- Political Data Science, Technical University of Munich, Munich, Germany
This study examines the role of coordinated inauthentic user behavior (CIUB) in the German COVID-19 discourse, focusing on the #nocovid hashtag. We analyze CIUB in relation to three dimensions of social media: communication, networks, and platforms. While our findings confirm the presence of coordinated behavior within the discourse, our analysis shows that CIUB itself did not significantly influence the debate. Instead, platform policies appear to have played a crucial role in shaping engagement by selectively amplifying or suppressing specific viewpoints. Using Granger causality analysis, we demonstrate that coordinated activity does not drive non-coordinated discourse. Conversely, an analysis of platform effects reveals that X’s algorithm systematically limited the visibility of anti-nocovid viewpoints. These findings highlight the need to reassess concerns about the influence of CIUB, shifting focus toward the role of platform policies in structuring online debates.
Introduction
Social media has become a dominant force in shaping public discourse, influencing political opinions, and amplifying narratives. It plays a crucial role in contemporary conflicts, such as the Russian-Ukraine war, the Israel-Palestinian war, and the COVID-19 pandemic, where competing narratives are propagated across platforms. One major concern in social media research is coordinated inauthentic user behavior (CIUB)—the organized and strategic dissemination of information by bots, trolls, or hyperactive users to manipulate public perception. CIUB is commonly associated with political propaganda, misinformation, and influence campaigns, often backed by state or non-state actors (Giglietto et al., 2019; Alizadeh et al., 2020).
Another related concern is social manipulation, which refers to the deliberate use of digital platforms to shape opinions, reinforce biases, or alter public perception through coordinated or algorithmic means. This manipulation can occur in various forms. Disinformation campaigns involve the intentional spread of false or misleading content (Lazer et al., 2018), while algorithmic amplification affects the visibility of content by prioritizing certain narratives over others based on engagement metrics (Hegelich et al., 2023). Another form of manipulation is coordinated inauthentic behavior, in which networks of bots or hyperactive users artificially boost specific viewpoints, increasing their prominence within a debate (Giglietto et al., 2019). Unlike organic discussions, where information spreads naturally among individuals, these forms of manipulation rely on strategic coordination—whether by human actors or automated scripts—to influence which narratives gain traction. It is important to distinguish between manipulation and its consequences; for example, while polarization and misinformation may result from manipulation, they are not inherently forms of it.
Extensive research has examined social media disinformation, focusing on detection methods, counterstrategies, and engagement patterns. Studies have explored computational approaches for identifying false information (Aïmeur et al., 2023; Wu et al., 2019), intervention strategies to reduce belief in misinformation (Saurwein and Spencer-Smith, 2020; Bode and Vraga, 2018), and the ways users engage with misleading content on different platforms (Shin et al., 2018; Allcott et al., 2019). The spread of false health information has also been a major topic of concern, particularly during the COVID-19 pandemic (Gabarron et al., 2021; Al-Zaman, 2021). While this body of literature provides valuable insights into how misinformation circulates, it does not fully explain its effects on public discourse. However, misinformation and coordinated inauthentic user behavior (CIUB) are distinct phenomena: misinformation refers to false or misleading content, whereas CIUB describes the deliberate, coordinated actions used to amplify narratives—whether true or false. Despite extensive research on disinformation, there remains a lack of empirical evidence assessing whether CIUB itself changes public opinion or whether its influence has been overstated. This gap is especially relevant when considering claims about the power of coordinated networks in shaping political and social debates.
This article examines the role of coordinated behavior in the German COVID-19 discourse on X, using the hashtag #nocovid as a case study. Prior research suggests that hyperactive and hyperconnected users disproportionately shape social media debates (Papakyriakopoulos et al., 2020), but it remains unclear whether their activity actually drives engagement among non-coordinated users or whether it circulates primarily within isolated clusters. Understanding the effectiveness of coordination in shaping online discourse requires a more precise categorization of the different functions of social media. To this end, we draw on the framework of functional differentiation proposed by Hegelich et al. (2023) and Dhawan et al. (2022), which divides social media into three key dimensions: communication, networks, and platforms. Each of these dimensions plays a distinct role in determining how information spreads and how narratives evolve.
This study is guided by three main research questions. First, was there coordinated, inauthentic user behavior (CIUB) in the German COVID-19 discourse? Second, did CIUB influence the German discourse on X? Finally, is there empirical evidence that platform policies, such as algorithmic ranking, affected the discourse? To answer these questions, we employ a computational approach to detect coordination using the CooRTweet package in R (Righetti and Balluff, 2024). To assess whether coordination influenced non-coordinated users, we conduct a Granger causality analysis (Granger, 1969; Shojaie and Fox, 2022), which tests whether past instances of coordinated activity can predict future non-coordinated engagement. Lastly, to evaluate the role of platform policies, we analyze the extent to which X’s algorithm may have differentially amplified or suppressed certain viewpoints within the #nocovid debate. By integrating these methods, we aim to provide a more nuanced understanding of CIUB’s role in shaping online discussions, challenging assumptions about its effectiveness while highlighting the broader influence of platform policies on discourse visibility.
Status quo
Understanding the role of coordinated inauthentic user behavior (CIUB) requires distinguishing between different aspects of social media dynamics. Following the framework proposed by Dhawan et al. (2022) in Restart Social Media?, we categorize social media into three key dimensions: communication, networks, and platforms. Each dimension plays a distinct role in the spread of disinformation, amplification of coordinated narratives, and structuring of online debates.
• Social media communication deals with echo chambers that support preexisting opinions and promote oversimplified, emotionally charged information that can create polarization. This dimension focuses on the content circulated within the networks.
• Social media networks refer to the connections between users, coined “small world networks.” The information flow within these networks is disproportionately influenced by a small number of hyperconnected and hyperactive users, which causes problems, including the dissemination of fake news. This dimension focuses on the structure of the network.
• Social media platforms focus on maximizing their economic revenue through machine learning algorithms that increase user interactions, resulting in shallow content that values instant gratification over meaningful connection. Platforms “over-fit” user preferences as a result of this excessive dependence on “meaningful interaction” measurements, which sets up a positive feedback loop. This dimension focuses on the business model of a given platform.
In the following, we map the existing literature on coordinated inauthentic user behavior for each of the three elements.
Social media communication
The COVID-19 pandemic underscored the complexity of information flow on social media, where misinformation and disinformation rapidly spread alongside credible information. The concept of an “infodemic” became particularly relevant, describing how an overwhelming volume of information—both true and false—creates confusion and undermines public trust (Tsao et al., 2021). Social media platforms attempted to counteract false claims through fact-checking and algorithmic interventions, yet studies show that CIUB actors quickly adapted, modifying their messaging strategies to evade moderation (Lee et al., 2023). A notable example is the shifting scientific consensus on COVID-19 transmission. In early 2020, posts suggesting SARS-CoV-2 was airborne were labeled as misinformation, only for the claim to be widely accepted by scientists in 2022 (Gisondi et al., 2022). This raises a critical question: when platforms suppress certain narratives, do they effectively combat misinformation, or do they also risk obstructing legitimate scientific debate?
Another significant instance of misinformation spread via CIUB was the 5G-Coronavirus conspiracy theory, which falsely claimed that 5G wireless networks were responsible for the spread of COVID-19. This theory gained traction on what was then called Twitter and other platforms, with the hashtag #5GCoronavirus trending in the United Kingdom for seven consecutive days between March 27 and April 4, 2020. Ahmed et al. (2020) conducted a social network analysis of this disinformation campaign and found that the most influential actors belonged to two distinct groups: a broadcast network, where a small number of users amplified the conspiracy theory to a wide audience, and an isolated group that engaged in fragmented discussions reinforcing their existing beliefs. Notably, their analysis showed a lack of authoritative voices actively countering the misinformation, allowing the narrative to spread unchecked.
Similarly, Graham et al. (2020) demonstrate how disinformation spreads virally through coordinated networks, showing that pandemic-related falsehoods—ranging from vaccine conspiracies to fabricated infection statistics—were strategically disseminated to exploit public fears and amplify distrust in governmental institutions. This case illustrates how CIUB actors leverage algorithmic amplification and social clustering to manufacture credibility for false claims, making misinformation harder to debunk. Wang et al. (2020) further examined pandemic-related sentiment on Sina Weibo, a major Chinese social media platform, revealing that fear and distrust toward health authorities dominated discussions. Their findings align with broader research showing that misinformation thrives in uncertain environments, where social media users rely on peer validation rather than institutional sources to assess credibility. The rapid dissemination of misinformation on both Western and Chinese social media highlights how CIUB actors capitalize on public anxieties to spread misleading narratives.
