- 1School of Basic Medical Sciences, Henan University, Kaifeng, China
- 2Department of Microbiology and Immunology, Faculty of Pharmacy, Zagazig University, Zagazig, Egypt
- 3School of Nursing and Health, Henan University, Kaifeng, China
- 4Institute of Cell Biology, Zhejiang University, Hangzhou, China
Tuberculosis (TB) remains a global health challenge, with timely and accurate diagnosis being critical for effective disease management and control. Recent advancements in the field of TB diagnostics have focused on the identification and utilization of blood-based biomarkers, offering a non-invasive, rapid, and scalable approach to disease detection. This review provides a comprehensive overview of the latest progress in blood-based biomarkers for TB, highlighting their potential to revolutionize diagnostic strategies. Furthermore, we explore emerging technologies such as NGS, PET-CT, Xpert and line probe assays, which have enhanced the sensitivity, specificity, and accessibility of biomarker-based diagnostics. The integration of artificial intelligence (AI) and machine learning (ML) in biomarker analysis is also examined, showcasing its potential to improve diagnostic accuracy and predictive capabilities. This review underscores the need for multidisciplinary collaboration and continued innovation to translate these promising technologies into practical, point-of-care solutions. By addressing these challenges, blood-based biomarkers and emerging technologies hold the potential to significantly improve TB diagnosis, ultimately contributing to global efforts to eradicate this devastating disease.
1 Introduction
Tuberculosis (TB) is a chronic infectious disease caused by Mycobacterium tuberculosis (Mtb), primarily leading to pulmonary infection (Miggiano et al., 2020). Other organs such as the brain, gut, and lymph nodes can also be infected, causing extra-pulmonary tuberculosis (Rodriguez-Takeuchi et al., 2019).According to the World Health Organization (WHO), tuberculosis stands as one of the world’s deadliest infectious diseases, with an estimated 10.6 million individuals infected in 2024 (Ghebreyesus, 2024). Moreover, 1.3 million people, including 167,000 individuals living with HIV, lost their lives to TB. About a quarter of the world’s population harbors latent tuberculosis infection (LTBI), and between 5% to 10% of those with LTBI are expected to develop symptoms and advance to active tuberculosis (aTB) (Getahun et al., 2015; Ying et al., 2022).
The widespread spread of tuberculosis is mainly due to the lack of a reliable, rapid, and accessible test for diagnosing TB (Nogueira et al., 2022). Traditional diagnostic methods, such as sputum smear microscopy, are commonly used in low- and middle-income countries due to their affordability and simplicity (Figure 1, Supplementary Table S1). However, these methods have significant limitations, including low sensitivity and a high detection threshold (requiring sputum with more than 1,000 bacilli/mL) (Steingart et al., 2006). Although sputum culture offers high sensitivity (>98%) and a lower detection threshold (>10 bacilli/mL), its lengthy culture duration of 2–8 weeks hinders timely diagnosis and treatment (Domínguez et al., 2023), potentially leading to further TB dissemination. Additionally, tuberculin skin tests (TST) or interferon-gamma release assays (IGRA) are recommended for identifying individuals with LTBI (Hamada et al., 2021). However, TST may yield false-positive results in individuals who have received the BCG vaccine or have been exposed to non-tuberculous mycobacteria (NTM), while IGRA may be less reliable in children under 5 years old and individuals with hematologic disorders or severe immunosuppression (Mandalakas et al., 2011). The emergence of drug-resistant strains of Mtb further complicates TB diagnosis and management (Farhat et al., 2024). In 2020, only 71% of patients with confirmed TB were tested for rifampin resistance, with less than half receiving appropriate resistance treatment and a success rate of merely 60% (Bagcchi, 2023). Drug-resistant TB can result in treatment challenges, recurrence of the disease, and the development of extensively drug-resistant tuberculosis (XDR-TB) (Seung et al., 2015), prolonging treatment duration and reducing cure rates (Farhat et al., 2024).
To address these challenges, the current recommendation is to utilize rapid molecular diagnostic tests, such as Xpert MTB/RIF Ultra and Truenat, as the initial diagnostic tool for individuals showing signs and symptoms of TB (Bagcchi, 2023). However, the progress of rapid molecular testing has been sluggish, and its implementation in low- and middle-income countries has been hindered by its prohibitive cost. In 2024, out of 6.4 million diagnosed patients, only 38% were detected using rapid molecular techniques, indicating a need for further advancement and the discovery of additional biomarkers (Carranza et al., 2020; Ghebreyesus, 2024.).
This manuscript provides a comprehensive overview of the strengths, limitations, and clinical applications of several traditional tuberculosis (TB) diagnostic methods. Additionally, it highlights the latest advancements in TB detection technologies, including PET-CT, Xpert MTB/RIF, next-generation sequencing (NGS), and line probe assays, which demonstrate exceptional sensitivity, accuracy, and versatility in various clinical settings. The study also explores the latest TB biomarkers discovered through advanced omics technologies like transcriptomics, metabolomics, and proteomics. These biomarkers are rigorously assessed for their sensitivity, specificity, and practical application, providing key insights into their potential to transform TB diagnosis and management.
2 Novel technologies for TB detection
In recent years, TB diagnostics have undergone continuous innovation, marked by groundbreaking advancements: The Xpert MTB/RIF nucleic acid amplification technology has notably enhanced diagnostic efficiency through rapid detection and simultaneous analysis of rifampicin resistance genes; AI/ML-based microscopy image analysis systems have achieved automated interpretation of sputum smears, substantially reducing manual errors; PET-CT metabolic-anatomical fusion imaging enables precise localization of active lesions, offering novel pathways for early diagnosis of extrapulmonary TB; and line probe assays (LPAs) facilitate rapid screening of multidrug-resistant tuberculosis (MDR-TB) via targeted analysis of drug resistance gene mutations (Figure 2, Supplementary Table S2).The synergistic integration of these technologies is propelling TB diagnosis and treatment toward precision medicine and intelligent healthcare paradigms (Khan et al., 2024).
2.1 Automated fluorescent microscopy for AI-driven tuberculosis detection
Sputum smear microscopy is the primary diagnostic method for a TB in low-income countries (Hung et al., 2007), with the two most commonly used detection methods being bright-field microscopy and fluorescence microscopy. Manual counting methods require trained inspectors and significant time to search for acid-fast bacilli (AFB) under microscopic vision (Kotei and Thirunavukarasu, 2022). However, implementing an automated system for reading microscopic slides can reduce subjectivity in results and improve the performance of smear microscopy (Mota Carvalho et al., 2023). MetaSystems’ automated fluorescent AFB slide scanner and analyzer represents an innovative commercial solution that integrates computer vision artificial intelligence (AI) with automated digital microscopy (DM) systems (Tomasello et al., 2022). The image acquisition and analysis of AFB slides are performed by the proprietary Metafer software platform, which incorporates a manufacturer-trained deep neural network (DNN) architecture. This advanced AI system, developed through supervised learning methodologies, employs a probabilistic scoring mechanism to accurately identify and classify objects with potential AFB characteristics (Desruisseaux et al., 2024). Among the 496 qualified smears that met the quality control criteria, the MetaSystems platform demonstrated a sensitivity of 97.0% and a specificity of 12.7% when used independently. When positive scans were utilized to assist technologists, the MetaSystems platform achieved a sensitivity of 70.7% and a specificity of 89.0%. Fu et al. further advanced the field by developing an automated microscope system (µ-Scan 1.1) powered by a convolutional neural network (CNN) algorithm, which achieved remarkable performance in detecting acid-fast bacilli (AFB) in tuberculosis sputum smears, with an accuracy of 95.2%, sensitivity of 85.7%, and specificity of 96.9%. In parallel, an innovative cough audio classifier was developed, leveraging AI and ML technologies to analyze cough sounds for tuberculosis detection. When tested in Tanzania, this classifier demonstrated promising results with a sensitivity of 80% and specificity of 90%, underscoring the significant potential of AI in delivering accessible diagnostic solutions, particularly in regions with constrained healthcare infrastructure (Fu et al., 2022).
2.2 PET-CT imaging for tuberculosis diagnosis
Chest X-ray, while maintaining its status as the most extensively employed screening modality for suspected pulmonary tuberculosis (PTB) cases, demonstrates significant limitations in detecting early-stage infections and extrapulmonary manifestations owing to its restricted sensitivity and lack of functional imaging capabilities (Feyisa et al., 2023; Sossen et al., 2023). CT and MRI are the preferred imaging techniques for assessing disease presence in specific body sites when sputum is negative or extrapulmonary tuberculosis is suspected (Skoura et al., 2015; Rodriguez-Takeuchi et al., 2019). Positron emission tomography-computed tomography (PET-CT) has emerged as a promising early diagnostic modality for tuberculosis, integrating the functional metabolic imaging capabilities of PET with the high-resolution anatomical details provided by CT, thereby establishing a comprehensive diagnostic framework for tuberculosis detection and evaluation. During the diagnostic procedure, patients undergo intravenous administration of the radioactive tracer 18F-fluorodeoxyglucose (18F-FDG), which serves as a metabolic marker. The PET scanner subsequently detects the positron emissions resulting from the radioactive decay of 18F-FDG, enabling both qualitative visualization and quantitative assessment of metabolic activity in tuberculosis lesions (Lamarca et al., 2019). When combined with CT’s superior anatomical imaging capabilities, this hybrid imaging modality facilitates precise localization and characterization of tuberculosis lesions, significantly enhancing diagnostic accuracy and enabling comprehensive evaluation of disease extent and progression (Bomanji et al., 2020). A comprehensive study conducted by Josef Yayan and colleagues revealed that FDG-PET-CT, while susceptible to interference from other inflammatory diseases, achieved an average diagnostic sensitivity of 82.6% and specificity of 67.3% in tuberculosis detection, demonstrating superior diagnostic performance with significantly higher sensitivity rates compared to conventional methods such as sputum testing (55%), sputum culture (70%), and chest X-ray imaging (72.5%) (Yayan et al., 2024).
2.3 Xpert for the detection of tuberculosis
The Xpert MTB/RIF assay (Xpert) and the MTB/RIF Ultra assay (Ultra) are the World Health Organization-recommended rapid molecular detection methods for tuberculosis (Bagcchi, 2023). These assays are utilized for initial diagnostic testing and rifampicin resistance testing in all patients showing signs and symptoms of tuberculosis (Chakravorty et al., 2017). Xpert MTB/RIF is an automated polymerase chain reaction (PCR) test conducted on the GeneXpert platform. Unlike traditional nucleic acid amplification (NAA) tests, Xpert MTB/RIF integrates sample processing, PCR amplification, and detection into a single self-contained test unit (Boehme et al., 2010). All detection steps are self-contained and isolated after sample introduction, with strong Mtb-killing ability upon test completion, enabling Xpert to effectively address biosafety concerns during the assay process.
