- 1Rheumatology Service, Instituto de Investigación Sanitaria La Princesa, Hospital Universitario de la Princesa, Madrid, Spain
- 2Department of Immunology and Oncology, Centro Nacional de Biotecnología/CSIC, Madrid, Spain
- 3Department of Cellular Biology, Facultad de Biología, Universidad Complutense de Madrid, Madrid, Spain
- 4Leeds Institute of Rheumatic and Musculoskeletal Medicine (LIRMM), The University of Leeds, Leeds, United Kingdom
- 5Institute of Parasitology and Biomedicine López-Neyra, CSIC, Granada, Spain
Rheumatoid arthritis (RA) is a chronic inflammatory disease characterized by an autoimmune response in the joints and an exacerbation of cytokine responses. A minority of patients with RA experience spontaneous remission, but most will show moderate/high disease activity, with aggressive joint damage and multiple systemic manifestations. There is thus is a great need to identify prognostic biomarkers for disease risk to improve diagnosis and prognosis, and to inform on the most appropriate therapy. Here we focused on suppressor of cytokine signaling 1 (SOCS1), a physiological negative regulator of cytokines that modulates cell activation. Using four independent cohorts of patients with arthritis, we characterized the correlation between SOCS1 mRNA levels and clinical outcome. We found a significant inverse correlation between SOCS1 mRNA expression and disease activity throughout the follow-up of patients with RA. Lower baseline SOCS1 levels were associated with poorer disease control in response to methotrexate and other conventional synthetic disease-modifying anti-rheumatic drugs in early arthritis, and to rituximab in established (active) RA. Moreover, we identified several single nucleotide polymorphisms in the SOCS1 gene that correlated with SOCS1 mRNA expression, and that might identify those patients with early arthritis that fulfill RA classification criteria. One of them, rs4780355, is in linkage disequilibrium with a microsatellite (TTTTC)3−5, mapped 0.9 kb downstream of the SNP, and correlated with reduced SOCS1 expression in vitro. Overall, our data support the association between SOCS1 expression and disease progression, disease severity and response to treatment in RA. These observations underlie the relevance of SOCS1 mRNA levels for stratifying patients prognostically and guiding therapeutic decisions.
Introduction
Rheumatoid arthritis (RA) is a systemic autoimmune disease characterized by chronic inflammation in diarthrodial joints. RA is known to have a very heterogeneous clinical course with a period of preclinical disease, and can be classified in several phases, from the initial genetic and environmental risk factors for RA, to the development of autoimmunity, arthralgia symptoms and inflammatory arthritis, and finally to established RA (1).
A small number of patients with inflammatory arthritis experience spontaneous resolution of symptoms, and some have a mild disease course with slow progression, which is diagnosed as undifferentiated arthritis (UA). Most patients with inflammatory arthritis nonetheless fulfill the European League Against Rheumatism (EULAR) 2010 criteria for RA and develop moderate-to-high disease activity and aggressive joint damage, often with systemic complications. In this scenario, clinical trials (2, 3) and daily clinical practice (4) have confirmed that early treatment with disease-modifying anti-rheumatic drugs (DMARDs) improves the outcome patients with early RA. To avoid the generalized use of aggressive therapies that could expose patients to unjustified risks of side effects and whose cost would needlessly burden National Health Services, much effort has been made to identify prognostic biomarkers for disease risk, to improve diagnosis and prognosis and to help guide the most appropriate therapy for each patient (5).
Cytokines were originally identified as soluble messengers in the context of the immune system, but are now known to be released by myriad cell types with multifunctional actions on cell proliferation, survival, apoptosis, differentiation and activation. Based on their role in the immune response, cytokines are classified as either pro- or anti-inflammatory (6), and some have key functions in different stages of RA (7). Cytokines act by engaging specific receptors constitutively associated with the Janus family tyrosine kinase–signal transducer and activator of transcription (JAK–STAT) signaling pathway. Upon ligand binding to receptors, JAKs are activated by transphosphorylation, and phosphorylate both the receptor and members of the STAT family of transcription factors. In turn, activated STATs dimerize and translocate to the nucleus to regulate gene expression (8). Dysregulated activation of the JAK–STAT pathway is associated with many diseases, including autoimmune disorders such as RA (9).
Cytokine-mediated signaling pathways are controlled precisely at several levels, including activation of phosphatases, upregulation of proteins that interfere with STAT binding to DNA, and expression of SOCS (suppressor of cytokine signaling) proteins, which suppress JAK activity, prevent STAT recruitment to the receptor, and induce substrate degradation (10–12). The SOCS proteins constitute a family of intracellular proteins comprising eight members that, among other processes, regulate cytokine-triggered signaling and cell activation status (13). While a recent genome-wide meta-analysis failed to identify a significant association between RA and SOCS1 (14), SOCS1 mRNA levels are significantly increased in peripheral blood T-cells and in synovial membranes of RA patients as compared with patients with osteoarthritis (15), pointing to a possible role for SOCS1 in RA.
In the present study, we used four independent cohorts of patients with arthritis to test for associations between SOCS1 expression and RA. We observed an inverse correlation between SOCS1 mRNA expression levels and disease activity, with lower baseline SOCS1 levels associating with poorer disease control. Genotyping analysis identified several single nucleotide polymorphisms (SNPs) in the SOCS1 gene that associate with RA development and with response to treatment. Finally, in vitro expression analysis indicated that the minor allele of one of these SNPs, rs4780355, correlated with reduced SOCS1 expression, supporting a functional relationship between this SNP and disease progression.
Patients, Materials, and Methods
Study Populations
Princesa Early Arthritis Register Longitudinal (PEARL) Study
The PEARL study includes patients referred to the Early Arthritis Clinic at the Hospital Universitario La Princesa, Madrid (Spain) (16). Inclusion criteria were at least one swollen joint and symptoms for <1 year. The register protocol included the collection of socio-demographic, clinical, and therapeutic data, as well as samples obtained in four scheduled visits (baseline, 6, 12, and 24 months). At the 24-month visit, a diagnosis of RA was established definitively based on the 1987 American College of Rheumatology criteria (17) as opposed to chronic UA (18) (Table 1). The PEARL study was approved by the Ethics Committee for Medical Research (CEIM, Hospital Universitario La Princesa. Instituto de Investigación Sanitaria La Princesa, Madrid; PI-518). All patients gave written informed consent. From the PEARL register, we selected both discovery and validation populations (Supplementary Table 1, Studied), The discovery group included patients that did (RA) or did not (UA) fulfill RA criteria at the end of follow-up, whereas the validation group included more stringent criteria for inclusion and all patients fulfilled the 1987 RA criteria at the end of follow-up. The main characteristics of the two groups are shown in Supplementary Table 2.
Leeds Cohorts
Leeds patients (Table 1) were included in two cohorts. The first cohort included 74 DMARD-naïve patients with early RA fulfilling the 2010 EULAR criteria that were selected from a prospective Early Arthritis Register (Table 1) and were treated in a standardized fashion with methotrexate at 15 mg/week, increasing to 25 mg/week over 8 weeks if remission was not achieved. Additional DMARDs (sulfasalazine or hydroxychloroquine) were used if low disease activity was not achieved by 3 months. The DAS28 (Disease Activity Score including a 28-joint count) was used as a 6-month outcome to classify response, with a DAS28 score <2.6 defining remission. The second cohort included 64 patients with established RA who showed an inadequate response to classic/synthetic DMARD treatment and were treated with rituximab (Table 1). Approval for the study with the Leeds cohorts was obtained from the North East Yorkshire Research Ethics Committee (PI: 07/S0703/68, 10/H1307/138). Written informed consent was obtained from all patients.
