- 1 Department of Radiology, University Hospital Southampton, Southampton, United Kingdom
- 2Department of Medical Imaging, Bournemouth University, Bournemouth, United Kingdom
- 3Department of Radiology, Oxford University Hospitals, Oxford, United Kingdom
- 4Department of Oncology, Oxford University, Oxford, United Kingdom
- 5Department of Metabolism, Digestion and Reproduction, Imperial College London, London, United Kingdom
- 6Department of Surgery, University Hospital Southampton, Southampton, United Kingdom
- 7Academic Unit of Cancer Sciences, University of Southampton, Southampton, United Kingdom
Colorectal liver metastases (CRLM) have heterogenous histopathological and immunohistochemical phenotypes, which are associated with variable responses to treatment and outcomes. However, this information is usually only available after resection, and therefore of limited value in treatment planning. Improved techniques for in vivo disease assessment, which can characterise the variable tumour biology, would support further personalization of management strategies. Advanced imaging of CRLM including multiparametric MRI and functional imaging techniques have the potential to provide clinically-actionable phenotypic characterisation. This includes assessment of the tumour-liver interface, internal tumour components and treatment response. Advanced analysis techniques, including radiomics and machine learning now have a growing role in assessment of imaging, providing high-dimensional imaging feature extraction which can be linked to clinical relevant tumour phenotypes, such as a the Consensus Molecular Subtypes (CMS). In this review, we outline how imaging techniques could reproducibly characterize the histopathological features of CRLM, with several matched imaging and histology examples to illustrate these features, and discuss the oncological relevance of these features. Finally, we discuss the future challenges and opportunities of CRLM imaging, with a focus on the potential value of advanced analytics including radiomics and artificial intelligence, to help inform future research in this rapidly moving field.
Introduction
Colorectal cancer (CRC) remains the second leading cause of cancer-related death in the developed world (1). Liver metastases are a major cause of death in patients with CRC and therefore optimising treatment of colorectal liver metastases (CRLM) is an important target for future research. Approximately 15% of patients will have synchronous liver metastases at initial diagnosis, with up to half developing liver metastases during their clinical course (2).
There are two main therapeutic strategies for CRLM. For those patients with polymetastatic disease, palliative systemic therapy is the mainstay of treatment. In patients with ‘oligometastatic’ disease (3), curative-intent surgical or image-guided treatment can be offered, often following neoadjuvant chemotherapy. Five-year survival rates following hepatic metastasectomy for CRLM are 28-49% (4), with some long-term survivors, in contrast to patients with polymetastatic disease who have a 1-year survival rate of approximately 55%, and 5-year survival rate of 3% (2). Historically, stricter surgical criteria have limited patient numbers proceeding to resection but, as evidence emerges that even patients with extensive disease derive benefit from local treatment (5), more patients are being offered treatment with curative intent. Selecting which patients who may benefit from radical treatment is an important challenge for clinicians, given that a significant proportion of patients undergoing metastasectomy suffer early relapse with incurable disease (4, 6). In the polymetastatic setting, multiple agents, including various cytotoxic regimens, targeted treatments and immunotherapies have transformed the options for patients without a curative option (7). Anticipating which agents will work optimally for each individual patient is critical, especially considering a counterintuitive response of patients to many of these therapies (8), which underlines the need for a more detailed assessment of colorectal metastases prior to commencing therapy.
The pathological literature describes the varied histopathological features of CRLM, both their internal architecture and their interface with the surrounding liver parenchyma (9, 10). Several histopathological and immunohistochemical phenotypes are associated with differential prognostic outcomes (10, 11). Unfortunately, histological phenotypic information is principally only available after resection, and is therefore of limited value in pretreatment prognostication, or when planning the neoadjuvant or polymetastatic treatment.
Improved techniques for in vivo disease assessment, which can characterise the variable tumour biology, would allow clinicians to plan personalized management strategies. Imaging already plays a central role in assessing the sites and burden of metastatic cancer both before and after treatment (12). Advanced imaging techniques, in particular multiparametric MRI (mpMRI) and functional imaging techniques, combined with novel image analysis techniques, have the potential to improve disease characterisation, and the advantage of being non-invasive, repeatable, and with the potential to assess all tumour sites.
In this narrative review, we outline how imaging techniques could reproducibly characterize the histopathological features of CRLM, with several matched imaging and histology examples to illustrate these features and discuss the oncological relevance of these features. We discuss the future opportunities and challenges of CRLM imaging, with a focus on the potential value of advanced analytics including radiomics and artificial intelligence, to help inform future research in this rapidly moving field. This review was informed by searching PubMed for relevant papers using search terms including ‘colorectal’, ‘liver metastas*’, ‘MRI’, ‘CT’, ‘PET’ and ‘imaging biomarker’ and a search of references.
Imaging of CRLM
Contrast enhanced CT (ceCT) is the mainstay of oncological imaging, and is the first line test for staging, surveillance and response assessment. Where there is diagnostic uncertainty, or precision about the number of metastases is crucial, mpMRI is the gold-standard technique for detecting and characterising focal liver lesions. Standard sequences include unenhanced T1- and T2-weighted sequences (including opposed-phase imaging), diffusion weighted imaging (DWI) and multiphase acquisitions following intravenous extracellular gadolinium chelate contrast media or liver-specific contrast agents. For CRLM, multiparametric liver MRI has superior per patient and per lesion sensitivity to CT (13, 14), and provides higher per lesion sensitivity than 18F-fluorodeoxyglucose-positron emission tomography/CT (FDG-PET/CT) (13). Additionally, it provides high quality anatomical information which is invaluable for treatment-planning. It is therefore recommended as part of routine imaging work-up for patients being staged prior to liver-directed therapy (15). 18F-fluorodeoxyglucose-positron emission tomography/CT (FDG-PET/CT) is often used in patients with CRLM to detect extrahepatic disease that would preclude a radical treatment approach (16).
Radiopathological Features of CRLM
Tumour Interface With Normal Liver
Arguably the most clinically-relevant histopathological feature amenable to evaluation by imaging is the interface between normal liver and tumour. Interface features could influence chemotherapy selection, in particular for antiangiogenic agents (17, 18), inform surgical approach (19) and improve risk stratification for recurrence and overall survival (20, 21). These features are also well covered in another recent review (22).
The growth pattern of CRLM has been subdivided into three patterns of interface with the liver parenchyma: ‘pushing’, ‘desmoplastic’ and ‘replacement’ (9). The ‘pushing’ pattern is characterized by direct abutment of tumour cells on the liver parenchyma, with expansile growth flattening the liver plates. The ‘desmoplastic’ interface (present in approximately 40%) is differentiated by a rim of desmoplastic, fibrotic stroma incorporating a lymphocytic infiltrate, numerous bile ducts and capillaries (Figure 1E). This classification includes the term ‘pseudo-encapsulated metastases’ and has been linked to improved outcomes compared with non-desmoplastic metastases (17, 18, 20, 21, 23). It has also been suggested a less aggressive surgical approach, with a narrower margin, can be attempted with desmoplastic lesions, thus sparing more normal liver (19). The ‘replacement’ growth pattern is characterised by intimate contact between tumour cells and hepatocytes with a preserved reticulin pattern within the tumour tissue. Growth of these lesions therefore appears to be via vascular co-option, rather than angiogenesis, which is supported by their proven poor response to anti-angiogenic agents (17, 18, 23).
