- 1Cell Signal Transduction Laboratory, Department of Preventive Medicine, Bioinformatics Center, School of Basic Medical Sciences, School of Software, Institute of Biomedical Informatics, Henan University, Kaifeng, China
- 2Department of Anesthesia, Stanford University, Stanford, CA, United States
- 3Henan Bioengineering Research Center, Zhengzhou, China
Bladder cancer (BC) is one of the most common malignant tumors in the urinary system. The discovery of prognostic biomarkers is still one of the major challenges to improve clinical treatment of BC patients. In order to assist biologists and clinicians in easily evaluating the prognostic potency of genes in BC patients, we developed a user-friendly Online consensus Survival tool for bladder cancer (OSblca), to analyze the prognostic value of genes. The OSblca includes gene expression profiles of 1,075 BC patients and their respective clinical follow-up information. The clinical follow-up data include overall survival (OS), disease specific survival (DSS), disease free interval (DFI), and progression free interval (PFI). To analyze the prognostic value of a gene, users only need to input the official gene symbol and then click the “Kaplan-Meier plot” button, and Kaplan-Meier curve with the hazard ratio, 95% confidence intervals and log-rank P-value are generated and graphically displayed on the website using default options. For advanced analysis, users could limit their analysis by confounding factors including data source, survival type, TNM stage, histological type, smoking history, gender, lymph invasion, and race, which are set up as optional parameters to meet the specific needs of different researchers. To test the performance of the web server, we have tested and validated its reliability using previously reported prognostic biomarkers, including KPNA2, TP53, and MYC etc., which had their prognostic values validated as reported in OSblca. In conclusion, OSblca is a useful tool to evaluate and discover novel prognostic biomarkers in BC. The web server can be accessed at http://bioinfo.henu.edu.cn/BLCA/BLCAList.jsp.
Introduction
As one of the most common malignant tumors of the urinary system, bladder cancer (BC) is estimated to cause about 549,393 new cases and 199,922 deaths worldwide in 2018 (1). Based on the clinic-pathological features, BC could be classified into two types: non-muscle invasive tumor (NMIBC, 70–80% of BC patient) and muscle-invasive tumor (MIBC, 20–30% of BC patient) (2, 3). Due to the relatively high rate of local recurrence and metastasis in MIBC patients, the treatment outcome is still poor, and the survival rate is lower than that of NMIBC patients. Although NMIBC patients have better survival rates than MIBC, 30–50% of NMIBC patients experience cancer recurrence (4). One of the major challenges to improve clinical outcomes of BC patients is to screen novel biomarkers for diagnosis and prognosis (5).
In recent years, a large number of prognostic biomarkers including DNA markers and protein markers have been reported (6–8). Some of the prognostic biomarkers, especially the ones involved in biological processes, are useful to identify high-risk patients, and could be used to predict the prognosis and treatment response. However, few biomarkers have been translated into clinics due to the lack of independent validation (5, 9, 10). With the advance of high through-put technologies, more and more studies analyzed the gene expression of cancer samples and uploaded these data on public databases such as The Cancer Genome Atlas (TCGA, https://portal.gdc.cancer.gov/) and Gene Expression Omnibus (GEO, https://www.ncbi.nlm.nih.gov/geo/). These data offer opportunities for the biomarker discovery, validation, and clinical application (11, 12). Unfortunately, until now, this convenient online tool is still unavailable to clinicians and biologists to evaluate and verify the prognostic value of the genes of interests in different datasets for BC.
To solve this problem, we developed an online web server named OSblca, which consists of gene expression profiles and relative clinical information of 1,075 bladder cancer patients from seven independent cohorts collected from TCGA and GEO databases. This web server enables researchers and clinicians to analyze the prognostic value of a gene of interest and accelerates the development of prognostic biomarkers.
Methods
Datasets Collection
Gene expression profiles and clinical follow-up information of bladder cancer patients were collected from TCGA and GEO databases. For TCGA dataset, level-3 gene expression profiling data (HiSeqV2) and clinical information of BC samples were downloaded in April 2018. In order to collect the relative datasets from GEO, keywords including “bladder cancer,” “prognosis,” “survival,” and “gene expression” were used to search in GEO database. Next, manual checks of the availability of data of mRNA expression, clinical survival information and at least 50 patients were performed.
