- 1School of Biomedical Engineering, Faculty of Medicine, Dalian University of Technology, Dalian, China
- 2Stem Cell Clinical Research Center, National Joint Engineering Laboratory, Regenerative Medicine, The First Affiliated Hospital of Dalian Medical University, Dalian, China
- 3Dalian Innovation Institute of Stem Cell and Precision Medicine, Dalian, China
- 4Department of Radiology, The First Affiliated Hospital of Dalian Medical University, Dalian, China
- 5Faculty of Information Technology, University of Jyväskylä, Jyväskylä, Finland
Vascular cognitive impairment (VCI) encompasses a diverse range of syndromes, including mild cognitive impairment and vascular dementia (VaD), primarily attributed to cerebrovascular lesions and vascular risk factors. Its prevalence ranks second only to Alzheimer’s disease (AD) in neuro diseases. The advancement of medical imaging technology, particularly magnetic resonance imaging (MRI), has enabled the early detection of structural, functional, metabolic, and cerebral connectivity alterations in individuals with VCI. This paper examines the utility of multimodal MRI in evaluating structural changes in the cerebral cortex, integrity of white matter fiber tracts, alterations in the blood–brain barrier (BBB) and glymphatic system (GS) activity, alteration of neurovascular coupling function, assessment of brain connectivity, and assessment of metabolic changes in patients with VCI.
Introduction
Vascular cognitive impairment (VCI) primarily arises from vascular risk factors and cerebrovascular diseases (van der Flier et al., 2018; Corriveau et al., 2016), encompassing mild cognitive impairment, vascular dementia (VaD), and varying levels of cognitive decline resulting from mixed pathologies such as comorbid Alzheimer’s disease (AD) (Zhang et al., 2019). VCI is further classified into four subtypes based on stroke history and clinicopathologic/imaging features: post-stroke vascular cognitive impairment (PSCI), subcortical ischemic vascular cognitive impairment (SVCI), multiple infarct vascular cognitive impairment, and mixed dementia with other neurodegenerative pathologies (Iadecola et al., 2019).
VCI may arise from various etiologies related to cerebrovascular diseases, such as cerebral small-vessel disease (CSVD), large-artery atherosclerosis, cerebral hemorrhage, cardiogenic embolism, and uncommon stroke etiologies (Dichgans and Leys, 2017). Currently, the etiology of VCI remains uncertain, with potential pathogenic mechanisms including neuronal injury resulting from cerebral hypoperfusion and hypoxia, destruction of myelin sheaths and loss of axons (Rosenberg et al., 2016; Du et al., 2019; Bálint et al., 2019), intracerebral homeostatic imbalance caused by dysfunction of the neurovascular unit such as disruption of the blood–brain barrier (BBB) (Lee et al., 2017; Parfenov et al., 2019), oxidative stress-induced damage to neurons, and the development of an intracerebral pro-inflammatory microenvironment in response to neuroinflammatory cascades (Parfenov et al., 2019; Liu et al., 2018). Moreover, patients with VCI exhibit abnormalities in lipid metabolism (Liu et al., 2020), glucose metabolism (Ma et al., 2018), and amino acid metabolism (Hosoki et al., 2023). Consequently, analyzing alterations in brain microstructure, function, metabolism and connectivity in VCI patients can inform targeted clinical interventions. Nevertheless, the evaluation of cognitive function changes in VCI patients in clinical settings predominantly relies on subjective cognitive scales, which are highly subjective and cannot reflect the changes in brain structure, function, metabolism, and connectivity in the process of cognitive dysfunction in VCI patients. The advancement of imaging technology has led to the utilization of multimodal magnetic resonance imaging (multi-MRI) technology in evaluating cognitive status in patients with VCI. Structural MRI (sMRI) sequences offer insights into brain morphology and structural alterations in VCI patients, while functional MRI (fMRI) can quantitatively analyze changes in cerebral perfusion, BBB permeability, cerebral hypoxic microenvironment, iron deposition, brain function connections, and glymphatic system (GS) activity, etc. in VCI patients. Metabolic MRI can provide accurate and quantitative assessments of glucose, lipid, and amino acid metabolism, as well as detect changes in tissue osmolality, neurotransmitters, mitochondrial function, etc. The integration of multimodal MRI for intelligent analysis of brain connectivity offers a novel approach to evaluating the brain network of patients with VCI in both resting and dynamic states. This review summarizes the current research progress in the utilization of multi-MRI techniques for the diagnosis and treatment of VCI (as shown in Figure 1).
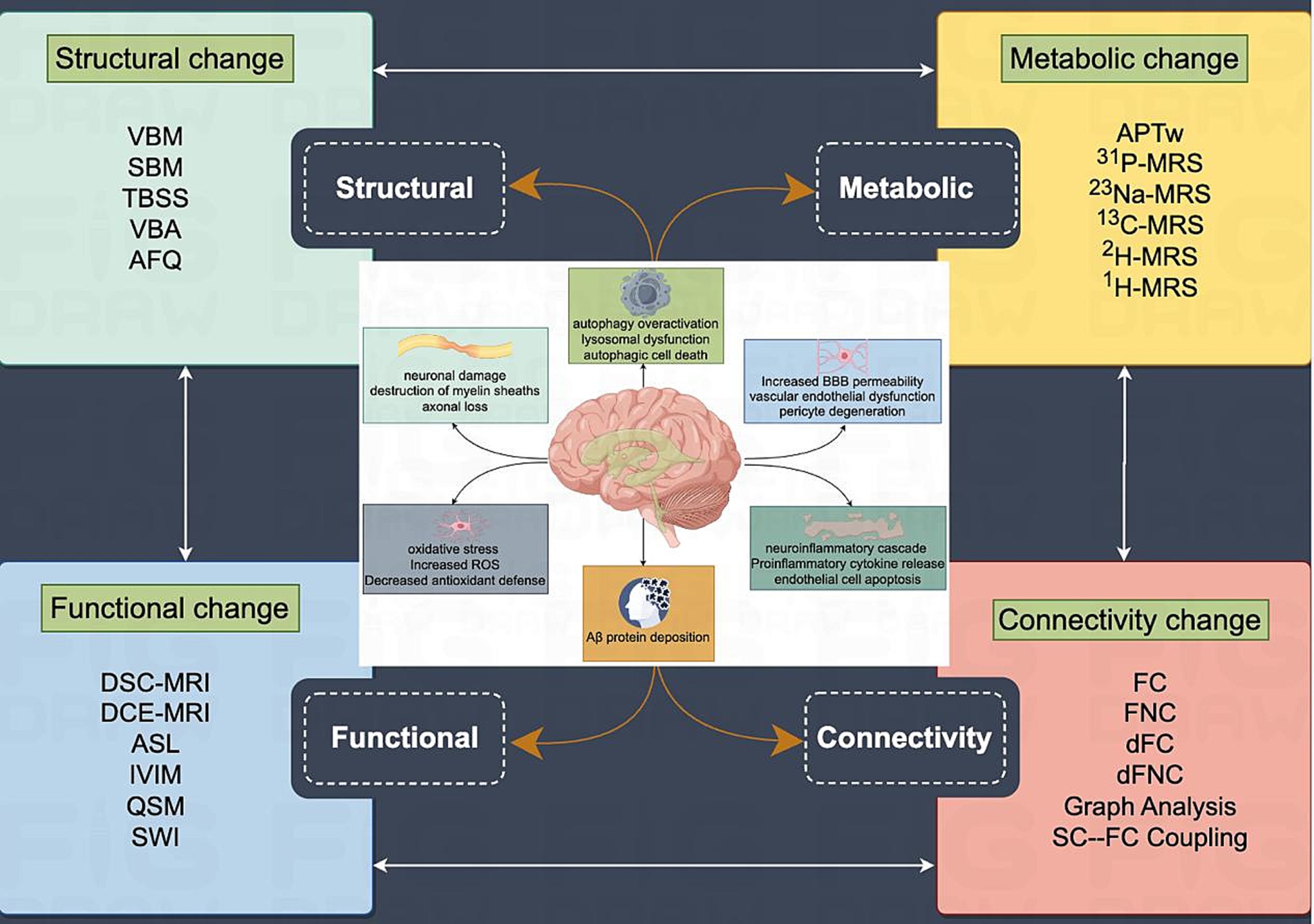
Figure 1. Pathophysiologic alterations and multimodal MRI assessment system for VCI: individuals diagnosed with VCI may exhibit pathophysiological alterations, including neuronal injury, myelin destruction, axonal loss, dysfunction of the neurovascular unit, oxidative stress-induced damage to neuronal cells, and activation of a neuroinflammatory cascade resulting in the formation of a pro-inflammatory microenvironment within the brain. These changes ultimately contribute to modifications in brain structure, function, metabolism, and connectivity. The advancement of MRI imaging technology has enabled the utilization of multimodal MRI and associated post-processing techniques to effectively delineate alterations in brain structure, function, metabolism, and connectivity. Images were produced using FigDraw software (www.figdraw.com) (DOI: PPARW86662).
Evaluation of the integrity of white matter fiber bundles in VCI patients
Chronic hypoperfusion and disruption of the neurovascular microenvironment have been shown to have detrimental consequences on neuronal homeostasis, resulting in neuronal death and chronic demyelination (Du et al., 2019; Liesz, 2019). The deficiency of myelin significantly compromises the integrity of white matter fiber tracts, ultimately leading to impaired cognitive function in the brain (Iadecola, 2013). Therefore, evaluating the integrity of white matter fiber bundles in patients with VCI can provide valuable insights into the degree of cognitive impairment present, thereby informing clinical interventions.
The rapid development of non-invasive neuroimaging technology has spawned a series of novel studies exploring the developmental patterns of the brain. MRI signals are used to obtain information such as image data, index parameters and other information, which can then discriminate the microstructural properties of different tissues. Currently, imaging studies probing tissue microstructure generally favor diffusion tensor imaging (DTI) metrics that respond to the diffusion properties of water molecules in white matter. DTI is a non-invasive neuroimaging technique that allows for the quantification of water molecule diffusion tensors and detailed evaluation of white matter fiber bundles. In recent years, DTI has been extensively utilized for the assessment of white matter microstructure (Le Bihan et al., 2001; Pierpaoli and Basser, 1996). Methods for analyzing the integrity of white matter fiber bundles using DTI primarily encompass region of interest (ROI)-based analysis, fiber bundle-based analysis, and voxel-based analysis. Fiber-based Tracer Spatial Statistical (TBSS) analysis is a voxel-based diffusion tensor imaging (DTI) data analysis technique employed to derive metrics such as fiber length, fiber integrity, and quantitative parameters, including fractional anisotropy (FA) and mean diffusivity (MD), of white matter fiber bundles through the mapping of brain white matter fibers (Smith et al., 2007). In contrast, the voxel-based analysis (VBA) analysis allows for statistical analysis of tensor metrics, including FA and MD values, computed during data preprocessing, to compare between-group differences at the whole-brain voxel level (McWilliam et al., 2023). The TBSS analysis method addresses issues related to alignment errors and smoothing kernel selection that are present in the VBA method, while also mitigating the bias in individual measurements and improving the accuracy of lesion localization compared to hand-drawn ROIs. However, the method’s skeletonization of fiber bundles disrupts the correspondence between diffusion parameters and actual anatomical structures, leading to challenges in result interpretation. Additionally, TBSS is limited in its ability to analyze specific fiber bundles. Furthermore, the TBSS method may not ensure precise analysis due to the potential discontinuity of the white matter skeleton in regions where fibers intersect (Smith et al., 2007). To address the aforementioned challenges associated with fiber bundle analysis in intersection regions, the implementation of automatic fiber quantification (AFQ) offers substantial improvements in the examination of white matter fiber intersections. This is achieved through the processes of fiber bundle segmentation, intersection identification, and the separation and quantification of fiber bundles (Yeatman et al., 2012). Concurrently, fiber bundle quantification, also known as tractometry, effectively captures the local microstructural heterogeneity within intersection regions, thereby facilitating the resolution of multifiber intersections with enhanced robustness and precision (Rheault et al., 2020). Recent research studies (Kim et al., 2016; Chen et al., 2018; Mascalchi et al., 2019; Liu et al., 2019b) have demonstrated that the TBSS analysis method offers a more objective quantitative assessment of white matter fibers, presenting a novel functional imaging evaluation approach for diagnosing and predicting the effectiveness of VCI. Kim et al. (2011) identified a relationship between cognitive and motor impairments and quantitative DTI metrics in individuals with SVCI utilizing the VBA technique. Specifically, they found that cognitive performance was linked to atypical DTI metrics in supratentorial regions, while motor deficits were associated with both supratentorial and infratentorial lesions. Additionally, Zhou et al. (2011) reported that patients with VCI exhibited decreased FA values across the entire brain, indicating more severe white matter damage that correlated with cognitive dysfunction. This was demonstrated through histogram analysis of DTI data and VBA techniques. Another study (Chenet al., 2020) demonstrated that diffusion indices of specific fiber bundles in patients with SVCI were correlated with changes in cognitive function, as determined through AFQ analysis. The DTI-based analysis demonstrates the ability to quantitatively, comprehensively, noninvasively, and objectively assess the integrity of white matter fiber bundles, providing valuable guidance for clinical treatment. However, DTI also has its limitations, its diffusion tensor model cannot effectively measure the white matter properties of fibre crossing regions, while in fact most brain regions contain complex cross-fibre structures. To solve this problem, scholars have proposed methods to improve image acquisition strategies and higher-order models, such as diffusion kurtosis imaging (DKI) and neuronal orientation dispersion and density imaging (NODDI).
