- 1College of Traditional Chinese Medicine, Southern Medical University, Guangzhou, China
- 2National Institute of Traditional Chinese Medicine Constitution and Preventive Medicine, Beijing University of Chinese Medicine, Beijing, China
- 3Department of Respiratory Medicine, Ningbo Hospital of Traditional Chinese Medicine, Zhejiang University of Chinese Medicine, Ningbo, China
- 4The Second Affiliated Hospital of Henan University of Chinese Medicine, Zhengzhou, China
Background: Asthma is a chronic inflammatory disorder arising from incompletely understood heterogenic gene–environment interactions. This study aims to investigate causal relationships among gut microbiota, skin microbiota, plasma metabolomics, white blood cells subtype, immune cells, inflammatory proteins, inflammatory cytokines, and asthma.
Methods: First, two-sample Mendelian randomization analysis was used to identify causal relationships. The summary statistics of 412 gut microbiota traits (N = 7 738), 150 skin microbiota traits (N = 579), 1 400 plasma metabolite traits (N = 8 299), white blood cells subtype counts (N = 746 667), 731 immune cell traits (N = 3 669), 91 circulating inflammatory proteins (N = 14 744), 41 inflammatory cytokine traits (N = 8 293), and asthma traits (N = 244 562) were obtained from publicly available genome-wide association studies. Inverse–variance weighted regression was used as the primary Mendelian randomization method. A series of sensitivity analyses was performed to test the robustness of causal estimates. Subsequently, mediation analysis was performed to identify the pathway from gut or skin microbiota to asthma mediated by plasma metabolites, immune cells, and inflammatory proteins.
Results: Mendelian randomization revealed the causal effects of 31 gut bacterial features (abundances of 19 bacterial pathways and 12 microbiota), 10 skin bacterial features, 108 plasma metabolites (81 metabolites and 27 ratios), 81 immune cells, five circulating inflammatory proteins, and three inflammatory cytokines and asthma. Moreover, the mediation analysis results supported the mediating effects of one plasma metabolite, five immunophenotypes, and one inflammatory protein on the gut or skin microbiota in asthma pathogenesis.
Conclusion: The findings of this study support a causal relationship among gut microbiota, skin microbiota, plasma metabolites, immune cells, inflammatory proteins, inflammatory cytokines, and asthma. Mediating pathways through which the above factors may affect asthma were proposed. The biomarkers and mediation pathways identified in this work provide new insights into the mechanism of asthma and contribute to its prevention and treatment.
1 Introduction
Asthma is a heterogeneous chronic respiratory disease defined by a history of respiratory symptoms (e.g., wheezing, shortness of breath, chest tightness, and coughing) that vary over time and in intensity together with variable expiratory airflow limitation. It affects nearly 10% of the global population, and its prevalence has continued to increase worldwide (1). Its prevalence, especially among children, has increased in numerous countries (2). This condition also presents challenges in terms of treatment efficacy and carries a substantial risk of exacerbation (3). Asthma imposes a significant disease burden, affecting all ages with increased mortality and reduced quality of life. Its pathogenesis, influenced by genetic and environmental factors, remains unclear, with genetic contributions estimated at 25%–80% (4). Given that asthma is a crucial public health concern associated with a high prevalence of morbidity and mortality, the study of its pathogenesis has certain importance.
The human gut microbiota is a dynamic ecosystem of microorganisms that interact with each other and the host. Dysbiosis not only impacts gut immunity but also influences lung health, contributing to the gut–lung axis concept (5). Gut microbiota influence lung immunity through immune cell differentiation and metabolite production affecting distal sites (6). The gut microbiota influences asthma susceptibility, with studies showing increased histamine-secreting microbes in asthma patients (7). In addition, research suggests that gut microbiota variation may help prevent or alleviate asthma by modulating inflammation, producing short-chain fatty acids, and regulating T cells (8). For example, the perturbed gut microbiota triggered by antibiotic use in individuals with asthma can be characterized by an exacerbated T helper (Th) 2 and Th1/Th17 immune response and diminished Treg population (9).
The mechanisms underlying the link between gut microbiota and asthma involve the complex metabolic and immune interactions of microbes, metabolites, and host immune responses (10). Moreover, gut microbiota is thought to play an important role in altering lung function, and several contributing pathways (Treg, iNKT, and Th17 cells) have been identified (6). Plasma metabolomics has identified key asthma-related metabolites (e.g., acetate, adenosine, alanine, and succinate) linked to hypoxia, oxidative stress, immunity, and lipid metabolism (11), with pediatric cases showing reduced citrate, ketone bodies, histidine, and glutamine (12), and aberrant purine metabolism in allergic asthma (13). Asthma arises from interactions between structural and immune cells triggered by environmental exposures (14), leading to airway obstruction mediated by chronic inflammation involving DCs, eosinophils, neutrophils, lymphocytes, ILCs, and mast cells. Altered microbiota disrupts immune homeostasis, impacting tolerance and inflammation (15). Airway inflammation drives hyperreactivity, mucus production, smooth muscle proliferation, and angiogenesis, worsening obstruction and lung function decline (16). Th2 CD4 activation promotes cytokine release (IL-4, IL-5, IL-13), leading to IgE synthesis, mast cell activation, and eosinophilic recruitment (17), with Th2–high inflammation commonly observed in allergic asthma (18).
Skin is colonized by diverse microbial communities and can be influenced over time in response to environmental factors. Skin microbiome plays an important role in tissue homeostasis and local immunity (19). One study found that Russian children had a higher abundance of Acinetobacter in their skin and nasal passages, which led to fewer cases of asthma and allergies, than Finnish individuals (20). A certain correlation exists between skin microbiota and environmental biodiversity in patients with allergies (21) Although the mechanistic role of skin dysbiosis in asthma pathogenesis remains uncertain, changes in the environment initiate dysbiosis in the skin along with the lung and gut, inducing functional and compositional changes in microbiota; such changes can affect the immunological mechanisms of allergic diseases, including asthma (22).
Observational studies make inferring true causality difficult given the presence of reverse causality and potential confounding factors. Mendelian randomization (MR) has a unique advantage in exploring the potential causal relationship between two traits on the basis of the Mendelian laws of inheritance and minimizes the effect of confounding factors on causal estimation (23). Mediation analysis can further evaluate the effects of an exposure on an outcome through a mediator (24). In contrast to airway microbiota, whose potential to modulate asthma is well recognized, gut and skin microbiota are anatomically distinct from the site of asthma occurrence but still play an important role in asthma. Meanwhile, the understanding of the effect of the skin microbiome on asthma is limited. In this study, we conducted a MR study based on recently published summary datasets of large genome-wide association studies (GWASs) to evaluate the causal relationship among gut microbiota, skin microbiota, plasma metabolites, circulating white blood cells (WBC), immune cells, circulating inflammatory proteins, inflammatory cytokines, and asthma and identify pathways from the gut or skin microbiota to asthma mediated by plasma metabolites, immune cells, and inflammatory proteins.
2 Methods
2.1 Study design
The study flowchart is illustrated in Figure 1. First, published GWAS summary data that included traits, such as gut microbiota, skin microbiota, plasma metabolites, circulating WBC, circulating inflammatory proteins, inflammatory cytokines, and asthma, were obtained (Supplementary Table S1). Second, two-sample MR analyses were used to evaluate the causal relationship among gut microbiota, skin microbiota, plasma metabolites, circulating WBC, immune cell traits, circulating inflammatory proteins, inflammatory cytokines, and asthma. In addition, gut microbiota, skin microbiota, and asthma were further analyzed through bidirectional two-sample MR. Furthermore, linkage disequilibrium score regression (LDSC) was performed to identify causal microbiota and gut bacterial pathways. Finally, two-step analyses were used to identify the mediation effect of plasma metabolites, circulating inflammatory proteins, and inflammatory cytokines on the relationship among gut microbiota, skin microbiota, and asthma. The Strengthening the Reporting of Observational Studies in Epidemiology Using MR checklist was completed for this observational study (Supplementary Table S2) (25). The GWAS data were obtained from publicly available datasets published between 2020 and 2023, and all data analyses were conducted in 2024.
