- 1Department of Community and Family Medicine, All India Institute of Medical Sciences, Deoghar (AIIMS Deoghar), Deoghar, India
- 2Faculty of Naturopathy and Yogic Sciences, SGT University, Gurugram, India
- 3Department of Community Medicine, Faculty of Medicine and Health Sciences, SGT University, Gurugram, India
Introduction: The COVID-19 pandemic triggered not only a public health crisis but also a parallel “infodemic”—an overwhelming flood of information, including false or misleading content. This phenomenon created confusion, mistrust, and hindered public health efforts globally. Understanding the dynamics of this infodemic is essential for improving future crisis communication and misinformation management.
Methods: This systematic review followed PRISMA 2020 guidelines. A comprehensive search was conducted across PubMed, Scopus, Web of Science, and Google Scholar for studies published between December 2019 and December 2024. Studies were included based on predefined criteria focusing on COVID-19-related misinformation causes, spread, impacts, and mitigation strategies. Data were extracted, thematically coded, and synthesized. The quality of studies was assessed using the AMSTAR 2 tool.
Results: Seventy-six eligible studies were analyzed. Key themes identified included the amplification of misinformation via digital platforms, especially social media; psychological drivers such as cognitive biases and emotional appeals; and the role of echo chambers in sustaining false narratives. Consequences included reduced adherence to public health measures, increased vaccine hesitancy, and erosion of trust in healthcare systems. Interventions like fact-checking, digital literacy programs, AI-based moderation, and trusted messengers showed varied effectiveness, with cultural and contextual factors influencing outcomes.
Discussion: The review highlights that no single strategy suffices to address misinformation. Effective mitigation requires a multi layered approach involving reactive (fact-checking), proactive (digital literacy, community engagement), and structural (policy and algorithm transparency) interventions. The review also underscores the importance of interdisciplinary collaboration and adaptive policies tailored to specific sociocultural settings.
Introduction
The COVID-19 pandemic not only posed a significant challenge to global healthcare systems but also gave rise to an unprecedented surge in misinformation, termed an “infodemic.” (Clemente-Suárez et al., 2022). This phenomenon, characterized by an overabundance of information—both accurate and false—created confusion, fear, and mistrust among the public (Infodemic, 2020). This study explores the factors driving COVID-19 misinformation, its spread via social media, its impact on public health, the effectiveness of mitigation efforts, and strategies to enhance resilience against misinformation in future health crises. Misinformation spreads through a structured process with multiple stages. Each stage influences how false information gains attention and persists.The spread begins with a source. This could be an individual, a social media post, or a coordinated disinformation campaign. The source may include manipulated content, misinterpretations, or deliberate fabrications. These false narratives are often designed to shape public opinion. Once misinformation is introduced, amplification occurs. Social media algorithms and human behavior contribute to its rapid spread. Emotionally charged misinformation spreads faster than factual content. This happens because social media platforms prioritize engagement and virality over accuracy. As misinformation spreads, it enters echo chambers. In these closed networks, people mainly interact with like-minded individuals. This limits their exposure to corrective information. As a result, misinformation becomes more persistent and harder to correct.To counter misinformation, intervention points are necessary. Real-time fact-checking, algorithm adjustments, and trusted messengers can help correct falsehoods. Healthcare professionals and community leaders play an important role in spreading accurate information. Digital literacy programs also help individuals assess the credibility of online content.The impact of misinformation depends on the effectiveness of interventions. If unchecked, false narratives continue to spread. However, timely corrections can increase public awareness and reduce the spread of misinformation. Understanding these processes helps in developing better strategies for preventing misinformation (Figure 1).Misinformation prevention involves multiple layers of intervention. The first layer focuses on reactive measures. Fact-checking and content moderation help counter misinformation after it has spread. Organizations verify claims, and AI systems flag or remove misleading content. However, these methods often face delays. People who already believe misinformation may also resist corrections. The second layer includes proactive strategies. These aim to stop misinformation before it spreads widely. Digital literacy programs teach people to evaluate information critically. Trusted messengers, like healthcare experts, help spread accurate information. The success of these strategies depends on cultural factors and people’s pre-existing beliefs. The third layer addresses structural solutions. This includes government regulations and AI-driven moderation. Some governments have introduced laws to hold social media platforms accountable. AI systems are also used to detect misinformation early. However, these measures must balance misinformation control with concerns about censorship and algorithmic biases. Regulations also vary across different countries. The final goal is to reduce misinformation and strengthen public resilience. Combining reactive, proactive, and structural interventions creates a more effective and sustainable response. Continuous research, policy improvements, and collaboration across different sectors are necessary. This will help address the evolving challenges of misinformation (Figure 2). The spread of misinformation during the pandemic created many challenges for public health. It made existing problems worse and reduced people’s willingness to follow preventive measures. As a result, governments and healthcare organizations struggle to provide accurate and timely information. This added pressure made it harder to manage the crisis effectively (Kisa and Kisa, 2024). The novelty of this study is that it takes an interdisciplinary approach by combining psychology, technology, and policy. It goes beyond public health-focused research by using a structured framework to analyze misinformation. Unlike previous studies, it highlights algorithmic transparency and content amplification. It also explores advanced solutions like AI-based detection and blockchain verification. These insights offer new ways to manage misinformation effectively. Addressing this infodemic became a crucial priority to mitigate its impact on the pandemic response and recovery (Ferreira Caceres et al., 2022; Bhattacharya et al., 2021; Rodrigues et al., 2024). Historically, misinformation has been a recurring challenge during health crises (Kisa and Kisa, 2024; Rodrigues et al., 2024). For instance, during the 1918 influenza pandemic, unfounded claims about cures and conspiracy theories about the origins of the virus proliferated through newspapers and word of mouth (Barry, 2004). Similarly, the Ebola outbreaks in West Africa saw widespread myths about the disease, leading to harmful practices like avoiding healthcare facilities (WHO, 2020b; Muzembo et al., 2022; Buseh et al., 2015). However, the COVID-19 infodemic was unique in its scale and intensity, driven by the global reach of digital platforms and the unprecedented speed of information dissemination (Pulido et al., 2020; WHO, 2020a). Understanding this phenomenon is critical for addressing future infodemics in an increasingly interconnected world.
Aim
The aim of this systematic review is to synthesize the existing body of literature on the infodemic during the COVID-19 pandemic, with a focus on identifying its causes, manifestations, and implications, as well as the strategies employed to combat it.
Methodology
The review adheres to the Preferred Reporting Items for Systematic Reviews and Meta-Analyses 2020 and the Quality of Reporting of Meta-analyses statement (Page et al., 2021). We explored the following research questions: 1. What were the key psychological, technological, and societal factors contributing to the spread of misinformation during the COVID-19 pandemic? 2. How did social media algorithms influence the amplification and dissemination of COVID-19 misinformation? 3. What were the public health consequences of misinformation on vaccine hesitancy and adherence to preventive measures? 4. How effective were fact-checking initiatives, regulatory measures, and public education campaigns in mitigating misinformation? 5. What strategies can improve public resilience against misinformation in future health crises?