Xpert MTB/RIF Ultra is an enhanced assay that features a newly designed cartridge and can be operated on the same device after a software update (Xie et al., 2024). Compared to Xpert MTB/RIF, Xpert Ultra includes two different multi-copy amplification targets and a larger DNA reaction chamber. The limit of detection (LOD) for TB testing with Ultra is 15.6 colony-forming units (CFUs) per mL in Mtb-spiked sputum, which is approximately 8 times higher in sensitivity than Xpert MTB/RIF (Chakravorty et al., 2017). Ultra is expected to improve TB case detection rates, especially in individuals with paucibacillary TB such as those with HIV co-infection, as well as in pediatric patients and those with extrapulmonary TB who typically have lower mycobacterial loads (Wang et al., 2022).
In a comprehensive meta-analysis conducted by Man-Qing Wang and colleagues, systematic evaluation of 187 fourfold tables derived from 72 independent studies demonstrated that Xpert MTB/RIF Ultra achieved an overall pooled sensitivity of 76% and specificity of 95% for pulmonary tuberculosis detection. Furthermore, the assay showed enhanced diagnostic performance in detecting rifampin resistance, with pooled sensitivity reaching 94% and specificity of 97% (Wang et al., 2025).
According to the WHO report, over 80% of rifampicin-resistant TB patients are also resistant to isoniazid, while the majority of rifampicin-resistant patients have MDR-TB (Gandhi et al., 2010). The use of Xpert has greatly enhanced the diagnosis of RIF-R and MDR tuberculosis, leading to a three- to eight-fold rise in global MDR-TB testing between 2010 and 2016 (Penn-Nicholson et al., 2022).Known as the only automated molecular assay of lower complexity, Xpert MTB/XDR is well-suited for a wider range of resistance testing and for use in lower-level laboratory networks (Tabriz et al., 2020; Pillay et al., 2022). This assay is proficient in detecting M. tuberculosis complex (MTBC) DNA and mutations linked to resistance against isoniazid, fluoroquinolones (ofloxacin, moxifloxacin, levofloxacin, gatifloxacin), second-line injectable drugs (amikacin, kanamycin, capreomycin), and ethionamide (Su et al., 2017; Pillay et al., 2022).
2.4 Line probe assay for the detection of tuberculosis
Line probe assays (LPAs) are a genotyping technique used for detecting drug-resistant Mtb, utilizing DNA-DNA hybridization technology and multiple probes to simultaneously identify common resistance mutations (Meaza et al., 2017). Currently endorsed by the WHO for the initial drug resistance screening of sputum smear-positive samples are line probes such as GenoType MTBDRplus, Nipro NTM+MDR-TB, and GenoType MTBDRsl (MacLean et al., 2020; Brankin et al., 2022). GenoType MTBDRplus and Nipro NTM+MDR-TB target mutations in the rpoB, katG, and inhA genes, along with the promoter region, to assess resistance to isoniazid and rifampicin (Ferreira Junior et al., 2014). On the other hand, GenoType MTBDRsl examines resistance to ethambutol, quinolones, and second-line injectables by detecting mutations in the gyrA, rrs, and embB genes (Brossier et al., 2010; Pinhata et al., 2023).
In a study involving 379 strains and 644 sputum samples (Meaza et al., 2017), the sensitivity and specificity of GenoType MTBDRplus and Nipro NTM+MDR-TB in detecting rifampin resistance ranged from 90.3%-98.2% to 97.8%-98.5% and 92%-96.5% to 97.5%-98.5%, respectively. For isoniazid resistance detection, the sensitivity and specificity were 89.1% and 95.4% to 98.8%-99.4% and 89.6%-94.9% to 97.6%-100%, respectively. Gardee et al. found that compared to phenotypic drug susceptibility testing, GenoType MTBDRsl version 2.0 showed 100% sensitivity for fluoroquinolone resistance and 89.2% sensitivity for second-line injectable drug resistance, with specificities of 98.9% and 98.5%, respectively (Bouzouita et al., 2021). LPAs provide a rapid diagnostic approach for XDR-TB and MDR-TB. However, due to incomplete understanding of the molecular mechanisms of anti-TB drug resistance, LPA targets are limited to common drug resistance mutations (Mindru et al., 2016).
2.5 Targeted next-generation sequencing for the diagnosis of tuberculosis
Genotypic drug susceptibility testing methods analyze mutations in the Mtb genome associated with drug resistance (Yan et al., 2023). Nucleic acid amplification tests (NAATs) are commonly used for this purpose due to their simplicity and ability to provide results quickly, thereby improving access to drug susceptibility testing (Cantera et al., 2019; Wang et al., 2023). However, NAATs are limited in their scope, targeting only a few known resistance mutations and a select range of drugs.
In contrast, targeted next-generation sequencing (NGS) technology combines gene amplification with high-throughput sequencing to detect resistance to multiple drugs in a single test (Bewicke-Copley et al., 2019; Satam et al., 2023). Targeted NGS can examine entire genes for specific resistance mutations, potentially offering greater accuracy. Furthermore, new targeted NGS assays can identify resistance to novel and repurposed drugs not covered by other molecular tests recommended by the WHO, making them a promising option for comprehensive resistance detection aligned with modern treatment protocols (Schwab et al., 2024).
A recent survey conducted across fifty-three countries, including those on the WHO’s list of high-burden tuberculosis countries, aimed to assess the diagnostic accuracy of targeted NGS for various drugs, including rifampicin, isoniazid, ethambutol, pyrazinamide, streptomycin, injectable drugs (amikacin, capreomycin, and kanamycin), moxifloxacin, and fluoroquinolones like levofloxacin and moxifloxacin (Schwab et al., 2024). The study found that targeted NGS demonstrated an overall sensitivity of 94.1% (95% CrI 90.9 - 96.3) and a specificity of 98.1% (97.0 - 98.9) for drug-resistant tuberculosis testing. In March 2024, the WHO updated its guidelines to include targeted NGS as a recommended tool for rapid diagnostics in tuberculosis detection, highlighting the growing importance and effectiveness of this technology in the fight against drug-resistant TB.
3 Potential blood-based biomarkers for tuberculosis diagnosis
Blood-based biomarkers for tuberculosis enable non-invasive, rapid, and highly sensitive diagnosis, with significant clinical potential (Li et al., 2025). Their non-invasive nature simplifies sample collection, avoiding the discomfort of traditional methods like sputum tests or biopsies, especially benefiting children, the elderly, and those unable to provide sputum. Blood-based methods (e.g., ELISA, PCR, mass spectrometry) deliver results in hours, speeding up diagnosis and enabling early treatment. Specific biomarkers (e.g., IP-10, RISK6) are elevated in active tuberculosis, improving sensitivity for low bacterial load cases (Pan et al., 2021). By integrating multiple biomarkers (e.g., cytokines, gene expression, metabolites), the accuracy and specificity of diagnosis can be further enhanced, effectively reducing misdiagnosis and missed diagnoses (Tables 1, 2). Additionally, blood testing technology is well-established, easy to standardize, and suitable for widespread use in resource-limited settings.
3.1 Cytokine-based biomarkers for tuberculosis diagnosis
Adaptive immunity plays a critical role in the progression of tuberculosis, a fact well-established and extensively documented (Jasenosky et al., 2015; de Martino et al., 2019). In the early stages of Mtb infection, cell-mediated immune responses are vital for containing the pathogen within a localized lung area (Li et al., 2019; Shaukat et al., 2023). In a study conducted by Muthya Pragun Acharya et al., 245 individuals were recruited and categorized into groups including PTB, EPTB, LTBI, healthy controls (HCs), cured tuberculosis (CTB), and sick controls (SCs). Host immune biomarkers in peripheral blood mononuclear cells were identified using multicolor flow cytometry in a cohort of 56 subjects. The clinical performance of these biomarkers was subsequently evaluated in a blind validation cohort of 165 subjects using whole blood (Acharya et al., 2021). The results indicated that the frequencies of cytokine-secreting Mtb-specific CD4 T cells with the CD38+CD27– phenotype clearly distinguished individuals with active tuberculosis from those without the disease. Among the cytokines tested, tumor necrosis factor-α (TNF-α) secretion from CD38+CD27-CD4+ T cells upon stimulation with ESAT6/CFP10 peptides demonstrated the best diagnostic accuracy, with a cutoff of 9.91% (exploratory results: 96.67% specificity, 88.46% sensitivity; validation results: 96.15% specificity, 90.16% sensitivity). Furthermore, this T cell subset could differentiate between treatment-naive TB patients and individuals who had been successfully treated for TB post-anti-TB therapy.
The persistent spread of drug-resistant TB remains one of the most urgent and formidable challenges confronting the global efforts to control TB. In 2023, Pavithra Sampath and colleagues identified two potential biomarkers capable of distinguishing between drug-sensitive and drug-resistant tuberculosis (Sampath et al., 2023). The study population included groups of HCs, individuals with LTBI, drug-sensitive tuberculosis (DS-TB), and drug-resistant tuberculosis (DR-TB), with 40 participants in each group, while those with other infections and comorbidities such as diabetes, HIV, HCV, and HBV were excluded from the study. The experiment initially confirmed that drug-resistant tuberculosis was linked to increased levels of chemokines in plasma using the Luminex Magpix multiplex detection system (Bio-Rad, Hercules, CA). Following this, CXCL10 and CXCL9 exhibited statistically significant differences across all four groups according to ROC analysis of individual variables. The findings were further validated through random forest (RF) analysis (Table 1).
3.2 Proteomics-based screening for diagnostic biomarkers of tuberculosis
Proteomics has emerged as a crucial tool for comprehensively analyzing cellular and organismal processes related to disease and its progression at the protein level (Kavallaris and Marshall, 2005; Al-Amrani et al., 2021). By profiling proteins, proteomics helps uncover the intricate connections among different cellular pathways, complementing both genomic studies and traditional biochemical methodologies (Pandey and Ghosh, 2024; Schiff et al., 2024).