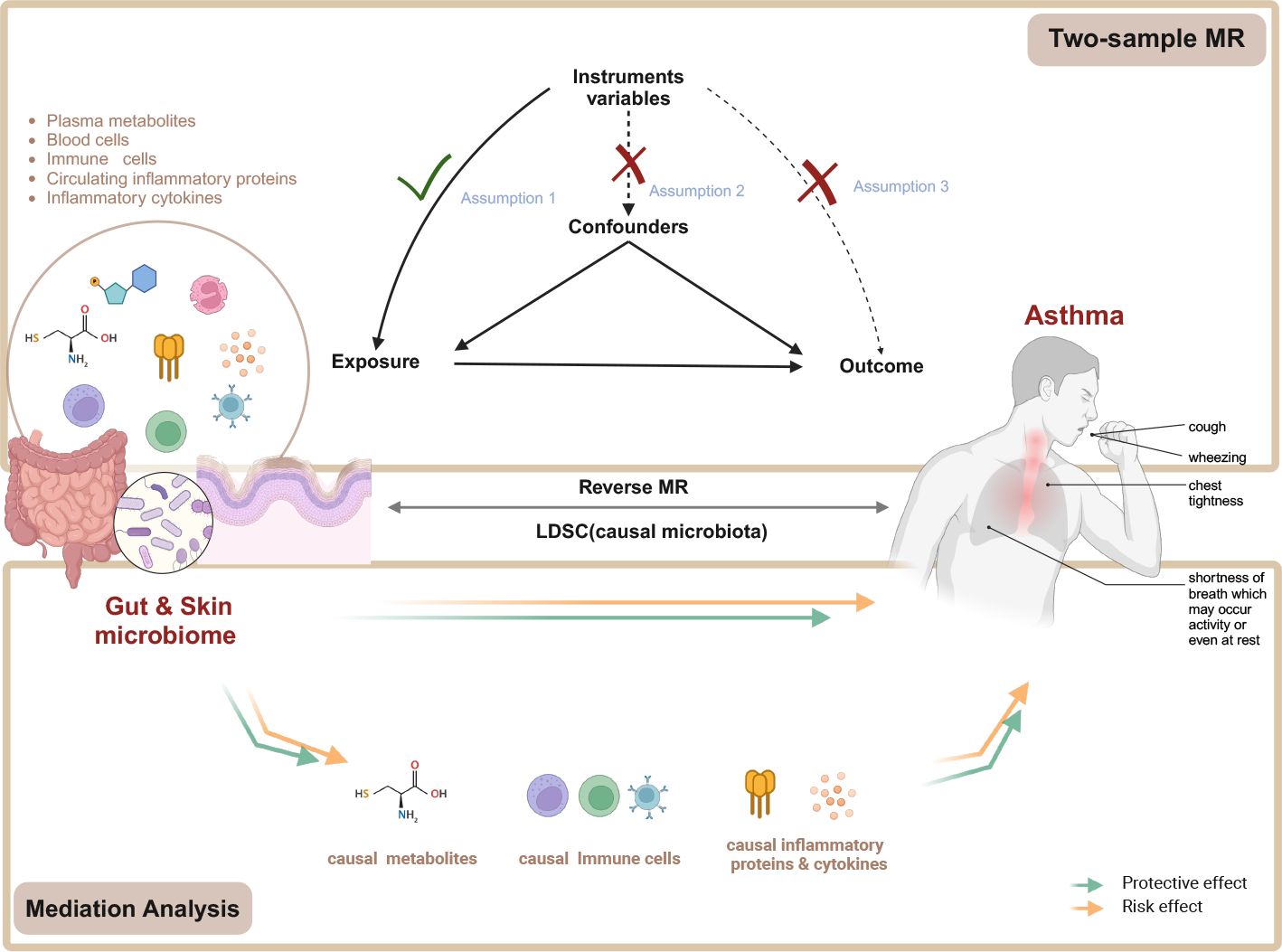
Figure 1. Flow chart of the study. Mendelian randomization study rationale: assumption 1, genetic instruments are associated with exposure; assumption 2, genetic instruments are not associated with confounders; assumption 3, genetic instruments are not associated with outcome, and genetic instruments act on outcome only through exposure. MR, Mendelian randomization. Created in BioRender. Wenqian, G. (2025) https://BioRender.com/w65a330.
2.2 Data sources
2.2.1 Source of GWAS data on gut microbiota
A GWAS dataset that included 7738 Dutch Microbiome Project participants whose microbiota data were quality-controlled with LifeLines (26) was used. A total of 207 taxa (five phyla, 10 classes, 13 orders, 26 families, 48 genera, and 105 species) and 205 pathways representing microbial composition and function were included in the subsequent analysis.
2.2.2 Source of GWAS data on skin microbiota
Data on skin microbiota in this study were obtained from the participants of two cross-sectional, population-based German cohorts, KORA FF4 (individuals = 324) and PopGen (individuals = 273), with a total of 1656 skin samples (27). Skin samples were taken from dry (dorsal and volar forearm [PopGen]), moist (antecubital fossa [KORA FF4 and PopGen]), and sebaceous (retro auricular fold [KORA FF4] and forehead [PopGen]) skin microenvironments. Microbial community profiles were obtained from the sequencing of the V1–V2 regions from the 16 S ribosomal RNA gene. Genome-wide association analyses were conducted on the univariate relative abundances of individual bacteria (amplicon sequence variants [ASVs]) and 79 nonredundant taxonomic groups ranging from genus to phylum levels.
2.2.3 Source of GWAS data on metabolites
The summary statistics of plasma metabolomics were acquired from the GWAS Catalog (https://www.ebi.ac.uk/gwas/) with the study accession numbers GCST90199621–GCST90201020. The latest study included 1091 plasma metabolites and 309 metabolite ratios from 8299 European individuals and involved 8299 samples and approximately 150 000 single-nucleotide polymorphism (SNP) loci (28). The above study included 850 known metabolites out of 1091 plasma metabolites, which could be divided into eight broad metabolic groups: lipids (395), amino acids (210), xenobiotics (130), nucleotides (33), cofactors and vitamins (31), carbohydrates (22), peptides (21), and energy (8). The remaining metabolites were partially characterized molecules (21) and unknown (220).
2.2.4 Source of GWAS data on WBC counts
Effect estimates for SNPs associated with WBC subtype counts, which included basophils, eosinophils, monocytes, lymphocytes, and neutrophils, were obtained from the Blood Cell Consortium meta-analysis involving 746 667 participants, including 184 424 individuals of non-EUR descent (29).
2.2.5 Source of immunity-wide GWAS data
The GWAS summary statistics for each immune trait used for cellular subpopulation analyses are publicly available from the GWAS Catalog (accession numbers GCST0001391–GCST0002121) (30). A total of 731 immunophenotypes, including absolute cell (AC) counts (n = 118), median fluorescence intensities (MFI) reflecting surface antigen levels (n = 389), morphological parameters (MPs) (n = 32), and relative cell (RC) counts (n = 192), were included. Specifically, the MFI, AC, and RC features contained B cells, CDCs, mature stages of T cells, monocytes, myeloid cells, TBNK (T, B, and natural killer cells), and Treg panels, whereas the MP feature contained cDC and TBNK panels. The original GWAS on immune traits was performed by using data from 3757 individuals through flow cytometry with the Sardinian founder population (31).
2.2.6 Source of GWAS data on circulating inflammatory proteins
The summary statistics of plasma metabolomics were acquired from the GWAS Catalog (https://www.ebi.ac.uk/gwas/) under the study accession numbers GCST90274758–GCST90274848. The study conducted a genome-wide protein quantitative trait locus study of 91 plasma proteins on 14824 participants measured by using the Olink Target platform (32).
2.2.7 Source of GWAS data on inflammatory cytokines
GWAS data for 41 inflammatory cytokines were collected from the University of Bristol (https://data.bris.ac.uk/data/dataset). They included three Finnish cohort studies (N = 8,293): the Cardiovascular Risk in Young Finns Study, FINRISK1997, and FINRISK2002 (33, 34).
2.2.8 Source of GWAS data on asthma
The GWAS summary-level data of asthma were extracted from FinnGen Biobank by the IEU open GWAS project, which included 42 163 European-descent cases and 202 399 European-descent controls.
2.3 Instrumental variable selection
In MR analysis, Instrumental variables (IVs) were utilized as mediators between exposure factors and outcomes to explore the causal relationship between exposure and outcomes. IVs are generally genetic variations, among which SNPs are the most used.
Strict quality control was performed on the SNPs to select valid IVs for MR analysis. Three basic assumptions of IVs must be satisfied. 1) Relevance assumption: IVs are related to the exposure studied. 2) Independence assumption: IVs are not associated with confounding factors. 3) Exclusion assumption: IVs do not directly affect the outcome. They can only affect the outcome by influencing exposure factors.
Three core assumptions were followed to identify the IVs needed:
1. The genome-wide significance threshold of P < 5 × 10−8 is used as a potential tool variable related to each exposure trait. When few whole-genome significance loci were found in the original GWAS results (35, 36), a loose P value of 1 × 10−5 was used to select SNPs associated with gut microbiota and plasma metabolomics, and a P value of 5 × 10−5 was applied to select SNPs associated with skin microbiota as candidate IVs.
2. SNPs related to outcome variables were excluded (P < 0.05).
3. Independent SNPs were then clumped to a linkage disequilibrium threshold of r2 < 0.001 in accordance with the 1000 Genomes reference panel.
4. The MR–Egger and MR pleiotropy residual sum and outlier (MR–PRESSO) test was used to assess horizontal pleiotropy. The pleiotropy effect was eliminated by removing outliers (37).
5. The strength of the selected SNPs was assessed by using the F-statistic, and variants with F statistic < 10 were excluded from the analysis to avoid weak instrumental bias. The F statistic formula is F = [R2 × (n − k − 1)]/[k × (1 − R2)], where R2 is the portion of the exposure variance explained by the IVs, n is the sample size, and k represents the number of IVs (38).
6. Steiger filtering was used to remove variants with evidence of a stronger association with the outcome than its association with the exposure.
2.4 Statistical analysis
2.4.1 Two-sample MR analysis
A two-sample MR was conducted to assess the causal relationship among gut microbiota, skin microbiota, plasma metabolites, WBC subtype counts, immune cell traits, inflammatory proteins, inflammatory cytokines, and asthma. Various methods were employed to estimate MR effects, ensuring robustness. Inverse–variance weighted (IVW) served as the primary approach (39) and was supplemented by the MR–Egger (40), weighted mode (41), and weighted median (42) methods, each tailored to different assumptions of instrument validity.
Sensitivity analyses were performed to verify the robustness of causality and thus determine whether heterogeneity and pleiotropy within IVs could bias the MR results. Heterogeneity testing was performed by using the MR–Egger and IVW methods. Cochrane’s Q statistic was utilized to assess heterogeneity among genetic instruments, with P > 0.05 indicating the absence of significant heterogeneity. The MR–Egger regression equation was employed to evaluate the horizontal pleiotropy of genetic instruments, with P > 0.05 suggesting the absence of horizontal pleiotropy (43). Furthermore, a powerful method, MR–PRESSO (37), in the MR–PRESSO package was utilized to exclude possible horizontal pleiotropic outliers that could substantially affect estimation results. Steiger filtering (44) was conducted to remove variants with evidence of a stronger association with the outcome than its association with the exposure. To test the robustness of our MR results, we performed a leave-one-out sensitivity analysis, sequentially removing each SNP and recalculating the causal estimates.