DKI sequence offers a more comprehensive assessment of the non-Gaussian diffusion behavior of water molecules compared to DTI sequences, enabling the quantitative evaluation of deviations between actual non-Gaussian diffusion displacements and ideal Gaussian diffusion displacements through kurtosis values. These values serve as indicators of the extent of restriction and heterogeneity in water molecule diffusion (Jensen and Helpern, 2010; Jensen et al., 2005). Liu et al. (2020) observed decreased kurtosis values in the left hippocampus of patient with SVCI, which were inversely associated with cognitive performance scores. Additionally, Fan et al. (2022) observed a decrease in kurtosis values within ROIs across various brain regions in patient with PSCI. These reduced kurtosis values were found to be significantly associated with cognitive scores in the patient population. DKI, with increased sensitivity to intracellular restricted diffusion, enhances the ability to detect subtle microstructural changes in tissues. This heightened sensitivity offers a more reliable method for assessing the integrity of white matter fiber bundles in patients with VCI.
In addition, the NODDI sequence has the advantages of higher specificity, better resolution and multi-parameter assessment compared with the traditional DTI sequence, which can analyse more complex brain tissue microstructures (Zhang et al., 2012). Beck et al. (2021) evaluated the age-dependence of diffusion metrics in the adult life cycle by six different diffusion models, including DTI, DKI and NODDI, and compared the age prediction accuracies of the six different diffusion models, and found that the more advanced diffusion models (e.g., the DKI and NODDI models) were more sensitive to age-related microstructural changes in the brain white matter. Complementing and extending the contribution of traditional DTI models.
Evaluation of blood–brain barrier permeability and changes in glymphatic system function in individuals with vascular cognitive impairment
The blood–brain barrier (BBB) plays a crucial role in controlling the passage of molecules from the bloodstream to the central nervous system, thereby maintaining the appropriate supply of essential nutrients and oxygen, shielding the brain from potentially harmful neurotoxic compounds circulating in the blood, and enhancing the removal of waste products across endothelial cells (Abbott et al., 2010); dysfunction in the PVS results in inadequate fluid flow within the ISF, impairing the elimination of neurotoxins and promoting the accumulation of detrimental substances (Tarasoff-Conway et al., 2015). In recent academic discourse, scholars have introduced the concept of the GS, a comprehensive brain perivascular fluid transport mechanism responsible for eliminating waste products from the ISF by enabling ISF-cerebrospinal fluid (CSF) exchange through aquaporin-4 (AQP-4) water channels located in the vascular end feet of astrocytes (Iliff et al., 2012; Iliff et al., 2013). Consequently, the functioning of the GS is essential for preserving brain fluid equilibrium across the lifespan. Prior research has demonstrated that reduced CBF and oxygen levels in individuals with VCI result in an increase in BBB permeability, the infiltration of plasma proteins into the perivascular spaces, impaired neurovascular coupling, and dysfunctional GS activity (Lee et al., 2017; Parfenov et al., 2019). Numerous studies have indicated that compromised GS function is correlated with various neurological conditions, such as AD (Rasmussen et al., 2018), diabetes (Jiang et al., 2017), traumatic brain injury (Iliff et al., 2014) and stroke (Gaberel et al., 2014), etc. GS dysfunction has been linked to sleep disorders (Xie et al., 2013) as well as to tau (Harrison et al., 2020) and β-amyloid (Aβ) (Eide et al., 2023) deposition, which are pathogenic mechanisms for cognitive impairment and dementia. In addition, the identification of the GS system has opened up avenues for exploring novel therapeutic approaches and targets, as well as for monitoring drug concentrations in central nervous system disorders where the BBB poses a challenge to effective treatment. Multi-modal MRI offers a noninvasive means of quantitatively assessing changes in BBB permeability and GS function in individuals with vascular cognitive impairment.
Assessment of BBB permeability changes
In the contemporary era, MRI brain perfusion weighted imaging (PWI) sequences have garnered significant attention as a focal point of research for evaluating alterations in BBB permeability. PWI can be categorized into two distinct types, one of which necessitates the use of exogenous contrast agent and encompasses dynamic susceptibility contrast MRI (DSC-MRI) and dynamic contrast-enhanced MRI (DCE-MRI). The other category of sequences for PWI is arterial spin-labeling (ASL) based MRI without the usage of exogenous contrast agents, which utilizes hydrogen protons in labeled arterial blood as an endogenous and freely diffusible tracer (Williams et al., 1992; Johnston et al., 2015). Intravoxel incoherent motion imaging (IVIM) can also provide perfusion information without the need for exogenous contrast agents (Federau et al., 2012) (As shown in Figure 2).
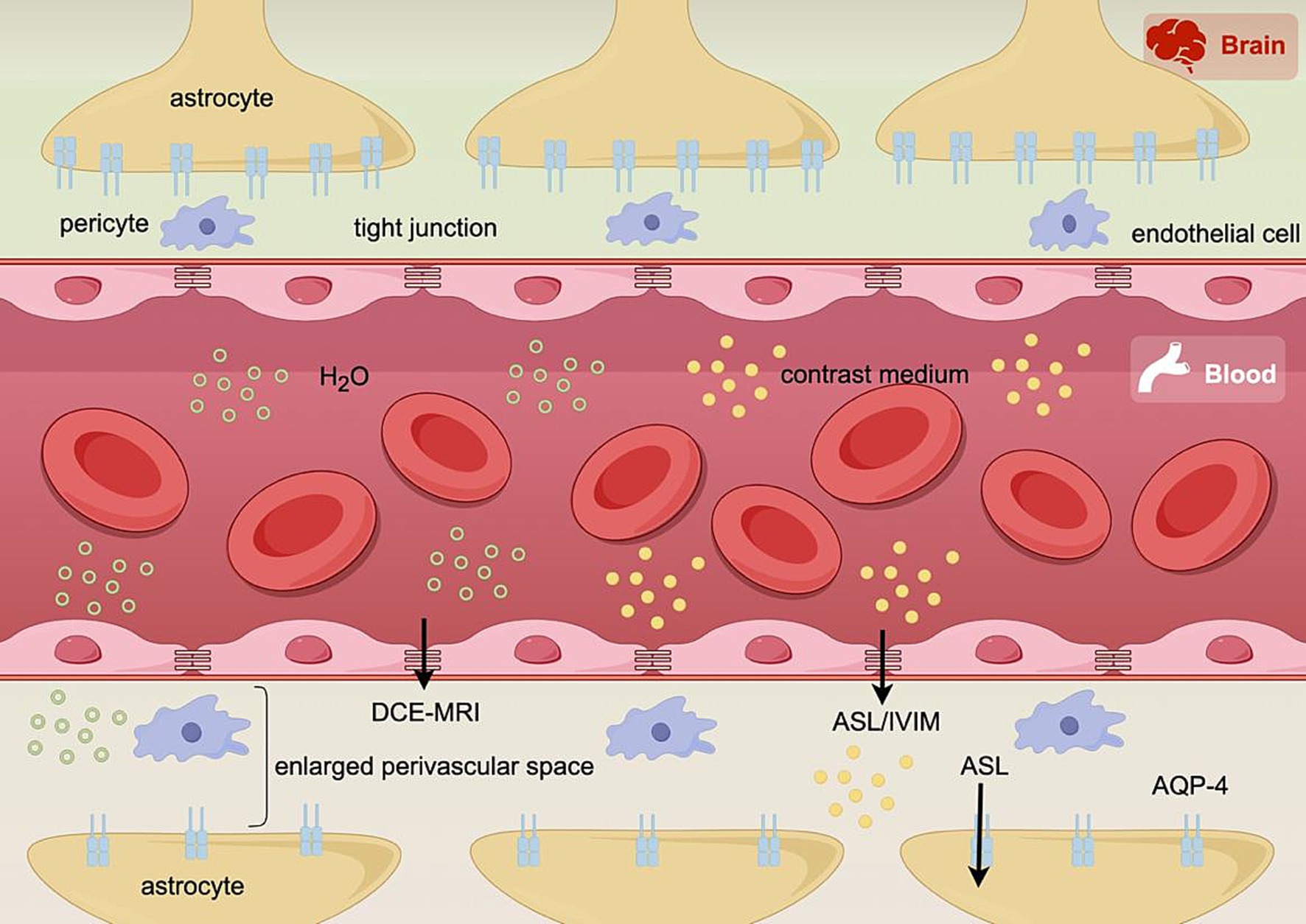
Figure 2. Schematic representation of the blood–brain barrier (BBB) and perivascular space (PVS): when the BBB permeability increases and the PVS widens, the rate of passive transport of contrast agent and water molecules from the intravascular to the extravascular space increases; at the same time, decreased aquaporin-4 (AQP-4) in astrocyte foot segments, which decreases the active transport of water molecules within the PVS and also makes the PVS wider and the GS dysfunction. These pathological changes can be characterized by the quantitative parameters of dynamic contrast-enhanced MRI (DCE-MRI) requiring exogenous contrast agent and arterial spin-labeled MRI (ASL) and intravoxel incoherent motion MRI (IVIM) without exogenous contrast agent, respectively. Images were produced using FigDraw software (www.figdraw.com) (DOI: AYSRP3949a).
DSC-MRI employs the BBB model to acquire semi-quantitative parameters such as cerebral blood flow (CBF), cerebral blood volume (CBV), mean transit time (MTT), and time to peak (TTP) (Kouwenberg et al., 2022; Boxerman et al., 2020); while DCE-MRI is primarily utilized for the assessment of vascular permeability, with key parameters including semi-quantitative parameters such as area under the curve (AUC), TTP, maximal signal intensity, maximal slope, and rate of contouring, etc., and quantitative parameters such as the volume transfer constant (Ktrans), the rate constant (Kep), and the fractional interstitial volume (Ve), etc. (Thrippleton et al., 2019). DCE-MRI is commonly utilized in clinical research as the preferred modality for evaluating changes in BBB permeability (Elschot et al., 2021). Several previous studies (Wang et al., 2023; Kerkhofs et al., 2021a) have confirmed that BBB disruption may be associated with the development of VCI and may precede the appearance of white matter lesions by quantitative parameters of DCE-MRI. A longitudinal study of Kerkhofs et al. (2021b) found that CSVD patients with higher baseline BBB permeability had more severe cognitive decline, further confirming the critical role of BBB disruption in the development of cognitive impairment.
ASL-labeled water molecules cross the BBB and passively diffuse into the brain tissue through tightly connected endothelial cell layers and active transport proteins (e.g., AQP-4 channels on astrocytes). Three modules were commonly used for the water labelling, namely, pulsed ASL (pASL), continuous ASL (CASL) and pseudo-continuous ASL (pCASL) (Fan et al., 2016; Wintermark et al., 2005). ASL-related techniques have the advantages of being non-invasive, contrast-free, and reproducible. ASL quantifies BBB permeability by water molecule transfer rate (Kw) and cerebral perfusion by CBF. Sun et al. (2016) observed a diffuse decrease in CBF in the brain of the SVCI group compared with HC group, which is similar to the findings of Schuff et al. (2009). A longitudinal study of van Dinther et al. (2024) found that lower baseline CBF was associated with cognitive decline in patients with VCI, an association that was most pronounced in attention/psychomotor speed, and that lower temporal and frontal CBF at baseline was associated with more severe memory loss. Neumann et al. (2022) also found that CBF decreases and arterial transit time (ATT) increases as cognitive severity increases in patients with CSVD. However, whether the correlation between CBF and cognitive level in patients with VCI, derived from longitudinal follow-up studies and clinical prediction modeling, is sufficient to indicate a causal relationship remains to be further investigated. In addition, the interference of factors such as cardiovascular disease that may accompany the increase in age makes the choice of post-labeling delay time (PLD) to have some impact on the results of the study. Thus, the commonly used PLD time of 1,800 ms may underestimate CBF in patients with cerebrovascular disease, and the ability to assess the correlation between CBF and the degree of cognitive impairment may be less accurate. Therefore, CBF alterations in VCI patients can be assessed using ASL sequences with multiple PLD times. The ASL sequence uses water molecules as the endogenous contrast agent, which has a much lower molecular weight than the contrast agent of the DCE-MRI sequence and can diffuse more readily into the BBB (Shao et al., 2020). Therefore, theoretically, altered water transport across the BBB should be detected earlier than contrast leakage. Finally, IVIM sequences can obtain perfusion coefficient (D*) value and perfusion fraction (f) value to reflect the microcirculatory perfusion and vascular permeability within the capillaries under low b-value conditions, which can also be used to assess the alteration of BBB permeability in VCI patients (Le Bihan et al., 1988). It can be seen that both types of PWI imaging sequences above can obtain information reflecting perfusion and BBB permeability, which provides an effective tool for studying cerebral underperfusion, cerebrovascular reactivity and BBB permeability in patients with VCI.
