- CNR Istituto Motori, Naples, Italy
The use of liquid or gaseous biofuels in reciprocating internal combustion engines (ICEs) is today a relevant issue as these systems are largely diffused for both steady power generation and transportation due to their flexibility and easiness of use. The improvement and perfect control of the combustion process under non-conventional fueling is mandatory to achieve high-energy efficiency without substantial changes to the architecture or the fuel supply system. In this perspective, the detailed characterization of multiphase reacting systems achievable though computational fluid dynamics (CFD) may give a decisive contribution. However, the assessed combustion models used for fossil fuels (diesel oil, gasoline, methane), tuned on the ground of a massive amount of experimental data, often results poor in predicting the actual behavior of renewable fuels whose composition and properties may change also according to technology for their production. Present work aims at filling some existing gaps in biofuel combustion modeling by performing investigations on two representative engine cases, for their characterization and performance enhancement. Two approaches are followed, namely through reduced chemical kinetics coupled with turbulence within a coherent flame schematization, and through a turbulent species transport approach with detailed kinetics. Simulations are first carried out on a compression ignition (CI) ICE. The formulation of a 3D CFD model is described to reproduce the performance of this engine in a dual-fuel mode with premixed syngas from biomass gasification and a biodiesel pilot injection leading to self-ignition. Pollutants formation and energy efficiency are calculated as syngas amount and the biodiesel start of injection (SOI) are varied. Attention is then focused on the implementation of renewable alcohol fuels (ethanol and butanol), as these lasts are receiving large interest due to low production costs. A validated reduced kinetic mechanism for PRF-ethanol-butanol combustion performs well in multi-component oxidation conditions, as well as in neat fuel oxidation conditions, in terms of ignition delay time, laminar flame speed and HCCI combustion conditions. The paper shows that CFD, even at different level of approximation, may describe into detail the combustion process and provide important guidelines for the design of new generation ICEs fuelled by biofuels.
Introduction
The reduction of greenhouse gas (GHG) emissions has become an important driver for the spread of the concept of bioenergy, in particular for Countries belonging to the Organization for Economic Cooperation and Development (OECD). Bioenergy derives from biological materials, and it is renewable as almost all can be traced back to energy from sunlight. Indeed, a substance used for bioenergy production is called a feedstock, as it can be converted into a biofuel in liquid or gaseous form. Examples comprise straw, sugarcane, wood waste, manure, and many other by-products form agricultural processes. Biomass feedstock can be used in different ways, e.g. by burning wood for heat, or as genetically modifying bacteria to create cellulosic ethanol. However, it is important that bioenergy is harnessed in a sustainable way.
Nowadays, bioenergy plays a prominent role only in the portfolio of a limited number of Countries. According to the International Energy Agency (IEA), in 2017, 90% of the whole capacity was located in 26 countries, where bioenergy supplied around 500 terawatt hours (TWh) of electricity, accounting for 2% of global electricity production (IEA Technology Roadmap, 2017). It is expected that by 2050, 3,100 TWh of electricity will be provided by bioenergy, i.e., 7.4% of world electricity generation, saving 1.3 Gt CO2 emissions per year, in addition to 0.7 Gt from biomass heat in industry and buildings, with very low life-cycle greenhouse gases (GHG) emissions (IEA Technology Roadmap, 2017).
A rapid growth of biofuel production has taken place in the last 10 years, supported by government policies and energy security concerns, and aimed at the sustainment and revitalization of the agricultural sector and the rural economy. In the transportation sector, a blending mandate, defining the proportion of biofuel to be used to power road vehicles, is a common support measure to enhance the bioenergy diffusion, often combined with other measures such as tax incentives. As a result, worldwide biofuel production rose from 16 billion liters (volumetric) in 2000 to 136.5 billion liters in 2017 (IEA Technology Roadmap, 2017). Current bioenergy consumption is largest in the heat sector, also for industrial applications, followed by bioenergy for electricity and transportation. While bioenergy for heat has kept a slowly growing trend, the bioenergy produced by biofuels in transportation is growing faster, as is the use of biomass for electricity generation purposes. A synoptic view of the current and recent worldwide situation of bioenergy consumption is given in Figure 1, as a function of the end use (left) and as regards the trend over the last years (right).
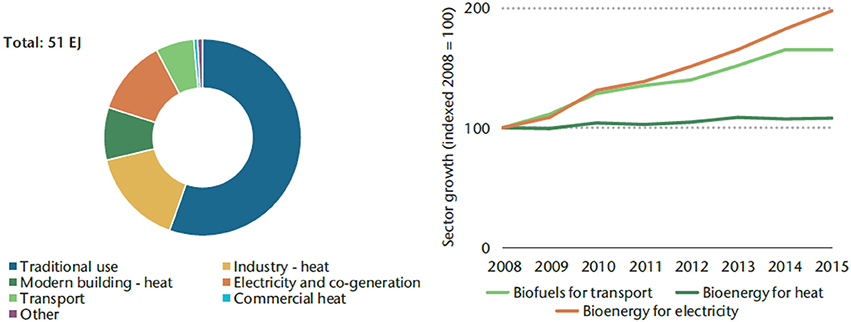
Figure 1. Consumption of biomass and waste resources by end use in 2015 (left) and modern bioenergy growth by sector, 2008–15 (right) (IEA Technology Road Map, 2017).
Based on the production technologies, biofuels are classified in:
• “First-generation” or conventional biofuels, derived from feedstock that can be consumed as human food, including biodiesel, from vegetable oils extraction (soybean, sunflower), with or without esterification, and alcohols (most commonly ethanol and butanol) from fermentation of simple sugars from starch crops (corn, wheat) or sugar crops (sugarcane);
• “Second-generation” or advanced biofuels, manufactured from different biomasses through different processing technologies as:
◦ Thermochemical conversion, as gasification, where carbon-based materials are converted into a synthesis fuel gas or syngas, this last being increasingly considered as a source for micro-cogeneration (combined heat and power, CHP) systems;
◦ Biochemical conversion through enzymatic-hydrolysis of lignocellulosic material. The sugars derived from this process are fermented into alcohol and then distilled into ethanol.
• Third-generation” biofuels derived from algae, as these produce an oil that can be easily refined into diesel or certain components of gasoline, or can be genetically manipulated to produce both alcohols or even gasoline and diesel fuel directly.
Worth mentioning are also processes aimed at producing fuels with very similar properties to diesel and kerosene. In particular, the Biomass-to-Liquids (BtL) diesel, also referred to as Fischer-Tropsch diesel (Kim et al., 2016), is produced by a two-step process: first, biomass is converted into syngas through gasification; after cleaning, the syngas catalytically undergoes a Fischer-Tropsch process, turning into a broad range of hydrocarbons in the liquid form, including synthetic diesel and bio-kerosene. The main technological challenges are related to the ones characterizing syngas production.
Westbrook (2013) provides a deep review on the effects of first-generation biofuels on the combustion processes and resulting emissions in ICEs. The most common petroleum fuels derive from different cuts during the distillation process of a petroleum refinery, as gasoline counts 4–10 carbon atoms per molecule, jet fuel counts 10–14 carbon atoms per molecule and diesel fuel counts around 15–22 carbon atoms per molecule. Therefore, as also pointed out by Bergthorson and Thomson (2015), a biofuel chosen for engine applications is largely inferred from this aspect, e.g., short-chain alcohols, as ethanol and butanol, are generally employed as gasoline replacements and knocking suppressors, while long-chain esters as biodiesel are used as diesel substitutes (Westbrook, 2013).