Up to now, the majority of mass spectrometry-based (MS-based) proteomics studies have removed numerous enriched protein components from plasma, resulting in the loss of biologically significant proteins. Consequently, candidate host proteins serving as TB biomarkers commonly exhibit high sensitivity but inadequate specificity. In a study led by Hannah F. Schiff et al., an optimized non-depletion untargeted proteomics method was employed to enhance the coverage of numerous enriched proteins. This approach enables the identification of new markers with both high sensitivity and specificity for tuberculosis (Schiff et al., 2024). Plasma samples from 11 aTB patients and 10 HCs in South Africa and Peru were initially subjected to proteomics analysis. Subsequently, through bioinformatics analysis that employed linear modeling and whole-gene correlation network analysis (WGCNA), a total of 118 differentially expressed proteins were identified. An independent patient cohort from the United Kingdom was later employed to validate the diagnostic potential of MS-identified plasma biomarkers, which included 32 patients with active TB and 30 individuals in a healthy control group. The final 6-protein marker combination, comprising FCGR3B, FETUB, LRG1, ADA2, CD14, and SELL, effectively differentiated patients with aTB from HCs and other infections (ORI) with high sensitivity and specificity. The AUC for TB and HCs was 0.972, with a sensitivity of 90.6% and a specificity of 90.0%, while the AUC for TB and ORI was 0.930, with a sensitivity of 90.6% and a specificity of 80.8% (Table 1).
Treatment for newly diagnosed TB consists of an intensive period of two months followed by a continuation period of four months. However, the absence of established criteria and biomarkers remains a challenge in effectively diagnosing cured TB. Qiqi Lu et al. utilized data-independent acquisition (DIA) to analyze the plasma protein expression profiles of TB patients at different treatment stages, which encompassed 35 newly diagnosed TB patients without treatment (group TB0), 35 TB patients after 2-month intensive-phase treatment (group TB2), 35 cured TB patients after 6-month intensive plus continuation phase treatment (group TB6), and 35 healthy controls (group HC) for comparison (Lu et al., 2022). Subsequent analysis of gene ontology (GO) function and Kyoto Encyclopedia of Genes and Genomes (KEGG) pathways indicates possible coagulation dysfunction, along with disruptions in vitamin and lipid metabolism, during tuberculosis treatment. By leveraging Machine Learning and Support Vector Machine (SVM) method, we ultimately identified four intersecting proteins (albumin [ALB, Swissprot: A0A0C4DGB6], haptoglobin [HP, Swissprot: P00738], Out at first protein homolog [OAF, Swissprot: E9PJ29], and retinol-binding protein 4 [RBP4, Swissprot: P02753]) as promising biomarkers for assessing the efficacy of pulmonary tuberculosis treatment. The efficacy assessment model, utilizing the four proteins, achieved an AUC of 0.963 with a sensitivity of 91.4% and specificity of 94.3% in distinguishing between TB0 and TB6 groups, while also demonstrating an AUC of 0.971, sensitivity of 88.6%, and specificity of 94.3% in distinguishing TB0 and HC groups.
3.3 Metabolomics–based screening for diagnostic biomarkers of tuberculosis
Metabolomics enables the quantitative profiling of high-throughput metabolite molecules (Cao et al., 2020). Through the analysis of metabolite changes, metabolomics can pinpoint specific differentially expressed metabolites that act as biomarkers for diagnostics, disease differentiation, and monitoring the effectiveness of treatment (Vinayavekhin et al., 2010).
The host plasma is abundant in lipids, which constitutes the primary source of nutrition for the growth and reproduction of Mtb. The high-throughput detection of alterations in the entire lipid metabolome of the host caused by Mtb infection was conducted by Jia-Xi Chen et al. using ultra-performance liquid chromatography-tandem mass spectrometry (UPLC-MS/MS) technology (Chen et al., 2021). The Orthogonal Partial Least Squares-Discriminant Analysis (OPLS-DA) model revealed that lipid metabolites between the TB0 group and the HC group were distinctly distinguishable, with 163 differential lipids identified. Additionally, comparisons among the TB0, TB2, and TB6 groups highlighted 25 lipid metabolites as differential. KEGG pathway analysis showed that the plasma from the TB0 and HC groups displayed differences in metabolic pathways linked to glycerophospholipid and sphingolipid metabolism and autophagy, with a noticeable enrichment in linolenic acid and arachidonic acid metabolic pathways as treatment progressed. Together, these metabolites constituted an efficacy evaluation model that accurately distinguished patients in the TB6 group from those in the TB0 group, achieving a perfect area under the curve (AUC) of 1.000. Specifically, the two lysophosphatidic acids, LPA (0:0/16:0) and LPA (0:0/18:0), were instrumental in differentiating cured and active TB patients, exhibiting an AUC of 1, with both sensitivity and specificity reaching 100%.
Due to the overlapping thresholds in drug susceptibility testing for anti-TB drugs, identifying pre-extensively (pre-XDR) and extensively drug-resistant tuberculosis (XDR-TB) poses a significant challenge. Pratchakan Chaiyachat and colleagues conducted a metabolomic analysis of one hundred and fifty Mtb isolates using ultra-high performance liquid chromatography coupled with electrospray ionization-quadrupole-time of flight-mass spectrometry (UHPLC-ESI-QTOF-MS/MS) (Chaiyachat et al., 2023). This analysis included fifty-four pre-XDR, sixty-three XDR-TB, and thirty-three pan-susceptible (pan-S) isolates. Twelve metabolic markers exhibiting the most significant differences between groups were identified. Notably, meso-hydroxyheme and itaconic anhydride demonstrated the ability to accurately classify the resistance status of a sample with 100% sensitivity and specificity. In addition, specific metabolites were identified in Mtb isolates resistant to ethionamide (ETO) and ethambutol (ETH).
3.4 LncRNAs-based biomarkers for tuberculosis diagnosis
Long non-coding RNAs (lncRNAs) are single noncoding RNA transcripts longer than 200 nucleotides, which are crucial elements in regulating gene expression (Figure 3). There is a growing body of evidence indicating that blood lncRNA expression profiles are closely linked to tuberculosis (TB), suggesting their potential as noninvasive biomarkers for TB detection (Wei et al., 2017; Mattick et al., 2023).
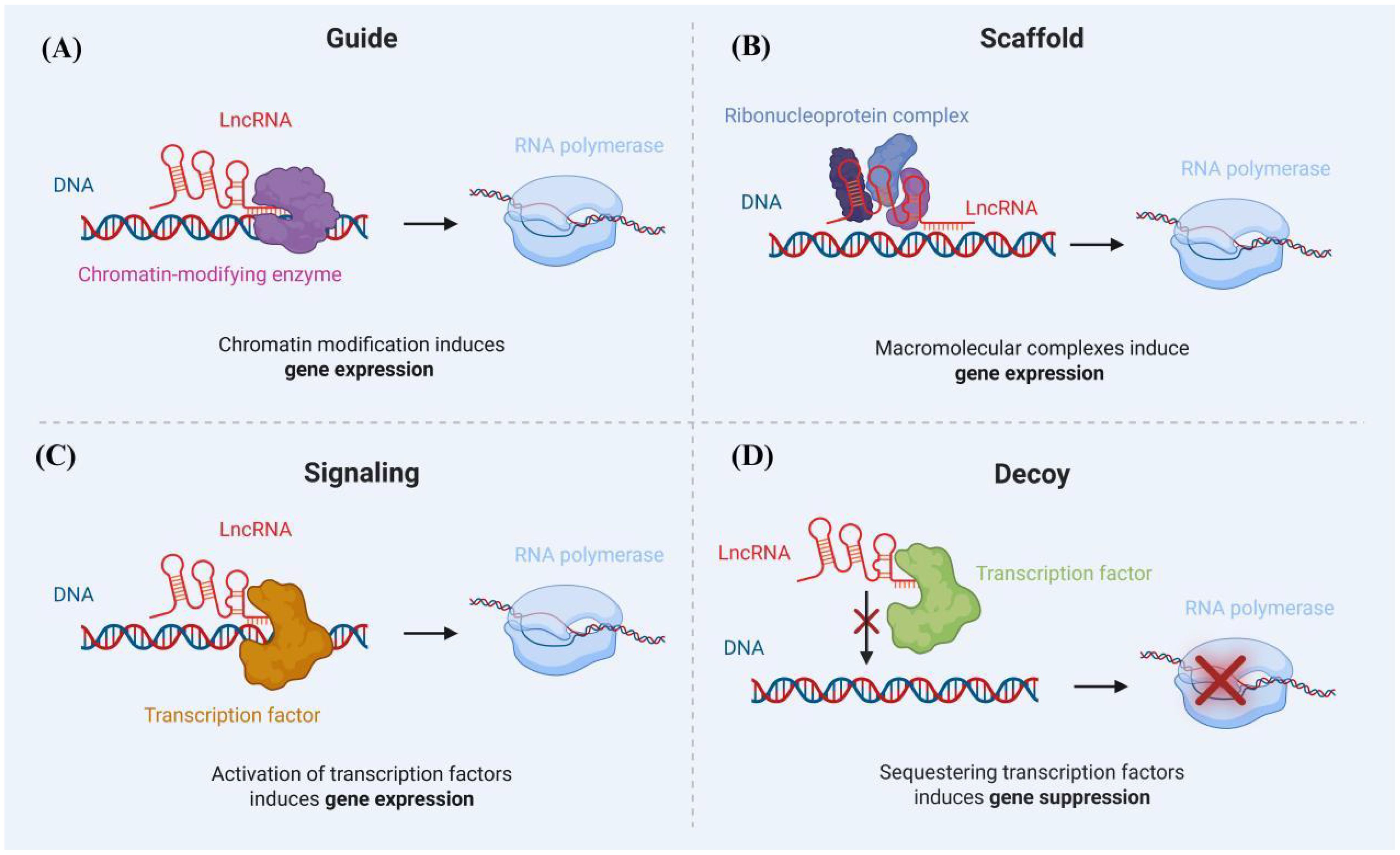
Figure 3. Mechanisms of lncRNA Action: (A) LncRNAs recruit chromatin-modifying enzymes to specific gene loci, whereby the modulation of chromatin status results in the activation or suppression of nearby genes. (B) LncRNAs engage in the formation of RNA-protein complexes (RNPs), facilitating either the promotion or repression of transcription. (C) LncRNAs recruit transcriptional machinery proteins to nearby target gene sites in order to enhance their transcriptional activity. (D) LncRNAs act as a decoy for the transcription factor, contributing to the repression of certain pro-apoptotic genes.
In research carried out by Zhong-liang Chen et al., plasma lncRNAs were scrutinized through microarray analysis, with a special focus on investigating the potential diagnostic significance of lncRNAs in TB (Chen et al., 2017). Through a thorough analysis, it was revealed that there were 511 differentially expressed lncRNAs (163 up-regulated and 348 down-regulated) as well as 411 differentially expressed mRNAs (127 up-regulated and 284 down-regulated) when comparing individuals with TB to healthy controls. GO, KEGG, and coding-noncoding co-expression (CNC) analyses revealed that the differentially expressed lncRNAs predominantly played a role in regulating alpha-beta T cell activation and the T cell receptor signaling pathway. Six lncRNAs (NR_038221, NR_003142, ENST00000568177, ENST00000570366, ENST00000422183, and ENST00000449589) were chosen for qPCR validation in 52 TB patients and healthy controls. However, there were no significant differences in the expression levels of ENST00000568177 and ENST00000449589 between TB patients and healthy control subjects. The final diagnostic model consisted of four distinct lncRNAs: NR_038221, NR_003142, ENST00000570366, and ENST00000422183, achieving an AUC of 0.845 with a sensitivity of 79.2% and a specificity of 75%. Furthermore, the lncRNA-mRNA-miRNA ceRNA network was developed to predict potential interactions between 85 mRNAs and 404 miRNAs with the identified lncRNAs (Table 2).