Evaluation of alterations in the activity of the glymphatic system
With the development of MRI techniques, the assessment of changes in GS activity can be objectively presented by quantitative MRI techniques, including MRI techniques with or without requirement of tracer injection. Intrathecal tracer injection is generally used to assess GS activity through animal models, which can reflect the GS clearance function over a long period of time, and is not affected by the BBB and blood circulation, but this method is invasive and prone to tracer deposition in the brain (Provenzano et al., 2019); intraventricular tracer injection is susceptible to the BBB and blood circulation, but is safer than intrathecal tracer injection and more commonly used in human beings (Mortensen et al., 2019; Kaur et al., 2020). MRI methods requiring tracer injection are based on the distribution of tracer in tissues and tissue characteristics to establish a pharmacokinetic model to simulate the process of MRI signal changes to achieve the assessment of GS activity, however, they are susceptible to the influence of magnetic field strength, magnetic field uniformity, and scanning parameters. Tracer-less methods, such as the dispersion correlation sequences, can be used for non-invasive assessment of GS activity due to their ability to track the movement of water molecules (Voorter et al., 2024). Besides, IVIM sequences can indirectly reflect fluid mobility within the PVS by changes in D* and f values under low b-value conditions; unfortunately, this method is susceptible to the influence of intercellular ISF (van der Thiel et al., 2021; Wong et al., 2020). Diffusion tensor imaging analysis along the perivascular space (DTI-ALPS) assesses the movement of water molecules in the direction of the PVS as measured by the diffusivity of water molecules measured by DTI sequences (Taoka et al., 2017). The DTI-ALPS analytical method can be used to express the ability of water diffusion along the direction of the PVS by means of the ALPS index, reflecting the GS activity of an individual patient: smaller ALPS index reflect, to some extent, GS impairment. However, this method is only applicable to areas adjacent to the lateral ventricles. Recently, several studies (Ke et al., 2022; Liang et al., 2023; Tian et al., 2023) have found that ALPS index were significantly lower and significantly associated with overall cognitive scores in patients with CSVD by the DTI-ALPS method; furthermore, a DTI-ALPS study (Song et al., 2022) in patients with SVCI showed that structural network efficiency mediated the link between impaired GS function and cognitive dysfunction in patients; Xu et al. (2022) analysis found that patients with cerebral amyloid angiopathy had reduced ALPS index and were associated with lower cognitive scores compared with HC group. In conclusion, GS dysfunction may be a potential pathologic mechanism for vascular cognitive dysfunction, and the ALPS index is expected to be an emerging MRI marker for it. However, the DTI-ALPS method needs to be aligned with magnetically sensitive weighted imaging (SWI). Zhang et al. (2021) performed a modification to obtain a modified ALPS (mALPS) index to reflect GS activity without SWI alignment. These DTI-based analysis methods have the advantages of being noninvasive, tracer-free, and requiring only one scan, but they can only reflect GS transient activity. There are also studies to quantify GS activity by ASL sequence (Ohene et al., 2019) and phase contrast method (Ichikawa et al., 2018). It is believed that with the continuous development of MRI imaging technology, more reliable methods to quantify GS activity can be provided.
MRI-visible perivascular spaces (PVSs) (Bouvy et al., 2014), free water (FW) fraction (Kamagata et al., 2022), and choroid plexus (ChP) volume (Zhang et al., 2021) had also been used to assess the GS function. The PVS is a fluid-filled cavity surrounding small blood vessels in the brain that aids in the exchange of ISF and CSF for waste removal (Brown et al., 2018). Enlarged perivascular spaces (EPVS) are characterized by round or oval smooth-edged structures larger than 2 mm in the perforating artery supply area, exhibiting equal signal intensity to CSF on MRI (Wardlaw et al., 2020). The early detection of PVS morphology is contingent upon the spatial resolution of imaging scans, with ultrahigh-field MRI offering the potential for higher resolution and the ability to detect smaller PVS at an early stage (Barisano et al., 2021). In the clinical assessment of the number of PVS, visual rating scales are usually chosen for this purpose (Rowley, 2013; Laveskog et al., 2018), and the most widely used one is the STRIVE criterion developed by Wardlaw et al. (2013): the quantification of PVS is conducted in the basal ganglia and the centrum semiovale, with classification into mild, moderate, and severe categories based on PVS count. However, the STRIVE criterion is subject to several limitations: (1) challenges in distinguishing between PVS and other cerebrovascular disease markers like lacunar infarcts or white matter lesions; (2) the time-consuming and subjective nature of manual counting, which increases the risk of detection bias; (3) the evaluation of PVS necessitates the utilization of high-resolution MRI images to ensure accuracy, limiting the feasibility of obtaining additional clinically relevant metrics, such as PVS volume; (4) the visual scale method is not suitable for longitudinal studies. In light of the aforementioned challenges, an increasing number of scholarly investigations (Dubost et al., 2019; Moses et al., 2023; Barisano et al., 2022) have been dedicated to the automated quantification of PVS, utilizing techniques such as intensity-based thresholding (Schwartz et al., 2019; Boespflug et al., 2018; Niazi et al., 2018), vascular filtering-based (Ballerini et al., 2018; Sepehrband et al., 2019), and machine learning (Dubost et al., 2019; Lian et al., 2018; Boutinaud et al., 2021). These methods are characterized by their speed, convenience, objectivity, and reproducibility. EPVS, a neuroimaging biomarker observed in individuals with CSVD, is closely linked to cognitive dysfunction. Zdanovskis et al. (2022) found that individuals in the SVCI group exhibited greater expansion of PVS in the centrum semiovale, basal ganglia, and midbrain regions compared to the HC group using visual rating scales. Future research utilizing automated quantification methods is anticipated to further investigate and assess early alterations in PVS number and volume in patients with VCI. ChP constitutes a critical component of the BBB (Damkier et al., 2013) with primary function is the production of cerebrospinal fluid; however, it also plays a pivotal role in mediating the brain’s clearance pathway, thereby contributing to the maintenance of cerebral homeostasis (Rees et al., 2016) and the functioning of the GS (Jessen et al., 2015). Meanwhile, recently, the FW diffusion MRI model has gained particular attention in CSVD (Duering et al., 2018; Li et al., 2024) as well as other brain diseases (Kamagata et al., 2022; Nägele et al., 2024). This model can be divided into FW compartment and tissue compartment, the FW compartment represents water molecules that are not restricted or directed. It is modeled by a tensor that is isotropic and has a fixed diffusion coefficient of water at 37°C. The tissue compartment represents all remaining water molecules, i.e., water molecules within or in close proximity to cellular structures. This includes intracellular water as well as extracellular water affected by physical barriers, such as axon membranes and myelin. Hence, the tissue compartment reflects white matte fiber organization (Duering et al., 2018).
Analysis of altered neurovascular coupling (NVC) function
The neurovascular unit (NVU), which consists of neurons, astrocytes, vascular smooth muscle cells (SMCs), endothelial cells (ECs), and pericytes (Abbott et al., 2006; Tam and Watts, 2010), plays a crucial role in the coupling of vascular and neuronal activity and is of increasing interest in the pathophysiology of VCI. Disruption of any neurovascular coupling (NVC) function component affects CBF regulation and neuronal function (Kisler et al., 2017). Thus, intact NVC function is critical and even minor damage can affect brain function and lead to cognitive decline (Toth et al., 2017). Recently, the analysis of NVC based on ASL and resting-state functional MRI (rs-fMRI) sequences has provided new perspectives for obtaining the functional status of NVC. Ruan et al. (2023) analyzed the associations between white matter lesion (WML) burden, cognitive function and NVC coefficients using mediation methods among the SVCI, PVCI, and HC groups, and found that compared with HC, NVC was significantly lower in the SVCI and PSCI groups at both whole-brain and brain-region levels, and NVC coefficients were also found to be lower in higher-order brain systems responsible for cognitive control and emotion regulation. Mediation analysis suggest that NVC mediates the relationship between WML burden and cognitive impairment. Liu et al. (2021) combined regional homogeneity (ReHo) values obtained by rs-fMRI post-processing with CBF from ASL sequence, using global ReHo-CBF correlation coefficients and ReHo/CBF ratios to detect an intrinsic link between neuronal activity and vascular responses in patients with SVCI, and found that patients in the SVCI group showed significantly lower overall ReHo-CBF coupling, as well as a markedly abnormal ReHo/CBF ratio mainly located in cognitively relevant brain regions and the ReHo/CBF ratio in the left precentral gyrus was positively correlated with the MMSE score. These findings suggest that patients with VCI exhibit abnormal neurovascular coupling early in the disease and during disease progression, which may be related to disease severity and cognitive impairment.
Assessment of brain connectivity
Connectivity analysis based on resting-state functional MRI of the brain
Rs-fMRI sequence is used to detect spontaneous brain activity in the resting state to explore brain function, by detecting spontaneous neuronal activity in the baseline state of the subject’s brain and determining the network connectivity of the relevant brain regions (Raimondo et al., 2021). Currently, the application of rs-fMRI sequence in evaluating brain connectivity in VCI patients is mainly divided into two categories: The first category is the indicators reflecting brain connectivity in the resting state: functional connectivity (FC), functional network connectivity (FNC) and graph theory analysis; and the second category is the connectivity analysis methods reflecting the dynamic change process of the brain: dynamic functional connectivity (dFC), dynamic functional network connectivity (dFNC), graph theory analysis, gradient analysis of brain networks, Granger causality analysis (GCA), multilayered brain networks, and other analysis methods.
Static brain functional connectivity and functional network connectivity analysis
Resting-state brain networks are generally analyzed by FC, FNC and graph theory analysis, in which FC and FNC are obtained by calculating the degree of correlation between two time series, positive correlation represents the existence of positive connections and vice versa, whereas the graph theory approach is based on FC, FNC for topological analysis.
The study of FC can be categorized into the voxel level and the ROI level. All of these FC analysis methods are based on linear models, while some studies have found that BOLD signals have nonlinear characteristics, therefore, FC analysis methods based on nonlinear models to obtain parameters such as maximum information coefficient (MIC), extended maximum information coefficient (EMIC), and so on, may be more suitable for the use of rs-fMRI to capture the complex interactions between brain regions. Chen et al. (2019) found that patients with SVCI had reduced FC between default mode network (DMN)-related brain regions and reduced negative connectivity with other network brain regions by the seed-based correlation analysis (SCA) method, at the same time, patients with SVCI were found to have impaired memory function associated with increased FC between DMN-related brain regions in the medial frontal lobe, whereas the patients’ impaired information processing was associated with reduced FC between DMN-related brain regions in the thalamus. Recently, other studies (Zuo et al., 2019; Sun et al., 2011) have found different trends of FC changes in different brain regions in patients with VCI, possibly due to subcortical ischemia disrupting directly and indirectly connected fiber bundles, which triggers weakened connectivity in some brain regions, whereas enhanced connectivity in some brain regions may be caused by compensatory compensation for cognitive impairment and neuroplasticity mechanisms in functional brain regions.
FNC analysis employs a strategy that combines model-driven and data-driven approaches (Jafri et al., 2008). First, grouped independent component analysis (ICA) is performed on rs-fMRI data from multiple subjects to derive subject-specific functional networks called resting-state networks (RSNs), commonly known as DMN, central executive network (CEN), salience network (SN), frontoparietal network (FPN), sensorimotor network (SMN), visual network (VN) and auditory network (AN) etc. (Mueller et al., 2014). Finally, the connectivity between any RSNs can then be obtained by calculating the connectivity metric, resulting in a connectivity matrix that includes the strength of connectivity between all networks. Wei et al. (2024) found different trends of changes in different RSNs interconnection models were found, along with different correlations between different RSNs interconnection models and MoCA scores, suggesting that there is a correlation between FNC changes and cognitive deficits in SVCI patients. Chen H. et al. (2023) also found increased FNC change between the VN and cerebellar domains in patients with SVCI, however, there was a reduction in FNC change between the cognitive control network and the cerebellar domains and a significant reduction in FNC within the sensorimotor domain. These studies also illustrate that the FNC approach can provide a more detailed delineation of brain regions reflecting the temporal connectivity between different brain regions by using a higher model order. In contrast to ROI-based approaches, FNC analysis employs a data-driven approach to generate functionally co-activated brain regions, whereas brain regions in ROI-based approaches are typically determined through a priori knowledge (Du et al., 2018).
In functional brain network analysis, relevant attributes such as local efficiency (Eloc), global efficiency (Eg), clustering coefficient (Cp), standardized clustering coefficients (γ), small-world attributes, characteristic path length (Lp), etc., can be obtained from graph theory analysis to evaluate the topological properties of brain networks (Farahani et al., 2019). Graph theory analysis helps to describe and quantify the topology of the brain, and can identify key nodes that are important and functional in the brain network. Several studies (Sang et al., 2018; Wang et al., 2019) have found that with the increase of cognitive impairment in VCI patients, the pattern of brain functional connectivity network is disrupted and the graph theory related properties are changed by rs-fMRI graph theory analysis method, which also indicates that the graph theory based brain functional network analysis method can provide a potential imaging biomarker for the early detection of cognitive impairments in VCI patients, however, most of the studies are descriptive and focus on pairwise interactions of binary compositional graphs, as the technology develops, the generative model, dynamic network, and algebraic topology will be the several promising directions for the future development.