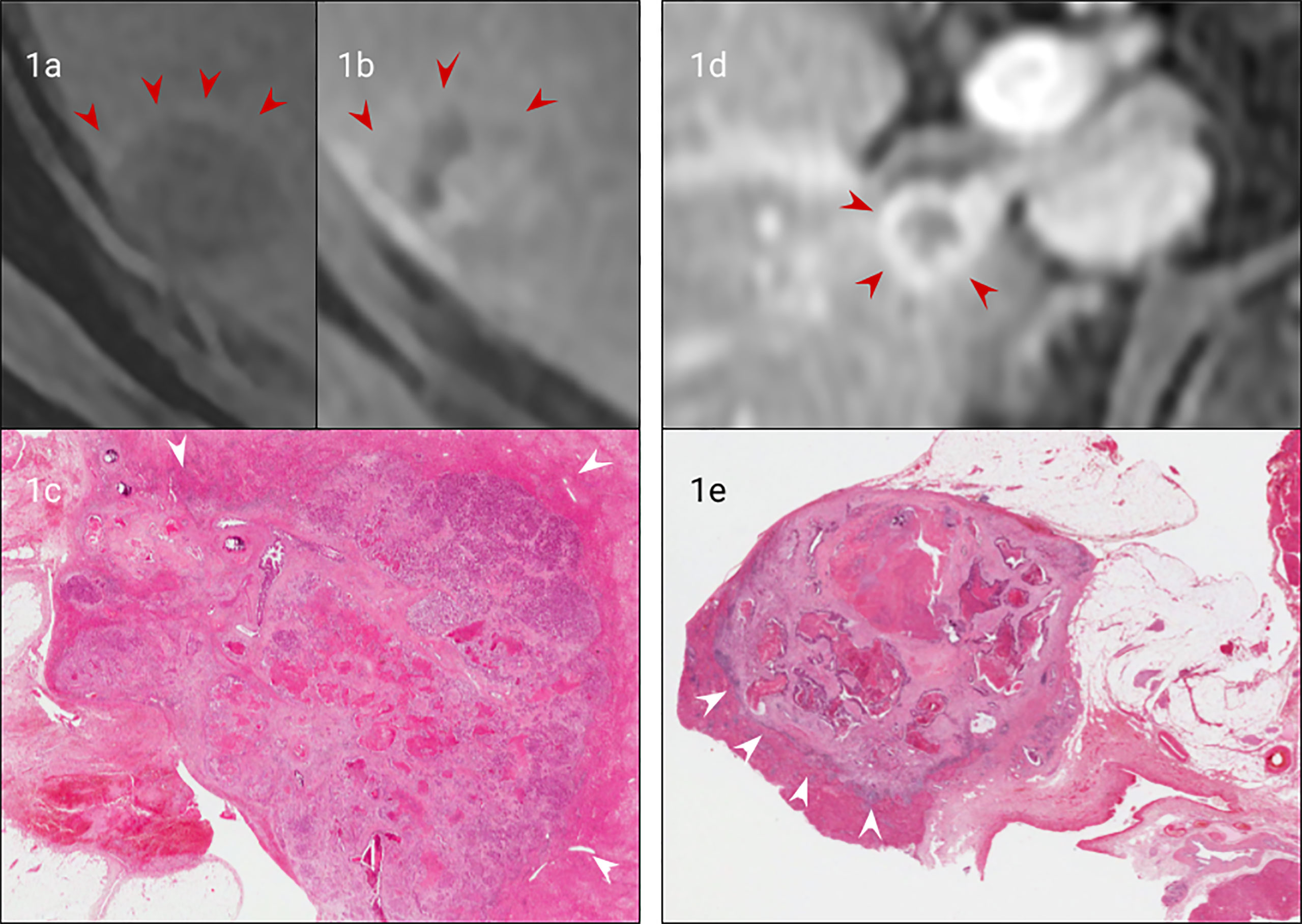
Figure 1 Matched imaging and histology of non-encapsulated (A–C) vs capsulated CRLM (A, D, E). Arterial phase gadolinium-enhanced T1 fat saturated MRI of a CRLM with early peripheral enhancement indicating compression of hepatocytes (arrows), (B). Portal venous gadolinium-enhanced T1 fat saturated MRI showing an absence of peripheral enhancement with isointensity to normal liver (arrows), (C). H&E staining (from (A, B) confirming no true capsule with peripheral compression of hepatocytes (arrows). (D). Portal venous phase gadolinium-enhanced T1 fat saturated MRI demonstrating clear peripheral enhancement of a fibrotic capsule (arrows), (E). H&E staining of the CRLM (from (D) confirming a true fibrotic capsule/desmoplastic interface (arrows).
There are several potential imaging correlates that could predict the presence of these patterns in vivo. The presence of a peripheral fibrotic capsule can be indicated by MRI (Figure 1D) (24–27), given typical MRI characteristics of fibrous tissue which is typically low T1 and T2 signal, and accumulates contrast on delayed contrast enhanced imaging (e.g. Figure 1). These features may reliably distinguish desmoplastic from other tumour types, although further research is required to establish the utility of this as a diagnostic tool.
Hepatocyte-specific contrast agents could also be useful in determining a ‘replacement’ growth pattern. This is similar to how microvascular invasion (MVI) can be identified with hepatocellular carcinoma (HCC) as reproducible hypoperfusion of liver parenchyma surrounding the tumour due to subtle tumour infiltration (28). However, this feature is currently untested in ‘replacement’ interface patterns of CRLM.
Vascular, Biliary, Lymphatic and Perineural Invasion
Vascular invasion is a common feature of colorectal cancer and its liver metastases. Intrahepatic portal venous invasion local to resected metastases occurs in approximately a quarter of cases (reported range 10-49%) and hepatic venous invasion in approximately one tenth of cases (reported range 5-24%) (10). Like primary colorectal cancer, venous invasion has been associated with poorer clinical outcomes (29). High resolution pelvic MRI has been shown to reliably identify extramural venous invasion of primary rectal cancer (30) and is now a useful prognostic marker. Similarly, although large vessel invasion is less common in CRLM, adjacent venous invasion can be similarly demonstrated (Figure 2).
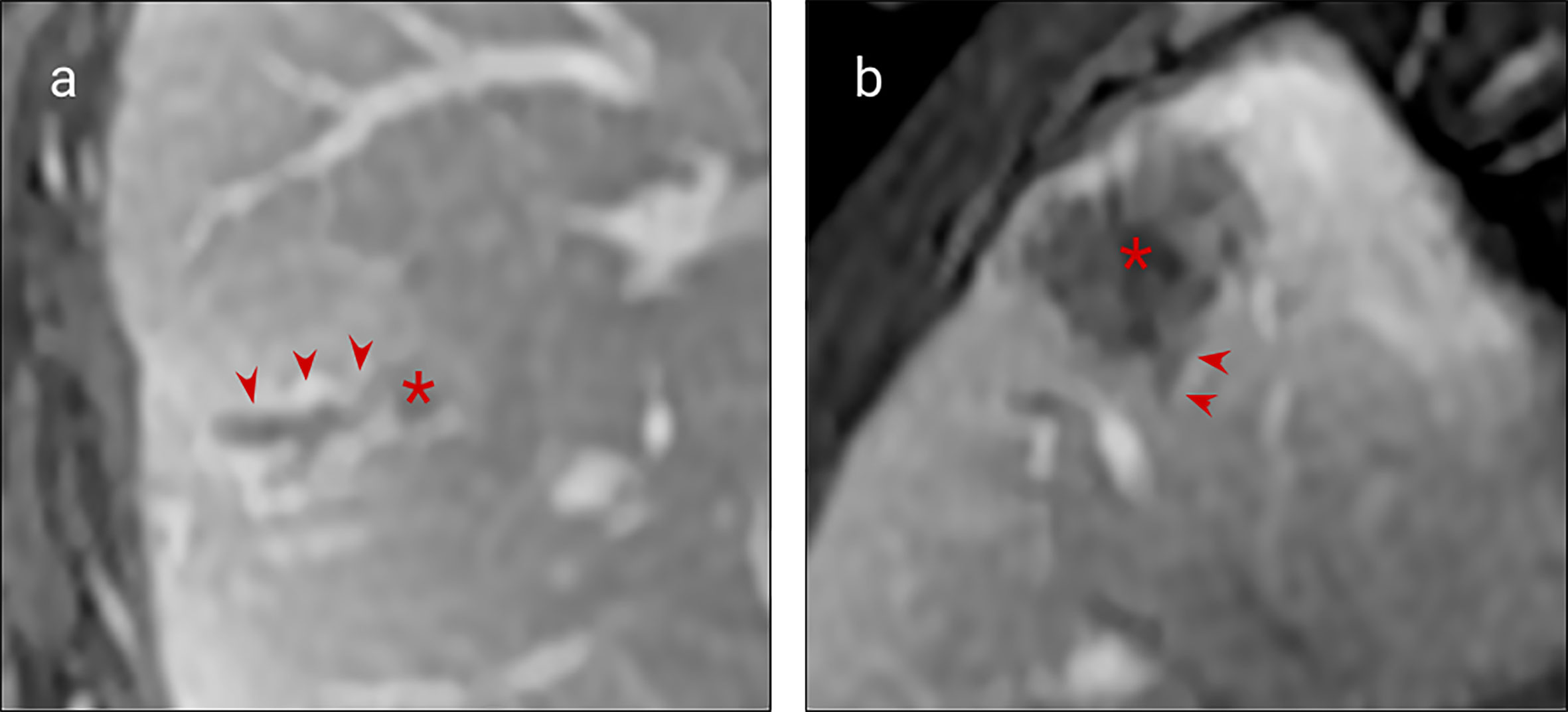
Figure 2 MRI examples of histologically confirmed CRLM with macroscopic vascular invasion. (A, B) Portal venous phase gadolinium-enhanced T1 fat saturated MRI shows CRLM lesions (asterisk) with a filling defect within a hepatic vein indicating macroscopic venous invasion (arrows).
Lymphatic invasion is a less common feature than venous invasion but has again been shown to be associated with poorer outcomes (31–33). Lymphatic vessels are smaller than the resolution of current imaging techniques, and therefore lymphatic invasion has not been reported to be directly accessible by MRI, although the presence of periportal, retroperitoneal or more distant lymphadenopathy may be a surrogate marker for this pathological feature. Neither biliary nor perineural invasion has been associated with adverse clinical outcomes (10) and there are no imaging studies correlating these features with MRI in CRLM.
Tumour Composition and Markers of Treatment Response
Features of Internal Composition
The internal composition of tumours differs, comprising variable proportions of tumour cells, fibrosis, necrotic material and, in some cases mucin and calcification. It is also influenced by adjuvant treatment (34, 35). These tumour components have typical MRI features (as shown in Table 1 and illustrated in Figure 3).
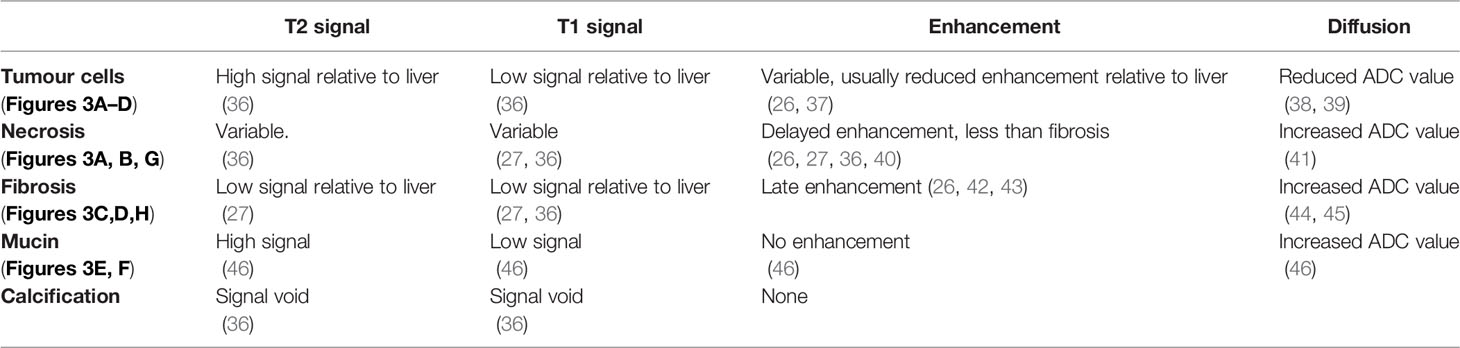
Table 1 (See corresponding Figure 3).
