- 1Department of Oncology, Complejo Hospitalario Universitario de Orense, Orense, Spain
- 2Department of Oncology, Hospital Álvaro Cunqueiro, Complejo Hospitalario Universitario de Vigo, Pontevedra, Spain
- 3Translational Oncology Research Group (ONCO-INVES), Galicia Sur Health Research Institute (IIS Galicia Sur), SERGAS-UVIGO, Pontevedra, Spain
- 4Department of Oncology, Complejo Asistencial Universitario de Burgos, Burgos, Spain
- 5Department of Oncology, Hospital Clínico Universitario de Valladolid, Valladolid, Spain
- 6Department of Oncology, Hospital Universitario La Paz, Madrid, Spain
- 7Department of Oncology, Donostialdea ESI/OSI Donostialdea, Donostia, Unibertsitate Ospitalea/Hospital Universitario Donostia, Donostia, Spain
- 8Department of Oncology, Complejo Hospitalario Universitario Insular Materno-Infantil, Las Palmas de Gran Canaria, Spain
- 9Department of Oncology, Institut Català d’Oncologia, Badalona, Spain
- 10Department of Oncology, Hospital de Sagunto, Valencia, Spain
- 11Outcomes10, Castellón, Spain
- 12Bristol Myers Squibb, Madrid, Spain
Introduction: The purpose of this investigation was to explore patients’ and oncologists’ preferences for the characteristics of a pharmacological regimen for patients with advanced renal cell carcinoma (aRCC).
Material and Methods: Cross-sectional observational study based on a discrete choice experiment (DCE) conducted in Spain. A literature review, a focus group with oncologists and interviews with patients informed the DCE design. Five attributes were included: progression survival gain, risk of serious adverse events (SAEs), health-related quality of life (HRQoL), administration mode, and treatment cost. Preferences were analyzed using a mixed-logit model to estimate relative importance (RI) of attributes (importance of an attribute in relation to all others), which was compared between aRCC patients and oncologists treating aRCC. Willingness to pay (WTP, payer: health system) for a benefit in survival or in risk reduction and maximum acceptable risk (MAR) in SAEs for improving survival were estimated from the DCE. Subgroup analyses were performed to identify factors that influence preference.
Results: A total of 105 patients with aRCC (77.1% male, mean age 65.9 years [SD: 10.4], mean time since RCC diagnosis 6.3 years [SD: 6.1]) and 67 oncologists (52.2% male, mean age 41.9 years [SD: 8.4], mean duration of experience in RCC 10.2 years [SD: 7.5]) participated in the study. The most important attribute for patients and oncologists was survival gain (RI: 43.6% vs. 54.7% respectively, p<0.05), followed by HRQoL (RI: 35.5% vs. 18.0%, respectively, p<0.05). MAR for SAEs was higher among oncologists than patients, while WTP (for the health system) was higher for patients. Differences in preferences were found according to time since diagnosis and education level (patients) or length of professional experience (oncologists).
Conclusion: Patients’ and oncologists’ preferences for aRCC treatment are determined mainly by the efficacy (survival gain) but also by the HRQoL provided. The results of the study can help to inform decision-making in the selection of appropriate aRCC treatment.
Introduction
Renal cell carcinoma (RCC) is among the most common cancers (1), representing 2.3% of all adult malignancies worldwide (2). RCCs arise from a variety of specialized cells located along the length of the nephron, giving rise to the diversity of histologic RCC types (3). The most common type of RCC is clear-cell RCC, that accounts for up to 75% of RCCs (4).
About 65% of patients with RCC have localized tumors, which are generally treated with surgery and may be cured by total or partial nephrectomy (1, 4). However, the remaining ~35% of patients who present with advanced RCC (aRCC) (which can be partially resected or destructed by surgery) or patients who relapse after local therapy, typically require systemic treatment (1, 5, 6). Nonetheless, aRCC has usually been refractory to conventional cytotoxic chemotherapy (7). Historically, cytokines, known for their limited activity and poor tolerability (8), were the only option available for aRCC. Notably, the management of RCC has been revolutionized in recent years, with the availability of drugs that improve survival for patients with advanced disease dramatically broadening (9, 10).
Compared to cytokines, targeted anti-angiogenic agents have demonstrated superior antitumor activity (11, 12). In addition, aRCC patients treated with sequences of targeted therapies have achieved extended survival (13). Although these treatments have demonstrated a clear progression-free survival benefit, only a small proportion of patients achieve long-term survival, highlighting the need for other therapeutic options with novel mechanisms of action that could potentially result in improved survival (14, 15). The introduction of immune checkpoint inhibitors that alter the interaction between immune cells and antigen-presenting cells, including tumor cells, has changed the treatment landscape for refractory solid tumors (10, 16), and has demonstrated improved overall survival in advanced melanoma (17), non-small-cell lung cancer (18, 19), and aRCC (20). Furthermore, tyrosine kinase inhibitor/immune checkpoint inhibitor combinations appear to be an important treatment option for patients with newly diagnosed aRCC, having shown improved response and overall survival in the first-line setting (21).