Beyond public health misinformation, political actors have also leveraged CIUB to manipulate discourse and control narratives. Research has shown that coordinated disinformation campaigns can be used for geopolitical influence, as seen in ISIS propaganda efforts, where social media messaging was tailored to appear uniquely relevant to Arabic-speaking recruits (Badawy and Ferrara, 2018). Similarly, the Russian Internet Research Agency (IRA) has been documented employing CIUB techniques to fabricate grassroots support for various geopolitical interests, including election interference and protest narratives (Pacheco et al., 2021). More recently, coordinated content dissemination was observed in the U.S. 2020 presidential election, when multiple Twitter users posted identical claims about leaving their spouses due to Biden’s victory—an artificial attempt to create the illusion of widespread discontent (Weber and Neumann, 2021). These cases demonstrate how CIUB is strategically deployed to construct deceptive narratives that appear organic.
Another prominent example of CIUB’s adaptability is the case of the Plandemic documentary, a widely debunked video that falsely claimed COVID-19 was a manufactured crisis. Despite being removed from major platforms, the documentary amassed over 8 million views across YouTube, Facebook, X (formerly Twitter), and Instagram before its takedown (Lee et al., 2023). This illustrates how CIUB actors continually evolve their strategies in response to countermeasures, finding new ways to sustain their influence even in the face of platform interventions.
The relationship between CIUB and political polarization is another crucial consideration. Studies suggest that rather than persuading neutral audiences, disinformation campaigns primarily reinforce preexisting ideological divisions (Shin et al., 2018). The phenomenon of echo chambers—where users are predominantly exposed to viewpoints that align with their beliefs—exacerbates this effect (Montag and Hegelich, 2020). Huszár et al. (2022) found that social media algorithms often amplify politically biased content, increasing the likelihood of ideological clustering. Similarly, Shahrezaye et al. (2020) demonstrated that a user’s political stance can often be inferred simply by analyzing their Facebook network, further emphasizing the role of social media in reinforcing political identities.
However, political elites also play a central role in shaping online discourse. Box-Steffensmeier and Moses (2021) found that elite figures strategically employed sentiment-driven messaging to engage with the public, reinforcing specific narratives about the pandemic. Their study highlights how sentiment—whether fear, reassurance, or urgency—was used to frame pandemic-related policies and responses. This aligns with broader concerns about how coordinated messaging, whether by political actors or coordinated inauthentic networks, can shape online debates, particularly in crisis situations where misinformation and political rhetoric often intertwine.
In addition to elite influence, user engagement patterns significantly shape the diffusion of disinformation. Tandoc et al. (2020) found that social media users respond to fake news in three key ways: endorsing it, rejecting it, or further disseminating it with commentary. However, even when users express skepticism, they may still unintentionally amplify misinformation, particularly when it aligns with their ideological positions. This suggests that CIUB does not necessarily create new beliefs but rather leverages existing cognitive biases to ensure the persistence and virality of misinformation. These dynamics indicate that the impact of disinformation is not merely a result of the content itself but also of how users engage with it.
Acclamation theory provides further insight into these engagement patterns, emphasizing that social media interactions are not purely about information-seeking but also about public signaling. Hegelich et al. (2023) argue that following an account or interacting with its content is an act of acclamation—a way for users to visibly declare allegiance to a particular group. Negative acclamation is also possible, where users deliberately engage with opposing content to signal disapproval. This has been observed in political contexts where users actively tag opponents to draw attention to conflicting viewpoints (Hegelich and Shahrezaye, 2015). The presence of both positive and negative acclamation on social media suggests that CIUB is not just a mechanism for spreading information; it is also a tool for identity signaling, which may contribute to increasing polarization in digital discourse.
Overall, social media communication is shaped not just by the content itself but by the coordinated efforts of actors who seek to manipulate its reach and interpretation. Whether through geopolitical campaigns, pandemic-related disinformation, or ideological echo chambers, CIUB plays a central role in shaping public discourse. While platform interventions can mitigate some forms of misinformation, the ability of CIUB actors to rapidly adapt and exploit new channels ensures that digital disinformation remains an evolving challenge.
Social media networks
Social media networks are not neutral spaces for information exchange; instead, they are highly asymmetric ecosystems where a small number of hyperactive users dominate the flow of information. Research shows that only 5%–10% of users generate up to 80% of political content, meaning that much of the digital debate is shaped by a concentrated subset of highly active participants (Papakyriakopoulos et al., 2020). These users often act as network hubs, exerting disproportionate influence on what appears to be organic public sentiment. This dynamic is further exacerbated by CIUB, as coordinated groups strategically exploit these network structures to manufacture the illusion of trends.
One of the most widely used CIUB tactics is Coordinated Link Sharing Behavior (CLSB), in which verified accounts, Facebook pages, or X users rapidly share identical content in a short period to manipulate platform algorithms and amplify visibility. Giglietto et al. (2019) documented the systematic use of CLSB during the 2018 and 2019 Italian elections, where coordinated networks were deployed to boost partisan content and artificially game engagement metrics. Building on this, Giglietto et al. (2020a) found that similar coordination tactics were employed during the Italian coronavirus outbreak, where networks selectively amplified certain news sources while suppressing others, shaping public discourse about the pandemic. These studies highlight how CLSB is not only used in electoral manipulation but also in crisis communication, reinforcing or distorting narratives in ways that affect public perception.
Beyond elections, CIUB has played a crucial role in framing social movements and protests. Giglietto et al. (2020b) demonstrated that CLSB is particularly effective in shaping news visibility—not by introducing new narratives but by amplifying existing partisan content to dominate media coverage. This was also evident in Brexit debates (Howard and Kollanyi, 2016), where synchronized hashtag usage and targeted engagement were used to steer public discourse. A similar strategy was employed in the #BLMKidnapping case, where far-right groups falsely linked a Chicago hate crime to the Black Lives Matter movement, amplifying the hashtag 480,000 times in a single day to distort public perception (Marwick, 2017). This exemplifies a technique known as “attention hacking,” where disinformation actors exploit platform algorithms by coordinating high-volume engagement to push misleading content into trending lists.
The disproportionate influence of hyperactive users plays a critical role in CIUB effectiveness. Rather than spreading information randomly, digital networks follow a small-world structure, where a few highly connected users act as central hubs (Papakyriakopoulos et al., 2020). These users—whether influencers, bots, or ideological activists—are capable of quickly disseminating content, ensuring that coordinated messaging gains traction. Luceri et al. (2020) found that far-right movements in Italy, such as Lega and Movimento 5 Stelle, systematically used CIUB techniques to spread inflammatory content about North African migration. This was achieved through coordinated retweeting, where bot networks amplified posts from a small set of influential accounts, boosting their reach beyond what would be organically possible (Del Vigna et al., 2019).
State-sponsored actors have also exploited small-world networks to further their geopolitical interests. Giglietto et al. (2020a) showed that during the COVID-19 pandemic in Italy, certain state-linked actors engaged in CLSB to strategically promote narratives favorable to their political agendas. Similarly, Luceri et al. (2020) documented how Russian and Chinese state-backed bots systematically amplified pandemic-related conspiracy theories, questioning vaccine safety and spreading doubts about COVID-19 origins. Meanwhile, Venezuelan troll networks were identified spreading anti-U.S. narratives, using specific fake news websites such as trumpnewss.com to target American audiences (Alizadeh et al., 2020). The Russian Internet Research Agency (IRA) has been one of the most extensively documented state-sponsored CIUB actors. Pacheco et al. (2021) found that IRA-linked accounts played a role in shaping narratives around the 2016 U.S. elections, the Syrian civil war, and Hong Kong protests, employing a combination of automated bots and human-run troll farms to fabricate grassroots support for specific agendas. The same techniques were later observed in the German 2016 elections, where Assenmacher et al. (2020) identified coordinated bot activity attempting to introduce anti-Merkel hashtags into trending discussions.
While CIUB is effective at manipulating online engagement, its actual impact on shifting political opinions remains contested. Research suggests that disinformation primarily affects engagement patterns rather than voter behavior, as CIUB often reinforces ideological divides rather than persuading neutral audiences (Shahrezaye et al., 2019). Giglietto et al. (2020b) argue that while CLSB can dominate media coverage, it does not necessarily change political attitudes; rather, it ensures that partisan perspectives become the most visible. This distinction is crucial for assessing whether CIUB fundamentally alters political landscapes or merely intensifies existing polarization.
In some cases, coordinated networks do succeed in mobilizing public action. For instance, the “Ice Bucket Challenge,” though not an example of disinformation, illustrates how social media coordination can be harnessed for legitimate social causes (Keller et al., 2019). Through a combination of celebrity endorsements, viral challenges, and networked visibility, the campaign successfully raised millions for ALS research. This example demonstrates that CIUB techniques are not inherently malicious—rather, their effects depend on the intentions of the actors deploying them.