However, the intrinsic variability of biomass feedstock, due to disparate growth and harvesting conditions, presents challenges for the characterization of derived biofuels used in ICEs applications, which require materials that are physically and chemically consistent with petroleum hydrocarbon fuels (Williams et al., 2016). The biomass conversion technology employed, along with the chemical and physical characteristics of the original feedstock, determine the structural features of the resulting biofuel, thus affecting the combustion efficiency and resulting noxious emissions when used in ICEs. The high variability of these different biofuels has led to the need of massive researches to study and improve the operation of ICEs fuelled with these renewable sources. In this perspective, the investigation of biofuel applications to ICEs and of many processes such as scavenging, fuel injection, mixture preparation, combustion, noxious emissions formation, heat transfer etc. can be performed through CFD methods. These have the capacity of simulating flow conditions that are hardly reproducible in experimental tests or are coupled with huge investment costs in the engine test bench equipment, and provide more detailed and complete information and an increased level of processes understanding due to the achievable high temporal or spatial resolution.
A review focusing on biodiesel combustion modeling is in the paper by Cheng et al. (2013), dealing with advances achieved through three different approaches, namely use of surrogate chemical kinetic mechanisms, mechanism reduction methods, and thermo-physical properties models. In the first approach, given a set of target properties, a surrogate fuel can be used to emulate a real one. Different surrogate criteria can be followed to achieve the more realistic description of the oxidation of a fuel. Matching the real fuel carbon content is the most used approach in many studies (Brakora et al., 2008; Herbinet et al., 2010; Luo et al., 2012), but also other possibilities exist, as matching the heating value (Brakora et al., 2008), the cetane number (Liu et al., 2013) or the ester content (Herbinet et al., 2010).
However, the suitability to 3D CFD modeling with kinetic mechanisms composed by a large number of reactions, although developed on surrogate species, is deeply limited due to the large computational effort required for their solution. Different reduction methods have been developed in the literature (Lu and Law, 2006; Pepiot-Desjardins and Pitsch, 2008; Sun et al., 2010; Wen and Zhong, 2012), aimed at reducing the number of reactions and species involved in the combustion mechanism, and at simultaneously avoiding the risks in producing oversimplified mechanisms that may lead to erroneous modeling results. An overview of these methods is presented in the first part of Section Development of a Reduced Chemical Mechanism for Combustion of Gasoline-Alcohols.
Lastly, thermo-physical properties models are developed on extensive experimental data to provide temperature-dependent correlations or properties software to calculate the thermo-physical features of biofuels from various feedstock sources (Yuan et al., 2003; Stringer et al., 2007).
Biodiesel oxidation is generally described through the development of surrogate or reduced models due to the many classes of involved molecules (branched and straight chain paraffins, alkenes, cyclic paraffins, aromatics). However, this approach can be obviously applied to other biofuels, such as alcohol molecules to be used in spark ignition (SI) engines. Indeed, although these lasts are composed by single classes of molecules, an accurate description of the reactions related to the combustion and noxious emissions formations allows a proper characterization of the effect of these oxygenated fuels in ICEs CFD applications, where generally multi-component interactions take place as they are blended with gasoline in different percentages for anti-knocking purposes.
The present work is aimed at performing two different representative investigations on internal combustion engines powered by biofuels, to give a contribution to their optimization and support their diffusion within various fields of application.
After a first section dedicated to the description of the main production technologies of each biofuel considered in present work, and their impact on ICEs performances, the first proposed method is focused on the employment of a properly developed 3D CFD model for the simulation of a CI engine under dual-fuel (syngas and biodiesel) mode. A parametric analysis is here conducted by varying the percentage of syngas trapped in the combustion chamber at intake valve close (IVC) time. The combustion simulation is realized through a reduced kinetic scheme of reaction since the syngas components, under simplified assumptions, can be added to the intermediate species of a coherent flame model (CFM), without altering the underlying path to product formation.
In the second part, a multi-component reduced kinetic model for SI engines under alcohol oxidation is developed and validated in terms of ignition delay time, laminar flame speed characteristics and HCCI combustion.
Enabled by kinetic models, CFD simulations can be used to optimize fuel formulations for advanced internal combustion engines so that maximum engine efficiency, fossil fuel substitution and low pollutant emissions goals can be achieved.
Biofuels Production Technologies and Impact on ICEs
Figure 2 summarizes the current status of development and the variety of approaches to biofuel production existing nowadays. There are various forms of biofuels and most of them are obtained through processes having various stages with also various end use purposes.
Biodiesel
Biodiesel has widely become commercially available all over the world due to its characteristics approximately similar to diesel fuel, in particular in terms of cetane number (Valentino et al., 2011). Typical biodiesel can be derived from vegetable oils through blending, emulsification, thermal cracking and transesterification methods. In particular, transesterification of vegetable oil with alcohol leads to the production of the Fatty Acid Methyl Esters (FAME), generally referred to as biodiesel.
However, biodiesel features five methyl esters components characterized by a longer carbon length than conventional diesel fuel (Shudo et al., 2005), thus the increased viscosity may lead to operating difficulties and filter plugging, especially under cold climate conditions. Moreover, biodiesel degrades up to four times faster than diesel fuel (Chiu et al., 2004), damaging the injection system components with detrimental effects as injector coking or injector deposits problems especially in high-pressure fuel pump (Chiu et al., 2004). On the other hand, biodiesel is renewable and the oxygen content, generally over 10% in mass, may effectively reduce engine-out emissions of unburnt hydrocarbons (UHC), carbon monoxide (CO) and particulate matter (PM) in modern CI engines (Zheng et al., 2008), with a slight increase in the nitrogen oxides (NOx) emissions (Monyem et al., 2001; Yamane et al., 2001; Dorado et al., 2003; Cheng et al., 2006).
Tamilselvan et al. (2017) provided a literature review on the state-of-art of performances, combustion and emission characteristics of diesel engines under biodiesel fuelling. Numerous experimental data on more than 30 biodiesel fuels were studied, revealing that the lower heating value of biodiesel produces lower net heat release, lower brake thermal efficiency and higher brake specific fuel consumption (BSFC) than diesel fuel. Moreover, lower exhaust emissions such as HC, CO and PM and higher CO2 and NOx were produced, due to enriched oxygen content and higher cetane number that improve combustion.
Alcohols
As regards alcohols, from the chemical point of view, they are characterized by a hydroxyl group (–OH) at one of the molecule carbons atoms. The most common alcohol, bio-ethanol, accounts for more than 85% of the total biofuel use, mainly derived from fermentation of sugars or from chemical reactions of ethylene with steam. The steps for wide-scale ethanol production are: sugars fermentation, dehydration, distillation. Before fermentation, some crops undergo saccharification or carbohydrates hydrolysis (as starch and cellulose) into sugars. The microbial fermentation of these lasts lead to ethanol. The two main plants components, cellulose and starch, are both composed of sugars, and can be in principle converted to sugars through fermentation. Water must be removed if ethanol is used as a fuel, generally through distillation, where the purity is limited to 94–95% due to the formation of a low-boiling water-ethanol azeotrope (a mixture of two or more liquids whose proportions cannot be altered or changed by simple distillation). Lastly, the azeotropic distillation method adds a hydrocarbon benzene to denature the ethanol and make it undrinkable.
The butanol alcohol can be produced from fossil fuels as well from the same agricultural feedstock as ethanol. Renewable butanol (bio-butanol) derives from the ABE fermentation process of carbohydrates (since its main products are acetone, butanol and ethanol with carbon dioxide and hydrogen by-products (Jones and Woods, 1986). First, there is a growth stage, where acetic and butyric acids are produced, and then the acid re-assimilation into acetone, butanol and ethanol occurs.
Today, ethanol is the most common alternative fuel to replace gasoline in SI engine (Catapano et al., 2016). The molecule of ethanol is compact and stable, thus very resistant to autoignition as large activation energy is needed. Moreover, its research octane number is higher than gasoline, so knocking risk is partially avoided by the higher latent heat of vaporization. Moreover, the small molecular size and the presence of an oxygen atom could contribute decreasing soot particle formation for gasoline direct injection (GDI) engines (Varde et al., 2007; Kalghatgi, 2014; Catapano et al., 2016), while NO formation should be taken under control. However, as regards butanol, it has the potentiality to overcome the disadvantages brought by lower-carbon alcohols, as it is characterized by a higher heating value, due to increased carbon content, thus an engine running on bio-butanol is characterized by a lower fuel consumption than ethanol. Moreover, butanol heat of vaporization is less than half compared to ethanol, thus butanol-fuelled engine have less cold start problems and allows mixture formation over shorter intervals of time. Finally, butanol alcohol is safer in terms of transportation and high temperatures use due to the lower saturation pressure and the higher flash point in relation to ethanol (Trindade, 2016).