Each of the treatments is associated with different clinical outcomes, administration modes, treatment-related adverse events, and costs (22), so decisions about therapy involve tradeoffs between the possible benefits and harms (9). The European Medicines Agency has indicated that in order to improve the management of treatment toxicities, it is important to better understand the extent to which patients are willing to tolerate AEs and, therefore, the relative importance patients give them (23). Additionally, aspects of patient comfort and preference are gaining greater attention when evaluating the best treatment concept for a patient in oncologic treatment (24). In this regard, it is unclear how the specific characteristics of recently approved immunotherapies such as mode and frequency of administration will affect patients and professionals’ preferences when choosing a treatment.
Most cancer patients favor joint decision-making with their doctor, rather than either party make a unilateral decision (25). Shared decision-making is an increasingly used model in medicine, which requires clinicians to understand their patients’ as well as their own preferences (26). For this purpose, there is a need to assess patients’ preferences (27) and enhance patient participation in the decision-making process (28, 29). A discrete choice experiment (DCE) is the stated preference survey format most commonly used to measure preferences for different healthcare interventions, offering a mechanism to facilitate shared decision-making between doctor and patient (30).
Limited evidence exists on which attributes of treatment regimens for aRCC are most important to patients and the extent to which the physician’s judgment matches the patient’s preferences (31–33). Thus, a better understanding of aRCC patients and oncologists’ treatment preferences could help to reduce the knowledge gap between them and consequently improve aRCC management and shared decision-making.
Materials and Methods
Study Design and Participants
This was an observational, multicenter, cross-sectional study conducted in Spain among patients with aRCC and oncologists.
Participating patients were recruited in nine Spanish hospitals by their physicians between August 2019 and July 2020. They were aged 18 or older; had been diagnosed with RCC at least two months prior to study inclusion; had aRCC at the time of the study; were receiving or had previously received pharmacological treatment for aRCC (the patient may have received different treatments); and were able to carry out the study tasks.
Oncologists practicing at Spanish public hospitals with more than two years’ experience in RCC management were identified by the sponsor and invited by e-mail to participate. The study questionnaire was available from July to August 2019.
The study was approved by the Ethics Committee of the Hospital General Universitario Gregorio Marañon (Madrid, Spain). Patients provided their written informed consent to participate in the study and oncologists voluntarily accepted to participate. No economic compensation was offered to participants.
The eligible patient population was estimated based on the population over 20 years of age in Spain in 2017 (37,315,882) (34), the prevalence of kidney cancer (0.011%) (35), and the proportion of patients with RCC (80%) (36). Thus, the eligible population for the study was 3,194 RCC patients. The oncologist population was established according to the number of oncologists treating RCC in Spain, based on the scientific committee’s estimate that about 1-2 oncologists per hospital treat RCC (including medium-large hospitals, i.e., >200 beds). Thus, the eligible population was 162 oncologists in Spain (37).
The minimum sample size necessary for the DCE was based on the estimation of proportions, coinciding with an approach proposed by Orme (38). The maximum variation criterion was applied (p=q=0.5), with a 95% confidence interval (Zα=1.96) and a 10% accuracy error. Based on this, a minimum necessary sample of 93 aRCC patients and 61 oncologists was estimated.
A steering committee consisting of two oncologists, experts in the management of RCC, led the study and provided scientific advice during its development.
Discrete Choice Experiment
DCE is a technique for eliciting individuals’ preferences in an indirect way (39), based on the premise that medical interventions can be described as combinations of different features or attributes. DCE involves asking respondents to choose between competing scenarios, each comparing two hypothetical treatment options with a series of defined attributes represented at various levels (e.g., an attribute of ‘route of administration’ at the level of ‘oral’). Using the choice data, the value that individuals attach to their constituent parts is estimated via probabilistic choice models, such as logit and probit models (39). DCE was applied according to the International Society for Pharmacoeconomics and Outcomes Research (ISPOR) Good Research Practices for Conjoint Analysis (30).
Attributes and Levels Selection
Attributes and their associated levels were initially identified by literature review. Key terms related to the disease (RCC), its treatment or treatment-related decision-making, and preferences studies were used to search the Pubmed/Medline international database (Supplementary Table 1). Studies assessing professionals’ or patients’ preferences for RCC attributes, published from March 15, 2009 up to March 15, 2019, were reviewed. A total of eight studies with potential attributes for inclusion in the DCE were identified (Supplementary Table 2).
The final selection of characteristics for use in the DCE was based on a focus group with eight oncologists and on semi-structured telephone interviews with five aRCC patients. The main purpose was to validate the potential attributes identified in the literature, to identify relevant attributes not retrieved, and to assess the comprehensibility of the attributes and levels proposed. As a result, five attributes, with a maximum of three levels each, were selected (Table 1).
