- 1Department of Neurosurgery, Tianjin Medical University General Hospital, Tianjin, China
- 2Tianjin Neurological Institute, Key Laboratory of Post Neuro-Injury Neuro-Repair and Regeneration in Central Nervous System, Ministry of Education, Tianjin, China
- 3Department of Clinical Laboratory, Tianjin Medical University General Hospital, Tianjin, China
- 4Department of Infectious Diseases, Tianjin Medical University General Hospital, Tianjin, China
Background: Pulmonary infection caused by multidrug-resistant Acinetobacter baumannii (MDR-AB) is a common and serious complication after brain injury. There are no definitive methods for its prediction and it is usually accompanied by a poor prognosis. This study aimed to construct and evaluate a nomogram based on patient data from the neurosurgical intensive care unit (NSICU) to predict the probability of MDR-AB pulmonary infection.
Methods: In this study, we retrospectively collected patient clinical profiles, early laboratory test results, and doctors’ prescriptions (66 variables). Univariate and backward stepwise regression analyses were used to screen the variables to identify predictors, and a nomogram was built in the primary cohort based on the results of a logistic regression model. Discriminatory validity, calibration validity, and clinical utility were evaluated using validation cohort 1 based on receiver operating characteristic curves, calibration curves, and decision curve analysis (DCA). For external validation based on predictors, we prospectively collected information from patients as validation cohort 2.
Results: Among 2115 patients admitted to the NSICU between December 1, 2019, and December 31, 2021, 217 were eligible for the study, including 102 patients with MDR-AB infections (102 cases) and 115 patients with other bacterial infections (115 cases). We randomly categorized the patients into the primary cohort (70%, N=152) and validation cohort 1 (30%, N=65). Validation cohort 2 consisted of 24 patients admitted to the NSICU between January 1, 2022, and March 31, 2022, whose clinical information was prospectively collected according to predictors. The nomogram, consisting of only six predictors (age, NSICU stay, Glasgow Coma Scale, meropenem, neutrophil to lymphocyte ratio, platelet to lymphocyte ratio), had significantly high sensitivity and specificity (primary cohort AUC=0.913, validation cohort 1 AUC=0.830, validation cohort 2 AUC=0.889) for early identification of infection and had great calibration (validation cohort 1,2 P=0.3801, 0.6274). DCA confirmed that the nomogram is clinically useful.
Conclusion: Our nomogram could help clinicians make early predictions regarding the onset of pulmonary infection caused by MDR-AB and implement targeted interventions.
Introduction
Acinetobacter spp. are key pathogens in nosocomial infections. In recent decades, Acinetobacter baumannii (AB) infection has become a global clinical challenge. Carbapenems are the treatment of choice for Acinetobacter infections, especially in critically ill patients (Chakravarty, 2020). With the advent of the multidrug resistance era, the prevalence of carbapenem-resistant AB has increased. Consequently, the World Health Organization (WHO) has ranked it as the world’s top pathogen, urgently requiring novel antibiotics (World Health Organization, 2017). The emergence of multidrug-resistant Acinetobacter baumannii (MDR-AB) poses greater challenges for clinical treatment. Almost all of these strains are resistant to commonly used antibiotics to varying degrees; hence, infections caused by such strains are difficult to eradicate (Ibrahim et al., 2021). Currently, MDR-AB can cause pulmonary, bloodstream, central nervous system, urinary tract, and abdominal infections; the most common of these are pulmonary infections (Kengkla et al., 2017).
Recent data show that the overall prevalence of multidrug resistant strains in patients with hospital-acquired pneumonia (HAP) and ventilator-associated pneumonia (VAP) caused by AB is approximately 79.9%, and the overall mortality rate can be as high as 56.2% (Lim et al., 2019). The annual incidence of pneumonia caused by MDR-AB in intensive care units (ICU) is increasing. Effectively controlling its incidence has attracted extensive attention from medical institutions (Russo et al., 2021).
Nomograms are predictive tools that create simple graphical representations of statistical predictive models and are used to generate numerical probabilities of clinical events (Cahlon et al., 2012). In recent years, studies using nomograms to predict the occurrence and prognosis of diseases have emerged. Nomograms have been constructed to predict pulmonary, bloodstream, urinary tract, and surgical wound infections (Tien et al., 2018; Chen et al., 2022; Yan et al., 2022). Zhang et al. conducted a retrospective study in which they developed a nomogram to predict the risk of acquiring carbapenem-resistant microorganism infections in patients admitted to the ICU for the first time (Zhang et al., 2022). With the coronavirus disease 2019 (COVID-19) affecting the world, Gong et al. constructed a nomogram for early identification of patients at risk of severe COVID-19 (Gong et al., 2020). However, nomograms predicting the risk of pulmonary infection caused by MDR-AB have received little attention. Based on the validation of traditional risk factors, we conducted a retrospective and prospective study to identify novel risk factors and their associated effects based on neurosurgical ICU (NSICU) profiles. Specifically, we created a scale to evaluate the probability of MDR-AB pneumonia in patients undergoing critical neurosurgical care, thereby guiding clinical practice.
Materials and methods
Patient recruitment
This study included patients admitted to the NSICU of Tianjin Medical University General Hospital (the highest-level hospital located in the center of Tianjin, China) between December 1, 2019, and December 31, 2021. Patients with the following conditions were excluded: non-infected or colonized patients; hospitalization for less than 7 days; diseases that severely affect blood chemistry indexes (such as leukemia, severe renal failure, severe liver failure, etc.), and cases with missing medical records and subject to medical disputes. Next, we prospectively collected information on patients admitted to the NSICU between January 1, 2022, and March 31, 2022, based on predictors (Figure 1).
Based on the draft standardized international terminology for multidrug resistant (MDR), extensively-drug resistant (XDR), and pan-drug resistant (PDR) bacteria proposed by experts from the United States and other countries in 2010, Magiorakos and other experts officially published the interim standard definitions of MDR, XDR, and PDR (Magiorakos et al., 2012). The categories of antimicrobials include aminoglycosides (e.g., gentamicin, tobramycin, or amikacin), antipseudomonal carbapenems (e.g., imipenem or meropenem), antipseudomonal fluoroquinolones (e.g., ciprofloxacin or levofloxacin), antipseudomonal penicillins + β-lactamase inhibitors (e.g., piperacillin-tazobactam or ticarcillin-clavulanic acid), extended-spectrum cephalosporins (e.g., ceftazidime, ceftriaxone, or cefepime), folate pathway inhibitors (e.g., trimethoprim-sulfamethoxazole), penicillin + β-lactamase inhibitors (e.g., ampicillin-sulbactam), polymyxins (e.g., colistin or polymyxin B), and tetracyclines (e.g., tetracycline, doxycycline, or minocycline). The criteria for MDR, XDR and PDR were defined based on the non-susceptibility to the above categories. The MDR criteria for Acinetobacter spp. was defined as acquired non-susceptibility to at least one agent from three or more antimicrobial categories listed above. XDR was defined as non-susceptibility to at least one agent from all but two or fewer antimicrobial categories. PDR was defined as non-susceptibility to all agents in all of the above antimicrobial categories. Therefore, a bacterial isolate characterized as XDR would also be characterized as MDR. Similarly, a bacterial isolate must be XDR to be further defined as PDR (Magiorakos et al., 2012).