Dynamic functional connectivity and functional network connectivity analysis
In recent years, more scholars believe that the brain’s information transfer is time-dynamic and condition-dependent (Chang and Glover, 2010), so purely relying on static analysis methods can not accurately describe the dynamic changes in the brain, and dynamic analysis methods can provide new perspectives on the dynamic functional activities of the brain: including dFC, dFNC, and the dynamic graph theory analysis, in addition to the analysis of the gradient analysis of the brain network, GCA, multi-layer brain network and other analytical methods.
dFC and dFNC are based on FC, ICA (Kiviniemi et al., 2011) and independent vector analysis (IVA) (Calhoun et al., 2014), using sliding time window (Madhyastha and Grabowski, 2014; Maes et al., 2022), joint time-frequency analysis (Yaesoubi et al., 2015) and other methods to transform the RSN into dynamic brain network, and then through K-means clustering and Gaussian mixture model clustering analysis to obtain multiple states, and finally to calculate the fractional occupancy of each state, the mean dwell time, the lifetime switching rate, and the transition probability matrix to realize the dynamic analysis of brain function. Currently, dFC analysis methods are mainly divided into two paths, one is to capture synchronous changes in paired regions, such as sliding window analysis and time-frequency analysis; the other is to identify synchronous change patterns at the multivariate level, such as co-activation (CAP), hidden Markov model (HMM), and multilayer brain network. Recently, fractional occupancy and mean lifetime of brain states in patients with SVCI have been found to be related to cognitive performance, as evidenced by higher fractional occupancy and longer mean lifetime in weakly-connected states and greater difficulty in switching from weakly-connected to more strongly-connected states (Sang et al., 2023). Xu et al. (2021) used dFC analysis method to categorize the brain states into seven different states, and observed that cognitive deficits in patients of state 3 with SVCI predominantly reduced the mean dwell time, with a strong overall positive correlation. Yin et al. (2022) analyzed the relationship between the temporal characteristics of dFC and cognitive impairment in patients with SVCI, and they classified the dFC status into sparse connectivity, strong connectivity, and intermediate patterns by K-means clustering analysis, and found that SVCI patients showed a higher fractional occupancy and longer mean dwell time in sparse connectivity, and a lower fractional occupancy and shorter dwell time in strong connectivity, which was similar to the finding of Chen F. et al. (2023). Meanwhile, this finding suggests that altered temporal properties of dFC in patients with SVCI may be a sensitive neuroimaging biomarker for early disease recognition. In addition to the above construction of dFC by the sliding window method, Schlemm et al. (2022) constructed dFC based on the co-activation pattern method, obtained DMN activation state, DMN inhibition state, VN activation state, VN inhibition state, and FPN state of the patients with SVCI by clustering analysis, and found that cognitive impairments were associated with the convergence of the mean dwell time of each state and the decrease of gradient between each pattern. Consistent sets of brain states and spatial alterations in functional connectivity that correlate with different states of consciousness or awareness can be identified by dFC, which provides additional options for analyzing brain connectivity in patients with VCI.
By integrating the aforementioned methods for analyzing dFC and dFNC, it has been observed that while the introduction of windows facilitates a dynamic assessment of brain connectivity, the selection of window length significantly influences the outcomes. Specifically, shorter window lengths tend to stabilize the relationship between the time series of two nodes within the window, thereby potentially increasing the calculated correlation. This phenomenon explains why dFC often yields more significant results, as static FC is analogous to using a window length equivalent to the entire scanning duration. However, it is important to note that correlations derived from excessively short window lengths may not accurately reflect genuine brain activity (Leonardi and Van De Ville, 2015). Furthermore, the optimal window length for assessing dFC between various nodes may vary. Zhang et al. (2018) proposed that a shorter window length is appropriate for analyzing intra-network connectivity, while a longer window length is preferable for examining inter-network connectivity. This distinction may arise from the influence of window length on the frequency of functional connectivity that can be detected. Specifically, a shorter window length is more conducive to observing connectivity associated with high-frequency signals, whereas a longer window length is more suitable for detecting connectivity related to low-frequency signals. Consequently, it is crucial to develop dynamic brain function analysis methods that mitigate the influence of window length. The CAP model addresses this by assessing the activation patterns of the entire brain at each sampling point, thereby describing the brain’s dynamic function in terms of instantaneous network states, which reflect the brain’s dynamic function at the smallest time scale (Liu and Duyn, 2013; Liu et al., 2018); the HMM model effectively solves the problem of the time window configuration by characterizing the brain activity as a sequence of different states deduced from the resting data problem (Zhang G. et al., 2020; Vidaurre et al., 2017); and the multilayer brain network analysis method can estimate a neighbourhood matrix for each time window, interconnecting all windows into a multilayer network, with each time window represented as a layer. This method constructs a hyper-adjacency matrix that captures both intra- and inter-layer connections, thereby offering a comprehensive perspective on dynamic interactions (Pedersen et al., 2018).
Properties analysis of DTI brain networks based on graph theory
Graph theory-based DTI brain network property analysis is based on brain partition mapping for deterministic or probabilistic fiber tracking between multiple brain regions two by two, using specific brain regions as nodes and structural connectivity indicators (fiber bundle bar count, probability, average FA value, etc.) between nodes as edges, which can construct structural brain networks and calculate small-world properties, Rich-club coefficients, degree centrality, etc., and utilize the graph theory to calculate the corresponding network properties to analyze brain structural connectivity (Rubinov and Sporns, 2010; Sporns, 2018). Kim et al. (2015) assessed the cognitive status of SVCI patients based on DTI graph theory analysis, and observed that an increase in white matter high-density volume or number of lumens was significantly associated with a decrease in network integration and an increase in network separation, and that changes in these network attributes were associated with poor performance of attention, language, visuospatial, memory, and frontal executive function performance was associated with poor performance. Tuladhar et al. (2017) also observed reduced structural network density, network strength, and efficiency in SVCI patients, and that the reduced connectivity was mainly manifested in the connectivity between rich-club nodes, with lower rich-club connectivity associated with lower psychomotor speed and executive function scores. Graph theory-based analysis of the structural network properties of the DTI brain can be used as a complement to the graph theory analysis of FC, and has some clinical applications in the assessment of patients with VCI.
Assessment of metabolic changes
Traditional proton-based magnetic resonance spectroscopy (1H-MRS) is capable of identifying metabolites such as choline (Cho), N-acetylaspartate (NAA), and creatine (Cr) within brain tissues in vivo, and has been extensively utilized for evaluating metabolic changes in the brains of individuals with VCI ((Barry Erhardt et al., 2019; Chen et al., 2016; Ross et al., 2005; Lin et al., 2021). Nonetheless, the complexity of the background, overlapping spectra, and the presence of pressurized water/lipids inherent to the 1H-MRS technique restrict its utility as a molecular imaging tool, hindering the ability to capture dynamic molecular metabolic processes. The emergence of multinuclear MRI, facilitated by advancements in MRS technology, enables the acquisition of diverse metabolite information within the human body, thereby augmenting the precision of detecting both physiological and pathological processes. This technology maintains spatial resolution while offering the unique capability of noninvasive, in vivo quantitative analysis of dynamic molecular metabolic processes, including the accurate quantification of energy metabolic pathways such as anaerobic glycolysis and the tricarboxylic acid cycle, and at the same time, can detect tissue osmotic pressure, neurotransmitters and mitochondrial function (Sun et al., 2023). Multinuclear MRI offers a distinct advantage in visualizing real-time dynamic metabolic processes across various pathological conditions. This technology is anticipated to offer novel opportunities for early disease detection, treatment efficacy evaluation, therapeutic decision-making, and drug development. Furthermore, it has the potential to facilitate the exploration of novel pathogenic mechanisms and is poised to become a valuable tool for advancing molecular biology, biochemistry, and metabolism research in human subjects.
Amide proton transfer weighted imaging (APTw) is an MRI technique utilizing chemical exchange saturation transfer (CEST) to assess protein properties at the cellular molecular level within tissues. The APTw sequence offers non-invasive, safe, and quantitative analysis, with changes in APT signal primarily influenced by the exchange rate between amide protons and water molecules. This exchange rate is closely linked to the concentration of proteins and peptides in the body, as well as the pH value (Milot, 2022; Zhou and Van, 2006).; APTw imaging has been extensively utilized in various areas of neuroscience research, including brain development (Zhang Z. et al., 2020; Zhang et al., 2016), cerebral vascular diseases (Sun et al., 2011; Yu et al., 2019), brain tumors (Joo et al., 2019; Guo et al., 2022), and neurodegenerative diseases (Chen X. et al., 2023; Guo et al., 2021), etc. Additionally, investigations have been conducted to determine if APT values could serve as a potential indicator of VCI. Mu et al. (2023) has demonstrated statistically significant differences in APT values of specific brain regions, such as the frontal white matter, hippocampus, amygdala, and thalamus, suggesting their potential utility as markers for VCI.
Sodium-23 MRS (23Na-MRS) is the sole noninvasive method capable of providing precise spatial quantification of sodium concentration in living tissue through imaging technology. This technique provides direct biochemical insights into cellular integrity and tissue viability, with minimal observable macroscopic alterations. Consequently, it can be utilized to monitor temporal changes in tissue viability during treatment and potentially serve as a biomarker for early preventative diagnosis in clinical settings (Madelin et al., 2014; Chen et al., 2022). 23Na-MRS has been used in neurological diseases such as AD, Huntington’s disease (HD) and multiple sclerosis (MS) (Reetz et al., 2012; Mellon et al., 2009). These investigations highlight the promise of 23Na-MRS for the timely detection and tracking of disease advancement and treatment efficacy in various neurological disorders, as indicated by the observation of heightened tissue sodium concentration (TSC) in the brain. Phosphorus-31 MRS (31P-MRS) enables noninvasive monitoring of cell membrane proliferation, mitochondrial processes involved in energy metabolism (Rzanny et al., 2003), tissue acid–base balance, tissue magnesium ion concentration, and specific nucleotides (Larkin et al., 2021). Zuo et al. (2015) identified variances in phosphocreatine (PCr) and phosphoric acid (Pi) levels through 31P-MRS assessments between individuals diagnosed with depression and a HC group, while Dudley et al. (2015) noted a notable decrease in PCr and adenosine triphosphate (ATP) levels in the gray matter of individuals with bipolar disorder (BD) using 31P-MRS. These findings underscore the utility of 31P-MRS in measuring mitochondrial metabolic irregularities. Meanwhile, 31P-MRS has shown promise as a valuable tool for assessing treatment response by serving as a biomarker for phospholipid metabolism. Specifically, the phospholipid monolipid (PME)/phospholipid diolipid (PDE) ratio in individuals with AD demonstrated an increase following a 4-week course of Souvenaid treatment (Rijpma et al., 2017). On the other hand, 31P-MRS has been utilized in research examining pH fluctuations in individuals post-traumatic brain injury (TBI) (Stovell et al., 2020). Hyperpolarized-13C-MRS enables the in vivo, non-invasive quantitative evaluation of key biochemical processes such as the tricarboxylic acid cycle and glucose metabolism in the human body (Mandal et al., 2022). Research utilizing hyperpolarized-13C-MRS has shown increased pyruvate-to-lactate conversion in longitudinal studies of MS mouse models (Guglielmetti et al., 2017b) and in acute and subacute phases of TBI mouse models (Guglielmetti et al., 2017a; DeVience et al., 2021). These findings offer valuable insights for assessing the effectiveness of anti-inflammatory treatments and managing patient care. The investigation into the utility of multinuclear MRI for evaluating changes in localized metabolic substances in the brains of patients with VCI is currently in its early stages. It is anticipated that advancements in magnetic resonance hardware systems, fast sequence methods, and accelerated algorithms such as compressed sensing and deep learning will contribute to the maturation of multi-nuclear MRI/MRS as a valuable tool for assessing metabolic alterations in the entire brain or specific regions of interest in patients with VCI, in the meantime, further research is needed to investigate the potential benefits of incorporating joint analysis with other functional MRI sequences and brain network analysis.
Summary and outlook
VCI, as a group of diseases mainly caused by cerebrovascular diseases, undergoes a progression from mVCI to VaD throughout the course of the disease, with structural, functional, metabolic, and cerebral connectivity changes occurring in different brain regions throughout the process, and with the development of neuroimaging, the comprehensive assessment and understanding of these changes has been greatly facilitated. However, we have found that the variation in results across studies may be related to a variety of factors such as patient selection and sample size, MRI field strength, scanning protocols or reproducibility on different scanning devices, and feasibility of implementation, in addition to the fact that patients may be at different stages of the disease, and a standardised protocol is therefore needed to increase the reproducibility of relevant studies, as in the case of the HARNESS protocol which standardises the effects of vascular factors on dementia and neurodegeneration effects on brain imaging protocols, contributing to increased study reproducibility. With the development of ultra-high field strength MRI equipment, which relies on its high resolution, good contrast between tissues and better signal-to-noise ratio to facilitate the detection of subtle lesions as well as finer blood vessels, it is becoming a new tool for CSVD studies. However, these new techniques still need to be validated to define their operational specifications before they can be widely disseminated. In addition, the application and development of multimodal MRI, neural networks, machine learning, and deep learning in image processing can further increase diagnostic accuracy, enabling the extraction of more imaging information from data that would otherwise not be easily accessible to provide additional support for diagnosis.
In addition, most of the neuroimaging studies on VCI are cross-sectional studies, and there is a lack of longitudinal studies to validate the results, so the longitudinal development and changes in the structure, function, metabolism, and connectivity of patients with VCI should be explored more deeply in future studies. Finally, with the exploratory development of transcriptional connectome association studies, it provides an important opportunity to bridge the gap between microscopic transcriptome features and macroscopic brain networks. However, the underlying molecular mechanisms of altered brain function associated with VCI patients remain unclear and await further exploration in future studies.
Author contributions
CM: Formal analysis, Resources, Supervision, Visualization, Writing – original draft. AL: Formal analysis, Supervision, Writing – review & editing. JiaL: Supervision, Writing – review & editing. XW: Formal analysis, Supervision, Writing – review & editing. FC: Formal analysis, Supervision, Visualization, Writing – review & editing. YL: Formal analysis, Supervision, Writing – review & editing. JinL: Formal analysis, Supervision, Visualization, Writing – review & editing.