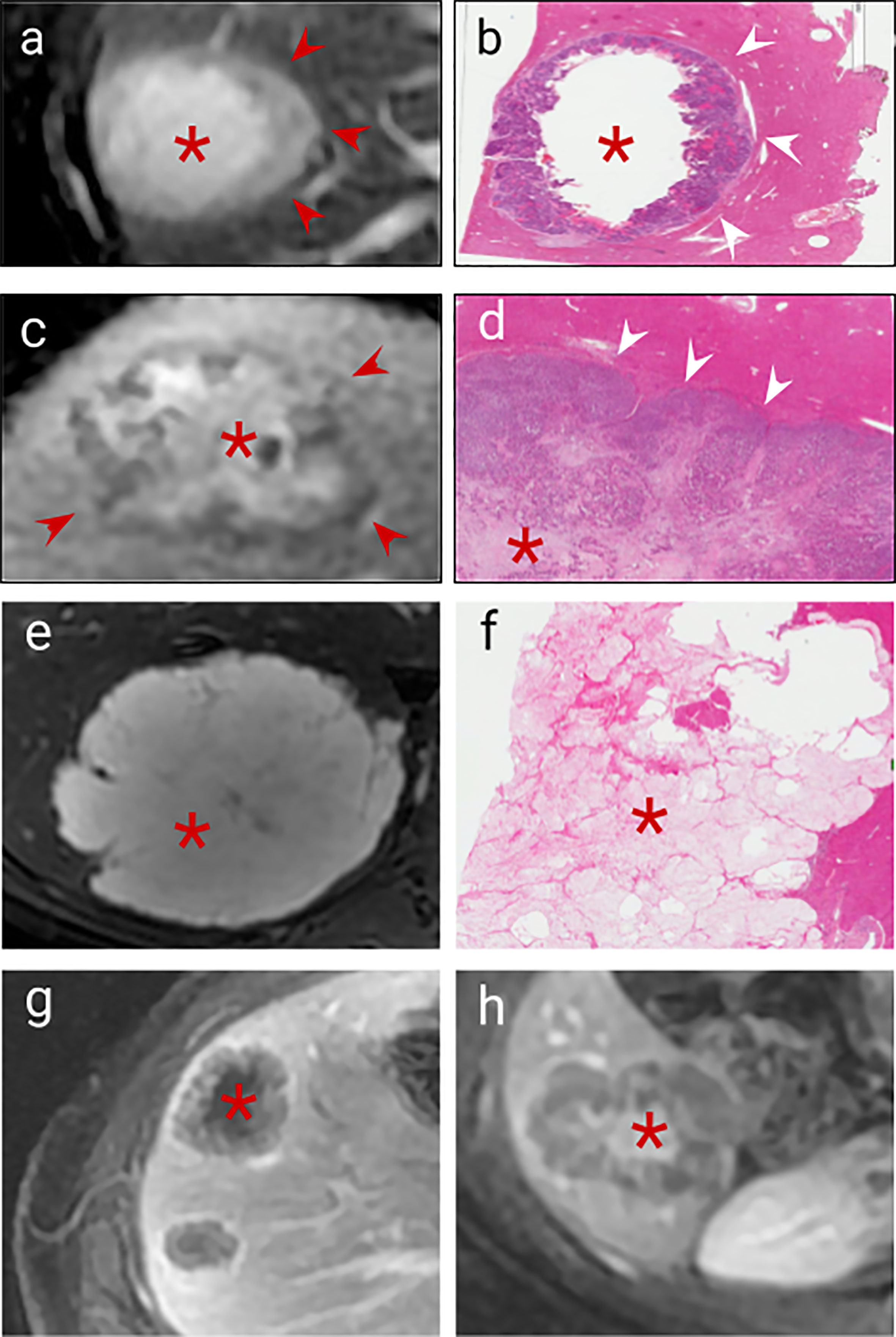
Figure 3 Imaging correlates of internal tumour composition. (A). T2 weighted non-enhanced MRI of a lesion displaying moderately high central T2 signal suggestive of central necrosis (asterisk) and surrounding viable tumour which is higher signal than normal liver (arrows), (B). H&E staining of this CRLM confirms peripheral viable tumour (arrows) with central liquefaction of the metastasis indicating classical Garland necrosis (asterisk), (C). Delayed phase gadolinium-enhanced T1 fat saturated MRI showing avid central delayed enhancement indicating fibrosis (asterisk) with surrounding viable tumour cells which enhance less than normal liver (arrows), (D). H&E staining of this CRLM confirming central fibrosis within the lesion (asteriks) and peripheral viable tumour cells (arrows), (E). T2 weighted sequence demonstrated homogenous high signal mucin (asterisk) (F). H&E staining confirming mucin containing metastasis (asterisk) (G). Delayed phase gadolinium-enhanced T1 fat saturated MRI showing centrally necrotic lesions (no delayed central enhancement, asteriks), (H). Delayed phase Gadolinium enhanced T1 fat saturated MRI showing late central enhancement indicating a centrally fibrotic lesion (asterisk) which contrasts with (G) (central necrosis).
Viable tumour cells contrast with normal liver on T1 and T2 weighted sequences (Table 1 and Figure 3A) and are generally hypoenhancing relative to background liver (Figures 3A, C, G) (26, 36–38). Necrosis is commonly found in chemo-naïve CRLMs, manifesting as T1 hyperintensity (differentiating it from other components) with variable enhancement, usually less than viable tumour and fibrosis (Figures 3A, B, G) (27, 40), with several histological subtypes recognised. CRLM often exhibit classical garland necrosis: areas of necrotic debris, sometimes referred to as ‘dirty’, ‘usual’ or intra-acinar necrosis, surrounded by a rim of viable tumour (Figures 3A, B) (27). Fibrosis demonstrates similar T1 and T2 signal characteristics to viable tumour, but typically has delayed gadolinium-enhancement (Figures 3C, D, H) (26, 42, 47) which differentiates it from tumour cells. Mucin is identified by pools of homogeneous high T2 and low T1 signal and absent enhancement (Figures 3E, F). Calcification characteristically presents signal voids on both T1 and T2-weighted sequences (Table 1) (36).
Defining the internal composition of metastases may help to categorise tumour biology and thus influence immunological and surgical strategy recently described Consensus Molecular Subtypes (CMS) of colorectal liver metastases (11), can be characterized by particular histopathological features and could be categorized by imaging. The molecular subtyping of liver metastases may be important even in patients when a resected primary tumour specimen is available, as significant discordance exists between primary and metastatic molecular subtypes (46, 48, 49). Within-patient heterogeneity is implicated as the root cause of a variable immunological response between primary and metastatic lesions (50, 51).
Pitroda et al. (11) have proposed three different CRLM subtypes [rather than the four classical colorectal molecular subtypes (52)]. They have identified a stromal metastasis subtype (with epithelial-mesenchymal transition and angiogenesis molecular signatures plus SMAD3 mutation association, subtype 3) which demonstrates significant baseline intratumoral fibrosis (11), in contrast to an immune-subtype (subtype 2) which demonstrates peritumoral lymphocyte infiltration and minimal intratumoural fibrosis (11). As outlined in Table 1, fibrosis is readily identified on MRI (27), whereas the degree of angiogenesis/microvascular density could be evaluated by Diffusion Weighted Imaging (DWI) and DCE-MRI enhancement (53–55). These imaging features are amenable to conventional visual, or radiomic derivation, and could provide a CMS prediction, and support personalised treatment. As yet, there have been no studies linking radiological imaging to CMS subtypes, although deep learning technologies have been applied to histological images to predict CMS subtypes and advanced imaging processing techniques (56), which are discussed in more detail below, have been used to classify other molecular features of CRLM.
Tumour Viability and Response
Chemotherapy and other systemic treatments, such as immunotherapy, are used in the palliative and neoadjuvant settings. Pre-treatment, proportions of viable and necrotic tumour occurs as a result of intralesional hypoxia and ischaemia as tumour growth outstrips angiogenesis or vascular co-option, and has not been found to be prognostically relevant (34, 57). However, markers suggesting reduction of viable tumour following treatment are useful indicators with a strong prognostic value (34, 57). Here imaging has an important advantage over histopathological assessment of CRLM; the ability to evaluate tumour response before and after treatment. Since the features of chemotherapy response, namely fibrosis and necrosis, are present in chemotherapy-naive metastases, evaluation of response without a baseline assessment becomes more challenging.