The patients were divided into an “MDR-AB pneumonia group” and an “other bacterial pneumonia group.” The inclusion criteria for the MDR-AB pneumonia group (diagnostic criteria) were as follows: (1) clinical symptoms and signs consistent with pneumonia (fever >38°C, increased white blood cell count to >12 × 109, cough, thick sputum with pulmonary rales, dyspnea, etc.) and new, progressive, or persistent pulmonary infiltrates and/or solid lesions on imaging (Klompas et al., 2012); and (2) positive sputum culture results: two or more sputum cultures showing pure MDR-AB growth or MDR-AB dominant growth (Horan et al., 2008). The inclusion criteria for the other bacterial pneumonia group were as follows: (1) clinical symptoms, signs, and imaging changes compatible with pneumonia; and (2) sputum culture showing growth of other bacteria or dominant growth of other bacteria. The culture results included more than 15 species of bacteria, including Neisseria, Klebsiella pneumoniae, Pseudomonas aeruginosa, and Staphylococcus (epidermidis, aureus, and hemolysis), except AB. The respiratory specimens collected included endotracheal aspiration (ETA), bronchoalveolar lavage fluid (BALF), and a protected specimen brush (PSB), in addition to the patient’s sputum. They were acquired from the patient’s lower respiratory tract and were fresh, yellow, purulent, and not contaminated by the upper respiratory tract. The qualifying criteria for a respiratory specimen were <10 epithelial cells and ≥ 25 leukocytes per microscopic field (Heineman et al., 1977).
Data collection
We retrospectively collected the patient’s data, including (1) patient demographics, such as sex, age, and underlying disease; (2) major diagnoses and comorbidities; (3) total duration of hospitalization and NSICU stay; (4) relevant scores and nursing status; (5) invasive operations; (6) ventilator usage time and whether tracheal intubation or tracheotomy was performed; and (7) application of antibiotics. For the MDR-AB pneumonia group, the type of antibiotic applied before the patient contracted MDR-AB pneumonia was recorded. For the other bacterial infection group, the type of antibiotic administered during the patient’s hospitalization was recorded; (8) early hospitalization (3–7 days after admission) blood test indicators such as white blood cell count (WBC), platelet count (PLT), absolute neutrophil count (NEU), absolute lymphocyte count (LYM), absolute monocyte count (MON), hemoglobin (HGB), globulin (GLO), albumin (ALB), alanine transaminase (ALT), aspartate transaminase (AST), creatinine (CREA), neutrophil to lymphocyte ratio (NLR), lymphocyte to monocyte ratio (LMR), platelet to lymphocyte ratio (PLR), C-reactive protein (CRP), procalcitonin (PCT), D-dimer, and glucose (GLU). This study met medical ethics standards (Declaration of Helsinki). The retrospective collection of data from the patients and their analyses was approved by the ethics committee of Tianjin Medical University General Hospital.
Statistical analyses
We randomly categorized the patients retrospectively into the primary cohort (70%) and validation cohort 1 (30%). The data from the primary cohort were used to construct nomograms and the data from the validation cohort 1 were used for internal validation. These variables are presented as means [standard deviation (SD)] or medians [interquartile range (IQR)]. The independent samples t-test or Mann–Whitney U test was used to compare two independent groups. Categorical variables are presented as numbers (percentage), and their differences among groups were compared using the chi-square test or Fisher’s exact test. Parameters identified as significant (P<0.05) in univariate analysis were incorporated into multivariate logistic regression analysis using a backward stepwise regression (BSR) approach. Optimal model selection was performed using the likelihood-ratio test using the Akaike information criterion (AIC). The identified independent factors were analyzed again using logistic regression models, and the results were expressed as odds ratios (OR) and 95% confidence intervals (CI). Finally, we constructed a nomogram based on the results of a logistic regression model and conducted a Hosmer–Lemeshow (HL) test to evaluate the calibration. The area under the receiver operating characteristic curve (AUC) was used to assess the recognition ability of the nomogram. According to the Youden Index, receiver operating characteristic (ROC) curves were used to determine the optimal cut-off point and its sensitivity and specificity. Decision curve analysis (DCA) curves were used to assess the clinical applicability of the nomogram. In addition, we used prospectively collected patient data as validation cohort 2 to perform external validation of the nomogram we constructed. All tests were two-tailed, and a P-value <0.05 was regarded as statistically significant.
Statistical analyses were performed using the SPSS software (version 25.0), and the nomogram was constructed using the R programming language (version 4.2.0; http://www.r-project.org/). The packages in R used in this study were “MASS,” “survival,” “rms,” “rmda,” “car,” “Hmisc,” “pROC,” and “ResourceSelection.”
Results
Clinical characteristics
We retrospectively collected the clinical data of 2115 patients. Overall, 217 patients who met the criteria were enrolled in the study (102 in the MDR-AB pneumonia group and 115 in the other bacterial pneumonia group). Nineteen patients with XDR-AB and 0 patients with PDR-AB were included in the MDR-AB pneumonia group. Pulmonary infection prevalence caused by MDR-AB in the NSICU was as high as 4.82%, with XDR-AB pneumonia accounting for 18.6%. A total of 66 variables were collected for each patient. Table S1 presents the clinical characteristics of the two groups. The 217 patients were randomly divided into 152 and 65 patients in the primary cohort (MDR-AB pneumonia group, N=74; other bacterial pneumonia group, N=78) and validation cohort 1 (MDR-AB pneumonia group, N=28; other bacterial pneumonia group, N=37), respectively. The clinical characteristics of the two cohorts and a comparison of the randomization assignments are presented in Table 1. The results showed satisfactory similarity in the prevalence of MDR-AB pneumonia in both cohorts (P = 0.542). In addition, the clinical parameters, use of antibiotics, and early hospitalization blood test indicators were comparable between the cohorts (all P > 0.05). In summary, the parameters selected for this study were homogeneous and comparable across cohorts, indicating that the clinical data were reliably sourced and collected with quality assurance.