Funding
The author(s) declare that financial support was received for the research and/or publication of this article. This study was supported by the Fourth Batch of Science and Technology Programme Targeted Projects in Liaoning Province in 2021 (No.2021JH2/10300135), Dalian Science and Technology Talent Innovation Support Project (No. 2023RY034).
Conflict of interest
The authors declare that the research was conducted in the absence of any commercial or financial relationships that could be construed as a potential conflict of interest.
Generative AI statement
The author(s) declare that no Gen AI was used in the creation of this manuscript.
Publisher’s note
All claims expressed in this article are solely those of the authors and do not necessarily represent those of their affiliated organizations, or those of the publisher, the editors and the reviewers. Any product that may be evaluated in this article, or claim that may be made by its manufacturer, is not guaranteed or endorsed by the publisher.
References
Abbott, N. J., Patabendige, A. A. K., Dolman, D. E. M., Yusof, S. R., and Begley, D. J. (2010). Structure and function of the blood-brain barrier. Neurobiol. Dis. 37, 13–25. doi: 10.1016/j.nbd.2009.07.030
Abbott, N. J., Rönnbäck, L., and Hansson, E. (2006). Astrocyte-endothelial interactions at the blood-brain barrier. Nat. Rev. Neurosci. 7, 41–53. doi: 10.1038/nrn1824
Bálint, A. R., Puskás, T., Menyhárt, Á., Kozák, G., Szenti, I., Kónya, Z., et al. (2019). Aging impairs cerebrovascular reactivity at preserved resting cerebral arteriolar tone and vascular density in the laboratory rat. Front. Aging Neurosci. 11:301. doi: 10.3389/fnagi.2019.00301
Ballerini, L., Lovreglio, R., Valdés Hernández, M. D. C., Ramirez, J., MacIntosh, B. J., Black, S. E., et al. (2018). Perivascular spaces segmentation in brain MRI using optimal 3D filtering. Sci. Rep. 8:2132. doi: 10.1038/s41598-018-19781-5
Barisano, G., Law, M., Custer, R. M., Toga, A. W., and Sepehrband, F. (2021). Perivascular space imaging at ultrahigh field MR imaging. Magn. Reson. Imaging Clin. N. Am. 29, 67–75. doi: 10.1016/j.mric.2020.09.005
Barisano, G., Lynch, K. M., Sibilia, F., Lan, H., Shih, N.-C., Sepehrband, F., et al. (2022). Imaging perivascular space structure and function using brain MRI. NeuroImage 257:119329. doi: 10.1016/j.neuroimage.2022.119329
Barry Erhardt, E., Pesko, J. C., Prestopnik, J., Thompson, J., Caprihan, A., and Rosenberg, G. A. (2019). Biomarkers identify the Binswanger type of vascular cognitive impairment. J. Cereb. Blood Flow Metab. 39, 1602–1612.
Beck, D., de Lange, A.-M. G., Maximov, I. I., Richard, G., Andreassen, O. A., Nordvik, J. E., et al. (2021). White matter microstructure across the adult lifespan: a mixed longitudinal and cross-sectional study using advanced diffusion models and brain-age prediction. NeuroImage 224:117441. doi: 10.1016/j.neuroimage.2020.117441
Boespflug, E. L., Schwartz, D. L., Lahna, D., Pollock, J., Iliff, J. J., Kaye, J. A., et al. (2018). MR imaging-based multimodal autoidentification of perivascular spaces (mMAPS): automated morphologic segmentation of enlarged perivascular spaces at clinical field strength. Radiology 286, 632–642. doi: 10.1148/radiol.2017170205
Boutinaud, P., Tsuchida, A., Laurent, A., Adonias, F., Hanifehlou, Z., Nozais, V., et al. (2021). 3D segmentation of perivascular spaces on T1-weighted 3 tesla MR images with a convolutional autoencoder and a U-shaped neural network. Front. Neuroinform. 15:641600. doi: 10.3389/fninf.2021.641600
Bouvy, W. H., Biessels, G. J., Kuijf, H. J., Kappelle, L. J., Luijten, P. R., and Zwanenburg, J. J. M. (2014). Visualization of perivascular spaces and perforating arteries with 7 T magnetic resonance imaging. Investig. Radiol. 49, 307–313. doi: 10.1097/RLI.0000000000000027
Boxerman, J. L., Quarles, C. C., Hu, L. S., Erickson, B. J., Gerstner, E. R., and Smits, M. (2020). Consensus recommendations for a dynamic susceptibility contrast MRI protocol for use in high-grade gliomas. Neuro-Oncology 22, 1262–1275. doi: 10.1093/neuonc/noaa141
Brown, R., Benveniste, H., Black, S. E., Charpak, S., Dichgans, M., Joutel, A., et al. (2018). Understanding the role of the perivascular space in cerebral small vessel disease. Cardiovasc. Res. 114, 1462–1473. doi: 10.1093/cvr/cvy113
Calhoun, V. D., Miller, R., Pearlson, G., and Adalı, T. (2014). The chronnectome: time-varying connectivity networks as the next frontier in fMRI data discovery. Neuron 84, 262–274. doi: 10.1016/j.neuron.2014.10.015
Chang, C., and Glover, G. H. (2010). Time-frequency dynamics of resting-state brain connectivity measured with fMRI. NeuroImage 50, 81–98. doi: 10.1016/j.neuroimage.2009.12.011
Chen, S. -Q., Cai, Q., Shen, Y. -Y., Xu, C. -X., Zhou, H., and Zhao, Z. (2016). Hydrogen proton magnetic resonance spectroscopy in multidomain amnestic mild cognitive impairment and vascular cognitive impairment without dementia. Am. J. Alzheimers Dis. Other Demen. 31, 422–429.
Chen, F., Chen, Q., Zhu, Y., Long, C., Lu, J., Jiang, Y., et al. (2023). Alterations in dynamic functional connectivity in patients with cerebral small vessel disease. Transl. Stroke Res. 15, 580–590. doi: 10.1007/s12975-023-01148-2
Chen, H.-J., Gao, Y.-Q., Che, C.-H., Lin, H., and Ruan, X.-L. (2018). Diffusion tensor imaging with tract-based spatial statistics reveals white matter abnormalities in patients with vascular cognitive impairment. Front. Neuroanat. 12:53. doi: 10.3389/fnana.2018.00053
Chen, X., Gong, T., Chen, T., Xu, C., Li, Y., Song, Q., et al. (2023). Altered glutamate-glutamine and amide proton transfer-weighted values in the hippocampus of patients with amnestic mild cognitive impairment: a novel combined imaging diagnostic marker. Front. Neurosci. 17:1089300. doi: 10.3389/fnins.2023.1089300
Chen, X., Huang, L., Ye, Q., Yang, D., Qin, R., Luo, C., et al. (2019). Disrupted functional and structural connectivity within default mode network contribute to WMH-related cognitive impairment. Neuroimage Clin. 24:102088. doi: 10.1016/j.nicl.2019.102088
Chen, Q., Shah, N. J., and Worthoff, W. A. (2022). Compressed sensing in sodium magnetic resonance imaging: techniques, applications, and future prospects. J. Magn. Reson. Imaging 55, 1340–1356. doi: 10.1002/jmri.28029
Chen, H., Xu, J., Lv, W., Hu, Z., Ke, Z., Qin, R., et al. (2023). Altered static and dynamic functional network connectivity related to cognitive decline in individuals with white matter hyperintensities. Behav. Brain Res. 451:114506. doi: 10.1016/j.bbr.2023.114506
Corriveau, R. A., Bosetti, F., Emr, M., Gladman, J. T., Koenig, J. I., Moy, C. S., et al. (2016). The science of vascular contributions to cognitive impairment and dementia (VCID): a framework for advancing research priorities in the cerebrovascular biology of cognitive decline. Cell. Mol. Neurobiol. 36, 281–288. doi: 10.1007/s10571-016-0334-7
Damkier, H. H., Brown, P. D., and Praetorius, J. (2013). Cerebrospinal fluid secretion by the choroid plexus. Physiol. Rev. 93, 1847–1892. doi: 10.1152/physrev.00004.2013
DeVience, S. J., Lu, X., Proctor, J. L., Rangghran, P., Medina, J. A., Melhem, E. R., et al. (2021). Enhancing metabolic imaging of energy metabolism in traumatic brain injury using hyperpolarized [1-13C]pyruvate and Dichloroacetate. Meta 11:335. doi: 10.3390/metabo11060335
Dichgans, M., and Leys, D. (2017). Vascular Cognitive Impairment. Circ. Res. 120, 573–591. doi: 10.1161/CIRCRESAHA.116.308426
Du, Y., Fu, Z., and Calhoun, V. D. (2018). Classification and prediction of brain disorders using functional connectivity: promising but challenging. Front. Neurosci. 12:525. doi: 10.3389/fnins.2018.00525
Du, B., Li, H., Zheng, H., Fan, C., Liang, M., Lian, Y., et al. (2019). Minocycline ameliorates depressive-like behavior and demyelination induced by transient global cerebral ischemia by inhibiting microglial activation. Front. Pharmacol. 10:1247. doi: 10.3389/fphar.2019.01247
Dubost, F., Yilmaz, P., Adams, H., Bortsova, G., Ikram, M. A., Niessen, W., et al. (2019). Enlarged perivascular spaces in brain MRI: automated quantification in four regions. NeuroImage 185, 534–544. doi: 10.1016/j.neuroimage.2018.10.026
Dudley, J. A., Lee, J.-H., Durling, M., Strakowski, S. M., and Eliassen, J. C. (2015). Age-dependent decreases of high energy phosphates in cerebral gray matter of patients with bipolar I disorder: a preliminary phosphorus-31 magnetic resonance spectroscopic imaging study. J. Affect. Disord. 175, 251–255. doi: 10.1016/j.jad.2015.01.026
Duering, M., Finsterwalder, S., Baykara, E., Tuladhar, A. M., Gesierich, B., Konieczny, M. J., et al. (2018). Free water determines diffusion alterations and clinical status in cerebral small vessel disease. Alzheimers Dement. 14, 764–774. doi: 10.1016/j.jalz.2017.12.007
Eide, Per Kristian, Lashkarivand, Aslan, Pripp, Are, Valnes, Lars Magnus, Hovd, Markus Herberg, Ringstad, Geir, et al. (2023). Plasma neurodegeneration biomarker concentrations associate with glymphatic and meningeal lymphatic measures in neurological disorders. Nat. Commun..14, 2084. doi: 10.1038/s41467-023-37685-5
Elschot, E. P., Backes, W. H., Postma, A. A., van Oostenbrugge, R. J., Staals, J., Rouhl, R. P. W., et al. (2021). A comprehensive view on MRI techniques for imaging blood-brain barrier integrity. Investig. Radiol. 56, 10–19. doi: 10.1097/RLI.0000000000000723
Fan, L., Ibrahim, F. E. E. M., Chu, X., Fu, Y., Yan, H., Wu, Z., et al. (2022). Altered microstructural changes detected by diffusion kurtosis imaging in patients with cognitive impairment after acute cerebral infarction. Front. Neurol. 13:802357. doi: 10.3389/fneur.2022.802357
Fan, A. P., Jahanian, H., Holdsworth, S. J., and Zaharchuk, G. (2016). Comparison of cerebral blood flow measurement with [15O]-water positron emission tomography and arterial spin labeling magnetic resonance imaging: a systematic review. J. Cereb. Blood Flow Metab. 36, 842–861. doi: 10.1177/0271678X16636393
Farahani, F. V., Karwowski, W., and Lighthall, N. R. (2019). Application of graph theory for identifying connectivity patterns in human brain networks: a systematic review. Front. Neurosci. 13:585. doi: 10.3389/fnins.2019.00585