Furthermore, in consideration of the potential chance to increase the overall type I error during multiple comparisons, false discovery rate (FDR) correction was implemented on the primary IVW results by using the Benjamini–Hochberg procedure. A significance threshold of FDR < 0.1 indicates a significant association, whereas PIVW < 0.05 but FDR > 0.1 implies a suggestive association (45). The source code used to analyze experiment results is publicly available at https://www.frontiersin.org/journals/immunology/articles/10.3389/fimmu.2025.1436888/abstract#supplementary-material.
All analyses were performed by using two-sample MR (version 0.6.0), MR (version 0.8.0), and MRPRESSO package (1.0) in R Software 4.3.3 (https://www.R-project.org).
2.4.2 Reverse MR analysis
Reverse MR analysis was performed to investigate whether asthma had a causal effect on gut microbiota and skin microbiota (PIVW < 0.05). In this context, asthma SNPs were regarded as IVs, asthma as exposure, and gut, and skin microbiological features as outcomes. The procedure for reverse MR analysis was similar to that used for MR analysis.
2.4.3 Metabolic pathway analysis
The HMDB IDs of known metabolites were retrieved from The Human Metabolome Database (https://hmdb.ca/) to identify known plasma metabolites (PIVW < 0.05), and enrichment analysis was conducted on the metabolic pathways associated with these metabolites by using MetaboAnalyst 5.0 (https://www.metaboanalyst.ca/). The pathway libraries selected for this analysis were the Small Molecule Pathway Database and Kyoto Encyclopedia of Genes and Genomes. The enrichment method employed was the hypergeometric test, and the significance level for metabolic pathway analysis was set at 0.01.
2.4.4 Linkage disequilibrium score regression analysis
Bivariate LDSC was performed by using GWAS summary statistics to show the genetic correlation among causal gut microbiota, causal skin microbiota, and asthma (46). χ2 statistics based on SNPs were regressed through LDSC regression to determine the heritability of a single trait and coheritability of two traits, which can identify whether confounding factors were present in MR analysis.
2.4.5 Mediation analysis
Mediation analysis can help explore potential mechanisms through which exposure affects outcomes. MR can be used to improve causal inference for mediation analysis (24). The mediation analysis in this study focused on asthma-related gut microbiota, skin microbiota, plasma metabolites, immune cell traits, inflammatory proteins, and inflammatory cytokines. The causal effect of exposure on the outcome (beta of IVW is c) was calculated before the two-step MR analysis was performed.
The mediation effect was calculated by using two-step MR as follows: mediation effect = beta (A) × beta (B). The total effect of gut microbiota on asthma was obtained through the previous two-sample MR and direct effect = (total effect − mediation effect). The mediation proportion was calculated by using the following formula: mediation proportion = (mediation effect/total effect) × 100%. The 95% confidence intervals (CI) for the mediation effects and proportions mediated were estimated by using the delta method.
3 Results
3.1 Causal effects of gut microbiota on asthma
Through two-sample MR analysis, we identified 31 suggestive associations between gut microbiota and asthma (PIVW < 0.05, FDR > 0.1; including the abundances of 19 gut bacterial pathways and 12 gut microbiota) (Figure 2; Supplementary Tables S3–5).
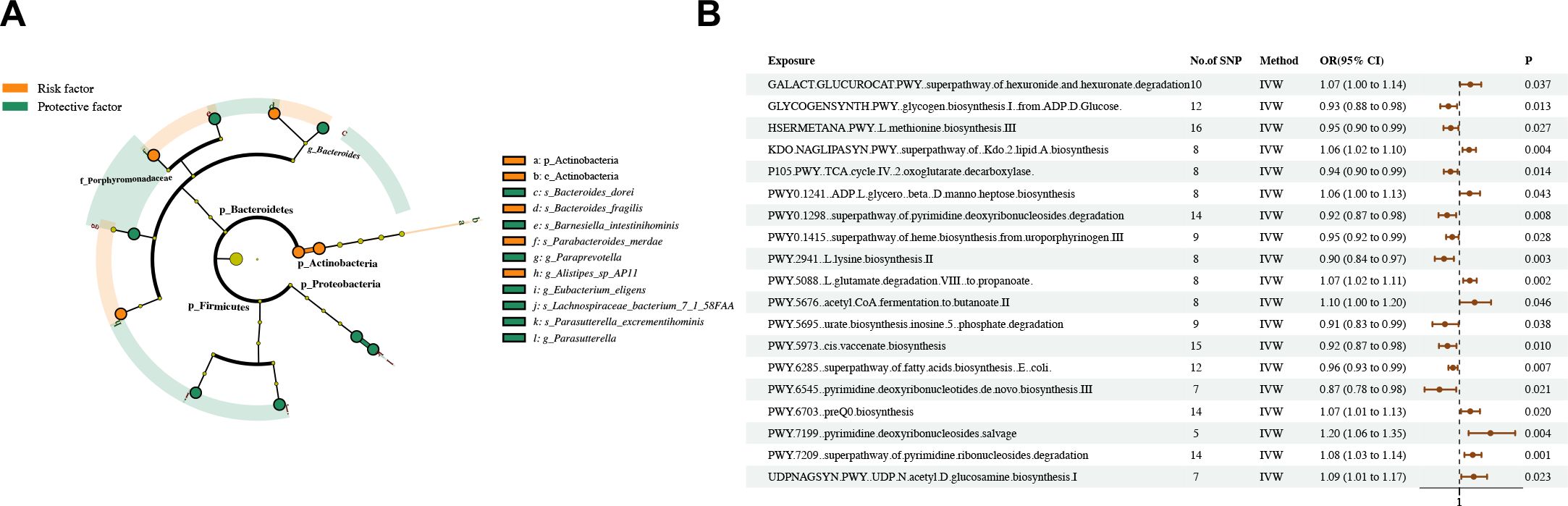
Figure 2. Causal estimates of MR between gut microbiota and asthma. (A) Estimates from the IVW analysis of gut microbiota abundance on asthma. (B) Estimates from the IVW analysis of gut bacterial pathway abundance on asthma.
The phylum Actinobacteria and class Actinobacteria in the phylum Actinobacteria had a positive causal relationship with asthma. The species Parabacteroides merdae, Alistipes sp AP11, and Bacteroides fragilis in the phylum Bacteroidetes had a positive causal relationship with asthma. By contrast, the genus Paraprevotella, species Barnesiella intestinihominis, and species Bacteroides dorei in the phylum Bacteroidetes had a negative causal relationship with asthma. The genus Parasutterella and species Parasutterella excrementihominis in the phylum Proteobacteria had a negative causal relationship with asthma. The species Eubacterium eligens and Lachnospiraceae bacterium 7 1 58FAA in the phylum Firmicutes had a negative causal relationship with asthma.
Dangerous and protective bacteria are partly independent and intertwined. The species Bacteroides dorei and Bacteroides_fragilis belong to the genus Bacteroides. The species Barnesiella intestinihominis and Parabacteroides merdae belong to the family Porphyromonadaceae. The above strains are members of the phylum Bacteroidetes together with the genus Paraprevotella and species Alistipes sp AP11. Meanwhile, the phyla Proteobacteria, Firmicutes, and Actinobacteria have an internal unified direction of influence on asthma (Figure 2A). The abundances of nine gut bacterial pathways had a positive causal relationship with asthma, whereas those of 10 gut bacterial pathways had a negative causal relationship (Figure 2B).
Sensitivity analysis further verified the robustness of the MR results (Supplementary Table S6). The Q statistics showed that only the species Bacteroides dorei had heterogeneity, whereas the remaining species did not show evidence of heterogeneity. Furthermore, the results of MR–Egger regression and MR–PRESSO did not reveal horizontal pleiotropy (dual validation was conducted on the abundances of 18 gut bacterial pathways and 11 gut microbiota). We did not find any reverse causality on the basis of the MR–Steiger test.
We conducted reverse MR and found a negative causal relationship between asthma and the family Streptococcaceae (odds ratio [OR] = 0.854, 95% CI [0.742–0.982], P = 0.027), genus Streptococcus (= 0.831, 95% CI [0.721–0.957], P = 0.010), family Oscillospiraceae (OR = 0.857, 95% CI [0.769–0.955], P = 0.005), genus Oscillibacter (OR = 0.857, 95% CI [0.769–0.955], P = 0.005), and species Oscillibacter unclassified (OR = 0.850, 95% CI [0.764–0.947], P = 0.003) (Supplementary Tables S7–10). Interestingly, all the above strains belonged to the phylum Firmicutes, which was negatively associated with asthma in forward and reverse analyses.
We then performed bivariate LDSC and identified a strong positive genetic correlation between the species Alistipes sp AP11 and asthma (rg = 0.06, P= 0.619) (Supplementary Tables S11).
3.2 Causal effects of skin microbiota on asthma
The results obtained through the IVW method suggested 10 causal relationships between skin microbiota and asthma (PIVW < 0.05, FDR > 0.1) (Figure 3; Supplementary Tables S12–14).
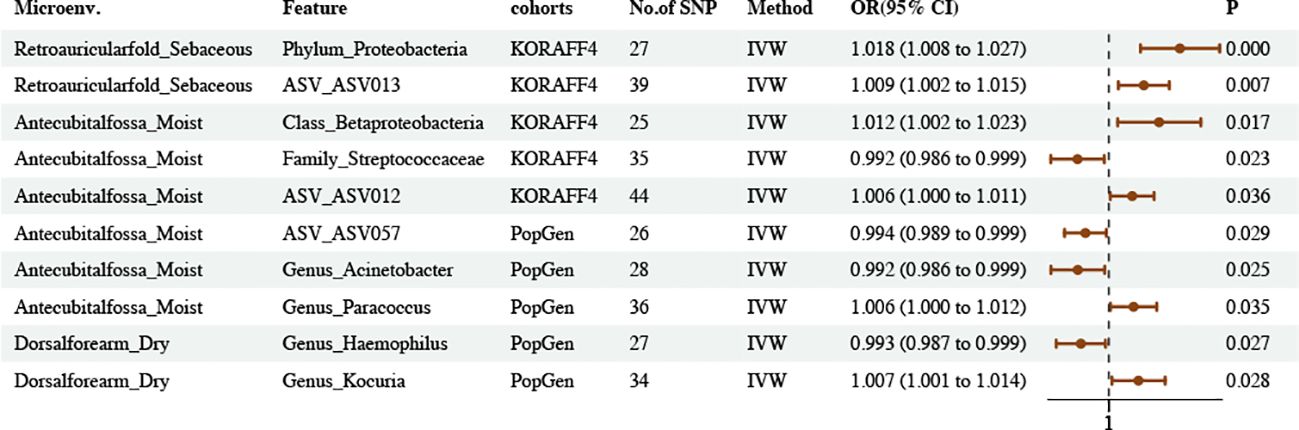
Figure 3. Causal estimates of MR between skin microbiota and asthma. Estimates from the IVW analysis of skin microbiota on asthma.