DNA Extraction, qRT-PCR, and PCR
For all samples, peripheral blood mononuclear cells (PBMCs) were isolated by Ficoll density gradient centrifugation (Histopaque-1077, Sigma-Aldrich, USA). For the PEARL study samples, total RNA (2 μg) obtained using the TRI Reagent (Sigma-Aldrich) was reverse-transcribed using the High-Capacity cDNA Reverse Transcription Kit (Applied Biosystems, USA). For the Leeds samples, RNA was extracted using a standard guanidium/phenol method and first-strand cDNA was synthesized using a High-Capacity cDNA Reverse Transcription Kit (Thermo Fisher, USA).
For SOCS1 analysis, and in order to confirm an amplification efficiency ~2, three 1:4 serial dilutions of each cDNA (corresponding to ~5 ng, 1.25 ng and 0.31 ng of the original RNA per well) were amplified in triplicate by qRT-PCR on an ABI Prism HT7900 sequence detection system using SYBR Green PCR Master Mix (both from Applied Biosystems) and 0.3 μM of the following primers:
SOCS1.5′: ACCCCGTCCTCCGCGACTAC; SOCS1.3′: TCCGCTCCCTCCAACC CAGG; β-actin.5′: AGCGAGCATCCCCCAAAGTT; β-actin.3′: GGGCACGAAGGCT CATCATT; GAPDH.5′: AGAAGGCTGGGGCTCATTTG; GAPDH.3′: AGGGGCCA TCCACAGTCTTC; HPRT1.5′: ACCAGTCAACAGGGGACATAAAAG; HPRT1.3′: GTCTGCATTGTTTTGCCAGTGTC. For relative quantification, the higher template concentration (5 ng) of each sample was used, normalized against the expression of ACTB and with the mean value obtained from 40 healthy donors (2−ΔΔCt) (19). GAPDH was used as a second internal control to validate ACTB levels. The same primers were used for SOCS1 in samples from the Leeds study, and HPRT was used as a housekeeping gene: HPRT.5′: GGAAAGAATGTCTTGATTGTGGAAG; HPRT.3′: AAGGAACCAGTCC GTCATATTAGG.
For in vitro phytohemagglutinin (PHA; Sigma, Spain) activation assays, ACTB and HPRT1 were used in a similar manner, although in this case normalization against HPRT1 levels was used for the figures shown here.
SOCS1 expression in the PEARL-validation group was analyzed using the Roche RealTime ready Custom Panels 384 and LightCycler 480 Probes Master with pre-plated primers from a Universal Probe Library (Roche, Germany). Assays were performed in triplicate and results were normalized to ACTB expression levels.
SOCS1 Genotyping
The Immunochip array on the Illumina iScan System (Illumina Inc., USA) was used to genotype 261 patients from the PEARL study. The chip array includes 196,000 SNPs with genetic positions according to the NCBI build 36 (hg18) map (Illumina manifest file Immuno_BeadChip_11419691_B.bpm). To control batch effects, stringent quality controls were applied to datasets prior to the final analysis. Raw data from Immunochips were filtered using PLINK v1.07 software as follows: low-quality SNPs were discarded when call rates were <95%, minor allele frequencies (MAFs) <0.01, and deviation from the Hardy-Weinberg equilibrium p < 0.001. Samples were also discarded when they showed <90% of successfully called SNPs. Ten SNPs were selected for subsequent genotyping in the 571 remaining PEARL early arthritis patients. The selected SOCS1 genetic variants (rs11074956, rs181582, rs149597, rs2021760, rs4780355, rs193779, rs243327, rs1559392, rs3844576, and rs243323) were genotyped using predesigned TaqMan probes (Applied Biosystems, assay ID: C___3189858_10, C___1004298_10, C___3189853_10, C__11185228_10, C___3189846_10, C___1004284_10, C___3189840_10, C___9697634_10, C___3189819_10, C___3189829_10, respectively). After PCR, the genotype of each sample was attributed automatically by measuring allele-specific fluorescence on a CFX Touch Real-Time PCR System using CFX 3.1 Manager (BioRad, USA). Duplicate samples and negative controls were included to verify genotyping accuracy.
PBMC Activation
To resolve the problem of the small volume of available blood obtained from patients, we first determined the optimal experimental conditions to activate PBMCs from both healthy donors and patients with RA. PBMCs were isolated as described and were activated with PHA (5 μg/ml). A peak of SOCS1 transcription was detected between 2 and 4 h post-stimulation, followed by a decline and then a second peak at ~16 h. We included NF-kB as an activation marker, as it is well-known to regulate inflammatory genes and cytokine production (20). Because the second upregulation peak might be the result of responses to multiple cytokines secreted by the distinct PBMC populations, we selected the early stimulation peak for comparative analysis.
Thus, PBMCs from patients, healthy donors or, when required, buffy coats, were isolated as above, seeded at 106 cells/ml, and stimulated with 5 μg/ml PHA for 3 h (21). Cells were then harvested for RNA extraction.
Luciferase Assay
Genomic DNA that included the SOCS1 polyadenylation signal and downstream sequences was cloned from DNA of buffy coats from healthy donors. Two clones with the rs4780355 minor allele (C) and two with the major allele (T) were selected for expression assays. A 790-base pair (bp) fragment comprising 68 bp upstream of the polyadenylation signal and rs4780355 (located 435 bp downstream), and a second fragment of 2.1 kbp with the same 5′ end and containing (TTTTC)3−5 located at 0.9 kbp 3′ of rs4780355, were PCR-amplified, individually cloned and sequenced verified. The fragments were cloned into the psiCHECK-2 vector (Promega, USA), between the Renilla luciferase coding sequence and its synthetic polyA site. The psiCHECK-2 plasmid has two different luciferase genes (Firefly and Renilla) driven by distinct promoters: the Renilla gene serves as a reporter, whereas the Firefly gene acts as an internal control. Plasmids were electroporated into Jurkat cells, which were cultured for 24 h (37°C, 5% CO2) and harvested. Cell lysates from 1–3 × 104 cells were analyzed for Renilla and Firefly luciferase activity using the Dual Luciferase Reporter Assay System (Promega) and data were normalized to Firefly luciferase activity.
Statistical Analysis
Statistical analyses were performed using Stata 14 for Windows (Stata Corp LP, USA) and Graphpad Prism 6 for Mac (GraphPad Software Inc., USA). Normally-distributed quantitative variables were expressed as the mean ± standard deviation, and non-normally-distributed variables were expressed as the median and interquartile range (IQR). In case of normal distribution, bivariate analyses were performed using a t-test; Mann-Whitney U, Kruskal-Wallis or p-trend tests were used for non-normally distributed variables. The χ2 test was used for qualitative variables.
Variables
SOCS1 gene expression, expressed as 2−ΔΔCt, does not show a Gaussian distribution, and so data were normalized through logarithmic transformation. When required, a variable SOCS1 baseline was used corresponding to low levels (low SOCS1), defined as those of samples from the baseline visit (PEARL or Leeds populations) below percentile 25 of their respective whole population.
Disease activity at the end of follow-up was defined by DAS28 cut-off values (22). In the case of the PEARL study, the EULAR response (22) was determined at visits at 6, 12, and 24 months relative to the baseline visit. A dichotomic variable “response” was developed for logistic regression by joining good and moderate EULAR responses.
Diagnosis at the end of follow-up in the PEARL population was expressed as a dichotomic variable between UA and RA as described above (see the Study Populations section).
Analyses
Cuzick's test was used to study SOCS1 evolution during follow-up and its association with disease activity level.
As several variables can contribute to slight modifications in SOCS1 expression, the descriptive analyses were followed by multivariate analyses based on generalized estimating equations (GEE) nested by patient and visit, using the xtgee command of Stata. This model allows a better-adjusted SOCS1 value for each patient. Briefly, we used multivariate models by adding all variables with p < 0.20 in the bivariate analysis. This was followed by manual backward-stepwise selection to fit the final models by sequentially removing variables with p > 0.20, except the variable diagnosis that was forced since UA was included only in the discovery subpopulation.