Syngas
Thermo-chemical conversion of solid biomass into another form of usable energy can be done through essentially three primary conversion technologies: through direct combustion, pyrolysis, and gasification. These primary conversion technologies can be also coupled with secondary conversion technologies for CHP production.
In particular, the solid fuel partial oxidation due to a gasifying agent (usually air, pure oxygen or steam) occurs during gasification process, to produce a renewable gaseous fuel called synthetic gas (syngas), this last composed by a mixture of carbon monoxide, hydrogen, methane, carbon dioxide, water vapor, nitrogen and liquid and solid by-products as char and TAR, and their composition largely vary according to the operative conditions chosen for the thermo-chemical conversion occurring in the reactor. La Villetta (2017), reported the influence of the main operating parameters, as gasifying agent, equivalence ratio, biomass moisture content and gasification temperature, on the quality of the producer gas.
Significant researches (Kaupp and Goss, 1981; Bade and Karim, 1999; Hasler and Nussbaumer, 1999; Sridhar et al., 2001; Li and Karim, 2005; Francisco et al., 2010; Pradhan et al., 2015) have been performed on studying and improving the operation of ICEs fuelled with syngas. Indeed, its quality is significantly poorer than gasoline and natural gas: the energy density of syngas is very low in comparison to natural gas, with a LHV ranging from 4 to 6 MJ/Nm3, that can be increased in the range of 9 to 13 MJ/Nm3 by using pure oxygen as gasifying agent (Francisco et al., 2010). Moreover, the producer gas low flame speed is more efficiently used in low speed engine, e.g., an engine with 1,500–2,500 rpm is ideal for producer gas application (Kaupp and Goss, 1981). The stoichiometric mass ratio in the air/producer gas mixture is between 1.0 and 1.2, compared to 17 for methane, thus an adequate mixing is needed for an engine to operate with high performance.
The estimated power reduction of a SI engine fuelled with syngas to about 40–50% is mainly due to the lower net calorific value of the mixture (Sridhar et al., 2001), whose 30% is due to the low energy density, while the rest is accounted by the pressure drop in the intake valves and piping. Therefore, the ignition timing in a spark ignition engine has to be advanced of about 30–40 degrees. On the other hand, CI engines have higher efficiency due to a greater compression ratio (Heywood, 1998), greater durability and generally require low maintenance than SI engines. Because of high compression ratio and low speeds, the derating of diesel engines running on producer gas is only between 15 and 30%. Therefore, syngas cannot be used in CI engines without a mean of initiating combustion, since the temperature at the end of the compression stroke is lower than the syngas autoignition temperature, but it is necessary to operate the engine in a dual-fuel mode, in which syngas is used as the primary fuel and is ignited by a pilot injection of biodiesel or diesel fuel (Costa et al., 2017).
CFD Analysis of CI Dual-Fuel Combustion With Biodiesel and Syngas
A 3D CFD model is developed within the AVL FireTM framework to reproduce the combustion process during the closed valve period of a CI engine fed with biodiesel fuel (B100) under different operative conditions and injection strategies (Costa et al., 2017). A turbocharged, water cooled, 4-valve, 4-cylinder CI diesel engine equipped with a cooled EGR system and a common rail injection system is considered. Engine characteristics are summarized in Table 1.
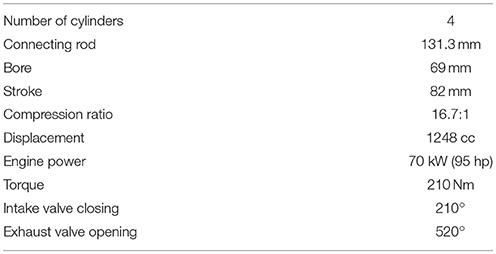
Table 1. Engine characteristics Costa et al. (2017).
The sub-models reproducing the spray behavior were assessed against an experimental database reported in the literature focused on the spray characterization of commercial biodiesel-diesel blends in a non-evaporating, high-density environment (Alfuso et al., 2005; Valentino et al., 2011). The injection pressure ranged between 97 and 115 MPa (Valentino et al., 2011). The injected fuel chosen in the software library, indicated as FAME-R, refers to biodiesel from rapeseed oil. The turbulent flow field follows the k-ζ-f model (Durbin, 1991; Hanjalic et al., 2004) in a Reynolds Averaged Navier Stokes (RANS) approach. The combustion chamber is modeled as shown in Figure 3; the mesh grid size and the imposed boundary conditions based on experimental data can be found in Costa et al. (2017).
The combustion sub-model implemented is the Extended Coherent Flame Model−3 Zones (ECFM-3Z) (Colin and Benkenida, 2003), tuned on the ground of experimental pressure cycles (Costa et al., 2017). This model belongs to the family of the Coherent Flame Models (CFM), based on the laminar flamelet concept assuming that the chemical time scales are way smaller than the turbulent ones, thus reactions take place within relatively thin layers separating the unburned gas from the fully burnt gas.
This engine is now hypothesized as switched to a dual-fuel operation, adding different percentages of syngas in the combustion chamber. Combustion phase is still described by the ECFM-3Z approach. Indeed, the fuel oxidation process in the flame is described through a reduced hydrocarbon oxidation scheme, followed in order to cover the high temperature oxidation process within the flame and in the post-flame region, respectively. Therefore, the syngas components, under simplified assumptions, can be added in the initial mixture in the combustion chamber and considered as intermediate species of the mechanism, without altering the underlying path to product formation.
Syngas components yield from gasification of woodchip biomass is derived through a properly developed thermo-chemical model (Costa and La Villetta, 2015). The in-cylinder pressure cycle, under only biodiesel fuelling [SOI equal to 357°, namely 3° Before Top Dead Centre (BTDC)] and at a speed of rotation of 2,750 RPM, is taken as reference condition in evaluating the effects of dual-fuel operation on engine performances.
In the perspective of considering the engine as part of a CHP unit, the influence of both the syngas percentage and start of injection is analyzed to optimize its performance according to the requested electrical or thermal load. The thermodynamic conditions of the air-syngas mixture at the IVC are obtained through the employment of a mixing component that allows to properly characterize the mixing of fresh air (at a temperature of 293 K) with different syngas percentages (supposed at a temperature of 333.15 K). Indeed, the temperature of the air-syngas mixture increases with the increasing syngas percentage considered. This lead to the counteracting effects of higher primary energy content in the combustion chamber but lower volumetric efficiency.
Thirty operative cases are considered in a range of variation of the SOI between 354° and 361°, while syngas percentages varies between 0 and 15% of the total trapped mass. This range defines the design of experiments (DOE) in the sub-sequent response surface method (RSM) study.
Figure 4 reports the evolution of the pressure curves and rate of heat release (ROHR) for nine reference cases. The amount of moisture content in the biomass is kept constant as its influence on the engine performances can be neglected (Costa et al., 2017). The reference case relative to only biodiesel injection is here neglected for the sake of clarity in the figure.
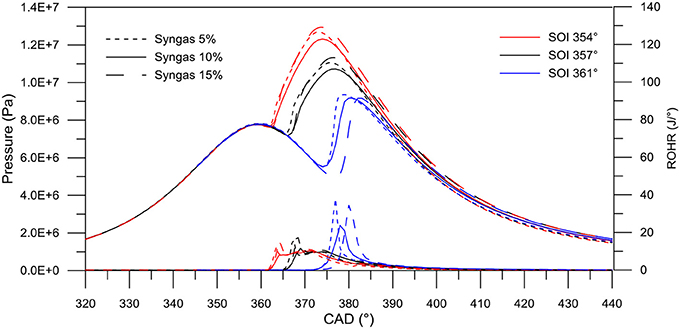
Figure 4. Numerical pressure cycle and ROHR for dual-fuel combustion with syngas in various percentages (source biomass with 16% moisture content) and different SOI.