Plasma lncRNA could potentially serve as a biomarker for accurately evaluating the recovery status of tuberculosis. Zhi-Bin Li et al. utilized lncRNA microarray analysis to identify differentially expressed plasma lncRNAs in untreated and cured TB individuals. They confirmed the expression levels of these lncRNAs using qPCR (Li et al., 2020). The findings revealed significant differences in the expression of lncRNAs uc.48+ and NR_105053 between untreated and cured tuberculosis groups. These lncRNAs were used to establish a predictive model for tuberculosis recovery. The model demonstrated a sensitivity of 90.00%, specificity of 86.36%, and an AUC value of 0.945. These lncRNAs could serve as biomarkers to differentiate between untreated TB patients and those who have been cured. Furthermore, the study predicted target genes of uc.48+ and NR_105053 by constructing co-expression networks between coding and non-coding genes, as well as an mRNA-lncRNA-miRNA interaction network.
3.5 MicRNAs–based biomarkers for tuberculosis diagnosis
MicroRNAs (miRNAs) are small non-coding RNAs, typically 18 to 24 nucleotides in length, They regulate gene expression at the post-transcriptional level and play a crucial role in various biological processes, including immune responses (Ying et al., 2008). Exosomal miRNAs have emerged as promising biomarkers, and in a study by Xuejiao Hu et al., they were combined with electronic health records (EHRs) for tuberculosis diagnosis (Gao et al., 2021). In the initial phase of the study, microarrays were utilized to analyze an exploratory cohort consisting of 11 active TB patients (7 PTB and 4 tuberculosis meningitis) and 8 HCs, aiming to identify differentially expressed exosomal miRNAs. Eleven candidate miRNAs, with miR-486 among them, were chosen from a pool of 102 differentially expressed exosomal miRNAs. Finally, six exosomal miRNAs (miR-20a, miR-20b, miR-26a, miR-106a, miR-191, and miR-486) were found to be differentially expressed in TB patients through qRT-PCR analysis. Subsequently, miRNAs and EHRs were employed to construct diagnostic models for PTB and Tuberculous Meningitis (TBM) in the selection cohort utilizing the Support Vector Machine (SVM) algorithm. The integrated “EHR+miRNA” model exhibited superior performance compared to using EHR data or miRNA data independently, achieving a diagnostic sensitivity of 0.94 and a specificity of 0.95 for TBM. For PTB, the sensitivity was 0.89, with a corresponding specificity (Table 2).
In a separate study, Muhammad Nasrum Massi and colleagues identified distinct expression patterns of miR-425-5p and miR-4523 in patients with active PTB, LTBI, and lymph node tuberculosis (LNTB) (Massi et al., 2023). The total study sample consisted of 23 patients with active PTB, 21 patients with LTBI, 21 patients with EPTB, and 25 HCs. The levels of hsa-miR-425-5p and hsa-miR-4523 in blood samples from various populations were quantified using RT-qPCR. The level of hsa-miR-425-5p miRNA expression in LNTB was found to be higher than that observed in LTBI. Additionally, the expression of hsa-miR-4523 miRNA was notably lower in PTB and LNTB compared to LTBI. ROC analysis of a single sample revealed that only mir-4523 had the capability to distinguish between LTBI and HCs, showcasing an AUC of 0.829 (Table 2).
3.6 CirRNAs–based biomarkers for tuberculosis diagnosis
Circular RNAs (circRNAs) represent a unique class of RNA characterized by the covalent linkage of their 3’ and 5’ ends, forming a closed-loop structure (Ruiz Esparza Garrido and Velázquez Flores, 2023) (Figure 4). Unlike linear RNAs, circRNAs, with their covalently closed loops, exhibit heightened resistance to RNase degradation, making them preferentially enriched during sample processing and superior candidates for molecular diagnostic biomarkers compared to other RNA types (Qu et al., 2015).
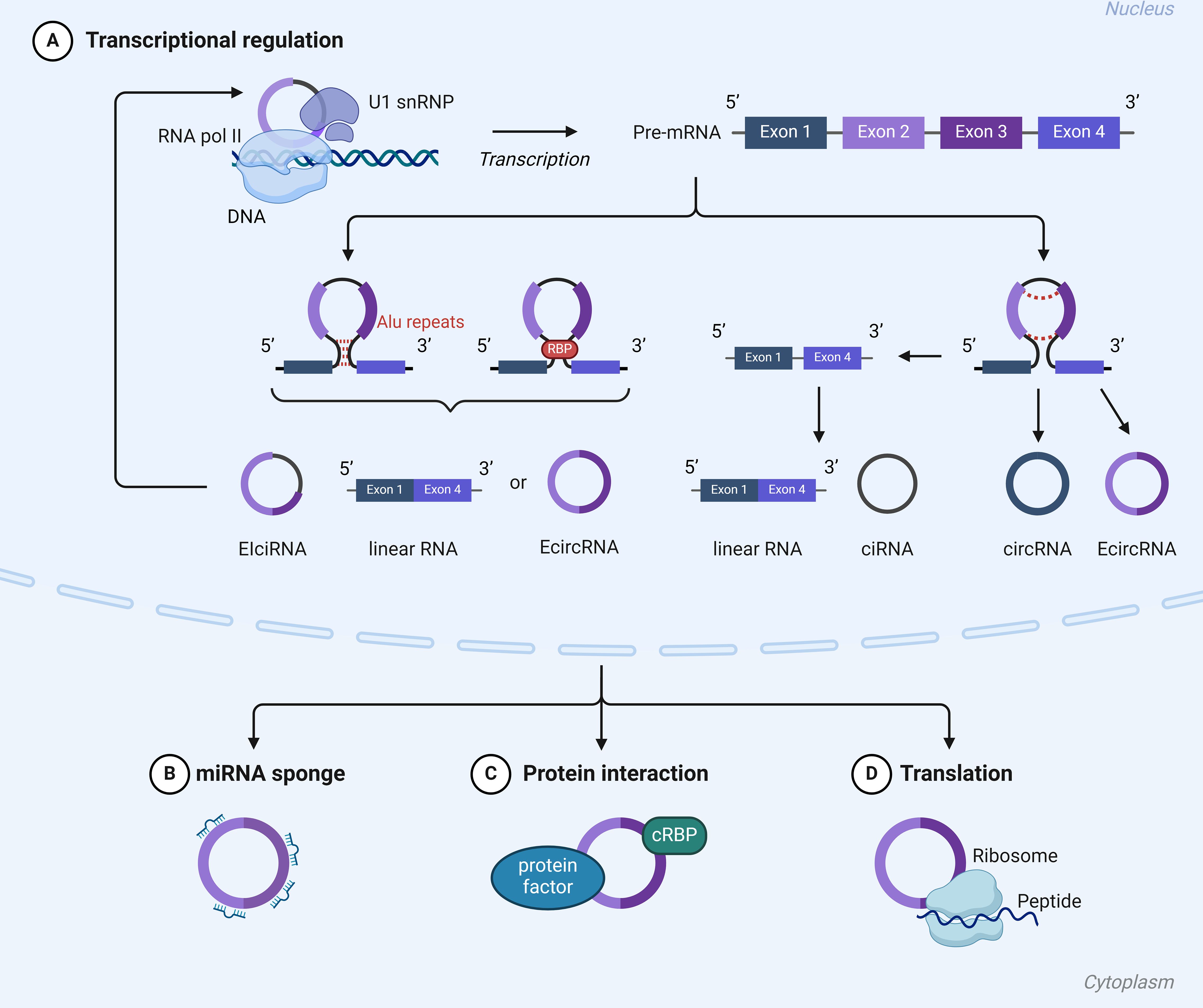
Figure 4. Mechanisms of action of cirRNA: (A) CircRNAs have the capability to directly interact with transcription complexes, thereby influencing the expression of parental genes. (B) CircRNAs have the ability to act as miRNA sponges. (C) circRNAs can interact with circRNA binding proteins (cRBPs) modulate their functions. (D) CircRNAs have the ability to encode peptides and proteins.
In a study by Zi-Kun Huang et al., differential circRNA expression was analyzed using microarray assay in three patients diagnosed with aTB and three HCs matched in terms of age and gender. A total of 37 circRNAs were identified as being differentially expressed between the two groups, comprising 13 up-regulated circRNAs and 24 down-regulated circRNAs. The three most significantly up-regulated and down-regulated circRNAs were chosen for validation using qRT-PCR in an independent cohort comprising 40 TB patients and 40 healthy controls. The findings suggested that hsa_circRNA_001937, hsa_circRNA_009024, and hsa_circRNA_005086 were elevated, while hsa_circRNA_102101, hsa_circRNA_104964, and hsa_circRNA_104296 were decreased in TB patients compared to the normal control group. The AUCs of the six candidate circRNAs were all above 0.750, with hsa_circRNA_001937 attaining the highest AUC of 0.873, outperforming the other five circRNAs in the study. hsa_circRNA_001937 was subsequently examined in a separate cohort comprising 115 TB patients, 40 pneumonia patients, 40 chronic obstructive pulmonary disease (COPD) patients, 40 lung cancer patients, and 90 control subjects. In the new cohort, the AUC of hsa_circRNA_001937 was 0.850, with sensitivity and specificity rates of 72.2% each; the expression level of hsa_circRNA_001937 in this cohort was significantly higher than that in patients with pneumonia and lung cancer (Table 2).
A thorough analysis integrating bioinformatics and molecular biology revealed that hsa_circ_0028883 holds promise as a potential biomarker for the diagnosis of active tuberculosis (Zhang et al., 2020). The study collected gene expression datasets for circRNA (GSE117563 and GSE106953), microRNA (miRNA, dataset GSE29190), and mRNA (GSE54992) from the Gene Expression Omnibus (GEO) database. A competing endogenous RNA (ceRNA) network was constructed based on potential interactions between circRNA, miRNA, and mRNA (Xie et al., 2018). GO and KEGG pathway analyses were used to predict their biological functions. To validate the results, qRT-PCR was performed to measure hsa_circ_0028883 and hsa-miR-409-5p levels in 20 active TB patients and 20 healthy donors. An ROC curve was then generated to evaluate hsa_circ_0028883’s diagnostic value. We discovered that hsa_circ_0028883 exhibited an impressive AUC value of 0.773 (Table 2).