Inclusion and exclusion criteria
The search terms were oriented according to the Population, Intervention, Comparison and Results (PICOS) approach, as shown in Table 1. Studies published between December 2019 and the present were included to ensure that the research focused on misinformation during the COVID-19 pandemic. Language: Only studies published in English were considered due to accessibility and consistency in analysis. Peer-reviewed journal articles, conference papers, and reputable preprints were included to ensure academic rigour. Studies specifically examining misinformation related to COVID-19, including its sources, spread mechanisms, psychological and social impacts, and mitigation strategies, were included. Studies published before December 2019 was excluded as they do not pertain to COVID-19 misinformation. Non-English studies were excluded due to language barriers and potential translation inconsistencies. Opinion pieces, editorials, blog posts, and non-peer-reviewed sources were excluded to maintain academic reliability. Studies that addressed misinformation in general but did not specifically focus on COVID-19 were excluded from the review.
Search methods
We designed the search strategy with an information specialist using medical subject headings and specific keywords (Table 2). We included articles published in English from December 2019 to December 2024, focusing on the infodemic during the COVID-19 pandemic. Non-English papers were excluded due to language bias, resource constraints for translation, limited accessibility, and inconsistent quality control across languages, which may affect the reproducibility and comparability of findings. We searched four databases (PubMed, Scopus, Web of Science, and Google Scholar) and explored the included studies reference lists. Potential limitations of including only these four databases can include database, exclusion of grey literature, publication bias and indexing limitations. Boolean operators like AND is used to narrow a search by including all specified terms, ensuring that results contain each keyword and OR is used to broaden a search by retrieving results that contain at least one of the specified terms. We first conducted the search on 4 December 2024, and we re-ran the search on 6 Jan 2024. After removing duplicates, two authors independently screened the title, abstract and full text of articles and included eligible articles for evaluation. An independent third author resolved any disagreements. We performed the screening process in Gurugram (Gurugram, Haryana, India).
Data collection and analysis
Two independent researchers extracted the general characteristics of each study and classified them into seven major themes: 1. The Role of Digital Platforms in Amplifying Misinformation, 2. Interventions to Combat the Infodemic. 3. The Role of Trusted Messengers 4. Proactive Regulation of Digital Platforms, 5.Enhancing Health and Media Literacy, 6.Bridging the Digital Divide, 7.Technological Innovations in Misinformation Management. We clustered articles based on similar properties associated with the stated objective and the reported outcomes. Although infodemics were primarily defined as the overabundance of information, usually with a negative connotation, we decided to report data from articles that also described the potential beneficial effects of the massive circulation of information and knowledge during health emergencies. We summarised the challenges and opportunities associated with infodemics and misinformation. A third author verified the retrieved data, and another author resolved any disagreements between the inter-reviewers.
Assessment of methodological quality
Two authors independently appraised the quality of the included articles using the AMSTAR 2 tool, which consists of 16 domains (Shea et al., 2017). Both reviewers conducted the screening and data extraction independently and in a blinded manner to minimize bias. Each categorical domain was rated using an online platform, and an overall assessment of critical and non-critical domains was obtained. Any inter-rater discrepancies were initially resolved through discussion, and if consensus could not be reached, a third reviewer was consulted for arbitration.
Data extraction
Data extraction followed a clear and structured process, with records initially identified through database searches and additional sources such as hand-searching and references. The PRISMA Flow Diagram outlined the screening process, beginning with 495 records identified through database searches, with an additional 127 duplicate records from other sources. After removing duplicates, 495 records were screened for relevance based on title and abstract. Of these, 310 were excluded because 216 were found to be irrelevant to the research topic, 48 were excluded due to language and accessibility barriers, and 46 lacked empirical data. Following this step, 185 reports were sought for retrieval, but 27 could not be accessed, leaving 158 reports for eligibility assessment.
At the eligibility stage, a detailed evaluation of the 158 reports was conducted, leading to the exclusion of 82 reports. These were removed due to inappropriate study design (Ahmed et al., 2020), lack of an integrated study (Islam et al., 2020), or lack of relevance to the research objectives (Guess et al., 2020). After this rigorous selection process, 76 studies met the inclusion criteria and were incorporated into the final review. The diagram visually represents the systematic approach used in study selection, ensuring transparency and reproducibility in the research process (Figure 3).
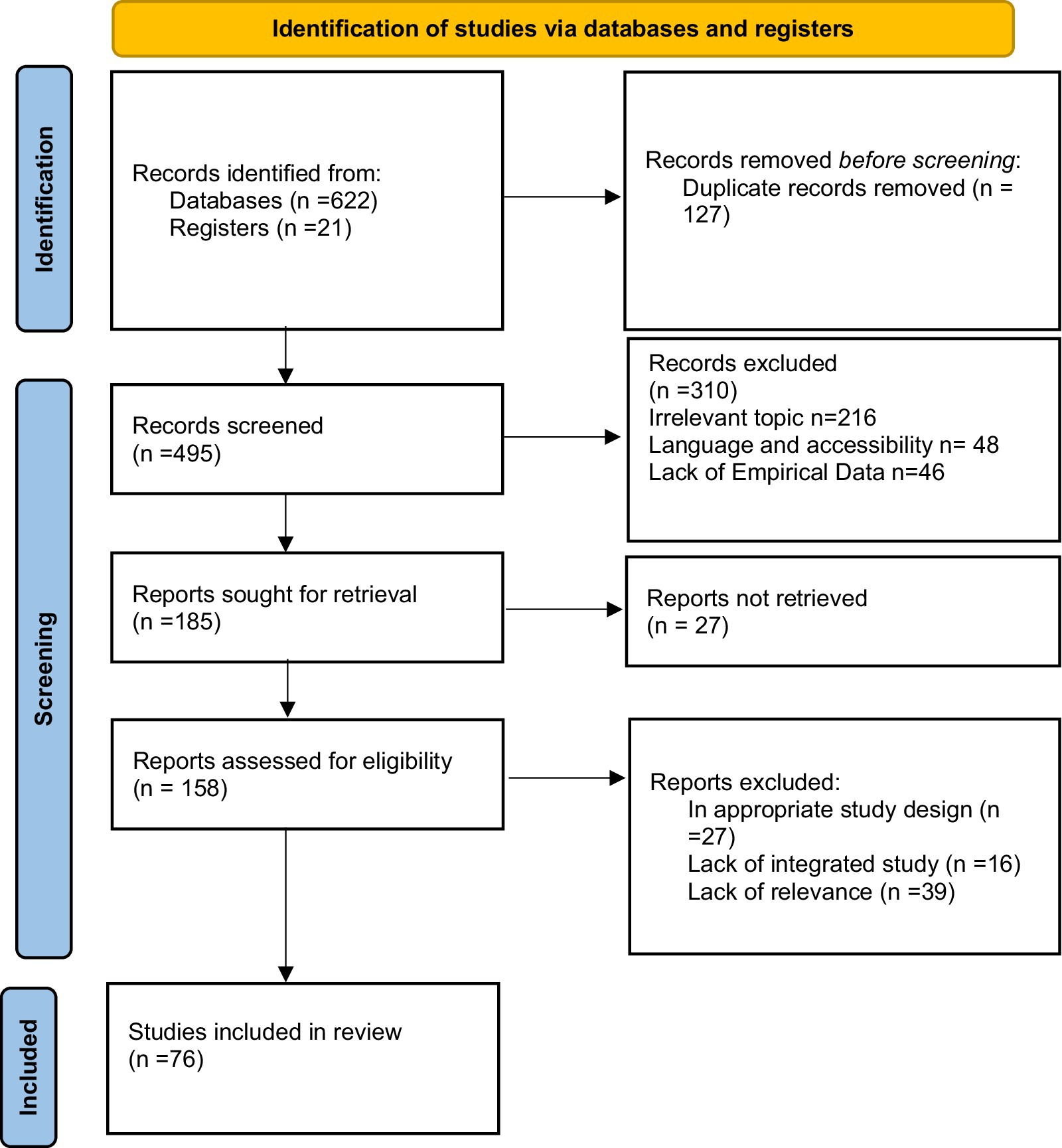
Figure 3. PRISMA 2020 flow diagram for new systematic reviews which included searches of databases and registers only.