We found that the phylum Proteobacteria and ASV ASV013 in skin samples taken from a sebaceous (retro auricular fold [KORA FF4]) skin microenvironment had a positive causal relationship with asthma. The class Betaproteobacteria and ASV ASV012 in skin samples taken from a moist (antecubital fossa [KORA FF4]) skin microenvironment had a positive causal relationship with asthma. The genus Paracoccus in skin samples taken from a moist (antecubital fossa [PopGen]) skin microenvironment had a positive causal relationship with asthma. The genus Kocuria in skin samples taken from a dry (volar forearm [PopGen]) skin microenvironment had a positive causal relationship with asthma.
The family Streptococcaceae in skin samples taken from a moist (Antecubital fossa KORAFF4]) skin microenvironment had a negative causal relationship with asthma. The ASV ASV057 and genus Acinetobacter in skin samples taken from a moist (Antecubital fossa [PopGen]) skin microenvironment had a negative causal relationship with asthma. The genus Haemophilus in skin samples taken from a dry (dorsal forearm [PopGen]) skin microenvironment had a negative causal relationship with asthma.
We performed reverse MR and found no causal relationship between asthma and skin microbiota. Sensitivity analysis further verified the robustness of the MR results. Furthermore, MR–Egger regression and MR–PRESSO did not reveal horizontal pleiotropy. We did not find any reverse causality on the basis of the MR–Steiger test (Supplementary Table S15).
3.3 Causal effects of plasma metabolites on asthma
The results obtained on the basis of the IVW method suggested 108 causal relationships between plasma metabolomics and asthma (PIVW < 0.05, corresponding to 81 unique plasma metabolite levels and 27 unique metabolic ratios) (Figures 4A, B; Supplementary Figure S1, Supplementary Tables S16–18). Plasma metabolites included amino acids (17), carbohydrates (2), cofactors and vitamins (3), lipids (30), nucleotides (1), partially characterized molecules (1), peptides (3), xenobiotics (4), and unknown (20).
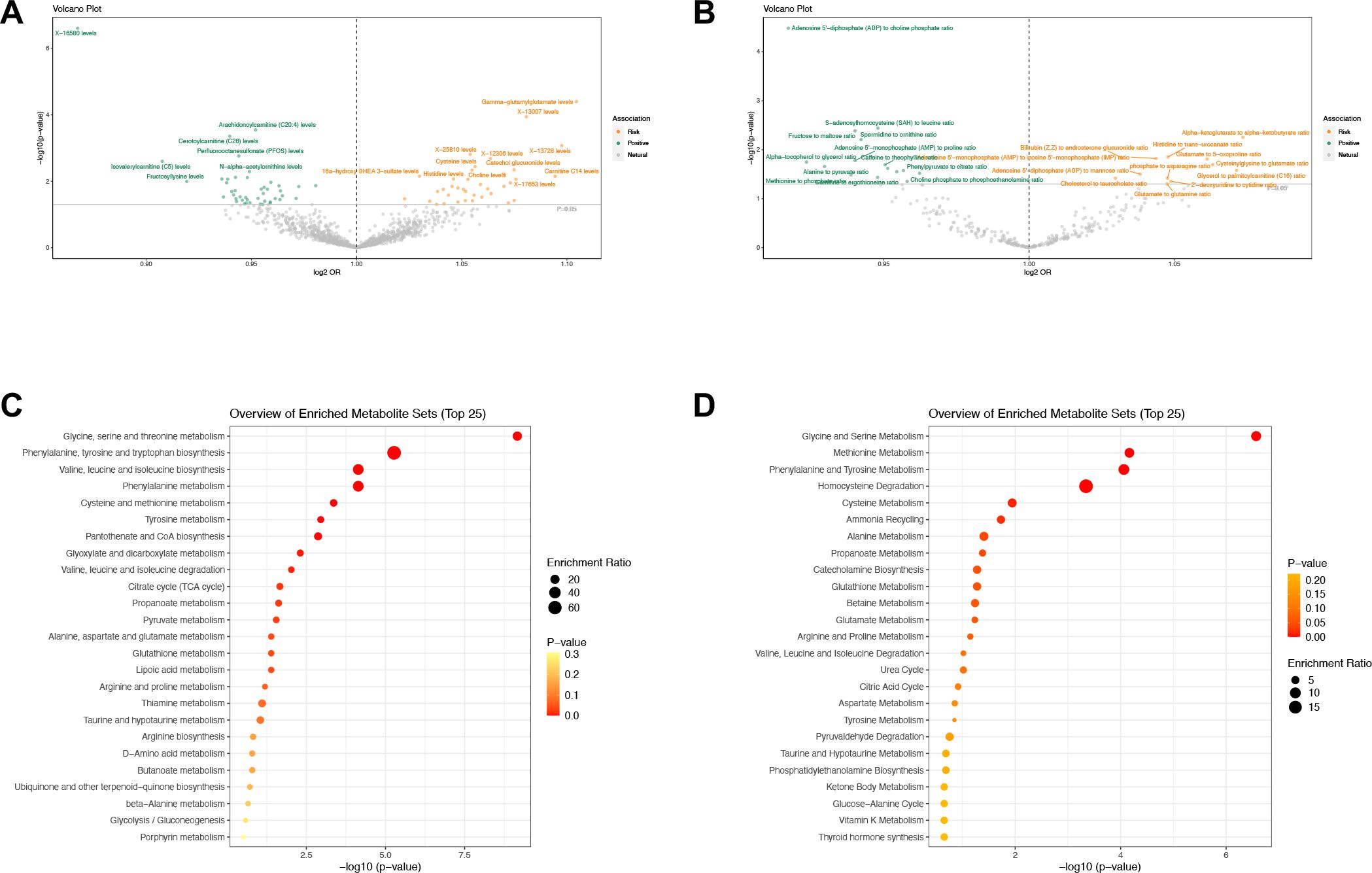
Figure 4. Causal estimates of MR between plasma metabolites and asthma. (A) Volcano plots of the IVW MR for the associations between plasma metabolites levels and asthma. (B) Volcano plots of the IVW MR for the associations between metabolic ratios and asthma. (C) The enrichment pathways of metabolites in KEGG. (D) The enrichment pathways of metabolites in SMPDE database.
However, following FDR correction, only four results maintained significant negative or positive causal relationships with asthma. Adenosine-5′-diphosphate-to-choline phosphate ratio maintained a significant negative causal relationship with asthma (OR = 0.9171, 95% CI [0.8804–0.9554], P = 0.00003307, FDR = 0.0186). X-16580 levels maintained a significant negative causal relationship with asthma (OR = 0.8674, 95% CI [0.8217–0.9155], P = 0.00000025, FDR = 0.0003). X-13007 levels maintained a significant positive causal relationship with asthma (OR = 1.0808, 95% CI [1.0389–1.1242], P = 0.00011440, FDR = 0.0400). Gamma-glutamyl glutamate levels maintained a significant positive causal relationship with asthma (OR = 1.1045, 95% CI [1.0534–1.1581], P = 0.00003978, FDR = 0.0186).
Sensitivity analysis further verified the robustness of the MR results. The Q statistics showed that nine plasma metabolites had heterogeneity, whereas the rest did not show evidence of heterogeneity. Furthermore, the results of MR–Egger regression and MR–PRESSO did not reveal horizontal pleiotropy (dual validation using 72 plasma metabolite levels and 23 metabolic ratios). We did not find any reverse causality on the basis of the MR–Steiger test (Supplementary Table S19).
We queried the HMDB IDs for the 81 known metabolites associated with asthma and conducted metabolic pathway enrichment analysis on 51 identifiable compounds. The enrichment results highlighted the metabolic pathways of glycine, serine and threonine metabolism; phenylalanine, tyrosine, and tryptophan biosynthesis; and methionine metabolism (Figures 4C, D; Supplementary Table S20).
3.4 Causal effects of WBC subtype counts and immune cell traits on asthma
The IVW method revealed 35 associations between 81 unique immune cell traits and asthma (PIVW < 0.05) (Figure 5; Supplementary Tables S21–24). Immune cell traits included B cells (10), cDC (16), maturation stages of T cells (11), monocytes (5), myeloid cells (16), TBNKs (13), and Tregs (10) (Supplementary Table S25). In the results of a further step following FDR correction, those marked with stars indicated the maintenance of significant negative or positive causal relationships with asthma. The MR results remained stable in sensitivity analyses, suggesting the absence of significant heterogeneity and horizontal pleiotropy (Supplementary Table S26).