Experimental Design and Survey Instrument
A paper- or web-based survey was completed by patients and oncologists, respectively. The patient survey included sociodemographic data (provided by the patient) and clinical variables (collected by the physician from medical records), and the choice tasks, self-reported by the patient (Supplementary Table 3). The oncologist survey included sociodemographic variables and the same choice sets as for patients (Supplementary Table 4).
An experimental design was constructed, consisting of a series of choice tasks from combinations of the attribute levels (called scenario alternatives), following ISPOR recommendations (30). Fractional factorial design (orthogonal main-effect matrix) generated 18 scenarios, with a mix-and-match algorithm used to generate the choice sets. To avoid participant fatigue, the 18 scenarios were divided in two blocks of nine multiple-choice sets, distributed across two versions of questionnaires. The experimental task asked participants to choose their preferred treatment scenario. Additionally, as is generally performed in DCEs (40), an initial control scenario was included to check whether the participant understands the exercise. In this scenario, one treatment was clearly superior to the other, so participants who answered this question incorrectly were excluded from analysis (41). Table 2 shows an example of the choice set.
Analyses
Sociodemographic and clinical variables were described using absolute and relative frequencies of response for qualitative variables, and statistics of centrality and dispersion for quantitative variables.
Preferences were analyzed applying a mixed logit model [Stata software (42)], which accounts for preference heterogeneity among respondents (43). It yields both a mean effect and a standard deviation (SD) of effects across the sample. Attributes with quantitative levels (survival gain, probability of suffering serious adverse events [SAEs], and cost) were transformed into linear variables. In these cases, the utility value for the unit was obtained. The regression coefficients, referred to as part-worth utilities, are interpreted as the utilities associated with each level within an attribute. These coefficients are not directly comparable between attributes. To this end, the relative importance (RI) of an attribute over the range of attributes included in the experiment was estimated for each participant as the range of part-worth utilities of an attribute (difference in part-worth utilities between the best or preferred level and the worst or least preferred level of the same attribute), divided by the sum of all ranges across attributes and multiplying by 100 (44). The mean RI of each attribute was calculated for each group of participants (patients and oncologists). Furthermore, to assess differences and similarities between patients’ and oncologists’ preferences for aRCC treatment attributes, individual IRs between patients and oncologists were compared.
To identify possible explanatory variables in patients’ and oncologists’ preferences, subgroup analyses comparing individual RIs for each attribute were performed according to the following variables and cut-offs for patients: gender, median age, education level (primary studies vs. secondary education or higher), median time from diagnosis, number of comorbidities (≤ or > 1 comorbidity), number of treatments received for RCC (≤ or > 1 treatment), route (oral vs. others), and frequency of administration of current treatment (1/2 times a day vs. others); and for oncologists: gender, median age, median years of professional experience, and median years in RCC management.
For comparisons, Student’s t-test or the Mann-Whitney U test were used, according to data distribution. For all statistical tests, statistical significance was set at p<0.05.
The maximum acceptable risk (MAR) that participants were willing to trade-off for a treatment benefit was estimated as the quotient between the utility associated with a clinical benefit attribute (1 month survival gain) and the utility associated with a 1% increased risk of SAEs (45, 46).
Willingness to pay (WTP) for a given clinical benefit (increased survival or reduced likelihood of SAEs), was estimated as the ratio of the partial utility of the attribute levels and the partial utility per incremental cost (47).
Results
Sociodemographic Characteristics of Participants
A total of 105 aRCC patients (77.1% male, mean age of 65.9 years [SD: 10.4], mean duration from RCC diagnosis 6.3 years [SD: 6.1]) and 67 oncologists (52.2% male, mean age 41.9 years [SD: 8.4], mean length of professional experience 13.6 years [SD: 7.8] and of experience in RCC management 10.2 years [SD: 7.5]) were included in the final data analysis (Table 3).
Preferences for RCC attributes
Items showing statistical significance (p<0.05) in both patients’ and oncologists’ preferences included levels of survival gain, SAE risk, level of intravenous administration every 15 days and worsening of HRQoL. Additionally, treatment cost was statistically significant (p<0.05) in the preferences of the oncologist group (Table 4). The signs of the coefficients indicate how this influence translates into preferences. In the case of SAEs, the results showed a greater preference for lower-risk scenarios. Survival gain coefficients indicated a greater preference for more years of survival. Intravenous administration every 15 days was less preferred than oral administration, and worsening of HRQoL was less preferred than improvement. The cost coefficients showed a greater preference for lower cost (only in the oncologist group). The main results of the mixed logit model are shown in Table 4.
Among all the attributes studied, both patients and oncologists assigned the highest RI to survival gain (43.6% and 54.7% respectively), followed by HRQoL (35.3% and 18.0%, respectively). Cost was the attribute with the lowest RI for both groups (0.6% and 5.5%, respectively) (Figure 1). Significant differences were found in the RI assigned to all attributes between patients and oncologists (p<0.05) (Figure 1).