To select the most relevant SNPs from the 10 identified as representative of genetic heterogeneity in the SOCS1 region, we used three independent models of logistic regression with the following dependent variables: (a) low SOCS1 expression at baseline visit adjusted by the relevant variables that affect SOCS1 expression, selected according to the longitudinal multivariate model (age, diagnosis, glucocorticoid treatment, disease activity level, and hemoglobin); (b) diagnosis at the end of follow-up; and (c) therapeutic response at the 12-month visit, adjusted by the variables that affect DAS28 (age and sex) (23). As the studied population showed a very heterogeneous background, we performed a sensitivity analysis based on ethnicity (not shown). No differences were observed after this adjustment, indicating the ancestry of the population does not contribute to the expression of SOCS1, independently of the contribution of the SNPs described here.
Results
Patient Characteristics
Baseline characteristics of patients are listed in Table 1. In the PEARL register, 71% of patients fulfilled the criteria for RA at the end of 2-year follow-up, whereas 29% were considered to have UA. The Leeds population included 74 DMARD-naïve patients with early RA and 64 patients with established RA.
All PEARL study patients were genotyped for various SNPs in SOCS1 (see Methods), but only a subpopulation (n = 143) with full follow-up and high-quality mRNA samples was used for SOCS1 expression analysis (Supplementary Table 1; studied population). No significant differences were observed in the disease characteristics between patients studied and not studied, which indicates that the studied population is fully representative of the entire register (Supplementary Table 1).
Low Baseline SOCS1 Expression as a Biomarker of Disease Severity in Patients With Inflammatory Arthritis
Using blood samples collected at different visits (n = 143 total), we studied SOCS1 expression in 104 patients from the PEARL register (discovery group; see Supplementary Table 2 for baseline characteristics). Despite a significant decrease in disease activity as a result of treatment adjusted over time (Figure 1A, Supplementary Table 3), we observed a significant reduction in SOCS1 mRNA expression during follow-up of patients with UA (Figure 1B; p = 0.013), but no significant changes in SOCS1 mRNA expression in patients with RA. When patients were classified by the DAS28 score (remission, low, moderate or high activity) and after pooling all visits, those with RA showed no difference in SOCS1 expression (Figure 1C), whereas a significant direct correlation between SOCS1 levels and disease activity was observed for patients with UA (Figure 1D; p = 0.027).
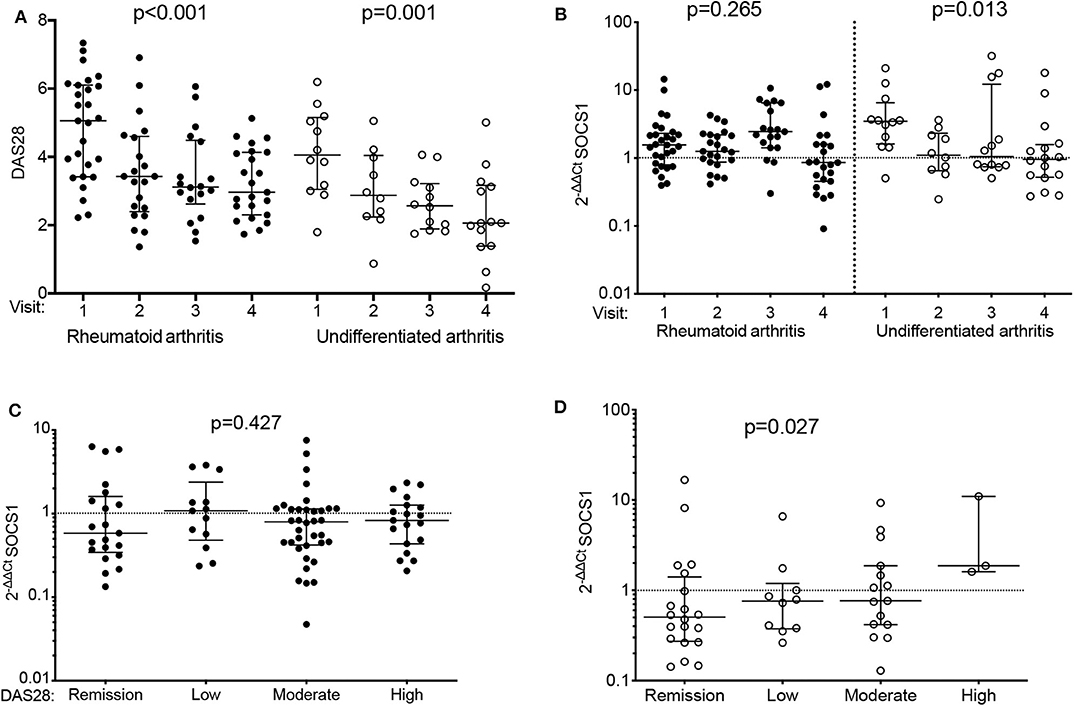
Figure 1. Differential SOCS1 expression throughout follow-up of patients with early arthritis by final diagnosis: discovery population. (A) Evolution of disease activity by diagnosis (rheumatoid arthritis [RA]: solid dots; undifferentiated arthritis [UA]: empty dots). (B) Variation of SOCS1 expression throughout follow-up in patients with RA (solid dots) or UA (empty dots). (C,D) SOCS1 expression according to disease activity (DA) assessed by DAS28 in visits of patients with RA (C) or UA (D). Data shown for mRNA SOCS1 levels normalized to ACTB and to mean SOCS1 expression levels in healthy donors (2−ΔΔCt). Error bars show medians and interquartile range. Statistical significance was determined with Cuzick's non-parametric trend test. RA patients, n = 70; UA patients, n = 34; healthy donors, n = 40.
In light of these observations, we replicated the study in a second PEARL group engaged in the 2011–2014 period. This patient group used more stringent criteria, and included only those patients with definite RA classification according to the 1987 criteria (17), and for whom sample availability included baseline and ≥2 visits (validation group; n = 39, 111 samples; see Supplementary Table 2 for baseline characteristics). Analysis of these samples confirmed a decrease in disease activity (Supplementary Figure 1A) that did not correlate with a reduction in SOCS1 expression either when patients were classified by follow-up visit number (Supplementary Figure 1B), by DAS28 score, (Supplementary Figure 1C), or when considered globally (Supplementary Figure 1D).
We detected some significant differences between the discovery and validation groups for sex, diagnosis, rheumatoid factor (RF) and antibodies to anti-citrullinated proteins (ACPA), likely due to the relatively low number of patients with definite RA in the validation group (Supplementary Table 2). As these variables could act as confounders in the analysis, we reanalyzed the pooled data using a longitudinal multivariate model nested by patients and visits. SOCS1 expression was significantly lower in patients older than 65 years, as well as in those with increased hemoglobin levels (Table 2). Glucocorticoids treatment and patients with UA tended to associate with higher SOCS1 expression (Table 2). The model adjusted by the aforementioned confounders showed significantly lower SOCS1 mRNA levels in samples from visits with moderate or high disease activity compared with those of patients in remission (Table 2). The correlation of SOCS1 values with those variables included in the multivariable analysis (age, glucocorticoids, disease activity and hemoglobin) is shown in Supplementary Figures 2A–D, and information on SOCS1 levels in RA vs. UA is included in Supplementary Figure 2E.
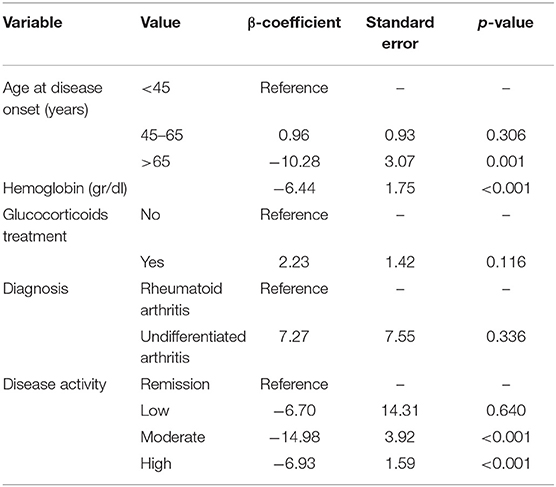
Table 2. Variables that affect SOCS1 expression: multivariate analysis performed in the pooled PEARL population.
