- 1Oral Sciences Research Group, Special Needs Unit, Department of Surgery and Medical-Surgical Specialties, School of Medicine and Dentistry, Universidade de Santiago de Compostela, Health Research Institute of Santiago (IDIS), Santiago de Compostela, Spain
- 2Oral Pathology and Rehabilitation Research Unit (UNIPRO), University Institute of Health Sciences (IUCS-CESPU), Gandra, Portugal
- 3Proteomic Unit, Health Research Institute of Santiago de Compostela (IDIS), Santiago de Compostela, Spain
Introduction: Proteomic techniques are useful to analyse the periodontal proteome in gingival crevicular fluid (GCF) and saliva. However, few investigations have assessed and compared the GCF and salivary proteomes. Therefore, this research aims to analyse the proteome structure and compare protein expression in these fluids between individuals with periodontal health and those with periodontitis.
Methods: GCF and saliva were collected from 44 periodontally healthy subjects and 41 with periodontitis (stages III-IV). Samples were analysed using sequential window acquisition of all theoretical mass spectra (SWATH-MS), and proteins were identified employing the UniProt database. The periodontal proteome structure was assessed using principal component analysis (PCA). Differential protein expression was defined as an adjusted p-value <0.05 combined with a fold-change ≥2 (upregulated) or ≤0.5 (downregulated).
Results: 250 abundant proteins were quantified in GCF and 377 in saliva (238 in common). The proteome structure was different in periodontitis compared to periodontal health in both oral fluids. In GCF, 63 (25.2%) proteins were differentially expressed, with 38 upregulated and 25 downregulated in periodontitis. The most overexpressed proteins were haemoglobin subunits (Hbs) beta (fold-change of 5.06) and alpha (4.35), carbonic anhydrase 1 (4.28), and protein S100-P (4.27). Among the underexpressed proteins, 14 were keratins, with type II cytoskeletal 6B being the most downregulated (0.10), together with glyceraldehyde-3-phosphate dehydrogenase (0.12) and zymogen granule protein 16 homolog B (0.13).
In saliva, 59 (15.7%) proteins were differentially expressed, with 55 upregulated and four downregulated in periodontitis. Twenty-nine proteins showed a fold-change ≥4, highlighting beta-2-microglobulin (44.14), keratin, type I cytoskeletal 13 (36.23), neutrophil defensin 1 (25.08), proteins S100-A9 (12.30), A8 (10.61), A12 (4.76), and P (4.72), annexin A1 (9.34), lysozyme C (4.98), immunoglobulin heavy constant alpha 1 (4.45), resistin (4.37), and Hbs beta (4.20) and alpha (4.06). The most downregulated protein was lipocalin-1 (0.35). Fourteen proteins were differentially expressed in GCF and saliva, where seven were keratins being underexpressed in GCF but overexpressed in saliva.
Conclusion: Periodontitis alters the periodontal proteome structure and the expression of numerous abundant proteins in GCF and saliva. However, proteins expressed vary qualitatively and quantitatively, indicating different expression patterns between oral fluids.
Introduction
Periodontitis is a chronic inflammatory disease that affects the gingiva, the alveolar bone, and the periodontal ligament surrounding the teeth (Kinane et al., 2017). Periodontitis not only has an impact on oral health but is also related to numerous systemic diseases, being especially relevant in their association with cardiovascular diseases or diabetes (Monsarrat et al., 2016). Today, periodontitis remains one of the most prevalent diseases worldwide, affecting almost 45% of the world’s population (Eke et al., 2020).
Several proteins have been shown to play an essential role in the initiation, progression, and severity of periodontitis (Bostanci and Belibasakis, 2018). However, other relevant proteins have not yet been identified, and their associations with the disease remain undiscovered (Bostanci and Belibasakis, 2018). Analyses using high-throughput proteomic techniques enable the periodontal proteome to be studied in greater detail, contributing to the determination of extensive and comprehensive protein profiles associated with periodontal diseases (Bostanci and Bao, 2017; Bostanci and Belibasakis, 2018).
Considering the range of proteomic techniques available, sequential window acquisition of all theoretical mass spectra (SWATH-MS) stands out as a particularly effective approach (Anjo et al., 2017). SWATH-MS is an untargeted, data-independent acquisition (DIA), label-free quantification technique designed to eliminate the variability introduced by chemical modifications, expand the dynamic range of quantification, and optimise the detection of data present in a sample (Frederick and Ciborowski, 2016). This method was developed to combine the proteome coverage potential of data-dependent acquisition (DDA) approaches with the quantitative consistency of targeted DIA proteomics (e.g., selected reaction monitoring – SRM) (Ludwig et al., 2018). These features make SWATH-MS a powerful strategy for discovering novel biomarkers (Anjo et al., 2017).
The most commonly used sample types for studying the periodontal proteome are gingival crevicular fluid (GCF) and saliva. Both can be easily collected in a non-invasive manner, making them particularly suitable for potential translation into clinical diagnostic tools (Guzman et al., 2014). The analysis of these fluids using various proteomic approaches has enabled the identification and quantification of numerous proteins with significantly different expression profiles depending on periodontal status (Ahmad et al., 2025). However, few studies have simultaneously analysed the GCF and salivary proteome collected from the same individuals (Grant et al., 2022; Tang et al., 2019). Additionally, SWATH-MS has previously proven useful in identifying novel GCF and salivary biomarkers with strong diagnostic potential for periodontitis (Blanco-Pintos et al., 2024, 2025a). Nevertheless, to date, SWATH-MS has not been employed to comprehensively study and compare the proteomic profile and protein expression between periodontal health and periodontitis.
Accordingly, this cross-sectional study aimed to (1) analyse the periodontal proteome structure and (2) compare differential protein expression in GCF and saliva between individuals with periodontal health and those with periodontitis using SWATH-MS.
Material and methods
The complete analysis protocol applied in the present study is detailed in Figure 1.
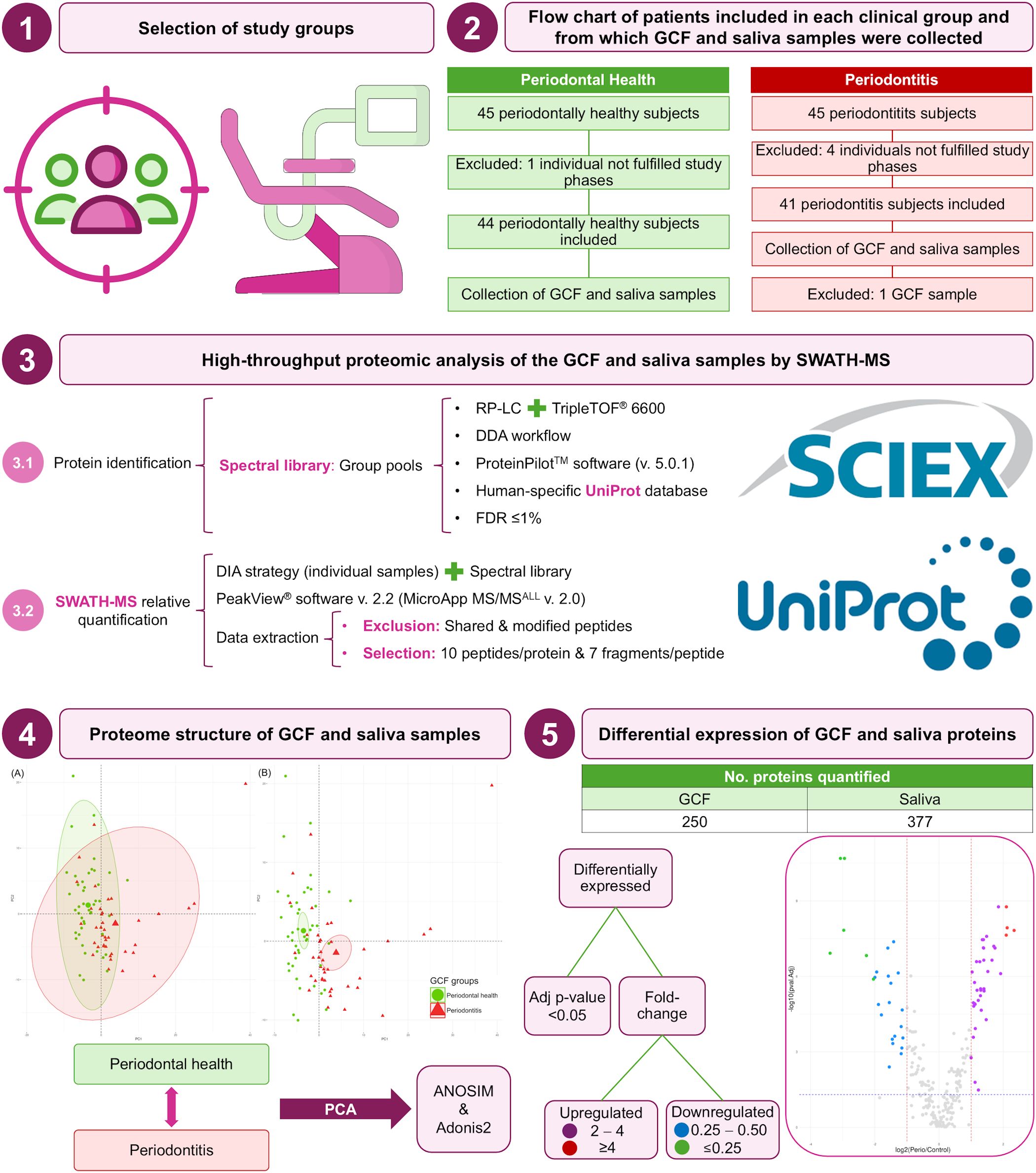
Figure 1. Complete analysis protocol of this study. Adj, adjusted; ANOSIM, analysis of the similarities; DDA, data-dependent acquisition; DIA, data-independent acquisition; FDR, false discovery rate; GCF, gingival crevicular fluid; LC, liquid-chromatography; No., number; PCA, principal component analysis; RP, reversed-phase; SWATH-MS, sequential window acquisition of all theoretical mass spectra; TOF, time-of-flight; v., version.
Selection of study groups
A convenience sample of 90 eligible participants, comprising 45 periodontally healthy subjects (control group) and 45 individuals affected by untreated periodontitis (periodontitis group) was recruited between 2018 and 2021 from 250 consecutive patients in the general population who were referred for assessment of their oral health status to the School of Medicine and Dentistry (Universidade de Santiago de Compostela, Spain) and the Instituto Superior de Ciências da Saúde-Norte, Cooperativa de Ensino Superior, Politécnico e Universitário (CESPU, Gandra, Paredes, Portugal).
Patients were selected if they fulfilled the following inclusion criteria: 1) aged 20 to 75; 2) had at least 10 natural teeth; 3) no previous periodontal treatment; 4) had no medical history of diabetes mellitus, hepatic or renal disease, or other severe medical conditions or transmittable illnesses; 5) had not taken systemic antimicrobials in the previous six months; 6) had no intake of antiinflammatory medication in the previous four months; 7) did not routinely use oral antiseptics; 8) had no history of alcohol or drug abuse; 9) were not pregnant or breastfeeding; and 10) used no orthodontic appliances.
Two experienced and previously calibrated dentists performed the periodontal diagnostics, with intra- and interexaminer intraclass correlation coefficients (ICC) greater than 0.950. Bleeding on probing (BOP) and the bacterial plaque level (BPL) were recorded for the entire mouth on a binary scale (presence/absence) at six sites per tooth. The probing pocket depth (PPD) and clinical attachment loss (CAL) were recorded using a PCP-UNC 15 probe at six sites per tooth. PPD was measured as the distance from the gingival margin to the base of the gingival sulcus. A positive value for gingival recession (REC) was assessed for the apical displacement of the gingival margin in relation to the cementoenamel junction. Considering an average biological width (BW) of 2 mm (Mulla et al., 2023), CAL was defined for three conditions: (1) PPD <4 mm and REC of 0 mm, CAL was equal to 0 mm; (2) PPD ≥4 mm and REC of 0 mm, CAL was equal to PPD subtracting BW; and (3) if REC >0 mm, CAL was PPD plus REC.
Standardised teeth radiographs were acquired to assess the alveolar bone status. The diagnosis of periodontitis was based on the clinical and radiographic information thus obtained. The control group included subjects who were periodontally healthy according to the criteria in the Classification of Periodontal Diseases and Conditions (Caton et al., 2018; Tonetti et al., 2018), i.e., BOP <10%, no location with a PPD ≥4 mm, and no radiographic evidence of alveolar bone loss. This classification was also used for the periodontitis group, which was composed of patients diagnosed with stage III or IV and grades B or C generalised periodontitis.
A questionnaire was used to evaluate the smoking habits of the participants, collecting information on their smoking status (non-smoker, former smoker, current smoker), the duration of their smoking status (as a former or current smoker), and the number of cigarettes consumed per day. Non-smokers were considered those who had never smoked, current smokers were those who had been smoking for at least one year, and former smokers were those who stopped doing so at least one year before, irrespective of how long they had been smoking.
The research was conducted following the principles of the Declaration of Helsinki (revised in 2013) on human experimentation studies (World Medical Association, 2013). The protocol was approved by the Galician Clinical Research Ethics Committee (registration numbers 2018/295 and 2021/417) and the Instituto Superior de Ciências da Saúde-Norte, CESPU (registration number 35/CEIUCS/2019). All the participants provided written informed consent to their involvement in the study.
Collection of the GCF and saliva samples and preparation for the mass spectrometry analysis
The GCF and saliva samples were collected one or two weeks after the initial examination. The GCF samples from the control and periodontal subjects were collected and pooled from five non-adjacent proximal sites. The samples were taken from teeth in quadrants 1 and 3 in the periodontally healthy controls. In patients with periodontitis, samples were collected from the sites with the deepest PPD values. Two paper strips were inserted into the gingival sulcus or periodontal pocket for 30 seconds. In cases of visible contamination with blood, samples were discarded, and new sites were sampled. The strips were collected and inserted into labelled tubes containing 300 mL of 0.01 M phosphate-buffered saline (PBS) with a pH of 7.2.
Immediately before the collection of the GCF samples, one ml of unstimulated whole saliva was collected from each participant using the spitting method at least one hour after the last meal and after the last brushing (Navazesh and Christensen, 1982). Subsequently, the GCF and saliva samples were stored at -80°C until further analysis.
The total protein concentrations of the samples were quantified using the reducing-agent and detergent-compatible (RC DC™) Protein Assay Kit (BIO-RAD, Hercules, CA, USA), following the manufacturer’s protocol. Approximately 50 μg of protein from each sample was precipitated using the methanol/chloroform (MeOH/CHCl3) method to remove potential contaminants such as deoxyribonucleic acid (DNA), ribonucleic acid (RNA), and lipids. Purified GCF and salivary proteins were loaded onto a 10% sodium dodecyl sulfate-polyacrylamide gel electrophoresis (SDS-PAGE) gel to concentrate them into a band for in-gel digestion. Electrophoresis was interrupted once the front had penetrated 3 mm into the gel (Bonzon-Kulichenko et al., 2011; Perez-Hernandez et al., 2013). The protein band was detected with SYPRO Ruby fluorescent staining (Lonza, Basel, Switzerland) and cut out. We then proceeded to manual in-gel tryptic digestion, as previously explained (Shevchenko et al., 1996), but with slight modifications. The gel pieces were reduced with 10 mM dithiothreitol (Sigma-Aldrich, St. Louis, Missouri, USA) in 50 mM ammonium bicarbonate (Sigma-Aldrich) and alkylated with 55 mM iodoacetamide (Sigma-Aldrich) in 50 mM ammonium bicarbonate.