This discussion of social media networks underscores the significant role of CIUB in shaping digital discourse, particularly through hyperactive users, state-sponsored disinformation, and engagement manipulation. While its influence on public opinion remains a subject of debate, its capacity to amplify certain narratives while suppressing others is undeniable. The structural nature of social media networks facilitates these activities, making them a central focus in understanding the broader impact of digital coordination strategies.
Social media platforms
Beyond user-driven coordination, social media platforms play a crucial role in shaping digital discourse through algorithmic ranking, content devaluation, and moderation policies. While these mechanisms are often justified as necessary to combat misinformation and maintain information integrity, they also raise concerns about selective suppression and the influence of platform governance on public debate. Platforms such as X use engagement-based algorithms to determine content visibility, amplifying posts that receive high interaction while limiting the reach of content flagged as misleading or harmful. However, the criteria for such classifications and the extent of their impact remain highly contested (Hegelich et al., 2023).
One of the most influential mechanisms in X’s content ranking system is the “social proof” filter, which prioritizes posts that have been engaged with by a user’s network. While this system is designed to promote relevance and reduce misinformation, it also reinforces dominant narratives by amplifying already popular content while suppressing alternative viewpoints. Hegelich et al. (2023) analyzed how this recommendation system functioned during U.S. political debates and found that content classified as misinformation—particularly from conservative sources—was systematically de-ranked, limiting its exposure. Similar concerns have been raised about the #nocovid debate, where our findings suggest that tweets opposing the nocovid strategy received fewer retweets per follower than those supporting it. While algorithmic suppression is one possible explanation, alternative factors—such as differences in audience composition, organic engagement levels, and media framing—must also be considered before attributing these disparities solely to platform intervention.
State regulations further complicate platform governance, as governments increasingly pressure tech companies to moderate content according to national policies. One notable example is Germany’s Network Enforcement Act (NetzDG), which requires social media platforms to remove flagged content within 24 h or face substantial fines (Gorwa, 2021). While proponents argue that such laws are necessary to 0combat hate speech and misinformation, critics warn that they risk over-censoring legitimate political discourse, particularly when implemented without transparent oversight. This regulatory pressure has led platforms to develop more aggressive moderation strategies, which, in turn, fuel accusations of political bias in content suppression.
The issue of shadow banning—where content is de-ranked or made less visible without notifying users—remains a point of contention. Some argue that platforms use these mechanisms to silence dissenting political views, particularly those that challenge mainstream narratives. On the other hand, platform companies such as X and Meta defend these practices as essential for maintaining the quality and integrity of public discourse, preventing coordinated inauthentic behavior from manipulating engagement metrics. The broader debate is not simply whether platforms suppress certain viewpoints, but whether their moderation policies are applied consistently across ideological lines. As social media companies continue refining their approaches to content governance, the tension between combating disinformation and ensuring a fair and open digital space remains unresolved.
Methods
To investigate coordinated inauthentic user behavior (CIUB) in the German COVID-19 discourse, we collected tweets from March 2020 to January 2023 using what was then called Twitter’s Academic API. The dataset consists of tweets containing trending COVID-19-related hashtags in Germany, including #nocovid, #longcovidkids (raising awareness of long Covid in children), #Querdenkersindterroristen (“lateral thinkers are terrorists,” used to criticize anti-lockdown movements), #Lauterbachmussweg (“Lauterbach must go,” calling for the resignation of Germany’s health minister Karl Lauterbach), and #Ichhabemitgemacht (“I went along with it,” a critical reflection on pandemic policies and public compliance). Among these hashtags, #nocovid was selected as the primary focus of our study because it uniquely represents a point of intense societal division. Unlike many hashtags that are predominantly associated with a single viewpoint, #nocovid was used by both proponents and opponents of strict pandemic measures, making it an ideal case for analyzing coordinated behavior across conflicting opinion clusters. The presence of coordination on both sides of the debate allowed us to examine whether coordinated activity influenced public discourse in a polarized environment. Additionally, this dual usage of the hashtag provided a clear visualization of social division, highlighting how the same digital space was leveraged by competing narratives to advance their agendas.
Due to recent restrictions on academic data access by X, the #nocovid dataset is limited in size. However, a qualitative review suggests that it captures central trends in the German COVID-19 debate, ensuring the validity of our analysis. In total, the dataset contains 102,147 tweets, with 4,523 users identified as engaging in coordinated activity at least three times within a 10-min window. The coordinated network consists of 4,523 nodes (users) and 712,718 edges (connections), revealing a complex web of interactions that highlights patterns of coordinated amplification. To detect coordination, we applied the CooRTweet package in R, which identifies five distinct coordination behaviors: coordinated link sharing, coordinated retweeting, coordinated hashtag usage, coordinated tweeting, and coordinated replying. Coordinated link sharing detects users who post the same link within a short timeframe, potentially indicating attempts to artificially amplify specific content. Coordinated retweeting identifies users who retweet the same post within a defined time interval, a known strategy in manipulation campaigns. Coordinated hashtag usage flags users who post identical hashtags simultaneously, often manipulate trending topics. Coordinated tweeting captures users who post the exact same text in rapid succession, behavior commonly associated with bot networks or scripted content distribution. Lastly, coordinated replying identifies accounts systematically replying to certain tweets, either to support or counter a narrative.
We established a minimum threshold of three instances within a 10-min window, to classify interactions as coordinated. This means that if at least three users engaged in the same retweeting, hashtag use, or replying pattern within this timeframe, their behavior was flagged as coordination. The results indicate that coordinated hashtag usage, coordinated replying (same user), and coordinated retweeting were the most significant forms of coordination, while coordinated link sharing, coordinated tweeting, and coordinated replying (same text) were not prevalent. The analysis revealed three distinct network components, primarily dominated by hyperactive users and suspected automated accounts. To quantify the influence of coordinated actors, we ranked users by their PageRank scores, a network centrality measure that highlights highly influential nodes within the network. We also analyzed their follower counts and classified them into different clusters based on coordination behavior. Moreover, we conducted a Granger causality test to examine whether peaks in coordinated tweeting predicted increases in non-coordinated tweeting. This test determines whether past values of one variable (coordinated tweets) help predict future values of another (non-coordinated tweets), thus identifying potential causal relationships and assessing whether coordinated behavior influenced broader discourse.
For this analysis, all tweets were converted into a time-series format, rounded to the nearest five-minute interval. The tweets were categorized into two time series: one consisting of coordinated tweets flagged under CIUB criteria and another comprising non-coordinated tweets representing organic discourse. The Granger causality test was then applied to investigate whether coordinated activity led to subsequent increases in non-coordinated tweeting across various time lags ranging from 5 to 60 min. If coordination were driving a broader discourse, we would expect to see a strong causal relationship where spikes in coordinated activity precede increased engagement in non-coordinated discussions. However, as outlined in the results section, our findings do not support this hypothesis, indicating that CIUB does not necessarily trigger widespread engagement beyond its immediate participants.
Beyond detecting coordination, we examined whether platform algorithms influenced engagement patterns across different opinion clusters. To test for potential algorithmic suppression, we calculated a retweet-to-follower ratio, which measures how many retweets a tweet receives per 100 followers. If one group consistently receives fewer retweets per follower than another, it could indicate algorithmic de-ranking or shadow banning.
For visualization, we logged the relation mean (retweets per follower) for each tweet and compared engagement trends between clusters supporting and opposing #nocovid. If the platform treated all tweets equally, we would expect similar engagement ratios across clusters when controlling for follower count. However, if one group systematically received lower engagement, it could suggest selective algorithmic filtering. The results presented in the next section explore whether such patterns emerged in the dataset.
This study acknowledges several limitations. Due to X’s policy changes, expanding the dataset is not possible. This issue has drawn criticism from the European Commission, which is currently investigating X’s reluctance to share data with researchers (Netzpolitik.org, 2024). The dataset is also subject to sampling bias, as emphasized by Pfeffer et al. (2018), meaning that our findings must be interpreted within the constraints of the available data. Furthermore, while we detect coordination, we do not claim that all identified activity is inauthentic. Some coordination may stem from genuine activism or shared ideological behavior, rather than deliberate manipulation.
Results
Social media communication
Was there any coordinated, inauthentic user behavior in the German COVID-19 discourse?
Yes, there is evidence of coordinated inauthentic user behavior in the German COVID-19 discussions on whether nocovid is a good strategy or not. It is not trivial to say from which side the coordination starts because it is used by both sides.