The method of histological tumour regression grading described by Rubbia-Brandt et al (34) is the most widely used for determining histological response to chemotherapy; it categorises tumour response according to the balance of fibrosis and residual tumour. This method has been adapted from response grading of other tumours such as rectal and oesophageal tumours (58). Imaging response evaluation using the widely adopted RECIST 1.1 criteria, is based on changes in overall lesion size without taking into account changes in tissue composition (59). This is a limitation of the RECIST 1.1 criteria as tumours can demonstrate a reduction in cellularity without a reduction in size (42) (e.g. Figures 4–6), and some tumours have a low proportion of viable tumour at the outset, limiting the reliability of RECIST assessment (60–62), and its prognostic value. A combined assessment of the viable tumour volume, using both lesion size and tissue characterization may improve treatment assessment, and is already used in tumours such as HCC (63). Ideally automated lesion segmentation, subsegmentation (64) and feature analysis would integrate this process into the imaging pathway.
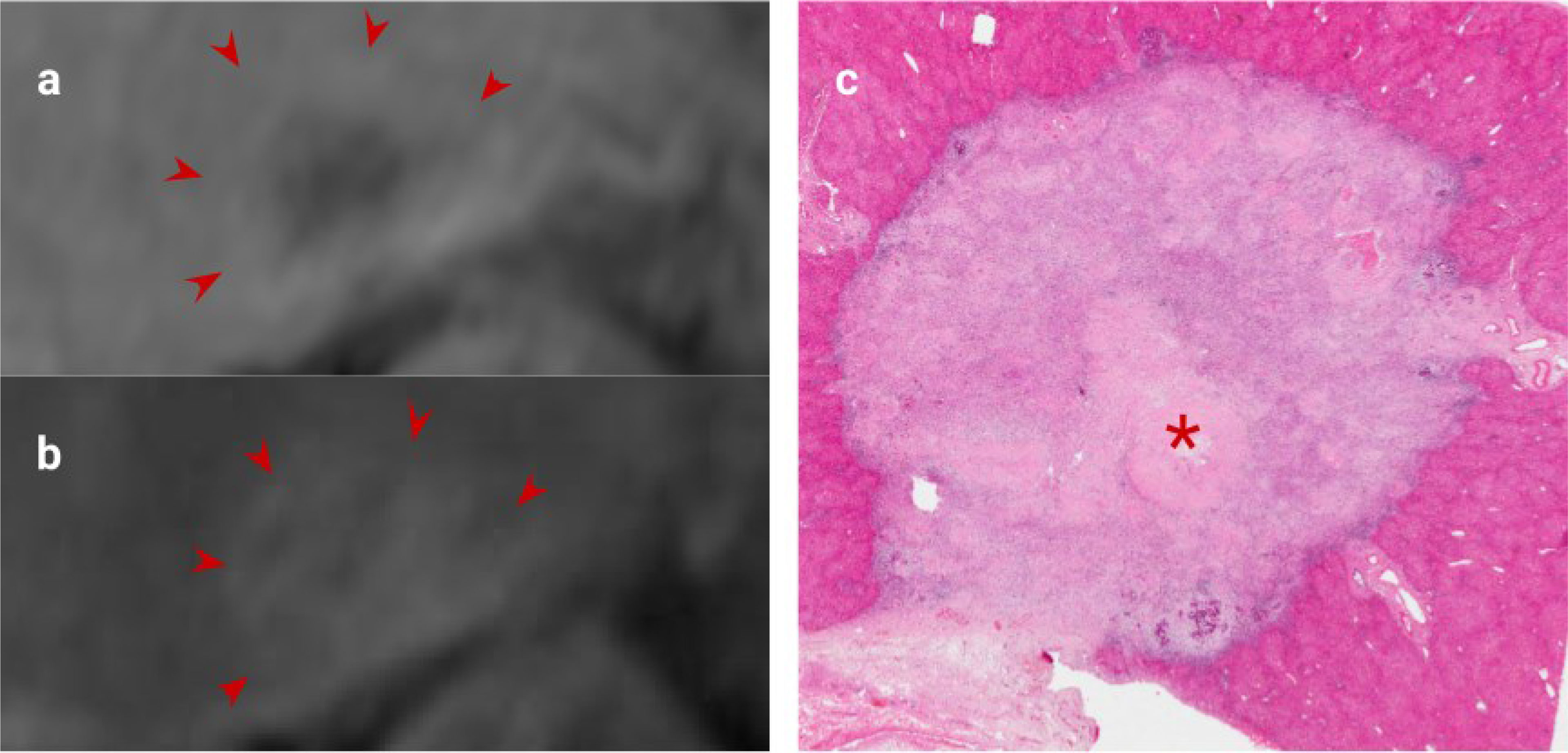
Figure 4 CRLM imaging features following excellent response to Chemotherapy. (A). Portal venous phase gadolinium-enhanced T1 fat saturated MRI showing decreased enhancement relative to liver (arrows), (B). Delayed phase MRI showing increased enhancement relative to liver (arrows), (C). H&E staining of this CRLM confirming almost complete fibrosis of the lesion indicating excellent chemotherapy response. '*' represents the metastasis of interest.
There is already evidence to support using morphological features to assess response of CRLM to chemotherapy. Lesion fibrosis, demonstrated as late gadolinium enhancement, which is the principle marker of response on pathological assessment, is a feature strongly linked to improved survival outcomes (Figure 4) (26, 42, 65). Similarly, CT/MRI morphological changes (illustrated in Figures 5 and 6) are better predictors of survival than RECIST [e.g (66–69)]. Adjuncts and alternatives to RECIST, including DWI and FDG-PET response evaluation have been investigated, but are not routinely deployed in clinical practice.
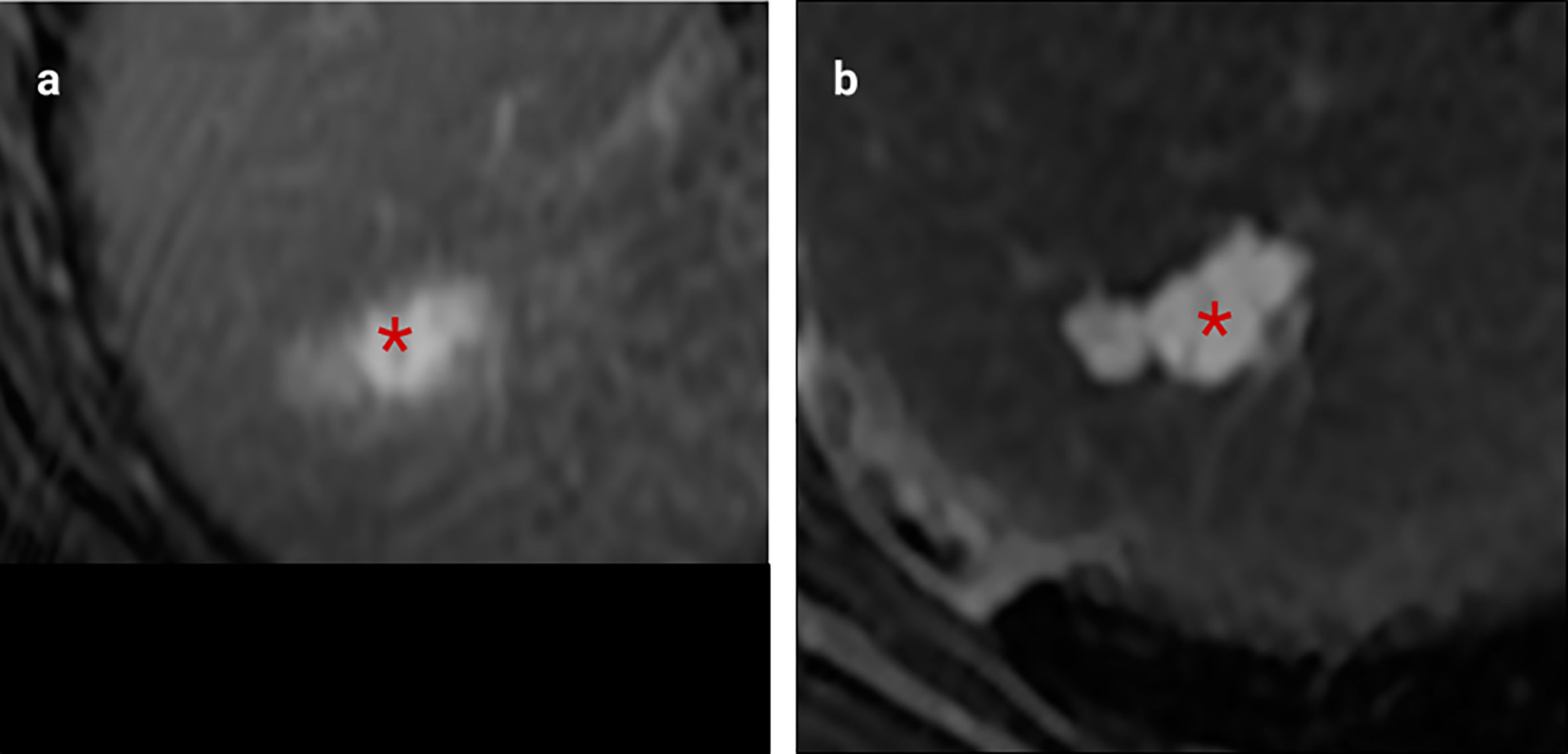
Figure 5 Example of excellent morphological response post-chemotherapy. Both images were obtained using the same MRI scanner performed at 1.5T (GE HDX Twinspeed MR scanner; GE, Milkwaukee, WI) with an 8-channel torso coil. (A) T2 weighted MRI showing a poorly defined CRLM with high T2 region of mucin (asterisk). (B). Following neoadjuvant treatment, this lesion demonstrates no change in size but now has a well-defined margin and replacement of intermediate signal cellular tumour with mucin, indicative of a good morphological response (asterisk). There was a complete pathological response at resection.