The gel pieces were then rinsed with 50 mM ammonium bicarbonate in 50% MeOH (HPLC grade, Scharlau, Barcelona, Spain), dehydrated by adding acetonitrile (ACN) (HPLC grade, Scharlau), and dried in a SpeedVac (Savant™). Modified porcine trypsin (Promega, Madison, Wisconsin, USA) was added to the dried gel pieces at a final concentration of 20 ng/μL in 20 mM ammonium bicarbonate and incubated at 37°C for 16 hours. Peptides were extracted three times by way of a 20-minute incubation in 40 μl of 60% ACN in 0.5% acetic acid (HCOOH). The resulting peptide extracts were pooled, concentrated in a SpeedVac, and stored at -20°C until use.
Protein quantification by SWATH-MS
Construction of MS/MS spectral libraries
To obtain a robust representation of the peptides and MS/MS present in the GCF and saliva study groups, four pools of around 10 samples from each group (periodontal health and periodontitis) were prepared after protein digestion using equal amounts of peptides from each sample. In more detail, after peptide resuspension in water plus 0.1% of formic acid with sonication per 10 minutes (the resuspension was made to obtain a µg/µl peptides solution), from each of the resuspended samples/peptides, we used three µl for create the corresponding pool to obtain the spectral library (Blanco-Pintos et al., 2024, 2025a, 2025b; Bugallo-Casal et al., 2025; Casado-Fernández et al., 2024; López-Valverde et al., 2024; Mondelo-Macía et al., 2024; Nóvoa et al., 2025; Rodrigues et al., 2024; Torres Iglesias et al., 2024; Vázquez-Mera et al., 2024). Four µl (around four µg) of each pool were separated using reversed-phase (RP) LC to identify the proteins and create the SWATH library.
The gradient was created with a micro-LC system (Eksigent Technologies nanoLC 400, SCIEX, Redwood City, California, USA) coupled with a TripleTOF® (time-of-flight) 6600 high-speed mass spectrometer (SCIEX) via a microflow ionisation source. A silica-based RP analytical column (C18CL 150×0.30 mm) with a particle size of 3 µm and a pore size of 120 Å (Eksigent, SCIEX) was employed. The samples were pre-washed on a YMC-TRIART C18 pre-column (YMC Technologies, Teknokroma, Barcelona, Spain), which had the same particle and pore sizes, and was connected in-line with the analytical column. The charge pump provided a 0.1% formic acid solution in water at a rate of 10 μl/min. The micropump generated a flow rate of 5 μl/min and operated under gradient elution conditions, employing 0.1% formic acid in water as mobile phase A and 0.1% formic acid in ACN as mobile phase B. Peptides were separated using a 40-minute gradient from 2% to 90% of mobile phase B. The injection volume was 4 μl (approximately 4 μg of protein).
The TripleTOF 6600 (SCIEX) was used for data acquisition, employing a DDA workflow. The source and interface conditions comprised an ion-source voltage (ISVF) of 5500V, a curtain gas (CUR) of 25, a collision energy (CE) of 10, and an ion-source gas 1 (GS1) of 25. The instrument was operated using the software Analyst TF 1.7.1 (SCIEX). Switching criteria were set for ions with a mass-to-charge ratio (m/z) ranging from 350 to 1400, a charge state of 2-5, a mass tolerance of 250 parts per million (ppm), and an abundance threshold of more than 200 counts per second (cps). Selected ions meeting these conditions were excluded for 15 seconds. The instrument was automatically calibrated every four hours using commercial PepCalMix tryptic peptides (SCIEX) as the external calibrants.
After the MS/MS analysis, the data files were processed using ProteinPilotTM 5.0.1 (SCIEX), which employs the ParagonTM algorithm for database search and Progroup™ for data grouping. Files were searched for peptide/protein identification against the UniProt human-specific database (UniProt Consortium, 2014) (https://www.uniprot.org/, UniProt release 2022_02, containing 20387 human proteins), specifying iodoacetamide at cysteine alkylation as a variable modification and methionine oxidation as a fixed modification. All known contaminants (keratins epidermal, trypsin…) were manually excluded. The false discovery rate (FDR) was set at ≤1% for both peptides and proteins (Aggarwal and Yadav, 2016). The MS/MS spectra of the identified peptides were used to generate a spectral library, which subsequently enabled the extraction of SWATH peaks using MicroApp MS/MSALL 2.0 for PeakView 2.2 (SCIEX). Only peptides with a confidence score above 99% (obtained by searching against the UniProt database) were included in the spectral library.
Relative quantification
The GCF and saliva samples were analysed individually, using a DIA method. These were then examined using the equipment and gradients described above for spectral library construction, although a SWATH-MS acquisition strategy was employed instead of a DDA approach. The method involves repeating an acquisition cycle. In particular, 100 TOF MS/MS scans (400 to 1500 m/z, high sensitivity mode, and 50 millisecond acquisition time) were performed using sequential, overlapping precursor isolation windows of varying widths (1 m/z overlap) that cover the entire mass range between 400 and 1250 m/z, obtained with a previous TOF MS scan (400–1500 m/z and 50 millisecond acquisition time) for each cycle. The total cycle time was 6.3 seconds. A SWATH variable window spreadsheet (SCIEX) was used to optimise the width of the 100 variable windows for each set of samples according to the ion density found in the DDA runs.
The extraction of specific data from the trace-ion chromatograms and fragments obtained using the SWATH-MS methodology was performed with the PeakView 2.2 software (SCIEX), utilising MicroApp MS/MSALL 2.0 and the previously created spectral library. Only peak area values integrated based on signal intensity from 10 peptides per protein and 7 fragments per peptide were selected. Shared and modified peptides were excluded from the processing. Five-minute windows and widths of 30 ppm were used to extract the ion chromatograms. SWATH-MS quantification was performed for all the proteins in the ion library identified by ProteinPilot™ with an FDR ≤1%. The retention times (RTs) of the selected peptides for each protein were realigned in each run according to the peptide-indexed RTs obtained in each sample throughout the chromatogram. The areas of each protein in each sample were acquired by combining the peak areas of the 10 peptides and the seven corresponding fragment ions.
The integrated peak areas (processed PeakView® mrkvw files) were exported directly to the MarkerView™ software (SCIEX) for the relative quantitative examination, which is an approach that has been used previously for SWATH-MS data analyses (Agra-Bermejo et al., 2020; Álvarez et al., 2020; Camino et al., 2020, 2022; Chantada-Vázquez et al., 2020; García-Vence et al., 2020; Novelle et al., 2021; Ortea et al., 2018). This export generated three files containing quantitative information on individual ions, the total intensity of the different ions for a particular peptide, and the total intensity of the different peptides for a specific protein. MarkerView™ utilises processing algorithms that accurately identify chromatographic and spectral peaks directly within the raw SWATH data. The software’s alignment of the data compensates for slight variations in mass and RT values, ensuring precise comparisons of identical compounds in different samples. To homogenise the data obtained, a most likely ratio (MLR) normalisation was performed (Colangelo et al., 2015).
Statistical analysis
For the calculation of the sample size, we considered the design of independent groups (periodontal health vs periodontitis), the possibility of using a nonparametric two-tailed test of mean differences between two independent groups, an effect size of 0.65, an alpha error of 0.05 and a statistical power of 0.80, a minimum sample size of 40 subjects in each group was required. Due to the possible loss of patients for various reasons, each study group initially consisted of 45 participants. Sample size calculation was performed with the G*Power program (version 3.1.9.4) (Faul et al., 2007).
Five of the 90 subjects selected were excluded for non-compliance with the project phases. Therefore, the clinical groups were finally composed of 44 periodontally healthy subjects and 41 patients with periodontitis. One GCF sample from those with periodontitis had to be excluded due to a processing error.
The R software (version 4.2.2) (R Core Team, 2022) was used to perform the statistical data analysis of the GCF and saliva samples from the study groups (control and periodontitis).
Comparison of clinical characteristics between control subjects and patients with periodontitis
The Shapiro-Wilk test was used to assess the distribution of quantitative variables. For variables with a normal distribution, the Student’s t-test was applied; otherwise, the Mann-Whitney U test was used. Fisher’s exact test and the Chi-squared test were used to assess the association between qualitative variables and the clinical conditions. A significance level of p<0.05 was established.
Periodontal proteome structure
Principal component analysis (PCA) was employed to visualise the clustering of the GCF and saliva samples in each analysis group. The FactoMineR software (version 2.8) (Lê et al., 2008) was used to perform the PCA, and then the amount of variability explained within each component was assessed using the factoextra package (version 1.0.7) (Kassambara and Mundt, 2020). The vegan software (version 2.6.4) (Oksanen et al., 2022) was used to perform two evaluations: 1) an analysis of the similarities (ANOSIM) between groups; and 2) an analysis of variance, using a similarity matrix via the adonis2 function of the non-parametric test permutational multivariate analysis of variance (PERMANOVA) to that end (Anderson, 2001). A significance level of p<0.05 was established.
Differential protein expression
Concerning all the proteins quantified in GCF and saliva, we evaluated the mean expression difference between the clinical groups using the non-parametric Mann-Whitney U test. The p-values obtained were adjusted using the mutoss package (version 0.1-13) (Team et al., 2023) with the Benjamini-Hochberg correction (Benjamini and Hochberg, 1995). The differential expression was statistically significant if the adjusted p-value was <0.05 (-log10 adjusted p-value=1.30).
Subsequently, the effect size of each protein in all the phases was calculated using the effsize package (version 0.8.1) (Torchiano, 2020) and Cohen’s d (Cohen, 1988). The expression ratio of each protein between conditions was calculated, and a fold-change ≥2 (log2 fold-change ≥1) or ≤0.5 (log2 fold-change ≤-1) was considered significant.
Volcano plots were created from the adjusted p-values and the differential expression of the log2 fold-change. Proteins with an adjusted p-value <0.05 and a log2 fold-change ≥1 (upregulated) or ≤-1 (downregulated) were differentially expressed.
Results
Clinical characteristics
Considering the clinical parameters, full-mouth BPL, BOP, PPD, and CAL levels were significantly higher in patients with periodontitis than in control subjects. Similarly, BOP, PPD and CAL levels from sampled GCF sites were significantly higher in periodontal patients (Table 1).
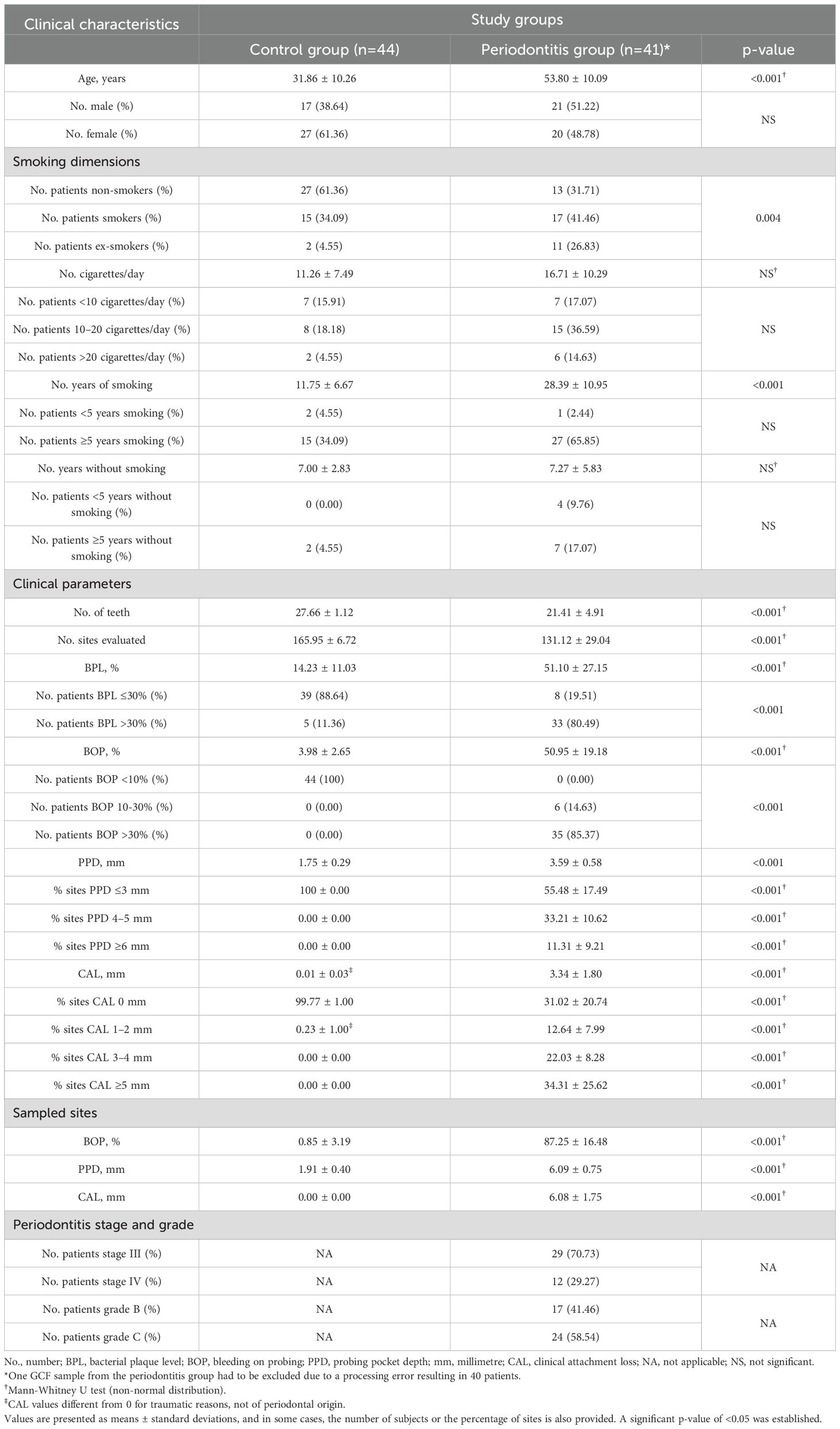
Table 1. Age, gender, smoking habit, and clinical characteristics of GCF and saliva sampled patients associated with periodontal status in the control group and periodontitis.
Proteins identified and quantified in GCF and saliva samples by SWATH-MS
The in silico spectral library contained 37985 transitions, 4556 peptides, and 427 proteins. Of these, 250 GCF and 377 salivary proteins were quantified by SWATH-MS using the previously defined criteria. Interestingly, 238 proteins were quantified in both oral fluids, representing 95.2% of the GCF proteins and 63.1% of those in saliva (Appendix S1).
Periodontal proteome structure in GCF and saliva
The periodontal proteome structure of the GCF and salivary proteins was evaluated using a PCA. The first two principal components or dimensions accounted for 37.3% of the total variance in GCF and 23.0% in saliva (Appendix S2).
The PCA plot of the GCF and saliva samples revealed distinct clustering between samples with good periodontal health and those with periodontitis. These findings were confirmed by ANOSIM and adonis2 tests, which showed significant differences in GCF and saliva between individuals with periodontitis and those with healthy periodontium (Figures 2, 3).