The hashtag #nocovid refers to a strategy or approach that aims to eliminate or reduce the spread of COVID-19 within a population. It involves implementing strict measures, such as lockdowns, travel restrictions, and extensive testing, to achieve low or zero cases of the virus. Certain individuals and groups advocate for this approach as an effective way to control the pandemic. The hashtag #ZeroCovid aligns with the same concept but is about eliminating COVID-19 entirely from a specific region or country. It emphasizes strict measures and a strong focus on preventing virus transmission to achieve zero cases.
In the data analyzed, we can observe the recurring use of the hashtags #Nocovid and #ZeroCovid. These hashtags are often accompanied by references to the protests in China, as indicated by the hashtag #ChinaProtests. China was mentioned in 10,072 tweets. The combination of these hashtags with references to protests in China suggests a commentary on the response to COVID-19 in different countries. It appears that the discussion is about the measures taken by the Chinese government to control the virus, drawing parallels to the advocacy for similar approaches in Germany. Tweets also mention conspiracy theories involving China and nocovid/ZeroCovid, suggesting ulterior motives, power expansion, and control. The tweets also discuss the role of China in the COVID-19 discourse in Germany and the potential influence of conspiracy. There were tweets that associated nocovid/ZeroCovid with a cult-like sect.
In addition to the mention of China, Jacinda Ardern is mentioned in 3451 tweets. In comparison to the frequency with which China was mentioned, those tweets were also worth looking at, as they were supporters of #ZeroCovid. This cluster cites countries such as Australia, New Zealand, and Taiwan, which have implemented different COVID-19 strategies, suggesting that their lockdowns are not equal to a Shanghai-style lockdown. Those tweets suggest advocacy for #ZeroCovid.
On the one hand, the accompanying hashtags, besides #ZeroCovid, are #Maskenpflicht (duty to mask), #Luftfilter (air filter), #ImpfenSchuetzt (vaccination protects), and #allesdichtmachen (close everything), which shows that those users are advocating this policy. It was important for them to distance themselves from the no-covid policy in China and highlight the success of this policy in other countries such as New Zealand, Australia, Japan, and South Korea.
On the other hand, there was also a cluster that supported #Bhakdi and #Wodarg. Sucharit Bhakdi and Wolfgang Wodarg are doctors who have been very critical of COVID-19 and think the pandemic is fake. They also highlighted that China had a spike in infections despite nocovid! They claim that “#ZeroCovid war und ist eindeutiger Totalitarismus, rücksichtslos und grundrechtsverletzend” (#ZeroCovid was and is clear totalitarianism, ruthless, and violating fundamental rights), and they also describe their opponents as fascists. The presence of contradictory positions surrounding the #Nocovid hashtag highlights the complexity and diversity of opinions within online discussions. The conflicting narratives are shown by their accompanying hashtags.
Another finding worth analyzing is the discourse shift over time within the use of #Nocovid, stressing the fluidity of online discussions and how they evolve with time and the change of circumstances. This adds an additional layer of analysis to what #Nocovid represents.
We qualitatively examine the user accounts with the highest PageRank score for the different network components. Within the first component, we observe only one user retweeting accounts such as SHomburg and prof_freedom, users who are clearly against the nocovid strategy. The account Birgit93Birgit had, at the time, an overall tweet count of 97,621 (active since December 2021 on X), a follower count of 672, and 131 other accounts that she follows. This is a hyperactive user who retweets around 400 tweets daily and has no account description. In summary, content-wise, the first component criticizes the implementation of this policy in China, citing coercive methods and fear propaganda. Critics argue that the nocovid strategy is unrealistic and ultimately destined to fail, as it does not account for the persistence of COVID-19, much like other endemic viruses. Discussions also reference Australia’s decision to abandon its COVID-19 restrictions and call for a restructuring of the Expert Council, which is perceived as being dominated by nocovid proponents. Overall, they conclude that nocovid remains a highly contested and potentially ineffective approach to pandemic management.
In the second component, however, the user with the highest PageRank score retweets content that supports the #nocovid strategy. For instance, the hashtags #YesToNocovid and #ZeroCovid accompanied the main hashtag #nocovid. The narrative described the strategy positions of nocovid as critical in combating potential situations, such as a return to settings without COVID-19 vaccines and medicines. The topic of education’s place in society and how to respond to criticism of the nocovid approach are also major points of discussion. The account Wunderwer had, at the time, an overall tweet count of 249,710 (since October 2011 active on X), 2,640 accounts they were following, and 1,620 followers. Their account description is: “GEGEN Durchseuchung von Kindern und Eltern, FÜR Aussetzen der Präsenzpflicht während der Pandemie”, which translates to: “AGAINST infection of children and parents, FOR suspension of compulsory attendance during the pandemic.”
The last prominent component contains 13 observations and 49 variables, mostly related to travel bots about COVID-19 and marketing campaigns related to India and tourism. Most tweets are retweets from “AmazonIndian2.” This bot has a follower count of 4,562 and is marketing travel luggage on Amazon.
In addition to the marketing bots, we identified automatization by third-party apps such as Twidere, Echofon, Tweetbot for iOS, and TweetCaster by using the source variable in the data. There were 113 automated sources in the first component that could be used to widely tweet and retweet content for the desired objectives. A total of 24 automated sources were in the second component. It is noteworthy that automated sources comprise an almost negligible portion of the total number of non-automated sources.
Social media network
Did coordination have an influence on the German discourse on X?
Coordinated tweets had no effect on non-coordinated behavior. We rule out that coordination makes news more viral. Figure 1 shows the rise of coordination within the nocovid dataset across a 5-min period for each form of coordination: Coretweet, Coreply, and Cohashtag. The percentage of tweets assigned to each kind of coordination in relation to the total number of tweets examined is shown on the y-axis. The graphs show how the proportion of tweets linked to each kind of coordination develops over time. The coretweet activity within nocovid remains consistently below 5%, from 2.5% to less than 5%, over the observed time intervals, which were 1 to 30 min. Coreply and cohashtag show diminishing percentages of coordination in relation to the total number of analyzed tweets, fewer than 0.5%.
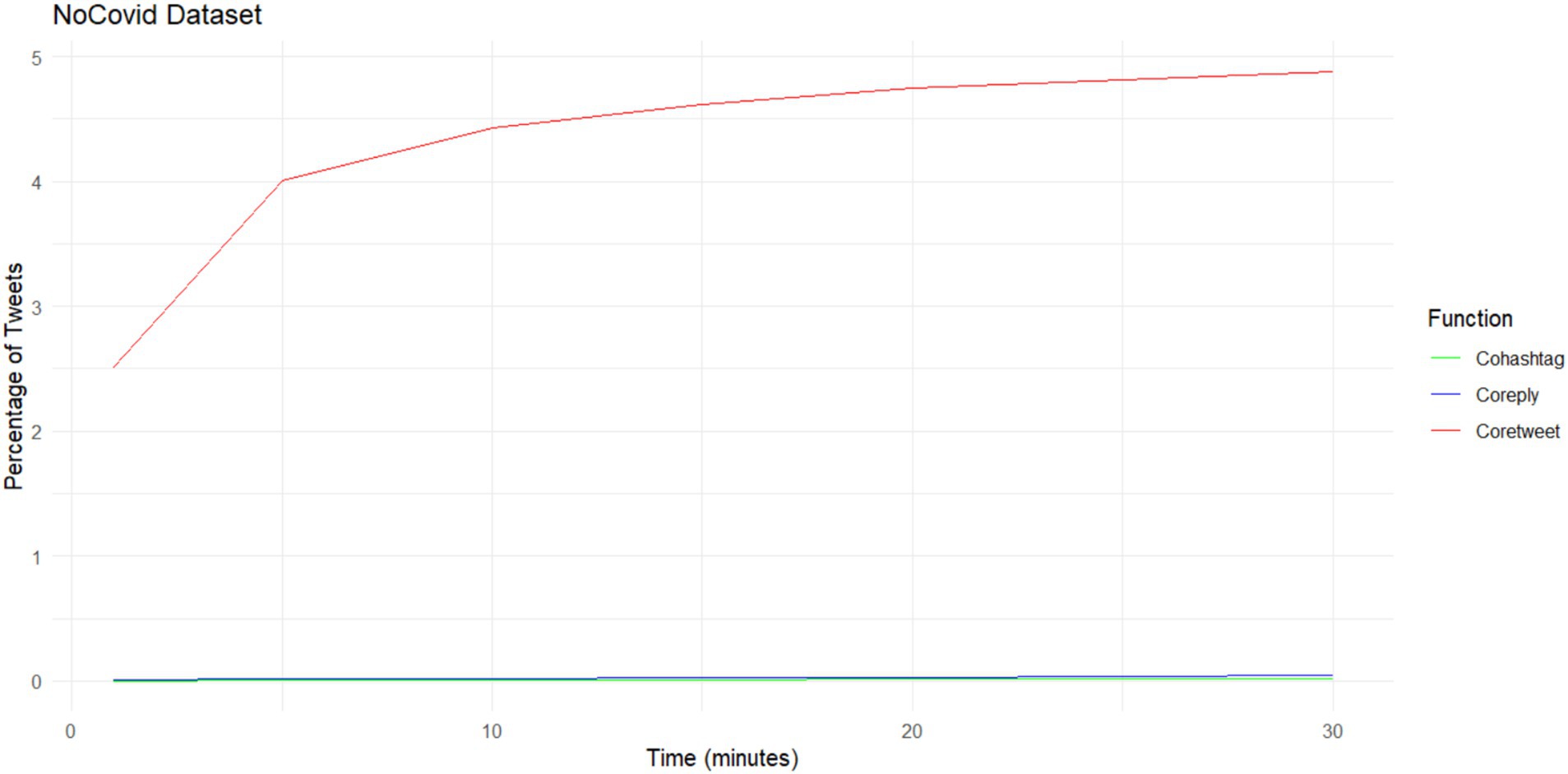
Figure 1. Percentage of coordinated tweets in each function. For each type of coordination—Coretweet, Coreply, and Cohashtag—the percentage of coordinated tweets relative to the total number of tweets analyzed is plotted across a 5-min period.