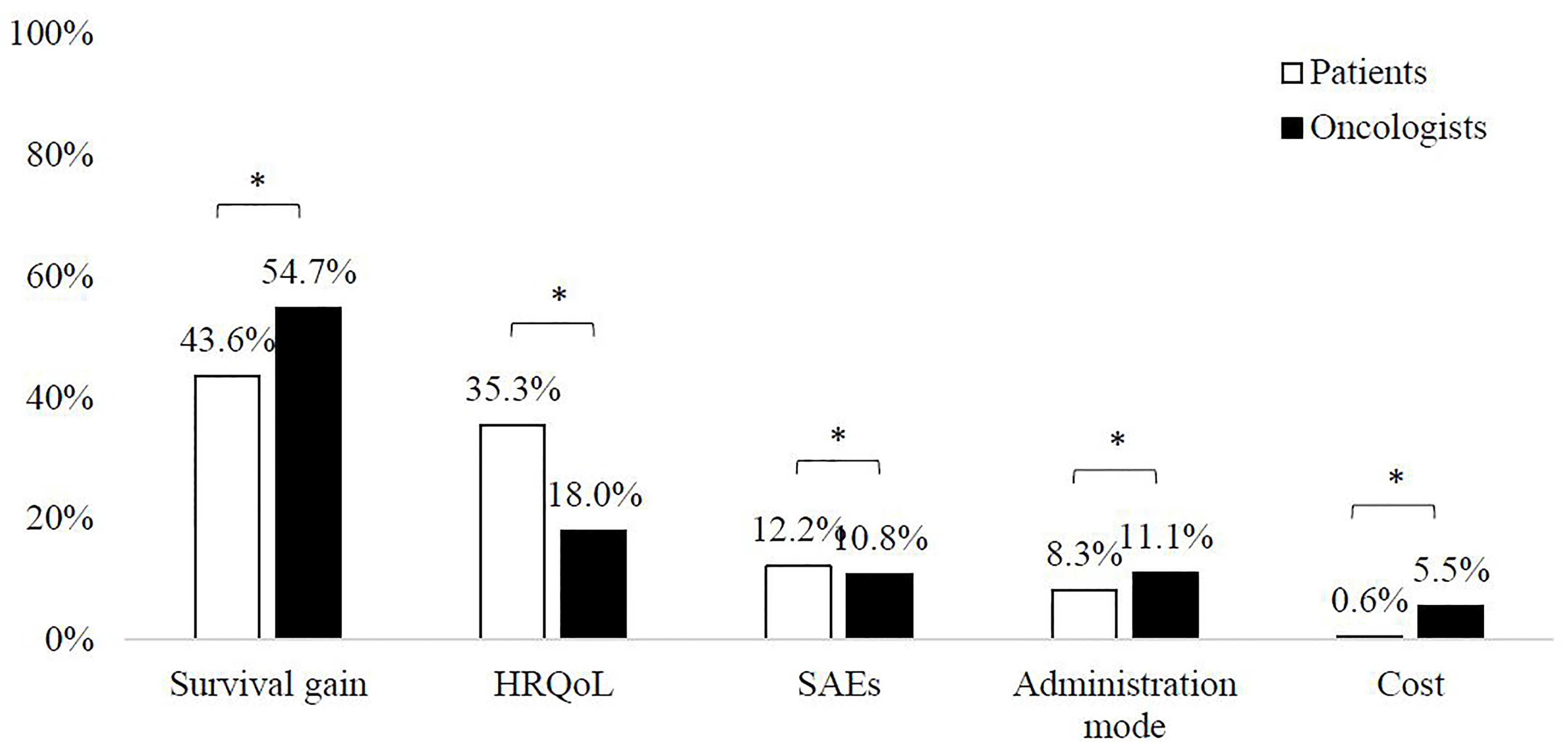
Figure 1 Relative importance of attributes *Significant difference between patients and oncologists (p < 0.05).
Determinants of Preferences
Regarding subgroup analyses to identify possible explanatory variables in patients’ and oncologists’ preferences, it was found that patients with less time since diagnosis (< 4 vs. 4 years) and oncologists with less professional experience (< 11 vs. ≥ 11 years) assign higher RI to SAEs, while patients with a lower education level (primary studies vs. secondary education or higher) assign higher RI to survival and lower RI to administration mode and treatment cost (all p<0.05). No other differences were observed (Supplementary Table 5).
Risk Tolerance and Willingness to Pay
Patients and oncologists were willing to accept an increase of 3.0% and 4.2% in SAE risk, respectively, in exchange for a one-month survival gain.
WTP was higher among patients than oncologists for increasing survival or lowering SAE risk (Table 5).
Discussion
The management of patients with aRCC has changed dramatically over the past few years as a result of the availability of multiple active agents. The near future will bring data on new agents and combinations of therapies administered to complement or even synergize with approved drugs and molecules currently under development. Hence, practitioners face new questions and challenges such as how to select the best drug for a given patient at a particular time. In this context, patients, physicians, and payers are seeking the highest value care for each patient, hence tools to compare the value of therapies have become necessary (1).