Data synthesis
A narrative synthesis approach was used to categorize studies into key themes: misinformation spread, impact, and mitigation. Findings were analyzed thematically, integrating qualitative insights and quantitative summaries. Contradictory results were examined for methodological or contextual variations. Intervention effectiveness—fact-checking, media literacy, and regulations—was compared across studies. Insights were mapped to existing misinformation frameworks, providing a comprehensive understanding of its dynamics and implications.
Results
The results of this review revealed that the COVID-19 infodemic manifested through various channels, including social media platforms, traditional news outlets, and interpersonal communication (Kisa and Kisa, 2024; Pulido et al., 2020). Social media emerged as a dominant vector for the dissemination of misinformation, with platforms such as Facebook, Twitter, and YouTube playing pivotal roles. For instance, Joseph et al. analyzed millions of posts across platforms and highlighted that misinformation on COVID-19 was shared at a rate comparable to factual information, often reaching large audiences due to algorithmic amplification (Joseph et al., 2022).
Common themes of misinformation included the origins of the virus, prevention and treatment measures, vaccine safety and efficacy, and conspiracy theories. For example, Islam et al. (2020) identified over 2,300 rumours, stigma, and conspiracy theories circulating across 87 countries, with a significant proportion related to unverified treatments such as ingesting disinfectants or using herbal remedies (Islam et al., 2020). This misinformation not only fuelled public confusion but also led to direct harm; a study by Aghababaeian et al. reported over 700 deaths and thousands of hospitalizations in Iran due to methanol poisoning linked to false beliefs about its protective effects against COVID-19 (Aghababaeian et al., 2020).
Empirical studies also highlighted the adverse effects of the infodemic on public health outcomes. Ferreira Caceres et al. found that exposure to COVID-19 misinformation significantly reduced adherence to preventive measures such as mask-wearing and social distancing (Ferreira Caceres et al., 2022). Health misinformation significantly erodes trust between patients and healthcare professionals, leading to scepticism about medical advice. This distrust negatively impacts patient adherence to treatments and public health measures (Kbaier et al., 2024). Health misinformation significantly undermines public trust in credible health sources due to insufficient health and digital literacy among users, which is exacerbated by socio-economic disparities. A study explored the long-term impact of an Israeli government digital literacy program for disadvantaged populations, as perceived by participants 1 year after course completion. Interviews conducted a year later revealed that participants primarily joined the program out of cognitive interest, particularly to learn internet applications, followed by career aspirations. Reported benefits included increased knowledge, greater confidence in using technology, empowerment, and improved self-efficacy. However, participants noted that without ongoing practice or instructor support, much of the acquired knowledge diminished over time, affecting the program’s lasting impact (Lev-On et al., 2020). Additionally, cultural contexts influence the reception of misinformation, making certain demographics more vulnerable (Ismail et al., 2022). Similarly, a survey by Pertwee et al. revealed that vaccine hesitancy increased in populations frequently exposed to anti-vaccine narratives online, with specific claims about microchip implantation and infertility driving mistrust in vaccine campaigns (Pertwee et al., 2022). The infodemic disproportionately affected vulnerable populations. For example, literacy barriers were evident in communities where access to credible sources of information was limited. A study conducted by Gaysynsky et al. demonstrated that individuals with lower health literacy were more likely to believe and share misinformation, exacerbating disparities in health outcomes (Gaysynsky et al., 2024). Vulnerable populations, particularly those in rural areas or low-income settings, were also found to be more susceptible to believing in conspiracy theories due to limited access to verified information sources (Kisa and Kisa, 2024) Quantitative analyses underscored the scale of the problem. Li et al. (2020) found that 25% of COVID-19-related YouTube videos contained misleading information, collectively amassing over 62 million views (Li et al., 2020). A similar study by Gallotti et al. (2020) reported that up to 40% of COVID-19-related tweets contained misinformation, often driven by bots and coordinated campaigns. Specific case studies, such as the “Pandemic” documentary, exemplify how misinformation campaigns gained traction and sowed widespread skepticism regarding public health interventions (Gallotti et al., 2020). Furthermore, Jon Agley and Yunyu Xiao identified a strong correlation between the virality of misinformation and public mistrust in health authorities, further complicating the pandemic response (Agley and Xiao, 2021). Localized examples also illustrate the impact of the infodemic. In India, misinformation about cow urine as a COVID-19 cure gained significant traction, leading to health risks and public confusion. Similarly, in the United States, conspiracy theories about 5G technology causing COVID-19 resulted in vandalism of telecommunications infrastructure, as documented by Ahmed et al. (2020).
Despite widespread misinformation, certain mitigation strategies showed effectiveness. Collaborative efforts between governments and social media companies to flag or remove false information were reported to reduce the virality of some narratives (Nature, 2021). A case study on Facebook’s partnership with fact-checking organizations demonstrated that labelling posts as misleading reduced their engagement rates by up to 80% (Aïmeur et al., 2023). However, these efforts often lagged behind the rapid spread of misinformation, highlighting the need for proactive measures.
Additionally, campaigns focusing on increasing health literacy emerged as pivotal. Studies by Paul Machete & Marita Turpin revealed that public awareness programs emphasizing critical thinking and source verification significantly reduced the likelihood of individuals sharing false information (Machete and Turpin, 2020). Similarly, tailored interventions targeting specific myths—such as WHO’s “MythBusters” initiative—proved effective in debunking common misconceptions, particularly when culturally contextualized messages were employed. A study by Birunda et al. proposed Automatic COVID-19 misinformation detection (ACOVMD) in Twitter using a self-trained semi-supervised hybrid deep learning model. The experimental results show that the proposed model achieves 80.92% accuracy and 98.15% accuracy in the 10 and 80% label-seen experiments, respectively (Birunda et al., 2024). A study by Lu et al. embraced uncertainty features within the information environment. It introduced a novel Environmental Uncertainty Perception (EUP) framework for detecting misinformation and predicting its spread on social media, which showed that the EUP alone achieved notably good performance, with detection accuracy at 0.753 and prediction accuracy at 0.71. This study makes a significant contribution to the literature by recognizing uncertainty features within information environments as a crucial factor for improving misinformation detection and spread-prediction algorithms during the pandemic (Lu et al., 2024). A study by Zhao et al. proposed a novel health misinformation detection model was which incorporated the central-level features (including topic features) and the peripheral-level features (including linguistic features, sentiment features, and user behavioral features). The model correctly detected about 85% of the health misinformation (Zhao et al., 2021).