Results show that an increasing syngas percentage results in a higher delay in the start of combustion, because of the CO slowing the combustion rate and prevailing on the enhancing effect of the H2 (Costa et al., 2017). Moreover, the relative increase in the primary energy content gives higher peaks of pressure and energy release, thus higher work done by the engine (as clearly reported later in the RSM analysis). Moreover, the effect of the SOI advancement clearly enhances the combustion efficiency, resulting in higher work produced.
The parametric analysis on the DOE space is used for the reconstruction of the Gaussian response surface in the DOE space. The results of this RSM (Gibbs and MacKay, 1996) in terms of mechanical and exhaust gases thermal power are shown in Figure 5 in the plane SOI–syngas percentage. The RSM method is used to analyse problems in which a target variable is influenced by a number of input variables having an unknown combined effect on it.
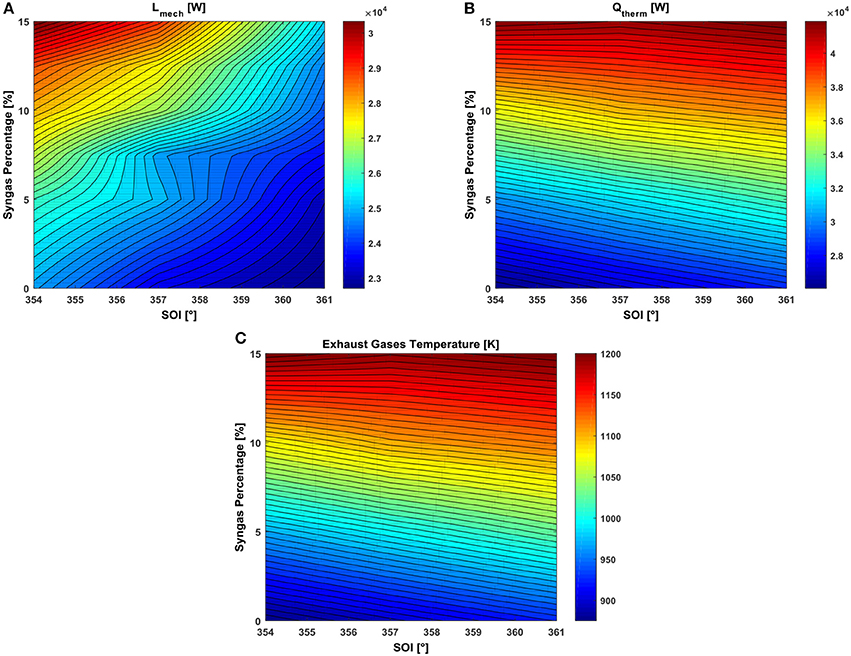
Figure 5. RSM plot in the SOI-syngas percentage plane of (A) closed valve engine power, (B) thermal power of the exhaust gases, and (C) exhaust gas temperature at EVO.
The engine mechanical power produced during the closed valve phase and the one related to the thermal energy of the exhaust gases (both expressed in Watt) are evaluated as:
where N is the engine speed in rev/min, exhaust is the exhaust gases flow rate expressed in kg/s while Tref is the reference temperature equal to 293.15 K. Each quantity takes into account the power produced by the whole engine, thus by the four cylinders.
In Figure 5A, the zone with higher mechanical power during the closed valve period is obtained for the most advanced SOI and the highest syngas percentage, thus the effect of the highest primary energy content in the cylinder overwhelm the one deriving from a lower volumetric efficiency. However, the highest thermal power of the exhaust gases evaluated at the exhaust valve open (EVO) is reached for a SOI of 361° (Figure 5B), consequent the highest temperature of the mixture (Figure 5C).
An analysis of the pollutant emissions can also be performed in this range. The numerically computed soot and NO emissions (expressed in ppm) at EVO are shown in Figure 6. NO formation follows the Zeldovich mechanism (Zeldovich et al., 1947), while the kinetic model developed at the University of Lund (Wang and Frenklach, 1997) is implemented to evaluate soot formation.
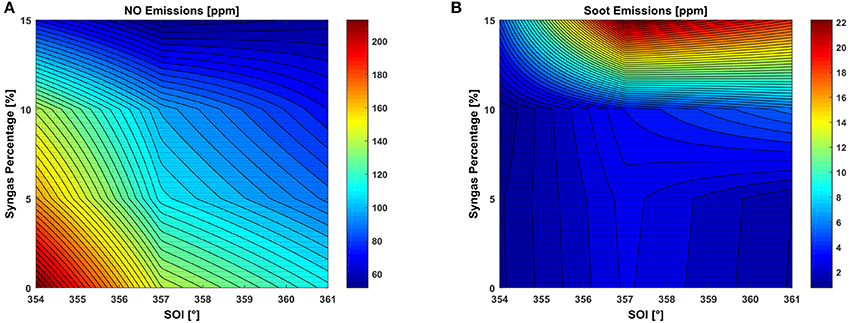
Figure 6. RSM plot in the SOI-syngas percentage plane of (A) NO emissions in ppm and (B) soot emissions in ppm at EVO.
As expected, higher NO values are visible in the zone where the SOI is the most advanced (354°) but also for the lowest syngas percentage trapped in the mixture. Indeed, the NO mass fraction is lower for high syngas percentages due to the reduced presence of oxygen, even though the maximum temperature reached in the combustion chamber is the highest (consequent to the highest pressure). Moreover, the classical trade-off between NO and soot is respected, as the lower amount of oxygen cannot oxidize the formed soot.
Finally, a comparison between the mechanical and thermal efficiencies is shown in Figure 7 for all the different dual-fuel operative conditions considered. These are defined respectively as the ratio, with respect to the primary energy content of the mixture, of the engine work produced during the closed valve phase or of the available energy at EVO. Although this last is obviously higher than the suitable amount that can be actually exploited, this quantity is considered valid as a comparison is made between different syngas amounts addition. Results show how increasing the syngas percentage in the combustion chamber, thus the primary energy content, enhances the mechanical power produced by the engine (Figure 5A) but also leads to a lower mechanical efficiency (Figure 7A), due to the reduced combustion efficiency consequent to the addition of CO. On the other hand, the addition of syngas increases the exhaust gases temperature, thus the engine thermal efficiency. Thus, as an overall conclusion, if CHP applications are considered, the users' demands shifts toward thermal energy production, instead than electrical, according to the increasing percentages of syngas, that result in a decrease of the combustion efficiency but in an increase of the thermal one.
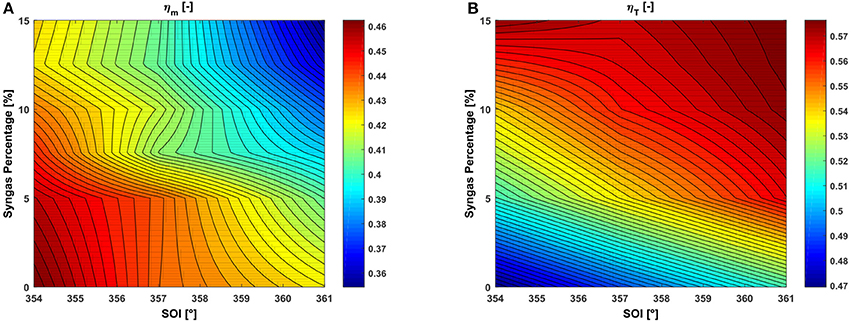
Figure 7. RSM plot in the SOI-syngas percentage plane of engine (A) mechanical and (B) thermal efficiencies.