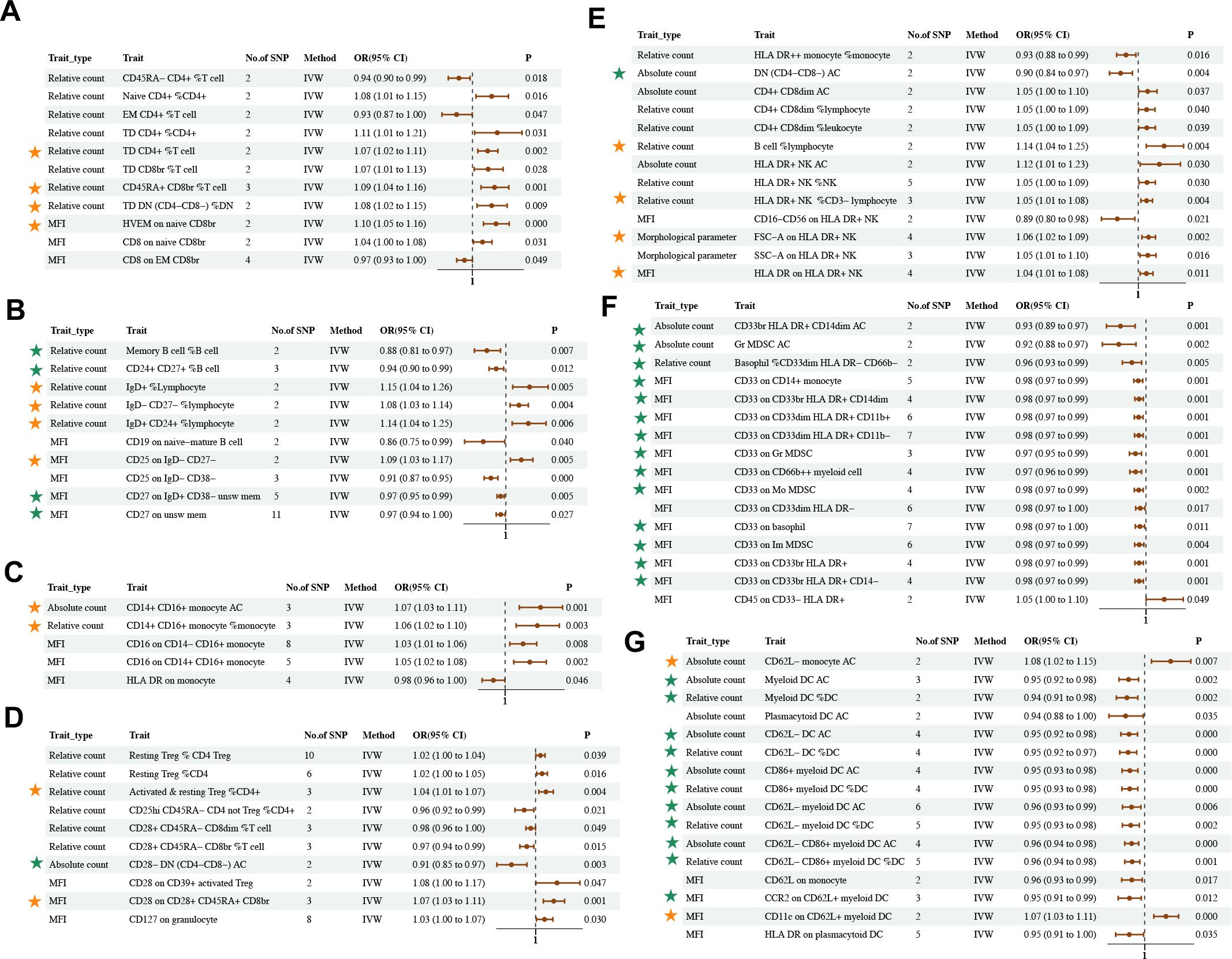
Figure 5. Causal estimates of MR between immune cell traits and asthma. Estimates from the IVW analysis of immune cell traits on asthma. Immune cell traits included (A) Maturation stages of T cell (B) B cell (C) Monocyte (D) Treg (E) TBNK (F) Myeloid cell (G) cDC. The results marked with green stars maintained significant negative causal relationship with asthma. The results marked with orange stars maintained significant positive causal relationship with asthma.
Although bivariate LDSC analysis identified a strong negative genetic association between eosinophil cell count and asthma, the MR analysis of eosinophil cell count in asthma identified heterogeneity and pleiotropy, which affected the results of causality (Supplementary Table S11).
3.5 Causal effects of inflammatory proteins and cytokines on asthma
The IVW method revealed five associations between inflammatory proteins and asthma and three associations between inflammatory cytokines and asthma (PIVW < 0.05) (Figures 6, 7; Supplementary Tables S27–30). Following FDR correction, four results maintained a significant positive causal relationship with asthma. CD40L receptor levels maintained a significant positive causal relationship with asthma (OR = 1.05, 95% CI [1.02–1.09], P = 0.003, FDR = 0.0588). IL-17C levels maintained a significant positive causal relationship with asthma (OR = 1.23, 95% CI [1.08–1.40], P = 0.002, FDR = 0.0554). Leukemia inhibitory factor receptor levels maintained a significant positive causal relationship with asthma (OR = 1.11, 95% CI [1.05–1.18], P = 0.000, FDR = 0.0057). IL-18 levels maintained a significant positive causal relationship with asthma (OR = 1.07, 95% CI [1.03–1.11], P = 0.001, FDR = 0.0518). The MR results remained stable in the sensitivity analyses, suggesting the absence of significant heterogeneity and horizontal pleiotropy (Supplementary Table S31).
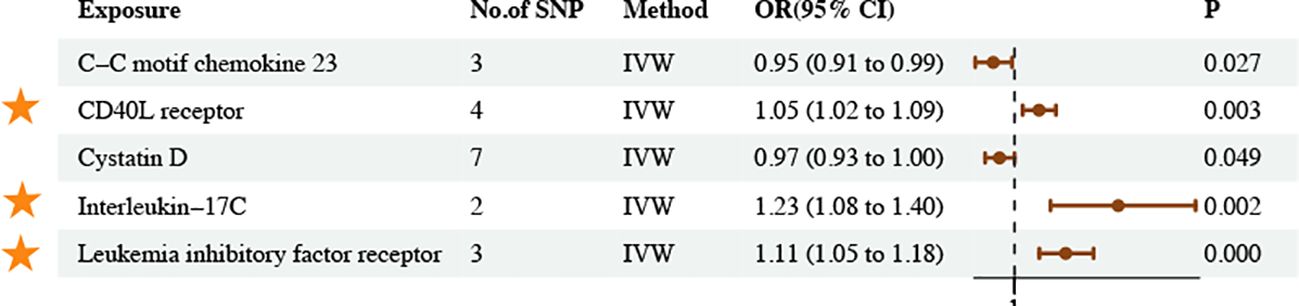
Figure 6. Causal estimates of MR between inflammatory proteins and asthma. Estimates from the IVW analysis of inflammatory proteins on asthma. The results marked with orange stars maintained significant positive causal relationship with asthma.

Figure 7. Causal estimates of MR between inflammatory cytokines and asthma. Estimates from the IVW analysis of inflammatory cytokines on asthma. The results marked with orange stars maintained significant positive causal relationship with asthma.
3.6 Mediation analysis results
We based our findings on asthma-associated gut microbiota, skin microbiota, metabolites, cells, proteins, and cytokines in two previously identified samples of MR to explore the potential mechanisms for the occurrence and development of asthma. We performed two-step MR analysis with plasma metabolites, immune cell traits, and inflammatory proteins and cytokines as mediator variables.
We found seven mediators that were significant intermediate variables linking gut microbiota or skin microbiota with asthma. The results showed that three gut bacterial pathways play a protective role in asthma through the mediation of IgD–CD27–B cell %lymphocyte, CD62L-DC %DC, HLA DR++ monocyte %monocyte, and perfluoro octane sulfonate levels. On the other hand, three bacteria played a predisposing role in asthma through the mediating effects of HLA DR+ natural killer %natural killer, and CD16 on CD14+ CD16+ monocyte and CD40L receptor levels (Figure 8; Supplementary Table S32).