Federau, C., Maeder, P., O’Brien, K., Browaeys, P., Meuli, R., and Hagmann, P. (2012). Quantitative measurement of brain perfusion with intravoxel incoherent motion MR imaging. Radiology. 265, 874–881. doi: 10.1148/radiol.12120584
Gaberel, T., Gakuba, C., Goulay, R., Martinez De Lizarrondo, S., Hanouz, J.-L., Emery, E., et al. (2014). Impaired glymphatic perfusion after strokes revealed by contrast-enhanced MRI: a new target for fibrinolysis? Stroke 45, 3092–3096. doi: 10.1161/STROKEAHA.114.006617
Guglielmetti, C., Chou, A., Krukowski, K., Najac, C., Feng, X., Riparip, L.-K., et al. (2017a). In vivo metabolic imaging of traumatic brain injury. Sci. Rep. 7:17525. doi: 10.1038/s41598-017-17758-4
Guglielmetti, C., Najac, C., Didonna, A., Van der Linden, A., Ronen, S. M., and Chaumeil, M. M. (2017b). Hyperpolarized 13C MR metabolic imaging can detect neuroinflammation in vivo in a multiple sclerosis murine model. Proc. Natl. Acad. Sci. USA 114, E6982–E6991. doi: 10.1073/pnas.1613345114
Guo, Z., Jiang, Y., Qin, X., Mu, R., Meng, Z., Zhuang, Z., et al. (2021). Amide proton transfer-weighted MRI might help distinguish amnestic mild cognitive impairment from a Normal elderly population. Front. Neurol. 12:707030. doi: 10.3389/fneur.2021.707030
Guo, H., Liu, J., Hu, J., Zhang, H., Zhao, W., Gao, M., et al. (2022). Diagnostic performance of gliomas grading and IDH status decoding a comparison between 3D amide proton transfer APT and four diffusion-weighted MRI models. J. Magn. Reson. Imaging 56, 1834–1844. doi: 10.1002/jmri.28211
Harrison, I. F., Ismail, O., Machhada, A., Colgan, N., Ohene, Y., Nahavandi, P., et al. (2020). Impaired glymphatic function and clearance of tau in an Alzheimer’s disease model. Brain 143, 2576–2593. doi: 10.1093/brain/awaa179
Hosoki, S., Hansra, G. K., Jayasena, T., Poljak, A., Mather, K. A., Catts, V. S., et al. (2023). Molecular biomarkers for vascular cognitive impairment and dementia. Nat. Rev. Neurol. 19, 737–753. doi: 10.1038/s41582-023-00884-1
Iadecola, C. (2013). The pathobiology of vascular dementia. Neuron 80, 844–866. doi: 10.1016/j.neuron.2013.10.008
Iadecola, C., Duering, M., Hachinski, V., Joutel, A., Pendlebury, S. T., Schneider, J. A., et al. (2019). Vascular cognitive impairment and dementia: JACC scientific expert panel. J. Am. Coll. Cardiol. 73, 3326–3344. doi: 10.1016/j.jacc.2019.04.034
Ichikawa, S., Motosugi, U., Okumura, A., Shimizu, T., and Onishi, H. (2018). Measurement of cerebrospinal fluid flow dynamics using phase contrast MR imaging with bilateral jugular vein compression: a feasibility study in healthy volunteers. Magn. Reson. Med. Sci. 17, 265–268. doi: 10.2463/mrms.tn.2017-0056
Iliff, J. J., Chen, M. J., Plog, B. A., Zeppenfeld, D. M., Soltero, M., Yang, L., et al. (2014). Impairment of glymphatic pathway function promotes tau pathology after traumatic brain injury. J. Neurosci. 34, 16180–16193. doi: 10.1523/JNEUROSCI.3020-14.2014
Iliff, J. J., Lee, H., Yu, M., Feng, T., Logan, J., Nedergaard, M., et al. (2013). Brain-wide pathway for waste clearance captured by contrast-enhanced MRI. J. Clin. Invest. 123, 1299–1309. doi: 10.1172/JCI67677
Iliff, J. J., Wang, M., Liao, Y., Plogg, B. A., Peng, W., Gundersen, G. A., et al. (2012). A paravascular pathway facilitates CSF flow through the brain parenchyma and the clearance of interstitial solutes, including amyloid β. Sci. Transl. Med. 4:147ra111. doi: 10.1126/scitranslmed.3003748
Jafri, M. J., Pearlson, G. D., Stevens, M., and Calhoun, V. D. (2008). A method for functional network connectivity among spatially independent resting-state components in schizophrenia. NeuroImage 39, 1666–1681. doi: 10.1016/j.neuroimage.2007.11.001
Jensen, J. H., and Helpern, J. A. (2010). MRI Quantification of Non-Gaussian Water Diffusion by Kurtosis Analysis. NMR Biomed. 23, 698–710. doi: 10.1002/nbm.1518
Jensen, J. H., Helpern, J. A., Ramani, A., Lu, H., and Kaczynski, K. (2005). Diffusional kurtosis imaging: the quantification of non-gaussian water diffusion by means of magnetic resonance imaging. Magn. Reson. Med. 53, 1432–1440. doi: 10.1002/mrm.20508
Jessen, N. A., Munk, A. S. F., Lundgaard, I., and Nedergaard, M. (2015). The Glymphatic system – a Beginner’s guide. Neurochem. Res. 40, 2583–2599. doi: 10.1007/s11064-015-1581-6
Jiang, Q., Zhang, L., Ding, G., Davoodi-Bojd, E., Li, Q., Li, L., et al. (2017). Impairment of the glymphatic system after diabetes. J. Cereb. Blood Flow Metab. 37, 1326–1337. doi: 10.1177/0271678X16654702
Johnston, M. E., Lu, K., Maldjian, J. A., and Jung, Y. (2015). Multi-TI arterial spin labeling MRI with variable TR and bolus duration for cerebral blood flow and arterial transit time mapping. IEEE Trans. Med. Imaging 34, 1392–1402. doi: 10.1109/TMI.2015.2395257
Joo, B., Han, K., Ahn, S. S., Choi, Y. S., Chang, J. H., Kang, S.-G., et al. (2019). Amide proton transfer imaging might predict survival and IDH mutation status in high-grade glioma. Eur. Radiol. 29, 6643–6652. doi: 10.1007/s00330-019-06203-x
Kamagata, K., Andica, C., Takabayashi, K., Saito, Y., Taoka, T., Nozaki, H., et al. (2022). Association of MRI indices of Glymphatic system with amyloid deposition and cognition in mild cognitive impairment and Alzheimer disease. Neurology 99:e2648. doi: 10.1212/WNL.0000000000201300
Kaur, J., Davoodi-Bojd, E., Fahmy, L. M., Zhang, L., Ding, G., Hu, J., et al. (2020). Magnetic resonance imaging and modeling of the Glymphatic system. Diagnostics (Basel). 10:344. doi: 10.3390/diagnostics10060344
Ke, Z., Mo, Y., Li, J., Yang, D., Huang, L., Yang, Z., et al. (2022). Glymphatic dysfunction mediates the influence of white matter hyperintensities on episodic memory in cerebral small vessel disease. Brain Sci. 12:1611. doi: 10.3390/brainsci12121611
Kerkhofs, D., Wong, S. M., Zhang, E., Staals, J., Jansen, J. F. A., van Oostenbrugge, R. J., et al. (2021a). Baseline blood-brain barrier leakage and longitudinal microstructural tissue damage in the periphery of white matter hyperintensities. Neurol. Int. 96, e2192–e2200. doi: 10.1212/WNL.0000000000011783
Kerkhofs, D., Wong, S. M., Zhang, E., Uiterwijk, R., Hoff, E. I., Jansen, J. F. A., et al. (2021b). Blood-brain barrier leakage at baseline and cognitive decline in cerebral small vessel disease: a 2-year follow-up study. Geroscience 43, 1643–1652. doi: 10.1007/s11357-021-00399-x
Kim, H. J., Im, K., Kwon, H., Lee, J. M., Ye, B. S., Kim, Y. J., et al. (2015). Effects of amyloid and small vessel disease on white matter network disruption. J. Alzheimers Dis. 44, 963–975. doi: 10.3233/JAD-141623
Kim, S. H., Park, J. S., Ahn, H.-J., Seo, S. W., Lee, J.-M., Kim, S. T., et al. (2011). Voxel-based analysis of diffusion tensor imaging in patients with subcortical vascular cognitive impairment: correlates with cognitive and motor deficits. J. Neuroimaging 21, 317–324. doi: 10.1111/j.1552-6569.2010.00527.x
Kim, G. H., Seo, S. W., Jung, K., Kwon, O.-H., Kwon, H., Kim, J. H., et al. (2016). The neural correlates of motor intentional disorders in patients with subcortical vascular cognitive impairment. J. Neurol. 263, 89–99. doi: 10.1007/s00415-015-7946-6
Kisler, K., Nelson, A. R., Montagne, A., and Zlokovic, B. V. (2017). Cerebral blood flow regulation and neurovascular dysfunction in Alzheimer disease. Nat. Rev. Neurosci. 18, 419–434. doi: 10.1038/nrn.2017.48
Kiviniemi, V., Vire, T., Remes, J., Elseoud, A. A., Starck, T., Tervonen, O., et al. (2011). A sliding time-window ICA reveals spatial variability of the default mode network in time. Brain Connect. 1, 339–347. doi: 10.1089/brain.2011.0036
Kouwenberg, V., van Santwijk, L., Meijer, F. J. A., and Henssen, D. (2022). Reliability of dynamic susceptibility contrast perfusion metrics in pre- and post-treatment glioma. Cancer Imaging 22:28. doi: 10.1186/s40644-022-00466-2
Larkin, J. R., Foo, L. S., Sutherland, B. A., Khrapitchev, A., and Tee, Y. K. (2021). Magnetic resonance pH imaging in stroke - combining the old with the new. Front. Physiol. 12:793741. doi: 10.3389/fphys.2021.793741
Laveskog, A., Wang, R., Bronge, L., Wahlund, L.-O., and Qiu, C. (2018). Perivascular spaces in old age: assessment, distribution, and correlation with white matter hyperintensities. AJNR Am. J. Neuroradiol. 39, 70–76. doi: 10.3174/ajnr.A5455
Le Bihan, D., Breton, E., Lallemand, D., Aubin, M. L., Vignaud, J., and Laval-Jeantet, M. (1988). Separation of diffusion and perfusion in intravoxel incoherent motion MR imaging. Radiology 168, 497–505. doi: 10.1148/radiology.168.2.3393671
Le Bihan, D., Mangin, J. F., Poupon, C., Clark, C. A., Pappata, S., Molko, N., et al. (2001). Diffusion tensor imaging: concepts and applications. J. Magn. Reson. Imaging 13, 534–546. doi: 10.1002/jmri.1076
Lee, J.-M., Park, J.-M., Song, M. K., Oh, Y. J., Kim, C.-J., and Kim, Y.-J. (2017). The ameliorative effects of exercise on cognitive impairment and white matter injury from blood-brain barrier disruption induced by chronic cerebral hypoperfusion in adolescent rats. Neurosci. Lett. 638, 83–89. doi: 10.1016/j.neulet.2016.12.018
Leonardi, N., and Van De Ville, D. (2015). On spurious and real fluctuations of dynamic functional connectivity during rest. NeuroImage 104, 430–436. doi: 10.1016/j.neuroimage.2014.09.007
Li, H., Jacob, M. A., Cai, M., Kessels, R. P. C., Norris, D. G., Duering, M., et al. (2024). Perivascular spaces, diffusivity along perivascular spaces, and free water in cerebral small vessel disease. Neurology 102:e209306. doi: 10.1212/WNL.0000000000209306
Lian, C., Zhang, J., Liu, M., Zong, X., Hung, S.-C., Lin, W., et al. (2018). Multi-channel multi-scale fully convolutional network for 3D perivascular spaces segmentation in 7T MR images. Med. Image Anal. 46, 106–117. doi: 10.1016/j.media.2018.02.009
Liang, T., Chang, F., Huang, Z., Peng, D., Zhou, X., and Liu, W. (2023). Evaluation of glymphatic system activity by diffusion tensor image analysis along the perivascular space (DTI-ALPS) in dementia patients. Br. J. Radiol. 96:20220315. doi: 10.1259/bjr.20220315
Liesz, A. (2019). The vascular side of Alzheimer’s disease. Science 365, 223–224. doi: 10.1126/science.aay2720
Lin, H., Jin, T., Chen, L., Dai, Y., Jia, W., He, X., et al. (2021). Longitudinal tracing of neurochemical metabolic disorders in working memory neural circuit and optogenetics modulation in rats with vascular cognitive impairment. Brain Res. Bull. 170, 174–186.