We hypothesized that patients with low SOCS1 expression and high disease activity at baseline might coincide with those unable to achieve adequate control of disease at follow-up. We thus tested whether low SOCS1 levels at baseline (values below the 25th percentile) were associated with higher disease activity after 2 years. In the combined PEARL patient subsets (n = 143), our analysis showed a trend for higher disease activity after 2 years in patients with low SOCS1 expression at baseline (Figure 2A, p = 0.053). The lack of significance was not unexpected given the heterogeneity of the cohorts evaluated. PEARL is a longitudinal observational register and includes patients with different treatments and even with intensified treatments in cases when the disease was not under control.
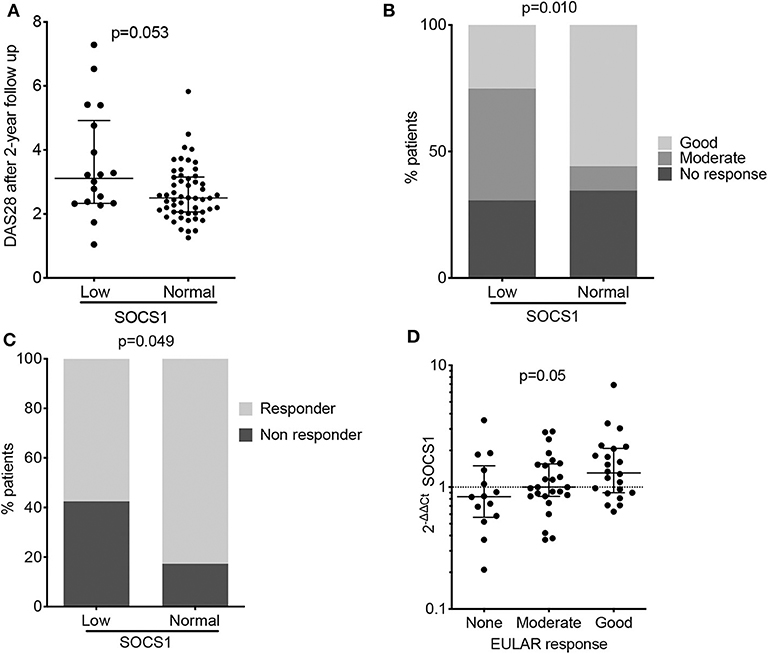
Figure 2. Baseline SOCS1 expression as a severity biomarker in patients with RA (PEARL study). (A) Disease activity estimated by DAS28 score after 2 years of follow-up, relative to baseline SOCS1 expression levels. Data shown as individual values with median and interquartile range. Statistical significance was established by the Mann-Whitney U test; low SOCS1 levels (n = 25) were defined as those values below percentile 25 at the baseline of PEARL population; the remaining patients were considered to have a normal SOCS1 level (n = 67). (B) Percentage of patients classified by EULAR response criteria after 12 months follow-up in PEARL subpopulations. Statistical significance was determined by the χ2 test. low SOCS1 levels (n = 25) were defined as those values below percentile 25 at the baseline of PEARL population; the remaining patients were considered to have a normal SOCS1 level (n = 67) as described for panel (A). (C) Percentage of responder and non-responder patients after 6 months rituximab infusion. Low SOCS1 levels (n = 14) were defined as those values below percentile 25 at the baseline of the Leeds established RA population; the remaining patients were considered to have a normal SOCS1 levels (n = 48). Statistical significance was determined as in (B). (D) Baseline SOCS1 expression relative to EULAR response criteria after 6-months rituximab infusion (Leeds study). Data shown for mRNA SOCS1 levels normalized to ACTB and to mean SOCS1 expression levels in healthy donors (2−ΔΔCt); error bars show medians and interquartile range; (n = 14 no response, n = 25 moderate response and n = 23 good response). Statistical significance was determined using Cuzick's non-parametric trend test.
SOCS1 Expression and Response to Therapy
Disease activity, a parameter strongly influenced by patient treatment, was heterogeneous throughout the PEARL follow-up (Supplementary Table 3). We nevertheless evaluated the potential of baseline SOCS1 expression to predict EULAR response at 6-, 12-, and 24-months of follow-up. Responder patients with lower SOCS1 mRNA levels at baseline showed poorer EULAR response rates in the three visits, although this was statistically significant only at the 12-month visit (Figure 2B; p = 0.010).
Data from the independent early, drug-naïve, Leeds RA cohort (n = 74) treated according to the treat-to-target protocol (i.e., the achievement of remission (24) with synthetic DMARDs (methotrexate dose escalation) confirmed that low SOCS1 baseline expression was associated with inability to achieve remission at 6 months (Table 3, p = 0.036). Receiver operating characteristic curve analysis (Supplementary Figure 3) demonstrated an area under the curve of 0.644 (95% confidence interval 0.514–0.775) for predicting remission. A regression analysis (Supplementary Table 4) showed the best predictive model (achieving 73% accuracy) used 4 variables (Age, TJC, CRP and SOCS1) although replacing TJC/CRP by DAS28 in a second model improved the regression but had no effect on accuracy. An AUROC analysis of the variables retained in the regression (Supplementary Table 5) showed that SOCS1 was the second best predictor of remission after TJC in this small early RA cohort (sensitivity/specificity 56%/67%, odd ratio 1.73, and positive/negative predictive value 78%/64%).
The association between SOCS1 expression and response to treatment was further investigated with the 64 patients from Leeds with established RA who were treated with rituximab (Table 3). This analysis revealed only one significant association between non-response to treatment and low baseline SOCS1 levels in this small cohort (Figure 2C, p = 0.049). Baseline SOCS1 levels also differed significantly depending on EULAR response classification 6-months post-rituximab treatment (Figure 2D; p = 0.050).
The rs4780355 Polymorphism in the SOCS1 Sequence Associates With Reduced SOCS1 Expression
Given the potential clinical relevance of determining SOCS1 mRNA levels in early RA, we tested whether genetic variability in the SOCS1 gene and adjacent areas influences SOCS1 expression and clinical parameters. Immunochip data (25) extracted for SNPs near the SOCS1 gene and also located at the two intergenic regions gave a total of 47 SNPs. We generated a linkage disequilibrium plot using Haploview software and defined three haplotype blocks of 2, 4 and 12 kb (Supplementary Figure 4).
After linkage disequilibrium analysis, 10 SNPs representative of the genetic variability in the SOCS1 gene and adjacent areas (rs11074956, rs181582, rs149597, rs2021760, rs4780355, rs193779, rs243327, rs1559392, rs3844576, and rs243323) were selected for subsequent genotyping in PEARL patients using predesigned TaqMan probes. The 10 SNPs were studied for association with baseline low SOCS1 mRNA, RA diagnosis at the end of follow-up, and response to treatment at 12 months of follow-up (Supplementary Table 6). The minor allele of four of these SNPs tended to associate with low SOCS1 expression; and another two SNPs with high levels at baseline (p < 0.150; Supplementary Table 6). We observed that from the four SNPs whose minor allele was associated with low SOCS1 expression, two were also linked to a higher odds ratio of RA diagnosis (p < 0.150), and one of these two (rs4780355) was linked to poor response (p < 0.150) (Supplementary Table 6), suggesting that this SNP might predict a poorer clinical course in patients with early arthritis. Regarding the relaxed p-values considered in these analyses, it should be mentioned that several SNPs in addition to rs4780355 might affect SOCS1 expression, limiting the feasibility of recruiting a sufficient number of patients per subgroup to allow the analysis of SOCS1 expression by haplotypes.