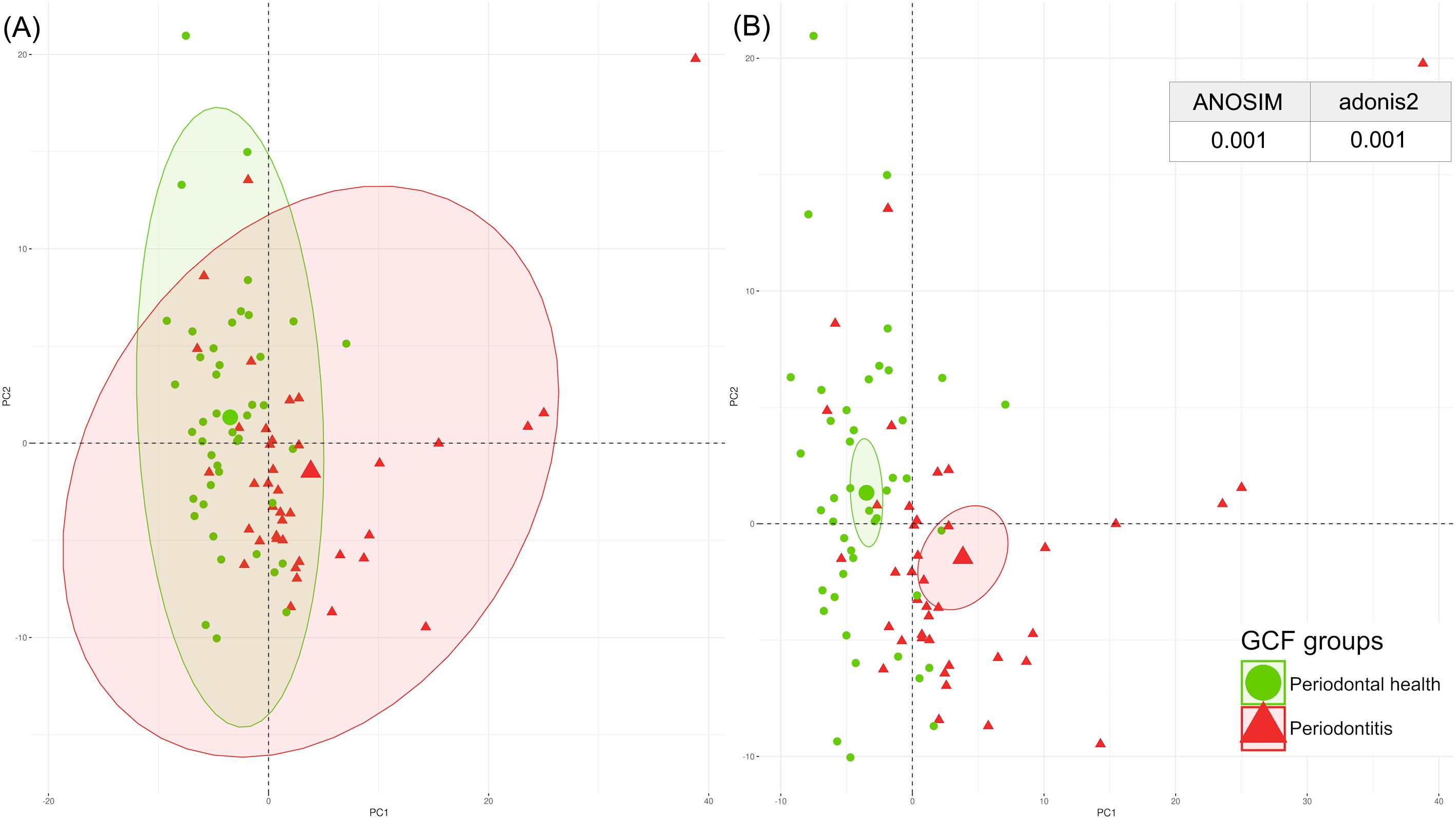
Figure 2. Spatial distribution of the GCF periodontal proteome by PCA, with p-values from ANOSIM and adonis2 tests categorised by periodontal health (green) and periodontitis (red). (A) Centroids of each clinical condition with their corresponding ranges of values; (B) Centroids of each clinical condition with their corresponding 95% confidence intervals. ANOSIM, analysis of similarities; GCF, gingival crevicular fluid; PCA, principal component analysis. A significant p-value of <0.05 was established.
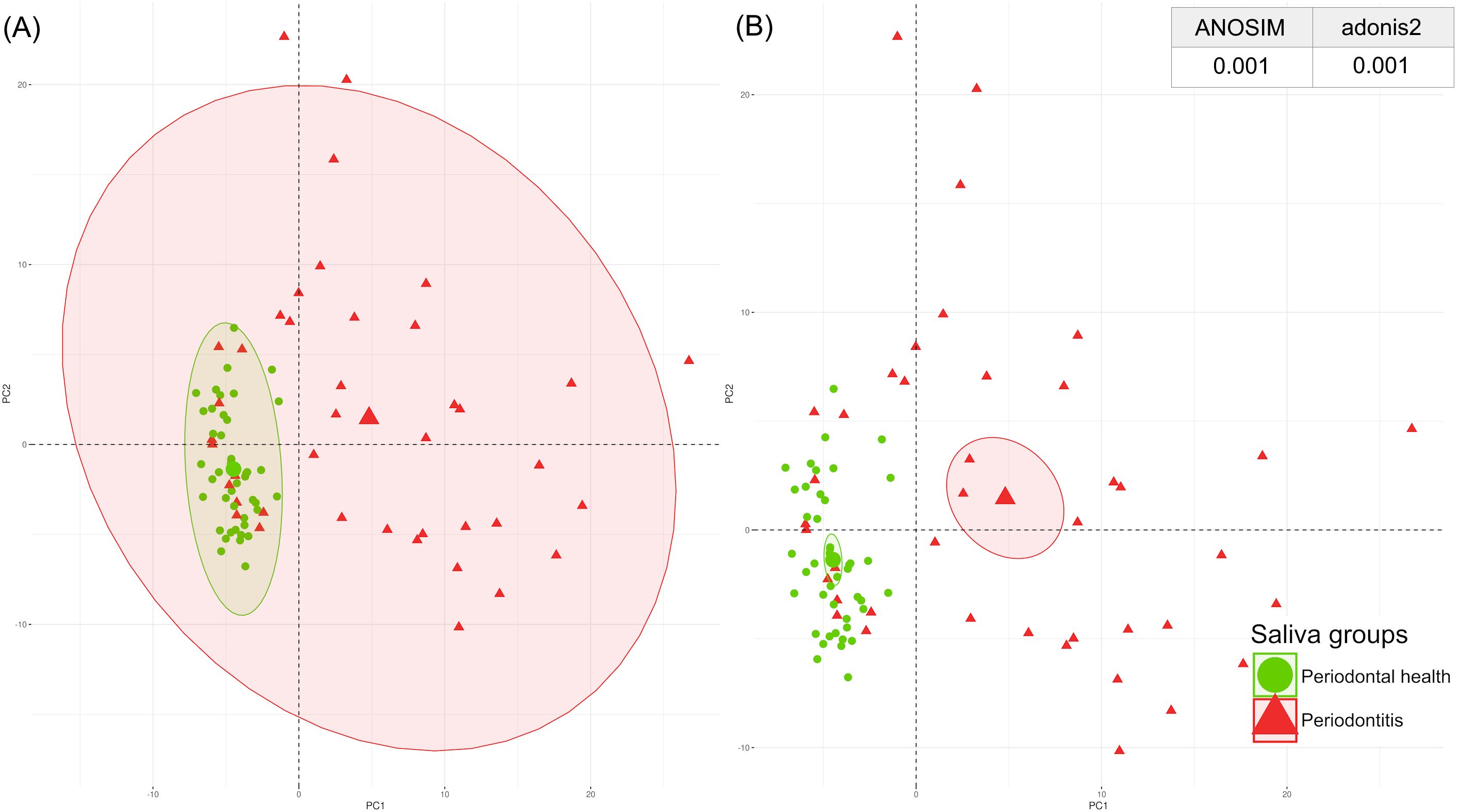
Figure 3. Spatial distribution of the saliva periodontal proteome by PCA with p-values from ANOSIM and adonis2 tests categorised by periodontal health (green) and periodontitis (red). (A) Centroids of each clinical condition with their corresponding ranges of values; (B) Centroids of each clinical condition with their corresponding 95% confidence intervals. ANOSIM, analysis of similarities; PCA, principal component analysis. A significant p-value of <0.05 was established.
Differential expression of the GCF proteins
Considering the expression of the 250 GCF proteins quantified and the previously established criteria, 63 (25.2%) were found to be differentially expressed in periodontitis compared to periodontal health. Of these, 38 (60.3%) were shown to be upregulated, and 25 (39.7%) were downregulated (Figure 4).
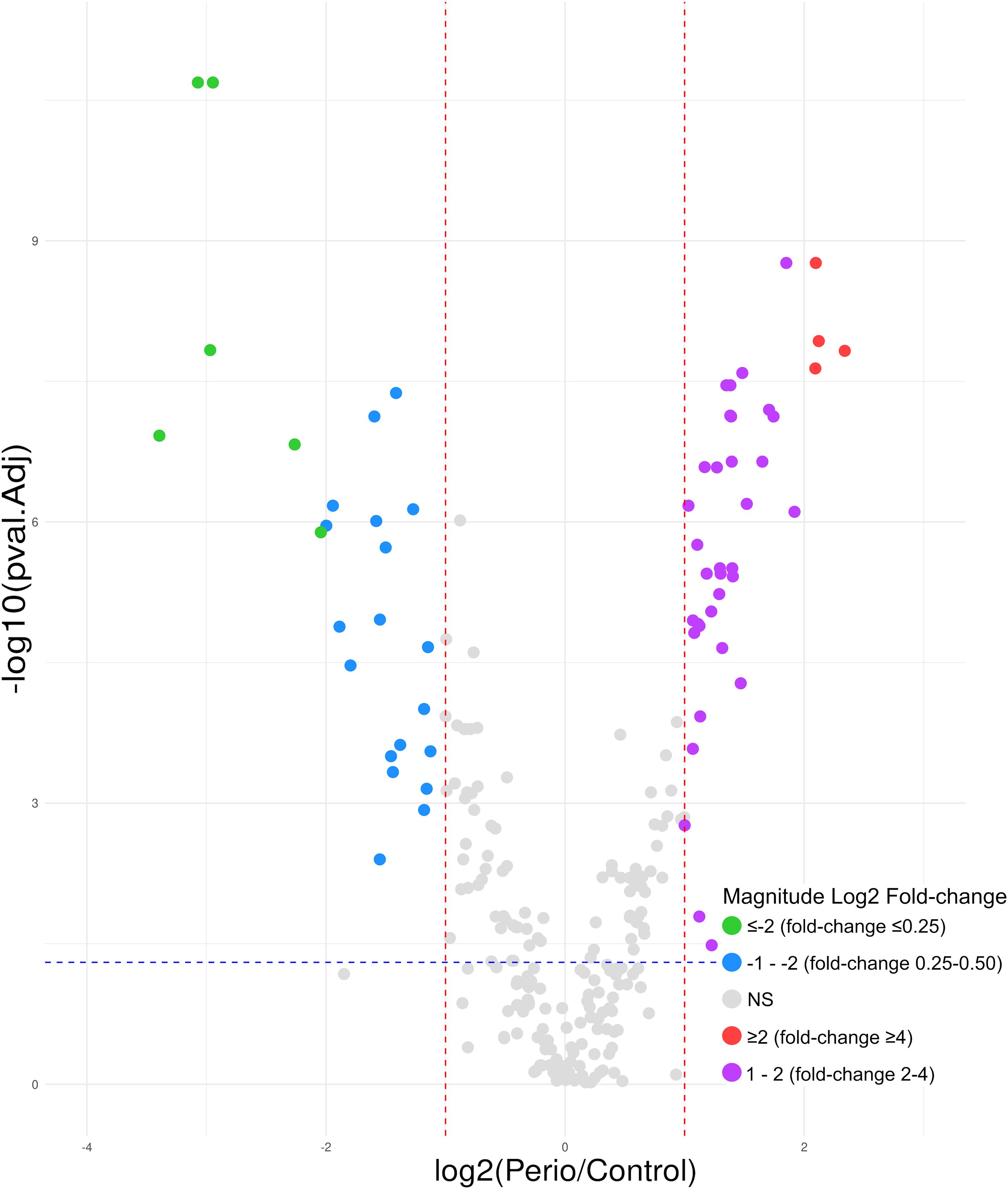
Figure 4. Volcano plot of the 63 GCF proteins differentially expressed in periodontitis compared to periodontal health; 38 upregulated (log2 fold-change ≥2 [red]= 4; 1 − 2 [purple]= 34) and 25 downregulated (log2 fold-change ≤-2 [green]= 6; -1 − -2 [blue]= 19). GCF, gingival crevicular fluid; log, logarithmic; NS, not significant. Differentially expressed proteins were considered if the adjusted p-value was <0.05 (−log10 p-value=1.30) (blue horizontal line) and a fold-change ≥2 (log2 fold-change ≥1) or ≤0.5 (log2 fold-change ≤-1) (red vertical lines). Magnitude Log2 fold-change: ≥2 (red)=fold-change ≥4; 1 − 2 (purple)=fold-change 2 − 4; ≤-2 (green)=fold-change ≤0.25; -1 − -2 (blue)=fold-change 0.25 − 0.50.
Among the 38 overexpressed proteins, four showed a ≥4-fold higher expression in periodontitis compared to periodontal health (log2 fold-change ≥2). Of these, haemoglobin subunits (Hb) beta and alpha showed the highest fold-change (5.06 and 4.35, respectively), followed by carbonic anhydrase 1 (4.28) and protein S100-P (4.27). The remaining 34 proteins showed at least 2-fold higher expression in periodontitis, highlighting immunoglobulins (Ig) heavy constant gamma 3 (3.78), kappa light chain (2.19), and heavy variable 5-51 (2.18), plasma protease C1 inhibitor (3.61), haptoglobin (3.35), and another Hb, delta (3.26) (Table 2).
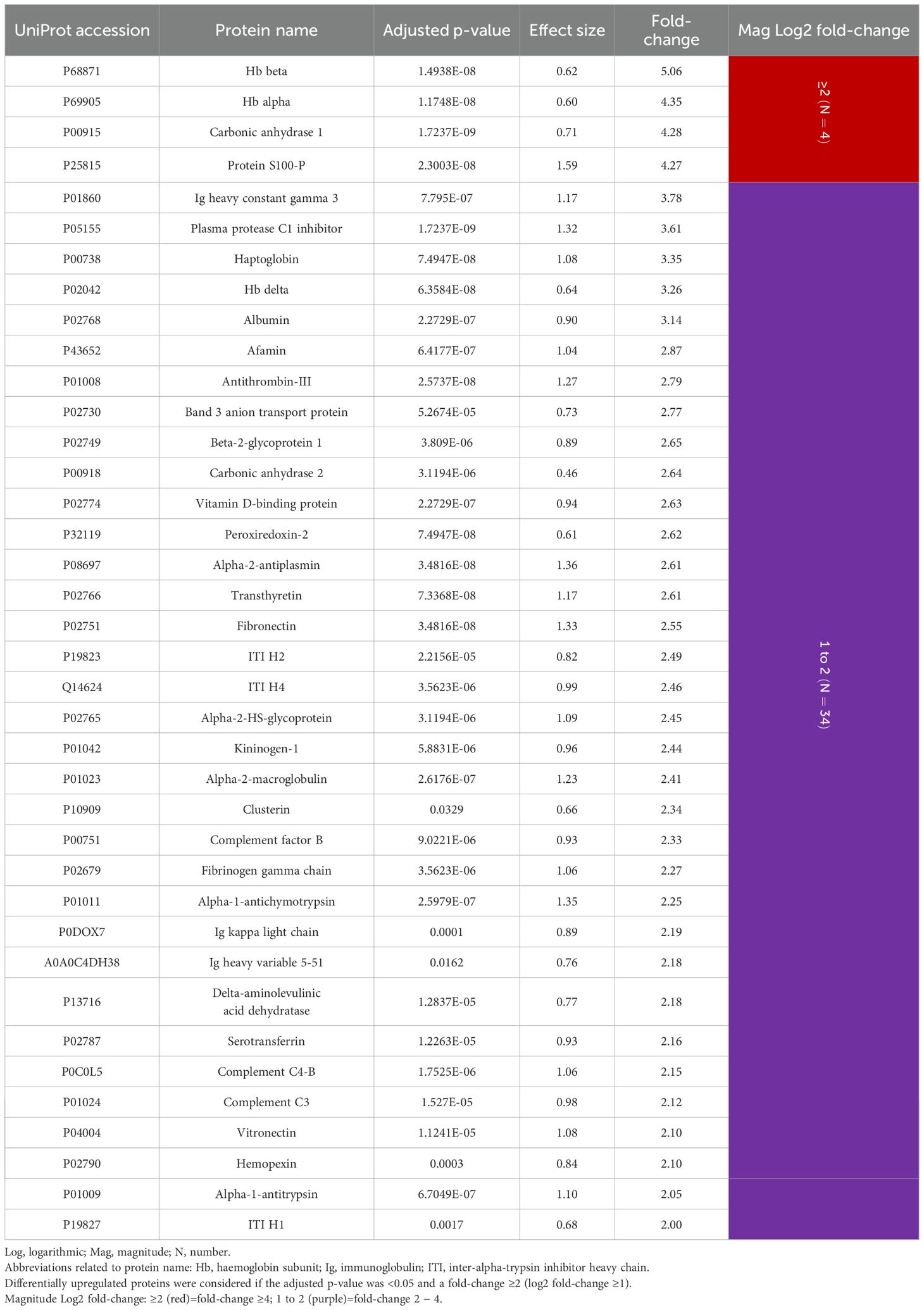
Table 2. GCF proteins differentially upregulated in periodontitis compared to periodontal health (N=38 proteins).