Variables selection using the BSR analysis
The BSR approach has a significant advantage in variable selection since it calculates all possible combinations of variables, and the final combination selected is the optimal combination based on the minimum AIC. In Table 1, the results of the univariate analysis show that 15 variables in the primary cohort were significantly different: total duration of hospitalization, NSICU stay, Glasgow Coma Scale (GCS) score, APACHE II score, ventilator usage time, tracheotomy, electronic bronchoscopy, lumbar puncture, meropenem, LYM, HGB, ALB, AST, NLR, and PLR (all P<0.05). We incorporated sex, age, and the 15 variables mentioned above in the BSR model. After eliminating the variables with poor predictive performance or multicollinearity, we screened six predictors. We then included them in a multivariable logistic regression, and the results showed that older age (OR=1.03; 95% CI=0.99–1.06), prolonged NSICU stay (OR=1.11; 95% CI=1.05–1.16), lower GCS score (OR=0.68; 95% CI=0.56–0.82), application of meropenem (OR=3.92; 95% CI=1.41–10.9), lower NLR (OR=0.90; 95% CI=0.83–0.98), and higher PLR (OR=1.01; 95% CI=1.00–1.02) were independent risk factors for pulmonary infections caused by MDR-AB (Table 2).
Construction, validation, and evaluation of the nomogram
As shown in Figure 2, we constructed a nomogram based on the multivariate logistic regression model. For the values of each predictor in the nomogram, a vertical line is drawn upward from each point, and the values of different variables can correspond to different scores on the topmost reference line (scores range from 0 to 100). The scores corresponding to each predictor were summed to obtain the total score, and a vertical line was dropped from the total point line to the bottom probability line to determine the probability of pulmonary infection caused by MDR-AB.
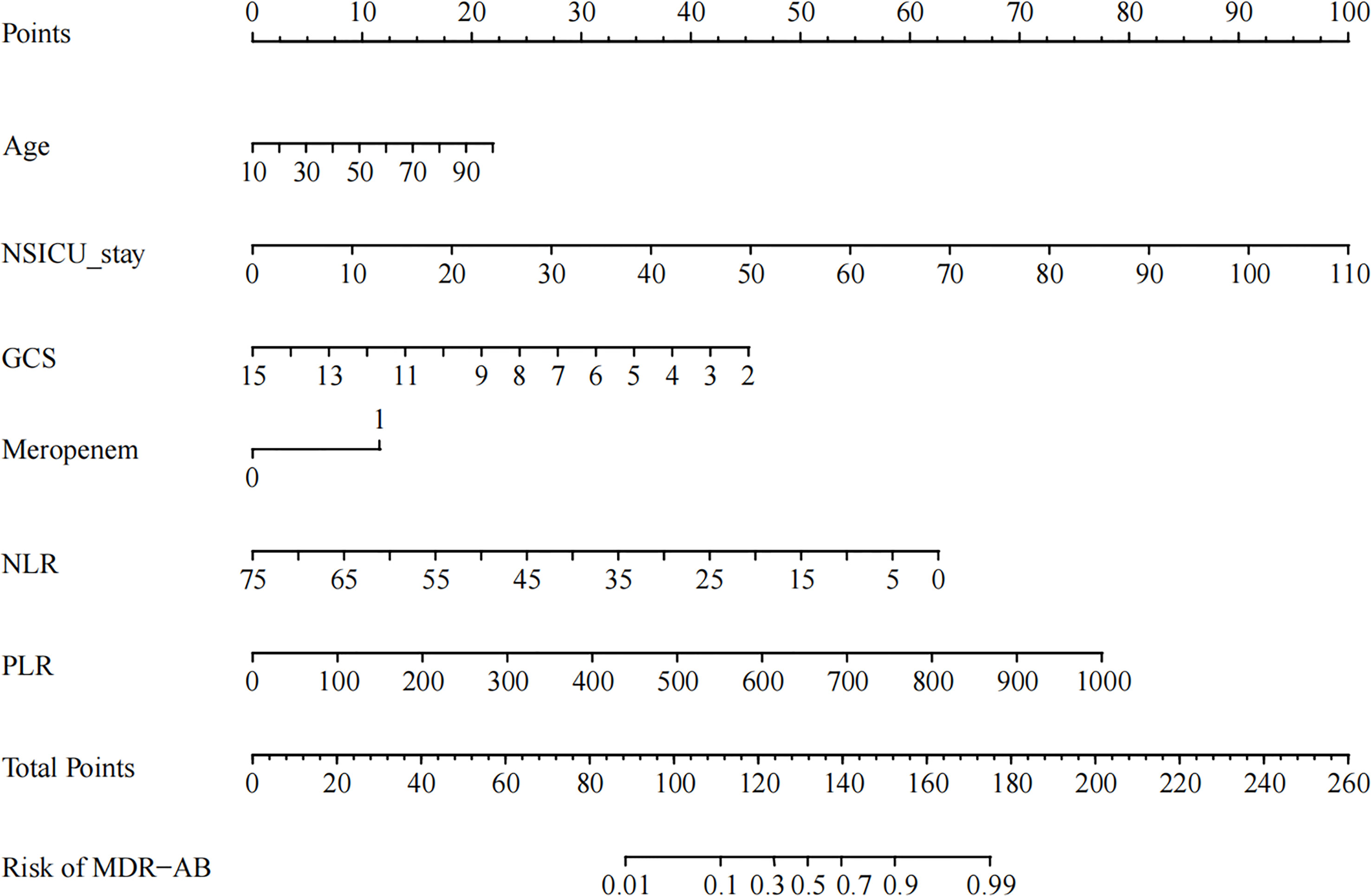
Figure 2 Example of prediction nomogram for the risk of pulmonary infection caused by multidrug-resistant Acinetobacter Baumannii (MDR-AB). NLR, neutrophil to lymphocyte ratio; PLR, platelet to lymphocyte ratio.
Figures 3A, B shows that the AUC of the nomogram was 0.913 (95% CI = 0.870–0.957) and 0.830 (95% CI = 0.728–0.932) for the primary cohort and validation cohort 1, respectively. In the primary cohort, the optimal cutoff for the nomogram was 0.384, with a sensitivity and specificity of 0.808 and 0.919, respectively. In validation cohort 1, the optimal cutoff was 0.520, sensitivity was 0.811, and specificity was 0.821. The above results show that our nomogram has good predictive ability.