4 Discussion
Significant progress has been made in tuberculosis diagnosis over the past few decades, transitioning from culture-based methods to faster, more accurate tests that are less labor-intensive and time-consuming, without the need for sophisticated biosafety labs (Walzl et al., 2018; Xie et al., 2018). Nonetheless, achieving the 2030 targets outlined in the World Health Organization’s End TB Strategy will necessitate ongoing technological advancements (Gupta-Wright and Denkinger, 2024).
Beyond improving diagnostic methods, it is crucial to collect and analyze biologic treatment data for TB patients during their treatment (Ahuja and Zaheer, 2025) (Supplementary Table S3). This involves documenting TB exposure history, symptom checks, and comorbidities. Key diagnostic tools like skin testing/IGRA, Xpert MTB/RIF, sputum culture, and chest X-rays should be carefully recorded. Additionally, the use of biologic agents such as TNF-α inhibitors and chemopreventive therapy, along with standard treatment protocols and immunosuppression data, must be thoroughly tracked (Naidu et al., 2023). TNF-α is a key biological agent for treating autoimmune diseases and a critical factor in maintaining the structure and function of tuberculous granulomas. Its inhibition can destabilize granulomas, leading to the reactivation of LTBI or the rapid progression of new Mtb infections to active tuberculosis. In terms of diagnosis, TNF-α inhibitors can suppress delayed-type hypersensitivity (DTH), resulting in a false-negative rate of up to 50-70% in the TST (de Oliveira Magalhães et al., 2024). Although the IGRA is not affected by DTH, long-term TNF-α inhibition may reduce IFN-γ release levels, increasing the risk of false negatives by 20-30%. Therefore, when using these drugs, it is essential to combine molecular testing (e.g., Xpert MTB/RIF) and imaging assessments to mitigate the interference of immune suppression on traditional diagnostic methods. The impact of chemopreventive therapy on tuberculosis diagnosis is equally significant. It may reduce the activity or quantity of Mtb, affecting the sensitivity of bacterial culture or molecular testing and leading to false-negative results. Additionally, chemopreventive therapy may modulate immune responses, compromising the accuracy of TST or IGRA and resulting in false negatives. When evaluating treatment efficacy or monitoring for relapse, chemopreventive therapy may also interfere with LTBI diagnosis. Therefore, interpreting diagnostic results requires integrating clinical context with other testing methods, taking into account the influence of chemopreventive therapy (Prakash Babu et al., 2023).
Furthermore, future research efforts should prioritize the development and application of cutting-edge technologies with the potential to transform TB diagnosis, such as Spatial CITE-seq for spatially resolved single-cell analysis, multimodal tri-omics for comprehensive molecular profiling, and perturb-DBiT for high-throughput functional genomics (Zhang et al., 2024). These innovative approaches hold promise for unraveling the complex mechanisms of TB pathogenesis and enabling more precise, early-stage detection. Despite the remarkable strides in tuberculosis (TB) diagnosis, several challenges persist. The prohibitive costs associated with many cutting-edge diagnostic technologies act as a significant barrier to their implementation in low-resource settings. Additionally, the detection of certain biomarkers necessitates sophisticated laboratory infrastructure and technical expertise, which are often unavailable in primary healthcare facilities. Future research endeavors should focus on elucidating the clinical utility of biomarkers, refining detection methodologies, and reducing costs to enhance accessibility (Baysoy et al., 2024).
Author contributions
YW: Investigation, Methodology, Writing – original draft, Writing – review & editing. ZY: Data curation, Formal analysis, Investigation, Methodology, Software, Supervision,Writing – original draft, Writing – review & editing. JjL: Data curation, Formal analysis, Investigation, Methodology, Writing – original draft, Writing – review & editing. JS: Investigation, Methodology, Software, Validation, Visualization, Writing – original draft, Writing – review & editing. HC: Investigation, Methodology, Writing – original draft, Writing – review & editing. SH: Investigation, Writing – review & editing. YD: Investigation, Methodology,Writing – review & editing. YW: Investigation, Writing – review & editing. ZY: Investigation, Methodology, Resources, Writing – review & editing. LX: Formal analysis, Investigation, Software, Visualization, Writing – review & editing. QL: Resources,Writing – review & editing, Investigation. XL: Conceptualization, Funding acquisition, Project administration, Resources, Writing – review & editing, Supervision. SG: Conceptualization, Funding acquisition, Project administration, Resources, Writing – review & editing. SL: Funding acquisition, Supervision, Writing – review & editing. JcL: Conceptualization, Funding acquisition, Project administration, Resources, Supervision, Writing – review & editing.
Funding
The author(s) declare that financial support was received for the research and/or publication of this article. This work was supported by the Key R&D and Promotion Projects of Henan Province (232102310208, 242102311172 and 242102310023); Henan University Laboratory Undergraduate Research Program (No. 20243305115); Key Scientific Research Project of Higher Education of Henan Province(No.23A310009); the key scientific research project of Henan Province, China (No. 242102311182); Kaifeng Science and Technology Development Program Projects (2303007); Provinces and ministries jointly build key projects of Henan Provincial Health Commission (SBGJ202402084).
Conflict of interest
The authors declare that the research was conducted in the absence of any commercial or financial relationships that could be construed as a potential conflict of interest.
Generative AI statement
The author(s) declare that no Generative AI was used in the creation of this manuscript.
Publisher’s note
All claims expressed in this article are solely those of the authors and do not necessarily represent those of their affiliated organizations, or those of the publisher, the editors and the reviewers. Any product that may be evaluated in this article, or claim that may be made by its manufacturer, is not guaranteed or endorsed by the publisher.
Supplementary material
The Supplementary Material for this article can be found online at: https://www.frontiersin.org/articles/10.3389/fcimb.2025.1567592/full#supplementary-material
References
Acharya, M. P., Pradeep, S. P., Murthy, V. S., Chikkannaiah, P., Kambar, V., Narayanashetty, S., et al. (2021). CD38+CD27-TNF-α + on Mtb-specific CD4+ T cells is a robust biomarker for tuberculosis diagnosis. Clin. Infect. Dis. 73, 793–801. doi: 10.1093/cid/ciab144
Ahuja, S., Zaheer, S. (2025). Advancements in pathology: Digital transformation, precision medicine, and beyond. J. Pathol. Inf. 16, 100408. doi: 10.1016/j.jpi.2024.100408
Al-Amrani, S., Al-Jabri, Z., Al-Zaabi, A., Alshekaili, J., Al-Khabori, M. (2021). Proteomics: Concepts and applications in human medicine. World J. Biol. Chem. 12, 57–69. doi: 10.4331/wjbc.v12.i5.57
Bagcchi, S. (2023). WHO’s global tuberculosis report 2022. Lancet Microbe 4, e20. doi: 10.1016/s2666-5247(22)00359-7
Balcells, M. E., Ruiz-Tagle, C., Tiznado, C., García, P., Naves, R. (2018). Diagnostic performance of GM-CSF and IL-2 in response to long-term specific-antigen cell stimulation in patients with active and latent tuberculosis infection. Tuberc. (Edinb) 112, 110–119. doi: 10.1016/j.tube.2018.08.006
Baysoy, A., Tian, X., Zhang, F., Renauer, P., Bai, Z., Shi, H., et al. (2024). Spatially Resolved in vivo CRISPR Screen Sequencing via Perturb-DBiT. bioRxiv, 2024.11.18.624106. doi: 10.1101/2024.11.18.624106
Bewicke-Copley, F., Arjun Kumar, E., Palladino, G., Korfi, K., Wang, J. (2019). Applications and analysis of targeted genomic sequencing in cancer studies. Comput. Struct. Biotechnol. J. 17, 1348–1359. doi: 10.1016/j.csbj.2019.10.004
Boehme, C. C., Nabeta, P., Hillemann, D., Nicol, M. P., Shenai, S., Krapp, F., et al. (2010). Rapid molecular detection of tuberculosis and rifampin resistance. N Engl. J. Med. 363, 1005–1015. doi: 10.1056/NEJMoa0907847
Bomanji, J., Sharma, R., Mittal, B. R., Gambhir, S., Qureshy, A., Begum, S. M. F., et al. (2020). PET/CT features of extrapulmonary tuberculosis at first clinical presentation: a cross-sectional observational (18)F-FDG imaging study across six countries. Eur. Respir. J. 55 (2), 1901951. doi: 10.1183/13993003.01959-2019
Bouzouita, I., Draoui, H., Cabibbe, A. M., Essalah, L., Bejaoui, S., Trovato, A., et al. (2021). Performance of the GenoType MTBDRsl V 2.0 for detecting second-line drugs resistance of Mycobacterium tuberculosis isolates in Tunisia. Res. Microbiol. 172, 103816. doi: 10.1016/j.resmic.2021.103816