Interestingly, of the 25 downregulated proteins, 14 were keratins, accounting for 56.0%. Considering a fold-change ≤0.25 (log2 fold-change ≤-2), which means a ≤4-fold lower expression in periodontitis, four were keratins: type II cytoskeletal 6B (0.10), type II cytoskeletal 6A (0.13), type II cytoskeletal 5 (0.21), and type I cytoskeletal 16 (0.24). The remaining 11 downregulated keratins exhibited a fold-change of 0.25-0.44. Other protein types that showed a fold-change ≤0.25 were glyceraldehyde-3-phosphate dehydrogenase (GAPDH) (0.12) and zymogen granule protein 16 homolog B (ZG16B) (0.13) (Table 3).
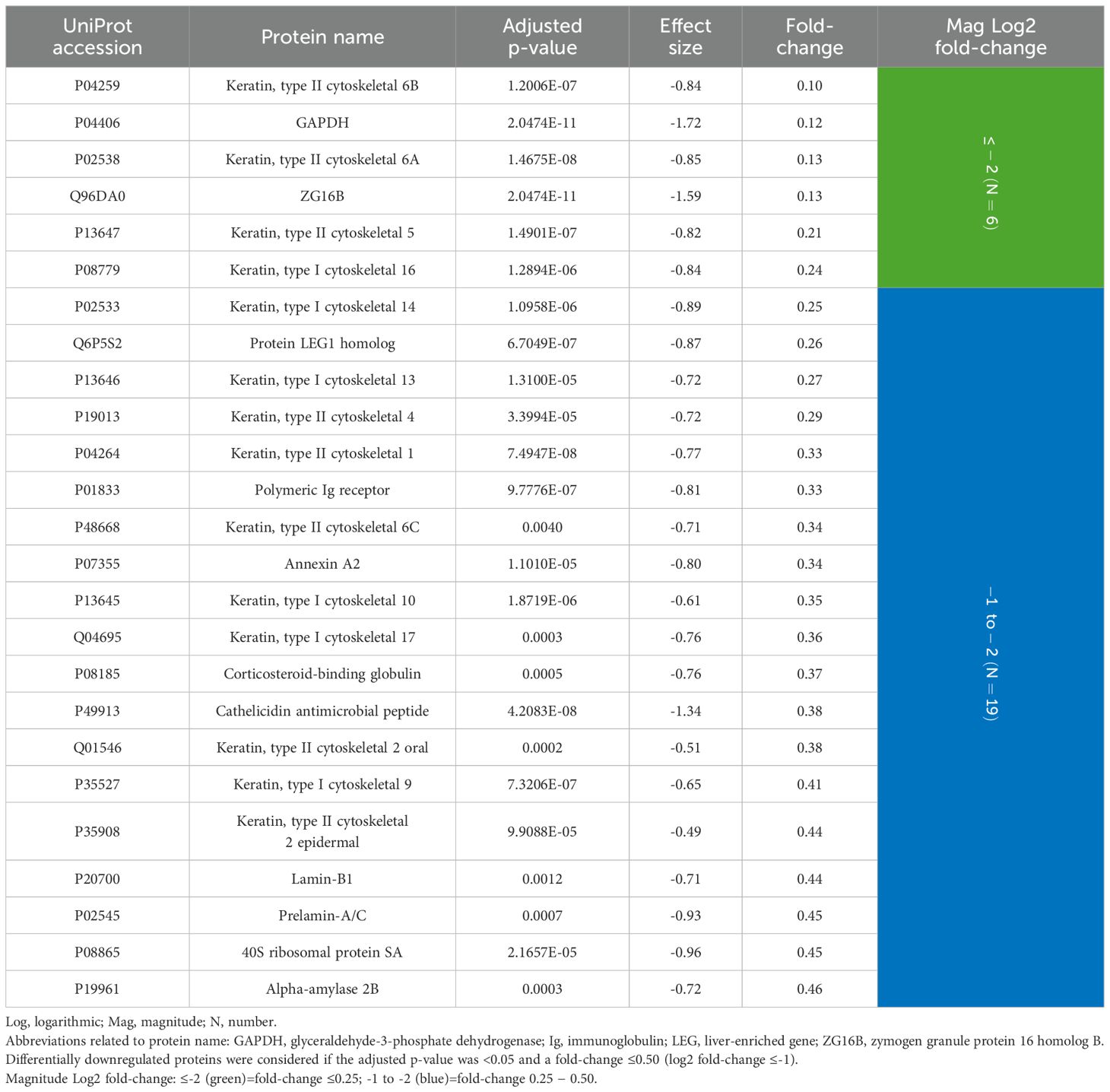
Table 3. GCF proteins differentially downregulated in periodontitis compared to periodontal health (N=25 proteins).
Differential expression of the salivary proteins
In saliva, of the 377 proteins quantified, 59 (15.7%) were differentially expressed, with 55 (93.2%) being upregulated and only four (6.8%) downregulated (Figure 5).
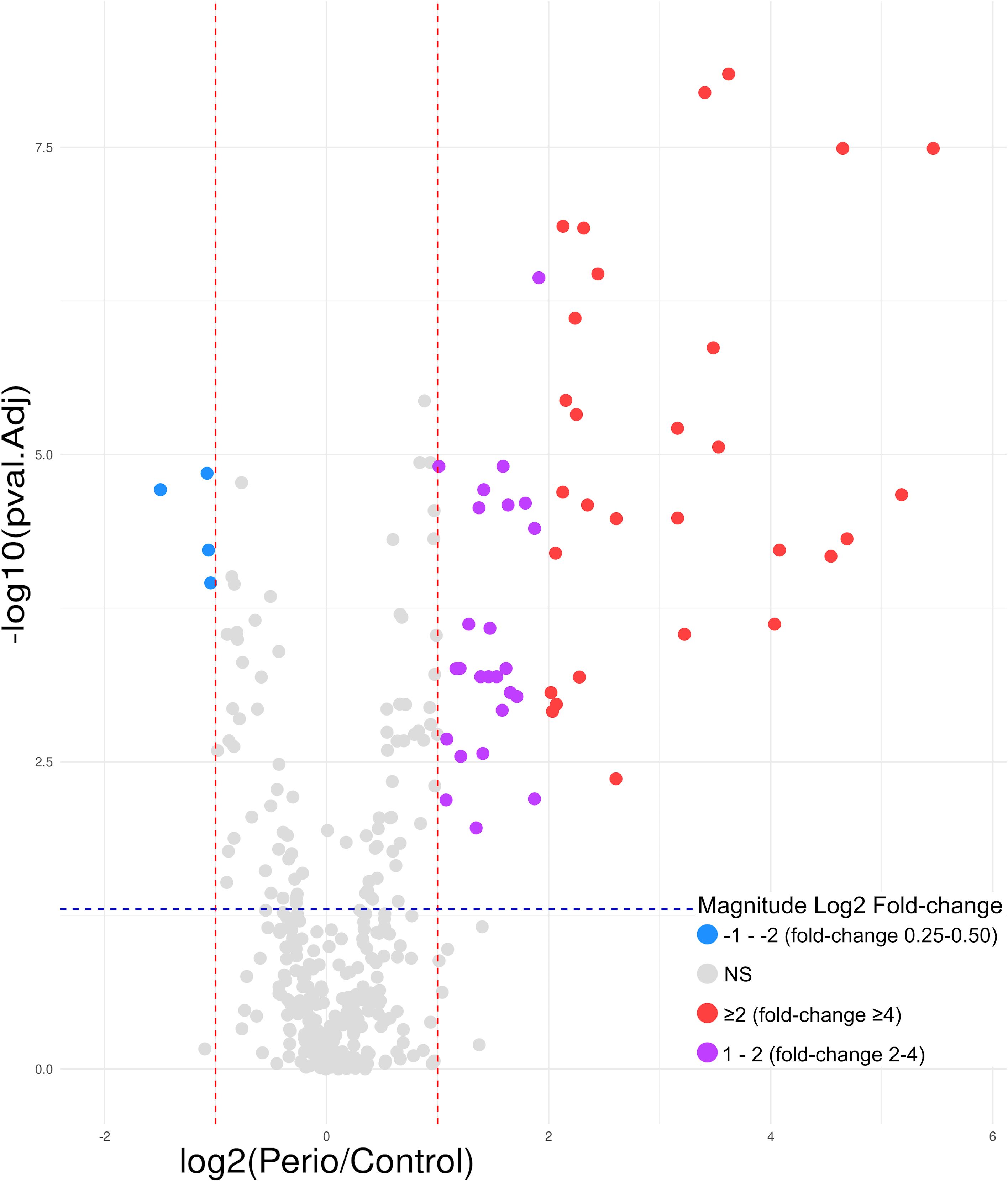
Figure 5. Volcano plot of the 59 saliva proteins differentially expressed in periodontitis compared to periodontal health; 55 upregulated (log2 fold-change ≥2 [red]= 29; 1 − 2 [purple]= 26) and 4 downregulated (log2 fold-change -1 − -2 [blue]). log, logarithmic; NS, not significant. Differentially expressed proteins were considered if the adjusted p-value was <0.05 (−log10 p-value=1.30) (blue horizontal line) and a fold-change ≥2 (log2 fold-change ≥1) or ≤0.5 (log2 fold-change ≤-1) (red vertical lines). Magnitude Log2 fold-change: ≥2 (red)=fold-change ≥4; 1 − 2 (purple)=fold-change 2 − 4; -1 − -2 (blue)=fold-change 0.25 − 0.50.
Consequently, the most differentially expressed proteins were upregulated, with beta-2-microglobulin exhibiting the greatest fold-change (44.14). Furthermore, in contrast to GCF, nine keratins were overexpressed in saliva in periodontitis, with seven exhibiting a fold-change of ≥ 4 and two between 2 and 4. Of these, keratin, type I cytoskeletal 13 showed the highest fold-change (36.23), and the remaining eight values ranged from 2.65 to 23.31. Other noteworthy proteins include the S100 family: A9 (12.30), A8 (10.61), A12 (4.76), P (4.72), and A6 (2.67), in addition to Ig heavy constant alpha 1 (4.45), alpha-2 heavy chain (2.59), and Hb beta (4.20) and alpha (4.06). Considering the remaining overexpressed proteins, of particular interest were neutrophil defensin 1 (25.08), annexin A1 (9.34), lysozyme C (4.98), resistin (4.37), cathepsin G (3.28), myeloperoxidase (2.77), and azurocidin (2.75) (Table 4).
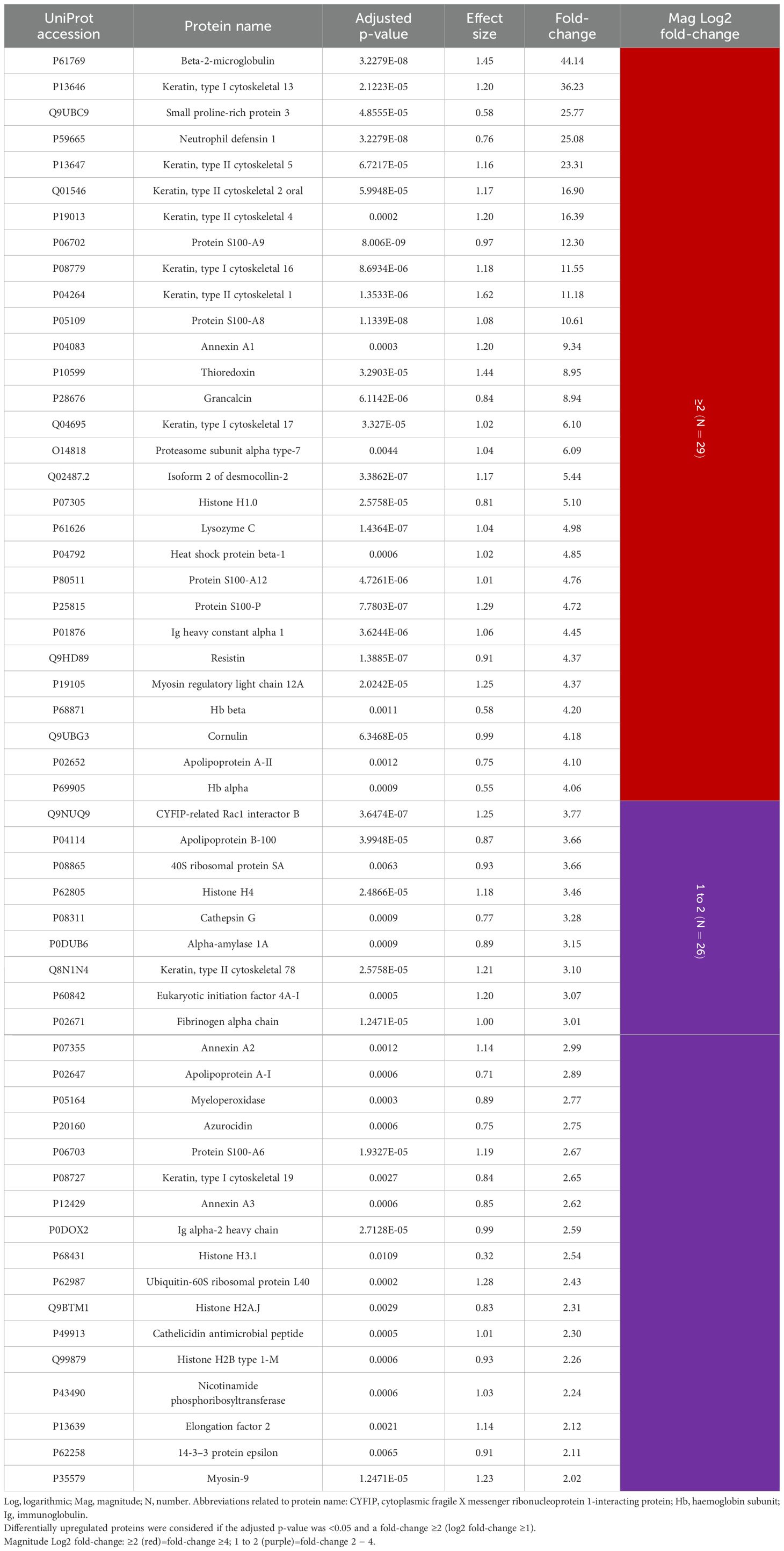
Table 4. Salivary proteins differentially upregulated in periodontitis compared to periodontal health (N=55 proteins).
The fold-change of the downregulated proteins was considerably lower, with no protein having a value of ≤0.25. The most underexpressed protein was lipocalin-1 with a fold-change of 0.35 (Table 5).