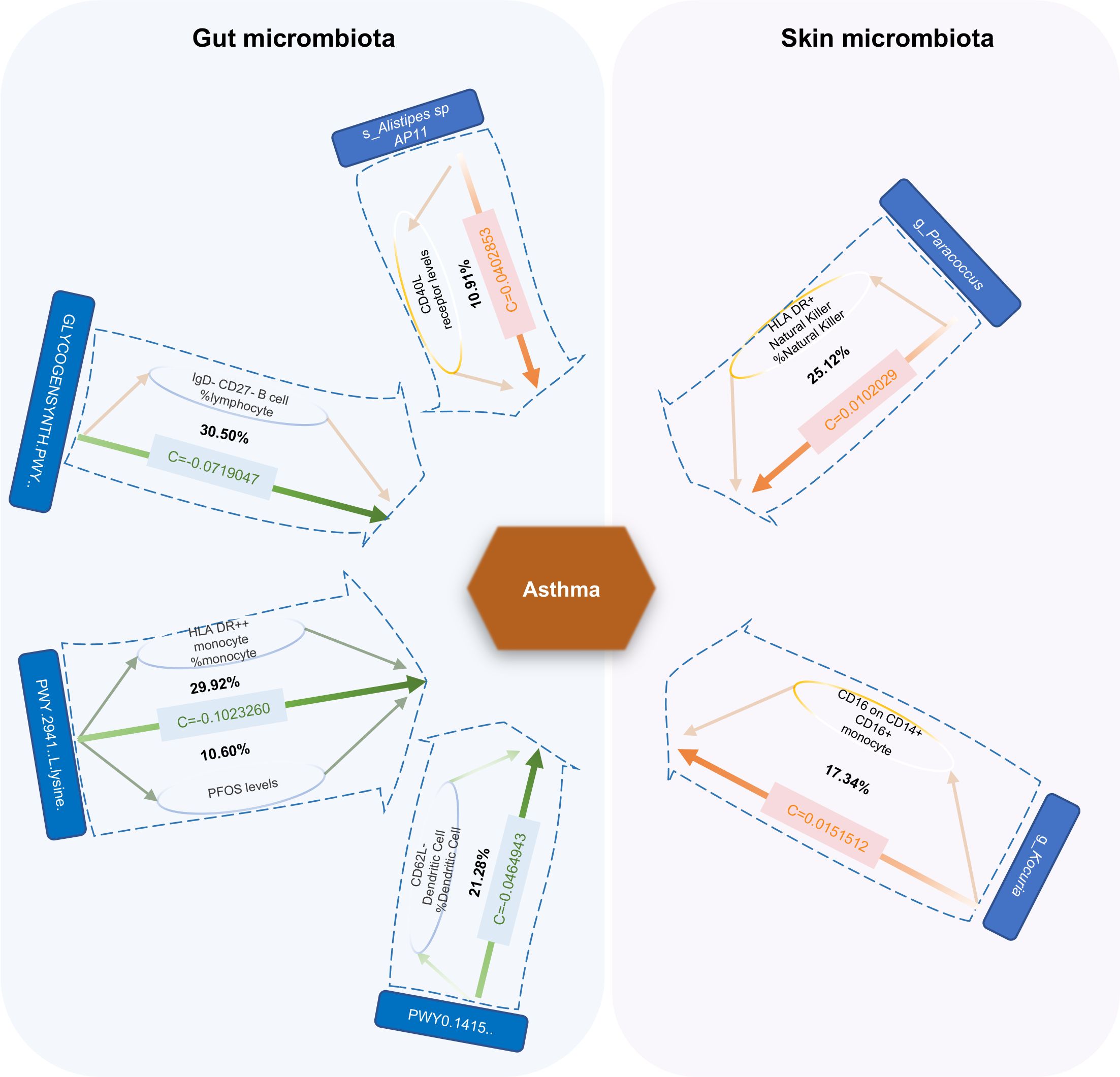
Figure 8. Mediation effect of gut microbiota or skin microbiota on asthma via plasma metabolites, immune cell traits, and inflammatory proteins. The green lines represent protective effects, and the orange lines represent risk effects. The mediation proportion is marked in the middle of the box. PFOS Perfluoro octane sulfonate; c Total effect; GLYCOGENSYNTH.PWY.glycogen.biosynthesis.I.from.ADP.D.Glucose; PWY.2941.L.lysine.biosynthesis.II; PWY0.1415.superpathway.of.heme.biosynthesis.from.uroporphyrinogen.III.
4 Discussion
In this study, we first assessed the causal relationship among gut microbiota, skin microbiota, plasma metabolome, WBC subtype counts, immune cell traits, circulating inflammatory proteins, inflammatory cytokines, and asthma by using MR analysis. We found potential causal associations among 31 gut bacterial features (abundances of 19 bacterial pathways and 12 microbiota), 10 skin bacterial features, 108 plasma metabolites (81 metabolites and 27 ratios), 81 immune cell traits, five circulating inflammatory proteins, three inflammatory cytokines, and asthma. Furthermore, the results of our mediation analysis supported the mediating effects of one plasma metabolite, five immunophenotypes, and one inflammatory protein on gut or skin microbiota in asthma pathogenesis.
A previous study found that the phylum Firmicutes showed significantly low abundance in children with asthma, and its dysbiosis may be associated with an increased risk of asthma (10). The negative association between the species Eubacterium eligens and Lachnospiraceae bacterium 7 1 58FAA in the phylum Firmicutes and asthma in our study was consistent with this previously reported finding. Another metabolomics-based study performed a comparative analysis on stool samples from children with asthma and healthy children aged 4–7 years. It found that children with allergic airway illnesses tended to have a considerably lower abundance of Firmicutes than those without. These results suggested that childhood rhinitis and asthma may be caused by a reduction in certain gut microbes in the phylum Firmicutes that are involved in the up-regulation of fecal amino acids (47). Therefore, through our study, we can infer whether the specific gut microbes in the phylum Firmicutes showing reductions may be the species Eubacterium eligens and Lachnospiraceae bacterium 7 1 58FAA. Data from the Canadian Healthy Infant Longitudinal Development Study demonstrated that the relative abundance of Rothia (phylum Actinobacteria) was considerably reduced in the gut microbiome of infants at risk for asthma in the first 100 days of life (48). We found that phylum Actinobacteria and class Actinobacteria in phylum Actinobacteria had a positive causal relationship with asthma likely due to the role of other bacteria in Actinobacteria. A positive association was found between Bacteroides fragilis colonization and asthma predictive index (49), and we obtained findings consistent with this result. The relative abundances of Bacteroidetes increased in the symptomatic eosinophilic asthma group (49). In our study, the effects of bacteria in Bacteroidetes on asthma are complicated, and the overall effects on asthma are unknown.
In healthy, but not in atopic, subjects, the relative abundance of Acinetobacter species was associated with the expression of anti-inflammatory molecules by PBMCs. Moreover, healthy subjects exhibited a robust balance between anti-inflammatory and Th1/Th2 gene expression, which was related to the composition of the skin microbiota. In cell assays and a mouse model, Acinetobacter species induced strong Th1 and anti-inflammatory responses by immune and skin cells and protected against allergic sensitization and lung inflammation through the skin. Acinetobacter species in the skin microbiota were found to protect against allergic sensitization and inflammation (19). These findings are similar to ours.
Patients with asthma were characterized by high levels of methionine, glutamine, and histidine and low levels of formate, methanol, acetate, choline, O-phosphocholine, arginine, and glucose (50). Similarly, we found that histidine (OR = 1.046, 95% CI [1.012–1.082], P = 0.009) and glutamine (OR = 1.053, 95% CI [1.013–1.094], P = 0.009) levels had a positive causal relationship with asthma. Metabolic pathway enrichment analysis on 51 identified compounds included methionine (Raw Pval = 0.0000682, FDR = 0.00284) and histidine (Raw Pval = 0.56, FDR = 1) metabolism.
Asthma is a highly heterogeneous disease with numerous endotypes based on discrete pathophysiological mechanisms. The complexity of asthma is due to the involvement of multiple cell types, including tissue-resident ILCs and other innate immune cells, such as bronchial epithelial cells, DCs, macrophages, and eosinophils (51). Numerous myeloid cells play a crucial role in asthma pathogenesis (52). Meanwhile, allergic asthma is the most common asthma phenotype (53). A considerable proportion of myeloid DCs rapidly disappear from circulation following allergen inhalation, suggesting that the margination of circulating myeloid DCs, as well as their recruitment into the airway mucosa, is an important feature of the immune response to inhaled allergens (54). Our findings are consistent with the above results. Eosinophils, a type of immune cell, play a critical role in the development and progression of asthma (55). Eosinophils are more than a marker of type 2 high asthma (14). Specifically, our bivariate LDSC analysis identified a strong negative genetic association between eosinophil cell counts and asthma.
The CD40 receptor and its ligand CD40L is one of the most critical molecular pairs of stimulatory immune checkpoints. Nonhematopoietic cells expressing CD40 can also engage CD40L and trigger a proinflammatory response (56). IL-17C has been known to participate in allergic inflammation. It is produced by distinct cellular sources and an essential autocrine cytokine that regulates innate epithelial immune responses (57). IL-17C is a member of the IL-17 family that is selectively induced in epithelia by bacterial challenge and inflammatory stimuli. IL-17C stimulates epithelial inflammatory responses, including the expression of proinflammatory cytokines, chemokines, and antimicrobial peptides, which are similar to those induced by IL-17A and IL-17F (58, 59). In addition, IL-18 may act as a potential mediator in the causal relationship between adult-onset asthma and ulcerative colitis (60). IL-18 expression in the lamina propria in biopsies from subjects with asthma did not differ from that in biopsies from controls but decreased in the epithelium (61). Recent work identified the association of IL-18 with the pathogenesis of asthma, wherein increased IL-18 expression was found in the serum of patients. Furthermore, IL-18 polymorphisms have been reported to be associated with susceptibility to asthma, suggesting that IL-18 may be therapeutically relevant to asthma (62). The above findings are similar to the results of our study.
Finally, we identified a few gut or skin bacteria on the basis of plasma metabolites, immune phenotype, and inflammatory proteins involved in the pathogenesis of asthma. Related research remains scarce, and additional studies are needed on the role of the above factors in asthma in the future. This study has certain limitations. First, under the currently set screening conditions, we obtained few SNPs for some immune cells. This situation may have led to a bias in the results. Second, given that our population data originated from individuals of European ancestry, the generalizability of our research results to other populations is limited. Third, asthma type analysis will provide a clear information basis for subsequent clinical and experimental studies. The validity of our study’s results should be further confirmed through additional experimental and clinical studies.
5 Conclusion
Our MR study identified 31 gut bacterial features (abundances of 19 bacterial pathways and 12 microbiota), 10 skin bacterial features, 108 plasma metabolites (81 metabolites and 27 ratios), 81 immune cells, five circulating inflammatory proteins, and three inflammatory cytokines involved in asthma. Moreover, the results of our mediation analysis supported the mediating effects of perfluoro octane sulfonate (PFOS, a plasma metabolite), IgD–CD27–B cells, CD62L-DCs, HLA DR++ monocytes, HLA DR+ natural killer cells, and CD16 on CD14+ CD16+ monocytes (five immunophenotypes), and CD40L receptor (an inflammatory protein) on gut or skin microbiota in asthma pathogenesis. Our findings contribute to the study of asthma mechanisms.
Data availability statement
The datasets presented in this study can be found in online repositories. The names of the repository/repositories and accession number(s) can be found in the article/Supplementary Material.
Author contributions
WG: Data curation, Methodology, Software, Writing – original draft, Conceptualization, Investigation, Project administration, Validation. EH: Writing – review & editing. HM: Writing – review & editing. JW: Writing – review & editing, Funding acquisition, Resources. QW: Writing – review & editing, Funding acquisition, Resources.