Development of OSblca
The OSblca web server was developed by Java script, and hosted by Tomcat 7.0 on Windows 2008. The database system that stores the gene expression and clinical data was handled by SQL Server 2008. The R package “RODBC” is used as a middleware to connect R and SQL. The input of OSblca web server must be the official gene symbol from NCBI (https://www.ncbi.nlm.nih.gov/). The outputs include Kaplan Meier (KM) survival curves, Hazard ratio (HR with 95% confidence interval) and log-rank P-value that are produced by R package “survival” (https://CRAN.R-project.org/package=survival). A gene could be regarded as a potential prognostic biomarker for BC patients when the log-rank P-value is < 0.05. OSblca can be accessed at http://bioinfo.henu.edu.cn/BLCA/BLCAList.jsp. A web server architecture diagram is presented in Figure 1A. The screenshot of the web server interface and the result are shown in Figure 1B.
Validation of Previously Published Prognostic Biomarkers in OSblca
In order to validate the performance of prognostic analysis in our web server, prognosis biomarkers for BC were searched in PubMed using the keywords “bladder cancer,” “survival,” “gene expression,” “biomarker,” and “prognosis.” The prognostic capabilities of these genes were evaluated in all cohorts, and all cutoff values in “splitting the patients” were tested in each cohort to get the best cutoff value.
Results
Clinical Characteristics of the Patients in OSblca
According to our criteria, in total 1,075 unique bladder cancer patients were collected from seven data sets including one TCGA cohort and six GEO cohorts. Survival information including overall survival (OS), disease specific survival (DSS), disease free interval (DFI), progression free interval (PFI) were gathered. No patient was lost to follow-up. Of the above, 935 patients have overall survival information, and the median overall survival time is 25.03 months. We also collected age, TNM stage, histological type, gender, smoking history, lymph invasion and race as confounding clinical factors. The average age is 68 ± 11. Distribution of TNM stages is as follows: stage I (n = 287, 29.64%), stage II (n = 233, 23.26%), stage III (n = 239, 23.85%), and stage IV (n = 209, 20.86%). The ratio of male to female of patients was close to 3:1. A summary of clinical properties for each dataset is presented in Table 1.
Survival Analysis of BC Patients Based on Clinical Characteristics
The Kaplan-Meier plots for the bladder cancer patients in OSblca stratified by TNM stage, histological type, gender, smoking history, lymph invasion, and race are presented in Figure 2. In these 1,075 patients, TNM stage, smoking history, lymph invasion, and histological type were significantly associated with overall survival (P < 0.0001, P = 0.0206, P < 0.0001, and P < 0.0001, respectively), which were consistent with previously reports (14–16). Nevertheless, gender and race showed no significant association with overall survival (P = 0.2260 and P = 0.5513).
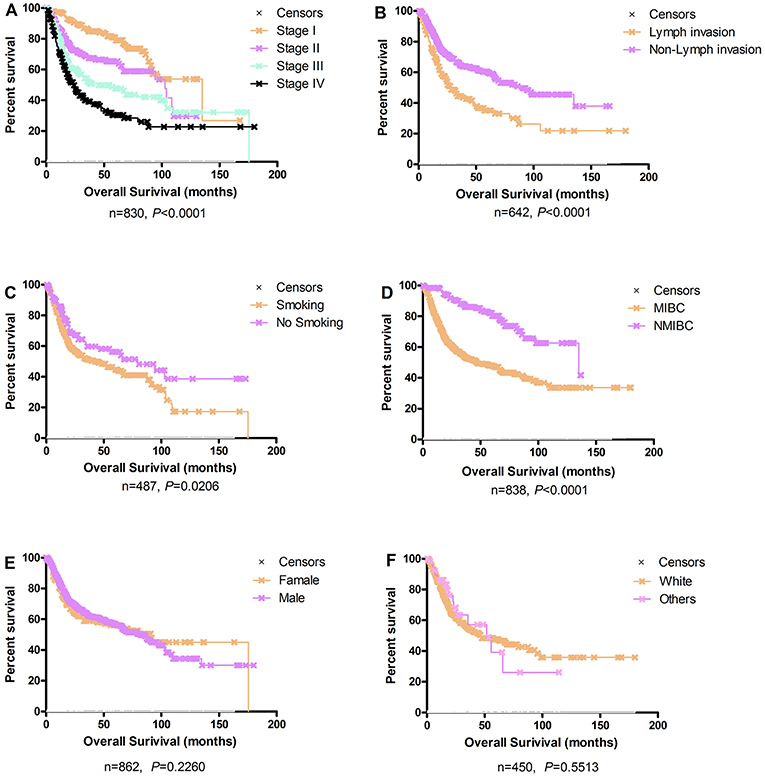
Figure 2. Survival analysis of clinical characteristics of the bladder cancer patients in OSblca. (A) TNM stage; (B) Lymph invasion; (C) Smoking history; (D) Histological type; (E) Gender; (F) Race.