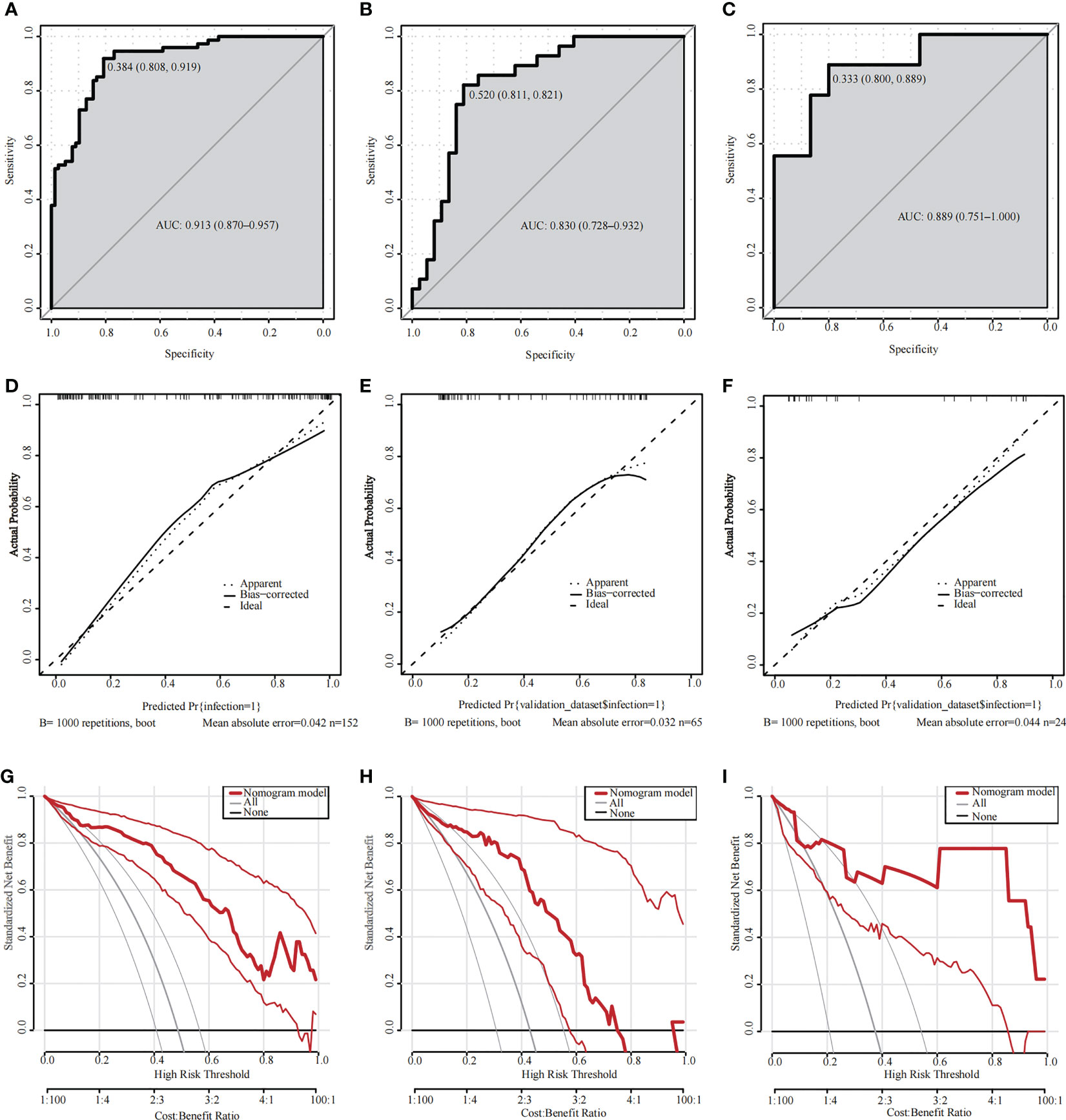
Figure 3 (A–C) ROC curves for validating the discrimination of the nomogram. (A) Primary cohort; (B) Validation cohort 1; (C) Validation cohort 2 (AUC=0.913 vs 0.830 vs 0.889). (D–F) Calibration curves for validating the calibration of the nomogram. (D) Primary cohort, HL test (χ2 = 0.3673 and P value=0.8322); (E) Validation cohort 1, HL test (χ2 = 0.2493 and P value=0.3801); (F) Validation cohort 2, HL test (χ2 = 0.93241 and P value=0.6274). (G–I) DCA curves for validating the net income of the nomogram. (G) Primary cohort; (H) Validation cohort 1; (I) Validation cohort 2. ROC, receiver operating characteristic; AUC, area under the ROC curve; HL, Hosmer–Lemeshow; DCA, Decision curve analysis.
Figures 3D, E shows that the calibration curves for the primary cohort and validation cohort 1 were similar to the 45-degree diagonal alignment. The HL test showed no significant difference (primary cohort: χ2 = 0.3673, P=0.8322; validation cohort 1: χ2 = 0.2493, P=0.3801), indicating that the nomogram had a satisfactory fit.
We then plotted a DCA curve to illustrate the value of the nomogram’s clinical application. Figures 3G, H shows that if the threshold probability is between 0.1 and 0.8 (in either cohort), the clinical intervention guided by the nomogram has a greater net benefit.
Finally, we prospectively collected data from 24 patients (MDR-AB pneumonia group, N=9; other bacterial pneumonia group, N=15) admitted to the NSICU as validation cohort 2 for external validation based on the six selected predictors (Table 3). The constructed nomogram had excellent discriminatory validity (Figure 3C, AUC=0.889), calibration validity (Figure 3F, HL test, P=0.6274), and clinical utility (Figure 3I, threshold probability, 0.1–1).
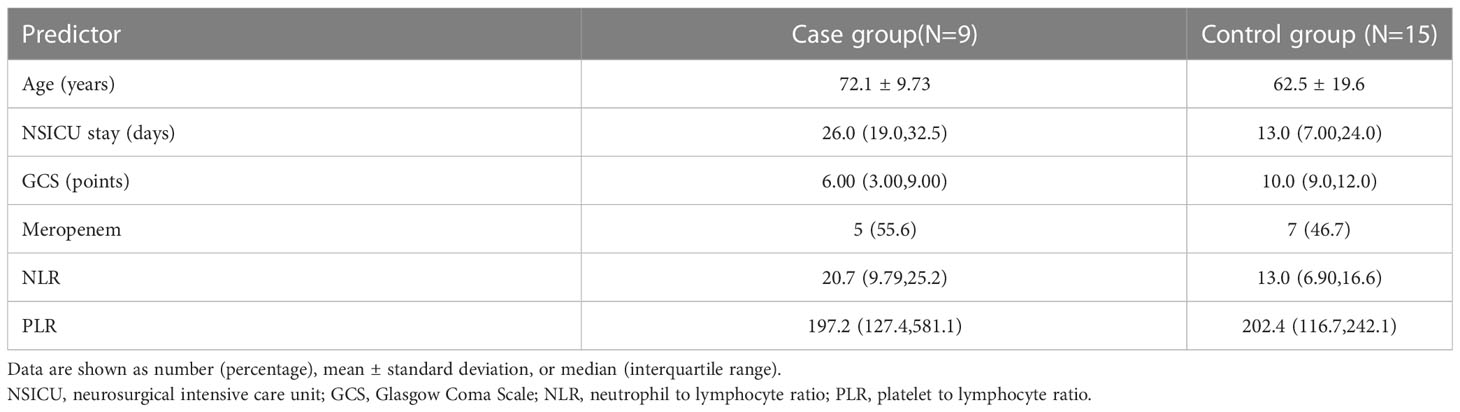
Table 3 Clinical characteristics of patients in the case and control groups in the validation cohort 2.