Funding
The author(s) declare that financial support was received for the research and/or publication of this article. General program of National Natural Science Foundation of China (No. 82174243), High level Key Discipline of National Administration of Traditional Chinese Medicine-Traditional Chinese constitutional medicine (No. zyyzdxk-2023251), the Fundamental Research Funds for the Central Universities (No.2023-JYB-JBZD-009).
Acknowledgments
We are grateful to all participants and study staff from the Biobank PopGen (Dr. Gunar Jacobs and team) and the KORA Studienzentrum (Dr. Margit Heier and team). We are grateful to Dutch Microbiome Project, UK Biobank, FinnGen, GWAS Catalog, and IEU OpenGWAS project for their selfless public sharing of GWAS summary data, which provides us with great convenience to carry out this research.
Conflict of interest
The authors declare that the research was conducted in the absence of any commercial or financial relationships that could be construed as a potential conflict of interest.
Publisher’s note
All claims expressed in this article are solely those of the authors and do not necessarily represent those of their affiliated organizations, or those of the publisher, the editors and the reviewers. Any product that may be evaluated in this article, or claim that may be made by its manufacturer, is not guaranteed or endorsed by the publisher.
Supplementary material
The Supplementary Material for this article can be found online at: https://www.frontiersin.org/articles/10.3389/fimmu.2025.1436888/full#supplementary-material
Abbreviations
MR, Mendelian randomization; WBC, white blood cells; DCs, dendritic cells; ILCs, innate lymphoid cells; AHR, airway hyperreactivity; Th, T helper; IL, interleukin; GWASs, genome-wide association studies; LDSC, linkage disequilibrium score regression; ASV, amplicon sequence variant; SNP, Single nucleotide polymorphism; AC, absolute cell; MFI, median fluorescence intensities; MPs, morphological parameters; RC, relative cell; TBNK - T, B, and natural killer cells; IV, Instrumental variable; MR–PRESSO, MR pleiotropy residual sum and outlier; IVW, Inverse–variance weighted; FDR, false discovery rate; CI, Confidence interval; OR, Odds ratio.
References
1. GBD 2015 Chronic Respiratory Disease Collaborators. Global, regional, and national deaths, prevalence, disability-adjusted life years, and years lived with disability for chronic obstructive pulmonary disease and asthma, 1990-2015: A systematic analysis for the Global Burden of Disease Study 2015. Lancet Respir Med. (2017) 5:691–706. doi: 10.1016/s2213-2600(17)30293-x
2. Reddel HK, Bacharier LB, Bateman ED, Brightling CE, Brusselle GG, Buhl R, et al. Global initiative for asthma strategy 2021: executive summary and rationale for key changes. Am J Respir Crit Care Med. (2022) 205:17–35. doi: 10.1164/rccm.202109-2205PP
3. Reddel HK, Bacharier LB, Bateman ED, Brightling CE, Brusselle GG, Buhl R, et al. Global initiative for asthma strategy 2021: executive summary and rationale for key changes. Eur Respir J. (2022) 59:2102730. doi: 10.1183/13993003.02730-2021
4. Li R, Guo Q, Zhao J, Kang W, Lu R, Long Z, et al. Assessing causal relationships between gut microbiota and asthma: evidence from two sample Mendelian randomization analysis. Front Immunol. (2023) 14:1148684. doi: 10.3389/fimmu.2023.1148684
5. Barcik W, Boutin RCT, Sokolowska M, Finlay BB. The role of lung and gut microbiota in the pathology of asthma. Immunity. (2020) 52:241–55. doi: 10.1016/j.immuni.2020.01.007
6. Ver Heul A, Planer J, Kau AL. The human microbiota and asthma. Clin Rev Allergy Immunol. (2019) 57:350–63. doi: 10.1007/s12016-018-8719-7
7. Barcik W, Pugin B, Westermann P, Perez NR, Ferstl R, Wawrzyniak M, et al. Histamine-secreting microbes are increased in the gut of adult asthma patients. J Allergy Clin Immunol. (2016) 138:1491–4.e7. doi: 10.1016/j.jaci.2016.05.049
8. Chunxi L, Haiyue L, Yanxia L, Jianbing P, Jin S. The gut microbiota and respiratory diseases: new evidence. J Immunol Res. (2020) 2020:2340670. doi: 10.1155/2020/2340670
9. Russell SL, Gold MJ, Reynolds LA, Willing BP, Dimitriu P, Thorson L, et al. Perinatal antibiotic-induced shifts in gut microbiota have differential effects on inflammatory lung diseases. J Allergy Clin Immunol. (2015) 135:100–9. doi: 10.1016/j.jaci.2014.06.027
10. Hufnagl K, Pali-Schöll I, Roth-Walter F, Jensen-Jarolim E. Dysbiosis of the gut and lung microbiome has a role in asthma. Semin Immunopathol. (2020) 42:75–93. doi: 10.1007/s00281-019-00775-y
11. Kelly RS, Dahlin A, McGeachie MJ, Qiu W, Sordillo J, Wan ES, et al. Asthma metabolomics and the potential for integrative omics in research and the clinic. Chest. (2017) 151:262–77. doi: 10.1016/j.chest.2016.10.008
12. Qu HQ, Glessner J, Qu J, Gilhool S, Mentch F, Campbell I, et al. Metabolomic profiling of samples from pediatric patients with asthma unveils deficient nutrients in African Americans. iScience. (2022) 25:104650. doi: 10.1016/j.isci.2022.104650
13. Yu M, Cui FX, Jia HM, Zhou C, Yang Y, Zhang HW, et al. Aberrant purine metabolism in allergic asthma revealed by plasma metabolomics. J Pharm BioMed Anal. (2016) 120:181–9. doi: 10.1016/j.jpba.2015.12.018
14. Hammad H, Lambrecht BN. The basic immunology of asthma. Cell. (2021) 184:1469–85. doi: 10.1016/j.cell.2021.02.016
15. Trujillo J, Lunjani N, Ryan D, O’Mahony L. Microbiome-immune interactions and relationship to asthma severity. J Allergy Clin Immunol. (2022) 149:533–4. doi: 10.1016/j.jaci.2021.12.774
16. Busse WW. Inflammation in asthma: the cornerstone of the disease and target of therapy. J Allergy Clin Immunol. (1998) 102:S17–22. doi: 10.1016/s0091-6749(98)70002-8
17. Lambrecht BN, Hammad H, Fahy JV. The cytokines of asthma. Immunity. (2019) 50:975–91. doi: 10.1016/j.immuni.2019.03.018
18. Fahy JV. Type 2 inflammation in asthma–present in most, absent in many. Nat Rev Immunol. (2015) 15:57–65. doi: 10.1038/nri3786
19. Fyhrquist N, Ruokolainen L, Suomalainen A, Lehtimäki S, Veckman V, Vendelin J, et al. Acinetobacter species in the skin microbiota protect against allergic sensitization and inflammation. J Allergy Clin Immunol. (2014) 134:1301–9.e11. doi: 10.1016/j.jaci.2014.07.059
20. Ruokolainen L, Paalanen L, Karkman A, Laatikainen T, von Hertzen L, Vlasoff T, et al. Significant disparities in allergy prevalence and microbiota between the young people in Finnish and Russian Karelia. Clin Exp Allergy. (2017) 47:665–74. doi: 10.1111/cea.12895
21. Hanski I, von Hertzen L, Fyhrquist N, Koskinen K, Torppa K, Laatikainen T, et al. Environmental biodiversity, human microbiota, and allergy are interrelated. Proc Natl Acad Sci U S A. (2012) 109:8334–9. doi: 10.1073/pnas.1205624109
22. Losol P, Sokolowska M, Chang YS. Interactions between microbiome and underlying mechanisms in asthma. Respir Med. (2023) 208:107118. doi: 10.1016/j.rmed.2023.107118
23. Emdin CA, Khera AV, Kathiresan S. Mendelian randomization. JAMA. (2017) 318:1925–6. doi: 10.1001/jama.2017.17219
24. Carter AR, Sanderson E, Hammerton G, Richmond RC, Davey Smith G, Heron J, et al. Mendelian randomisation for mediation analysis: current methods and challenges for implementation. Eur J Epidemiol. (2021) 36:465–78. doi: 10.1007/s10654-021-00757-1
25. Skrivankova VW, Richmond RC, Woolf BAR, Yarmolinsky J, Davies NM, Swanson SA, et al. Strengthening the reporting of observational studies in epidemiology using Mendelian randomization: the strobe-Mr statement. JAMA. (2021) 326:1614–21. doi: 10.1001/jama.2021.18236
26. Lopera-Maya EA, Kurilshikov A, van der Graaf A, Hu S, Andreu-Sánchez S, Chen L, et al. Effect of host genetics on the gut microbiome in 7,738 participants of the dutch microbiome project. Nat Genet. (2022) 54:143–51. doi: 10.1038/s41588-021-00992-y
27. Moitinho-Silva L, Degenhardt F, Rodriguez E, Emmert H, Juzenas S, Möbus L, et al. Host genetic factors related to innate immunity, environmental sensing and cellular functions are associated with human skin microbiota. Nat Commun. (2022) 13:6204. doi: 10.1038/s41467-022-33906-5
28. Chen Y, Lu T, Pettersson-Kymmer U, Stewart ID, Butler-Laporte G, Nakanishi T, et al. Genomic atlas of the plasma metabolome prioritizes metabolites implicated in human diseases. Nat Genet. (2023) 55:44–53. doi: 10.1038/s41588-022-01270-1