Discussion
Infodemics spread and impact on public health
The COVID-19 infodemic, a term describing the rapid and widespread dissemination of misinformation and disinformation during the pandemic, is a complex issue influenced by societal, technological, and psychological factors (WHO, 2020a). While digital platforms played a pivotal role in amplifying misinformation, the effectiveness of interventions to mitigate its impact remains debatable. A critical analysis of the mechanisms driving misinformation spread, the limitations of current strategies, and areas requiring further research is necessary to formulate a more comprehensive response.The amplification of misinformation on digital platforms can be attributed to algorithmic biases that prioritize engagement over accuracy. Studies by Cinelli et al. (2020) and Vosoughi et al. (2018) suggest that emotionally charged misinformation spreads more rapidly than factual content (Cinelli et al., 2020; Vosoughi et al., 2018). However, these studies primarily focus on Western social media landscapes, raising concerns about their generalizability to regions with different digital ecosystems and media consumption patterns. Additionally, while social media platforms have introduced measures to curb misinformation, such as fact-checking partnerships, the effectiveness of these interventions is inconsistent.
Effectiveness of different mitigation strategies
While AI can rapidly identify disinformation campaigns, its reliance on pattern recognition increases the likelihood of false positives, particularly when distinguishing between satire and harmful misinformation (Pennycook and Rand, 2022). This contrasts with human fact-checking efforts, which, though slower, provide nuanced contextual understanding. The interplay between these approaches remains a contentious debate, with some scholars arguing for a hybrid model that combines AI efficiency with human oversight to balance speed and accuracy (Zhang et al., 2023). Others highlight the susceptibility of AI systems to adversarial manipulation, where misinformation creators adapt content to evade automated detection, raising concerns about long-term sustainability. Meanwhile, human fact-checking, despite its strengths in contextual analysis, faces challenges related to scalability and biases introduced by individual or institutional perspectives. The debate between AI-driven and human-led approaches underscores the need for a more integrated strategy that considers the strengths and weaknesses of both methodologies. For instance, Pennycook and Rand (2021) found that flagged misinformation was less likely to be shared, yet Guess et al. (2020) demonstrated that such efforts had minimal impact on users entrenched in misinformation echo chambers (Pennycook and Rand, 2021; Guess et al., 2020).
Additionally, fact-checking is not always effective in changing the beliefs of individuals deeply embedded in misinformation echo chambers. Echo chambers, where people are repeatedly exposed to like-minded opinions and selective information, reinforce pre-existing biases and make individuals resistant to correction, even when presented with credible evidence. Psychological factors play a key role in this resistance (WHO, 2020b). Confirmation bias leads people to seek, interpret, and remember information in ways that align with their existing beliefs while dismissing contradictory facts. The backfire effect can also occur, where direct confrontation with fact-checks strengthens rather than weakens false beliefs. Emotional investment in misinformation, particularly when linked to identity or ideology, further reinforces resistance to correction (Pennycook and Rand, 2022). Technological factors also contribute to this challenge. Social media algorithms prioritize engagement, often amplifying misleading content and reinforcing belief systems within closed networks. When fact-checks appear in such environments, they may be rejected outright or perceived as biased attacks, especially if they come from sources that individual’s distrust. Research suggests that while fact-checking remains a valuable strategy, it must be combined with other approaches for greater impact. Media literacy programs can help people critically evaluate information before they form rigid beliefs. Narrative-based corrections, where misinformation is debunked through storytelling rather than direct contradiction, have shown promise in overcoming resistance. Encouraging open dialogue and trust-building within communities may also help reduce misinformation’s grip (Kbaier et al., 2024; Cinelli et al., 2020). This contradiction suggests that fact-checking alone is insufficient, particularly when cognitive biases and ideological predispositions influence information consumption.
Another major intervention—trusted messengers—has shown promise in countering misinformation, yet its success is contingent on cultural and contextual factors. Research by MacKay et al. highlights the credibility of healthcare professionals and community leaders in disseminating accurate information (MacKay et al., 2022). However, the assumption that trust in these figures translates to behavioral change is problematic. A case study from India by Sundaram et al. demonstrated that community health workers effectively addressed vaccine hesitancy through direct engagement (Sundaram et al., 2023). Nevertheless, this approach may not be scalable in urban or digitally interconnected populations, where misinformation circulates rapidly and personal interactions are limited (Journal of Primary Care Specialties, 2021). Furthermore, there is insufficient research on whether trust in experts extends to digital platforms, where misinformation thrives.
Regulatory measures targeting digital platforms have also been proposed to curb the infodemic, yet their implementation remains contentious. Germany’s NetzDG law mandates the removal of illegal content within 24 h, a model cited as effective in reducing hate speech (Library of Congress, 2021; Human Rights Watch, 2018a). However, concerns about censorship and freedom of expression complicate its adoption on a global scale. Furthermore, the lack of transparency in how platforms determine what constitutes misinformation raises ethical and practical dilemmas. A standardized, international approach to content moderation is necessary, yet the feasibility of such a framework remains uncertain given the divergent regulatory environments across countries (Trengove et al., 2022).
The contradiction between the effectiveness of digital literacy campaigns and the persistence of misinformation-related beliefs presents a critical challenge in misinformation research. Some studies advocate for digital literacy as a key intervention, arguing that training individuals to critically evaluate information sources reduces their susceptibility to false claims (Finland Toolbox, 2024). Programs like Finland’s media education initiative have been highlighted as promising models that integrate critical thinking into curricula, fostering long-term resilience against misinformation. However, other studies suggest that belief persistence—where individuals cling to preexisting views despite corrective information—undermines the impact of such initiatives. Cognitive biases, such as the backfire effect and motivated reasoning, may lead people to reject or reinterpret corrective messages in ways that reinforce their existing beliefs. This discrepancy raises questions about whether digital literacy efforts can significantly alter misinformation consumption patterns or whether they merely benefit those already inclined toward critical engagement. Furthermore, there is a need to examine how digital literacy interventions interact with different sociocultural and psychological factors, as well as their scalability in diverse populations. Addressing these contradictions requires a more nuanced approach that accounts for cognitive resistance to factual corrections and the broader social dynamics of misinformation spread.