Development of a Reduced Chemical Mechanism for Combustion of Gasoline-Alcohols
The approaches based on the development of biofuel kinetic models are largely followed to overcome the uncertainties and the complexity of the combustion occurring for this kind of fuels, as they are generally blended with gasoline in different percentages in SI engines for anti-knocking purposes. However, the chemical complexity and the uncertainties related to the measurements of these components lead researchers to rely on the idea of surrogate fuel model, that mimics the real fuel chemical and physical characteristics, employing less number of hydrocarbons components and requiring less computational time. Indeed, each fuel component should require its own set of combustion species and reactions but the resulting mechanism would be computationally prohibitive.
Thus, detailed mechanisms need to be reduced to the smallest possible size, but still leading to an accurate prediction of specific targets in the domain of interest. The skeletal reduction process identifies all reactions and species having little influence on the targets, removing them definitively from the mechanism, thus reducing the number of differential equations that have to be solved and accelerating the computation of source terms. Another route is replacing some differential equations with algebraic relations through quasi-steady-state assumption or species lumping. Despite the route chosen, it is essential to correctly identify the species that do not play an important role during the chemical processes (Stagni et al., 2013).
The skeletal reduction can be accomplished through the implementation of different methods such as Directed Relation Graph (DRG) (Lu and Law, 2006), DRG with error propagation (DRGEP) (Pepiot-Desjardins and Pitsch, 2008), path flux analysis (PFA) (Sun et al., 2010), computational singular perturbation (CSP) (Wen and Zhong, 2012) to eliminate the unimportant reactions and species. All these methods derive from the automatic approach DRG developed by Lu and Law (2006) for the generation of skeletal mechanisms at different level of complexities and domains of applicability. Given a threshold parameter ε, nodes of the DRG method correspond to species present in the mechanism. Thus, a direct edge exists from species A to species B only if the normalized interaction coefficient rAB, quantifying their mutual dependence, is greater than ε (Brakora et al., 2008). Generally, the DRG approach is almost the most effective methods among these methods, because their use is not only straightforward and effective treatment strategy but also need less computation cost (Liu et al., 2016).
As regards species lumping method, the critical issue is determining which species to lump, classifying the contribution of each selected species to the lumped ones, and estimating their kinetic parameters (Stagni et al., 2013). In the chemical lumping method, species are lumped according to their chemical structure (Liguras and Allen, 1992), while mathematically based approaches rely on formal transformation rules between original and lumped variables (Pinto et al., 1999). The first approach requires wide chemical expertise, e.g., at high temperatures, the large alkyl radicals and the reacting mixture interactions are weak, thus allowing their direct substitution with their primary decomposition or isomerization products. To be fair, H-abstraction or recombination reactions of heavy radicals may also take place, but due to their lower weight with respect to the decomposition ones, it is allowed to drastically reduce the total number of radicals and reactions to be considered (e.g., all the intermediate alkyl radicals higher than C4 can be instantaneously transformed into their final products). Similarly, in the low temperature mechanism, this method lumps together the primary intermediate radicals classes (alkenes, carbonyl hydroperoxides, cyclic esters) into single corresponding species (Stagni et al., 2013).
This section presents a reduced multi-component kinetic model for primary reference fuels (PRF, as n-heptane and isooctane), ethanol and butanol oxidation mechanisms, developed and validated against experimental data in terms of ignition delay time, laminar flame speed, and Homogeneous Charge Compression Ignition (HCCI) engine combustion. The reduced PRF mechanism developed by Wang et al. (2013) is integrated with an ethanol reduced oxidation mechanism, this last performed using the DRG (Lu and Law, 2006) method and sensitivity analysis approach. Then, this mechanism is integrated with a reduced butanol mechanism obtained after a combination of lumping, DRG and sensitivity analysis approaches (Piazzullo et al., 2017). The entire model consists of 197 species and 634 reactions.
Mechanisms Reduction and Validation
Among the various methods proposed to identify the most unimportant species and reaction pathways in a detailed kinetic mechanism and to reduce the number of variables (thus the computational effort), DRG method has been suggested to help automation of reduction procedures, thus it is used in the present work as a reduction tool.
The ethanol detailed mechanism developed by Mittal et al. (2014), consisting of 110 species and 540 reactions, is used as starting reference. Through the DRG approach, a new reduced mechanism consisting of 26 species and 118 reactions is generated, while the adjustment of reactions rate constants of selected reactions is performed against experimental data from literature in terms of ignition delay time and laminar flame speed. The primary oxidation pathways of C2H5OH → sC2H4OH → CH3CHO → CH3CO → CH3 are maintained in the present reduced mechanism due to its importance as demonstrated by Marinov (1999). This reduced mechanism is then integrated with a PRF mechanism developed and validated by Wang et al. (2014), keeping all the common species and reactions already present in the PRF mechanism. The final PRFE mechanism consists of 82 species and 313 reactions.
Moreover, the butanol detailed mechanism of Sarathy et al. (2014) (426 species and 2,335 reactions) is first reduced by applying the DRG method. Then, the lumping method is applied to the branching reactions of the primary butanol molecules, followed by reaction rate constants adjustment based on the ignition delay curve sensitivity analysis to match experimental validation data. Lumping approach generates mechanisms with drastically reduced number of species and reactions at the minimal expense of accuracy. In this approach, attempt to retain the isomers reaction pathways effects is made considering multiple decomposition reactions of the lumped species that involve the products from the detailed reaction pathways of the original isomers. However, butanol starting isomers and their immediate radicals are not lumped, and the fuel radical isomers to be retained are selected based on the reaction rate to generate the radicals (Piazzullo et al., 2017).
On the contrary, the H-abstraction reactions from butenols (C4H7OH) and their subsequent decomposition reactions were lumped to a single species and reaction pathway regardless of parent butanol isomers, as those reactions are mainly high-temperature reactions and the reaction rate difference between the butenol isomers is relatively small. The reduction processes are limited to the reactions involving 4-carbon number (C4) species, while the C1-C3 reactions and H2-CO sub-mechanisms of the detailed mechanism are retained to facilitate the integration of the butanol mechanism into the PRFE mechanism. The final PRFBE mechanism consists of 195 species and 692 reactions. It is obviously validated against experimental measurements of ignition delay and laminar flame speed for neat iso-octane, n-heptane, ethanol, four butanol isomers, and their blends, but for the sake of brevity, the validations shown here are performed with the final mechanism. Other results can be found in Piazzullo et al. (2017).
Figure 8 shows the validation in terms of ignition delay times at constant volume n-heptane/iso-octane/ethanol/air mixtures compared with shock tube test data of (Haas et al., 2009). Moreover, Figure 9 shows the ignition delay times of butanol-isomers/air mixture at constant volume (Weber et al., 2011). Equivalence ratio ranges from 0.5 to 2, while pressures from 10 to 50 atm.
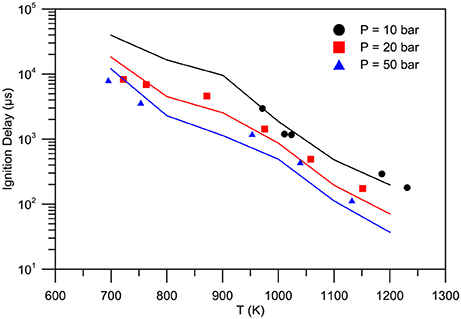
Figure 8. Experimental (symbols) - numerical (lines) comparison of ignition delay times for ethanol/PRF blend (volume fractions: 18% n-heptane, 62% iso-octane, 20% ethanol): P = 10 bar (spheres), P = 20 bar (squares), P = 50 bar (triangles).
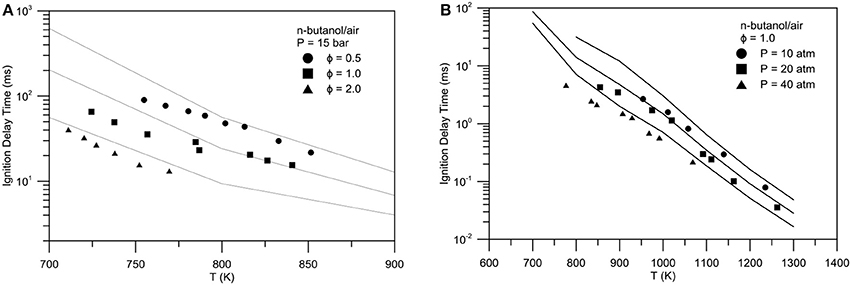
Figure 9. Experimental (symbols)-numerical (lines) comparison of ignition delay times for n-butanol/air mixture: (A) Pressure = 15 bar, equivalence ratio = 0.5 (spheres), 1.0 (squares), 2.0 (triangles), (B) equivalence ratio = 1.0, P = 10 atm (spheres), P = 20 atm (squares), P = 40 atm (triangles).