For the components within the network, the number of components for each of the functions is plotted against time (Figure 2). In the example of nocovid, the coretweet function shows fluctuating trends with a slight increase initially, followed by a decrease, then stabilization, peaking at 4 components and slightly declining thereafter. Coreply exhibits a rising trend from 3 to 10 components over time, whereas Cohashtag starts at 0 components (due to lack of coordination within the first minute), gradually increasing to 5. The fewer the components, the better the network’s cohesiveness, and therefore information flow can increase, with fewer separated islands of groups and stronger linkages and interactions.
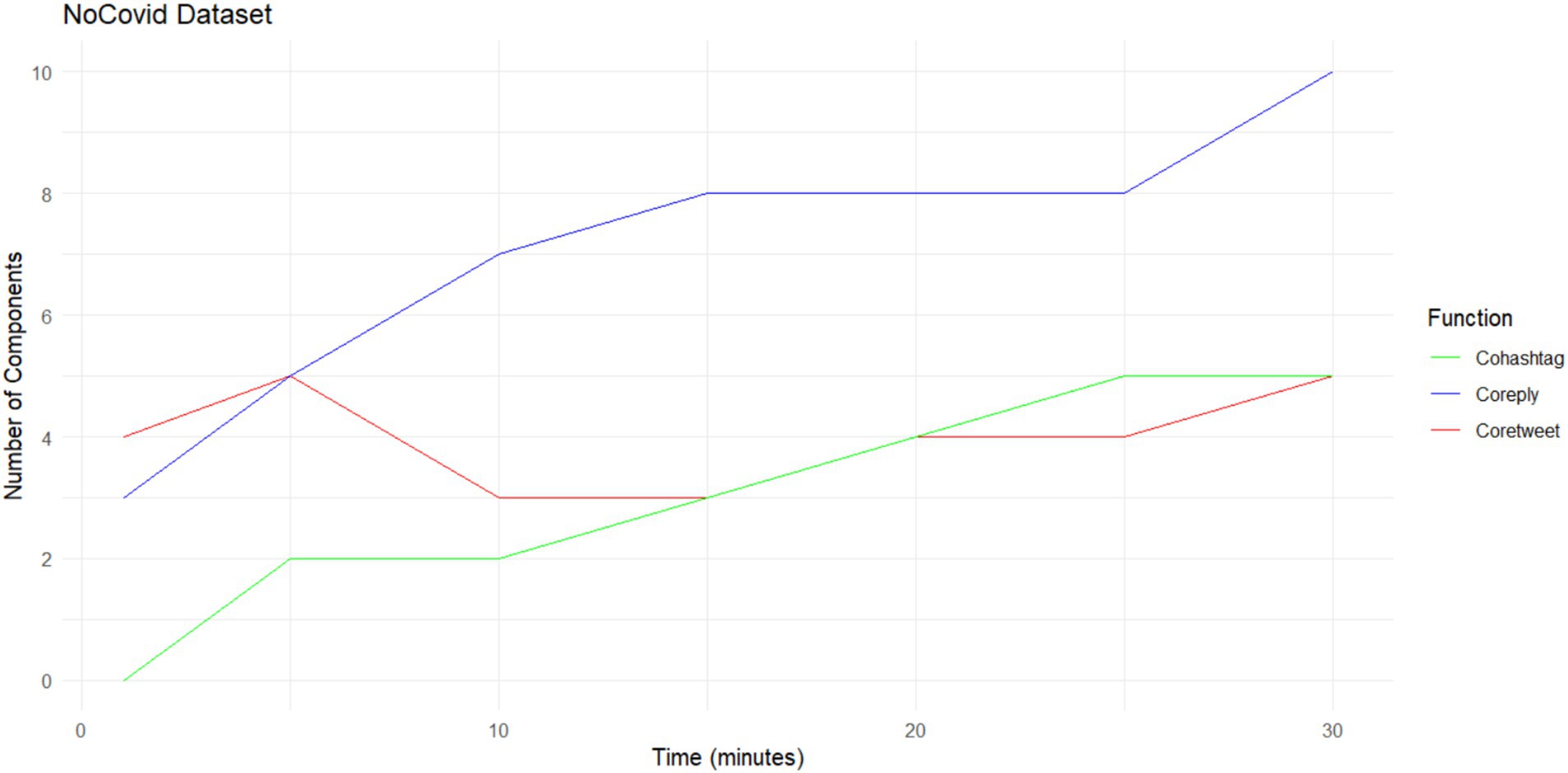
Figure 2. Number of network components in each function. The number of network components for each type of coordination—Coretweet, Coreply, and Cohashtag—is plotted against time.
However, the question remains: what influence does coordination have on the entire discussion, and does it drive non-coordination? In the following analysis, we focus on the function coretweet, as it is noticeably the most prominent coordination within nocovid. To answer the question above, first we generated timestamp data from two distinct time series, representing tweets classified as coordinated or non-coordinated, respectively. Converting these timestamps to POSIXct objects allowed for additional processing. We then rounded the timestamps to the nearest 5-min period to facilitate further research and aggregate tweet activity into distinct time bins. We then counted the number of tweets that appeared in each rounded timestamp bin. Two time series datasets were produced as a result, each of which showed the frequency of coordinated and non-coordinated activity tweets within 5-min intervals. We converted these datasets into zoo objects, a specific type of R data structure for managing time-series data, by using the “zoo” package. We then combined the two-time series into a single zoo object so that we could directly compare the temporal patterns of coordinated and non-coordinated tweet activity. We also generated detrended time series by differencing successive observations to identify underlying trends.
Finally, to evaluate the possible causative association between coordinated and non-coordinated X activity, we performed a Granger causality study (Granger, 1969; Shojaie and Fox, 2022). This approach makes it possible to understand the temporal dynamics and any interactions between these two types of tweet behavior.
This means that predicting non-coordinated tweets in the nocovid data cannot be improved by adding lagged coordination values beyond what can be obtained by simply considering the lagged values of the non-coordinated tweets (Figure 3). To determine if one time series (coordinated = true) helps predict another time series (coordinated = false), two models are compared using the Granger causality test. The first model uses the lags of the two variables (no coordination and with coordination) to predict the effect on the non-coordinated tweets. For the second model, no coordination lags are the sole variable used to predict non-coordination. To find out if adding the lags of coordinated tweets enhances the prediction of non-coordinated tweets, the test compares the fit of these two models. At 0.5905 and 0.4069, the p-value exceeds the conventional significance criterion of 0.05. Therefore, we are unable to reject the null hypothesis.