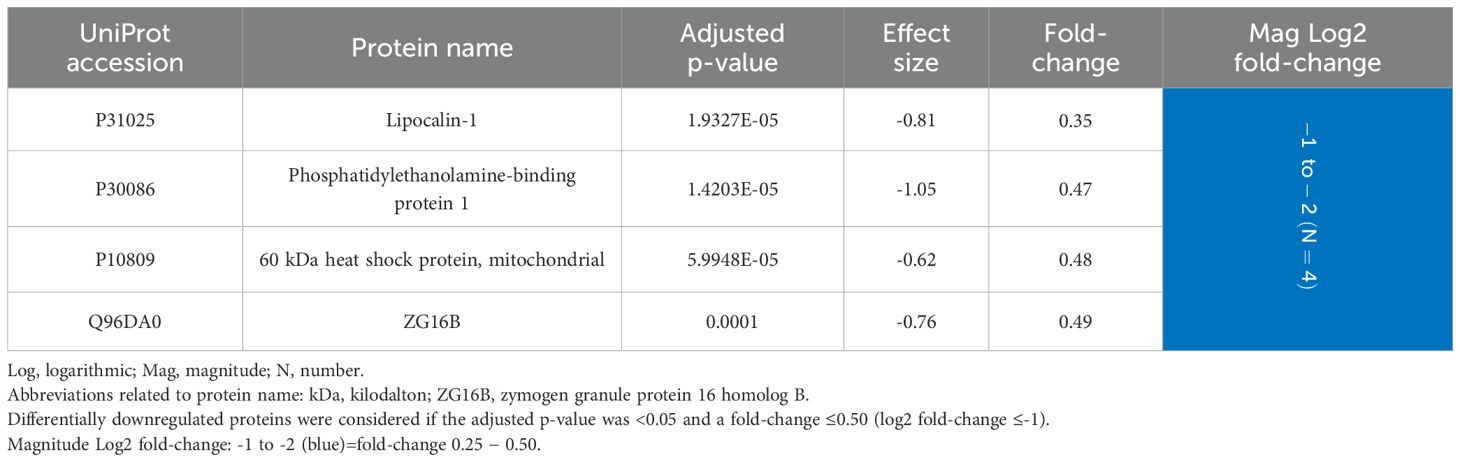
Table 5. Salivary proteins differentially downregulated in periodontitis compared to periodontal health (N=4 proteins).
Comparison of differentially expressed proteins in GCF and saliva
Comparing the results obtained in GCF and saliva, 14 proteins were differentially expressed in both sample types, representing 22.2% and 23.7% of the total proteins differentially expressed, respectively.
As previously noted, Hb beta and alpha were overexpressed in GCF and saliva (GCF: 5.06 and 4.35; saliva: 4.20 and 4.06, respectively), in addition to protein S100-P (4.27; 4.72). Furthermore, ZG16B was found to be downregulated in both sample types (0.13; 0.49).
However, the remaining 10 proteins were underexpressed in GCF but overexpressed in saliva. Interestingly, seven of these proteins were keratins (types I cytoskeletal 13, 16, and 17, and types II cytoskeletal 1, 2 oral, 4, and 5) with a fold-change in GCF of 0.21-0.38, whereas in saliva it was 6.10-36.23. The remaining three proteins were annexin A2 (0.34; 2.99), cathelicidin antimicrobial peptide (0.38; 2.30), and 40S ribosomal protein SA (0.45; 3.66) (Table 6).
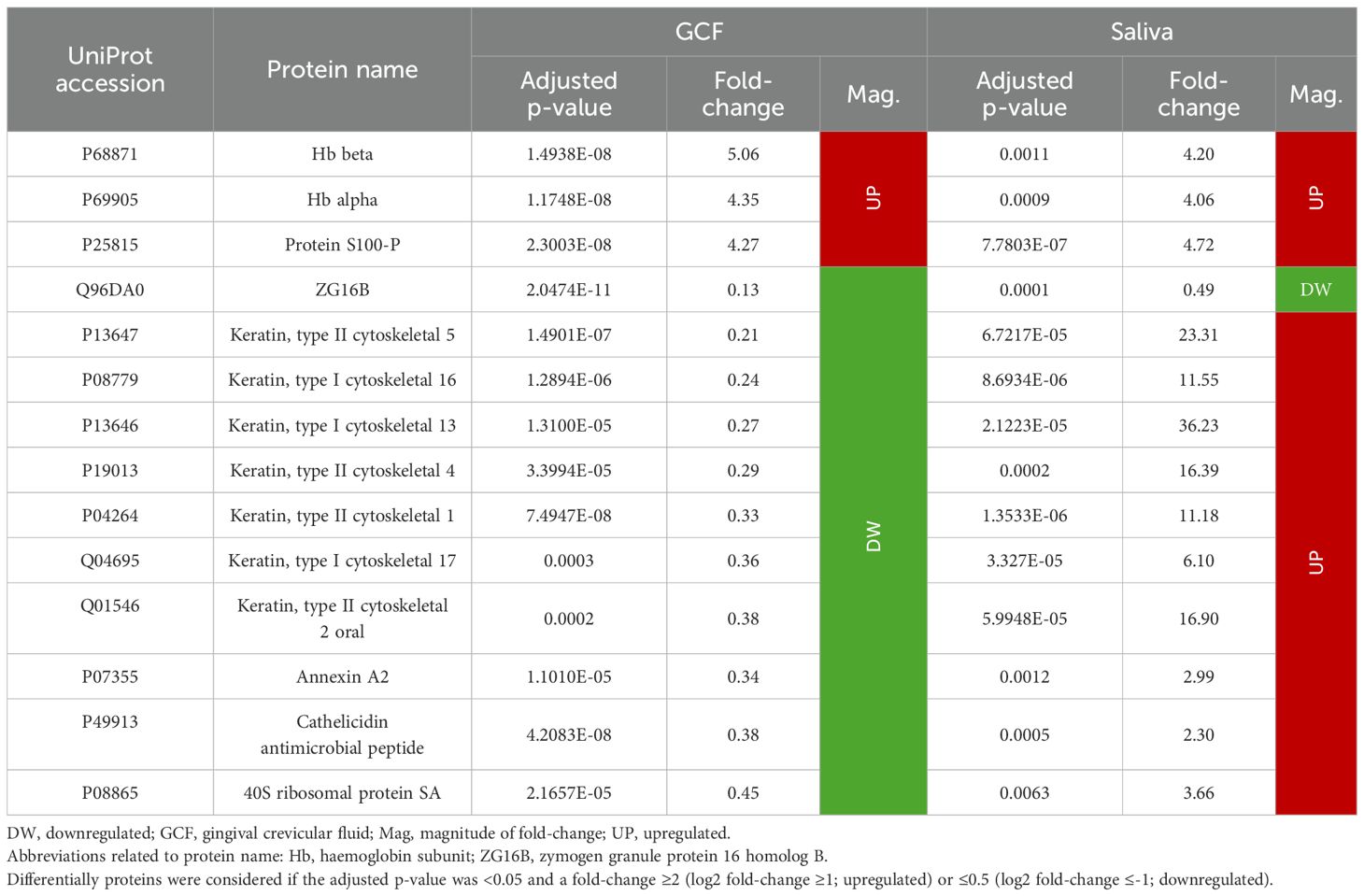
Table 6. Proteins differentially expressed in GCF and saliva in periodontitis compared to periodontal health (N=14 proteins).
Discussion
The analysis of GCF and saliva samples using SWATH-MS enabled the quantification of 250 abundant proteins in GCF and 377 in saliva. In both fluids, the structure of the periodontal proteome differed between individuals with periodontitis and those with a healthy periodontium. Regarding protein expression, approximately 25% of GCF proteins and 16% of salivary proteins were found to be differentially expressed. In both fluids, most of these proteins were overexpressed in periodontitis—60% vs. 40% in GCF and 93% vs. 7% in saliva, respectively. Furthermore, although 95% of GCF proteins and 63% of salivary proteins were quantified in both sample types, the most differentially expressed proteins varied, and only about 22% of them were shared between the two oral fluids.
Methodological differences in periodontal proteome analysis
To date, the periodontal proteome has been evaluated using different proteomic techniques (Rizal et al., 2020). However, this is the first study to use SWATH-MS to analyse the structure and differential protein expression of the periodontal proteome.
Sample size is a relevant limitation in previous proteomics research, with most studies including ≤20 subjects per clinical group (Bellei et al., 2022; Bostanci et al., 2018; Casarin et al., 2023; Grant et al., 2022; Guzman et al., 2018; Mertens et al., 2018; Tang et al., 2019; Yi et al., 2021). In contrast, the present study included a notably larger sample size, with more than 40 patients. Increasing the sample size is crucial for enhancing statistical robustness and improving the ability to detect true differences in protein expression levels between clinical conditions (Ahmad et al., 2025).
The periodontal proteome has been primarily studied in gingival crevicular fluid (GCF) and saliva samples (Guzman et al., 2014), as both oral fluids are valuable for investigating the oral and systemic environments (Ahmad et al., 2025). However, there is a tendency to justify the selection of one fluid over the other, since most studies have evaluated the periodontal proteome in either GCF or saliva (Bellei et al., 2022; Bostanci et al., 2018; Casarin et al., 2023; Hartenbach et al., 2020; Kim et al., 2021; Yi et al., 2021). Only two studies have analysed both sample types (Grant et al., 2022; Tang et al., 2019). These studies focused on assessing the diagnostic accuracy of proteins without comparing the structural and expression differences between the GCF and saliva proteomes.
Analysis of GCF and saliva samples using SWATH-MS yielded a spectral library of 427 proteins, with 250 and 377 proteins quantified in each fluid, respectively, based on stringent criteria that required at least 10 peptides per protein and seven fragments per peptide. Although this number of quantified proteins is lower compared to previous studies, those studies applied less stringent criteria, quantifying proteins based on as few as two unique peptides (Bostanci et al., 2018; Grant et al., 2022; Kim et al., 2021; Silbereisen et al., 2024; Yi et al., 2021).
Although we could have employed more extensive libraries or adopted a library-free DIA approach (Demichev et al., 2020; Rosenberger et al., 2014), building our own spectral library ensured accurate protein identification by focusing on the most abundant proteins. This approach lays the groundwork for further validation using less sensitive methods, which could ultimately support clinical applications.
Comparing GCF and saliva, saliva samples have a higher number of proteins (Grant et al., 2022; Tang et al., 2019). This is because saliva also comprises proteins from the salivary glands (Guzman et al., 2014). Moreover, 238 proteins were common to both oral fluids, representing 95% of those in GCF and 63% in saliva. This reflects that most GCF components are excreted from the pocket through saliva and can be identified in both fluids (Bostanci and Bao, 2017).
Grant et al. reported that about 30% of the total proteins were present in both fluids (Grant et al., 2022). This lower proportion may be attributed to the different proteomic techniques used (isobaric tag for relative and absolute quantitation -iTRAQ-) and the analysis of stimulated saliva samples (Grant et al., 2022). Conversely, we collected unstimulated saliva as the stimulation of salivary flow may produce inappropriate glycoprotein glycosylation (Buzalaf et al., 2020).
The criteria applied in previous publications to consider a protein as differentially expressed were based on a p-value <0.05, a minimum fold-change, or both (Bellei et al., 2022; Bostanci et al., 2018; Casarin et al., 2023; Hartenbach et al., 2020; Kim et al., 2021; Shin et al., 2019). However, our study was more demanding, being the first to apply the Benjamini-Hochberg correction for controlling false positives (Benjamini and Hochberg, 1995). This variability in the criteria applied, as well as the different proteomics techniques employed, complicates the comparison of the results obtained with other studies.
Using a more permissive statistical threshold may increase the number of differentially expressed proteins detected. However, in scenarios involving distinct clinical states—such as periodontal health and advanced periodontitis—it is more relevant to prioritise the most strongly differentially expressed proteins. Conversely, when comparing closely related conditions, such as periodontal health and gingivitis, applying a less stringent threshold may be necessary to detect subtle variations in protein expression.
Periodontal proteome structure in GCF and saliva
The periodontal proteome structure was different between periodontitis and periodontal health in both sample types, consistent with previous research (Shin et al., 2019; Yi et al., 2021). Our research group demonstrated that smoking status does not alter the distribution of the GCF periodontal proteome in periodontitis (Blanco-Pintos et al., 2025b). Therefore, periodontitis is a more relevant condition for modifying the structure of the periodontal proteome. However, future research should investigate the impact of other clinical variables, such as age, on the spatial distribution of the periodontal proteome.
Differential expression of GCF proteins
The functions and possible relationship to periodontitis of the most relevant GCF proteins differentially expressed are specified in Table 7.
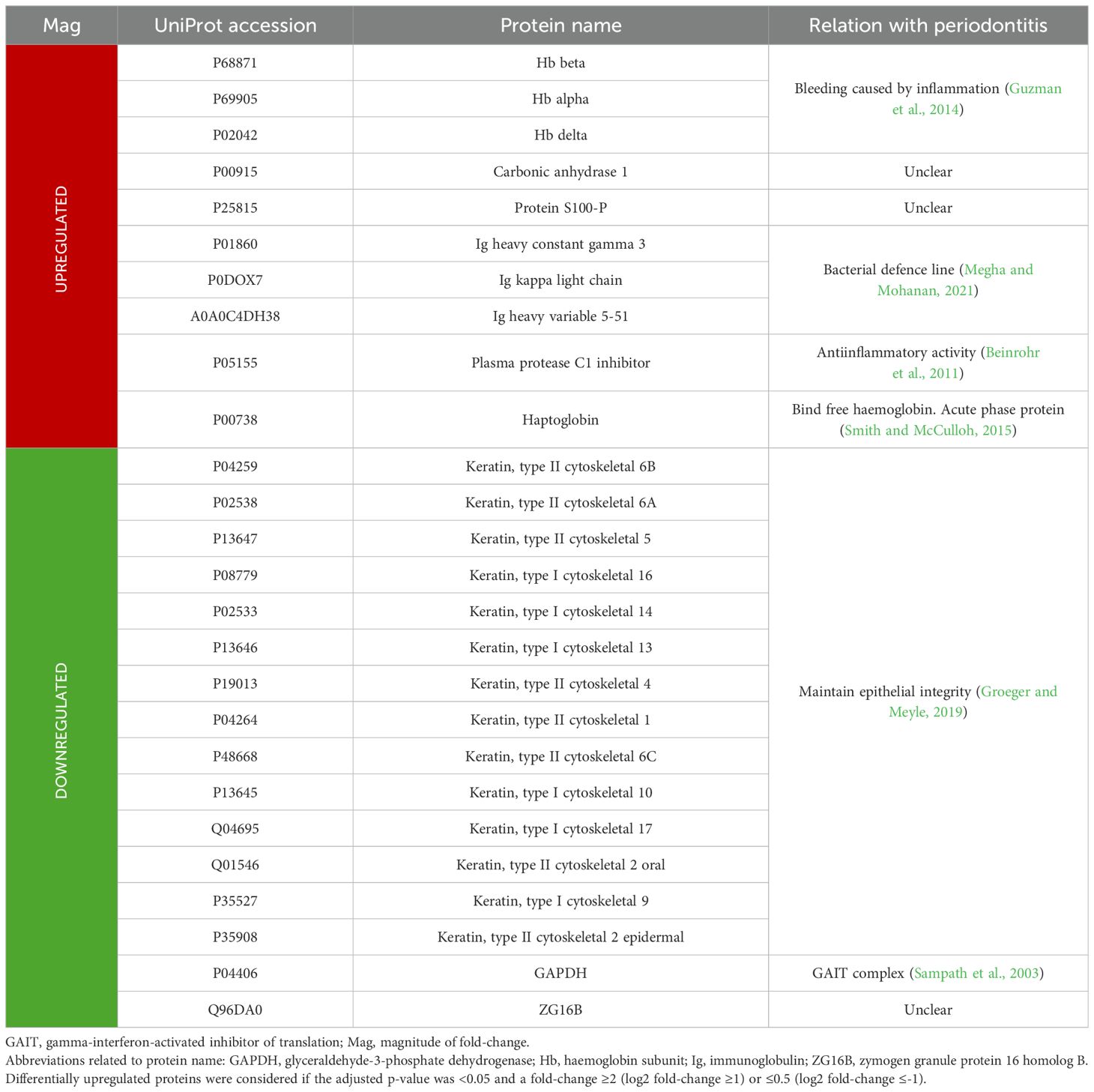
Table 7. Functions and relationship to periodontitis of the most relevant GCF proteins differentially expressed in periodontitis compared to periodontal health.