Stated preference surveys, such as DCE, are one of the most reliable and valid techniques available for quantifying participants’ preferences in healthcare (48). Accumulated evidence on patients’ and healthcare professionals’ preferences for RCC treatment characteristics is scarce. Greater knowledge of these preferences could help to identify relevant treatment characteristics for patients and healthcare professionals, improve patient management and encourage shared decision-making (49–51).
In this study, survival gain and improved HRQoL were the treatment attributes most highly valued by patients and oncologists. SAE risk was the third attribute in importance for patients, over administration mode. For oncologists, SAE risk and administration mode had similar RI. Cost of treatment (for the health system) was the attribute with lowest value for both groups. These results suggest that, in the RCC treatment decision, survival gain and improved HRQoL are essential for both patients and oncologists. Clinical benefit is an important part of value assessment, and in aRCC, overall survival has become the primary indicator of patient benefit. Beyond the general treatment goal of patients living longer, patient-specific factors also require consideration in the assessment of value (1). In this regard, HRQoL is increasingly recognized as a crucial aspect in overall patient outcomes (52). These factors have a major influence on decision-making and, hence, on the determination of the value of a treatment (1). Nonetheless, our results point out that improved HRQoL continues to be much more relevant for patients than for oncologists.
Previous studies have shown different results regarding patient preferences in RCC. Similar to our findings, Wong et al. and Mohamed et al. (31, 33) found that efficacy (PFS) was the most important attribute; while Park et al. (53) observed that patients considered AEs (hand-foot skin reaction, bone marrow suppression, gastrointestinal perforation, and bleeding) more important than PFS. Patients seemed to give greater importance to AEs that are more prevalent, while more serious but less prevalent AEs were considered less important (31, 33).
Preferences regarding treatment efficacy and AEs depend on both the magnitude of the benefit/risk and the patient’s own experience. Thus, patients who experience AEs of treatment tend to require greater clinical benefit than those who do not (54). In our study, patients with a longer time since diagnosis (and oncologists with longer professional experience) assigned lower importance to SAEs. This might implicate that these patients weigh the risks and benefits differently, in that they appear to accept more AEs as the price for being able to spend as much time as possible with their partners (24). In addition, it has been described that the weight of preferences may vary over the course of treatment (54). On the other hand, patients with a lower education level assigned greater importance to survival and a lower value to the administration mode and treatment cost. Other studies have also found an influence of educational level on preferences; for example cancer survivors’ preference for patient-centered care was associated with a higher educational level (55), the preference for the format in which prognostic information is provided for advanced cancer patients was influenced by the number of years of school education (56), and multiple sclerosis patients with higher education were less concerned about adverse events (57).
Notably, despite the key role of healthcare professionals in shared decision-making and treatment prescription in RCC, very few studies address the preferences of healthcare professionals. Park et al. found that the preferences of patients and healthcare professionals differed significantly. While patients gave more importance to AEs (as previously mentioned), physicians assigned a higher value to PFS, followed by route of administration and skin reactions. In fact, compared to patients, physicians would be willing to accept almost 10 times the risk of skin reaction and bone marrow suppression (1.3% vs. 9.6% and 1.0% vs. 11.8%, respectively) (53). In our study, the RI assigned to all treatment attributes was significantly different between the two groups of participants, with HRQoL and SAE risk being more highly valued by patients, and survival gain, administration mode and treatment cost more highly valued by oncologists. Therefore, given the lower value attributed to SAEs, oncologists were willing to accept a slightly higher increase in SAE risk than patients in exchange for 1-month survival gain (4.2% oncologists and 3.0% patients). In contrast, Gonzalez et al. showed that although efficacy was the most important attribute for both physicians and patients, the former gave greater importance to fatigue, skin reactions or other attributes such as co-payment (22). These results would be in line with those observed by Lawrence et al., in which the survival times judged sufficient to warrant treatment (e.g. adverse effects) were significantly higher among physicians (9) compared to patients (54). Of note, our study specifically addressed SAEs and not mild or general AEs, unlike these latter studies. Overall, these results highlight the relevance for physicians to recognize that their patients may have preferences that differ from their own when considering the potential benefits and harms of treatment.
In addition, given the high costs of these new treatments, physicians need to assess which drugs or combinations provide the best value for patients and the healthcare system and are thus worth pursuing (1). In publicly-funded National Healthcare Systems, prescribing physicians can be considered as serving a dual role, acting as patients’ advocates but also as society’s gatekeepers of the use of resources (58). We found that, despite cost (for the healthcare system) being the attribute with lower RI, it has an influence on oncologists’ (although not for patients) preferences. Consequently, WTP for survival gain or risk reduction was higher among patients.