The present mechanism is also validated with laminar flame speed predictions. Figure 10 shows comparison of laminar flame speed predictions and experimental data (Marinov, 1999) for ethanol/air mixtures for a range of unburned gas pressures of 0.1–0.2 MPa, unburned gas temperatures of 300–453 K, and equivalence ratios of 0.7–1.4. Figure 11 shows the laminar flame speed propagation for various butanol/air mixtures. The proposed model well predicts the experimental data for all four isomers for a temperature of 343 K and atmospheric pressure by Sarathy et al. (2014).
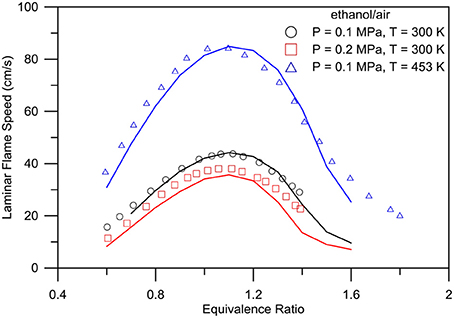
Figure 10. Experimental (symbols) - numerical (lines) comparison of laminar flame speeds for ethanol/air mixture. Operative conditions: P = 0.1 MPa, T = 300 K (spheres), P = 0.2 MPa, T = 300 K (squares), P = 0.1 MPa, T = 453 K (triangles).
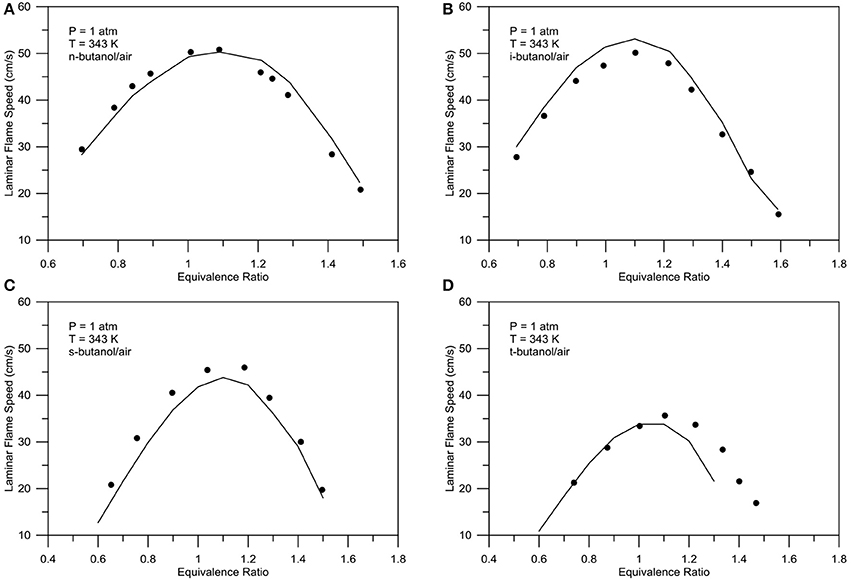
Figure 11. Experimental (symbols) - numerical (lines) comparison of laminar flame speed for n-butanol (A), iso-butanol (B), s-butanol (C), t-butanol (D) blends with air. Pressure = 1 atm, T = 343 K.
Lastly, a 3D CFD code, KIVA3VR2-CHEMKIN was developed to perform numerical simulations of n-butanol/n-heptane/air HCCI simulation. Uniform pre-mixture at the intake valve closure is assumed, while computation is done with a 0.5-degree sector grid of a flat piston engine cylinder (Viggiano and Magi, 2012). Engine features and initial and boundary conditions are reported in Table 2. Figures 12 compare pressure and heat release predictions and experimental measurements. Generally, the reduced mechanism correctly reproduces the in-cylinder pressures and heat release rates for all cases. The auto-ignition and combustion processes are deemed to be well reproduced with the present mechanism. Lastly, Figure 13 shows the composition, in volume fractions, of the main species characterizing the exhaust gases produced in the HCCI combustion simulation performed as a function of the equivalence ratio.
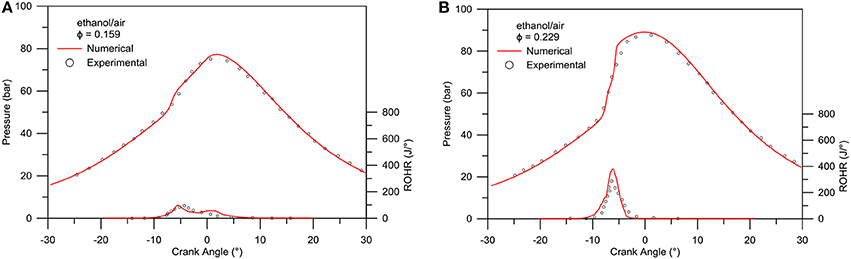
Figure 12. Experimental (symbols) - numerical (lines) comparison of pressures and rate of heat release for ethanol/air mixtures: (A) φ = 0.159, (B) φ = 0.229.
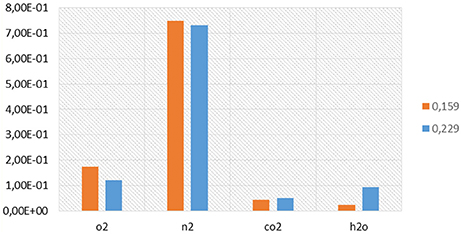
Figure 13. Volume fractions of the main species present in the exhausts composition at EVO for the HCCI combustion simulations performed under ethanol/air mixture for an equivalence ratio of 0.159 (orange) and 0.229 (blue).
Conclusions
Nowadays, fossil fuels combustion is the dominant global source of pollutant emissions and related negative effects on environment and climate change. Thus, the need to act with appropriate measures to achieve economic, social and environmental objectives is strong as the optimization of energy conversion systems, the reduction of fuels consumption and the containment of pollutant emissions released into the atmosphere (particulate matter, nitrogen oxides NOx, unburned hydrocarbons, carbon dioxide CO2).
In the last decades, efforts have been made to protect our planet from further deterioration, leading the way to a large-scale seeking for renewable sources of energy. A viable option is resorting to biofuels.
Present work is focused on the development and description of different numerical approaches for the investigation and optimization of energy systems powered by biofuels. CFD combustion modeling is taken into account following two different approaches: the first based on the implementation of the ECFM-3Z combustion model with reduced chemistry to reproduce the performances of a CI engine for CHP production operating in a dual-fuel mode with premixed syngas and biodiesel pilot injection; the second through a species transport approach with detailed kinetics for alcohols oxidation.
These approaches are here presented to provide suitable solutions, with low computational efforts, to overcome the issues deriving from the high variability in quality and compositions of different biofuels, that cannot be solved through the implementation of the already assessed combustion models developed for fossil fuels combustion.
Increasing percentages of syngas decrease combustion efficiency, while exhaust gas temperature and the consequent thermal efficiency is increased for retarded SOI. Therefore, these parameters can be chosen according to the users' demands shifted toward thermal or electrical energy, if CHP applications are considered. Moreover, syngas addition reduces NO formation but increases soot production: this last can be reduced with a retarded SOI.
Moreover, a reduced reaction kinetics mechanism for PRF-ethanol-butanol combustion generated by combining different reduced mechanisms for alcohols oxidation performs well in multi-component oxidation conditions, as well as in neat fuel oxidation conditions. Future development of this mechanism will be focused on the addition of reactions relevant to the NO formation, and aromatic compounds as toluene for the soot production, to assess its performance in 3D CFD SI engine combustion simulations.
