning system. Future Gener. Comput. Syst. 75, 206–215. doi:10.1016/J.FUTURE.2016.10.009
Zambrano, A., Perez, I., Palau, C., and Esteve, M. (2014). Quake detection system using smartphone-based wireless sensor network for early warning IEEE international conference on pervasive computing and communication workshops. Budapest, Hungary: PERCOM WORKSHOPS, 297–302. 2014, doi:10.1109/PerComW.2014.6815221
Zhao, Y., Deng, P., Liu, J., Wang, M., and Wan, J. (2021). LCANet: Lightweight context-aware attention networks for earthquake detection and phase-picking on IoT edge devices. IEEE Syst. J. 16, 4024–4035. doi:10.1109/JSYST.2021.3114689
Zou, Z.-X., Zhang, M., He, X.-D., Lin, S.-F., Dong, Z.-Y., and Sun, K. (2019). Seismic monitoring network based on mems sensors. Earthq. Sci. 32 (3–4), 179–185. doi:10.29382/eqs-2019-0179-08
Appendix
Keywords: earthquake early warning (EEW), low-cost seismometers, MEMS, warning systems, systematic literature review (SLR), earthquake resilience, earthquake detection
Citation: Chandrakumar C, Prasanna R, Stephens M and Tan ML (2022) Earthquake early warning systems based on low-cost ground motion sensors: A systematic literature review. Front. Sens. 3:1020202. doi: 10.3389/fsens.2022.1020202
Received: 15 August 2022; Accepted: 04 October 2022;
Published: 03 November 2022.
Edited by:
Edmundo Monteiro, University of Coimbra, PortugalReviewed by:
Wento Zhang, Institute of Semiconductors (CAS), ChinaHimanshu Mittal, Ministry of Earth Sciences, India
Francesco Finazzi, University of Bergamo, Italy
Copyright © 2022 Chandrakumar, Prasanna, Stephens and Tan. This is an open-access article distributed under the terms of the Creative Commons Attribution License (CC BY). The use, distribution or reproduction in other forums is permitted, provided the original author(s) and the copyright owner(s) are credited and that the original publication in this journal is cited, in accordance with accepted academic practice. No use, distribution or reproduction is permitted which does not comply with these terms.
*Correspondence: Chanthujan Chandrakumar, Y2NoYW5kcmEyQG1hc3NleS5hYy5ueg==