Hb beta and alpha were the most overexpressed proteins, with Hb delta also being up to five times increased in periodontitis. It is reported that, despite discarding highly visible blood-contaminated GCF samples, there is a greater tendency for spontaneous bleeding in periodontitis due to inflammation of periodontal tissues (Guzman et al., 2014).
Consequently, haptoglobin was also found to be upregulated (fold-change >3), because it binds to free haemoglobin. Furthermore, haptoglobin is an acute-phase protein, and its expression is increased in infectious and inflammatory processes (Smith and McCulloh, 2015). Previous proteomic studies have also demonstrated the overexpression of these proteins in periodontitis (Bellei et al., 2022; Carneiro et al., 2014; Tang et al., 2019).
Moreover, the Ig’s heavy constant gamma 3, kappa light chain, and heavy variable 5–51 were also shown to be upregulated (fold-change >2). Igs are essential components of the immune system for recognising and neutralising pathogens by binding to their antigens (Megha and Mohanan, 2021). Therefore, in periodontitis, their expression is increased due to bacterial dysbiosis and inflammation of periodontal tissues (Bellei et al., 2022; Carneiro et al., 2014; Marinho et al., 2019; Yi et al., 2021).
Considering the remaining upregulated proteins, the plasma protease C1 inhibitor (fold-change >3.5) is particularly noteworthy. This protein functions as an essential antiinflammatory protein by regulating the complement system (Beinrohr et al., 2011). However, carbonic anhydrase 1 and protein S100-P, they were overexpressed in periodontitis (fold-change around 4.3 for both), as in previous studies (Baliban et al., 2012; Silva-Boghossian et al., 2013); however, their relationship with periodontitis is still unclear.
Conversely, the most differentially expressed proteins were downregulated, as keratin, type II cytoskeletal 6B showed a fold-change of 0.10, implying a 10-fold lower expression in periodontitis compared to periodontal health. Moreover, the majority (56%) of the 25 proteins underexpressed in periodontitis were different types of keratins. Keratins are essential structural proteins in the epithelial barrier, providing resistance to microbial invasion (Groeger and Meyle, 2019). The decrease in keratins in GCF periodontitis samples may be related to periodontal tissue degradation, compromising the integrity of the epithelium and making the tissues more vulnerable to bacterial invasion (Groeger and Meyle, 2019).
Apart from keratins, the most underexpressed proteins include GAPDH and ZG16B with also a fold-change of around 0.10. GAPDH is a key enzyme in glycolysis (Tisdale, 2002), but it is also part of the gamma interferon-activated inhibitor of translation (GAIT) complex. This complex may contribute to the resolution of the inflammatory response following cytokine activation (Sampath et al., 2003). Therefore, its underexpression may be associated with reduced antiinflammatory activity of the GAIT complex. However, the function of ZG16B and its potential relationship with periodontitis remain unclear.
Differential expression of the salivary proteins
In saliva, the most differentially expressed protein was beta-2-microglobulin, which was approximately 44 times more abundant in patients with periodontitis than in healthy subjects. This protein is a component of major histocompatibility complex (MHC) class I molecules and is released during inflammatory processes due to its crucial role in the immune system and antigen presentation (Bernier, 1980; Sreejit et al., 2014) (Table 8).
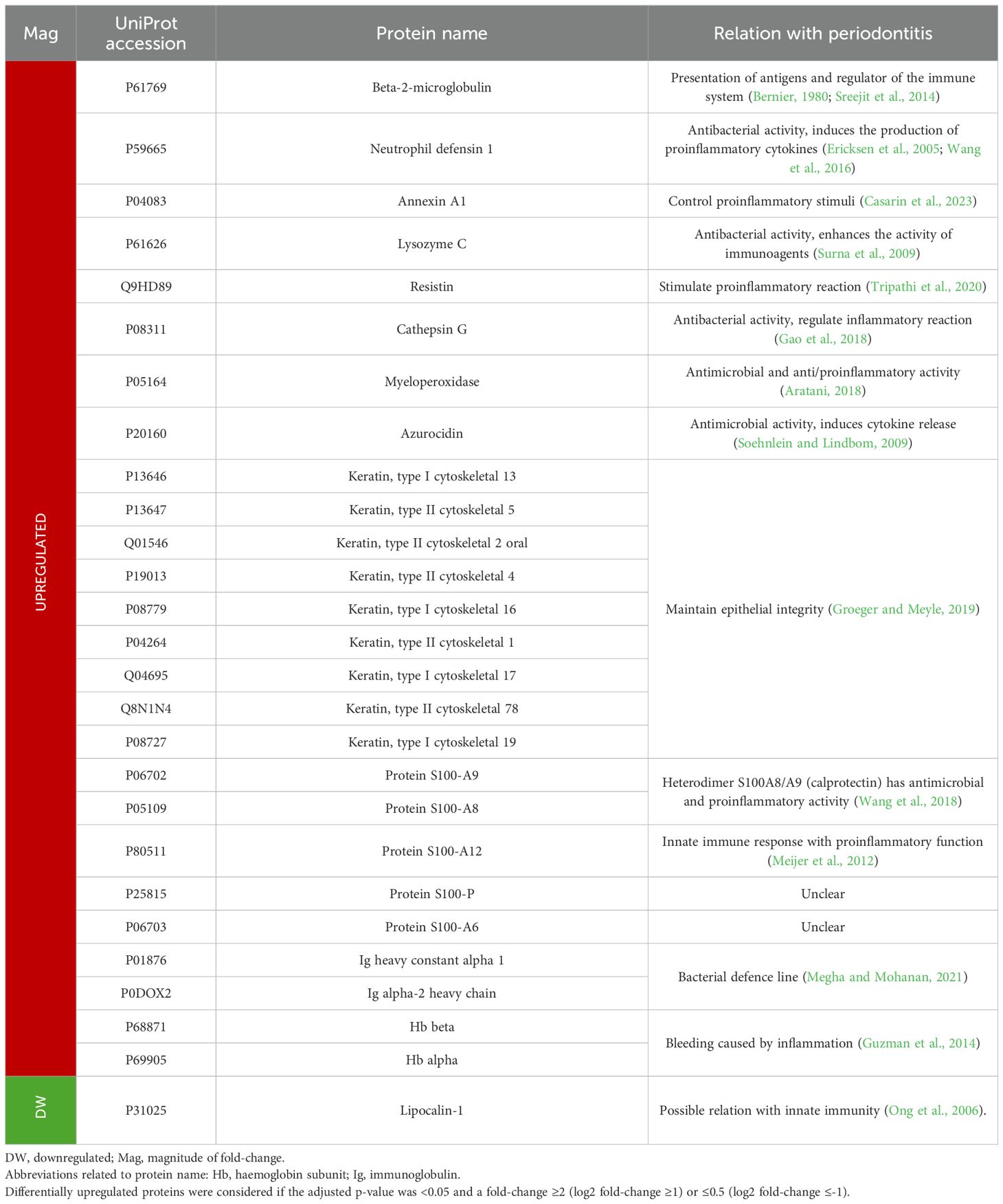
Table 8. Functions and relationship to periodontitis of the most relevant salivary proteins differentially expressed in periodontitis compared to periodontal health.
Other proteins that stand out are neutrophil defensin 1, annexin A1, lysozyme C, resistin, cathepsin G, myeloperoxidase, and azurocidin, which were upregulated between 2.8 and 25.1-fold in periodontitis. These proteins participate in the immune system by having proinflammatory (promoting cytokine secretion) and/or antiinflammatory functions (Aratani, 2018; Casarin et al., 2023; Gao et al., 2018; Soehnlein and Lindbom, 2009; Surna et al., 2009; Tripathi et al., 2020; Wang et al., 2016). Additionally, some of them have also demonstrated antimicrobial activity (Ericksen et al., 2005) (Table 8). Thus, overexpression of these proteins can indicate the dysregulation of the host immune response in periodontitis.
Similarly, other proteins with proinflammatory and antimicrobial functions that were overexpressed were those belonging to the S100 family, A8, A9, and A12, with a fold-change >4.5 (Meijer et al., 2012; Wang et al., 2018). Moreover, S100-P and S100-A6 were also found to be upregulated, as in previous studies (Grant et al., 2022; Salazar et al., 2013), although their possible relationship with periodontitis has not yet been discovered.
Furthermore, as in GCF, two Igs (heavy constant alpha 1 and alpha-2 heavy chain), as well as Hb beta and alpha, were overexpressed in periodontitis. However, keratins showed a different expression pattern, as nine keratins were found to be upregulated in saliva.
Conversely, only four salivary proteins were found to be downregulated in periodontitis. Moreover, the difference in expression with respect to healthy subjects was lower than that shown by the overexpressed proteins. Lipocalin-1 was the most underexpressed saliva protein with a nearly three times lower expression in periodontitis. This protein is involved in taste perception but also has a possible relationship with innate immunity (Ong et al., 2006).
Periodontal proteome expression in GCF and saliva: similarities and differences
As mentioned above, the protein composition of GCF and saliva samples was similar, as 95% of abundant GCF proteins and 63% of abundant salivary proteins were quantified in both oral fluids. However, the proportion of differentially expressed proteins was higher in GCF than in saliva (25% vs. 16%).
Furthermore, although more upregulated than downregulated proteins were found in both sample types in periodontitis, the distribution was more balanced in GCF (60% vs. 40%), whereas saliva showed a strong predominance of overexpressed proteins (93% vs. 7%). Additionally, the most differentially expressed proteins in GCF were underexpressed in periodontitis, with expression levels up to 10-fold lower. In contrast, in saliva, 11 proteins showed a 10-fold or greater increase in expression in periodontitis, with the highest difference reaching a 44-fold increase.
When comparing the differentially expressed proteins in both sample types, keratins are particularly noteworthy, as previously mentioned. Of the 14 proteins differentially expressed in both oral fluids, seven were keratins that were underexpressed in GCF but overexpressed in saliva (Table 9). This contrasting pattern may result from keratin exfoliation from the periodontal pocket due to epithelial barrier breakdown, leading to increased concentrations in saliva (Bostanci and Bao, 2017).
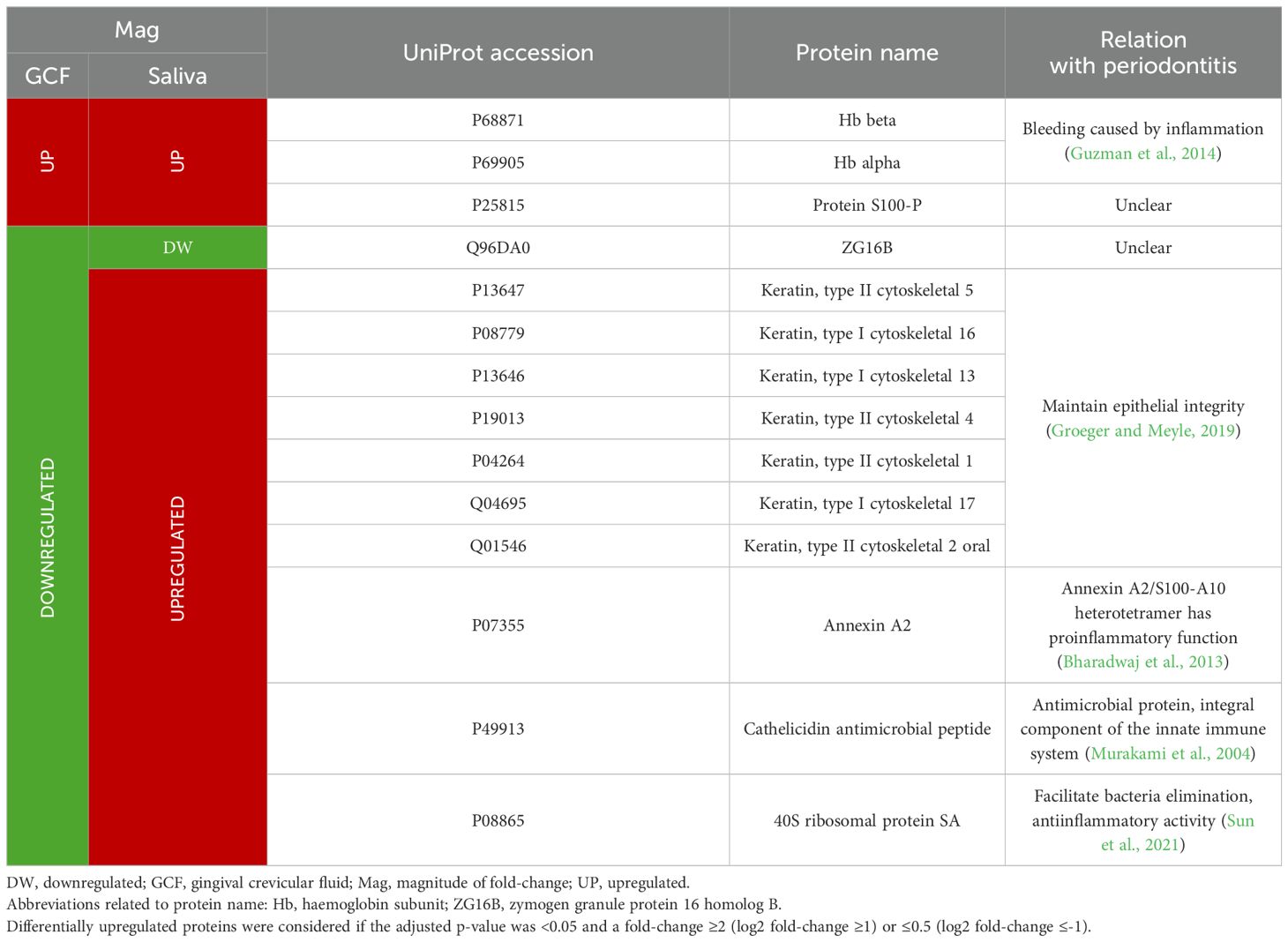
Table 9. Functions and relationship to periodontitis of the GCF and salivary proteins differentially expressed in periodontitis compared to periodontal health in both oral fluids.
A similar opposing expression pattern between GCF and saliva was observed for annexin A2, cathelicidin antimicrobial peptide, and 40S ribosomal protein SA. These proteins play roles in the immune response, exhibiting antiinflammatory, proinflammatory, and antimicrobial activities (Bharadwaj et al., 2013; Murakami et al., 2004; Sun et al., 2021).
Implications for practice and research perspectives
To better understand the periodontal proteome in GCF and saliva across periodontal health and periodontitis, we included a heterogeneous population with respect to age and smoking status. Proteomic analyses have shown that both factors influence protein expression (Blanco-Pintos et al., 2025b; Zhang et al., 2022). This diversity enhances the generalisability of the findings by helping to isolate the specific impact of periodontitis on the proteome. However, the influence of clinical variables on the periodontal proteome in GCF and saliva should be further investigated. This would allow for a more accurate evaluation and comparison of their effects on the proteome in periodontitis across both oral fluids.
Additionally, we analysed two distinct clinical conditions: periodontal health and advanced periodontitis (stages III–IV). Future research should also investigate the periodontal proteome in earlier disease stages, such as initial periodontitis (stages I–II) and gingivitis, in both GCF and saliva. This would help clarify how the proteome is progressively altered from health to disease, and how these molecular changes are reflected in both oral fluids.
From a clinical perspective, differentially expressed proteins cannot be considered biomarkers without diagnostic accuracy analyses (Gürsoy and Kantarci, 2022), such as those previously published by our research group (Blanco-Pintos et al., 2024, 2025a). Consistently, nearly all proteins demonstrating excellent diagnostic accuracy for detecting periodontitis were differentially expressed in both GCF and saliva. This includes proteins such as ZG16B and carbonic anhydrase 1, whose relationship with periodontitis remains unclear. As a result, functional studies aimed at elucidating the role of these proteins in the pathogenesis of periodontitis are of particular interest.
Moreover, the proteins with the highest diagnostic capacity differed between GCF and saliva, just as the most differentially expressed proteins varied between these fluids. This study is the first to demonstrate that while similar proteins are quantified in both GCF and saliva, their expression patterns differ qualitatively and quantitatively, underscoring the potential need for different molecular biomarkers in each oral fluid.