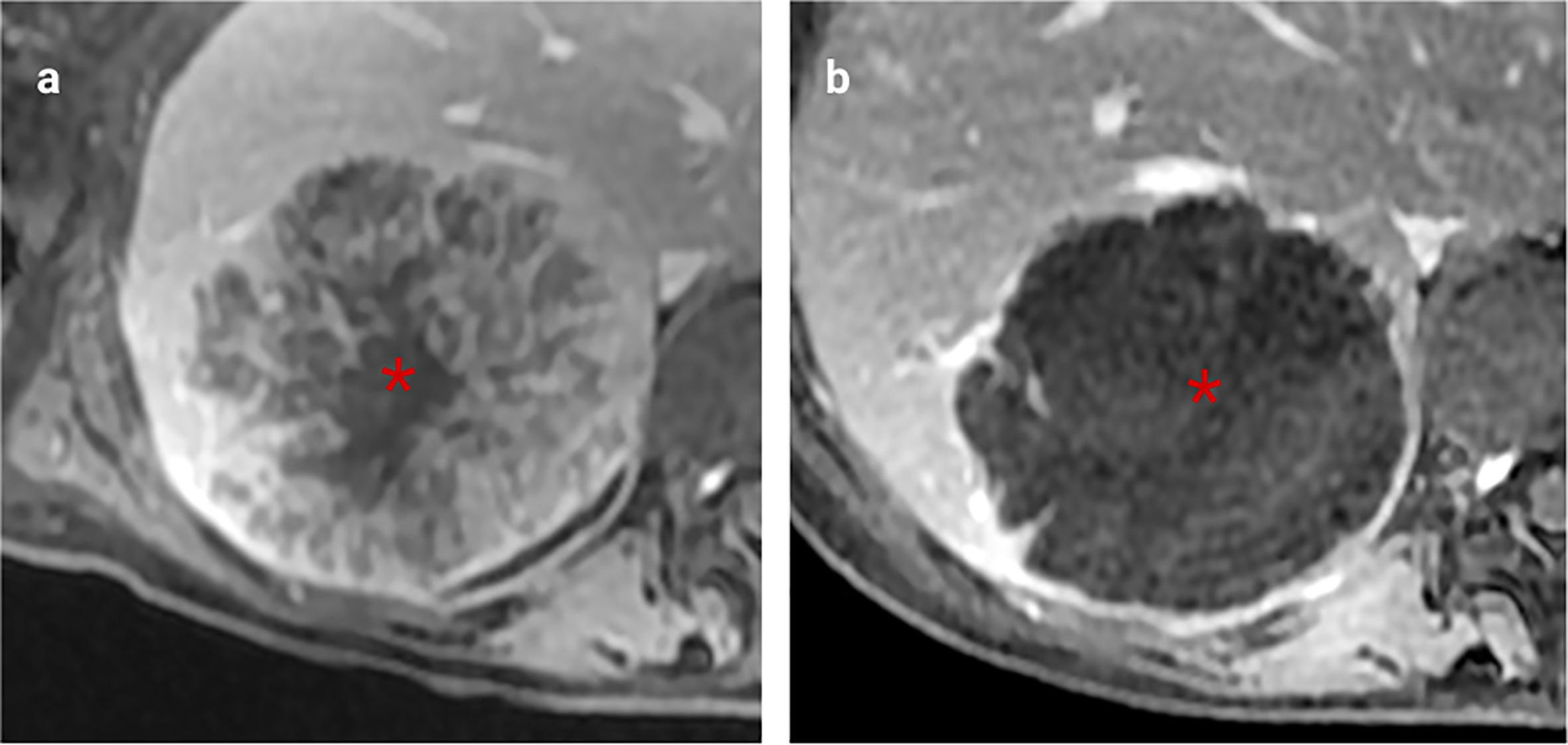
Figure 6 Excellent morphological response to chemotherapy. (A). Heterogenously enhancing CRLM on portal venous phase T1-weighted MRI (asterisk). (B), Post-chemotherapy MRI shows the lesion has become homogenous without a reduction in size, indicating a significant decrease in viable tumour cells.
Towards Comprehensive Imaging Characterization of CRLM: Opportunities and Challenges
The major benefit of imaging is the capacity to perform repeated, in vivo assessment of multisite disease, which is a particular advantage in the metastatic setting. In this article, we have described the basis for more complete morphological characterization of CRLM (22). A further strength of imaging is the potential to perform multiparametric imaging, including functional imaging techniques. Functional imaging allows physiological evaluation of tumours, and can aid determination of histological features, and support treatment selection and response. For example, assessment of angiogenesis and microvascular density is possible by diffusion weighted imaging (DWI) (53) and functional vascular assessment by DCE-MRI (54, 55). These techniques could also be used in identifying molecular subtypes of CRLM. Response to antiangiogenic treatment can be monitored with DCE-MRI (54, 70) and this can improve treatment stratification in clinical practice and trials.
Molecular imaging techniques, in particular PET/CT, can provide further functional assessments of CRLM. FDG-PET is well-established in oncological practice, and routinely acquired in staging oligometastatic CRC, principally for detecting disease that would be beyond the scope of local therapy. However, FDG-uptake can also be used as marker of hypoxia, and as a prognostic marker and for response evaluation (71). The potential applications for novel molecular agents to image specific disease features and process are diverse, with hypoxia imaging agents such as 18F-FMISO (72) and antibody-based imaging for CEA (73) are under investigation in the research setting.
However, despite the potential for multiparametric structural, functional and molecular imaging to provide a more comprehensive assessment of CRLM, there are limitations. While these techniques might be valuable, and are often integrated into trials, complex multimodality assessments are challenging in the clinical workflow, and reliably and repeatedly combining information from multiparametric imaging is difficult for human observers. A second major technical challenge to the use of imaging for assessing tissue features of CRLM is the achievable spatial resolution. For MRI, the in-plane spatial resolution is typically in the region of 1mm2. Although higher spatial resolutions can be achieved, an important feature of liver imaging is managing respiratory and, to a lesser extent, cardiac motion, which limits acquisition time and spatial resolution achievable in the upper abdomen. This issue is compounded through tissue features which can be substantially smaller than the imaging resolution, which may preclude accurate assessment of some features and place a limit on the achievable performance of imaging.
These two key challenges may be overcome by developments in image acquisition and analysis techniques. MRI acquisition will increasingly use computer-assisted techniques to decrease acquisition time and improve image resolution, for example through the use of ‘super-resolution’ techniques, which may improve the potential for tissue feature assessment by imaging (74). Secondly, improved motion correction and co-registration techniques can help overcome issues with between-acquisition motion (75).
The most promising opportunity within radiology is the incorporation of machine learning in image interpretation. Analysis of tumour features has historically relied on expert radiological assessment of imaging features. However, modern radiomic image analysis can be used to extract high-dimensional data from medical imaging (76), and machine learning techniques can be used for both automated segmentation (77) and feature analysis (78) to produce imaging biomarkers from medical imaging. These analysis strategies can more easily combine multiparametric imaging than a human observer, will remove observer variability, and can become an automated component of the image interpretation pipeline, which would be critical for clinical implementation in patient care.
Several key hurdles must be overcome before radiomics and machine learning becomes robust enough to influence patient care in daily clinical practice. Firstly, many radiomics and machine learning studies on CRLM have been conducted on relatively small datasets (42, 66, 76, 78). Studies conducted on small datasets therefore are at put a Radiomic algorithm at risk of ‘overfitting’ the data (creating an algorithm too specific to a limited pool of data), reducing the generalisability of the study findings (79, 80). The scientific community needs large annotated databases to derive and validate image analysis tools, however the practicality and ethics of sharing scans across multiple institutions, acquired through different and evolving techniques, is an ongoing challenge (79). Aside from acquiring larger, accurately labelled datasets, development of advanced radiomic techniques hold promise for minimising this issue (including various unsupervised clustering methods), but they are unlikely to be the solution without improved sources of data (79, 81, 82).
Even assuming a robust algorithm and analysis platform is developed in the research setting, adapting them into a convenient final product for use in the routine clinical workflow is a further challenge. In addition, many radiomic techniques are time-consuming from an operator perspective with careful lesion contouring required (76, 81). Automated segmentation technologies and other assisted analysis tools will be crucial to ensure workflow ensure clinical adoption.
An important consideration for imaging biomarkers in cancer is the development of alternative techniques for in vivo tumour assessment, in particular circulating tumour biomarkers such as circulating tumour DNA (83). However, as these tissues do not allow spatial localization of tumours, it is likely, particularly in the metastatic setting, that imaging can provide complementary information, and the combination of technologies can offer a more comprehensive toolkit to accurately phenotype disease.
Conclusion
This review has highlighted the potential for advanced imaging to provide in vivo characterization of clinically relevant histopathological features of CRLM. We have outlined the imaging findings of these histopathological features, focusing on the tumour-liver interface, intralesional component analysis, and morphological response assessment following systemic or liver-directed treatment. In vivo assessment of the tumour-liver interface has the potential to play and important role in defining the surgical approach and chemotherapy selection. As well as improving our characterisation of response to chemotherapy, imaging analysis of internal tumour components could play an increasingly important role as predictors of CRLM molecular subtypes. However, in the absence of studies providing robust validation of imaging techniques to report these features in practice, our assessment of CRLM by imaging is limited to documenting their size, number and location.
Advanced analysis methods, such as radiomics and machine learning, will be crucial tools in developing and validating novel imaging biomarkers for CRLM. However, these rely on curated and annotated datasets of sufficient size to build reliable algorithms, which is likely to require the cross-institutional collaborations that have been achieved in pathology and molecular biology.