Discussion
In this study, conducted to identify novel risk factors of MDR-A-caused pulmonary infections in the NSICU and their associated effects, we found a very high incidence of pulmonary infections caused by MDR-AB in the NSICU and a high percentage of XDR-AB pneumonia among them. In addition to causing prolonged hospitalization and greatly increasing the economic burden on the patient’s family, the infections also cause complications and higher mortality rates (Zhen et al., 2021). Therefore, there is an urgent need for early detection and control of pulmonary infections caused by MDR-AB. Our study was based on the clinical data of NSICU patients, and the data were analyzed using an effective mathematical modeling approach. The final results indicated that older age, prolonged NSICU stay, lower GCS score, application of meropenem, lower NLR, and higher PLR were independent risk factors for pulmonary infection caused by MDR-AB. Subsequently, we developed a predictive nomogram consisting of these six predictors, with significantly high sensitivity and specificity for the early identification of infection (primary cohort AUC=0.913, validation cohort 1 AUC=0.830, validation cohort 2 AUC=0.889). DCA showed that the nomogram has great potential for clinical use. To the best of our knowledge, this is the first study to construct a nomogram for predicting the risk of pulmonary infection caused by MDR-AB.
To date, several studies have reported the risk factors for pulmonary infection due to single- and MDR-AB, all based on hospital-integrated ICU or pediatric ICUs (PICUs). Risk factors include the severity of the underlying disease, duration of hospital stay, duration of ICU stay, mechanical ventilation, invasive procedures, and prior antibiotic use (Munoz-Price and Weinstein, 2008; Cai et al., 2012; Zheng et al., 2013; Tsakiridou et al., 2014; Li et al., 2017; Ren et al., 2019). Some studies have established predictive models based on relevant clinical symptoms (such as anemia and hypoalbuminemia), clinical indicators (such as duration of hospitalization and ventilator usage), and biological indicators (such as LYM, NLR, and MLR), which can indicate the onset of pneumonia and even predict the prognosis of the patient (Seligman et al., 2006; Cataudella et al., 2017; Huang et al., 2018; Wu et al., 2021). Zhang et al. developed a nomogram from a single center that predicted the risk of death in patients with AB infection. This study included 10 factors from participants and did not involve laboratory indicators (Zhang et al., 2020). However, there are few studies on the early prediction of pulmonary infections caused by MDR-AB, and the research components are incomplete.
Most patients in the NSICU suffer from acute and critical diseases with relatively rapid progression and a high risk of deterioration. Neurosurgical disorders result in relatively poor consciousness and low physical activity in patients. In addition, it is challenging for many to be independent and have difficulty in actively cooperating with the clinical treatment. These factors contribute to the higher prevalence of AB in NSICUs (Ryu et al., 2017). Our study showed that older age significantly increased the risk of MDR-AB pneumonia. Compared with patients in other wards, the average age of patients admitted to the NSICU is higher, most have impaired consciousness including lethargy and coma, and they remain on bed rest for a longer period. Some patients also have symptoms, such as irritability and delirium, which cause difficulties in administering nursing care and treatment. However, in the primary cohort of this study, there was no statistically significant difference with respect to diseases such as hypertension, diabetes, coronary artery disease, and obsolete cerebral infarction. This suggests that the patient’s diagnosis and underlying disease at admission were not the primary cause of MDR-AB pulmonary infection, relative to patients with pulmonary infections caused by other bacteria. Moreover, it substantiates that case and control groups included in this study are comparable and that the experimental results are more authentic.
The GCS is an accessible physiological scoring system that can assess the degree of impaired consciousness in patients on admission (Alvarez et al., 1998). In our study, GCS was identified as a predictor of pulmonary infection caused by MDR-AB, and the risk of infection decreased with increasing GCS scores. AB is a conditionally pathogenic bacterium present in the respiratory and gastrointestinal tracts. A low GCS score indicates that the patient is in a poor state of consciousness, and brainstem reflexes may be delayed or absent. This can greatly prolong the patient’s time remaining bedridden, which in turn reduces the patient’s ability to expel sputum and ventilate, causing AB from the respiratory and gastrointestinal tracts to infect the lungs (Chen et al., 2013). With a prolonged stay in the NSICU, the immunity of elderly patients is reduced. If there are no effective nursing measures, such as turning over and expelling sputum in time, it could lead to hypostatic pneumonia (Ji et al., 2022).
Many studies have suggested that longer hospital stays can increase the risk of pulmonary infections in patients with AB (Munoz-Price and Weinstein, 2008). We did not confirm this notion in this study; however, we only found that pulmonary infection caused by MDR-AB was associated with a prolonged stay in the NSICU. This could be due to the fact that the infection exacerbates neurological symptoms and leaves the patient in a state of prolonged unconsciousness. The modes of transmission of AB include contact (direct and indirect), droplet, and airborne modes, and prolonged exposure of patients to the NSICU, which can significantly increase the chance of infection. Elderly patients admitted to the NSICU are at an increased risk of infection, as their immunity decreases throughout the disease course (Yakupogullari et al., 2016). Patients are transferred back to the general neurosurgery ward for further treatment after their condition improves. The degree of bacterial resistance and invasiveness in general wards is lower than that in ICUs, which can effectively prevent the occurrence of MDR-AB pulmonary infections (Donowitz et al., 1982).