Usage of OSblca
The main function that OSblca provides is to evaluate and verify the prognostic value for a given gene. “Gene symbol,” “Data source,” “Survival,” and “Split patients” are set as the four main parameters. The input dialog box of “Gene symbol” is on the upper left of the OSblca page (Figure 3A). A red prompting message will show up when the input is not an official gene symbol. “Data source” provides eight options including independent analysis in one of seven cohorts and in a combined cohort consisting of all the BC patients from seven cohorts. The users can choose to evaluate the prognosis of a given gene in an individual cohort or in a combined cohort according to their needs. Under “Survival” option, four prognostic terms including OS, DSS, DFI, and PFI are provided. In the “Split patients” dialog box, user can select different thresholds of gene expression levels to divide patients into two subgroups for input gene. After then, by clicking the “Kaplan-Meier plot” button, OSblca server will take the request and return the analysis results, which are graphically displayed and presented with HR, 95% CI and log-rank P-value (Figure 3B).
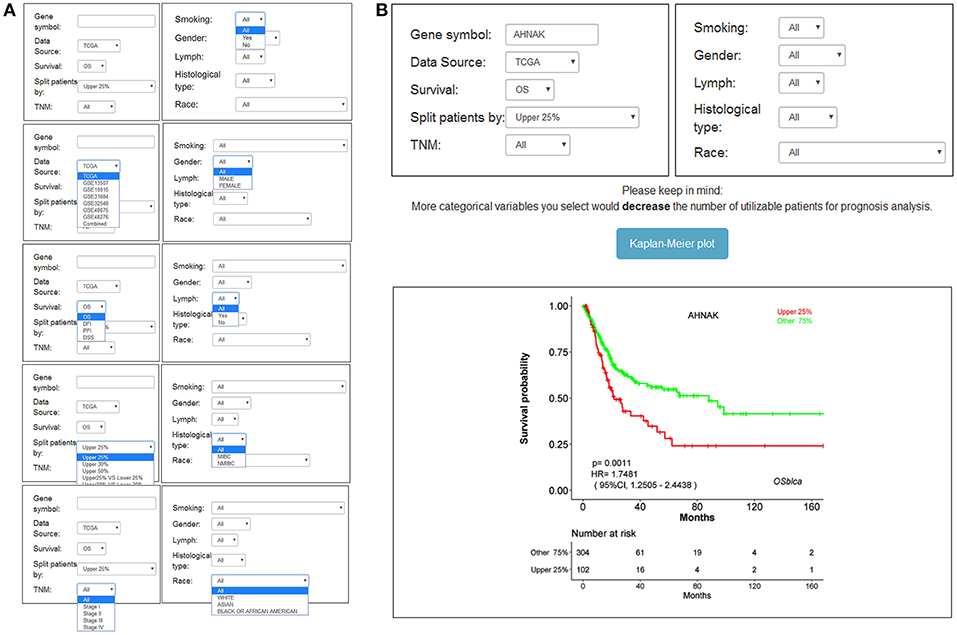
Figure 3. Input and output interface of OSblca. (A) The options of main input parameters and clinical factors of OSblca; (B) The output interface of OSblca.
In order to meet the specific needs, six confounding clinical factors including TNM stage, smoking history, gender, lymph, histological type, and race, were set as optional filter factors in the prognostic analysis. As showed in Figure 3A, each factor has 2–5 options for users to choose from.