Brankin, A., Seifert, M., Georghiou, S. B., Walker, T. M., Uplekar, S., Suresh, A., et al. (2022). In silico evaluation of WHO-endorsed molecular methods to detect drug resistant tuberculosis. Sci. Rep. 12, 17741. doi: 10.1038/s41598-022-21025-6
Brossier, F., Veziris, N., Aubry, A., Jarlier, V., Sougakoff, W. (2010). Detection by GenoType MTBDRsl test of complex mechanisms of resistance to second-line drugs and ethambutol in multidrug-resistant Mycobacterium tuberculosis complex isolates. J. Clin. Microbiol. 48, 1683–1689. doi: 10.1128/jcm.01947-09
Cantera, J. L., White, H., Diaz, M. H., Beall, S. G., Winchell, J. M., Lillis, L., et al. (2019). Assessment of eight nucleic acid amplification technologies for potential use to detect infectious agents in low-resource settings. PloS One 14, e0215756. doi: 10.1371/journal.pone.0215756
Cao, G., Song, Z., Hong, Y., Yang, Z., Song, Y., Chen, Z., et al. (2020). Large-scale targeted metabolomics method for metabolite profiling of human samples. Anal. Chim. Acta 1125, 144–151. doi: 10.1016/j.aca.2020.05.053
Carranza, C., Pedraza-Sanchez, S., de Oyarzabal-Mendez, E., Torres, M. (2020). Diagnosis for latent tuberculosis infection: new alternatives. Front. Immunol. 11. doi: 10.3389/fimmu.2020.02006
Chaiyachat, P., Kaewseekhao, B., Chaiprasert, A., Kamolwat, P., Nonghanphithak, D., Phetcharaburanin, J., et al. (2023). Metabolomic analysis of Mycobacterium tuberculosis reveals metabolic profiles for identification of drug-resistant tuberculosis. Sci. Rep. 13, 8655. doi: 10.1038/s41598-023-35882-2
Chakrabarty, S., Kumar, A., Raviprasad, K., Mallya, S., Satyamoorthy, K., Chawla, K. (2019). Host and MTB genome encoded miRNA markers for diagnosis of tuberculosis. Tuberc. (Edinb) 116, 37–43. doi: 10.1016/j.tube.2019.04.002
Chakravorty, S., Simmons, A. M., Rowneki, M., Parmar, H., Cao, Y., Ryan, J., et al. (2017). The new Xpert MTB/RIF ultra: improving detection of mycobacterium tuberculosis and resistance to rifampin in an assay suitable for point-of-care testing. mBio 8 (3), e00812-17. doi: 10.1128/mBio.00812-17
Chen, J., Han, Y.-S., Yi, W.-J., Huang, H., Li, Z.-B., Shi, L.-Y., et al. (2020). Serum sCD14, PGLYRP2 and FGA as potential biomarkers for multidrug-resistant tuberculosis based on data-independent acquisition and targeted proteomics. J. Cell. Mol. Med. 24, 12537–12549. doi: 10.1111/jcmm.v24.21
Chen, J. X., Han, Y. S., Zhang, S. Q., Li, Z. B., Chen, J., Yi, W. J., et al. (2021). Novel therapeutic evaluation biomarkers of lipid metabolism targets in uncomplicated pulmonary tuberculosis patients. Signal Transduct. Target Ther. 6, 22. doi: 10.1038/s41392-020-00427-w
Chen, Z. L., Wei, L. L., Shi, L. Y., Li, M., Jiang, T. T., Chen, J., et al. (2017). Screening and identification of lncRNAs as potential biomarkers for pulmonary tuberculosis. Sci. Rep. 7, 16751. doi: 10.1038/s41598-017-17146-y
Chen, J., Wu, L., Lv, Y., Liu, T., Guo, W., Song, J., et al. (2022). Screening of long non-coding RNAs biomarkers for the diagnosis of tuberculosis and preliminary construction of a clinical diagnosis model. Front. Microbiol. 13. doi: 10.3389/fmicb.2022.774663
Collins, J. M., Walker, D. I., Jones, D. P., Tukvadze, N., Liu, K. H., Tran, V. T., et al. (2018). High-resolution plasma metabolomics analysis to detect Mycobacterium tuberculosis-associated metabolites that distinguish active pulmonary tuberculosis in humans. PloS One 13, e0205398. doi: 10.1371/journal.pone.0205398
de Martino, M., Lodi, L., Galli, L., Chiappini, E. (2019). Immune response to mycobacterium tuberculosis: A narrative review. Front. Pediatr. 7. doi: 10.3389/fped.2019.00350
de Oliveira Magalhães, V., Bonfiglioli, K. R., Gomes, C. M. F., Bonfá, E., de Medeiros-Ribeiro, A. C., Saad, C. G. S., et al. (2024). Tuberculin skin test repetition after TNF-α inhibitors in patients with chronic inflammatory arthritis: a long-term retrospective cohort in endemic area. Adv. Rheumatol. 64, 70. doi: 10.1186/s42358-024-00406-7
Desruisseaux, C., Broderick, C., Lavergne, V., Sy, K., Garcia, D. J., Barot, G., et al. (2024). Retrospective validation of MetaSystems’ deep-learning-based digital microscopy platform with assistance compared to manual fluorescence microscopy for detection of mycobacteria. J. Clin. Microbiol. 62, e0106923. doi: 10.1128/jcm.01069-23
Domínguez, J., Boeree, M. J., Cambau, E., Chesov, D., Conradie, F., Cox, V., et al. (2023). Clinical implications of molecular drug resistance testing for Mycobacterium tuberculosis: a 2023 TBnet/RESIST-TB consensus statement. Lancet Infect. Dis. 23, e122–e137. doi: 10.1016/s1473-3099(22)00875-1
Fang, Y., Zhao, J., Wang, X., Wang, X.-F., Wang, L., Liu, L., et al. (2021). Identification of differentially expressed lncRNAs as potential plasma biomarkers for active tuberculosis. Tuberculosis 128, 102065. doi: 10.1016/j.tube.2021.102065
Farhat, M., Cox, H., Ghanem, M., Denkinger, C. M., Rodrigues, C., Abd El Aziz, M. S., et al. (2024). Drug-resistant tuberculosis: a persistent global health concern. Nat. Rev. Microbiol. 22, 617–635. doi: 10.1038/s41579-024-01025-1
Ferreira Junior, S. L., Dalla Costa, E. R., Santos, P. G., Gomes, H. M., Silva, M. S., Esteves, L. S., et al. (2014). In house reverse membrane hybridisation assay versus GenoType MTBDRplus and their performance to detect mutations in the genes rpoB, katG and inhA. Mem. Inst. Oswaldo Cruz 109, 307–314. doi: 10.1590/0074-0276130469
Feyisa, D. W., Ayano, Y. M., Debelee, T. G., Schwenker, F. (2023). Weak localization of radiographic manifestations in pulmonary tuberculosis from chest X-ray: A systematic review. Sensors (Basel) 23 (15), 6781. doi: 10.3390/s23156781
Fu, H. T., Tu, H. Z., Lee, H. S., Lin, Y. E., Lin, C. W. (2022). Evaluation of an AI-based TB AFB smear screening system for laboratory diagnosis on routine practice. Sensors (Basel) 22 (21), 8497. doi: 10.3390/s22218497
Fu, Y., Wang, J., Qiao, J., Yi, Z. (2019). Signature of circular RNAs in peripheral blood mononuclear cells from patients with active tuberculosis. J. Cell. Mol. Med. 23, 1917–1925. doi: 10.1111/jcmm.2019.23.issue-3
Gandhi, N. R., Nunn, P., Dheda, K., Schaaf, H. S., Zignol, M., van Soolingen, D., et al. (2010). Multidrug-resistant and extensively drug-resistant tuberculosis: a threat to global control of tuberculosis. Lancet 375, 1830–1843. doi: 10.1016/s0140-6736(10)60410-2
Gao, S. H., Chen, C. G., Zhuang, C. B., Zeng, Y. L., Zeng, Z. Z., Wen, P. H., et al. (2021). Integrating serum microRNAs and electronic health records improved the diagnosis of tuberculosis. J. Clin. Lab. Anal. 35, e23871. doi: 10.1002/jcla.23871
Garay-Baquero, D. J., White, C. H., Walker, N. F., Tebruegge, M., Schiff, H. F., Ugarte-Gil, C., et al. (2020). Comprehensive plasma proteomic profiling reveals biomarkers for active tuberculosis. JCI Insight 5 (18), e137427. doi: 10.1172/jci.insight.137427
Getahun, H., Matteelli, A., Abubakar, I., Aziz, M. A., Baddeley, A., Barreira, D., et al. (2015). Management of latent Mycobacterium tuberculosis infection: WHO guidelines for low tuberculosis burden countries. Eur. Respir. J. 46, 1563–1576. doi: 10.1183/13993003.01245-2015
Gupta-Wright, A., Denkinger, C. M. (2024). Advances in TB diagnostics: A critical element for the elimination toolkit. Indian J. Med. Res. 159, 391–394. doi: 10.25259/ijmr_261_2024
Hamada, Y., Cirillo, D. M., Matteelli, A., Penn-Nicholson, A., Rangaka, M. X., Ruhwald, M. (2021). Tests for tuberculosis infection: landscape analysis. Eur. Respir. J. 58 (5), 2100167. doi: 10.1183/13993003.00167-2021
Han, Y.-S., Chen, J.-X., Li, Z.-B., Chen, J., Yi, W.-J., Huang, H., et al. (2021). Identification of potential lipid biomarkers for active pulmonary tuberculosis using ultra-high-performance liquid chromatography-tandem mass spectrometry. Exp. Biol. Med. 246, 387–399. doi: 10.1177/1535370220968058
He, J., Xiong, J., Huang, Y. (2024). miR-29 as diagnostic biomarkers for tuberculosis: a systematic review and meta-analysis. Front. Public Health 12. doi: 10.3389/fpubh.2024.1384510
Hu, X., Liao, S., Bai, H., Gupta, S., Zhou, Y., Zhou, J., et al. (2020). Long noncoding RNA and predictive model to improve diagnosis of clinically diagnosed pulmonary tuberculosis. J. Clin. Microbiol. 58 (7), e01973-19. doi: 10.1128/jcm.01973-19
Hu, X., Liao, S., Bai, H., Wu, L., Wang, M., Wu, Q., et al. (2019). Integrating exosomal microRNAs and electronic health data improved tuberculosis diagnosis. EBioMedicine 40, 564–573. doi: 10.1016/j.ebiom.2019.01.023
Huang, Z., Su, R., Qing, C., Peng, Y., Luo, Q., Li, J. (2018b). Plasma Circular RNAs hsa_circ_0001953 and hsa_circ_0009024 as Diagnostic Biomarkers for Active Tuberculosis. Front. Microbiol. 9. doi: 10.3389/fmicb.2018.02010
Huang, Z., Su, R., Yao, F., Peng, Y., Luo, Q., Li, J. (2018c). Circulating circular RNAs hsa_circ_0001204 and hsa_circ_0001747 act as diagnostic biomarkers for active tuberculosis detection. Int. J. Clin. Exp. Pathol. 11, 586–594.