In previous studies from our research group, the diagnostic accuracy of GCF proteins was found to be higher than that of salivary proteins (Blanco-Pintos et al., 2024, 2025a). This difference may be attributed to the higher protein composition in saliva, which leads to greater inter-subject variability (Guzman et al., 2014). Clinically, both oral fluids are applicable, as while GCF biomarkers are probably more relevant in clinical practice alongside traditional diagnostic parameters, saliva, in addition to the clinical setting, is more suitable for large-scale population screenings and self-assessment (Guzman et al., 2014).
However, these results require validation in future studies. Commonly used validation techniques, such as enzyme-linked immunosorbent assay (ELISA), Western blot, and protein arrays, have certain limitations, including higher detection limits for some proteins compared to proteomic approaches, the requirement for highly specific antibodies, and an inability to provide a comprehensive analysis of the proteome (Jayasena et al., 2016). Therefore, it may be more appropriate to use SWATH-MS in an independent patient cohort or to employ other proteomic techniques such as SRM (Bostanci et al., 2018).
In conclusion, the periodontal proteome structure in GCF and saliva differs between individuals with periodontitis and those with periodontal health. Differential expression analysis reveals that a considerable proportion of abundant proteins in both sample types are altered in periodontitis, being higher in GCF. Moreover, the most differentially expressed proteins vary between these oral fluids both qualitatively and quantitatively. Therefore, although periodontitis induces changes in the periodontal proteome in GCF and saliva, the expression patterns differ between these fluids.
Data availability statement
The datasets presented in this study can be found in online repositories. The names of the repository/repositories and accession number(s) can be found below: https://www.ebi.ac.uk/pride/archive/, PXD043474 (GCF samples) and PXD043491 (saliva samples).
Ethics statement
The studies involving humans were approved by Galician Clinical Research Ethics Committee (registration numbers 2018/295 and 2021/417) Instituto Superior de Ciências da Saúde-Norte, CESPU (registration number 35/CEIUCS/2019). The studies were conducted in accordance with the local legislation and institutional requirements. The participants provided their written informed consent to participate in this study.
Author contributions
BT: Conceptualization, Investigation, Methodology, Visualization, Writing – original draft. RA: Investigation, Methodology, Writing – review & editing. SB: Investigation, Visualization, Writing – original draft. SN: Investigation, Visualization, Writing – original draft. RM: Investigation, Methodology, Resources, Writing – review & editing. BS: Data curation, Formal analysis, Methodology, Writing – review & editing. BC: Data curation, Methodology, Software, Writing – review & editing. TI: Conceptualization, Funding acquisition, Project administration, Resources, Supervision, Validation, Writing – review & editing.
Funding
The author(s) declare that financial support was received for the research and/or publication of this article. This study has been funded by the Instituto de Salud Carlos III (ISCIII) through the project PI21/00588 and co-funded by the European Union (EU).
Conflict of interest
The authors declare that the research was conducted in the absence of any commercial or financial relationships that could be construed as a potential conflict of interest.
Generative AI statement
The author(s) declare that no Generative AI was used in the creation of this manuscript.
Publisher’s note
All claims expressed in this article are solely those of the authors and do not necessarily represent those of their affiliated organizations, or those of the publisher, the editors and the reviewers. Any product that may be evaluated in this article, or claim that may be made by its manufacturer, is not guaranteed or endorsed by the publisher.
Supplementary material
The Supplementary Material for this article can be found online at: https://www.frontiersin.org/articles/10.3389/fcimb.2025.1576906/full#supplementary-material
References
Aggarwal, S., Yadav, A. K. (2016). False discovery rate estimation in proteomics. Methods Mol. Biol. 1362, 119–128. doi: 10.1007/978-1-4939-3106-4_7
Agra-Bermejo, R. M., Cacho-Antonio, C., Rozados-Luis, A., Couselo-Seijas, M., Fernandez, A. L., Martinez-Cereijo, J. M., et al. (2020). CD5L, macrophage apoptosis inhibitor, was identified in epicardial fat-secretome and regulated by isoproterenol from patients with heart failure. Front. Physiol. 11. doi: 10.3389/fphys.2020.00620
Ahmad, P., Escalante-Herrera, A., Marin, L. M., Siqueira, W. L. (2025). Progression from healthy periodontium to gingivitis and periodontitis: Insights from bioinformatics-driven proteomics - A systematic review with meta-analysis. J. Periodontal. Res. 60, 8–29. doi: 10.1111/jre.13313
Álvarez, V. J., Bravo, S. B., Chantada-Vazquez, M. P., Colón, C., De Castro, M. J., Morales, M., et al. (2020). Characterization of new proteomic biomarker candidates in mucopolysaccharidosis Type IVA. Int. J. Mol. Sci. 22, 226. doi: 10.3390/ijms22010226
Anderson, M. J. (2001). A new method for non-parametric multivariate analysis of variance. Austral. Ecol. 26, 32–46. doi: 10.1111/j.1442-9993.2001.01070.pp.x
Anjo, S. I., Santa, C., Manadas, B. (2017). SWATH-MS as a tool for biomarker discovery: From basic research to clinical applications. Proteomics 17, 1600278–n/a. doi: 10.1002/pmic.201600278
Aratani, Y. (2018). Myeloperoxidase: Its role for host defense, inflammation, and neutrophil function. Arch. Biochem. Biophys. 640, 47–52. doi: 10.1016/j.abb.2018.01.004
Baliban, R. C., Sakellari, D., Li, Z., DiMaggio, P. A., Garcia, B. A., Floudas, C. A. (2012). Novel protein identification methods for biomarker discovery via a proteomic analysis of periodontally healthy and diseased gingival crevicular fluid samples. J. Clin. Periodontol. 39, 203–212. doi: 10.1111/j.1600-051X.2011.01805.x
Beinrohr, L., Murray-Rust, T. A., Dyksterhuis, L., Závodszky, P., Gál, P., Pike, R. N., et al. (2011). Serpins and the complement system. Methods Enzymol. 499, 55–75. doi: 10.1016/B978-0-12-386471-0.00004-3
Bellei, E., Bertoldi, C., Monari, E., Bergamini, S. (2022). Proteomics disclose the potential of gingival crevicular fluid (GCF) as a source of biomarkers for severe periodontitis. Materials 15, 2161. doi: 10.3390/ma15062161
Benjamini, Y., Hochberg, Y. (1995). Controlling the false discovery rate: A practical and powerful approach to multiple testing. J. R. Stat. Soc B. Stat. Methodol. 57, 289–300. doi: 10.1111/j.2517-6161.1995.tb02031.x
Bernier, G. M. (1980). β2-microglobulin: Structure, function and significance. Vox. Sang. 38, 323–327. doi: 10.1111/j.1423-0410.1980.tb04500.x
Bharadwaj, A., Bydoun, M., Holloway, R., Waisman, D. (2013). Annexin A2 heterotetramer: structure and function. Int. J. Mol. Sci. 14, 6259–6305. doi: 10.3390/ijms14036259
Blanco-Pintos, T., Regueira-Iglesias, A., Kuz, I., Sánchez-Barco, A., Seijas-Otero, N., Chantada-Vázquez, M. P., et al. (2025b). Impact of smoking habit on the subgingival proteome in patients with periodontitis. J. Periodontol. 96, 217–229. doi: 10.1002/JPER.24-0062
Blanco-Pintos, T., Regueira-Iglesias, A., Relvas, M., Alonso-Sampedro, M., Bravo, S. B., Balsa-Castro, C., et al. (2025a). Diagnostic accuracy of novel protein biomarkers in saliva to detect periodontitis using untargeted ‘SWATH’ mass spectrometry. J. Clin. Periodontol. 52, 199–214. doi: 10.1111/jcpe.14103
Blanco-Pintos, T., Regueira-Iglesias, A., Relvas, M., Alonso-Sampedro, M., Chantada-Vázquez, M. P., Balsa-Castro, C., et al. (2024). Using SWATH-MS to identify new molecular biomarkers in gingival crevicular fluid for detecting periodontitis and its response to treatment. J. Clin. Periodontol. 51, 1342–1358. doi: 10.1111/jcpe.14037
Bonzon-Kulichenko, E., Pérez-Hernández, D., Núñez, E., Martínez-Acedo, P., Navarro, P., Trevisan-Herraz, M., et al. (2011). A robust method for quantitative high-throughput analysis of proteomes by 18O labeling. Mol. Cell. Proteomics 10, M110.003335. doi: 10.1074/mcp.M110.003335
Bostanci, N., Bao, K. (2017). Contribution of proteomics to our understanding of periodontal inflammation. Proteomics 17, 1500518–n/a. doi: 10.1002/pmic.201500518
Bostanci, N., Belibasakis, G. N. (2018). Gingival crevicular fluid and its immune mediators in the proteomic era. Periodontol. 2000. 76, 68–84. doi: 10.1111/prd.12154
Bostanci, N., Selevsek, N., Wolski, W., Grossmann, J., Bao, K., Wahlander, A., et al. (2018). Targeted proteomics guided by label-free quantitative proteome analysis in saliva reveal transition signatures from health to periodontal disease. Mol. Cell. Proteomics. 17, 1392–1409. doi: 10.1074/mcp.RA118.000718
Bugallo-Casal, A., Muiño, E., Bravo, S. B., Hervella, P., Arias-Rivas, S., Rodríguez-Yáñez, M., et al. (2025). NOTCH3 variant position affects the phenotype at the pluripotent stem cell level in CADASIL. Neuromol. Med. 27, 18. doi: 10.1007/s12017-025-08840-6
Buzalaf, M. A. R., Ortiz, A. C., Carvalho, T. S., Fideles, S. O. M., Araújo, T. T., Moraes, S. M., et al. (2020). Saliva as a diagnostic tool for dental caries, periodontal disease and cancer: is there a need for more biomarkers? Expert. Rev. Mol. Diagn. 20, 543–555. doi: 10.1080/14737159.2020.1743686
Camino, T., Lago-Baameiro, N., Bravo, S. B., Molares-Vila, A., Sueiro, A., Couto, I., et al. (2022). Human obese white adipose tissue sheds depot-specific extracellular vesicles and reveals candidate biomarkers for monitoring obesity and its comorbidities. Transl. Res. 239, 85–102. doi: 10.1016/j.trsl.2021.01.006
Camino, T., Lago-Baameiro, N., Bravo, S. B., Sueiro, A., Couto, I., Santos, F., et al. (2020). Vesicles shed by pathological murine adipocytes spread pathology: Characterization and functional role of insulin resistant/hypertrophied adiposomes. Int. J. Mol. Sci. 21, 2252. doi: 10.3390/ijms21062252
Carneiro, L. G., Nouh, H., Salih, E. (2014). Quantitative gingival crevicular fluid proteome in health and periodontal disease using stable isotope chemistries and mass spectrometry. J. Clin. Periodontol. 41, 733–747. doi: 10.1111/jcpe.12262
Casado-Fernández, L., Laso-García, F., Piniella, D., Gómez-de Frutos, M. C., Otero-Ortega, L., Bravo, S. B., et al. (2024). The proteomic signature of circulating extracellular vesicles following intracerebral hemorrhage: Novel insights into mechanisms underlying recovery. Neurobiol. Dis. 201, 106665. doi: 10.1016/J.NBD.2024.106665
Casarin, R. C. V., Salmon, C. R., Stolf, C. S., Paz, H. E. S., Rangel, T. P., Domingues, R. R., et al. (2023). Salivary annexin A1: A candidate biomarker for periodontitis. J. Clin. Periodontol. 50, 942–951. doi: 10.1111/jcpe.13803
Caton, J. G., Armitage, G., Berglundh, T., Chapple, I. L. C., Jepsen, S., Kornman, K. S., et al. (2018). A new classification scheme for periodontal and peri-implant diseases and conditions – Introduction and key changes from the 1999 classification. J. Clin. Periodontol. 45, S1–S8. doi: 10.1111/jcpe.12935
Chantada-Vázquez, M. P., Castro López, A., García Vence, M., Vázquez-Estévez, S., Acea-Nebril, B., Calatayud, D. G., et al. (2020). Proteomic investigation on bio-corona of Au, Ag and Fe nanoparticles for the discovery of triple negative breast cancer serum protein biomarkers. J. Proteomics. 212, 103581. doi: 10.1016/j.jprot.2019.103581
Colangelo, C. M., Ivosev, G., Chung, L., Abbott, T., Shifman, M., Sakaue, F., et al. (2015). Development of a highly automated and multiplexed targeted proteome pipeline and assay for 112 rat brain synaptic proteins. Proteomics 15, 1202–1214. doi: 10.1002/pmic.201400353
Demichev, V., Messner, C. B., Vernardis, S. I., Lilley, K. S., Ralser, M. (2020). DIA-NN: neural networks and interference correction enable deep proteome coverage in high throughput. Nat. Methods 17, 41–44. doi: 10.1038/s41592-019-0638-x
Eke, P. I., Borgnakke, W. S., Genco, R. J. (2020). Recent epidemiologic trends in periodontitis in the USA. Periodontol. 2000. 82, 257–267. doi: 10.1111/prd.12323
Ericksen, B., Wu, Z., Lu, W., Lehrer, R. I. (2005). Antibacterial activity and specificity of the six human {alpha}-defensins. Antimicrob. Agents Chemother. 49, 269–275. doi: 10.1128/AAC.49.1.269-275.2005
Faul, F., Erdfelder, E., Lang, A., Buchner, A. (2007). G*Power 3: A flexible statistical power analysis program for the social, behavioral, and biomedical sciences. Behav. Res. Methods 39, 175–191. doi: 10.3758/bf03193146
Frederick, K., Ciborowski, P. (2016). “SWATH-MS: Data acquisition and analysis,” in Proteomic Profiling and Analytical Chemistry. Eds. Ciborowski, P., Silberring, J. (Amsterdam: Elsevier), 161–173. doi: 10.1016/B978-0-444-63688-1.00009-4
Gao, S., Zhu, H., Zuo, X., Luo, H. (2018). Cathepsin G and its role in inflammation and autoimmune diseases. Arch. Rheumatol. 33, 498–504. doi: 10.5606/ArchRheumatol.2018.6595
García-Vence, M., Chantada-Vázquez, M. P., Cameselle-Teijeiro, J. M., Bravo, S. B., Núñez, C. (2020). A novel nanoproteomic approach for the identification of molecular targets associated with thyroid tumors. Nanomaterials 10, 2370. doi: 10.3390/nano10122370
Grant, M. M., Taylor, J. J., Jaedicke, K., Creese, A., Gowland, C., Burke, B., et al. (2022). Discovery, validation, and diagnostic ability of multiple protein-based biomarkers in saliva and gingival crevicular fluid to distinguish between health and periodontal diseases. J. Clin. Periodontol. 49, 622–632. doi: 10.1111/jcpe.13630
Groeger, S., Meyle, J. (2019). Oral mucosal epithelial cells. Front. Immunol. 10. doi: 10.3389/fimmu.2019.00208
Gürsoy, U. K., Kantarci, A. (2022). Molecular biomarker research in periodontology: A roadmap for translation of science to clinical assay validation. J. Clin. Periodontol. 49, 556–561. doi: 10.1111/jcpe.13617
Guzman, Y. A., Sakellari, D., Arsenakis, M., Floudas, C. A. (2014). Proteomics for the discovery of biomarkers and diagnosis of periodontitis: A critical review. Expert. Rev. Proteomics. 11, 31–41. doi: 10.1586/14789450.2014.864953
Guzman, Y. A., Sakellari, D., Papadimitriou, K., Floudas, C. A. (2018). High-throughput proteomic analysis of candidate biomarker changes in gingival crevicular fluid after treatment of chronic periodontitis. J. Periodontal. Res. 53, 853–860. doi: 10.1111/jre.12575
Hartenbach, F. A. R. R., Velasquez, É., Nogueira, F. C. S., Domont, G. B., Ferreira, E., Colombo, A. P. V. (2020). Proteomic analysis of whole saliva in chronic periodontitis. J. Proteomics. 213, 103602. doi: 10.1016/j.jprot.2019.103602
Jayasena, T., Poljak, A., Braidy, N., Zhong, L., Rowlands, B., Muenchhoff, J., et al. (2016). Application of targeted mass spectrometry for the quantification of sirtuins in the central nervous system. Sci. Rep. 6, 35391. doi: 10.1038/srep35391
Kassambara, A., Mundt, F. (2020). factoextra: Extract and visualize the results of multivariate data analyses. R package. Available online at: https://CRAN.R-project.org/package=factoextra (Accessed November 14, 2024).