The resistance mechanisms of AB to carbapenem antibiotics include the production of carbapenemase to hydrolyze the antibiotic drug, reduced affinity for penicillin-binding proteins, downregulation of outer membrane protein expression, and active excretion of the drug from the bacteria (Duraes et al., 2018). Related studies have shown that the use of carbapenems is a risk factor for infection with AB pneumonia (Falagas and Kopterides, 2006). Carbapenems include imipenem, meropenem, and biapenem; however, our study showed that the application of meropenem increased the risk of infection with MDR-AB pneumonia. This may be due to the low use of both imipenem and biexpenem in the departments where we conducted our study, making the amount of clinical data too small to be statistically meaningful. Neurosurgery is one of the most challenging and delicate procedures among all surgical systems. Most of the patients admitted are of advanced age with underlying diseases and are immunocompromised, with high susceptibility to postoperative intracranial infections (Xiao et al., 2022). Owing to the low success rate of bacterial cultures in cerebrospinal fluid (approximately 20%) and the high mortality rate of intracranial infections, meropenem is commonly used in the treatment of empirical central infections in post-neurosurgical patients, and its application rate in the clinic is increasing (Tunkel et al., 2017; Schneider et al., 2022). Our study found that meropenem application increased the risk of MDR-AB pneumonia, whereas all generations of cephalosporins and vancomycin were not associated with an increased risk. The use of meropenem should be controlled in clinical practice. For surgical patients, we can apply first or second-generation cephalosporins (cefuroxime, cefoxitin) for infection prevention in the perioperative period, and the course of treatment usually does not exceed 24 hours. The patient may be treated empirically with a third or fourth-generation cephalosporin (ceftriaxone, cefepime) instead of meropenem and combined with vancomycin first if the possibility of intracranial infection complications is considered. This allows for full coverage against both gram-positive and gram-negative bacteria. If the treatment is ineffective or after the sensitive antibiotic for the bacteria is confirmed by cerebrospinal fluid culture, meropenem can be replaced or added. In addition, empirical anti-infective therapy should be administered with cephalosporins or carbapenems, as appropriate, considering local epidemiologic data, as well as the distribution and resistance of pathogenic bacteria.
Inflammatory indicators such as NLR, LMR, and PLR, whose reliability and accessibility are established in almost all medical disciplines, can be used as markers of the immune response to various infectious and non-infectious stimuli (Russell et al., 2019). In this study, early NLR and PLR were screened as independent risk factors by stepwise regression and included in our nomogram. Although NLR was ultimately identified as a protective factor, it acted in conjunction with PLR in this nomogram to affect the final probability of infection.
The age and GCS score of the patient cannot be controlled by physicians. Relevant security authorities such as senior care facilities and traffic police can improve their measures and efficiency, focus on elderly patients, prevent the occurrence of traumatic brain injury, and reduce the severity of its symptoms. It is important for physicians to control the duration of hospitalization, standardize the use of meropenem, and pay attention to early inflammatory indicators to reduce the incidence of MDR-AB pulmonary infection.
This study has several strengths. Firstly, our study provides a convenient and practical quantitative prediction tool based on only six predictors that are readily available from routine clinical and blood tests. Secondly, the inclusion and exclusion criteria were rigorously developed for this study, and sufficient study subjects and data on factors were collected. Finally, our nomogram was validated extensively and showed good discrimination, calibration, and robust clinical utility and stability.
This study has some limitations: Firstly, this study retrospectively documented patient information from a single center for 2 years and did not use external data for validation. A multicenter study with a larger sample size is needed to validate the results of this study. Secondly, the predictive model we proposed was based on the NSICU of Tianjin Medical University General Hospital. This is an exploration of a quantitative model that may not be widely applicable to various disciplines, and the coefficients of the relevant factors in the model may vary from case to case.
In conclusion, our nomogram incorporated patient age, duration of NSICU stay, GCS, early NLR, and PLR. The occurrence of pulmonary infections caused by MDR-AB can be predicted at the early stage of hospitalization. According to the predicted results, it is possible to advise patients with sputum bacterial culture and drug susceptibility testing at an early stage. Switching from empirical treatment to targeted treatment before the diagnosis of MDR-AB pulmonary infection can improve patients’ recovery and survival rates. Overall, our study will help in early prediction and implementation of targeted interventions against pulmonary infection caused by MDR-AB for better patient management.
Data availability statement
The raw data supporting the conclusions of this article will be made available by the authors, without undue reservation.
Ethics statement
The study was approved by the Ethics Committee of Tianjin Medical University General Hospital. Owing to the retrospective nature of this study, there was no interference in the patients’ diagnosis and treatment, although a small amount included some prospectively collected clinical information of the patients for validation; therefore, obtaining informed consent from the patients was waived by the ethics committee. This study complied with the Declaration of Helsinki and adhered to the principles of medical ethics. The authors kept all patient information strictly confidential.
Author contributions
RJ and DW contributed substantially to the collection of literature, study design, data interpretation, and manuscript writing. RJ, JS, JL, and CP reviewed and revised the articles and performed the quality assessment. WJ, ML, MN, CW, TL, YC, JF, and SD performed data collection and statistical analyses. ZS, YF, and JY interpreted the data and studied the oversight. All authors contributed to the article and approved the submitted version.
Funding
This study was supported by the National Natural Science Foundation of China (Nos. 82071390, 82001323, and 82101434), Science and Technology Project of Tianjin (No. 19YFZCSY00650), and Tianjin Key Medical Discipline (Specialty) Construction Project.
Acknowledgments
We would like to thank all members of staff in the NSICU and medical record department for their support in this study.
Conflict of interest
The authors declare that the research was conducted in the absence of any commercial or financial relationships that could be construed as a potential conflict of interest.
Publisher’s note
All claims expressed in this article are solely those of the authors and do not necessarily represent those of their affiliated organizations, or those of the publisher, the editors and the reviewers. Any product that may be evaluated in this article, or claim that may be made by its manufacturer, is not guaranteed or endorsed by the publisher.