Validation of Previously Published BC Biomarkers
To test the reliability of prognosis prediction in our web server, we evaluated 21 prognostic biomarkers from 16 previously reported literatures in the OSblca web server, including KPNA2, TP53, and MYC (17–32). As shown in Table 2, 17 out of 21 (82%) previous reported prognostic biomarkers were showed to have significant prognostic potency in OSblca, while the remaining four previously reported prognostic biomarkers did not reach significance in OSblca. Among the 17 validated prognostic biomarkers, 11 genes showed significant prognostic abilities in the combined cohort.
Discussion
The discovery of prognostic biomarkers is a hot topic in translational research. In the current study, we present a convenient web server to assist researchers and clinicians to quickly screen and evaluate the prognostic value of genes in different cohorts of BC. As shown in a straightforward web interface, people without much bioinformatics experience can easily navigate OSblca to investigate genes of interests. In addition, users can perform survival analysis filtered by one or several factors according to the specific research purposes of their needs.
The validation of previously reported prognostic biomarkers in OSblac showed that our web tool is reliable and can be used in prognostic analysis for BC patients. Notably, 11 genes, such as KPNA2 and TP53, were confirmed as prognostic biomarkers in the combined cohort, which indicated that these genes may be more widely applied as prognostic candidates for BC patients.
In summary, OSblca is a free online survival analysis web server that allows clinicians and researchers to rapidly analyze the prognostic value of a given gene in BC. We will keep updating OSblca to make it more powerful for the users.
Data Availability
Publicly available datasets were analyzed in this study. This data can be found here: http://bioinfo.henu.edu.cn/BLCA/BLCAList.jsp.
Author Contributions
GZ, QW, MY, and XG collected data, developed the server, and drafted the paper. QY, YD, XS, YA, and HD set up the server and performed the analyses. LX, WZ, and YW contributed to data analysis and paper writing. All authors edited and approved the final manuscript.
Funding
This work was supported by the National Natural Science Foundation of China (No.81602362), the program for Science and Technology Development in Henan Province (No.162102310391), the supporting grants of Henan University (No.2015YBZR048; No.B2015151), the program for Innovative Talents of Science and Technology in Henan Province (No.18HASTIT048), and Yellow River Scholar Program (No.H2016012), Kaifeng Science and Technology Major Project (No.18ZD008).
Conflict of Interest Statement
The authors declare that the research was conducted in the absence of any commercial or financial relationships that could be construed as a potential conflict of interest.
References
1. Bray F, Ferlay J, Soerjomataram I, Siegel RL, Torre LA, Jemal A. Global cancer statistics 2018: GLOBOCAN estimates of incidence and mortality worldwide for 36 cancers in 185 countries. CA Cancer J Clin. (2018) 68:394–424. doi: 10.3322/caac.21492
2. Knowles MA, Hurst CD. Molecular biology of bladder cancer: new insights into pathogenesis and clinical diversity. Nat Rev Cancer. (2015) 15:25–41. doi: 10.1038/nrc3817
4. Babjuk M, Bohle A, Burger M, Capoun O, Cohen D, Comperat EM, et al. EAU guidelines on non-muscle-invasive urothelial carcinoma of the bladder: update 2016. Eur Urol. (2017) 71:447–61. doi: 10.1016/j.eururo.2016.05.041
5. Netto GJ. Molecular biomarkers in urothelial carcinoma of the bladder: are we there yet? Nat Rev Urol. (2011) 9:41–51. doi: 10.1038/nrurol.2011.193