The current study is subject to certain limitations. Although DCE is the recommended approach and is widely used to assess patient preferences for treatment characteristics, there is always a risk of a gap between stated and revealed preferences (30, 59); therefore, the study participants might make other choices in real life. Additionally, other attributes not included may also be relevant. However, this potential bias was prevented by selecting the most important attributes from the literature and using the input of an expert focus group (oncologists) and interviews with RCC patients. The selection of different levels may provide other utility values and relative importance. Another limitation is the inherent selection bias of getting the information from patients and oncologists willing to participate in the study, whose views might differ from those of other patients or oncologists. Finally, sample size requirements in DCE are also a controversial issue. No general recommendations exist, so sample sizes differ substantially between studies and the method used is not usually reported (48). The sample of participants in this study was representative of the number of Spanish RCC patients and Spanish oncologists experts in RCC. In this regard, the findings of the study significantly reflect preferences for RCC treatment attributes in Spain and should be interpreted within the context of the study. Above all, the comparison between patients’ subjective thoughts and oncologists’ data increases the strength of the results and provides new insights into the scarcely studied subject of oncologists’ preferences in RCC.
In summary, this study shows that, for both aRCC patients and oncologists, the most important treatment attributes are survival gain and improved HRQoL. Remarkably, SAE risk and administration mode are also important attributes to consider, while treatment cost is of least relevance. Patients would be more willing to accept that the health system should assume higher costs for improving survival and reducing SAE risk than oncologists. The results of the study can contribute to improved decision-making in the selection of an appropriate RCC treatment.
Data Availability Statement
The original contributions presented in the study are included in the article/Supplementary Material, further inquiries can be directed to the corresponding author.
Ethics Statement
The studies involving human participants were reviewed and approved by Ethics Committee of the Hospital General Universitario Gregorio Marañon (Madrid, Spain). Patients provided their written informed consent to participate in the study.
Author Contributions
OF, ML-Q, GC, DSdP, ÁP, LB, AG, OE, and SB contributed equally to the design of the research and to data retrieval. CG-R and SA contributed equally to the conception and design of the research, the analysis and interpretation of the data and drafted the manuscript. VP and CP-S contributed equally to the conception and design of the research and to data interpretation. All authors critically reviewed the manuscript, agree to be fully accountable for ensuring the integrity and accuracy of the work, and read and approved the final draft.
Funding
The study was funded by Bristol Myers Squibb.
Conflict of Interest
OF has performed a consulting or advisory role for Astellas Pharma, Roche, Pfizer, Bristol-Myers-Squibb, Sanofi, EUSA Pharma, Sanoffi; has received speaking honoraria form Pierre-Fabre, Novartis, Bristol-Myers-Squibb, Ipsen, Roche, Astellas Pharma, Bayer, Janssen; and has received travel/accommodation expenses from Bristol-Myers-Squibb, Ipsen, Astellas. SB has received travel grants and/or honoraria for educational activities from BMS, Roche, Pfizer and MSD. ML-Q has participated in speaker’s bureaus for Roche, Boehringer Ingelheim, Janssen-Cilag, Ipsen, MSD, Novartis, Astra Zeneca, Lilly, Pfizer, Astellas Pharma; has performed a consulting or advisory role for MSD, AstraZeneca, BMS, Boehringer Ingelheim, Roche, Ipsen, EUSA Pharma, Eisai, Pfizer; and has received travel and accommodation expenses from MSD, BMS, AstraZeneca, Pfizer, Roche, Lilly. ÁP has received funding for research, travel grants and/or speaker fees from Pfizer, Novartis, EUSA Pharma, Ipsen, BMS, MSD, Merck, Janssen, Astellas, Bayer, Sanofi, Clovis and Roche. AG has received honoraria for educational activities from Bristol-Myers Squibb, Eusa Pharma, Ipsen, MSD, Pfizer and Roche-Genentech. CG-R and SA work for an independent research entity and have received fees for their contribution to the development of the project and the writing of the manuscript. VP and CP-S work at Bristol-Myers Squibb.
The remaining authors declare that the research was conducted in the absence of any commercial or financial relationships that could be construed as a potential conflict of interest.
The reviewer RMB declared a past co-authorship with the authors OF and ÁP to the handling editor.
Publisher’s Note
All claims expressed in this article are solely those of the authors and do not necessarily represent those of their affiliated organizations, or those of the publisher, the editors and the reviewers. Any product that may be evaluated in this article, or claim that may be made by its manufacturer, is not guaranteed or endorsed by the publisher.
Supplementary Material
The Supplementary Material for this article can be found online at: https://www.frontiersin.org/articles/10.3389/fonc.2021.773366/full#supplementary-material
References
1. Posadas EM, Limvorasak S, Figlin RA. Targeted Therapies for Renal Cell Carcinoma. Nat Rev Nephrol (2017) 13:496–511. doi: 10.1038/nrneph.2017.82