Author Contributions
DM was involved with literature review, and writing and editing the manuscript. MT was involved with literature review, and writing and editing the manuscript. FG was involved with writing and editing the manuscript. DB was involved with writing and editing the manuscript. RG was involved with writing and editing the manuscript. JP was involved with writing and editing the manuscript. AH was involved with writing and editing the manuscript. JF was involved with literature review, writing and editing the manuscript. All authors contributed to the article and approved the submitted version.
Conflict of Interest
The authors declare that the research was conducted in the absence of any commercial or financial relationships that could be construed as a potential conflict of interest.
Publisher’s Note
All claims expressed in this article are solely those of the authors and do not necessarily represent those of their affiliated organizations, or those of the publisher, the editors and the reviewers. Any product that may be evaluated in this article, or claim that may be made by its manufacturer, is not guaranteed or endorsed by the publisher.
References
1. Bray F, Jemal A, Grey N, Ferlay J, Forman D. Global Cancer Transitions According to the Human Development Index (2008-2030): A Population-Based Study. Lancet Oncol (2012) 13(8):790–801. doi: 10.1016/S1470-2045(12)70211-5
2. Manfredi S, Lepage C, Hatem C, Coatmeur O, Faivre J, Bouvier A-M. Epidemiology and Management of Liver Metastases From Colorectal Cancer. Ann Surg (2006) 244(2):254–9. doi: 10.1097/01.sla.0000217629.94941.cf
3. Hellman S, Weichselbaum RR. Oligometastases. J Clin Oncol (1995) 13:8–10. doi: 10.1200/jco.1995.13.1.8
4. Kansas GP, Taylor A, Primrose JN, Langeberg W, Kelsh MA, Mowat F, et al. Survival After Liver Resection in Metastatic Colorectal Cancer: Review and Meta-Analysis of Prognostic Factors. Clin Epidemiol (2012) 4(1):283–301. doi: 10.2147/clep.s34285
5. Adam R, Wicherts DA, de Haas RJ, Ciacio O, Lévi F, Paule B, et al. Patients With Initially Unresectable Colorectal Liver Metastases: Is There a Possibility of Cure? J Clin Oncol (2009) 27:1829–35. doi: 10.1200/jco.2008.19.9273
6. Gomez D, Sangha VK, Morris-Stiff G, Malik HZ, Guthrie AJ, Toogood GJ, et al. Outcomes of Intensive Surveillance After Resection of Hepatic Colorectal Metastases. Br J Surg (2010) 97:1552–60. doi: 10.1002/bjs.7136
7. Van Cutsem E, Cervantes A, Adam R, Sobrero A, Van Krieken JH, Aderka D, et al. ESMO Consensus Guidelines for the Management of Patients With Metastatic Colorectal Cancer. Ann Oncol (2016) 27(8):1386–422. doi: 10.1093/annonc/mdw235
8. Bridgewater JA, Pugh SA, Maishman T, Eminton Z, Mellor J, Whitehead A, et al. Systemic Chemotherapy With or Without Cetuximab in Patients With Resectable Colorectal Liver Metastasis (New EPOC): Long-Term Results of a Multicentre, Randomised, Controlled, Phase 3 Trial. Lancet Oncol (2020) 21(3):398–411. doi: 10.1016/S1470-2045(19)30798-3
9. Vermeulen PB, Colpaert C, Salgado R, Royers R, Hellemans H, Van den Heuvel E, et al. Liver Metastases From Colorectal Adenocarcinomas Grow in Three Patterns With Different Angiogenesis and Desmoplasia [Internet]. Vol 195 J Pathol (2001) 195(3):336–42. doi: 10.1002/path.966
10. Knijn N, de Ridder JAM, Punt CJA, de Wilt JHW, Nagtegaal ID. Histopathological Evaluation of Resected Colorectal Cancer Liver Metastases: What Should be Done? Histopathology (2013) 63(2):149–56. doi: 10.1111/his.12124
11. Pitroda SP, Khodarev NN, Huang L, Uppal A, Wightman SC, Ganai S, et al. Integrated Molecular Subtyping Defines a Curable Oligometastatic State in Colorectal Liver Metastasis. Nat Commun (2018) 9. doi: 10.1038/s41467-018-04278-6
12. Franklin JM, Sharma RA, Harris AL, Gleeson FV. Imaging Oligometastatic Cancer Before Local Treatment. Lancet Oncol (2016) 17(9):e406–14. doi: 10.1016/S1470-2045(16)30277-7
13. Niekel MC, Bipat S, Stoker J. Diagnostic Imaging of Colorectal Liver Metastases With CT, MR Imaging, FDG PET, and/or FDG PET/CT: A Meta-Analysis of Prospective Studies Including Patients Who Have Not Previously Undergone Treatment. Radiology (2010) 257(3):674–84. doi: 10.1148/radiol.10100729
14. Mainenti PP, Stanzione A, Guarino S, Romeo V, Ugga L, Romano F, et al. Colorectal Cancer: Parametric Evaluation of Morphological, Functional and Molecular Tomographic Imaging. World J Gastroenterol (2019) 25(35):5233–56. doi: 10.3748/wjg.v25.i35.5233
15. Adams RB, Aloia TA, Loyer E, Pawlik TM, Taouli B, Vauthey J-N, et al. Selection for Hepatic Resection of Colorectal Liver Metastases: Expert Consensus Statement. HPB (2013) 15(2):91–103. doi: 10.1111/j.1477-2574.2012.00557.x
16. Maffione AM, Lopci E, Bluemel C, Giammarile F, Herrmann K, Rubello D. Diagnostic Accuracy and Impact on Management of (18)F-FDG PET and PET/CT in Colorectal Liver Metastasis: A Meta-Analysis and Systematic Review. Eur J Nucl Med Mol Imaging (2015) 42(1):152–63. doi: 10.1007/s00259-014-2930-4
17. Lazaris A, Amri A, Petrillo SK, Zoroquiain P, Ibrahim N, Salman A, et al. Vascularization of Colorectal Carcinoma Liver Metastasis: Insight Into Stratification of Patients for Anti-Angiogenic Therapies. Hip Int (2018) 4(3):184–92. doi: 10.1002/cjp2.100
18. Frentzas S, Simoneau E, Bridgeman VL, Vermeulen PB, Foo S, Kostaras E, et al. Vessel Co-Option Mediates Resistance to Anti-Angiogenic Therapy in Liver Metastases. Nat Med (2016) 22(11):1294–302. doi: 10.1038/nm.4197
19. Oliveira RC, Alexandrino H, Cipriano MA, Tralhão JG. Liver Metastases and Histological Growth Patterns: Biological Behavior and Potential Clinical Implications-Another Path to Individualized Medicine? J Oncol (2019) 2019:6280347. doi: 10.1155/2019/6280347
20. Eefsen RL, Vermeulen PB, Christensen IJ, Laerum OD, Mogensen MB, Rolff HC, et al. Growth Pattern of Colorectal Liver Metastasis as a Marker of Recurrence Risk. Clin Exp Metastasis (2015) 32(4):369–81. doi: 10.1007/s10585-015-9715-4
21. Pinheiro RS, Herman P, Lupinacci RM, Lai Q, Mello ES, Coelho FF, et al. Tumor Growth Pattern as Predictor of Colorectal Liver Metastasis Recurrence. Am J Surg (2014) 207:493–8. doi: 10.1016/j.amjsurg.2013.05.015
22. Paulatto L, Burgio MD, Sartoris R, Beaufrère A, Cauchy F, Paradis V, et al. Colorectal Liver Metastases: Radiopathological Correlation. Insights Into Imaging (2020) 11. doi: 10.1186/s13244-020-00904-4
23. Siriwardana PN, Luong TV, Watkins J, Turley H, Ghazaley M, Gatter K, et al. Biological and Prognostic Significance of the Morphological Types and Vascular Patterns in Colorectal Liver Metastases (CRLM). Medicine (2016) 95:e2924. doi: 10.1097/md.0000000000002924
24. Farace P, Merigo F, Fiorini S, Nicolato E, Tambalo S, Daducci A, et al. DCE-MRI Using Small-Molecular and Albumin-Binding Contrast Agents in Experimental Carcinomas With Different Stromal Content. Eur J Radiol (2011) 78(1):52–9. doi: 10.1016/j.ejrad.2009.04.043
25. Chen P, Wang Y, Fang X, Wang X, Wang G. Prognostic Significance of Peritumoral Fibrosis After Resection of Pancreatic Head Cancer. Oncol Lett (2020) 19(2):1235–40. doi: 10.3892/ol.2019.11181
26. Cheung HMC, Karanicolas PJ, Hsieh E, Coburn N, Maraj T, Kim JK, et al. Late Gadolinium Enhancement of Colorectal Liver Metastases Post-Chemotherapy Is Associated With Tumour Fibrosis and Overall Survival Post-Hepatectomy. Eur Radiol (2018) 28:3505–12. doi: 10.1007/s00330-018-5331-4
27. Outwater E, Tomaszewski JE, Daly JM, Kressel HY. Hepatic Colorectal Metastases: Correlation of MR Imaging and Pathologic Appearance. Radiol (1991) 180(2):327–32. doi: 10.1148/radiology.180.2.2068294