Supplementary material
The Supplementary Material for this article can be found online at: https://www.frontiersin.org/articles/10.3389/fcimb.2023.1152512/full#supplementary-material
References
Alvarez, M., Nava, J. M., Rue, M., Quintana, S. (1998). Mortality prediction in head trauma patients: performance of Glasgow coma score and general severity systems. Crit. Care Med. 26 (1), 142–148. doi: 10.1097/00003246-199801000-00030
Cahlon, O., Brennan, M. F., Jia, X., Qin, L. X., Singer, S., Alektiar, K. M. (2012). A postoperative nomogram for local recurrence risk in extremity soft tissue sarcomas after limb-sparing surgery without adjuvant radiation. Ann. Surg. 255 (2), 343–347. doi: 10.1097/SLA.0b013e3182367aa7
Cai, X. F., Sun, J. M., Bao, L. S., Li, W. B. (2012). Risk factors and antibiotic resistance of pneumonia caused by multidrug resistant acinetobacter baumannii in pediatric intensive care unit. World J. Emerg. Med. 3 (3), 202–207. doi: 10.5847/wjem.j.1920-8642.2012.03.008
Cataudella, E., Giraffa, C. M., Di Marca, S., Pulvirenti, A., Alaimo, S., Pisano, M., et al. (2017). Neutrophil-To-Lymphocyte ratio: an emerging marker predicting prognosis in elderly adults with community-acquired pneumonia. J. Am. Geriatr. Soc. 65 (8), 1796–1801. doi: 10.1111/jgs.14894
Chakravarty, B. (2020). Genetic mechanisms of antibiotic resistance and virulence in acinetobacter baumannii: background, challenges and future prospects. Mol. Biol. Rep. 47 (5), 4037–4046. doi: 10.1007/s11033-020-05389-4
Chen, B., Grothe, C., Schaller, K. (2013). Validation of a new neurological score (FOUR score) in the assessment of neurosurgical patients with severely impaired consciousness. Acta Neurochir (Wien) 155 (11), 2133–2139. doi: 10.1007/s00701-013-1854-2
Chen, Z., Zhong, M., Xu, Z., Ye, Q., Xie, W., Gao, S., et al. (2022). Development and validation of a nomogram based on geriatric nutritional risk index to predict surgical site infection among gynecologic oncology patients. Front. Nutr. 9. doi: 10.3389/fnut.2022.864761
Donowitz, L. G., Wenzel, R. P., Hoyt, J. W. (1982). High risk of hospital-acquired infection in the ICU patient. Crit. Care Med. 10 (6), 355–357. doi: 10.1097/00003246-198206000-00001
Duraes, F., Pinto, M., Sousa, E. (2018). Medicinal chemistry updates on bacterial efflux pump modulators. Curr. Med. Chem. 25 (42), 6030–6069. doi: 10.2174/0929867325666180209142612
Falagas, M. E., Kopterides, P. (2006). Risk factors for the isolation of multi-drug-resistant acinetobacter baumannii and pseudomonas aeruginosa: a systematic review of the literature. J. Hosp Infect. 64 (1), 7–15. doi: 10.1016/j.jhin.2006.04.015
Gong, J., Ou, J., Qiu, X., Jie, Y., Chen, Y., Yuan, L., et al. (2020). A tool for early prediction of severe coronavirus disease 2019 (COVID-19): a multicenter study using the risk nomogram in wuhan and guangdong, China. Clin. Infect. Dis. 71 (15), 833–840. doi: 10.1093/cid/ciaa443
Heineman, H. S., Chawla, J. K., Lopton, W. M. (1977). Misinformation from sputum cultures without microscopic examination. J. Clin. Microbiol. 6 (5), 518–527. doi: 10.1128/jcm.6.5.518-527.1977
Horan, T. C., Andrus, M., Dudeck, M. A. (2008). CDC/NHSN surveillance definition of health care-associated infection and criteria for specific types of infections in the acute care setting. Am. J. Infect. Control 36 (5), 309–332. doi: 10.1016/j.ajic.2008.03.002
Huang, Y., Liu, A., Liang, L., Jiang, J., Luo, H., Deng, W., et al. (2018). Diagnostic value of blood parameters for community-acquired pneumonia. Int. Immunopharmacol 64, 10–15. doi: 10.1016/j.intimp.2018.08.022
Ibrahim, S., Al-Saryi, N., Al-Kadmy, I. M. S., Aziz, S. N. (2021). Multidrug-resistant acinetobacter baumannii as an emerging concern in hospitals. Mol. Biol. Rep. 48 (10), 6987–6998. doi: 10.1007/s11033-021-06690-6
Ji, Y., Yang, X., Wang, J., Cai, W., Gao, F., Wang, H. (2022). Factors influencing the physical restraint of patients in the neurosurgical intensive care unit. Clin. Nurs. Res. 31 (1), 46–54. doi: 10.1177/10547738211016874
Kengkla, K., Kongpakwattana, K., Saokaew, S., Apisarnthanarak, A., Chaiyakunapruk, N. (2017). Comparative efficacy and safety of treatment options for MDR and XDR acinetobacter baumannii infections: a systematic review and network meta-analysis. J. Antimicrobial Chemother. 73 (1), 22–32. doi: 10.1093/jac/dkx368
Klompas, M., Kleinman, K., Khan, Y., Evans, R. S., Lloyd, J. F., Stevenson, K., et al. (2012). Rapid and reproducible surveillance for ventilator-associated pneumonia. Clin. Infect. Dis. 54 (3), 370–377. doi: 10.1093/cid/cir832
Li, Y. J., Pan, C. Z., Fang, C. Q., Zhao, Z. X., Chen, H. L., Guo, P. H., et al. (2017). Pneumonia caused by extensive drug-resistant acinetobacter baumannii among hospitalized patients: genetic relationships, risk factors and mortality. BMC Infect. Dis. 17 (1), 371. doi: 10.1186/s12879-017-2471-0
Lim, S. M. S., Abidin, A. Z., Liew, S. M., Roberts, J. A., Sime, F. B. (2019). The global prevalence of multidrug-resistance among acinetobacter baumannii causing hospital-acquired and ventilator-associated pneumonia and its associated mortality: a systematic review and meta-analysis. J. Infection 79 (6), 593–600. doi: 10.1016/j.jinf.2019.09.012
Magiorakos, A. P., Srinivasan, A., Carey, R. B., Carmeli, Y., Falagas, M. E., Giske, C. G., et al. (2012). Multidrug-resistant, extensively drug-resistant and pandrug-resistant bacteria: an international expert proposal for interim standard definitions for acquired resistance. Clin. Microbiol. Infect. 18 (3), 268–281. doi: 10.1111/j.1469-0691.2011.03570.x
Munoz-Price, L. S., Weinstein, R. A. (2008). Acinetobacter infection. N Engl. J. Med. 358 (12), 1271–1281. doi: 10.1056/NEJMra070741
Organization, W. H. (2017). WHO publishes list of bacteria for which new antibiotics are urgently needed (Geneva: World Health Organization). Available at: http://www.who.int/mediacentre/news/releases/2017/bacteria-antibiotics-needed/en/.