6. Sapre N, Herle P, Anderson PD, Corcoran NM, Hovens CM. Molecular biomarkers for predicting outcomes in urothelial carcinoma of the bladder. Pathology. (2014) 46:274–82. doi: 10.1097/PAT.0000000000000110
7. Xylinas E, Kluth LA, Lotan Y, Daneshmand S, Rieken M, Karakiewicz PI, et al. Blood- and tissue-based biomarkers for prediction of outcomes in urothelial carcinoma of the bladder. Urol Oncol. (2014) 32:230–42. doi: 10.1016/j.urolonc.2013.06.009
8. Xie L, Dang Y, Guo J, Sun X, Xie T, Zhang L, et al. High KRT8 expression independently predicts poor prognosis for lung adenocarcinoma patients. Genes. (2019) 10:E36. doi: 10.3390/genes10010036
9. Bolenz C, Lotan Y. Molecular biomarkers for urothelial carcinoma of the bladder: challenges in clinical use. Nat Clin Pract Urol. (2008) 5:676–85. doi: 10.1038/ncpuro1259
10. van Rhijn BW, van der Poel HG, van der Kwast TH. Urine markers for bladder cancer surveillance: a systematic review. Eur Urol. (2005) 47:736–48. doi: 10.1016/j.eururo.2005.03.014
11. Guo X, Tang Y, Zhu W. Distinct esophageal adenocarcinoma molecular subtype has subtype-specific gene expression and mutation patterns. BMC Genomics. (2018) 19:769. doi: 10.1186/s12864-018-5165-0
12. Choi W, Ochoa A, McConkey DJ, Aine M, Hoglund M, Kim WY, et al. Genetic alterations in the molecular subtypes of bladder cancer: illustration in the cancer genome atlas dataset. Eur Urol. (2017) 72:354–65. doi: 10.1016/j.eururo.2017.03.010
13. Liu J, Lichtenberg T, Hoadley KA, Poisson LM, Lazar AJ, Cherniack AD, et al. An integrated TCGA pan-cancer clinical data resource to drive high-quality survival outcome analytics. Cell. (2018) 173:400–16e11. doi: 10.1016/j.cell.2018.02.052
14. Proctor I, Stoeber K, Williams GH. Biomarkers in bladder cancer. Histopathology. (2010) 57:1–13. doi: 10.1111/j.1365-2559.2010.03592.x
15. Samanic C, Kogevinas M, Dosemeci M, Malats N, Real FX, Garcia-Closas M, et al. Smoking and bladder cancer in Spain: effects of tobacco type, timing, environmental tobacco smoke, and gender. Cancer Epidemiol Biomarkers Prev. (2006) 15:1348–54. doi: 10.1158/1055-9965.EPI-06-0021
16. Lotan Y, Gupta A, Shariat SF, Palapattu GS, Vazina A, Karakiewicz PI, et al. Lymphovascular invasion is independently associated with overall survival, cause-specific survival, and local and distant recurrence in patients with negative lymph nodes at radical cystectomy. J Clin Oncol. (2005) 23:6533–9. doi: 10.1200/JCO.2005.05.516
17. Jensen JB, Munksgaard PP, Sorensen CM, Fristrup N, Birkenkamp-Demtroder K, Ulhoi BP, et al. High expression of karyopherin-alpha2 defines poor prognosis in non-muscle-invasive bladder cancer and in patients with invasive bladder cancer undergoing radical cystectomy. Eur Urol. (2011) 59:841–8. doi: 10.1016/j.eururo.2011.01.048
18. Kramer MW, Escudero DO, Lokeshwar SD, Golshani R, Ekwenna OO, Acosta K, et al. Association of hyaluronic acid family members (HAS1, HAS2, and HYAL-1) with bladder cancer diagnosis and prognosis. Cancer. (2011) 117:1197–209. doi: 10.1002/cncr.25565
19. Youssef RF, Shariat SF, Kapur P, Kabbani W, Ghoneim T, King E, et al. Expression of cell cycle-related molecular markers in patients treated with radical cystectomy for squamous cell carcinoma of the bladder. Human Pathol. (2011) 42:347–55. doi: 10.1016/j.humpath.2010.07.012
20. Schultz L, Albadine R, Hicks J, Jadallah S, DeMarzo AM, Chen YB, et al. Expression status and prognostic significance of mammalian target of rapamycin pathway members in urothelial carcinoma of urinary bladder after cystectomy. Cancer. (2010) 116:5517–26. doi: 10.1002/cncr.25502
21. Kauffman EC, Robinson BD, Downes MJ, Powell LG, Lee MM, Scherr DS, et al. Role of androgen receptor and associated lysine-demethylase coregulators, LSD1 and JMJD2A, in localized and advanced human bladder cancer. Mol Carcinog. (2011) 50:931–44. doi: 10.1002/mc.20758