Author Contributions
MC contributed to the development, correction and writing of the whole paper. DP contributed to the development and writing of the whole paper.
Conflict of Interest Statement
The authors declare that the research was conducted in the absence of any commercial or financial relationships that could be construed as a potential conflict of interest.
References
Alfuso, S., Allocca, L., Caputo, G., and Corcione, F. E. (2005). Experimental Investigation of a Spray from a Multi-Jet Common Rail Injection System for Small Engines, SAE Technical Paper No. 2005-24-90.
Bade, S. O. S., and Karim, G. A. (1999). Hydrogen as an additive to methane for spark ignition engine applications. Int. J. Hydrogen Energy 24, 577–586. doi: 10.1016/S0360-3199(98)00103-7
Bergthorson, J. M., and Thomson, M. J. (2015). A review of the combustion and emissions properties of advanced transportation biofuels and their impact on existing and future engines. Renewable Sus. Energy Rev. 42, 1393–1417. doi: 10.1016/j.rser.2014.10.034
Brakora, J. L., Ra, Y., Reitz, R. D., McFarlane, J., and Daw, C. S. (2008). Development and validation of a reduced reaction mechanism for biodiesel-fueled engine simulations. SAE Inter. J. Fuels Lubricants 1, 675–702. doi: 10.4271/2008-01-1378
Catapano, F., Sementa, P., and Vaglieco, B. M. (2016). Air-fuel mixing and combustion behavior of gasoline-ethanol blends in a GDI wall-guided turbocharged multi-cylinder optical engine. Renewable Energy 96, 319–332. doi: 10.1016/j.renene.2016.04.087
Cheng, A. S., Upatnieks, A., and Mueller, C. J. (2006). Investigation of the impact of biodiesel fuelling on NOx emissions using an optical direct injection diesel engine. Int. J Engine Res. 7, 297–318. doi: 10.1243/14680874JER05005
Cheng, X., Ng, H. K., Gan, S., and Ho, J. H. (2013). Advances in computational fluid dynamics (CFD) modeling of in-cylinder biodiesel combustion. Energy Fuels 27, 4489–4506. doi: 10.1021/ef4005237
Chiu, C. W., Schumacher, L. G., and Suppes, G. J. (2004). Impact of cold flow improvers on soybean biodiesel blend. Biomass Bioenergy 27, 485–491. doi: 10.1016/j.biombioe.2004.04.006
Colin, O., and Benkenida, A. (2003). The 3-Zones extended coherent flame model (ecfm-3z) for computing premixed/diffusion combustion. Oil Gas Sci. Technol. Rev. IFP Energies Nouvelles 58, 47–62. doi: 10.2516/ogst:2004043
Costa, M., La Villetta, M., Massarotti, N., and Piazzullo, D. (2017). Numerical analysis of a compression ignition engine powered in the dual-fuel mode with syngas and biodiesel. Energy 137, 969–979. doi: 10.1016/j.energy.2017.02.160
Costa, M., La Villetta, M, and Massarotti, N. (2015). Optimal tuning of a thermo-chemical equilibrium model for downdraft biomass gasifiers. Chem. Eng. Trans. 37, 343–348. doi: 10.3303/CET1543074
Dorado, M. P., Ballesteros, E., Arnal, J. M., and Gomez, J. (2003). Exhaust emissions from a diesel engine fuelled with transesterified waste olive oil. Fuel 82, 1311–1315. doi: 10.1016/S0016-2361(03)00034-6
Durbin, P. A. (1991). Near-wall turbulence closure modelling without damping functions. J. Theor. Comput. Fluid Dyn. 3, 1–13.
Francisco, R. W. Jr., Rua, F., Costa, M., Catapan, R. C., and Oliveira, A. A. M. (2010). On the combustion of hydrogen-rich gaseous fuels with low calorific value in a porous burner. Energy Fuels 24, 880–887. doi: 10.1021/ef9010324
Gibbs, M. N., and MacKay, D. J. C. (1996). Efficient Implementation of Gaussian Processes for Interpolation, Department of Physics. Cavendish Laboratory, Cambridge University, Technical Report.
Haas, F. M., Chaos, M., and Dryer, F. L. (2009). Low and intermediate temperature oxidation of ethanol and ethanol–PRF blends: an experimental and modeling study. Combustion Flame 156, 2346–2350. doi: 10.1016/j.combustflame.2009.08.012
Hanjalic, K., Popovac, M., and Hadziabdic, M. (2004). A robust near-wall elliptic-relaxation eddy-viscosity turbulence model for CFD. Int. J. Heat. Fluid Flow 25, 1047–1051. doi: 10.1016/j.ijheatfluidflow.2004.07.005
Hasler, P., and Nussbaumer, T. (1999). Gas cleaning for IC engine applications from fixed bed biomass gasification. Biomass Bioenergy 16, 385–395.
Herbinet, O., Pitz, W. J., and Westbrook, C. K. (2010). Detailed chemical kinetic mechanism for the oxidation of biodiesel fuels blend surrogate. Comb. Flame 157, 893–908. doi: 10.1016/j.combustflame.2009.10.013
IEA Technology Roadmap (2017). IEA Technology Roadmap. Available online at: https://www.iea.org/publications/freepublications/ publication/Technology_Roadmap_Delivering_Sustainable_Bioenergy.pdf (Accessed Jan 8, 2018).
Jones, D. T., and Woods, D. R. (1986). Acetone-butanol fermentation revisited. Microbiol. Rev. 50, 484–524.
Kaupp, A., and Goss, J. R. (1981). State of the Art Report for Small Scale (to 50 kW) Gas Producer-Engine Systems. US Forest Service.
Kim, Y. D., Yang, C. W., and Kim, B. J. (2016). Fischer–Tropsch diesel production and evaluation as alternative automotive fuel in pilot-scale integrated biomass-to-liquid process. Appl. Energy 180, 301–312. doi: 10.1016/j.apenergy.2016.07.095
La Villetta, M. (2017). Biomass-to-Energy Systems: Techno-Economic Aspects and Modelling Approaches for Characterization and Improvement of Performance. Ph.D. thesis, University of Naples Parthenope.
Li, H., and Karim, G. A. (2005). Exhaust emissions from an SI engine operating on gaseous fuel mixtures containing hydrogen. Inter. J. Hydrogen Energy 30, 1491–1499. doi: 10.1016/j.ijhydene.2005.05.007
Liguras, D. K., and Allen, D. T. (1992). Comparison of lumped and molecular modeling of hydropyrolysis. Ind. Eng. Chem. Res. 31, 45–53. doi: 10.1021/ie00001a007
Liu, C., Zuo, Z., and Feng, H. (2016). Systematic reduction of the detailed kinetic mechanism for the combustion of n-Butane. J. Chem. 2016:8153582. doi: 10.1155/2016/8153582
Liu, W., Sivaramakrishnan, R., Davis, M. J., Som, S., Longman, D. E., and Lu, T. F. (2013). Development of a reduced biodiesel surrogate model for compression ignition engine modeling. Proc. Comb. Inst. 34, 401–409. doi: 10.1016/j.proci.2012.05.090
Lu, T., and Law, C. K. (2006). Linear time reduction of large kinetic mechanisms with directed relation graph: n-Heptane and iso-octane. Comb. Flame 144, 24–36. doi: 10.1016/j.combustflame.2005.02.015
Luo, Z., Plomer, M., Lu, T., Som, S., Longman, D. E., Sarathy, S. M., et al. (2012). A reduced mechanism for biodiesel surrogates for compression ignition engine applications. Fuel 99, 143–153. doi: 10.1016/j.fuel.2012.04.028
Marinov, N. M. (1999). A detailed chemical kinetic model for high temperature ethanol oxidation. Int. J. Chem. Kinet. 31, 183–220. doi: 10.1002/(SICI)1097-4601(1999)31:3<183::AID-KIN3>3.0.CO;2-X
Mittal, G., Burke, S. M., Davies, V. A., and Parajuli, B. (2014). Autoignition of ethanol in a rapid compression machine. Comb. Flame 161, 1164–1171. doi: 10.1016/j.combustflame.2013.11.005
Monyem, A., Van gerpen, J. H., and Canakci, M. (2001). The effect of timing and oxidation on emissions from biodiesel-fueled engines, Trans. Am. Soc. Agric. Eng. 44, 35–42. doi: 10.13031/2013.2301
Pepiot-Desjardins, P., and Pitsch, H. (2008). An efficient error-propagation-based reduction method for large chemical kinetic mechanisms. Comb. Flame 154, 67–81. doi: 10.1016/J.COMBUSTFLAME.2007.10.020
Piazzullo, D., Costa, M., Ra, Y., Rocco, V., and Ullal, A. (2017). “Development of a reduced chemical mechanism for combustion of gasoline-biofuels,” in SAE Technical Paper 2017-24-0039.