29. Chen MH, Raffield LM, Mousas A, Sakaue S, Huffman JE, Moscati A, et al. Trans-ethnic and ancestry-specific blood-cell genetics in 746,667 individuals from 5 global populations. Cell. (2020) 182:1198–213.e14. doi: 10.1016/j.cell.2020.06.045
30. Orrù V, Steri M, Sidore C, Marongiu M, Serra V, Olla S, et al. Complex genetic signatures in immune cells underlie autoimmunity and inform therapy. Nat Genet. (2020) 52:1036–45. doi: 10.1038/s41588-020-0684-4
31. Sidore C, Busonero F, Maschio A, Porcu E, Naitza S, Zoledziewska M, et al. Genome sequencing elucidates Sardinian genetic architecture and augments association analyses for lipid and blood inflammatory markers. Nat Genet. (2015) 47:1272–81. doi: 10.1038/ng.3368
32. Zhao JH, Stacey D, Eriksson N, Macdonald-Dunlop E, Hedman ÅK, Kalnapenkis A, et al. Genetics of circulating inflammatory proteins identifies drivers of immune-mediated disease risk and therapeutic targets. Nat Immunol. (2023) 24:1540–51. doi: 10.1038/s41590-023-01588-w
33. Ahola-Olli AV, Würtz P, Havulinna AS, Aalto K, Pitkänen N, Lehtimäki T, et al. Genome-wide association study identifies 27 loci influencing concentrations of circulating cytokines and growth factors. Am J Hum Genet. (2017) 100:40–50. doi: 10.1016/j.ajhg.2016.11.007
34. Kalaoja M, Corbin LJ, Tan VY, Ahola-Olli AV, Havulinna AS, Santalahti K, et al. The role of inflammatory cytokines as intermediates in the pathway from increased adiposity to disease. Obes (Silver Spring). (2021) 29:428–37. doi: 10.1002/oby.23060
35. Sanna S, van Zuydam NR, Mahajan A, Kurilshikov A, Vich Vila A, Võsa U, et al. Causal relationships among the gut microbiome, short-chain fatty acids and metabolic diseases. Nat Genet. (2019) 51:600–5. doi: 10.1038/s41588-019-0350-x
36. Yun Z, Guo Z, Li X, Shen Y, Nan M, Dong Q, et al. Genetically predicted 486 blood metabolites in relation to risk of colorectal cancer: A Mendelian randomization study. Cancer Med. (2023) 12:13784–99. doi: 10.1002/cam4.6022
37. Verbanck M, Chen CY, Neale B, Do R. Detection of widespread horizontal pleiotropy in causal relationships inferred from Mendelian randomization between complex traits and diseases. Nat Genet. (2018) 50:693–8. doi: 10.1038/s41588-018-0099-7
38. Burgess S, Thompson SG. Avoiding bias from weak instruments in Mendelian randomization studies. Int J Epidemiol. (2011) 40:755–64. doi: 10.1093/ije/dyr036
39. Burgess S, Scott RA, Timpson NJ, Davey Smith G, Thompson SG. Using published data in Mendelian randomization: A blueprint for efficient identification of causal risk factors. Eur J Epidemiol. (2015) 30:543–52. doi: 10.1007/s10654-015-0011-z
40. Bowden J, Davey Smith G, Burgess S. Mendelian randomization with invalid instruments: effect estimation and bias detection through egger regression. Int J Epidemiol. (2015) 44:512–25. doi: 10.1093/ije/dyv080
41. Hartwig FP, Davey Smith G, Bowden J. Robust inference in summary data Mendelian randomization via the zero modal pleiotropy assumption. Int J Epidemiol. (2017) 46:1985–98. doi: 10.1093/ije/dyx102
42. Bowden J, Davey Smith G, Haycock PC, Burgess S. Consistent estimation in Mendelian randomization with some invalid instruments using a weighted median estimator. Genet Epidemiol. (2016) 40:304–14. doi: 10.1002/gepi.21965
43. Burgess S, Thompson SG. Interpreting findings from Mendelian randomization using the Mr-Egger method. Eur J Epidemiol. (2017) 32:377–89. doi: 10.1007/s10654-017-0255-x
44. Holmes MV, Ala-Korpela M, Smith GD. Mendelian randomization in cardiometabolic disease: challenges in evaluating causality. Nat Rev Cardiol. (2017) 14:577–90. doi: 10.1038/nrcardio.2017.78
45. Benjamini Y, Hochberg Y. Controlling the false discovery rate: A practical and powerful approach to multiple testing. J R Stat society: Ser B (Methodological). (1995) 57:289–300. doi: 10.1111/j.2517-6161.1995.tb02031.x
46. Bulik-Sullivan BK, Loh PR, Finucane HK, Ripke S, Yang J, Patterson N, et al. Ld score regression distinguishes confounding from polygenicity in genome-wide association studies. Nat Genet. (2015) 47:291–5. doi: 10.1038/ng.3211
47. Chiu CY, Cheng ML, Chiang MH, Kuo YL, Tsai MH, Chiu CC, et al. Gut microbial-derived butyrate is inversely associated with Ige responses to allergens in childhood asthma. Pediatr Allergy Immunol. (2019) 30:689–97. doi: 10.1111/pai.13096
48. Arrieta MC, Stiemsma LT, Dimitriu PA, Thorson L, Russell S, Yurist-Doutsch S, et al. Early infancy microbial and metabolic alterations affect risk of childhood asthma. Sci Transl Med. (2015) 7:307ra152. doi: 10.1126/scitranslmed.aab2271
49. Vael C, Nelen V, Verhulst SL, Goossens H, Desager KN. Early intestinal bacteroides fragilis colonisation and development of asthma. BMC Pulm Med. (2008) 8:19. doi: 10.1186/1471-2466-8-19
50. Jung J, Kim SH, Lee HS, Choi GS, Jung YS, Ryu DH, et al. Serum metabolomics reveals pathways and biomarkers associated with asthma pathogenesis. Clin Exp Allergy. (2013) 43:425–33. doi: 10.1111/cea.12089
51. Wang X, Kong Y, Zheng B, Zhao X, Zhao M, Wang B, et al. Tissue-resident innate lymphoid cells in asthma. J Physiol. (2023) 601:3995–4012. doi: 10.1113/jp284686
52. Lambrecht BN, Persson EK, Hammad H. Myeloid cells in asthma. Microbiol Spectr. (2017) 5. doi: 10.1128/microbiolspec.MCHD-0053-2016
53. Akar-Ghibril N, Casale T, Custovic A, Phipatanakul W. Allergic endotypes and phenotypes of asthma. J Allergy Clin Immunol Pract. (2020) 8:429–40. doi: 10.1016/j.jaip.2019.11.008
54. Upham JW, Denburg JA, O’Byrne PM. Rapid response of circulating myeloid dendritic cells to inhaled allergen in asthmatic subjects. Clin Exp Allergy. (2002) 32:818–23. doi: 10.1046/j.1365-2222.2002.01375.x
55. Shen K, Zhang M, Zhao R, Li Y, Li C, Hou X, et al. Eosinophil extracellular traps in asthma: implications for pathogenesis and therapy. Respir Res. (2023) 24:231. doi: 10.1186/s12931-023-02504-4
56. Karnell JL, Rieder SA, Ettinger R, Kolbeck R. Targeting the Cd40-Cd40l pathway in autoimmune diseases: humoral immunity and beyond. Adv Drug Delivery Rev. (2019) 141:92–103. doi: 10.1016/j.addr.2018.12.005
57. Ramirez-Carrozzi V, Sambandam A, Luis E, Lin Z, Jeet S, Lesch J, et al. Il-17c regulates the innate immune function of epithelial cells in an autocrine manner. Nat Immunol. (2011) 12:1159–66. doi: 10.1038/ni.2156
58. McGeachy MJ, Cua DJ, Gaffen SL. The Il-17 family of cytokines in health and disease. Immunity. (2019) 50:892–906. doi: 10.1016/j.immuni.2019.03.021
59. Olcott CM, Han JK, Cunningham TD, Franzese CB. Interleukin-9 and interleukin-17c in chronic rhinosinusitis. Int Forum Allergy Rhinol. (2016) 6:841–7. doi: 10.1002/alr.21745
60. Zou X, Lu RL, Liao B, Liu SJ, Dai SX. Causal relationship between asthma and ulcerative colitis and the mediating role of interleukin-18: A bidirectional Mendelian study and mediation analysis. Front Immunol. (2023) 14:1293511. doi: 10.3389/fimmu.2023.1293511
61. Kaur D, Chachi L, Gomez E, Sylvius N, Brightling CE. Interleukin-18, Il-18 binding protein and Il-18 receptor expression in asthma: A hypothesis showing Il-18 promotes epithelial cell differentiation. Clin Transl Immunol. (2021) 10:e1301. doi: 10.1002/cti2.1301
Keywords: asthma, gut microbiota, skin microbiota, plasma metabolites, immune cells, inflammatory proteins, inflammatory cytokines, Mendelian randomization
Citation: Guo W, Hong E, Ma H, Wang J and Wang Q (2025) Effect of the gut microbiome, skin microbiome, plasma metabolome, white blood cells subtype, immune cells, inflammatory proteins, and inflammatory cytokines on asthma: a two-sample Mendelian randomized study and mediation analysis. Front. Immunol. 16:1436888. doi: 10.3389/fimmu.2025.1436888
Received: 22 May 2024; Accepted: 03 March 2025;
Published: 21 March 2025.
Edited by:
Zhengrui Li, Shanghai Jiao Tong University, ChinaReviewed by:
Ancuta Lupu, Grigore T. Popa University of Medicine and Pharmacy, RomaniaYu Shuo Pan, Affiliated Hospital of Liaoning University of Traditional Chinese Medicine, China
Copyright © 2025 Guo, Hong, Ma, Wang and Wang. This is an open-access article distributed under the terms of the Creative Commons Attribution License (CC BY). The use, distribution or reproduction in other forums is permitted, provided the original author(s) and the copyright owner(s) are credited and that the original publication in this journal is cited, in accordance with accepted academic practice. No use, distribution or reproduction is permitted which does not comply with these terms.
*Correspondence: Ji Wang, ZG9jdG9yd2FuZzIwMDlAMTI2LmNvbQ==; Qi Wang, d2FuZ3FpNzEwQDEyNi5jb20=