Both rs11074956 and rs4780355 have been associated with some autoimmune and inflammatory diseases (26, 27), but we focused on rs4780355 (T/C SOCS1) because it was the most consistent with the considered criteria (Supplementary Table 6), and it is located near the SOCS1 3′ untranslated region (UTR) (Supplementary Figure 5), allowing the study of its influence on SOCS1 expression in in vitro transcription assays.
To begin to examine the implications of rs4780355 (T/C SOCS1) for SOCS1, we first isolated PBMCs from homozygous C/C and T/T, and heterozygous C/T patients, and measured SOCS1 mRNA levels before and after in vitro activation of cells with PHA. PHA stimulation mimics T-cell receptor activation (28), and therefore does not restrict the analysis to a single cytokine, thus amplifying the possibilities of an effect triggered by SOCS1. Although SOCS1 is expressed in control conditions (29), its expression is upregulated following cell activation (13). Results showed that SOCS1 mRNA induction in PHA-activated PBMCs was lower for homozygous (C/C) patients than for heterozygous (C/T) patients (p = 0.048), and there was also a trend for lower expression when compared with homozygous (T/T) patients (p = 0.1) (Figure 3A). Hematological analysis of these patients showed no differences in the numbers of lymphocytes or monocytes (Supplementary Figures 6A,B).
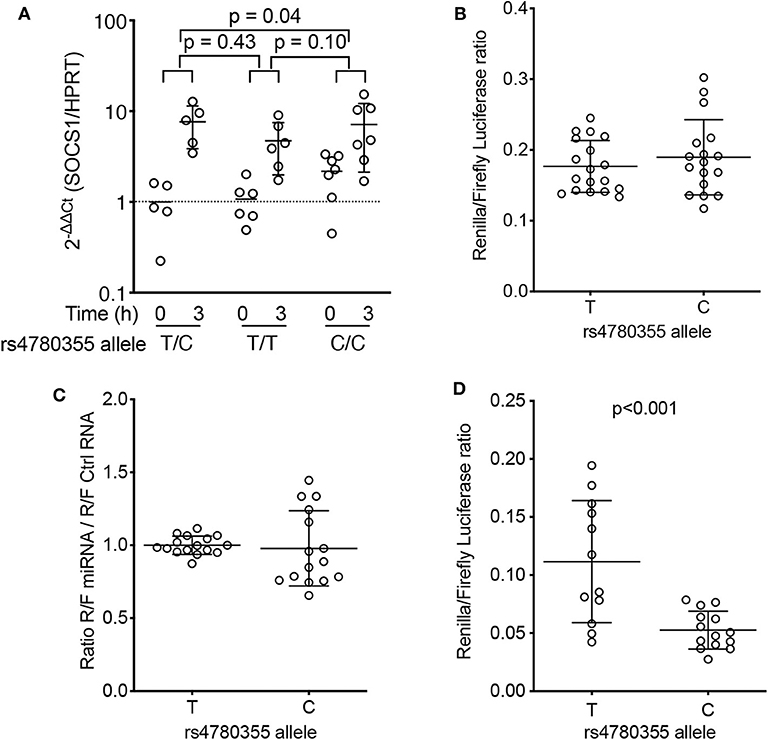
Figure 3. Effects of rs4780355-linked genomic DNA features on SOCS1 expression. (A) Peripheral blood mononuclear cells from 18 patients with RA (five T/C heterozygous, seven C/C homozygous and six T/T homozygous for rs4780355) were PHA-activated for 3 h. SOCS1 levels were determined by qRT-PCR (see Methods) before and after 3 h treatment. Values (2−ΔΔCt) are normalized to ACTB and the mean value from heterozygous samples at t = 0; p-values from unpaired t-tests are indicated. (B) Luciferase assays. Two independent constructs for each genotype, which included the SOCS1 polyadenylation site and rs4780355 SNP, were used for Jurkat cell transfection; the Renilla/Firefly luciferase ratio was measured 24 h later. (C) miR-498 or control RNA (MISSION® siRNA Universal Negative Control #2, Sigma) were co-transfected with plasmid constructs. Renilla/Firefly ratios obtained with miR-498 were normalized to control miRNA values. Results from two independent clones and triplicate measurements. (D) Two independent clones, including the polyadenylation site, rs4780355 SNP (C or T) and its associated microsatellite [[TTTTC]3 or [TTTTC]5, respectively], were tested for each haplotype in two experiments with triplicate samples. Statistical significance was determined by the Mann-Whitney U test.
We next used an in vitro system to examine the role of this polymorphism in SOCS1 expression. The SOCS1 3′UTR (containing the T or C allele region) was cloned into the psiCHECK-2 vector (see Methods). Jurkat cell transfection assays showed no significant differences in the ratio of Renilla/Firefly luciferase between constructs with T or C alleles (four independent clones for each allele; Figure 3B). Analysis of the miRDB database (www.mirdb.org) pointed to the DNA region containing the rs4780355 polymorphism as a potential target for the microRNA miR-498 (Supplementary Figure 7). This microRNA is downregulated in CD4+ T-cells in patients with RA (30), which suggests a role in RA pathogenesis. We therefore transfected Jurkat cells with the luciferase constructs for each allele in the presence of miR-498 or an miR-control. Data showed no significant difference in luciferase activity between the two conditions (Figure 3C), indicating the lack of a direct effect of the polymorphism or a potential role for miR-498 in SOCS1 expression.
Detailed analysis of the DNA sequence surrounding the rs4780355 polymorphism identified a microsatellite followed by a variable T-string, GCT[TTTTC](3−5)T(11−19)GC, 0.9 kbp downstream of the polymorphism. The observed sequence variations were deposited in NCBI's ClinVar database SCV000999906 (Supplementary Figure 8). In Spanish and British populations, allele C is linked (R2 = 0.9456) to the short microsatellite (three repeats), whereas allele T is associated with the long form (five repeats) (Supplementary Figure 9). We thus generated a construct containing the SOCS1 3′UTR (including the T or C allele region and the associated microsatellites) cloned into the psiCHECK-2 vector. Jurkat cell transfection showed a significant reduction in the Renilla/Firefly luciferase ratio when the construct contained the C allele, that is, the short form of the microsatellite (Figure 3D). These data suggest that the effect of rs4780355 on SOCS1 expression is related to presence of the microsatellite (TTTTC)3−5 and the variable T-string in the 3′UTR region.
Discussion
Genome-wide association studies have found multiple SNPs on genes that encode cytokines, their receptors and/or signaling molecules, associating with inflammatory and autoimmune disease risk (31, 32). This is also the case for RA, an autoimmune disease for which cytokine-based therapies are included in the armamentarium of drugs for the treatment of patients with an inadequate response to conventional DMARDs (33–36). Baricitinib and tofacitinib, two small-molecule JAK inhibitors that block JAK/STAT signaling, have recently been approved for RA treatment (7). Physiologically, cytokine activation also triggers downregulatory mechanisms that protect cells from hyperactivation. Cytokines promote the expression of SOCS proteins that not only block JAK/STAT signaling, but also trigger protein degradation by the proteasome (37). Here we show that patients with early arthritis who are unable to upregulate SOCS1 expression are more likely to be classified as having RA and have a higher risk of not achieving remission and/or failing to respond to treatment. Our data concur with previous observations showing that Socs1−/− mice are hypersusceptible to IL-1-mediated acute inflammatory arthritis and have increased joint damage, with no change in the time course of arthritis (38). In a similar line, Stat1−/− mice show exacerbated zymosan-induced arthritis, possibly due to reduced SOCS1 expression (39). Ectopic expression of SOCS1 abolishes IFN-β-mediated STAT1α stimulation and prevents IFN-β-induced expression of CD40 (40), a protein involved in RA pathogenesis (7). In addition to its effect on the JAK-STAT pathway, SOCS1 interacts with the adaptor protein GRB2, the phosphoprotein VAV, calcineurin, and IL-1 receptor-associated kinase, and thereby suppresses signaling through KIT, T-cell receptor, and Toll-like receptors 2 and 4 (41, 42). SOCS1 inhibits MYD88 adaptor-like protein degradation and thus regulates NF-kB activation and, accordingly, Socs1−/− mice show increased susceptibility to chronic LPS-induced inflammation (43, 44). This pathway is involved in the expression of several inflammatory mediators, including TNF-α, IL-1β, IL-6, and type I interferon, all useful targets for biological therapy for RA (45, 46).