Liu, Y., Chan, D. K. Y., Thalamuthu, A., Wen, W., Jiang, J., Paradise, M., et al. (2020). Plasma lipidomic biomarker analysis reveals distinct lipid changes in vascular dementia. Comput. Struct. Biotechnol. J. 18, 1613–1624. doi: 10.1016/j.csbj.2020.06.001
Liu, X., Cheng, R., Chen, L., Gong, J., Luo, T., and Lv, F. (2021). Altered neurovascular coupling in subcortical ischemic vascular disease. Front. Aging Neurosci. 13:598365. doi: 10.3389/fnagi.2021.598365
Liu, X., Cheng, R., Chen, L., Luo, T., Lv, F., Gong, J., et al. (2019b). Alterations of white matter integrity in subcortical ischemic vascular disease with and without cognitive impairment: a TBSS study. J. Mol. Neurosci. 67, 595–603. doi: 10.1007/s12031-019-01266-3
Liu, X., and Duyn, J. H. (2013). Time-varying functional network information extracted from brief instances of spontaneous brain activity. Proc. Natl. Acad. Sci. USA 110, 4392–4397. doi: 10.1073/pnas.1216856110
Liu, D., Li, K., Ma, X., Li, Y., Bu, Q., Pan, Z., et al. (2020). Correlations between the microstructural changes of the medial temporal cortex and mild cognitive impairment in patients with cerebral small vascular disease (cSVD): a diffusion kurtosis imaging study. Front. Neurol. 10:1378. doi: 10.3389/fneur.2019.01378
Liu, X., Zhang, N., Chang, C., and Duyn, J. H. (2018). Co-activation patterns in resting-state fMRI signals. NeuroImage 180, 485–494. doi: 10.1016/j.neuroimage.2018.01.041
Liu, Y., Zhang, Y., Zheng, X., Fang, T., Yang, X., Luo, X., et al. (2018). Galantamine improves cognition, hippocampal inflammation, and synaptic plasticity impairments induced by lipopolysaccharide in mice. J. Neuroinflammation 15:112. doi: 10.1186/s12974-018-1141-5
Ma, H. R., Sheng, L. Q., Pan, P. L., Wang, G. D., Luo, R., Shi, H. C., et al. (2018). Cerebral glucose metabolic prediction from amnestic mild cognitive impairment to Alzheimer’s dementia: a meta-analysis. Transl. Neurodegener. 7:9. doi: 10.1186/s40035-018-0114-z
Madelin, G., Lee, J.-S., Regatte, R. R., and Jerschow, A. (2014). Sodium MRI: methods and applications. Prog. Nucl. Magn. Reson. Spectrosc. 79, 14–47. doi: 10.1016/j.pnmrs.2014.02.001
Madhyastha, T. M., and Grabowski, T. J. (2014). Age-related differences in the dynamic architecture of intrinsic networks. Brain Connect. 4, 231–241. doi: 10.1089/brain.2013.0205
Maes, C., Cuypers, K., Peeters, R., Sunaert, S., Edden, R. A. E., Gooijers, J., et al. (2022). Task-related modulation of sensorimotor GABA+ levels in association with brain activity and motor performance: a multimodal MRS-fMRI study in young and older adults. J. Neurosci. 42, 1119–1130. doi: 10.1523/JNEUROSCI.1154-21.2021
Mandal, P. K., Guha Roy, R., Samkaria, A., Maroon, J. C., and Arora, Y. (2022). In vivo 13C magnetic resonance spectroscopy for assessing brain biochemistry in health and disease. Neurochem. Res. 47, 1183–1201. doi: 10.1007/s11064-022-03538-8
Mascalchi, M., Salvadori, E., Toschi, N., Giannelli, M., Orsolini, S., Ciulli, S., et al. (2019). DTI-derived indexes of brain WM correlate with cognitive performance in vascular MCI and small-vessel disease. A TBSS study. Brain Imaging Behav. 13, 594–602. doi: 10.1007/s11682-018-9873-5
McWilliam, A., Palma, G., Abravan, A., Acosta, O., Appelt, A., Aznar, M., et al. (2023). Voxel-based analysis: roadmap for clinical translation. Radiother. Oncol. 188:109868. doi: 10.1016/j.radonc.2023.109868
Mellon, E. A., Pilkinton, D. T., Clark, C. M., Elliott, M. A., Witschey, W. R., Borthakur, A., et al. (2009). Sodium MR imaging detection of mild Alzheimer disease: preliminary study. AJNR Am. J. Neuroradiol. 30, 978–984. doi: 10.3174/ajnr.A1495
Milot, L. (2022). Amide proton transfer-weighted MRI: insight into Cancer cell biology. Radiology 305, 135–136. doi: 10.1148/radiol.221376
Mortensen, K. N., Sanggaard, S., Mestre, H., Lee, H., Kostrikov, S., Xavier, A. L. R., et al. (2019). Impaired Glymphatic transport in spontaneously hypertensive rats. J. Neurosci. 39, 6365–6377. doi: 10.1523/JNEUROSCI.1974-18.2019
Moses, J., Sinclair, B., Law, M., O’Brien, T. J., and Vivash, L. (2023). Automated methods for detecting and quantitation of enlarged perivascular spaces on MRI. J. Magn. Reson. Imaging 57, 11–24. doi: 10.1002/jmri.28369
Mu, R., Qin, X., Zheng, W., Yang, P., Huang, B., Li, X., et al. (2023). Amide proton transfer could be a surrogate imaging marker for predicting vascular cognitive impairment. Brain Res. Bull. 204:110793. doi: 10.1016/j.brainresbull.2023.110793
Mueller, S., Costa, A., Keeser, D., Pogarell, O., Berman, A., Coates, U., et al. (2014). The effects of methylphenidate on whole brain intrinsic functional connectivity. Hum. Brain Mapp. 35, 5379–5388. doi: 10.1002/hbm.22557
Nägele, F. L., Petersen, M., Mayer, C., Bönstrup, M., Schulz, R., Gerloff, C., et al. (2024). Longitudinal microstructural alterations surrounding subcortical ischemic stroke lesions detected by free-water imaging. Hum. Brain Mapp. 45:e26722. doi: 10.1002/hbm.26722
Neumann, K., Günther, M., Düzel, E., and Schreiber, S. (2022). Microvascular impairment in patients with cerebral small vessel disease assessed with arterial spin labeling magnetic resonance imaging: a pilot study. Front. Aging Neurosci. 14:871612. doi: 10.3389/fnagi.2022.871612
Niazi, M., Karaman, M., Das, S., Zhou, X. J., Yushkevich, P., and Cai, K. (2018). Quantitative MRI of perivascular spaces at 3T for early diagnosis of mild cognitive impairment. AJNR Am. J. Neuroradiol. 39, 1622–1628. doi: 10.3174/ajnr.A5734
Ohene, Y., Harrison, I. F., Nahavandi, P., Ismail, O., Bird, E. V., Ottersen, O. P., et al. (2019). Non-invasive MRI of brain clearance pathways using multiple echo time arterial spin labelling: an aquaporin-4 study. NeuroImage 188, 515–523. doi: 10.1016/j.neuroimage.2018.12.026
Parfenov, V. A., Ostroumova, O. D., Ostroumova, T. M., Kochetkov, A. I., Fateeva, V. V., Khacheva, K. K., et al. (2019). Vascular cognitive impairment: pathophysiological mechanisms, insights into structural basis, and perspectives in specific treatments. Neuropsychiatr. Dis. Treat. 15, 1381–1402. doi: 10.2147/NDT.S197032
Pedersen, M., Zalesky, A., Omidvarnia, A., and Jackson, G. D. (2018). Multilayer network switching rate predicts brain performance. Proc. Natl. Acad. Sci. USA 115, 13376–13381. doi: 10.1073/pnas.1814785115
Pierpaoli, C., and Basser, P. J. (1996). Toward a quantitative assessment of diffusion anisotropy. Magn. Reson. Med. 36, 893–906. doi: 10.1002/mrm.1910360612
Provenzano, D. A., Pellis, Z., and DeRiggi, L. (2019). Fatal gadolinium-induced encephalopathy following accidental intrathecal administration: a case report and a comprehensive evidence-based review. Reg. Anesth. Pain Med. 44, 721–729. doi: 10.1136/rapm-2019-100422
Raimondo, L., Oliveiraĺcaro, A. F., Heij, J., Priovoulos, N., Kundu, P., Leoni, R. F., et al. (2021). Advances in resting state fMRI acquisitions for functional connectomics. NeuroImage 243:118503. doi: 10.1016/j.neuroimage.2021.118503
Rasmussen, M. K., Mestre, H., and Nedergaard, M. (2018). The glymphatic pathway in neurological disorders. Lancet Neurol. 17, 1016–1024. doi: 10.1016/S1474-4422(18)30318-1
Rees, H. D., Dunn, A. J., and Iuvone, P. M. (2016). The choroid plexus-cerebrospinal fluid interface in Alzheimer’s disease: more than just a barrier. Neural Regen. Res. 11:534. doi: 10.4103/1673-5374.180372
Reetz, K., Romanzetti, S., Dogan, I., Saß, C., Werner, C. J., Schiefer, J., et al. (2012). Increased brain tissue sodium concentration in Huntington’s disease - a sodium imaging study at 4 T. NeuroImage 63, 517–524. doi: 10.1016/j.neuroimage.2012.07.009
Rheault, F., De Benedictis, A., Daducci, A., et al. (2020). Tractostorm: The what, why, and how of tractography dissection reproducibility. Hum. Brain. Mapp. 41, 1859–1874. doi: 10.1002/hbm.24917
Rijpma, A., van der Graaf, M., Lansbergen, M. M., Meulenbroek, O., Cetinyurek-Yavuz, A., Sijben, J. W., et al. (2017). The medical food Souvenaid affects brain phospholipid metabolism in mild Alzheimer’s disease: results from a randomized controlled trial. Alzheimers Res. Ther. 9:51. doi: 10.1186/s13195-017-0286-2
Rosenberg, G. A., Wallin, A., Wardlaw, J. M., Markus, H. S., Montaner, J., Wolfson, L., et al. (2016). Consensus statement for diagnosis of subcortical small vessel disease. J. Cereb. Blood Flow Metab. 36, 6–25. doi: 10.1038/jcbfm.2015.172
Ross, A. J., Sachdev, P. S., Wen, W., Valenzuela, M. J., and Brodaty, H. (2005). 1H MRS in stroke patients with and without cognitive impairment [published correction appears in Neurobiol Aging. 2006 Mar;27(3):513]. Neurobiol. Aging. 26, 873–882. doi: 10.1016/j.neurobiolaging.2004.07.008
Rowley, H. A. (2013). The alphabet of imaging in acute stroke: does it spell improved selection and outcome? Stroke 44, S53–S54. doi: 10.1161/STROKEAHA.113.001939
Ruan, Z., Sun, D., Zhou, X., Yu, M., Li, S., Sun, W., et al. (2023). Altered neurovascular coupling in patients with vascular cognitive impairment: a combined ASL-fMRI analysis. Front. Aging Neurosci. 15:1224525. doi: 10.3389/fnagi.2023.1224525
Rubinov, M., and Sporns, O. (2010). Complex network measures of brain connectivity: uses and interpretations. NeuroImage 52, 1059–1069. doi: 10.1016/j.neuroimage.2009.10.003
Rzanny, R., Klemm, S., Reichenbach, J. R., Pfleiderer, S. O. R., Schmidt, B., Volz, H.-P., et al. (2003). 31P-MR spectroscopy in children and adolescents with a familial risk of schizophrenia. Eur. Radiol. 13, 763–770. doi: 10.1007/s00330-002-1565-1
Sang, L., Chen, L., Wang, L., Zhang, J., Zhang, Y., Li, P., et al. (2018). Progressively disrupted brain functional connectivity network in subcortical ischemic vascular cognitive impairment patients. Front. Neurol. 9:94. doi: 10.3389/fneur.2018.00094
Sang, L., Wang, L., Zhang, J., Qiao, L., Li, P., Zhang, Y., et al. (2023). Progressive alteration of dynamic functional connectivity patterns in subcortical ischemic vascular cognitive impairment patients. Neurobiol. Aging 122, 45–54. doi: 10.1016/j.neurobiolaging.2022.11.009
Schlemm, E., Frey, B. M., Mayer, C., Petersen, M., Fiehler, J., Hanning, U., et al. (2022). Equalization of brain state occupancy accompanies cognitive impairment in cerebral small vessel disease. Biol. Psychiatry 92, 592–602. doi: 10.1016/j.biopsych.2022.03.019
Schuff, N., Matsumoto, S., Kmiecik, J., Studholme, C., Du, A., Ezekiel, F., et al. (2009). Cerebral blood flow in ischemic vascular dementia and Alzheimer’s disease, measured by arterial spin-labeling magnetic resonance imaging. Alzheimers Dement. 5, 454–462. doi: 10.1016/j.jalz.2009.04.1233
Schwartz, D. L., Boespflug, E. L., Lahna, D. L., Pollock, J., Roese, N. E., and Silbert, L. C. (2019). Autoidentification of perivascular spaces in white matter using clinical field strength T1 and FLAIR MR imaging. NeuroImage 202:116126. doi: 10.1016/j.neuroimage.2019.116126
Sepehrband, F., Barisano, G., Sheikh-Bahaei, N., Cabeen, R. P., Choupan, J., Law, M., et al. (2019). Image processing approaches to enhance perivascular space visibility and quantification using MRI. Sci. Rep. 9:12351. doi: 10.1038/s41598-019-48910-x
Shao, X., Jann, K., Ma, S. J., Yan, L., Montagne, A., Ringman, J. M., et al. (2020). Comparison between blood-brain barrier water exchange rate and permeability to gadolinium-based contrast agent in an elderly cohort. Front. Neurosci. 14:571480. doi: 10.3389/fnins.2020.571480
Smith, S. M., Johansen-Berg, H., Jenkinson, M., Rueckert, D., Nichols, T. E., Miller, K. L., et al. (2007). Acquisition and voxelwise analysis of multi-subject diffusion data with tract-based spatial statistics. Nat. Protoc. 2, 499–503. doi: 10.1038/nprot.2007.45
Song, H., Ruan, Z., Gao, L., Lv, D., Sun, D., Li, Z., et al. (2022). Structural network efficiency mediates the association between glymphatic function and cognition in mild VCI: a DTI-ALPS study. Front. Aging Neurosci. 14:974114. doi: 10.3389/fnagi.2022.974114
Sporns, O. (2018). Graph theory methods: applications in brain networks. Dialogues Clin. Neurosci. 20, 111–121. doi: 10.31887/DCNS.2018.20.2/osporns
Stovell, M. G., Mada, M. O., Carpenter, T. A., Yan, J.-L., Guilfoyle, M. R., Jalloh, I., et al. (2020). Phosphorus spectroscopy in acute TBI demonstrates metabolic changes that relate to outcome in the presence of normal structural MRI. J. Cereb. Blood Flow Metab. 40, 67–84. doi: 10.1177/0271678X18799176