28. Lee S, Kim SH, Lee JE, Sinn DH, Park CK. Preoperative Gadoxetic Acid-Enhanced MRI for Predicting Microvascular Invasion in Patients With Single Hepatocellular Carcinoma. J Hepatol (2017) 67(3):526–34. doi: 10.1016/j.jhep.2017.04.024
29. Hayashi M, Inoue Y, Komeda K, Shimizu T, Asakuma M, Hirokawa F, et al. Clinicopathological Analysis of Recurrence Patterns and Prognostic Factors for Survival After Hepatectomy for Colorectal Liver Metastasis. BMC Surg (2010) 10:27. doi: 10.1186/1471-2482-10-27
30. Brown G, Radcliffe AG, Newcombe RG, Dallimore NS, Bourne MW, Williams GT. Preoperative Assessment of Prognostic Factors in Rectal Cancer Using High-Resolution Magnetic Resonance Imaging. Br J Surg (2003) 90:355–64. doi: 10.1002/bjs.4034
31. Shirabe K, Takenaka K, Gion T, Fujiwara Y, Shimada M, Yanaga K, et al. Analysis of Prognostic Risk Factors in Hepatic Resection for Metastatic Colorectal Carcinoma With Special Reference to the Surgical Margin. Br J Surg (1997) 84(8):1077–80. doi: 10.1002/BJS.1800840810
32. Sasaki A, Aramaki M, Kawano K, Yasuda K, Inomata M, Kitano S. Prognostic Significance of Intrahepatic Lymphatic Invasion in Patients With Hepatic Resection Due to Metastases From Colorectal Carcinoma. Cancer (2002) 95(1):105–11. doi: 10.1002/cncr.10655
33. Lupinacci RM, Mello ES, Pinheiro RS, Marques G, Coelho FF, Kruger JAP, et al. Intrahepatic Lymphatic Invasion But Not Vascular Invasion is a Major Prognostic Factor After Resection of Colorectal Cancer Liver Metastases. World J Surg (2014) 38:2089–96. doi: 10.1007/s00268-014-2511-5
34. Rubbia-Brandt L, Giostra E, Brezault C, Roth AD, Andres A, Audard V, et al. Importance of Histological Tumor Response Assessment in Predicting the Outcome in Patients With Colorectal Liver Metastases Treated With Neo-Adjuvant Chemotherapy Followed by Liver Surgery. Ann Oncol (2007) 18(2):299–304. doi: 10.1093/annonc/mdl386
35. Eefsen RL, Engelholm L, Willemoe GL, Van den Eynden GG, Laerum OD, Christensen IJ, et al. Microvessel Density and Endothelial Cell Proliferation Levels in Colorectal Liver Metastases From Patients Given Neo-Adjuvant Cytotoxic Chemotherapy and Bevacizumab. Int J Cancer (2016) 138(7):1777–84. doi: 10.1002/ijc.29904
36. Milot L, Guindi M, Gallinger S, Moulton CA, Brock KK, Dawson LA, et al. MR Imaging Correlates of Intratumoral Tissue Types Within Colorectal Liver Metastases: A High-Spatial-Resolution Fresh Ex Vivo Radiologic-Pathologic Correlation Study. Radiol (2010) 254(3):747–54. doi: 10.1148/radiol.09090508
37. Colagrande S, Castellani A, Nardi C, Lorini C, Calistri L, Filippone A. The Role of Diffusion-Weighted Imaging in the Detection of Hepatic Metastases From Colorectal Cancer: A Comparison With Unenhanced and Gd-EOB-DTPA Enhanced MRI. Eur J Radiol (2016) 85(5):1027–34. doi: 10.1016/j.ejrad.2016.02.011
38. Geschwind J-FH, Artemov D, Abraham S, Omdal D, Huncharek MS, McGee C, et al. Chemoembolization of Liver Tumor in a Rabbit Model: Assessment of Tumor Cell Death With Diffusion-Weighted MR Imaging and Histologic Analysis. J Vasc Interventional Radiol (2000) 11:1245–55. doi: 10.1016/s1051-0443(07)61299-8
39. Sica GT, Ji H, Ros PR. CT and MR Imaging of Hepatic Metastases. Am J Roentgenol (2000) 174:691–8. doi: 10.2214/ajr.174.3.1740691
40. Koh TS, Thng CH, Hartono S, Dominguez LTM, Lim TKH, Huynh H, et al. Assessment of Tumor Necrotic Fraction by Dynamic Contrast-Enhanced MRI: A Preclinical Study of Human Tumor Xenografts With Histopathologic Correlation. NMR Biomed (2014) 27(4):486–94. doi: 10.1002/nbm.3090
41. Chiaradia M, Baranes L, Van Nhieu JT, Vignaud A, Laurent A, Decaens T, et al. Intravoxel Incoherent Motion (IVIM) MR Imaging of Colorectal Liver Metastases: Are We Only Looking at Tumor Necrosis? J Magnetic Resonance Imaging (2014) 39:317–25. doi: 10.1002/jmri.24172
42. Shur J, Orton M, Connor A, Fischer S, Moulton C, Gallinger S, et al. A Clinical-Radiomic Model for Improved Prognostication of Surgical Candidates With Colorectal Liver Metastases. J Surg Oncol (2020) 121:357–64. doi: 10.1002/jso.25783
43. Murata S, Matsushima S, Sato Y, Yamaura H, Kato M, Hasegawa T, et al. Predicting Chemotherapeutic Response for Colorectal Liver Metastases Using Relative Tumor Enhancement of Gadoxetic Acid Disodium-Enhanced Magnetic Resonance Imaging. Abdom Radiol (NY) (2018) 43(12):3301–6. doi: 10.1007/s00261-018-1615-z
44. Wagner M, Doblas S, Daire J-L, Paradis V, Haddad N, Leitão H, et al. Diffusion-Weighted MR Imaging for the Regional Characterization of Liver Tumors. Radiol (2012) 264(2):464–72. doi: 10.1148/radiol.12111530
45. Scurr ED, Collins DJ, Temple L, Karanjia N, Leach MO, Koh D-M. Appearances of Colorectal Hepatic Metastases at Diffusion-Weighted MRI Compared With Histopathology: Initial Observations. Br J Radiol (2012) 85(1011):225–30. doi: 10.1259/bjr/11597735
46. Lee JE, Kim SH, Lee S, Choi S-Y, Hwang JA, Woo S-Y. Differentiating Metastatic Mucinous Colorectal Adenocarcinomas From Simple Cysts of the Liver Using Contrast-Enhanced and Diffusion-Weighted MRI. Br J Radiol (2018) 91:20180303. doi: 10.1259/bjr.20180303
47. Cheung HMC, Karanicolas PJ, Coburn N, Seth V, Law C, Milot L. Delayed Tumour Enhancement on Gadoxetate-Enhanced MRI Is Associated With Overall Survival in Patients With Colorectal Liver Metastases. Eur Radiol (2019) 29(2):1032–8. doi: 10.1007/s00330-018-5618-5
48. Lau YC, Schmeier S, Frizelle F, Purcell R. Consensus Molecular Subtypes of Primary Colon Tumors and Their Hepatic Metastases. Future Sci OA (2021) 7(7):FSO722. doi: 10.2144/fsoa-2021-0021
49. Choi JY, Choi S, Lee M, Park YS, Sung JS, Chang WJ, et al. Clinical Implication of Concordant or Discordant Genomic Profiling Between Primary and Matched Metastatic Tissues in Patients With Colorectal Cancer. Cancer Res Treat (2020) 52(3):764–78. doi: 10.4143/crt.2020.044
50. Zou Y, Hu X, Zheng S, Yang A, Li X, Tang H, et al. Discordance of Immunotherapy Response Predictive Biomarkers Between Primary Lesions and Paired Metastases in Tumours: A Systematic Review and Meta-Analysis. EBioMed (2021) 63:103137. doi: 10.1016/j.ebiom.2020.103137
51. Stintzing S, Wirapati P, Lenz H-J, Neureiter D, Fischer von Weikersthal L, Decker T, et al. Consensus Molecular Subgroups (CMS) of Colorectal Cancer (CRC) and First-Line Efficacy of FOLFIRI Plus Cetuximab or Bevacizumab in the FIRE3 (AIO KRK-0306) Trial. Ann Oncol (2019) 30(11):1796–803. doi: 10.1093/annonc/mdz387
52. Guinney J, Dienstmann R, Wang X, de Reyniès A, Schlicker A, Soneson C, et al. The Consensus Molecular Subtypes of Colorectal Cancer. Nat Med (2015) 21(11):1350–6. doi: 10.1038/nm.3967
53. Ullrich RT, Jikeli JF, Diedenhofen M, Böhm-Sturm P, Unruh M, Vollmar S, et al. In-Vivo Visualization of Tumor Microvessel Density and Response to Anti-Angiogenic Treatment by High Resolution MRI in Mice. PloS One (2011) 6:e19592. doi: 10.1371/journal.pone.0019592
54. Morgan B, Thomas AL, Drevs J, Hennig J, Buchert M, Jivan A, et al. Dynamic Contrast-Enhanced Magnetic Resonance Imaging as a Biomarker for the Pharmacological Response of PTK787/ZK 222584, an Inhibitor of the Vascular Endothelial Growth Factor Receptor Tyrosine Kinases, in Patients With Advanced Colorectal Cancer and Liver Metastases: Results From Two Phase I Studies. J Clin Oncol (2003) 21(21):3955–64. doi: 10.1200/JCO.2003.08.092
55. De Bruyne S, Van Damme N, Smeets P, Ferdinande L, Ceelen W, Mertens J, et al. Value of DCE-MRI and FDG-PET/CT in the Prediction of Response to Preoperative Chemotherapy With Bevacizumab for Colorectal Liver Metastases. Br J Cancer (2012) 106(12):1926–33. doi: 10.1038/bjc.2012.184