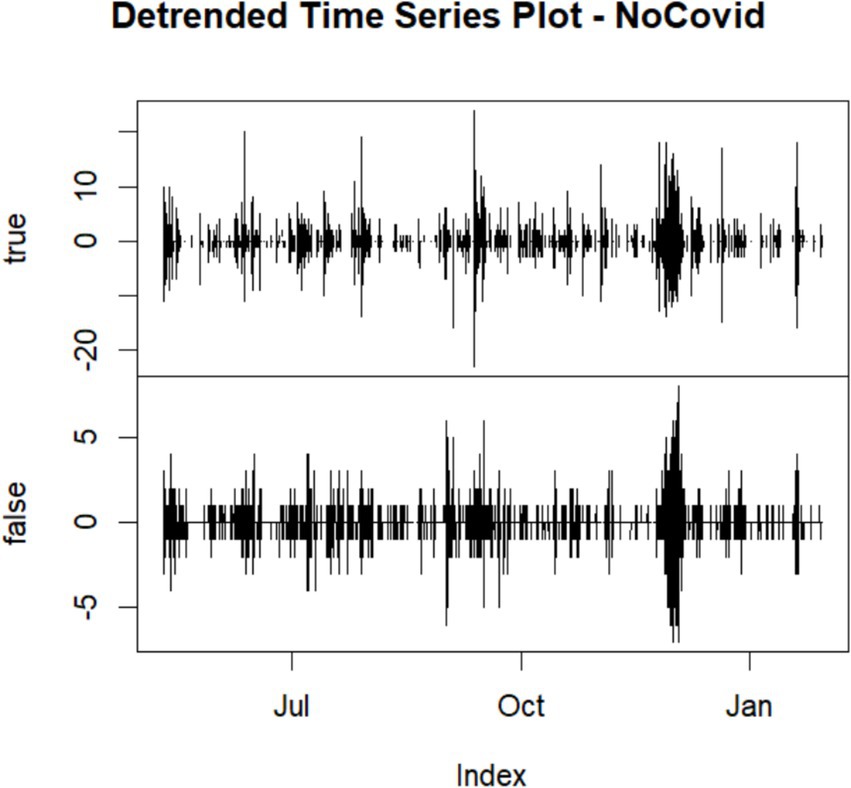
Figure 3. Granger causality analysis. We plot the granger causality analysis results to visualize if one time series (coordinated = True) helps predict another time series (coordinated = False).
Social media platform
Was there empirical evidence that the platform’s policy affected the discourse? There is clear evidence that platforms influence political discourse through decisions such as throttling outreach or shadow banning. We investigate the influence of social media platforms on content visibility and potential tweet suppression in different opinion clusters.
We can demonstrate that the non-coordinated tweets are unaffected by coordinated behavior itself. However, the platform affects the various clusters’ outreach in that the nocovid critical cluster has a clearly constricted reach. Only tweets that were retweeted as a result of coordinated behavior are taken into account. We split the number of followers of the users who posted the initial tweet by the frequency of retweets for each of these tweets, since users with higher follower counts in both clusters may receive higher visibility on the platform, contributing to higher engagement on their tweets (Figure 4). We call this variable the relation mean. We consider the number of retweets for every coordinated tweet, but we also account for the user’s follower base when calculating the weight of each tweet.
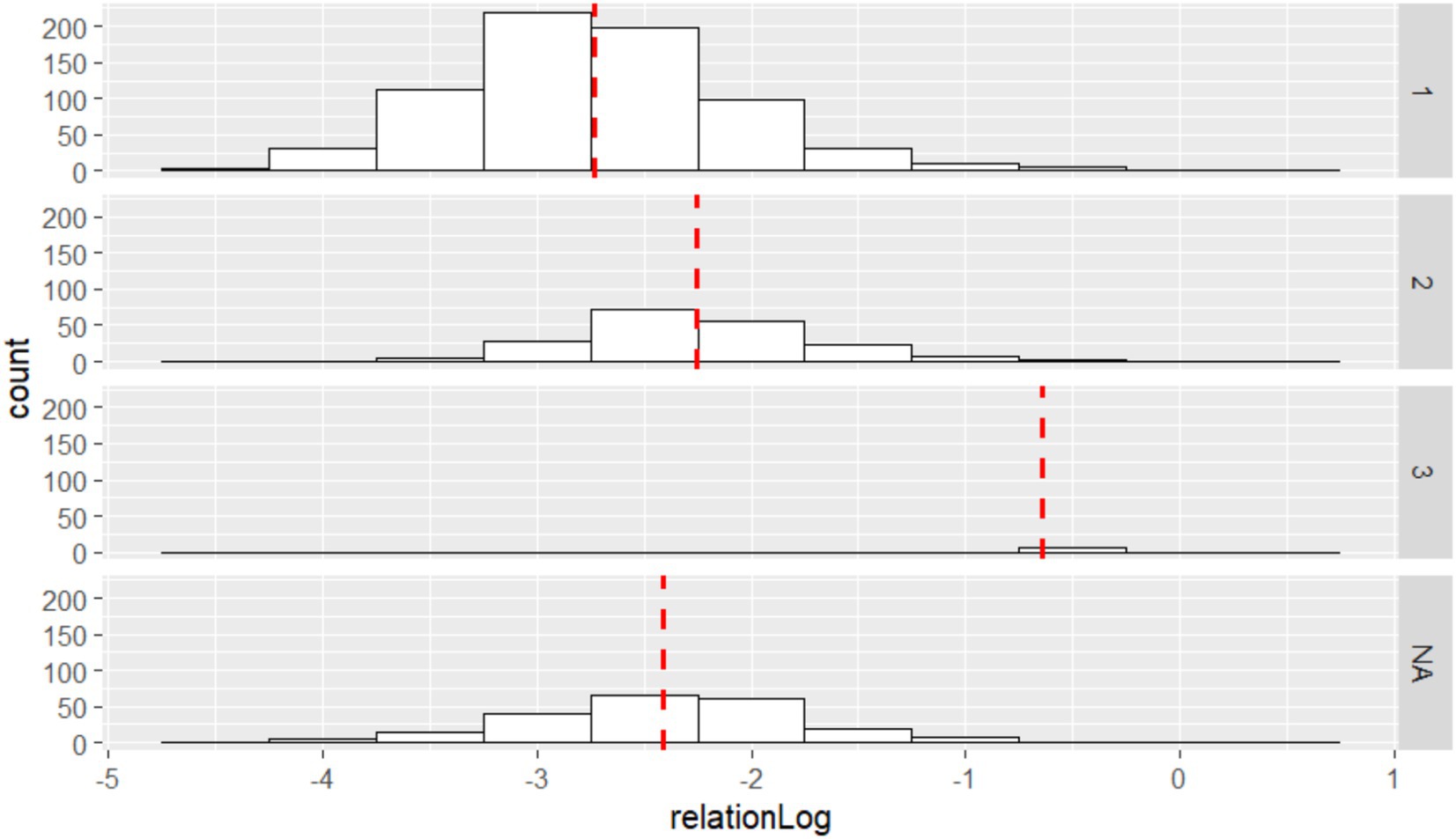
Figure 4. Opinion clusters and their outreach. The numbers on the right scale correspond to the different opinion clusters. Only tweets that were retweeted because of coordinated behavior are considered. We split the number of followers of the users who posted the initial tweet by the frequency of retweets for each of these tweets. We call this variable the relation mean, which we then log, for the purpose of visualization. We consider the number of retweets for every coordinated tweet, but we also account for the user’s follower base when calculating the weight of each tweet.
We have a comparison group of tweets that aren’t connected to any of these elements for comparison. In comparison to the control group, the pro-nocovid strategy cluster clearly benefits from the platform, but the anti-covid strategy cluster does not. The weighted follower mean value is represented by the red line. The X algorithm places a lot of weight on what they refer to as social proof, which is why followers are so crucial. A user’s likelihood of seeing another user’s tweets increases significantly if they follow them. For this reason, we weigh the follower count. Therefore, a user receives roughly two retweets for each 100 followers in the pro-nocovid bubble. Whereas, for every 100 followers, a user in the anti-nocovid cluster receives 0.5 retweets. In other words, component 1 yields a relation mean of 0.0098 retweets per follower, whereas component 2 yields twice that much (approx. 0.0188). Given that the marketing bots in component 3 follow and retweet one another, it makes sense that they score 0.24.
Clearly, what this demonstrates is that the platform handles tweets with varying content differently. Speaking up against nocovid results in fewer retweets than speaking out in support of the nocovid strategy. This can only be explained by the influence of the algorithms, as we weigh it based on the number of followers a user has, making it independent of the social network.
Discussion
Social media communication
Simple quantitative methods, such as counting hashtags, are insufficient for understanding public opinion on social media, particularly in the presence of coordinated inauthentic user behavior (CIUB). Hashtag usage does not necessarily reflect organic sentiment, as both proponents and opponents of a given position can employ the same hashtags to advance contrasting arguments. This is evident in the case of #nocovid, where coordination occurred on both sides of the debate, making it difficult to determine genuine public sentiment through traditional social media analysis. The presence of marketing bots further distorts the dataset, as some automated accounts are designed to boost unrelated content, such as commercial advertisements, without any thematic connection to the discourse. This highlights a fundamental limitation of computational social science methods that rely purely on statistical data analysis without semantic interpretation.