Furthermore, inconsistencies in research findings highlight the need for adaptive policy frameworks. Policymakers must consider variations in audience responses, the influence of social media algorithms, and the trustworthiness of fact-checking organizations when designing interventions. A rigid, one-size-fits-all approach may fail to address the complexity of misinformation spread. Instead, policies should be evidence-driven and flexible, incorporating ongoing research to refine strategies over time.
Artificial intelligence models have shown promise in identifying disinformation campaigns. However, their reliance on pattern recognition can lead to challenges, such as distinguishing between satire and harmful misinformation. For instance, a study revealed that existing fake news detectors are more likely to flag AI-generated content as false, while often misclassifying human-written fake news as genuine, indicating a bias in detection mechanisms (Ghiurău and Popescu, 2025). The advent of generative AI technologies has facilitated the creation of deepfakes—highly realistic but fabricated content—which poses significant threats to information integrity. These AI-generated media have been implicated in spreading false information across various domains, including politics and health, complicating efforts to maintain information accuracy (Sunil et al., 2025). Automated content moderation systems, while efficient, can inadvertently perpetuate biases present in their training data. This can lead to unjust outcomes, such as the disproportionate removal of content from certain groups. Therefore, incorporating human oversight is crucial to mitigate these biases and ensure fair content moderation practices.
Despite the various strategies employed to combat the COVID-19 infodemic, significant gaps remain in understanding the psychological mechanisms that drive misinformation adoption and resistance to correction. Future research should prioritize comparative studies that examine the effectiveness of interventions across different sociocultural contexts. Additionally, longitudinal studies assessing the durability of fact-checking, media literacy programs, and regulatory measures would provide deeper insights into sustainable solutions. Regulatory measures targeting digital platforms have also been proposed to curb the infodemic, yet their implementation remains contentious. Germany’s NetzDG law mandates the removal of illegal content within 24 h, a model cited as effective in reducing hate speech. However, concerns about censorship and freedom of expression complicate its adoption on a global scale.
Here is a comparative table (Table 3) of different misinformation countermeasures, detailing their effectiveness and limitations (Table 4).
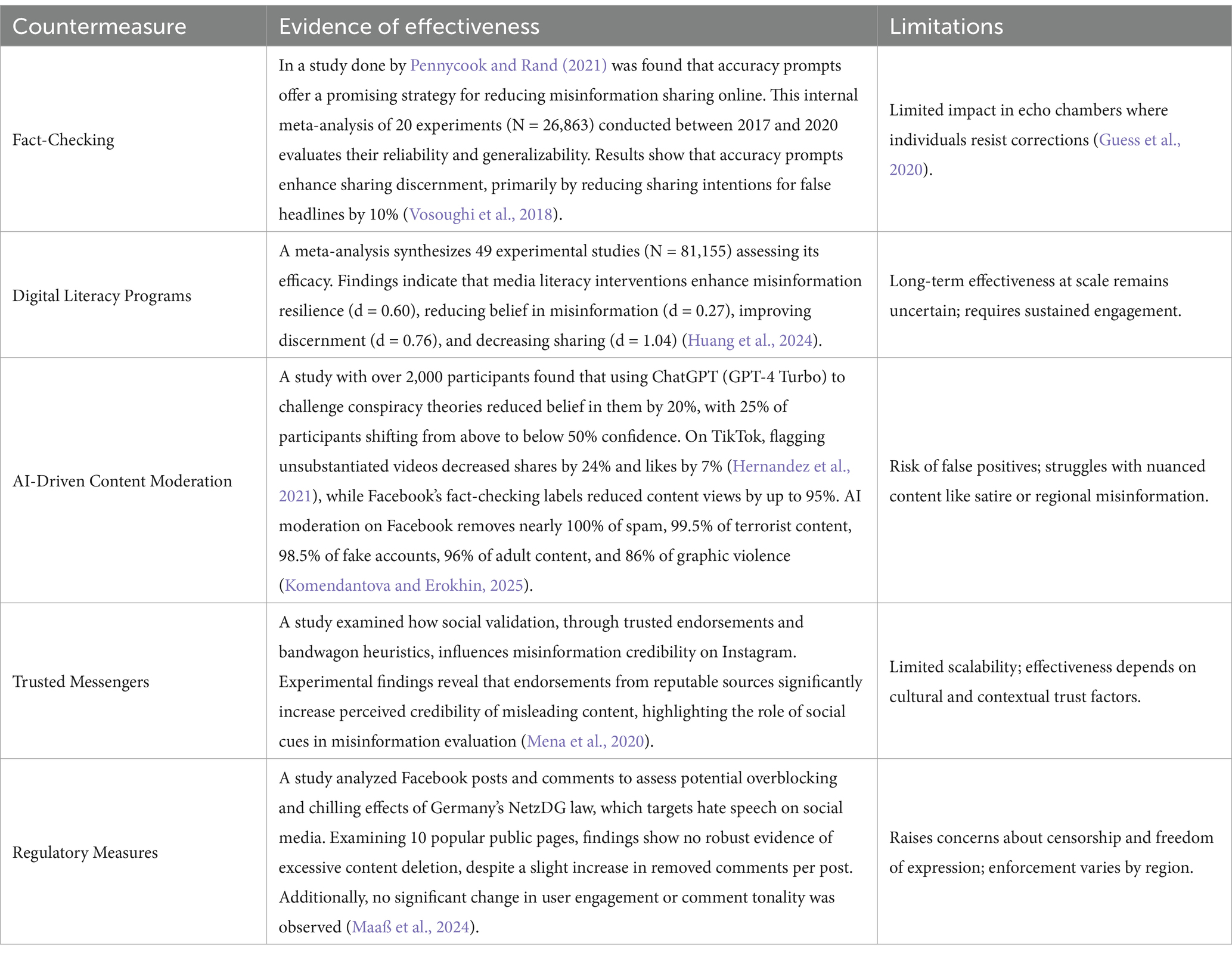
Table 3. Comparative table of different misinformation countermeasures, detailing their effectiveness and limitations.
Strategies to enhance resilience against infodemics in future health crisis
Recommendations for future research
To effectively address the challenges posed by infodemics, future research must focus on a multi-faceted approach that combines technological, behavioral, social, and policy-driven strategies (Rodrigues et al., 2024; WHO, 2020b; Briand et al., 2021). Given the complex nature of misinformation and its far-reaching consequences, particularly in times of crises like the COVID-19 pandemic, it is essential to identify and explore key areas of intervention. The following areas are crucial to the ongoing effort to combat misinformation, each addressing different dimensions of the issue:
Algorithm transparency
Research into algorithm transparency should aim at developing frameworks for ethical algorithm design. This could involve making algorithmic processes more understandable and accessible to the public, ensuring that platforms are held accountable for the content they promote. Platform-driven interventions, such as X’s Community Notes and Facebook’s misinformation labels, aim to curb misinformation through algorithmic adjustments and user-driven corrections (Tan, 2022; Yu et al., 2024; Dujeancourt and Garz, 2023).