Sun, Y., Cao, W., Ding, W., Wang, Y., Han, X., Zhou, Y., et al. (2016). Cerebral blood flow alterations as assessed by 3D ASL in cognitive impairment in patients with subcortical vascular cognitive impairment: a marker for disease severity. Front. Aging Neurosci. 8:211. doi: 10.3389/fnagi.2016.00211
Sun, P. Z., Cheung, J. S., Wang, E., and Lo, E. H. (2011). Association between pH-weighted endogenous amide proton chemical exchange saturation transfer MRI and tissue lactic acidosis during acute ischemic stroke. J. Cereb. Blood Flow Metab. 31, 1743–1750. doi: 10.1038/jcbfm.2011.23
Sun, Y., Qin, L., Zhou, Y., Xu, Q., Qian, L., Tao, J., et al. (2011). Abnormal functional connectivity in patients with vascular cognitive impairment, no dementia: a resting-state functional magnetic resonance imaging study. Behav. Brain Res. 223, 388–394. doi: 10.1016/j.bbr.2011.05.006
Sun, P., Wu, Z., Lin, L., Hu, G., Zhang, X., and Wang, J. (2023). MR-Nucleomics: the study of pathological cellular processes with multinuclear magnetic resonance spectroscopy and imaging in vivo. NMR Biomed. 36:e4845. doi: 10.1002/nbm.4845
Tam, S. J., and Watts, R. J. (2010). Connecting vascular and nervous system development: angiogenesis and the blood-brain barrier. Annu. Rev. Neurosci. 33, 379–408. doi: 10.1146/annurev-neuro-060909-152829
Taoka, T., Masutani, Y., Kawai, H., Nakane, T., Matsuoka, K., Yasuno, F., et al. (2017). Evaluation of glymphatic system activity with the diffusion MR technique: diffusion tensor image analysis along the perivascular space (DTI-ALPS) in Alzheimer’s disease cases. Jpn. J. Radiol. 35, 172–178. doi: 10.1007/s11604-017-0617-z
Tarasoff-Conway, J. M., Carare, R. O., Osorio, R. S., Glodzik, L., Butler, T., Fieremans, E., et al. (2015). Clearance systems in the brain-implications for Alzheimer disease. Nat. Rev. Neurol. 11, 457–470. doi: 10.1038/nrneurol.2015.119
Thrippleton, M. J., Backes, W. H., Sourbron, S., Ingrisch, M., van Osch, M. J. P., Dichgans, M., et al. (2019). Quantifying blood-brain barrier leakage in small vessel disease: review and consensus recommendations. Alzheimers Dement. 15, 840–858. doi: 10.1016/j.jalz.2019.01.013
Tian, Y., Cai, X., Zhou, Y., Jin, A., Wang, S., Yang, Y., et al. (2023). Impaired glymphatic system as evidenced by low diffusivity along perivascular spaces is associated with cerebral small vessel disease: a population-based study. Stroke Vasc. Neurol. 8, 413–423. doi: 10.1136/svn-2022-002191
Toth, P., Tarantini, S., Csiszar, A., and Ungvari, Z. (2017). Functional vascular contributions to cognitive impairment and dementia: mechanisms and consequences of cerebral autoregulatory dysfunction, endothelial impairment, and neurovascular uncoupling in aging. Am. J. Physiol. Heart Circ. Physiol. 312, H1–H20. doi: 10.1152/ajpheart.00581.2016
Tuladhar, A. M., Lawrence, A., Norris, D. G., Barrick, T. R., Markus, H. S., and de Leeuw, F. E. (2017). Disruption of rich club organisation in cerebral small vessel disease. Hum. Brain Mapp. 38, 1751–1766. doi: 10.1002/hbm.23479
van der Flier, W. M., Skoog, I., Schneider, J. A., Pantoni, L., Mok, V., Chen, C. L. H., et al. (2018). Vascular cognitive impairment. Nat. Rev. Dis. Primers 4:18003. doi: 10.1038/nrdp.2018.3
van der Thiel, M. M., Freeze, W. M., Verheggen, I. C. M., Wong, S. M., de Jong, J. J. A., Postma, A. A., et al. (2021). Associations of increased interstitial fluid with vascular and neurodegenerative abnormalities in a memory clinic sample. Neurobiol. Aging 106, 257–267. doi: 10.1016/j.neurobiolaging.2021.06.017
van Dinther, M., Hooghiemstra, A. M., Bron, E. E., Versteeg, A., Leeuwis, A. E., Kalay, T., et al. (2024). Lower cerebral blood flow predicts cognitive decline in patients with vascular cognitive impairment. Alzheimers Dement. 20, 136–144. doi: 10.1002/alz.13408
Vidaurre, D., Smith, S. M., and Woolrich, M. W. (2017). Brain network dynamics are hierarchically organized in time. Proc. Natl. Acad. Sci. USA. 114, 12827–12832. doi: 10.1073/pnas.1705120114
Voorter, P. H. M., van Dinther, M., Jansen, W. J., Postma, A. A., Staals, J., Jansen, J. F. A., et al. (2024). Blood–brain barrier disruption and perivascular spaces in small vessel disease and neurodegenerative diseases: a review on MRI methods and insights, Blood–brain barrier disruption and perivascular spaces in small vessel disease and neurodegenerative diseases: a review onMRIMethods and insights. J. Magn. Reson. Imaging 59, 397–411. doi: 10.1002/jmri.28989
Wang, J., Chen, Y., Liang, H., Niedermayer, G., Chen, H., Li, Y., et al. (2019). The role of disturbed small-world networks in patients with white matter lesions and cognitive impairment revealed by resting state function magnetic resonance images (rs-fMRI). Med. Sci. Monit. 25, 341–356. doi: 10.12659/MSM.913396
Wang, X., Shi, Y., Chen, Y., Gao, Y., Wang, T., Li, Z., et al. (2023). Blood-brain barrier breakdown is a sensitive biomarker of cognitive and language impairment in patients with white matter Hyperintensities. Neurol. Ther. 12, 1745–1758. doi: 10.1007/s40120-023-00527-z
Wardlaw, J. M., Benveniste, H., Nedergaard, M., et al. (2020). Perivascular spaces in the brain: anatomy, physiology and pathology. Nat. Rev. Neurol. 16, 137–153. doi: 10.1038/s41582-020-0312-z
Wardlaw, J. M., Smith, E. E., Biessels, G. J., Cordonnier, C., Fazekas, F., Frayne, R., et al. (2013). Neuroimaging standards for research into small vessel disease and its contribution to ageing and neurodegeneration. Lancet Neurol. 12, 822–838. doi: 10.1016/S1474-4422(13)70124-8
Wei, H.-L., Wei, C., Yu, Y.-S., Yu, X., Chen, Y., Li, J., et al. (2024). Dysfunction of the triple-network model is associated with cognitive impairment in patients with cerebral small vessel disease. Heliyon 10:e24701. doi: 10.1016/j.heliyon.2024.e24701
Williams, D. S., Detre, J. A., Leigh, J. S., and Koretsky, A. P. (1992). Magnetic resonance imaging of perfusion using spin inversion of arterial water. Proc. Natl. Acad. Sci. USA 89, 212–216. doi: 10.1073/pnas.89.1.212
Wintermark, M., Sesay, M., Barbier, E., Borbély, K., Dillon, W. P., and Eastwood, J. D. (2005). Comparative overview of brain perfusion imaging techniques. Stroke 36, e83–e99. doi: 10.1161/01.STR.0000177884.72657.8b
Wong, S. M., Backes, W. H., Drenthen, G. S., Zhang, C. E., Voorter, P. H. M., Staals, J., et al. (2020). Spectral diffusion analysis of Intravoxel incoherent motion MRI in cerebral small vessel disease. J. Magn. Reson. Imaging 51, 1170–1180. doi: 10.1002/jmri.26920
Xie, L., Kang, H., Xu, Q., Chen, M. J., Liao, Y., Thiyagarajan, M., et al. (2013). Sleep drives metabolite clearance from the adult brain. Science 342, 373–377. doi: 10.1126/science.1241224
Xu, Y., Shang, H., Lu, H., Zhang, J., Yao, L., and Long, Z. (2021). Altered dynamic functional connectivity in subcortical ischemic vascular disease with cognitive impairment. Front. Aging Neurosci. 13:758137. doi: 10.3389/fnagi.2021.758137
Xu, J., Su, Y., Fu, J., Wang, X., Nguchu, B. A., Qiu, B., et al. (2022). Glymphatic dysfunction correlates with severity of small vessel disease and cognitive impairment in cerebral amyloid angiopathy. Eur. J. Neurol. 29, 2895–2904. doi: 10.1111/ene.15450
Yaesoubi, M., Allen, E. A., Miller, R. L., and Calhoun, V. D. (2015). Dynamic coherence analysis of resting fMRI data to jointly capture state-based phase, frequency, and time-domain information. NeuroImage 120, 133–142. doi: 10.1016/j.neuroimage.2015.07.002
Yeatman, J. D., Dougherty, R. F., Myall, N. J., Wandell, B. A., and Feldman, H. M. (2012). Tract profiles of white matter properties: automating fiber-tract quantification. PLoS One 7:e49790. doi: 10.1371/journal.pone.0049790
Yin, W., Zhou, X., Li, C., You, M., Wan, K., Zhang, W., et al. (2022). The clustering analysis of time properties in patients with cerebral small vessel disease: a dynamic connectivity study. Front. Neurol. 13:913241. doi: 10.3389/fneur.2022.913241
Yu, L., Chen, Y., Chen, M., Luo, X., Jiang, S., Zhang, Y., et al. (2019). Amide proton transfer MRI signal as a surrogate biomarker of ischemic stroke recovery in patients with supportive treatment. Front. Neurol. 10:104. doi: 10.3389/fneur.2019.00104
Zdanovskis, N., Platkājis, A., Kostiks, A., Šneidere, K., Stepens, A., Naglis, R., et al. (2022). Combined score of perivascular space dilatation and white matter Hyperintensities in patients with Normal cognition, mild cognitive impairment, and dementia. Medicina (Kaunas) 58:887. doi: 10.3390/medicina58070887
Zhang, C., Baum, S. A., Adduru, V. R., Biswal, B. B., and Michael, A. M. (2018). Test-retest reliability of dynamic functional connectivity in resting state fMRI. NeuroImage 183, 907–918. doi: 10.1016/j.neuroimage.2018.08.021
Zhang, G., Cai, B., Zhang, A., Stephen, J. M., Wilson, T. W., Calhoun, V. D., et al. (2020). Estimating dynamic functional brain connectivity with a sparse hidden Markov model. IEEE Trans. Med. Imaging 39, 488–498. doi: 10.1109/TMI.2019.2929959
Zhang, H., Kang, H., Zhao, X., Jiang, S., Zhang, Y., Zhou, J., et al. (2016). Amide proton transfer (APT) MR imaging and magnetization transfer (MT) MR imaging of pediatric brain development. Eur. Radiol. 26, 3368–3376. doi: 10.1007/s00330-015-4188-z
Zhang, H., Schneider, T., Wheeler-Kingshott, C. A., and Alexander, D. C. (2012). NODDI: practical in vivo neurite orientation dispersion and density imaging of the human brain. Neuroimage. 61, 1000–1016. doi: 10.1016/j.neuroimage.2012.03.072
Zhang, X., Su, J., Gao, C., Ni, W., Gao, X., Li, Y., et al. (2019). Progression in vascular cognitive impairment: pathogenesis, neuroimaging evaluation, and treatment. Cell Transplant. 28, 18–25. doi: 10.1177/0963689718815820
Zhang, Z., Zhang, C., Yao, J., Gao, F., Gong, T., Jiang, S., et al. (2020). Amide proton transfer-weighted magnetic resonance imaging of human brain aging at 3 tesla. Quant. Imaging Med. Surg. 10, 727–742. doi: 10.21037/qims.2020.02.22
Zhang, W., Zhou, Y., Wang, J., Gong, X., Chen, Z., Zhang, X., et al. (2021). Glymphatic clearance function in patients with cerebral small vessel disease. NeuroImage 238:118257. doi: 10.1016/j.neuroimage.2021.118257
Zhou, Y., Null, Q.-X., Qin, L., Qian, L., Cao, W., and Xu, J. (2011). A primary study of diffusion tensor imaging-based histogram analysis in vascular cognitive impairment with no dementia. Clin. Neurol. Neurosurg. 113, 92–97. doi: 10.1016/j.clineuro.2010.09.007
Zhou, J., and Van, Z. P. C. M. (2006). Chemical exchange saturation transfer imaging and spectroscopy. Prog. Nucl. Magn. Reson. Spectrosc. 48, 109–136. doi: 10.1016/j.pnmrs.2006.01.001
Zuo, C. S., Lin, P., Vitaliano, G., Wang, K., Villafuerte, R., and Lukas, S. E. (2015). A preliminary evaluation of the correlation between regional energy phosphates and resting state functional connectivity in depression. Neuroimage Clin. 9, 348–354. doi: 10.1016/j.nicl.2015.08.020
Keywords: vascular cognitive impairment, glymphatic system, diffusion tensor imaging, resting-state functional MRI, dynamic functional connectivity, structure–function coupling
Citation: Ma C, Liu A, Liu J, Wang X, Cong F, Li Y and Liu J (2025) A window into the brain: multimodal MRI assessment of vascular cognitive impairment. Front. Neurosci. 19:1526897. doi: 10.3389/fnins.2025.1526897
Edited by:
Ardalan Aarabi, University of Picardie Jules Verne, FranceReviewed by:
Wentai Zhang, Peking Union Medical College Hospital (CAMS), ChinaMario Versaci, Mediterranea University of Reggio Calabria, Italy
Copyright © 2025 Ma, Liu, Liu, Wang, Cong, Li and Liu. This is an open-access article distributed under the terms of the Creative Commons Attribution License (CC BY). The use, distribution or reproduction in other forums is permitted, provided the original author(s) and the copyright owner(s) are credited and that the original publication in this journal is cited, in accordance with accepted academic practice. No use, distribution or reproduction is permitted which does not comply with these terms.
*Correspondence: Ying Li, bGl5aW5nX3h4QDE2My5jb20=; Jing Liu, bGl1amluZ0BkbXUuZWR1LmNu