Another critical issue in computational social science is the assumption that network connections equate to influence. In reality, not all social media users actively engage in political discourse, and a small fraction of hyperactive users disproportionately shape conversations. Research by Papakyriakopoulos et al. (2020) indicates that approximately 5% of users generate 25% of political content on Facebook, while 10% of users are responsible for 80% of shared content. This uneven distribution suggests that social media networks do not represent a broad public dialogue but are instead dominated by highly engaged users who reinforce specific narratives. Without analyzing the coordination dynamics and discourse structures, merely counting hashtags or engagement metrics provides an incomplete picture of public opinion.
While coordinated user activity amplifies certain perspectives, its ability to persuade broader audiences remains uncertain. Our findings suggest that information does not diffuse freely; rather, it circulates within preexisting ideological clusters. This means that while CIUB can shape visibility and engagement, its influence on actual belief formation is more difficult to quantify. Thus, without a qualitative analysis of discourse, quantitative metrics alone are insufficient for understanding the real impact of coordinated behavior on social media.
Social media network
One of the most pressing questions in computational social science is whether CIUB actually influences public opinion or simply reinforces existing divisions. While numerous studies have documented the prevalence of coordinated networks (Righetti, 2025), it remains unclear whether these efforts expand their reach beyond their existing base or primarily serve as amplification mechanisms within pre-existing ideological groups. Our research suggests that, despite high levels of coordination within the #nocovid debate, there was no significant evidence that coordinated tweets triggered increased engagement from non-coordinated users. The Granger causality test did not establish a causal link between CIUB and a subsequent rise in non-coordinated discourse. This challenges the assumption that coordinated disinformation necessarily expands beyond its originating network to shape broader public opinion.
However, this does not mean that CIUB has no impact. While we did not find evidence of direct persuasion or large-scale organic spread, CIUB likely contributes to polarization by reinforcing existing beliefs within ideological echo chambers (Shahrezaye et al., 2019). Additionally, from a theoretical standpoint, coordinated activity plays a role in acclamation dynamics—where social media users signal belonging to a political or ideological group rather than engaging in genuine deliberation (Hegelich et al., 2023). This is particularly relevant in right-wing populist movements, where coordinated networks serve as tools for identity reinforcement rather than for broad persuasion.
Another key finding is that not all coordination is politically or socially meaningful. Our analysis identified a third cluster in the coordinated network consisting of marketing bots promoting Amazon travel products and luggage. These bots had no thematic connection to the COVID-19 discourse, illustrating that some instances of coordination are driven by commercial motives rather than political influence. This reinforces the need for careful differentiation between politically relevant CIUB and other forms of coordinated activity that may distort engagement metrics but lack substantive impact on public discourse.
Social media platform
Our results provide strong evidence that platform policies influence political discourse by determining which content gains visibility. The discrepancy in outreach between different opinion clusters suggests that X’s content moderation strategies play an active role in shaping the spread of information. While algorithmic interventions such as shadow banning, de-ranking, and misinformation tagging are intended to curb the spread of false or harmful content, they also raise concerns about potential biases in content suppression.
X employs several algorithmic mechanisms that influence content visibility. One of the most significant is the “Social Proof” filter, which ensures that users primarily see content engaged with by others in their network (Hegelich et al., 2023). This means that dominant narratives within a user’s network are likely to be reinforced, while contrarian viewpoints may struggle to gain traction. While this filtering mechanism helps mitigate the spread of misinformation, it also has the unintended effect of limiting the reach of minority perspectives, including those critical of dominant public health policies such as nocovid strategies.
In the case of the #nocovid debate, our findings indicate that tweets opposing nocovid policies received fewer retweets per follower compared to those supporting them. While algorithmic suppression may be one contributing factor, it is essential to consider alternative explanations such as differences in engagement behavior, media framing, and audience size. Our study does not provide definitive proof that platform bias was the sole cause of this discrepancy, but it highlights a clear pattern that warrants further investigation.
Beyond algorithmic interventions, state regulations also influence content moderation practices. Germany’s Network Enforcement Act (NetzDG) mandates that platforms remove flagged content within 24 h or face financial penalties (Gorwa, 2021). While this legislation is intended to combat hate speech and disinformation, critics argue that it may lead to over-censorship of legitimate political discourse. This raises a broader question: Do platform moderation policies apply evenly across different political perspectives, or do they disproportionately affect certain viewpoints?
The debate over shadow banning further complicates this issue. While some argue that platforms use de-ranking mechanisms to silence dissenting opinions, companies such as X and Meta defend these practices as necessary to maintain information integrity. Le Merrer et al. (2021) suggest that shadow banning functions primarily as an algorithmic process to limit the spread of potentially harmful or misleading content, rather than as an intentional political suppression tool. However, as Savolainen (2022) highlights, much of the controversy surrounding shadow banning stems from perceived rather than proven suppression. The opacity of platform algorithms fosters what she terms “algorithmic folklore,” where users attribute content suppression to deliberate bias, even in cases where de-ranking is due to broader engagement patterns or automated moderation rules. This ambiguity raises critical concerns about governance and trust, as user perceptions of bias can be just as influential as actual moderation policies in shaping digital discourse.
Data availability statement
The raw data supporting the conclusions of this article will be made available by the authors, without undue reservation.
Ethics statement
Ethical approval was not required for the study involving human data in accordance with the local legislation and institutional requirements. Written informed consent was not required, for either participation in the study or for the publication of potentially/indirectly identifying information, in accordance with the local legislation and institutional requirements. The social media data was accessed and analyzed in accordance with the platform’s terms of use and all relevant institutional/national regulations.
Author contributions
HS: Conceptualization, Data curation, Formal analysis, Funding acquisition, Investigation, Methodology, Project administration, Resources, Software, Supervision, Validation, Visualization, Writing – original draft, Writing – review & editing. SH: Conceptualization, Funding acquisition, Project administration, Supervision, Writing – review & editing.
Funding
The author(s) declare that financial support was received for the research and/or publication of this article. This work was supported by Federal Ministry of Education and Research (BMBF)—NEOVEX: 13N16050.
Conflict of interest
The authors declare that the research was conducted in the absence of any commercial or financial relationships that could be construed as a potential conflict of interest.
Generative AI statement
The authors declare that no Gen AI was used in the creation of this manuscript.
Publisher’s note
All claims expressed in this article are solely those of the authors and do not necessarily represent those of their affiliated organizations, or those of the publisher, the editors and the reviewers. Any product that may be evaluated in this article, or claim that may be made by its manufacturer, is not guaranteed or endorsed by the publisher.
References
Ahmed, W., Vidal-Alaball, J., Downing, J., and Seguí, F. L. (2020). COVID-19 and the 5G conspiracy theory: social network analysis of X data. J. Med. Internet Res. 22:e19458. doi: 10.2196/19458
Aïmeur, E., Amri, S., and Brassard, G. (2023). Fake news, disinformation and misinformation in social media: a review. Soc. Netw. Anal. Min. 13:30. doi: 10.1007/s13278-023-01028-5
Al-Zaman, M. S. (2021). COVID-19-related social media fake news in India. Journalism and Media, 2, 100–114. doi: 10.3390/journalmedia2010007
Alizadeh, M., Shapiro, J. N., Buntain, C., and Tucker, J. A. (2020). Content-based features predict social media influence operations. Sci. Adv. 6:eabb5824. doi: 10.1126/sciadv.abb5824
Allcott, H., Gentzkow, M., and Yu, C. (2019). Trends in the diffusion of misinformation on social media. Research and Politics, 6, 1–8. doi: 10.1177/2053168019848554
Assenmacher, D., Adam, L., Trautmann, H., and Grimme, C. (2020). Towards real-time and unsupervised campaign detection in social media. Proceedings of the thirty-third international Florida artificial intelligence research society conference (FLAIRS 2020).