2. International agency for research on cancer. Data Source: GLOBOCAN 2020 (2021). Available at: http://gco.iarc.fr/.
4. Lázaro M, Valderrama BP, Suárez C, de-Velasco G, Beato C, Chirivella I, et al. SEOM Clinical Guideline for Treatment of Kidney Cancer (2019). Clin Transl Oncol (2020) 22:256–69. doi: 10.1007/s12094-019-02285-7
5. Janzen NK, Kim HL, Figlin RA, Belldegrun AS. Surveillance After Radical or Partial Nephrectomy for Localized Renal Cell Carcinoma and Management of Recurrent Disease. Urol Clin North Am (2003) 30:843–52. doi: 10.1016/S0094-0143(03)00056-9
6. Lam JS, Leppert JT, Belldegrun AS, Figlin RA. Novel Approaches in the Therapy of Metastatic Renal Cell Carcinoma. World J Urol (2005) 23:202–12. doi: 10.1007/s00345-004-0466-0
7. Chavan S, Bray F, Lortet-Tieulent J, Goodman M, Jemal A. International Variations in Bladder Cancer Incidence and Mortality. Eur Urol (2014) 66:59–73. doi: 10.1016/j.eururo.2013.10.001
8. Coppin C, Porzsolt F, Awa A, Kumpf J, Coldman A, Wilt T. Immunotherapy for Advanced Renal Cell Cancer. Cochrane Database Syst Rev (2005) (1):CD001425. doi: 10.1002/14651858.CD001425.pub2
9. Lawrence NJ, Martin A, Davis ID, Troon S, Sengupta S, Hovey E, et al. What Survival Benefits are Needed to Make Adjuvant Sorafenib Worthwhile After Resection of Intermediate- or High-Risk Renal Cell Carcinoma? Clinical Investigators’ Preferences in the SORCE Trial. Kidney Cancer (2018) 2:123–31. doi: 10.3233/KCA-180038
10. Motzer RJ, Jonasch E, Dror Michaelson M, Nandagopal L, Gore JL, George S, et al. NCCN Guidelines Insights: Kidney Cancer, Version 2.2020. JNCCN J Natl Compr Cancer Netw (2019) 17:1278–85. doi: 10.6004/jnccn.2019.0054
11. Li P, Wong YN, Armstrong K, Haas N, Subedi P, Davis-Cerone M, et al. Survival Among Patients With Advanced Renal Cell Carcinoma in the Pretargeted Versus Targeted Therapy Eras. Cancer Med (2016) 5:169–81. doi: 10.1002/cam4.574
12. Carmichael C, Yuh BE, Sun V, Lau C, Hsu J, Saikia J, et al. Quality of Life in Patients With Metastatic Renal Cell Carcinoma: Assessment of Long-Term Survivors. Clin Genitourin Cancer (2013) 11:149–54. doi: 10.1016/j.clgc.2012.09.006
13. Ko JJ, Choueiri TK, Rini BI, Lee JL, Kroeger N, Srinivas S, et al. First-, Second-, Third-Line Therapy for mRCC: Benchmarks for Trial Design From the IMDC. B. R J Cancer (2014) 110:1917–22. doi: 10.1038/bjc.2014.25
14. Escudier B, Porta C, Schmidinger M, Algaba F, Patard JJ, Khoo V, et al. Renal Cell Carcinoma: ESMO Clinical Practice Guidelines for Diagnosis, Treatment and Follow-Up. Ann Oncol (2014) 25 Suppl 3:iii49–56. doi: 10.1093/annonc/mdu259
15. Finley DS, Pantuck AJ, Belldegrun AS. Tumor Biology and Prognostic Factors in Renal Cell Carcinoma. Oncologist (2011) 16(Suppl 2):4–13. doi: 10.1634/theoncologist.2011-S2-04
16. Brahmer JR, Drake CG, Wollner I, Powderly JD, Picus J, Sharfman WH, et al. Phase I Study of Single-Agent Anti-Programmed Death-1 (MDX-1106) in Refractory Solid Tumors: Safety, Clinical Activity, Pharmacodynamics, and Immunologic Correlates. J Clin Oncol (2010) 28:3167–75. doi: 10.1200/JCO.2009.26.7609
17. Topalian SL, Hodi FS, Brahmer JR, Gettinger SN, Smith DC, McDermott DF, et al. Safety, Activity, and Immune Correlates of Anti-PD-1 Antibody in Cancer. N Engl J Med (2012) 366:2443–54. doi: 10.1056/NEJMoa1200690
18. Borghaei H, Paz-Ares L, Horn L, Spigel DR, Steins M, Ready NE, et al. Nivolumab Versus Docetaxel in Advanced Nonsquamous Non-Small-Cell Lung Cancer. N Engl J Med (2015) 373:1627–39. doi: 10.1056/NEJMoa1507643
19. Brahmer J, Reckamp KL, Baas P, Crinò L, Eberhardt WE, Poddubskaya E, et al. Nivolumab Versus Docetaxel in Advanced Squamous-Cell Non-Small-Cell Lung Cancer. N Engl J Med (2015) 373:123–35. doi: 10.1056/NEJMoa1504627
20. Motzer RJ, Rini BI, McDermott DF, Redman BG, Kuzel TM, Harrison MR, et al. Nivolumab for Metastatic Renal Cell Carcinoma: Results of a Randomized Phase II Trial. J Clin Oncol (2015) 33:1430–7. doi: 10.1200/JCO.2014.59.0703
21. Garje R, An J, Greco A, Vaddepally RK, Zakharia Y. The Future of Immunotherapy-Based Combination Therapy in Metastatic Renal Cell Carcinoma. Cancers (Basel) (2020) 12:1–13. doi: 10.3390/cancers12010143
22. González JM, Doan J, Gebben DJ, Boeri M, Fishman M. Comparing the Relative Importance of Attributes of Metastatic Renal Cell Carcinoma Treatments to Patients and Physicians in the United States: A Discrete-Choice Experiment. Pharmacoeconomics (2018) 36:973–86. doi: 10.1007/s40273-018-0640-7
23. Eichler HG. Regulatory Decision-Making in the Context of Personalized Medicine: Consequences for Authorization and Pharmacovigilance. 2nd Annual Health Economics and Personalized Medicine Symposium. Luxemburg (2013).