In humans, SOCS1 expression is associated with CD4+ T-cell resistance to the immunosuppressive effect of IL-10, which is detected before the cells migrate to synovial tissue (47). Expression of miR-155, a microRNA that targets SOCS1, has been detected in PBMCs from patients with RA, and is involved in TNFα and IL-1β upregulation (48). Correlation between DNA methylation of SOCS1 and the degree of inflammation, assessed by TNFα and IL-6 levels, was also shown in patients with HLA-B27+ spondylitis (49).
Non-activated immune cells express low levels of SOCS, which is rapidly and transiently upregulated after cytokine activation (13). We found that patients with UA in remission had low levels of SOCS1 that increased in parallel to disease activity. By contrast, SOCS1 mRNA levels did not correlate with disease activity in patients with RA, but those with the lowest baseline levels showed the poorest clinical evolution. Genetic variants that affect SOCS1 expression thus might alter the course of RA. In our analysis, several SOCS1 gene variants affected its mRNA levels. Three SNPs (rs11074956, rs4780355, and rs243323) were associated with low SOCS1 expression and a tendency for poorer clinical outcomes. In an in vitro luciferase-based assay, we detected lower SOCS1 expression in Jurkat cells expressing the minor allele of rs4780355. This SNP is located in Chr16:11254001 (GRCh38.p7) within the 3′UTR of the SOCS1 gene. In silico analysis indicated that the SOCS1 sequence involving the rs4780355 polymorphism is a potential target for miR-498. Interestingly, miR-498 directly targets the 3′UTR of STAT3 and is downregulated in CD4+ T-cells of patients with RA (30). We hypothesized that it might also target the SOCS1 3′UTR region, triggering SOCS1 downregulation and thus cytokine hyperactivation. However, based on our in vitro luciferase-based assays using cells transfected with miR-498, we discarded this hypothesis.
We found that rs4780355 is in linkage disequilibrium with expansions of simple sequence repeats, microsatellites, in a non-coding region near the SOCS1 3′UTR. In the population origin of our cohorts, the C allele is linked to the shortest form of the microsatellite, whereas the T allele is associated with five repeats. The in vitro luciferase-based assays linked the C allele to reduced SOCS1 expression when cells bore the minor allele of rs4780355. RNA misprocessing has been reported for a number microsatellite-expansion diseases (50), and in other cases microsatellites cause gene silencing, possibly due to impairment of transcriptional elongation (51). RNA polymerase II transcription frequently terminates 500 or more bp downstream of the poly(A) signal (52). Intron or UTR retention during RNA processing could affect nuclear retention, nucleocytoplasmic transport, and cytoplasmic turnover and is a conserved regulatory mechanism that affects a wide range of cellular events (53, 54). Our luciferase-based assays suggest that the presence of the shorter microsatellite influences mRNA processing and thus promotes reduced protein expression. Although more experiments are needed to clarify the mechanism, we speculate that it involves RNA stability and/or transcriptional termination efficiency.
The current paradigm of RA management holds that disease is better controlled when very early DMARD treatment is established (55). New lines of research indicate that early treatment of patients is associated with better disease outcomes (56); however, the clinical spectrum of RA is very heterogeneous and the discovery of new severity biomarkers will be needed to establish tailored treatments. Our data suggest that measuring SOCS1 mRNA levels could be a technically reliable and robust procedure with which to predict those patients who will have a poorer clinical evolution. This is supported by data obtained in the discovery population, validated in a separate group of patients selected using more stringent RA criteria, and replicated in two independent populations for early and established RA.
Our observations, nonetheless, indicate some limitations to implementing the use of this biomarker, including determining the appropriate baseline low levels of SOCS1. Prior adjustment by age and glucocorticoid use is also needed to allow efficient patient classification. In addition, SOCS1 expression did not discriminate between patients in remission regarding pre-arthritis stages or treatment effects. Genotyping of rs11074956, rs4780355, and rs243323, whose minor alleles were associated with lower SOCS1 baseline levels, might overcome these limitations.
Some authors propose that personalized medicine for RA is currently not possible (57), and data from daily clinical practice suggest that earlier treatment for inflammation improves clinical outcome (5). Our findings point to new candidates for the development of composite biomarker indices with greater predictive ability for RA severity that could be useful to guide patient care from establishment of the diagnosis.
Data Availability Statement
The datasets generated for this study can be found in the ClinVar, SCV000999906.
Ethics Statement
The studies involving human participants were reviewed and approved by The PEARL study was approved by the local Research Ethics Committee (PI-518). All patients gave informed consent. The Leeds cohorts approval was obtained from local Research Ethics Committees for both groups (REC: 07/S0703/68, 10/H1307/138). Informed consent was obtained from all individuals. The patients/participants provided their written informed consent to participate in this study.
Author Contributions
AL, RV, IS, NA, PL, CM, and RG performed the experiments. IS, AM, and JM analyzed the genetic variability of SOCS1 gene from Immunochip data. PE, EV, RM, and FP recruited the patients of the Leeds cohorts and obtained the samples. AT-M, AO, and IG-A recruited the patients of the PEARL study and obtained the samples. RV, FP, and IG-A performed the statistical analysis. RV, IG-A, and MM wrote the manuscript. IG-A and MM conceived the study. All authors contributed to the article and approved the submitted version.
Funding
This work was supported in part by grants from the Spanish Ministry of Economy and Competitiveness/FEDER (SAF 2017-82940-R; PI14/00442, PI17/00027, PI18/00371), the RETICS Program of the Instituto de Salud Carlos III (RIER: RD16/0012/0006; RD16/0012/0008; RD16/0012/0011; RD16/0012/0013) and the European Union (Masterswitch FP7-Health-2007-B-223404). We also obtained funds from the Spanish Natonal Research Council for open access publication fees.
Conflict of Interest
The authors declare that the research was conducted in the absence of any commercial or financial relationships that could be construed as a potential conflict of interest.
Acknowledgments
We thank C. Mark for editorial assistance.
Supplementary Material
The Supplementary Material for this article can be found online at: https://www.frontiersin.org/articles/10.3389/fimmu.2020.01336/full#supplementary-material
References
1. van Steenbergen HW, Huizinga TW, van der Helm-van Mil AH. The preclinical phase of rheumatoid arthritis: what is acknowledged and what needs to be assessed? Arthritis Rheum. (2013) 65:2219–32. doi: 10.1002/art.38013
2. Boers M. Understanding the window of opportunity concept in early rheumatoid arthritis. Arthritis Rheum. (2003) 48:1771–4. doi: 10.1002/art.11156
3. van Nies JA, Krabben A, Schoones JW, Huizinga TW, Kloppenburg M, van der Helm-van Mil AH. What is the evidence for the presence of a therapeutic window of opportunity in rheumatoid arthritis? A systematic literature review. Ann Rheum Dis. (2014) 73:861–70. doi: 10.1136/annrheumdis-2012-203130
4. Toledano E, Ortiz AM, Ivorra-Cortes J, Montes N, Beltran A, Rodriguez-Rodriguez L, et al. Are rheumatologists adhering to the concepts window of opportunity and treat-to-target? Earlier and more intense disease-modifying anti-rheumatic drug treatment over time in patients with early arthritis in the PEARL study. Clin Exp Rheumatol. (2018) 36:382–8.