Badawy, A., and Ferrara, E. (2018). The rise of jihadist propaganda on social networks. J. Comput. Soc. Sci. 1, 453–470. doi: 10.1007/s42001-018-0015-z
Bode, L., and Vraga, E. K. (2018). See something, say something: correction of global health misinformation on social media. Health Commun. 33, 1131–1140. doi: 10.1080/10410236.2017.1331312
Box-Steffensmeier, J. M., and Moses, L. (2021). Meaningful messaging: sentiment in elite social media communication with the public on the COVID-19 pandemic. Sci. Adv. 7:eabg2898. doi: 10.1126/sciadv.abg2898
Dhawan, S., Hegelich, S., Sindermann, C., and Montag, C. (2022). Re-start social media, but how? Telemat. Inform. Rep. 8:100017. doi: 10.1016/j.teler.2022.100017
Gabarron, E., Oyeyemi, S. O., and Wynn, R. (2021). COVID-19-related misinformation on social media: a systematic review. Bull. World Health Organ. 99, 455–463A. doi: 10.2471/BLT.20.276782
Giglietto, F., Righetti, N., and Marino, G. (2019). Understanding coordinated and inauthentic link sharing behavior on Facebook in the run-up to 2018 general election and 2019 European election in Italy. doi: 10.31235/osf.io/3jteh
Giglietto, F., Righetti, N., and Marino, G. (2020a). Detecting coordinated link sharing behavior on Facebook during the Italian coronavirus outbreak. Paper presented at AoIR 2020: The 21st annual conference of the Association of Internet Researchers. Virtual Event: AoIR. Available online at: http://spir.aoir.org
Giglietto, F., Righetti, N., and Marino, G. (2020b). It takes a village to manipulate the media: coordinated link sharing behavior during 2018 and 2019 Italian elections. Inf. Commun. Soc. 23, 867–891. doi: 10.1080/1369118X.2020.1739732
Gisondi, M. A., Barber, R., Faust, J. S., Raja, A., Strehlow, M. C., Westafer, L. M., et al. (2022). A deadly infodemic: social media and the power of COVID-19 misinformation. Journal of medical Internet research, 24:e35552. doi: 10.2196/35552
Gorwa, R. (2021). Elections, institutions, and the regulatory politics of platform governance: the case of the German NetzDG. Telecommun. Policy 45:102145. doi: 10.1016/j.telpol.2021.102145
Graham, T., Bruns, A., Zhu, G., and Campbell, R. (2020). Like a virus: The coordinated spread of coronavirus disinformation.
Granger, C. W. (1969). Investigating causal relations by econometric models and cross-spectral methods. Econometrica 37, 424–438. doi: 10.2307/1912791
Hegelich, S., Dhawan, S., and Sarhan, H. (2023). Twitter as political acclamation. Front. Pol. Sci. 5:1150501. doi: 10.3389/fpos.2023.1150501
Hegelich, S., and Shahrezaye, M. (2015). The communication behavior of German MPs on X: preaching to the converted and attacking opponents. Eur. Policy Anal. 1, 155–174. doi: 10.18278/epa.1.2.8
Howard, P. N., and Kollanyi, B. (2016). Bots, #StrongerIn, and #Brexit: computational propaganda during the UK-EU referendum. International Journal of Communication, 10, 5032–5055. doi: 10.3030/648311
Huszár, F., Ktena, S. I., O’Brien, C., Belli, L., Schlaikjer, A., and Hardt, M. (2022). Algorithmic amplification of politics on X. Proc. Natl. Acad. Sci. 119:e2025334119. doi: 10.1073/pnas.2025334119
Keller, F. B., Schoenebeck, S. Y., and Pater, J. A. (2019). How coordinated campaigns steer collective attention: the case of the ALS ice bucket challenge. Proceedings of the ACM on human-computer interaction, 3(CSCW), 1–21.
Lazer, D. M., Baum, M. A., Benkler, Y., Berinsky, A. J., Greenhill, K. M., Menczer, F., et al. (2018). The science of fake news. Science 359, 1094–1096. doi: 10.1126/science.aao2998
Le Merrer, E., Morgan, B., and Trédan, G. (2021) Setting the record straighter on shadow banning. In IEEE INFOCOM 2021-IEEE conference on computer communications (pp. 1–10). IEEE.
Lee, E. W., Bao, H., Wang, Y., and Lim, Y. T. (2023). From pandemic to Plandemic: examining the amplification and attenuation of COVID-19 misinformation on social media. Soc. Sci. Med. 328:115979. doi: 10.1016/j.socscimed.2023.115979
Luceri, L., Cardoso, F., and Giordano, S. (2020). Down the bot hole: actionable insights from a 1-year analysis of bots activity on X. arXiv preprint arXiv: 2010.15820.
Marwick, A. E. (2017). Media manipulation and disinformation online. Data and Society Research Institute. Available at: https://datasociety.net/pubs/oh/DataAndSociety_MediaManipulationAndDisinformationOnline.pdf
Montag, C., and Hegelich, S. (2020). Understanding detrimental aspects of social media use: will the real culprits please stand up? Front. Sociol. 5:599270. doi: 10.3389/fsoc.2020.599270
Netzpolitik.org. (2024). Vorläufiges Ergebnis: X/X verstößt gegen EU-Regeln für Plattformen. Available online at: https://netzpolitik.org/2024/vorlaeufiges-ergebnis-x-X-verstoesst-gegen-eu-regeln-fuer-plattformen/
Pacheco, D., Hui, P. M., Torres-Lugo, C., Truong, B. T., Flammini, A., and Menczer, F. (2021). Uncovering coordinated networks on social media: methods and case studies. In proceedings of the international AAAI conference on web and social media (Vol. 15, pp. 455–466).
Papakyriakopoulos, O., Serrano, J. C. M., and Hegelich, S. (2020). Political communication on social media: a tale of hyperactive users and bias in recommender systems. Online Soc. Netw. Media 15:100058. doi: 10.1016/j.osnem.2019.100058
Pfeffer, J., Mayer, K., and Morstatter, F. (2018). Tampering with X’s sample API. EPJ Data Sci. 7:50. doi: 10.1140/epjds/s13688-018-0178-0
Righetti, N. (2025). The multiple nuances of online firestorms: the case of a pro-Vietnam attack on the Facebook digital embassy of China in Italy amidst the pandemic. Ital. Sociol. Rev. 15:1. doi: 10.13136/isr.v15i1.850
Saurwein, F., and Spencer-Smith, C. (2020). Combating disinformation on social media: Multilevel governance and distributed accountability in Europe. Digital Journalism, 8, 820–841. doi: 10.1080/21670811.2020.1765401
Savolainen, L. (2022). The shadow banning controversy: perceived governance and algorithmic folklore. Media Cult. Soc. 44, 1091–1109. doi: 10.1177/01634437221077174
Shahrezaye, M., Meckel, M., and Hegelich, S. (2020). Estimating the political orientation of Twitter users using network embedding algorithms. J. Appl. Bus. Econ. 22, 53–62. doi: 10.33423/jabe.v22i14.3965
Shahrezaye, M., Papakyriakopoulos, O., Serrano, J. C. M., and Hegelich, S. (2019). Measuring the ease of communication in bipartite social endorsement networks: a proxy to study the dynamics of political polarization. In proceedings of the 10th international conference on social media and society (pp. 158–165).
Shin, J., Jian, L., Driscoll, K., and Bar, F. (2018). The diffusion of misinformation on social media: temporal pattern, message, and source. Comput. Hum. Behav. 83, 278–287. doi: 10.1016/j.chb.2018.02.008
Shojaie, A., and Fox, E. B. (2022). Granger causality: a review and recent advances. Ann. Rev. Stat. Appl. 9, 289–319. doi: 10.1146/annurev-statistics-040120-010930
Tandoc, E. C., Lim, D., and Ling, R. (2020). Diffusion of disinformation: how social media users respond to fake news and why. Journalism 21, 381–398. doi: 10.1177/1464884919868325
Tsao, S. F., Chen, H., Tisseverasinghe, T., Yang, Y., Li, L., and Butt, Z. A. (2021). What social media told us in the time of COVID-19: a scoping review. Lancet Digit. Health 3, e175–e194. doi: 10.1016/S2589-7500(20)30315-0
Wang, T., Lu, K., Chow, K. P., and Zhu, Q. (2020). COVID-19 sensing: negative sentiment analysis on social media in China via BERT model. IEEE Access 8, 138162–138169. doi: 10.1109/ACCESS.2020.3012595
Weber, D., and Neumann, F. (2021). Amplifying influence through coordinated behavior in social networks. Soc. Netw. Anal. Min. 11:111. doi: 10.1007/s13278-021-00815-2
Keywords: coordinated inauthentic behavior, social media network (SMN), social media communication, social media platform, disinformation
Citation: Sarhan H and Hegelich S (2025) There was coordinated inauthentic user behavior in the COVID-19 German X-discourse, but did it really matter? Front. Commun. 10:1510144. doi: 10.3389/fcomm.2025.1510144
Edited by:
Pablo Santaolalla Rueda, International University of La Rioja, SpainReviewed by:
Francisco José Murcia Verdú, University of Castilla-La Mancha, SpainWill Grant, Australian National University, Australia
Copyright © 2025 Sarhan and Hegelich. This is an open-access article distributed under the terms of the Creative Commons Attribution License (CC BY). The use, distribution or reproduction in other forums is permitted, provided the original author(s) and the copyright owner(s) are credited and that the original publication in this journal is cited, in accordance with accepted academic practice. No use, distribution or reproduction is permitted which does not comply with these terms.
*Correspondence: Habiba Sarhan, aGFiaWJhLnNhcmhhbkB0dW0uZGU=