22. Wu P, Liu S, Zhang W, Zhang Y, Zhu G, Wei D, et al. Low-level Ki-67 expression as an independent predictor of bladder tumour recurrence in patients with primary upper tract urothelial carcinoma after radical nephroureterectomy. Jpn J Clin Oncol. (2015) 45:1175–81. doi: 10.1093/jjco/hyv139
23. Harshman LC, Bepler G, Zheng Z, Higgins JP, Allen GI, Srinivas S. Ribonucleotide reductase subunit M1 expression in resectable, muscle-invasive urothelial cancer correlates with survival in younger patients. BJU Int. (2010) 106:1805–11. doi: 10.1111/j.1464-410X.2010.09327.x
24. Aziz A, Lessard A, Moore K, Hovington H, Latulippe E, Larue H, et al. Improved cancer specific-survival in patients with carcinoma invading bladder muscle expressing cyclo-oxygenase-2. BJU Int. (2011) 108:531–7. doi: 10.1111/j.1464-410X.2010.09909.x
25. Chan OTM, Furuya H, Pagano I, Shimizu Y, Hokutan K, Dyrskjot L, et al. Association of MMP-2, RB and PAI-1 with decreased recurrence-free survival and overall survival in bladder cancer patients. Oncotarget. (2017) 8:99707–21. doi: 10.18632/oncotarget.20686
26. Jager T, Becker M, Eisenhardt A, Tilki D, Totsch M, Schmid KW, et al. The prognostic value of cadherin switch in bladder cancer. Oncol Rep. (2010) 23:1125–32. doi: 10.3892/or_00000741
27. Shiina H, Igawa M, Shigeno K, Yamasaki Y, Urakami S, Yoneda T, et al. Clinical significance of mdm2 and p53 expression in bladder cancer. A comparison with cell proliferation and apoptosis. Oncology. (1999) 56:239–47. doi: 10.1159/000011971
28. Levidou G, Saetta AA, Karlou M, Thymara I, Pratsinis H, Pavlopoulos P, et al. D-type cyclins in superficial and muscle-invasive bladder urothelial carcinoma: correlation with clinicopathological data and prognostic significance. J Cancer Res Clin Oncol. (2010) 136:1563–71. doi: 10.1007/s00432-010-0814-y
29. Canesin G, Gonzalez-Peramato P, Palou J, Urrutia M, Cordon-Cardo C, Sanchez-Carbayo M. Galectin-3 expression is associated with bladder cancer progression and clinical outcome. Tumour Biol. (2010) 31:277–85. doi: 10.1007/s13277-010-0033-9
30. Guo G, Xu Y, Gong M, Cao Y, An R. USP28 is a potential prognostic marker for bladder cancer. Tumour Biol. (2014) 35:4017–22. doi: 10.1007/s13277-013-1525-1
31. Mizutani Y, Katsuoka Y, Bonavida B. Prognostic significance of second mitochondria-derived activator of caspase (Smac/DIABLO) expression in bladder cancer and target for therapy. Int J Oncol. (2010) 37:503–8. doi: 10.3892/ijo_00000699
Keywords: bladder cancer, prognostic biomarker analysis, web server, kaplan-meier curve, cox regression model
Citation: Zhang G, Wang Q, Yang M, Yuan Q, Dang Y, Sun X, An Y, Dong H, Xie L, Zhu W, Wang Y and Guo X (2019) OSblca: A Web Server for Investigating Prognostic Biomarkers of Bladder Cancer Patients. Front. Oncol. 9:466. doi: 10.3389/fonc.2019.00466
Received: 05 March 2019; Accepted: 15 May 2019;
Published: 04 June 2019.
Edited by:
Prashant Patel, University of Birmingham, United KingdomReviewed by:
Piotr Bryniarski, Medical University of Silesia, PolandVadim S. Koshkin, University of California, San Francisco, United States
Copyright © 2019 Zhang, Wang, Yang, Yuan, Dang, Sun, An, Dong, Xie, Zhu, Wang and Guo. This is an open-access article distributed under the terms of the Creative Commons Attribution License (CC BY). The use, distribution or reproduction in other forums is permitted, provided the original author(s) and the copyright owner(s) are credited and that the original publication in this journal is cited, in accordance with accepted academic practice. No use, distribution or reproduction is permitted which does not comply with these terms.
*Correspondence: Xiangqian Guo, eHFndW9AaGVudS5lZHUuY24=
†These authors have contributed equally to this work