Behavioural insights
Understanding the psychological factors behind the belief and sharing of misinformation is essential for designing interventions that target the root causes of these behaviors. Psychological theories of belief formation, cognitive biases, and social influence can provide crucial insights into why people are so easily influenced by misinformation. For instance, cognitive biases such as confirmation bias, where individuals seek out information that confirms their pre-existing beliefs, play a critical role in the spread of falsehoods. Emotional responses to misinformation, such as fear or anger, also contribute to its virality, as these emotions increase engagement with content (Pennycook and Rand, 2021; Munusamy et al., 2024).
Global collaboration
Misinformation is not bound by borders, and its effects are global. The widespread nature of misinformation, especially on platforms like Facebook, Twitter, and YouTube, means that disinformation can easily cross geographical, political, and cultural boundaries. As a result, tackling the infodemic requires global cooperation and coordination among researchers, policymakers, and technology companies. Future research should focus on fostering international partnerships to share data, research findings, and best practices in combating misinformation (Adams et al., 2023; Desai et al., 2022; New WHO Review Finds, 2022).
Community-based strategies
While global and national interventions are crucial, community-based strategies are also vital for combating misinformation, especially in regions with limited access to digital literacy resources. Misinformation spreads rapidly in local communities through word-of-mouth, local media, and interpersonal interactions. Therefore, it is essential to evaluate the efficacy of grassroots efforts in building trust and promoting accurate information within communities (Oxford Academic, 2022; Borges Do Nascimento et al., 2022; Stover et al., 2024).
Policy impact
Regulatory and policy measures have been among the most widely discussed approaches to tackling misinformation (Tan, 2022). However, the effectiveness of these measures remains a subject of debate. Some countries have implemented stringent laws aimed at curbing the spread of false information. For example, Singapore’s Protection from Online Falsehoods and Manipulation Act (POFMA) empowers authorities to issue correction orders to platforms and individuals found spreading falsehoods. While such measures have been successful in curbing some forms of misinformation, they have also raised concerns about censorship and the suppression of dissenting voices (Human Rights Watch, 2018b; Protection from Online Falsehoods and Manipulation Act, 2021; Nannini et al., 2024).
However, the future research avenues discussed above may face feasibility challenges. Finally, by assessing strategies to enhance public resilience against misinformation, this research highlights the importance of digital literacy, institutional collaboration, and proactive policy frameworks. These findings provide a foundation for developing robust misinformation mitigation strategies applicable to future health crises, reinforcing the necessity of a multidisciplinary approach in addressing digital misinformation.
Data availability statement
The original contributions presented in the study are included in the article/supplementary material, further inquiries can be directed to the corresponding author.
Author contributions
SB: Writing – original draft, Writing – review & editing. AS: Writing – original draft, Writing – review & editing.
Funding
The author(s) declare that no financial support was received for the research and/or publication of this article.
Conflict of interest
The authors declare that the research was conducted in the absence of any commercial or financial relationships that could be construed as a potential conflict of interest.
Generative AI statement
The author(s) declare that Gen AI was used in the creation of this manuscript. Generative AI was used. The author(s) verify and take full responsibility for the use of generative AI in the preparation of this manuscript.
Publisher’s note
All claims expressed in this article are solely those of the authors and do not necessarily represent those of their affiliated organizations, or those of the publisher, the editors and the reviewers. Any product that may be evaluated in this article, or claim that may be made by its manufacturer, is not guaranteed or endorsed by the publisher.
References
Adams, Z., Osman, M., Bechlivanidis, C., and Meder, B. (2023). (why) is misinformation a problem? Perspect. Psychol. Sci. 18, 1436–1463. doi: 10.1177/17456916221141344
Aghababaeian, H., Hamdanieh, L., and Ostadtaghizadeh, A. (2020). Alcohol intake in an attempt to fight COVID-19: a medical myth in Iran. Alcohol Fayettev N. 88, 29–32. doi: 10.1016/j.alcohol.2020.07.006
Agley, J., and Xiao, Y. (2021). Misinformation about COVID-19: evidence for differential latent profiles and a strong association with trust in science. BMC Public Health 21:89. doi: 10.1186/s12889-020-10103-x
Ahmed, W., Vidal-Alaball, J., Downing, J., and López Seguí, F. (2020). COVID-19 and the 5G conspiracy theory: social network analysis of twitter data. J. Med. Internet Res. 22:e19458. doi: 10.2196/19458
Aïmeur, E., Amri, S., and Brassard, G. (2023). Fake news, disinformation and misinformation in social media: a review. Soc. Netw. Anal. Min. 13:30. doi: 10.1007/s13278-023-01028-5
Barry, J. M. (2004). The site of origin of the 1918 influenza pandemic and its public health implications. J. Transl. Med. 2:3. doi: 10.1186/1479-5876-2-3
Bhattacharya, S., Saleem, S. M., and Singh, A. (2021). COVID-19 vaccine: A battle with the uncertainties and infodemics. J. Primary Care Specialties. 2, 21–3.
Birunda, S. S., Devi, R. K., Muthukannan, M., and Babu, M. M. (2024). ACOVMD: Automatic COVID‐19 misinformation detection in Twitter using self‐trained semi‐supervised hybrid deep learning model. Int. Soc. Sci. J. 74, 713–30. doi: 10.1111/issj.124754
Borges Do Nascimento, I. J., Pizarro, A. B., Almeida, J. M., Azzopardi-Muscat, N., Gonçalves, M. A., Björklund, M., et al. (2022). Infodemics and health misinformation: a systematic review of reviews. Bull. World Health Organ. 100, 544–561. doi: 10.2471/BLT.21.287654
Briand, S. C., Cinelli, M., Nguyen, T., Lewis, R., Prybylski, D., Valensise, C. M., et al. (2021). Infodemics: a new challenge for public health. Cell 184, 6010–6014. doi: 10.1016/j.cell.2021.10.031
Buseh, A. G., Stevens, P. E., Bromberg, M., and Kelber, S. T. (2015). The Ebola epidemic in West Africa: challenges, opportunities, and policy priority areas. Nurs. Outlook 63, 30–40. doi: 10.1016/j.outlook.2014.12.013
Cinelli, M., Quattrociocchi, W., Galeazzi, A., Valensise, C. M., Brugnoli, E., Schmidt, A. L., et al. (2020). The COVID-19 social media Infodemic. Sci. Rep. 10:16598. doi: 10.1038/s41598-020-73510-5
Clemente-Suárez, V. J., Navarro-Jiménez, E., Simón-Sanjurjo, J. A., Beltran-Velasco, A. I., Laborde-Cárdenas, C. C., Benitez-Agudelo, J. C., et al. (2022). Mis–dis information in COVID-19 health crisis: a narrative review. Int. J. Environ. Res. Public Health 19:5321. doi: 10.3390/ijerph19095321
Desai, A. N., Ruidera, D., Steinbrink, J. M., Granwehr, B., and Lee, D. H. (2022). Misinformation and disinformation: the potential disadvantages of social Media in Infectious Disease and how to combat them. Clin. Infect. Dis. 74, e34–e39. doi: 10.1093/cid/ciac109
Dujeancourt, E., and Garz, M. (2023). The effects of algorithmic content selection on user engagement with news on twitter. Inf. Soc. 39, 263–281. doi: 10.1080/01972243.2023.2230471
Ferreira Caceres, M. M., Sosa, J. P., Lawrence, J. A., Sestacovschi, C., Tidd-Johnson, A., MHU, R., et al. (2022). The impact of misinformation on the COVID-19 pandemic. AIMS Public Health 9, 262–277. doi: 10.3934/publichealth.2022018
Finland Toolbox (2024). Taruutriainen. Media literacy and education in Finland. Available online at: https://toolbox.finland.fi/life-society/media-literacy-and-education-in-finland/ (Accessed December 22, 2024).