Ren, J., Li, X., Wang, L., Liu, M., Zheng, K., Wang, Y. (2019). Risk factors and drug resistance of the MDR acinetobacter baumannii in pneumonia patients in ICU. Open Med. (Wars) 14, 772–777. doi: 10.1515/med-2019-0090
Russell, C. D., Parajuli, A., Gale, H. J., Bulteel, N. S., Schuetz, P., de Jager, C. P. C., et al. (2019). The utility of peripheral blood leucocyte ratios as biomarkers in infectious diseases: a systematic review and meta-analysis. J. Infect. 78 (5), 339–348. doi: 10.1016/j.jinf.2019.02.006
Russo, A., Bassetti, M., Bellelli, V., Bianchi, L., Marincola Cattaneo, F., Mazzocchetti, S., et al. (2021). Efficacy of a fosfomycin-containing regimen for treatment of severe pneumonia caused by multidrug-resistant acinetobacter baumannii: a prospective, observational study. Infect. Dis. Ther. 10 (1), 187–200. doi: 10.1007/s40121-020-00357-8
Ryu, J. A., Yang, J. H., Chung, C. R., Suh, G. Y., Hong, S. C. (2017). Impact of neurointensivist Co-management on the clinical outcomes of patients admitted to a neurosurgical intensive care unit. J. Korean Med. Sci. 32 (6), 1024–1030. doi: 10.3346/jkms.2017.32.6.1024
Schneider, F., Gessner, A., El-Najjar, N. (2022). Efficacy of vancomycin and meropenem in central nervous system infections in children and adults: current update. Antibiot. (Basel) 11 (2), 173. doi: 10.3390/antibiotics11020173
Seligman, R., Meisner, M., Lisboa, T. C., Hertz, F. T., Filippin, T. B., Fachel, J. M., et al. (2006). Decreases in procalcitonin and c-reactive protein are strong predictors of survival in ventilator-associated pneumonia. Crit. Care 10 (5), R125. doi: 10.1186/cc5036
Tien, N., Lin, T. H., Hung, Z. C., Lin, H. S., Wang, I. K., Chen, H. C., et al. (2018). Diagnosis of bacterial pathogens in the urine of urinary-Tract-Infection patients using surface-enhanced raman spectroscopy. Molecules 23 (12), 3374. doi: 10.3390/molecules23123374
Tsakiridou, E., Makris, D., Daniil, Z., Manoulakas, E., Chatzipantazi, V., Vlachos, O., et al. (2014). Acinetobacter baumannii infection in prior ICU bed occupants is an independent risk factor for subsequent cases of ventilator-associated pneumonia. BioMed. Res. Int. 2014, 193516. doi: 10.1155/2014/193516
Tunkel, A. R., Hasbun, R., Bhimraj, A., Byers, K., Kaplan, S. L., Scheld, W. M., et al. (2017). 2017 infectious diseases society of america’s clinical practice guidelines for healthcare-associated ventriculitis and meningitis. Clin. Infect. Dis. 64 (6), e34–e65. doi: 10.1093/cid/ciw861
Wu, J., Wang, X., Zhou, M., Chen, G. B., Du, J., Wang, Y., et al. (2021). The value of lymphocyte-to-monocyte ratio and neutrophil-to-lymphocyte ratio in differentiating pneumonia from upper respiratory tract infection (URTI) in children: a cross-sectional study. BMC Pediatr. 21 (1), 545. doi: 10.1186/s12887-021-03018-y
Xiao, Q., Zhang, H., Wu, X., Qu, J., Qin, L., Wang, C. (2022). Augmented renal clearance in severe infections-an important consideration in vancomycin dosing: a narrative review. Front. Pharmacol. 13. doi: 10.3389/fphar.2022.835557
Yakupogullari, Y., Otlu, B., Ersoy, Y., Kuzucu, C., Bayindir, Y., Kayabas, U., et al. (2016). Is airborne transmission of acinetobacter baumannii possible: a prospective molecular epidemiologic study in a tertiary care hospital. Am. J. Infect. Control 44 (12), 1595–1599. doi: 10.1016/j.ajic.2016.05.022
Yan, C., Hao, P., Wu, G., Lin, J., Xu, J., Zhang, T., et al. (2022). Machine learning-based combined nomogram for predicting the risk of pulmonary invasive fungal infection in severely immunocompromised patients. Ann. Transl. Med. 10 (9), 514. doi: 10.21037/atm-21-4980
Zhang, J., Liu, W., Shi, W., Cui, X., Liu, Y., Lu, Z., et al. (2022). A nomogram with six variables is useful to predict the risk of acquiring carbapenem-resistant microorganism infection in ICU patients. Front. Cell Infect. Microbiol. 12. doi: 10.3389/fcimb.2022.852761
Zhang, H., Zhao, Y., Zheng, Y., Kong, Q., Lv, N., Liu, Y., et al. (2020). Development and validation of a model for predicting the risk of death in patients with acinetobacter baumannii infection: a retrospective study. Infect. Drug Resist. 13, 2761–2772. doi: 10.2147/IDR.S253143
Zhen, X., Lundborg, C. S., Sun, X., Zhu, N., Gu, S., Dong, H. (2021). Correction to: economic burden of antibiotic resistance in China: a national level estimate for inpatients. Antimicrob. Resist. Infect. Control 10 (1), 64. doi: 10.1186/s13756-021-00934-7
Keywords: Acinetobacter baumannii, pulmonary infection, multidrug-resistant, nomogram, early diagnosis, neurosurgical intensive care unit
Citation: Wu D, Sha Z, Fan Y, Yuan J, Jiang W, Liu M, Nie M, Wu C, Liu T, Chen Y, Feng J, Dong S, Li J, Sun J, Pang C and Jiang R (2023) Evaluating the efficiency of a nomogram based on the data of neurosurgical intensive care unit patients to predict pulmonary infection of multidrug-resistant Acinetobacter baumannii. Front. Cell. Infect. Microbiol. 13:1152512. doi: 10.3389/fcimb.2023.1152512
Received: 27 January 2023; Accepted: 12 April 2023;
Published: 25 April 2023.
Edited by:
Marc Leone, Aix Marseille Université, FranceReviewed by:
Nazan Tuna, Namik Kemal University, TürkiyeCarole Eldin, Assistance Publique Hôpitaux de Marseille, France
Copyright © 2023 Wu, Sha, Fan, Yuan, Jiang, Liu, Nie, Wu, Liu, Chen, Feng, Dong, Li, Sun, Pang and Jiang. This is an open-access article distributed under the terms of the Creative Commons Attribution License (CC BY). The use, distribution or reproduction in other forums is permitted, provided the original author(s) and the copyright owner(s) are credited and that the original publication in this journal is cited, in accordance with accepted academic practice. No use, distribution or reproduction is permitted which does not comply with these terms.
*Correspondence: Rongcai Jiang, amlhbmcxMTYyMTZAMTYzLmNvbQ==; Jian Sun, c3VuamlhbjIyMjJAMTI2LmNvbQ==; Chongjie Pang, ZWNobzA2NDVAc2luYS5jb20=
†These authors have contributed equally to this work