5. Robinson WH, Mao R. Biomarkers to guide clinical therapeutics in rheumatology? Curr Opin Rheumatol. (2016) 28:168–75. doi: 10.1097/BOR.0000000000000250
6. Burska Boissinot M, Ponchel F. Cytokines as biomarkers in rheumatoid arthritis. Mediators Inflamm. (2014) 2014:545493. doi: 10.1155/2014/545493
7. McInnes IB, Schett G. Pathogenetic insights from the treatment of rheumatoid arthritis. Lancet. (2017) 389:2328–2337. doi: 10.1016/S0140-6736(17)31472-1
8. Villarino AV, Kanno Y, Ferdinand JR, O'Shea JJ. Mechanisms of Jak/STAT signaling in immunity and disease. J Immunol. (2015) 194:21–7. doi: 10.4049/jimmunol.1401867
9. Malemud CJ. Intracellular Signaling Pathways in Rheumatoid Arthritis. J Clin Cell Immunol. (2013) 4:160. doi: 10.4172/2155-9899.1000160
10. Alexander WS. Suppressors of cytokine signalling (SOCS) in the immune system. Nat Rev Immunol. (2002) 2:410–6. doi: 10.1038/nri818
11. Nicola NA, Greenhalgh CJ. The suppressors of cytokine signaling (SOCS) proteins: important feedback inhibitors of cytokine action. Exp Hematol. (2000) 28:1105–12. doi: 10.1016/S0301-472X(00)00525-7
12. Yasukawa H, Sasaki A, Yoshimura A. Negative regulation of cytokine signaling pathways. Annu Rev Immunol. (2000) 18:143–64. doi: 10.1146/annurev.immunol.18.1.143
13. Krebs DL, Hilton DJ. SOCS proteins: negative regulators of cytokine signaling. Stem Cells. (2001) 19:378–87. doi: 10.1634/stemcells.19-5-378
14. Acosta-Herrera M, Kerick M, Gonzalez-Serna D, Myositis Genetics C, Scleroderma Genetics C, et al. Genome-wide meta-analysis reveals shared new loci in systemic seropositive rheumatic diseases. Ann Rheum Dis. (2019) 78:311–9. doi: 10.1136/annrheumdis-2018-214127
15. Isomaki P, Alanara T, Isohanni P, Lagerstedt A, Korpela M, Moilanen T, et al. The expression of SOCS is altered in rheumatoid arthritis. Rheumatology. (2007) 46:1538–46. doi: 10.1093/rheumatology/kem198
16. Gonzalez-Alvaro Ortiz AM, Alvaro-Gracia JM, Castaneda S, Diaz-Sanchez B, Carvajal I, et al. Interleukin 15 levels in serum may predict a severe disease course in patients with early arthritis. PLoS ONE. (2011) 6:e29492. doi: 10.1371/journal.pone.0029492
17. Arnett FC, Edworthy SM, Bloch DA, McShane DJ, Fries JF, Cooper NS, et al. The American Rheumatism Association 1987 revised criteria for the classification of rheumatoid arthritis. Arthritis Rheum. (1988) 31:315–24. doi: 10.1002/art.1780310302
18. Verpoort KN, van Dongen H, Allaart CF, Toes RE, Breedveld FC, Huizinga TW. Undifferentiated arthritis–disease course assessed in several inception cohorts. Clin Exp Rheumatol. (2004) 22:S12–7.
19. Livak KJ, Schmittgen TD. Analysis of relative gene expression data using real-time quantitative PCR and the 2[-Delta Delta C(T)] Method. Methods. (2001) 25:402–8. doi: 10.1006/meth.2001.1262
20. Thaker YR, Schneider H, Rudd CE. TCR and CD28 activate the transcription factor NF-kappaB in T-cells via distinct adaptor signaling complexes. Immunol Lett. (2015) 163:113–9. doi: 10.1016/j.imlet.2014.10.020
21. Chen X, Xu Q, Li X, Wang L, Yang L, Chen Z, et al. Molecular and Phenotypic Characterization of Nine Patients with STAT1 GOF Mutations in China. J Clin Immunol. (2019) 40:82–95. doi: 10.1007/s10875-019-00688-3
22. Fransen J, van Riel PL. The disease activity score and the EULAR response criteria. Clin Exp Rheumatol. (2005) 23:S93–9.
23. Gonzalez-Alvaro Ortiz AM, Seoane IV, Garcia-Vicuna R, Martinez C, Gomariz RP. Biomarkers predicting a need for intensive treatment in patients with early arthritis. Curr Pharm Des. (2015) 21:170–81. doi: 10.2174/1381612820666140825123104
24. Smolen J, Breedveld F, Burmester G, Bykerk V, Dougados M, Emery P, et al. Treating rheumatoid arthritis to target: 2014 update of the recommendations of an international task force. Ann Rheum Dis. (2016) 75:3–15. doi: 10.1136/annrheumdis-2015-207524
25. Cortes A, Brown MA. Promise and pitfalls of the immunochip. Arthritis Res Ther. (2011) 13:101. doi: 10.1186/ar3204
26. Martinez A, Perdigones N, Cenit MC, Espino L, Varade J, Lamas JR, et al. Chromosomal region 16p13: further evidence of increased predisposition to immune diseases. Ann Rheum Dis. (2010) 69:309–11. doi: 10.1136/ard.2008.098376
27. Ellinghaus D, Ellinghaus E, Nair RP, Stuart PE, Esko T, Metspalu A, et al. Combined analysis of genome-wide association studies for Crohn disease and psoriasis identifies seven shared susceptibility loci. Am J Hum Genet. (2012) 90:636–47. doi: 10.1016/j.ajhg.2012.02.020
28. Ceuppens JL, Baroja ML, Lorre K, Van Damme J, Billiau A. Human T cell activation with phytohemagglutinin. The function of IL-6 as an accessory signal. J Immunol. (1988) 141:3868–74.