Gallotti, R., Valle, F., Castaldo, N., Sacco, P., and De Domenico, M. (2020). Assessing the risks of ‘infodemics’ in response to COVID-19 epidemics. Nat. Hum. Behav. 4, 1285–1293. doi: 10.1038/s41562-020-00994-6
Gaysynsky, A., Senft Everson, N., Heley, K., and Chou, W. Y. S. (2024). Perceptions of Health Misinformation on Social Media: Cross-Sectional Survey Study. JMIR Infodemiol. 4:e51127. doi: 10.2196/51127
Ghiurău, D., and Popescu, D. E. (2025). Distinguishing reality from AI: approaches for detecting synthetic content. Computer 14:1. doi: 10.3390/computers14010001
Guess, A. M., Lerner, M., Lyons, B., Montgomery, J. M., Nyhan, B., Reifler, J., et al. (2020). A digital media literacy intervention increases discernment between mainstream and false news in the United States and India. Proc. Natl. Acad. Sci. USA 117, 15536–15545. doi: 10.1073/pnas.1920498117
Hernandez, R. G., Hagen, L., Walker, K., O’Leary, H., and Lengacher, C. (2021). The COVID-19 vaccine social media infodemic: healthcare providers’ missed dose in addressing misinformation and vaccine hesitancy. Hum Vaccin Immunother. 17, 2962–2964. doi: 10.1080/21645515.2021.1912551 [Epub April 23, 2021].
Huang, G., Jia, W., and Yu, W. (2024). Media literacy interventions improve resilience to misinformation: a meta-analytic investigation of overall effect and moderating factors. Commun. Res. 4:00936502241288103. doi: 10.1177/00936502241288103
Human Rights Watch. (2018a). Germany: Flawed Social Media Law. Available online at: https://www.hrw.org/news/2018/02/14/germany-flawed-social-media-law (Accessed December 22, 2024).
Human Rights Watch (2018b). Singapore: ‘Fake News’ Law Curtails Speech. Available online at: https://www.hrw.org/news/2021/01/13/singapore-fake-news-law-curtails-speech (Accessed December 22, 2024).
Infodemic (2020). Available online at: https://www.who.int/health-topics/infodemic#tab=tab_1 (Accessed March 7, 2025).
Islam, M. S., Sarkar, T., Khan, S. H., Mostofa Kamal, A. H., Hasan, S. M. M., Kabir, A., et al. (2020). COVID-19–related Infodemic and its impact on public health: a global social media analysis. Am. J. Trop. Med. Hyg. 103, 1621–1629. doi: 10.4269/ajtmh.20-0812
Ismail, N., Kbaier, D., Farrell, T., and Kane, A. (2022). The experience of health professionals with misinformation and its impact on their job practice: qualitative interview study. JMIR Form Res. 6:e38794. doi: 10.2196/38794
Joseph, A. M., Fernandez, V., Kritzman, S., Eaddy, I., Cook, O. M., Lambros, S., et al. (2022). COVID-19 misinformation on social media: a scoping review. Cureus 14:e24601. doi: 10.7759/cureus.24601
Journal of Primary Care Specialties. (2021). Available online at: https://journals.lww.com/jopc/fulltext/2021/02010/covid_19_vaccine__a_battle_with_the_uncertainties.5.aspx (Accessed March 25, 2025).
Kbaier, D., Kane, A., McJury, M., and Kenny, I. (2024). Prevalence of health misinformation on social media-challenges and mitigation before, during, and beyond the COVID-19 pandemic: scoping literature review. J. Med. Internet Res. 26:e38786. doi: 10.2196/38786
Kisa, S., and Kisa, A. (2024). A comprehensive analysis of COVID-19 misinformation, public health impacts, and communication strategies: scoping review. J. Med. Internet Res. 26:e56931. doi: 10.2196/56931
Komendantova, N., and Erokhin, D. (2025). Artificial Intelligence Tools in Misinformation Management during Natural Disasters. Public Organ. Rev. 28, 1–25. doi: 10.1007/s11115-025-00815-2
Lev-On, A., Steinfeld, N., Abu-Kishk, H., and Naim, S. (2020). The long-term effects of digital literacy programs for disadvantaged populations: analyzing participants’ perceptions. J. Inf. Commun. Ethics Soc. 19, 146–162. doi: 10.1108/JICES-02-2020-0019
Li, H. O. Y., Bailey, A., Huynh, D., and Chan, J. (2020). YouTube as a source of information on COVID-19: a pandemic of misinformation? BMJ Glob. Health 5:e002604. doi: 10.1136/bmjgh-2020-002604
Library of Congress. (2021). Germany: Network Enforcement Act Amended to Better Fight Online Hate Speech. Available online at: https://www.loc.gov/item/global-legal-monitor/2021-07-06/germany-network-enforcement-act-amended-to-better-fight-online-hate-speech/ (Accessed December 22, 2024).
Lu, J., Zhang, H., Xiao, Y., and Wang, Y. (2024). An environmental uncertainty perception framework for misinformation detection and spread prediction in the COVID-19 pandemic: artificial intelligence approach. JMIR AI 3:e47240. doi: 10.2196/47240
Maaß, S., Wortelker, J., and Rott, A. (2024). Evaluating the regulation of social media: an empirical study of the German NetzDG and Facebook. Telecommun. Policy 48:102719. doi: 10.1016/j.telpol.2024.102719
Machete, P., and Turpin, M. (2020). “The use of critical thinking to identify fake news: a systematic literature review” in Responsible design, implementation and use of information and communication technology. eds. M. Hattingh, M. Matthee, H. Smuts, I. Pappas, Y. K. Dwivedi, and M. Mäntymäki (Cham: Springer International Publishing), 235–246.
MacKay, M., Colangeli, T., Thaivalappil, A., Del Bianco, A., McWhirter, J., and Papadopoulos, A. (2022). A review and analysis of the literature on public health emergency communication practices. J. Community Health 47, 150–162. doi: 10.1007/s10900-021-01032-w
Mena, Paul, Barbe, Danielle, and Chan, Sylvia (2020). Misinformation on Instagram: The Impact of Trusted Endorsements on Message Credibility. Available online at: https://journals.sagepub.com/doi/full/10.1177/2056305120935102 (Accessed March 25, 2025).