Huang, Z.-K., Yao, F.-Y., Xu, J.-Q., Deng, Z., Su, R.-G., Peng, Y.-P., et al. (2018a). Microarray expression profile of circular RNAs in peripheral blood mononuclear cells from active tuberculosis patients. Cell. Physiol. Biochem. 45, 1230–1240. doi: 10.1159/000487454
Hung, N. V., Sy, D. N., Anthony, R. M., Cobelens, F. G., van Soolingen, D. (2007). Fluorescence microscopy for tuberculosis diagnosis. Lancet Infect. Dis. 7, 238–239; author reply 239-240. doi: 10.1016/s1473-3099(07)70059-2
Jasenosky, L. D., Scriba, T. J., Hanekom, W. A., Goldfeld, A. E. (2015). T cells and adaptive immunity to Mycobacterium tuberculosis in humans. Immunol. Rev. 264, 74–87. doi: 10.1111/imr.12274
Jiang, J., Li, Z., Chen, C., Jiang, W., Xu, B., Zhao, Q. (2021). Metabolomics strategy assisted by transcriptomics analysis to identify potential biomarkers associated with tuberculosis. Infect. Drug Resist. 14, 4795–4807. doi: 10.2147/idr.S330493
Kaushik, A. C., Wu, Q., Lin, L., Li, H., Zhao, L., Wen, Z., et al. (2021). Exosomal ncRNAs profiling of mycobacterial infection identified miRNA-185-5p as a novel biomarker for tuberculosis. Brief Bioinform. 22 (6), bbab210. doi: 10.1093/bib/bbab210
Kavallaris, M., Marshall, G. M. (2005). Proteomics and disease: opportunities and challenges. Med. J. Aust. 182, 575–579. doi: 10.5694/j.1326-5377.2005.tb06817.x
Khan, A., Khan, N., Singh, R. (2024). Tuberculosis diagnosis versus GeneXpert(®)MTB/RIF formats. Bioanalysis 16, 843–848. doi: 10.1080/17576180.2024.2349423
Kotei, E., Thirunavukarasu, R. (2022). Computational techniques for the automated detection of mycobacterium tuberculosis from digitized sputum smear microscopic images: A systematic review. Prog. Biophys. Mol. Biol. 171, 4–16. doi: 10.1016/j.pbiomolbio.2022.03.004
Koyuncu, D., Niazi, M. K. K., Tavolara, T., Abeijon, C., Ginese, M. L., Liao, Y., et al. (2021). CXCL1: A new diagnostic biomarker for human tuberculosis discovered using Diversity Outbred mice. PloS Pathog 17, e1009773. doi: 10.1371/journal.ppat.1009773
Lamarca, A., Barriuso, J., Chander, A., McNamara, M. G., Hubner, R. A., ÓReilly, D., et al. (2019). (18)F-fluorodeoxyglucose positron emission tomography ((18)FDG-PET) for patients with biliary tract cancer: Systematic review and meta-analysis. J. Hepatol. 71, 115–129. doi: 10.1016/j.jhep.2019.01.038
Li, Z. B., Han, Y. S., Wei, L. L., Shi, L. Y., Yi, W. J., Chen, J., et al. (2020). Screening and identification of plasma lncRNAs uc.48+ and NR_105053 as potential novel biomarkers for cured pulmonary tuberculosis. Int. J. Infect. Dis. 92, 141–150. doi: 10.1016/j.ijid.2020.01.005
Li, Z., Hu, Y., Zou, F., Gao, W., Feng, S., Chen, G., et al. (2025). Assessing the risk of TB progression: Advances in blood-based biomarker research. Microbiol. Res. 292, 128038. doi: 10.1016/j.micres.2024.128038
Li, Y., Wang, X., Teng, D., Chen, H., Wang, M., Wang, J., et al. (2019). Identification of the ligands of TCRγδ by screening the immune repertoire of γδT cells from patients with tuberculosis. Front. Immunol. 10. doi: 10.3389/fimmu.2019.02282
Lu, Q., Liu, J., Yu, Y., Liang, H. F., Zhang, S. Q., Li, Z. B., et al. (2022). ALB, HP, OAF and RBP4 as novel protein biomarkers for identifying cured patients with pulmonary tuberculosis by DIA. Clin. Chim. Acta 535, 82–91. doi: 10.1016/j.cca.2022.08.002
Luo, H. L., Peng, Y., Luo, H., Zhang, J. A., Liu, G. B., Xu, H., et al. (2020). Circular RNA hsa_circ_0001380 in peripheral blood as a potential diagnostic biomarker for active pulmonary tuberculosis. Mol. Med. Rep. 21, 1890–1896. doi: 10.3892/mmr.2020.10992
MacLean, E., Kohli, M., Weber, S. F., Suresh, A., Schumacher, S. G., Denkinger, C. M., et al. (2020). Advances in molecular diagnosis of tuberculosis. J. Clin. Microbiol. 58 (1), 17741. doi: 10.1128/jcm.01582-19
Mamishi, S., Mahmoudi, S., Banar, M., Hosseinpour Sadeghi, R., Marjani, M., Pourakbari, B. (2019). Diagnostic accuracy of interferon (IFN)-γ inducible protein 10 (IP-10) as a biomarker for the discrimination of active and latent tuberculosis. Mol. Biol. Rep. 46, 6263–6269. doi: 10.1007/s11033-019-05067-0
Mandalakas, A. M., Detjen, A. K., Hesseling, A. C., Benedetti, A., Menzies, D. (2011). Interferon-gamma release assays and childhood tuberculosis: systematic review and meta-analysis. Int. J. Tuberc. Lung Dis. 15, 1018–1032. doi: 10.5588/ijtld.10.0631
Massi, M. N., Hidayah, N., Handayani, I., Iskandar, I. W., Djannah, F., Angria, N., et al. (2023). microRNA hsa-miR-425-5p and hsa-miR-4523 expressions as biomarkers of active pulmonary tuberculosis, latent tuberculosis infection, and lymph node tuberculosis. Noncoding RNA Res. 8, 527–533. doi: 10.1016/j.ncrna.2023.07.001
Mattick, J. S., Amaral, P. P., Carninci, P., Carpenter, S., Chang, H. Y., Chen, L.-L., et al. (2023). Long non-coding RNAs: definitions, functions, challenges and recommendations. Nat. Rev. Mol. Cell Biol. 24, 430–447. doi: 10.1038/s41580-022-00566-8
Meaza, A., Kebede, A., Yaregal, Z., Dagne, Z., Moga, S., Yenew, B., et al. (2017). Evaluation of genotype MTBDRplus VER 2.0 line probe assay for the detection of MDR-TB in smear positive and negative sputum samples. BMC Infect. Dis. 17, 280. doi: 10.1186/s12879-017-2389-6
Meng, Z., Wang, M., Guo, S., Zhou, Y., Lyu, M., Hu, X., et al. (2021). Novel long non-coding RNA and LASSO prediction model to better identify pulmonary tuberculosis: A case-control study in China. Front. Mol. Biosci. 8. doi: 10.3389/fmolb.2021.632185
Miggiano, R., Rizzi, M., Ferraris, D. M. (2020). Mycobacterium tuberculosis pathogenesis, infection prevention and treatment. Pathogens 9 (5), 385. doi: 10.3390/pathogens9050385
Mindru, R., Spinu, V., Popescu, O. (2016). LPA or GeneXpert in the diagnosis of multidrug-resistant tuberculosis. Pneumologia 65, 76–80.
Mota Carvalho, T. F., Santos, V. L. A., Silva, J. C. F., Figueredo, L. J. A., de Miranda, S. S., Duarte, R. O., et al. (2023). A systematic review and repeatability study on the use of deep learning for classifying and detecting tuberculosis bacilli in microscopic images. Prog. Biophys. Mol. Biol. 180-181, 1–18. doi: 10.1016/j.pbiomolbio.2023.03.002
Naidu, A., Nayak, S. S., Lulu, S. S., Sundararajan, V. (2023). Advances in computational frameworks in the fight against TB: The way forward. Front. Pharmacol. 14. doi: 10.3389/fphar.2023.1152915
Nogueira, B. M. F., Krishnan, S., Barreto-Duarte, B., Araújo-Pereira, M., Queiroz, A. T. L., Ellner, J. J., et al. (2022). Diagnostic biomarkers for active tuberculosis: progress and challenges. EMBO Mol. Med. 14, e14088. doi: 10.15252/emmm.202114088
Pan, L. P., Gao, M. Q., Jia, H. Y., Huang, M. L., Wei, R. R., Sun, Q., et al. (2021). Diagnostic performance of a novel Mycobacterium Tuberculosis specific T-Cell based assay for tuberculosis. Zhonghua Jie He He Hu Xi Za Zhi 44, 443–449. doi: 10.3760/cma.j.cn112147-20200821-00916
Pandey, D., Ghosh, D. (2024). Proteomics-based host-specific biomarkers for tuberculosis: The future of TB diagnosis. J. Proteomics 305, 105245. doi: 10.1016/j.jprot.2024.105245
Penn-Nicholson, A., Georghiou, S. B., Ciobanu, N., Kazi, M., Bhalla, M., David, A., et al. (2022). Detection of isoniazid, fluoroquinolone, ethionamide, amikacin, kanamycin, and capreomycin resistance by the Xpert MTB/XDR assay: a cross-sectional multicentre diagnostic accuracy study. Lancet Infect. Dis. 22, 242–249. doi: 10.1016/s1473-3099(21)00452-7
Pillay, S., Steingart, K. R., Davies, G. R., Chaplin, M., De Vos, M., Schumacher, S. G., et al. (2022). Xpert MTB/XDR for detection of pulmonary tuberculosis and resistance to isoniazid, fluoroquinolones, ethionamide, and amikacin. Cochrane Database Syst. Rev. 5, Cd014841. doi: 10.1002/14651858.CD014841.pub2
Pinhata, J. M. W., Brandao, A. P., Gallo, J. F., Oliveira, R. S., Ferrazoli, L. (2023). GenoType MTBDRsl for detection of second-line drugs and ethambutol resistance in multidrug-resistant Mycobacterium tuberculosis isolates at a high-throughput laboratory. Diagn. Microbiol. Infect. Dis. 105, 115856. doi: 10.1016/j.diagmicrobio.2022.115856
Prakash Babu, S., Ezhumalai, K., Raghupathy, K., Sundaresan, M., Jain, K., Narasimhan, P. B., et al. (2023). Comparison of IGRA and TST in the diagnosis of latent tuberculosis among women of reproductive age in South India. Indian J. Tuberc. 70, 12–16. doi: 10.1016/j.ijtb.2022.03.011
Qu, S., Yang, X., Li, X., Wang, J., Gao, Y., Shang, R., et al. (2015). Circular RNA: A new star of noncoding RNAs. Cancer Lett. 365, 141–148. doi: 10.1016/j.canlet.2015.06.003
Rodriguez-Takeuchi, S. Y., Renjifo, M. E., Medina, F. J. (2019). Extrapulmonary tuberculosis: pathophysiology and imaging findings. Radiographics 39, 2023–2037. doi: 10.1148/rg.2019190109
Ruiz Esparza Garrido, R., Velázquez Flores, M. (2023). Circular RNAs: the next level of gene regulation. Am. J. Transl. Res. 15, 6122–6135.