56. Sirinukunwattana K, Domingo E, Richman SD, Redmond KL, Blake A, Verrill C, et al. Image-Based Consensus Molecular Subtype (imCMS) Classification of Colorectal Cancer Using Deep Learning. Gut (2021) 70(3):544–54. doi: 10.1136/gutjnl-2019-319866
57. Serrablo A, Paliogiannis P, Pulighe F, Moro SS-M, Borrego-Estella V, Attene F, et al. Impact of Novel Histopathological Factors on the Outcomes of Liver Surgery for Colorectal Cancer Metastases. Eur J Surg Oncol (2016) 42(9):1268–77. doi: 10.1016/j.ejso.2016.02.013
58. Mandard AM, Dalibard F, Mandard JC, Marnay J, Henry-Amar M, Petiot JF, et al. Pathologic Assessment of Tumor Regression After Preoperative Chemoradiotherapy of Esophageal Carcinoma. Clinicopathologic Correlations. Cancer (1994) 73(11):2680–6. doi: 10.1002/1097-0142(19940601)73:11<2680::aid-cncr2820731105>3.0.co;2-c
59. Eisenhauer EA, Therasse P, Bogaerts J, Schwartz LH, Sargent D, Ford R, et al. New Response Evaluation Criteria in Solid Tumours: Revised RECIST Guideline (Version 1.1). Eur J Cancer (2009) 45(2):228–47. doi: 10.1016/j.ejca.2008.10.026
60. Lohith G. Radiomic Imaging Analysis-To Renovate RECIST Criteria? Cancer Ther Oncol Int J (2017) 6. doi: 10.19080/ctoij.2017.06.555679
61. Villaruz LC, Socinski MA. The Clinical Viewpoint: Definitions, Limitations of RECIST, Practical Considerations of Measurement [Internet]. Vol 19 Clin Cancer Res (2013) p:2629–36. doi: 10.1158/1078-0432.ccr-12-2935
62. Barnacle AM, McHugh K. Limitations With the Response Evaluation Criteria in Solid Tumors (RECIST) Guidance in Disseminated Pediatric Malignancy. Pediatr Blood Cancer (2006) 46:127–34. doi: 10.1002/pbc.20344
63. Choi J-Y, Lee J-M, Sirlin CB. CT and MR Imaging Diagnosis and Staging of Hepatocellular Carcinoma: Part I. Development, Growth, and Spread: Key Pathologic and Imaging Aspects. Radiology (2014) 272:635–54. doi: 10.1148/radiol.14132361
64. Franklin JM, Irving B, Papiez BW, Kallehauge JF, Wang LM, Goldin RD, et al. Tumour Subregion Analysis of Colorectal Liver Metastases Using Semi-Automated Clustering Based on DCE-MRI: Comparison With Histological Subregions and Impact on Pharmacokinetic Parameter Analysis. Eur J Radiol (2020) 126:108934. doi: 10.1016/j.ejrad.2020.108934
65. Cheung HMC, Kim JK, Hudson J, Coburn N, Karanicolas PJ, Law C, et al. Late Gadolinium MRI Enhancement of Colorectal Liver Metastases Is Associated With Overall Survival Among Nonsurgical Patients. Eur Radiol (2019) 29:3901–7. doi: 10.1007/s00330-019-06177-w
66. Dohan A, Gallix B, Guiu B, Le Malicot K, Reinhold C, Soyer P, et al. Early Evaluation Using a Radiomic Signature of Unresectable Hepatic Metastases to Predict Outcome in Patients With Colorectal Cancer Treated With FOLFIRI and Bevacizumab. Gut (2020) 69(3):531–9. doi: 10.1136/gutjnl-2018-316407
67. Mazard T, Boonsirikamchai P, Overman MJ, Asran MA, Choi H, Herron D, et al. Comparison of Early Radiological Predictors of Outcome in Patients With Colorectal Cancer With Unresectable Hepatic Metastases Treated With Bevacizumab. Gut (2018) 67(6):1095–102. doi: 10.1136/gutjnl-2017-313786
68. Chun YS. Association of Computed Tomography Morphologic Criteria With Pathologic Response and Survival in Patients Treated With Bevacizumab for Colorectal Liver Metastases. Jama (2009) 302:2338. doi: 10.1001/jama.2009.1755
69. Boonsirikamchai P, Asran MA, Maru DM, Vauthey J-N, Kaur H, Kopetz S, et al. CT Findings of Response and Recurrence, Independent of Change in Tumor Size, in Colorectal Liver Metastasis Treated With Bevacizumab. Am J Roentgenol (2011) 197:W1060–6. doi: 10.2214/ajr.11.6459
70. Khan M, Loree JM, Advani SM, Ning J, Li W, Pereira AAL, et al. Prognostic Implications of Mucinous Differentiation in Metastatic Colorectal Carcinoma Can Be Explained by Distinct Molecular and Clinicopathologic Characteristics. Clin Colorectal Cancer (2018) 17(4):e699–709. doi: 10.1016/j.clcc.2018.07.005
71. Xia Q, Liu J, Wu C, Song S, Tong L, Huang G, et al. Prognostic Significance of (18)FDG PET/CT in Colorectal Cancer Patients With Liver Metastases: A Meta-Analysis. Cancer Imaging (2015) 15:19. doi: 10.1186/s40644-015-0055-z
72. Lee ST, Muralidharan V, Tebbutt N, Wong P, Fang C, Liu Z, et al. Prevalence of Hypoxia and Correlation With Glycolytic Metabolism and Angiogenic Biomarkers in Metastatic Colorectal Carcinoma. Eur J Nucl Med Mol Imaging (2020) 48:1585–92. doi: 10.1007/s00259-020-05074-5
73. Hong H, Sun J, Cai W. Radionuclide-Based Cancer Imaging Targeting the Carcinoembryonic Antigen. Biomark Insights (2008) 3:435–51. doi: 10.4137/BMI.S1124
74. Yakkundi SV, Subha DP. Convolutional LSTM: A Deep Learning Approach for Dynamic MRI Reconstruction. 2020 4th Int Conf Trends Electron Inf (ICOEI) (48184) (2020) 48184:1011–5. doi: 10.1109/icoei48184.2020.9142982
75. Papież BW, Franklin JM, Heinrich MP, Gleeson FV, Brady M, Schnabel JA. GIFTed Demons: Deformable Image Registration With Local Structure-Preserving Regularization Using Supervoxels for Liver Applications. J Med Imaging (2018) 5:1. doi: 10.1117/1.jmi.5.2.024001
76. Aerts HJWL, Velazquez ER, Leijenaar RTH, Parmar C, Grossmann P, Carvalho S, et al. Decoding Tumour Phenotype by Noninvasive Imaging Using a Quantitative Radiomics Approach. Nat Commun (2014) 5:4006. doi: 10.1038/ncomms5006
77. Vorontsov E, Cerny M, Régnier P, Di Jorio L, Pal CJ, Lapointe R, et al. Deep Learning for Automated Segmentation of Liver Lesions at CT in Patients With Colorectal Cancer Liver Metastases. Radiology: Artif Intell (2019) 1:180014. doi: 10.1148/ryai.2019180014
78. Liang M, Cai Z, Zhang H, Huang C, Meng Y, Zhao L, et al. Machine Learning-Based Analysis of Rectal Cancer MRI Radiomics for Prediction of Metachronous Liver Metastasis. Acad Radiol (2019) 26(11):1495–504. doi: 10.1016/j.acra.2018.12.019
79. Sala E, Mema E, Himoto Y, Veeraraghavan H, Brenton JD, Snyder A, et al. Unravelling Tumour Heterogeneity Using Next-Generation Imaging: Radiomics, Radiogenomics, and Habitat Imaging. Clin Radiol (2017) 72(1):3–10. doi: 10.1016/j.crad.2016.09.013
80. Dietterich T. Overfitting and Undercomputing in Machine Learning. ACM Computing Surveys (1995) 27:326–7. doi: 10.1145/212094.212114
81. Chen J, Milot L, Cheung HMC, Martel AL. Unsupervised Clustering of Quantitative Imaging Phenotypes Using Autoencoder and Gaussian Mixture Model. Lect Notes Comput Sci (2019) 1:575–82. doi: 10.1007/978-3-030-32251-9_63
Keywords: colorectal (colon) cancer, liver, metastasis, radiomic biomarkers, MRI, computed tomography
Citation: Maclean D, Tsakok M, Gleeson F, Breen DJ, Goldin R, Primrose J, Harris A and Franklin J (2021) Comprehensive Imaging Characterization of Colorectal Liver Metastases. Front. Oncol. 11:730854. doi: 10.3389/fonc.2021.730854
Received: 25 June 2021; Accepted: 15 November 2021;
Published: 07 December 2021.
Edited by:
Joseph Ross Mitchell, Independent Researcher, Tampa, FL, United StatesReviewed by:
Jianan Chen, University of Toronto, CanadaKunal Bharat Gala, Tata Memorial Hospital, India
Copyright © 2021 Maclean, Tsakok, Gleeson, Breen, Goldin, Primrose, Harris and Franklin. This is an open-access article distributed under the terms of the Creative Commons Attribution License (CC BY). The use, distribution or reproduction in other forums is permitted, provided the original author(s) and the copyright owner(s) are credited and that the original publication in this journal is cited, in accordance with accepted academic practice. No use, distribution or reproduction is permitted which does not comply with these terms.
*Correspondence: Drew Maclean, ZGZ3bWFjbGVhbkBkb2N0b3JzLm9yZy51aw==