Kim, J. S., Cho, I., Kim, K., Hwang, Y. S. (2021). Identification of galectin-10 as a biomarker for periodontitis based on proteomic analysis of gingival crevicular fluid. Mol. Med. Rep. 23, 123. doi: 10.3892/mmr.2020.11762
Kinane, D. F., Stathopoulou, P. G., Papapanou, P. N. (2017). Periodontal diseases. Nat. Rev. Dis. Primers. 3, 17039–17038. doi: 10.1038/nrdp.2017.38
Lê, S., Josse, J., Husson, F. (2008). FactoMineR: An R package for multivariate analysis. J. Stat. Software 25, 1–18. doi: 10.18637/jss.v025.i01
López-Valverde, L., Vázquez-Mosquera, M. E., Colón-Mejeras, C., Bravo, S. B., Barbosa-Gouveia, S., Álvarez, J. V., et al. (2024). Characterization of the plasma proteomic profile of Fabry disease: Potential sex- and clinical phenotype-specific biomarkers. Transl. Res. 269, 47–63. doi: 10.1016/j.trsl.2024.02.006
Ludwig, C., Gillet, L., Rosenberger, G., Amon, S., Collins, B. C., Aebersold, R. (2018). Data-independent acquisition-based SWATH-MS for quantitative proteomics: a tutorial. Mol. Syst. Biol. 14 Suppl, e8126–e8n/a. doi: 10.15252/msb.20178126
Marinho, M. C., Pacheco, A. B. F., Costa, G. C. V., Ortiz, N. D., Zajdenverg, L., Sansone, C. (2019). Quantitative gingival crevicular fluid proteome in type 2 diabetes mellitus and chronic periodontitis. Dis. 25, 588–595. doi: 10.1111/odi.12996
Megha, K. B., Mohanan, P. V. (2021). Role of immunoglobulin and antibodies in disease management. Int. J. Biol. Macromol. 169, 28–38. doi: 10.1016/j.ijbiomac.2020.12.073
Meijer, B., Gearry, R. B., Day, A. S. (2012). The role of S100A12 as a systemic marker of inflammation. Int. J. Inflam. 2012, 907078–907076. doi: 10.1155/2012/907078
Mertens, B., Orti, V., Vialaret, J., Gibert, P., Relaño-Ginés, A., Lehmann, S., et al. (2018). Assessing a multiplex-targeted proteomics approach for the clinical diagnosis of periodontitis using saliva samples. Bioanalysis 10, 35–45. doi: 10.4155/bio-2017-0218
Mondelo-Macía, P., García-González, J., León-Mateos, L., Abalo, A., Bravo, S., Chantada Vazquez, M. P., et al. (2024). Identification of a proteomic signature for predicting immunotherapy response in patients with metastatic non-small cell lung cancer. Mol. Cell. Proteomics. 23, 100834. doi: 10.1016/J.MCPRO.2024.100834
Monsarrat, P., Blaizot, A., Kémoun, P., Ravaud, P., Nabet, C., Sixou, M., et al. (2016). Clinical research activity in periodontal medicine: A systematic mapping of trial registers. J. Clin. Periodontol. 43, 390–400. doi: 10.1111/jcpe.12534
Mulla, S. A., Patil, A., Mali, S., Jain, A., Sharma, D., Jaiswal, H. C., et al. (2023). Exploring the biological width in dentistry: A comprehensive narrative review. Curēus 15, e42080. doi: 10.7759/cureus.42080
Murakami, M., Lopez-Garcia, B., Braff, M., Dorschner, R. A., Gallo, R. L. (2004). Postsecretory processing generates multiple cathelicidins for enhanced topical antimicrobial defense. J. Immunol. 172, 3070–3077. doi: 10.4049/jimmunol.172.5.3070
Navazesh, M., Christensen, C. M. (1982). A comparison of whole mouth resting and stimulated salivary measurement procedures. J. Dent. Res. 61, 1158–1162. doi: 10.1177/00220345820610100901
Novelle, M. G., Bravo, S. B., Deshons, M., Iglesias, C., García-Vence, M., Annells, R., et al. (2021). Impact of liver-specific GLUT8 silencing on fructose-induced inflammation and omega oxidation. iScience 24, 102071. doi: 10.1016/j.isci.2021.102071
Nóvoa, E., da Silva Lima, N., Gonzalez-Rellan, M. J., Chantada-Vazquez, M. P., Verheij, J., Rodriguez, A., et al. (2025). Mitochondrial antiviral signaling protein enhances MASLD progression through the ERK/TNFα/NFκβ pathway. Hepatology. 81:1535–1552. doi: 10.1097/hep.0000000000000930
Oksanen, J., Simpson, G., Blanchet, F., Kindt, R., Legendre, P., Minchin, P., et al. (2022). vegan: Community ecology package. R package. Available online at: https://CRAN.R-project.org/package=vegan (Accessed November 14, 2024).
Ong, S. T., Shan Ho, J. Z., Ho, B., Ding, J. L. (2006). Iron-withholding strategy in innate immunity. Immunobiology 211, 295–314. doi: 10.1016/j.imbio.2006.02.004
Ortea, I., Ruiz-Sánchez, I., Cañete, R., Caballero-Villarraso, J., Cañete, M. D. (2018). Identification of candidate serum biomarkers of childhood-onset growth hormone deficiency using SWATH-MS and feature selection. J. Proteomics. 175, 105–113. doi: 10.1016/j.jprot.2018.01.003
Perez-Hernandez, D., Gutiérrez-Vázquez, C., Jorge, I., López-Martín, S., Ursa, A., Sánchez-Madrid, F., et al. (2013). The intracellular interactome of tetraspanin-enriched microdomains reveals their function as sorting machineries toward exosomes. J. Biol. Chem. 288, 11649–11661. doi: 10.1074/jbc.M112.445304
R Core Team (2022). R: A language and environment for statistical computing (Vienna, Austria: R Foundation for Statistical Computing). Available at: https://www.R-project.org/ (Accessed November 14, 2024).
Rizal, M. I., Soeroso, Y., Sulijaya, B., Assiddiq, B. F., Bachtiar, E. W., Bachtiar, B. M. (2020). Proteomics approach for biomarkers and diagnosis of periodontitis: systematic review. Heliyon 6, e04022. doi: 10.1016/j.heliyon.2020.e04022
Rodrigues, J. S., Chenlo, M., Bravo, S. B., Perez-Romero, S., Suarez-Fariña, M., Sobrino, T., et al. (2024). dsRNAi-mediated silencing of PIAS2beta specifically kills anaplastic carcinomas by mitotic catastrophe. Nat. Commun. 15, 3736. doi: 10.1038/s41467-024-47751-1
Rosenberger, G., Koh, C. C., Guo, T., Röst, H. L., Kouvonen, P., Collins, B. C., et al. (2014). A repository of assays to quantify 10,000 human proteins by SWATH-MS. Sci. Data. 1, 140031. doi: 10.1038/sdata.2014.31
Salazar, M. G., Jehmlich, N., Murr, A., Dhople, V. M., Holtfreter, B., Hammer, E., et al. (2013). Identification of periodontitis associated changes in the proteome of whole human saliva by mass spectrometric analysis. J. Clin. Periodontol. 40, 825–832. doi: 10.1111/jcpe.12130
Sampath, P., Mazumder, B., Seshadri, V., Fox, P. L. (2003). Transcript-selective translational silencing by gamma interferon is directed by a novel structural element in the ceruloplasmin mRNA 3′ untranslated region. Mol. Cell. Biol. 23, 1509–1519. doi: 10.1128/MCB.23.5.1509-1519.2003
Shevchenko, A., Wilm, M., Vorm, O., Mann, M. (1996). Mass spectrometric sequencing of proteins from silver-stained polyacrylamide gels. Anal. Chem. 68, 850–858. doi: 10.1021/ac950914h
Shin, M., Kim, Y., Shin, Y. J., Ko, B. J., Kim, S., Kim, H. (2019). Deep sequencing salivary proteins for periodontitis using proteomics. Clin. Invest. 23, 3571–3580. doi: 10.1007/s00784-018-2779-1
Silbereisen, A., Bao, K., Wolski, W., Nanni, P., Kunz, L., Afacan, B., et al. (2024). Probing the salivary proteome for prognostic biomarkers in response to non-surgical periodontal therapy. J. Clin. Periodontol. 52, 56–67. doi: 10.1111/jcpe.13990
Silva-Boghossian, C. M., Colombo, A. P. V., Tanaka, M., Rayo, C., Xiao, Y., Siqueira, W. L. (2013). Quantitative proteomic analysis of gingival crevicular fluid in different periodontal conditions. PloS One 8, e75898. doi: 10.1371/journal.pone.0075898
Smith, A., McCulloh, R. J. (2015). Hemopexin and haptoglobin: allies against heme toxicity from hemoglobin not contenders. Front. Physiol. 6. doi: 10.3389/fphys.2015.00187
Soehnlein, O., Lindbom, L. (2009). Neutrophil-derived azurocidin alarms the immune system. J. Leukoc. Biol. 85, 344–351. doi: 10.1189/jlb.0808495
Sreejit, G., Ahmed, A., Parveen, N., Jha, V., Valluri, V. L., Ghosh, S., et al. (2014). The ESAT-6 protein of Mycobacterium tuberculosis interacts with beta-2-microglobulin (β2M) affecting antigen presentation function of macrophage. PloS Pathog. 10, e1004446. doi: 10.1371/journal.ppat.1004446
Sun, Q., Li, N., Jia, L., Guo, W., Jiang, H., Liu, B., et al. (2021). Ribosomal protein SA-positive neutrophil elicits stronger phagocytosis and neutrophil extracellular trap formation and subdues pro-inflammatory cytokine secretion against Streptococcus suis serotype 2 infection. Front. Immunol. 11. doi: 10.3389/fimmu.2020.585399
Surna, A., Kubilius, R., Sakalauskiene, J., Vitkauskiene, A., Jonaitis, J., Saferis, V., et al. (2009). Lysozyme and microbiota in relation to gingivitis and periodontitis. Med. Sci. Monit. 15, CR66–CR73.
Tang, H., Yuan, C., Ma, Z., Zhu, C., Tong, P., Gallagher, J. E., et al. (2019). The potentiality of salivary peptide biomarkers for screening patients with periodontal diseases by mass spectrometry. Clin. Chim. Acta 495, 278–286. doi: 10.1016/j.cca.2019.04.076
Team, M. C., Blanchard, G., Dickhaus, T., Hack, N., Konietschke, F., Rohmeyer, K., et al. (2023). mutoss: Unified multiple testing procedures (Wien: R package). Available at: https://CRAN.R-project.org/package=mutoss (Accessed November 14, 2024).
Tisdale, E. J. (2002). Glyceraldehyde-3-phosphate dehydrogenase is phosphorylated by protein kinase ciota/lambda and plays a role in microtubule dynamics in the early secretory pathway. J. Biol. Chem. 277, 3334. doi: 10.1074/jbc.M109744200
Tonetti, M. S., Greenwell, H., Kornman, K. S. (2018). Staging and grading of periodontitis: Framework and proposal of a new classification and case definition. J. Clin. Periodontol. 45, S149–S161. doi: 10.1111/jcpe.12945
Torchiano, M. (2020). effsize: Efficient effect size computation (Wien: R package). Available at: https://CRAN.R-project.org/package=effsize (Accessed November 14, 2024).
Torres Iglesias, G., López-Molina, M. P., Botella, L., Laso-García, F., Chamorro, B., Fernández-Fournier, M., et al. (2024). Differential protein expression in extracellular vesicles defines treatment responders and non-responders in multiple sclerosis. Int. J. Mol. Sci. 25, 10761. doi: 10.3390/ijms251910761
Tripathi, D., Kant, S., Pandey, S., Ehtesham, N. Z. (2020). Resistin in metabolism, inflammation, and disease. Febs. J. 287, 3141–3149. doi: 10.1111/febs.15322
UniProt Consortium (2014). Activities at the universal protein resource (UniProt). Nucleic Acids Res. 42, D191–D198. doi: 10.1093/nar/gkt1140
Vázquez-Mera, S., Martelo-Vidal, L., Miguéns-Suárez, P., Bravo, S. B., Saavedra-Nieves, P., Arias, P., et al. (2024). Exploring CD26–/lo subpopulations of lymphocytes in asthma phenotype and severity: A novel CD4+ T cell subset expressing archetypical granulocyte proteins. Allergy 79, 3005–3021. doi: 10.1111/all.16327
Wang, F., Qiao, L., Lv, X., Trivett, A., Yang, R., Oppenheim, J. J., et al. (2016). Alarmin human α defensin HNP1 activates plasmacytoid dendritic cells by triggering NF-κB and IRF1 signaling pathways. Cytokine 83, 53–60. doi: 10.1016/j.cyto.2016.03.015
Wang, S., Song, R., Wang, Z., Jing, Z., Wang, S., Ma, J. (2018). S100A8/A9 in inflammation. Front. Immunol. 9. doi: 10.3389/fimmu.2018.01298
World Medical Association (2013). World medical association declaration of Helsinki: Ethical principles for medical research involving human subjects. JAMA 310, 2191–2194. doi: 10.1001/jama.2013.281053
Yi, J., Shen, Y., Yang, Y., Shen, C., Liu, B., Qiao, L., et al. (2021). Direct MALDI-TOF profiling of gingival crevicular fluid sediments for periodontitis diagnosis. Talanta 225, 121956. doi: 10.1016/j.talanta.2020.121956
Keywords: periodontitis, gingival crevicular fluid, saliva, oral fluids, protein expression, SWATH-MS, mass spectrometry, proteomics
Citation: Blanco-Pintos T, Regueira-Iglesias A, Suárez-Rodríguez B, Seijas-Otero N, Relvas M, Bravo SB, Balsa-Castro C and Tomás I (2025) Characterisation of the periodontal proteome in gingival crevicular fluid and saliva using SWATH-MS. Front. Cell. Infect. Microbiol. 15:1576906. doi: 10.3389/fcimb.2025.1576906
Received: 28 February 2025; Accepted: 07 April 2025;
Published: 02 May 2025.
Edited by:
Lucinda Janete Bessa, Egas Moniz Center for Interdisciplinary Research (CiiEM), PortugalReviewed by:
Ganesan Saibaba, Hebrew University of Jerusalem, IsraelStefania Bergamini, University of Modena and Reggio Emilia, Italy
Copyright © 2025 Blanco-Pintos, Regueira-Iglesias, Suárez-Rodríguez, Seijas-Otero, Relvas, Bravo, Balsa-Castro and Tomás. This is an open-access article distributed under the terms of the Creative Commons Attribution License (CC BY). The use, distribution or reproduction in other forums is permitted, provided the original author(s) and the copyright owner(s) are credited and that the original publication in this journal is cited, in accordance with accepted academic practice. No use, distribution or reproduction is permitted which does not comply with these terms.
*Correspondence: Inmaculada Tomás, aW5tYWN1bGFkYS50b21hc0B1c2MuZXM=; Alba Regueira-Iglesias, YWxiYS5yZWd1ZWlyYS5pZ2xlc2lhc0BzZXJnYXMuZXM=
†ORCID: Alba Regueira-Iglesias, orcid.org/0000-0002-6549-7738
Inmaculada Tomás, orcid.org/0000-0002-3317-0853