Sampath, P., Rajamanickam, A., Thiruvengadam, K., Natarajan, A. P., Hissar, S., Dhanapal, M., et al. (2023). Plasma chemokines CXCL10 and CXCL9 as potential diagnostic markers of drug-sensitive and drug-resistant tuberculosis. Sci. Rep. 13, 7404. doi: 10.1038/s41598-023-34530-z
Satam, H., Joshi, K., Mangrolia, U., Waghoo, S., Zaidi, G., Rawool, S., et al. (2023). Next-generation sequencing technology: current trends and advancements. Biol. (Basel) 12 (5), 286. doi: 10.3390/biology12070997
Schiff, H. F., Walker, N. F., Ugarte-Gil, C., Tebruegge, M., Manousopoulou, A., Garbis, S. D., et al. (2024). Integrated plasma proteomics identifies tuberculosis-specific diagnostic biomarkers. JCI Insight 9 (8), e173273. doi: 10.1172/jci.insight.173273
Schwab, T. C., Perrig, L., Göller, P. C., Guebely de la Hoz, F. F., Lahousse, A. P., Minder, B., et al. (2024). Targeted next-generation sequencing to diagnose drug-resistant tuberculosis: a systematic review and meta-analysis. Lancet Infect. Dis. 24, 1162–1176. doi: 10.1016/s1473-3099(24)00263-9
Seung, K. J., Keshavjee, S., Rich, M. L. (2015). Multidrug-resistant tuberculosis and extensively drug-resistant tuberculosis. Cold Spring Harb. Perspect. Med. 5, a017863. doi: 10.1101/cshperspect.a017863
Shaukat, S. N., Eugenin, E., Nasir, F., Khanani, R., Kazmi, S. U. (2023). Identification of immune biomarkers in recent active pulmonary tuberculosis. Sci. Rep. 13, 11481. doi: 10.1038/s41598-023-38372-7
Skoura, E., Zumla, A., Bomanji, J. (2015). Imaging in tuberculosis. Int. J. Infect. Dis. 32, 87–93. doi: 10.1016/j.ijid.2014.12.007
Sossen, B., Richards, A. S., Heinsohn, T., Frascella, B., Balzarini, F., Oradini-Alacreu, A., et al. (2023). The natural history of untreated pulmonary tuberculosis in adults: a systematic review and meta-analysis. Lancet Respir. Med. 11, 367–379. doi: 10.1016/s2213-2600(23)00097-8
Steingart, K. R., Ng, V., Henry, M., Hopewell, P. C., Ramsay, A., Cunningham, J., et al. (2006). Sputum processing methods to improve the sensitivity of smear microscopy for tuberculosis: a systematic review. Lancet Infect. Dis. 6, 664–674. doi: 10.1016/s1473-3099(06)70602-8
Su, K. Y., Yan, B. S., Chiu, H. C., Yu, C. J., Chang, S. Y., Jou, R., et al. (2017). Rapid sputum multiplex detection of the M. tuberculosis complex (MTBC) and resistance mutations for eight antibiotics by nucleotide MALDI-TOF MS. Sci. Rep. 7, 41486. doi: 10.1038/srep41486
Sun, W., He, X., Zhang, X., Wang, X., Lin, W., Wang, X., et al. (2022). Diagnostic value of lncRNA NORAD in pulmonary tuberculosis and its regulatory role in Mycobacterium tuberculosis infection of macrophages. Microbiol. Immunol. 66, 433–441. doi: 10.1111/1348-0421.12986
Tabriz, N. S., Skak, K., Kassayeva, L. T., Omarkulov, B. K., Grigolashvili, M. A. (2020). Efficacy of the Xpert MTB/RIF assay in multidrug-resistant tuberculosis. Microb. Drug Resist. 26, 997–1004. doi: 10.1089/mdr.2019.0326
Tan, Y., Tan, Y., Li, J., Hu, P., Guan, P., Kuang, H., et al. (2021). Combined IFN-γ and IL-2 release assay for detect active pulmonary tuberculosis: a prospective multicentre diagnostic study in China. J. Transl. Med. 19, 289. doi: 10.1186/s12967-021-02970-8
Tomasello, G., Foroughi, F., Padron, D., Moreno, A., Banaei, N. (2022). Evaluation of MetaSystems automated fluorescent microscopy system for the machine-assisted detection of acid-fast bacilli in clinical samples. J. Clin. Microbiol. 60, e0113122. doi: 10.1128/jcm.01131-22
Vinayavekhin, N., Homan, E. A., Saghatelian, A. (2010). Exploring disease through metabolomics. ACS Chem. Biol. 5, 91–103. doi: 10.1021/cb900271r
Vrieling, F., Alisjahbana, B., Sahiratmadja, E., van Crevel, R., Harms, A. C., Hankemeier, T., et al. (2019). Plasma metabolomics in tuberculosis patients with and without concurrent type 2 diabetes at diagnosis and during antibiotic treatment. Sci. Rep. 9, 18669. doi: 10.1038/s41598-019-54983-5
Walzl, G., McNerney, R., du Plessis, N., Bates, M., McHugh, T. D., Chegou, N. N., et al. (2018). Tuberculosis: advances and challenges in development of new diagnostics and biomarkers. Lancet Infect. Dis. 18, e199–e210. doi: 10.1016/s1473-3099(18)30111-7
Wang, G., Huang, M., Jing, H., Jia, J., Dong, L., Zhao, L., et al. (2022). The practical value of Xpert MTB/RIF ultra for diagnosis of pulmonary tuberculosis in a high tuberculosis burden setting: a prospective multicenter diagnostic accuracy study. Microbiol. Spectr. 10, e0094922. doi: 10.1128/spectrum.00949-22
Wang, M., Liu, H., Ren, J., Huang, Y., Deng, Y., Liu, Y., et al. (2023). Enzyme-assisted nucleic acid amplification in molecular diagnosis: A review. Biosens. (Basel) 13. doi: 10.3390/bios13020160
Wang, L., Xie, B., Zhang, P., Ge, Y., Wang, Y., Zhang, D. (2019). LOC152742 as a biomarker in the diagnosis of pulmonary tuberculosis infection. J. Cell. Biochem. 120, 8949–8955. doi: 10.1002/jcb.v120.6
Wang, M. Q., Zheng, Y. F., Hu, Y. Q., Huang, J. X., Yuan, Z. X., Wu, Z. Y., et al. (2025). Diagnostic accuracy of Xpert MTB/RIF Ultra for detecting pulmonary tuberculosis and rifampicin resistance: a systematic review and meta-analysis. Eur. J. Clin. Microbiol. Infect. Dis 44 (3), 681–702. doi: 10.1007/s10096-024-05032-1
Wei, J. W., Huang, K., Yang, C., Kang, C. S. (2017). Non-coding RNAs as regulators in epigenetics (Review). Oncol. Rep. 37, 3–9. doi: 10.3892/or.2016.5236
Xie, L., Dang, Y., Xie, J. (2018). Post-translational modification and regulatory network of Mycobacterium tuberculosis antibiotic resistance. Sheng Wu Gong Cheng Xue Bao 34, 1279–1287. doi: 10.13345/j.cjb.170530
Xie, Y. L., Eichberg, C., Hapeela, N., Nakabugo, E., Anyango, I., Arora, K., et al. (2024). Xpert MTB/RIF Ultra versus mycobacterial growth indicator tube liquid culture for detection of Mycobacterium tuberculosis in symptomatic adults: a diagnostic accuracy study. Lancet Microbe 5, e520–e528. doi: 10.1016/s2666-5247(24)00001-6
Yan, M., Zhao, Z., Wu, T., Liu, T., Xu, G., Xu, H., et al. (2023). Highly sensitive detection of complicated mutations of drug resistance in mycobacterium tuberculosis using a simple, accurate, rapid, and low-cost tailored-design competitive wild-type blocking assay. Small Methods 7, e2201322. doi: 10.1002/smtd.202201322
Yayan, J., Rasche, K., Franke, K. J., Windisch, W., Berger, M. (2024). FDG-PET-CT as an early detection method for tuberculosis: a systematic review and meta-analysis. BMC Public Health 24, 2022. doi: 10.1186/s12889-024-19495-6
Yi, Z., Gao, K., Li, R., Fu, Y. (2018). Dysregulated circRNAs in plasma from active tuberculosis patients. J. Cell Mol. Med. 22, 4076–4084. doi: 10.1111/jcmm.13684
Ying, S. Y., Chang, D. C., Lin, S. L. (2008). The microRNA (miRNA): overview of the RNA genes that modulate gene function. Mol. Biotechnol. 38, 257–268. doi: 10.1007/s12033-007-9013-8
Ying, C., He, C., Xu, K., Li, Y., Zhang, Y., Wu, W. (2022). Progress on diagnosis and treatment of latent tuberculosis infection. Zhejiang Da Xue Xue Bao Yi Xue Ban 51, 691–696. doi: 10.3724/zdxbyxb-2022-0445
Zhang, D., Rubio Rodríguez-Kirby, L. A., Lin, Y., Song, M., Wang, L., Wang, L., et al. (2024). Spatial dynamics of mammalian brain development and neuroinflammation by multimodal tri-omics mapping. bioRxiv, 2024.07.28.605493. doi: 10.1101/2024.07.28.605493
Zhang, X., Zhang, Q., Wu, Q., Tang, H., Ye, L., Zhang, Q., et al. (2020). Integrated analyses reveal hsa_circ_0028883 as a diagnostic biomarker in active tuberculosis. Infect. Genet. Evol. 83, 104323. doi: 10.1016/j.meegid.2020.104323
Zhang, Y., Zhang, X., Zhao, Z., Zheng, Y., Xiao, Z., Li, F. (2019). Integrated bioinformatics analysis and validation revealed potential immune-regulatory miR-892b, miR-199b-5p and miR-582-5p as diagnostic biomarkers in active tuberculosis. Microb. Pathog 134, 103563. doi: 10.1016/j.micpath.2019.103563
Keywords: tuberculosis, blood-based biomarkers, diagnostic technologies, artificial intelligence, global health
Citation: Yang Z, Li J, Shen J, Cao H, Wang Y, Hu S, Du Y, Wang Y, Yan Z, Xie L, Li Q, Gomaa SE, Liu S, Li X and Li J (2025) Recent progress in tuberculosis diagnosis: insights into blood-based biomarkers and emerging technologies. Front. Cell. Infect. Microbiol. 15:1567592. doi: 10.3389/fcimb.2025.1567592
Received: 14 February 2025; Accepted: 07 April 2025;
Published: 08 May 2025.
Edited by:
Ying Luo, University of Texas Southwestern Medical Center, United StatesReviewed by:
Alin Laurentiu Tatu, Dunarea de Jos University, RomaniaFu Gao, Yale University, United States
Bide Chen, Duke University, United States
Zixu Wang, Bicycle Therapeutics, United Kingdom
Li Meng, University of Texas Southwestern Medical Center, United States
Copyright © 2025 Yang, Li, Shen, Cao, Wang, Hu, Du, Wang, Yan, Xie, Li, Gomaa, Liu, Li and Li. This is an open-access article distributed under the terms of the Creative Commons Attribution License (CC BY). The use, distribution or reproduction in other forums is permitted, provided the original author(s) and the copyright owner(s) are credited and that the original publication in this journal is cited, in accordance with accepted academic practice. No use, distribution or reproduction is permitted which does not comply with these terms.
*Correspondence: Shejuan Liu, MTAyMTAwMTVAaGVudS5lZHUuY24=; Xianghui Li, MTAxOTAxNThAdmlwLmhlbnUuZWR1LmNu
†These authors have contributed equally to this work