Pinto, F., Costa, P., Gulyurtlu, I., and Cabrita, I. (1999). Pyrolysis of plastic wastes. 1. effect of plastic waste composition on product yield. J. Anal. Appl. Pyrolysis 51, 39–55. doi: 10.1016/S0165-2370(99)00008-X
Pradhan, A., Baredar, P., and Kumar, A. (2015). Syngas as an alternative fuel used in internal combustion engines: a review. J. Pure Appl. Sci. Technol. 5, 51–66. doi: 10.9734/AIR/2017/32896
Sarathy, S. M., Oßwald, P., Hansen, N., and Kohse-Höinghaus, K. (2014). Alcohol combustion chemistry. Prog. Energy Comb. Sci. 44. 40–102. doi: 10.1016/j.pecs.2014.04.003
Shudo, T., Fujibe, A., Kazahaya, M., and Aoyagi, Y. (2005). “The cold flow performance and the combustion characteristics with ethanol blended biodiesel fuel,” in SAE Technical Paper No 2005-01-3707.
Sridhar, G., Paul, P. J., and Mukunda, H. S. (2001). Biomass derived producer gas as a reciprocating engine fuel- an experimental analysis. Biomass Bioenergy 21, 61–72. doi: 10.1016/S0961-9534(01)00014-9
Stagni, A., Cuoci, A., Frassoldati, A., Faravelli, T., and Ranzi, E. (2013). Lumping and reduction of detailed kinetic schemes: an effective coupling. Ind. Eng. Chem. Res. 53, 9004–9016. doi: 10.1021/ie403272f
Stringer, V., McCrady, J., Hansen, A., and Lee, C. F. (2007). Modeling Biodiesel Spray Breakup with Well-Defined Fuel Properties. Chicago, IL: ILASS-Americas.
Sun, W., Chen, Z., Gou, X., and Ju, Y. (2010). A path flux analysis method for the reduction of detailed chemical kinetic mechanisms. Comb. Flame 157, 1298–1307. doi: 10.1016/j.combustflame.2010.03.006
Tamilselvan, P., Nallusamy, N., and Rajkumar, S. (2017). A comprehensive review on performance, combustion and emission characteristics of biodiesel fuelled diesel engines. Renewable. Sus. Energy Rev. 79, 1134–1159. doi: 10.1016/j.rser.2017.05.176
Trindade, W. R. D. S. (2016). N-butanol as Biofuel Characteristics and Evaluation of its Application in Internal Combustion Engines through 1D Simulation. Master Thesis, Universidade Estadual de Campinas.
Valentino, G., Allocca, L., Iannuzzi, S., and Montanaro, A. (2011). Biodiesel/mineral diesel fuel mixtures: spray evolution and engine performance and emissions characterization. Energy 36, 3924–3932. doi: 10.1016/j.energy.2010.10.052
Varde, K., Jones, A., Knutsen, A., Mertz, D., and Yu, P. (2007). Exhaust emissions and energy release rates from a controlled spark ignition engine using ethanol blends. Proc. Inst. Mech. Eng. Part D J. Automob. Eng. 221, 933–941. doi: 10.1243/09544070JAUTO179
Viggiano, A., and Magi, V. (2012). A comprehensive investigation on the emissions of ethanol HCCI engines. Appl. Energy 93, 277–287. doi: 10.1016/j.apenergy.2011.12.063
Wang, H., and Frenklach, M. (1997). A detailed kinetic modeling study of aromatics formation in laminar premixed acetylene and ethylene flames. Comb. Flame 110, 173–221.
Wang, H., Yao, M., and Reitz, R. D. (2013). Development of a reduced primary reference fuel mechanism for ic engine combustion simulations. Energy Fuels 27, 7843–7853. doi: 10.1021/ef401992e
Wang, H., Zheng, Z., Yao, M., and Reitz, R. D. (2014). An experimental and numerical study on the effects of fuel properties on the combustion and emissions of low-temperature combustion diesel engines. Comb. Sci. Technol. 186, 1795–1815. doi: 10.1080/00102202.2014.920836
Weber, B. W., Kumar, K., Zhang, Y., and Sung, C. J. (2011). Autoignition of n-butanol at elevated pressure and low-to-intermediate temperature. Combustion Flame 158, 809–819. doi: 10.1016/j.combustflame.2011.02.005
Wen, F., and Zhong, B. (2012). Skeletal mechanism generation based on eigenvalue analysis method. Acta Physico Chem. Sinica 28, 1306–1312.
Westbrook, C. K. (2013). Biofuels combustion. Annu. Rev. Phys. Chem. 64, 201–219. doi: 10.1146/annurev-physchem-040412-110009
Williams, C. L., Westover, T. L., Emerson, R. M., Tumuluru, J. S., and Li, C. (2016). Sources of biomass feedstock variability and the potential impact on biofuels production. BioEnergy Res. 9, 1–14. doi: 10.1007/s12155-015s-9694-y
Yamane, K., Ueta, A., and Shimamoto, Y. (2001). “Influence of physical and chemical properties of biodiesel fuel on injection, combustion and exhaust emission characteristics in a DI-CI engine,” in Proceedings of the 5th International Symposium on Diagnostics and Modelling of Combustion in Internal Combustion Engines (Nagoya: COMODIA), 402–409.
Yuan, W., Hansen, A. C., and Zhang, Q. (2003). Predicting the physical properties of biodiesel for combustion modeling. Trans. ASAE 46, 1487–1493. doi: 10.13031/2013.15631
Zeldovich, Y. B., Sadovnikov, P. Y., and Frank-Kamenetskii, D. A. (1947). Oxidation of Nitrogen in Combustion. Moscow: Academy of Sciences of USSR, Institute of Chemical Physics.
Keywords: CFD, biofuels, biodiesel, syngas, dual-fuel, CHP, alcohols
Citation: Costa M and Piazzullo D (2018) Biofuel Powering of Internal Combustion Engines: Production Routes, Effect on Performance and CFD Modeling of Combustion. Front. Mech. Eng. 4:9. doi: 10.3389/fmech.2018.00009
Received: 09 April 2018; Accepted: 26 July 2018;
Published: 22 August 2018.
Edited by:
Evangelos G. Giakoumis, National Technical University of Athens, GreeceReviewed by:
John Nuszkowski, University of North Florida, United StatesKhanh Duc Cung, Argonne National Laboratory (DOE), United States
Hu Wang, Tianjin University, China
Copyright © 2018 Costa and Piazzullo. This is an open-access article distributed under the terms of the Creative Commons Attribution License (CC BY). The use, distribution or reproduction in other forums is permitted, provided the original author(s) and the copyright owner(s) are credited and that the original publication in this journal is cited, in accordance with accepted academic practice. No use, distribution or reproduction is permitted which does not comply with these terms.
*Correspondence: Daniele Piazzullo, ZGFuaWVsZS5waWF6enVsbG9Ac3R1ZGVudHMudW5pcm9tYTIuZXU=