29. Giotis ES, Ross CS, Robey RC, Nohturfft A, Goodbourn S, Skinner MA. Constitutively elevated levels of SOCS1 suppress innate responses in DF-1 immortalised chicken fibroblast cells. Sci Rep. (2017) 7:17485. doi: 10.1038/s41598-017-17730-2
30. Li J, Wan Y, Guo Q, Zou L, Zhang J, Fang Y, et al. Altered microRNA expression profile with miR-146a upregulation in CD4+ T cells from patients with rheumatoid arthritis. Arthritis Res Ther. (2010) 12:R81. doi: 10.1186/ar3006
31. Gutierrez-Arcelus M, Rich SS, Raychaudhuri S. Autoimmune diseases - connecting risk alleles with molecular traits of the immune system. Nat Rev Genet. (2016) 17:160–74. doi: 10.1038/nrg.2015.33
32. Vandenbroeck K. Cytokine gene polymorphisms and human autoimmune disease in the era of genome-wide association studies. J Interferon Cytokine Res. (2012) 32:139–51. doi: 10.1089/jir.2011.0103
33. Singh JA, Saag KG, Bridges SL Jr, Akl EA, Bannuru RR, Sullivan MC, et al. 2015 American College of rheumatology guideline for the treatment of rheumatoid arthritis. Arthritis Rheumatol. (2016) 68:1–26. doi: 10.1002/art.39480
34. Smolen JS, Landewe R, Bijlsma J, Burmester G, Chatzidionysiou K, Dougados M, et al. EULAR recommendations for the management of rheumatoid arthritis with synthetic and biological disease-modifying antirheumatic drugs: 2016 update. Ann Rheum Dis. (2017) 76:960–77. doi: 10.1136/annrheumdis-2016-210715
35. Rider P, Carmi Y, Cohen I. Biologics for Targeting Inflammatory Cytokines, Clinical Uses, and Limitations. Int J Cell Biol. (2016) 2016:9259646. doi: 10.1155/2016/9259646
36. Sundberg TB, Xavier RJ, Schreiber SL, Shamji AF. Small-molecule control of cytokine function: new opportunities for treating immune disorders. Curr Opin Chem Biol. (2014) 23:23–30. doi: 10.1016/j.cbpa.2014.08.013
37. Ungureanu D, Saharinen P, Junttila I, Hilton DJ, Silvennoinen O. Regulation of Jak2 through the ubiquitin-proteasome pathway involves phosphorylation of Jak2 on Y1007 and interaction with SOCS-1. Mol Cell Biol. (2002) 22:3316–26. doi: 10.1128/MCB.22.10.3316-3326.2002
38. Egan PJ, Lawlor KE, Alexander WS, Wicks IP. Suppressor of cytokine signaling-1 regulates acute inflammatory arthritis and T cell activation. J Clin Invest. (2003) 111:915–24. doi: 10.1172/JCI16156
39. de Hooge AS, van de Loo FA, Koenders MI, Bennink MB, Arntz OJ, Kolbe T, et al. Local activation of STAT-1 and STAT-3 in the inflamed synovium during zymosan-induced arthritis: exacerbation of joint inflammation in STAT-1 gene-knockout mice. Arthritis Rheum. (2004) 50:2014–23. doi: 10.1002/art.20302
40. Qin H, Wilson CA, Lee SJ, Benveniste EN. IFN-beta-induced SOCS-1 negatively regulates CD40 gene expression in macrophages and microglia. FASEB J. (2006) 20:985–7. doi: 10.1096/fj.05-5493fje
41. De Sepulveda P, Okkenhaug K, Rose JL, Hawley RG, Dubreuil P, Rottapel R. Socs1 binds to multiple signalling proteins and suppresses steel factor-dependent proliferation. EMBO J. (1999) 18:904–15. doi: 10.1093/emboj/18.4.904
42. Kinjyo Hanada T, Inagaki-Ohara K, Mori H, Aki D, Ohishi M, et al. SOCS1/JAB is a negative regulator of LPS-induced macrophage activation. Immunity. (2002) 17:583–91. doi: 10.1016/S1074-7613(02)00446-6
43. Mansell A, Smith R, Doyle SL, Gray P, Fenner JE, Crack PJ, et al. Suppressor of cytokine signaling 1 negatively regulates Toll-like receptor signaling by mediating Mal degradation. Nat Immunol. (2006) 7:148–55. doi: 10.1038/ni1299
44. Nakagawa R, Naka T, Tsutsui H, Fujimoto M, Kimura A, Abe T, et al. SOCS-1 participates in negative regulation of LPS responses. Immunity. (2002) 17:677–87. doi: 10.1016/S1074-7613(02)00449-1
45. Wang N, Liang H, Zen K. Molecular mechanisms that influence the macrophage m1-m2 polarization balance. Front Immunol. (2014) 5:614. doi: 10.3389/fimmu.2014.00614
46. Yu CF, Peng WM, Schlee M, Barchet W, Eis-Hubinger AM, Kolanus W, et al. SOCS1 and SOCS3 Target IRF7 Degradation To Suppress TLR7-Mediated Type I IFN Production of Human Plasmacytoid Dendritic Cells. J Immunol. (2018) 200:4024–35. doi: 10.4049/jimmunol.1700510
47. Yamana J, Yamamura M, Okamoto A, Aita T, Iwahashi M, Sunahori K, et al. Resistance to IL-10 inhibition of interferon gamma production and expression of suppressor of cytokine signaling 1 in CD4+ T cells from patients with rheumatoid arthritis. Arthritis Res Ther. (2004) 6:R567–77. doi: 10.1186/ar1445
48. Li X, Tian F, Wang F. Rheumatoid arthritis-associated microRNA-155 targets SOCS1 and upregulates TNF-alpha and IL-1beta in PBMCs. Int J Mol Sci. (2013) 14:23910–21. doi: 10.3390/ijms141223910
49. Lai NS, Chou JL, Chen GC, Liu SQ, Lu MC, Chan MW. Association between cytokines and methylation of SOCS-1 in serum of patients with ankylosing spondylitis. Mol Biol Rep. (2014) 41:3773–80. doi: 10.1007/s11033-014-3242-2
50. Sznajder LJ, Michalak M, Taylor K, Cywoniuk P, Kabza M, Wojtkowiak-Szlachcic A, et al. Mechanistic determinants of MBNL activity. Nucleic Acids Res. (2016) 44:10326–42. doi: 10.1093/nar/gkw915
51. Punga T, Buhler M. Long intronic GAA repeats causing Friedreich ataxia impede transcription elongation. EMBO Mol Med. (2010) 2:120–9. doi: 10.1002/emmm.201000064
52. Rosonina E, Kaneko S, Manley JL. Terminating the transcript: breaking up is hard to do. Genes Dev. (2006) 20:1050–6. doi: 10.1101/gad.1431606
53. Braunschweig U, Barbosa-Morais NL, Pan Q, Nachman EN, Alipanahi B, Gonatopoulos-Pournatzis T, et al. Widespread intron retention in mammals functionally tunes transcriptomes. Genome Res. (2014) 24:1774–86. doi: 10.1101/gr.177790.114
54. Jung H, Lee D, Lee J, Park D, Kim YJ, Park WY, et al. Intron retention is a widespread mechanism of tumor-suppressor inactivation. Nat Genet. (2015) 47:1242–8. doi: 10.1038/ng.3414
55. van Nies JA, Tsonaka R, Gaujoux-Viala C, Fautrel B, van der Helm-van Mil AH. Evaluating relationships between symptom duration and persistence of rheumatoid arthritis: does a window of opportunity exist? Results on the Leiden early arthritis clinic and ESPOIR cohorts. Ann Rheum Dis. (2015) 74:806–12. doi: 10.1136/annrheumdis-2014-206047
56. Van Steenbergen HW, Da Silva JA, Huizinga TW, Van Der Helm-van AH. Preventing progression from arthralgia to arthritis: targeting the right patients. Nat Rev Rheumatol. (2018) 14:32–41. doi: 10.1038/nrrheum.2017.185
Keywords: rheumatoid arthritis, disease activity, cytokines, inflammation, biomarkers
Citation: Lamana A, Villares R, Seoane IV, Andrés N, Lucas P, Emery P, Vital EM, Triguero-Martínez A, Marquez A, Ortiz AM, Maxime R, Martínez C, Martín J, Gomariz RP, Ponchel F, González-Álvaro I and Mellado M (2020) Identification of a Human SOCS1 Polymorphism That Predicts Rheumatoid Arthritis Severity. Front. Immunol. 11:1336. doi: 10.3389/fimmu.2020.01336
Received: 11 December 2019; Accepted: 26 May 2020;
Published: 26 June 2020.
Edited by:
Dimitrios Petrou Bogdanos, University of Thessaly, GreeceReviewed by:
Mrinal K. Sarkar, University of Michigan, United StatesJillian M. Richmond, University of Massachusetts Medical School, United States
Copyright © 2020 Lamana, Villares, Seoane, Andrés, Lucas, Emery, Vital, Triguero-Martínez, Marquez, Ortiz, Maxime, Martínez, Martín, Gomariz, Ponchel, González-Álvaro and Mellado. This is an open-access article distributed under the terms of the Creative Commons Attribution License (CC BY). The use, distribution or reproduction in other forums is permitted, provided the original author(s) and the copyright owner(s) are credited and that the original publication in this journal is cited, in accordance with accepted academic practice. No use, distribution or reproduction is permitted which does not comply with these terms.
*Correspondence: Isidoro González-Álvaro, isidoro.ga@ser.es; Mario Mellado, mmellado@cnb.csic.es
†These authors have contributed equally to this work