Munusamy, S., Syasyila, K., Shaari, A. A. H., Pitchan, M. A., Kamaluddin, M. R., and Jatnika, R. (2024). Psychological factors contributing to the creation and dissemination of fake news among social media users: a systematic review. BMC Psychol. 12:673. doi: 10.1186/s40359-024-02129-2
Muzembo, B. A., Ntontolo, N. P., Ngatu, N. R., Khatiwada, J., Suzuki, T., Wada, K., et al. (2022). Misconceptions and rumors about Ebola virus disease in sub-Saharan Africa: a systematic review. Int. J. Environ. Res. Public Health 19:4714. doi: 10.3390/ijerph19084714
Nannini, L., Bonel, E., Bassi, D., and Maggini, M. J. (2024). Beyond phase-in: assessing impacts on disinformation of the EU digital services act. AI and Ethics. 11, 1–29. doi: 10.1007/s43681-024-00467-w
Nature (2021). Shifting attention to accuracy can reduce misinformation online. Available online at: https://www.nature.com/articles/s41586-021-03344-2 (Accessed December 22, 2024).
New WHO Review Finds. (2022). Infodemics and misinformation negatively affect people’s health behaviours. Available online at: https://www.who.int/europe/news-room/01-09-2022-infodemics-and-misinformation-negatively-affect-people-s-health-behaviours--new-who-review-finds (Accessed December 2022).
Oxford Academic. (2022). Misinformation across digital divides: theory and evidence from Northern Ghana. African Affairs. Available online at: https://academic.oup.com/afraf/article/121/483/161/6575724 (Accessed December 2022).
Page, M. J., JE, M. K., Bossuyt, P. M., Boutron, I., Hoffmann, T. C., Mulrow, C. D., et al. (2021). The PRISMA 2020 statement: an updated guideline for reporting systematic reviews. BMJ 372:n71. doi: 10.1136/bmj.n71
Pennycook, G., and Rand, D. G. (2021). The psychology of fake news. Trends Cogn. Sci. 25, 388–402. doi: 10.1016/j.tics.2021.02.007
Pennycook, G., and Rand, D. G. (2022). Accuracy prompts are a replicable and generalizable approach for reducing the spread of misinformation. Nat. Commun. 13:2333. doi: 10.1038/s41467-022-30073-5
Pertwee, E., Simas, C., and Larson, H. J. (2022). An epidemic of uncertainty: rumors, conspiracy theories and vaccine hesitancy. Nat. Med. 28, 456–459. doi: 10.1038/s41591-022-01728-z
Protection from Online Falsehoods and Manipulation Act (2021). Available online at: https://www.pofmaoffice.gov.sg/regulations/protection-from-online-falsehoods-and-manipulation-act/ (Accessed December 22, 2024).
Pulido, C. M., Villarejo-Carballido, B., Redondo-Sama, G., and Gómez, A. (2020). COVID-19 infodemic: more retweets for science-based information on coronavirus than for false information. Int. Sociol. 35, 377–392. doi: 10.1177/0268580920914755
Rodrigues, F., Newell, R., Rathnaiah Babu, G., Chatterjee, T., Sandhu, N. K., and Gupta, L. (2024). The social media Infodemic of health-related misinformation and technical solutions. Health Policy Technol. 13:100846. doi: 10.1016/j.hlpt.2024.100846
Shea, B. J., Reeves, B. C., Wells, G., Thuku, M., Hamel, C., Moran, J., et al. (2017). AMSTAR 2: a critical appraisal tool for systematic reviews that include randomised or non-randomised studies of healthcare interventions, or both. BMJ 358:j4008. doi: 10.1136/bmj.j4008
Stover, J., Avadhanula, L., and Sood, S. (2024). A review of strategies and levels of community engagement in strengths-based and needs-based health communication interventions. Front. Public Health 12:1231827. doi: 10.3389/fpubh.2024.1231827
Sundaram, S. P., Devi, N. J., Lyngdoh, M., Medhi, G. K., and Lynrah, W. (2023). Vaccine Hesitancy and Factors Related to Vaccine Hesitancy in COVID-19 Vaccination among a Tribal Community of Meghalaya: A Mixed Methods Study. Journal of Patient Experience. 10:23743735231183673. doi: 10.1177/23743735231183673
Sunil, R., Mer, P., Diwan, A., Mahadeva, R., and Sharma, A. (2025). Exploring autonomous methods for deepfake detection: a detailed survey on techniques and evaluation. Heliyon 11:e42273. doi: 10.1016/j.heliyon.2025.e42273
Tan, C. (2022). Regulating disinformation on twitter and Facebook. Griffith Law Rev. 31, 513–536. doi: 10.1080/10383441.2022.2138140
Trengove, M., Kazim, E., Almeida, D., Hilliard, A., Zannone, S., and Lomas, E. (2022). A critical review of the Online Safety Bill. Patterns 3:100544. doi: 10.1016/j.patter.2022.100544
Vosoughi, S., Roy, D., and Aral, S. (2018). The spread of true and false news online. Science 359, 1146–1151. doi: 10.1126/science.aap9559
WHO. (2020a). Managing the COVID-19 infodemic: Promoting healthy behaviours and mitigating the harm from misinformation and disinformation. Available online at: https://www.who.int/news/item/23-09-2020-managing-the-covid-19-infodemic-promoting-healthy-behaviours-and-mitigating-the-harm-from-misinformation-and-disinformation (Accessed December 22, 2024).
WHO (2020b). Factors that contributed to undetected spread. Available online at: https://www.who.int/news-room/spotlight/one-year-into-the-ebola-epidemic/factors-that-contributed-to-undetected-spread-of-the-ebola-virus-and-impeded-rapid-containment (Accessed December 22, 2024).
Yu, J., Bekerian, D. A., and Osback, C. (2024). Navigating the digital landscape: challenges and barriers to effective information use on the internet. Encyclopedia 4, 1665–1680. doi: 10.3390/encyclopedia4040109
Zhang, J., Pan, Y., Lin, H., Sun, Z., Wu, P., and Tu, J. (2023). Infodemic: Challenges and solutions in topic discovery and data process. Arch. Public Health 81:166. doi: 10.1186/s13690-023-01179-z
Keywords: infodemic, misinformation, COVID-19, pandemics, health communication, social media, public health, epidemiology
Citation: Bhattacharya S and Singh A (2025) Unravelling the infodemic: a systematic review of misinformation dynamics during the COVID-19 pandemic. Front. Commun. 10:1560936. doi: 10.3389/fcomm.2025.1560936
Edited by:
Yi Luo, Montclair State University, United StatesReviewed by:
Ashwani Kumar Upadhyay, Symbiosis International University, IndiaDhouha Kbaier, The Open University, United Kingdom
Copyright © 2025 Bhattacharya and Singh. This is an open-access article distributed under the terms of the Creative Commons Attribution License (CC BY). The use, distribution or reproduction in other forums is permitted, provided the original author(s) and the copyright owner(s) are credited and that the original publication in this journal is cited, in accordance with accepted academic practice. No use, distribution or reproduction is permitted which does not comply with these terms.
*Correspondence: Sudip Bhattacharya, ZHJzdWRpcDgxQGdtYWlsLmNvbQ==