24. Spaich S, Kinder J, Hetjens S, Fuxius S, Gerhardt A, Sütterlin M. Patient Preferences Regarding Chemotherapy in Metastatic Breast Cancer-a Conjoint Analysis for Common Taxanes. Front Oncol (2018) 8:1–11. doi: 10.3389/fonc.2018.00535
25. Hubbard G, Kidd L, Donaghy E. Preferences for Involvement in Treatment Decision Making of Patients With Cancer: A Review of the Literature. Eur J Oncol Nursing: Off J Eur Oncol Nurs Soc (2008) 12(4):299–318. doi: 10.1016/j.ejon.2008.03.004
26. Colligan E, Metzler A, Tiryaki E. Shared Decision-Making in Multiple Sclerosis : A Review. Mult Scler (2017) 23:185–90. doi: 10.1177/1352458516671204
27. daCosta DiBonaventura M, Copher R, Basurto E, Faria C, Lorenzo R. Patient Preferences and Treatment Adherence Among Women Diagnosed With Metastatic Breast Cancer. Am Heal Drug Benefits (2014) 7:386–96.
28. Tamirisa NP, Goodwin JS, Kandalam A, Linder SK, Weller S, Turrubiate S, et al. Patient and Physician Views of Shared Decision Making in Cancer. Heal Expect (2017) 20(6):1248–53. doi: 10.1111/hex.12564
30. Bridges JF, Hauber AB, Marshall D, Lloyd A, Prosser LA, Regier DA, et al. Conjoint Analysis Applications in Health–a Checklist: A Report of the ISPOR Good Research Practices for Conjoint Analysis Task Force. Value Heal (2011) 14:403–13. doi: 10.1016/j.jval.2010.11.013
31. Mohamed AF, Hauber AB, Neary MP. Patient Benefit-Risk Preferences for Targeted Agents in the Treatment of Renal Cell Carcinoma. Pharmacoeconomics (2011) 29:977–88. doi: 10.2165/11593370-000000000-00000
32. Park MH, Jo C, Bae EY. Lee EK. A Comparison of Preferences of Targeted Therapy for Metastatic Renal Cell Carcinoma Between the Patient Group and Health Care Professional Group in South Korea. Value Heal (2012) 15:933–9. doi: 10.1016/j.jval.2012.05.008
33. Wong MK, Mohamed AF, Hauber AB, Yang J, Liu Z, Rogerio J. Patients Rank Toxicity Against Progression Free Survival in Second-Line Treatment of Advanced Renal Cell Carcinoma. J Med Econ (2012) 15:1–14. doi: 10.3111/13696998.2012.708689
34. National Statistics Institute. Cifras De Población a 1 Enero 2017. Available at: http://www.ine.es/jaxiT3/Tabla.htm?t=9663&L=0 (Accessed july 2017).
35. International Agency for Research on Cancer. 2012 Country Factsheets. Available at: http://eco.iarc.fr/eucan/CountrySearch.aspx (Accessed July 2017).
36. Escudier B, Kataja V. Renal Cell Carcinoma: ESMO Clinical Practice Guidelines for Diagnosis, Treatment and Follow-Up Clinical Practice Guidelines. Ann Oncol (2010) 21: (Supplement 5):137–9. doi: 10.1093/annonc/mdq206
37. Ministry of Health, Consumer Affairs and Social Welfare. Available at: https://www.mscbs.gob.es/ciudadanos/prestaciones/centrosServiciosSNS/hospitales/docs/CNH2017.pdf.
38. Orme B. Sample Size Issues for Conjoint Analysis, 2nd ed. In: Orme B, ed. Getting Started with Conjoint Analysis: Strategies for Product Design and Pricing Research. Madison,WI: Research Publishers LLC (2010), 57–66.
39. Erdem S, Thompson C. Prioritising Health Service Innovation Investments Using Public Preferences: A Discrete Choice Experiment. BMC Health Serv Res (2014) 14:360. doi: 10.1186/1472-6963-14-360
40. Mattmann M, Logar I, Brouwer R. Choice Certainty, Consistency, and Monotonicity in Discrete Choice Experiments. J Environ Econ Policy (2018) 0:1–19. doi: 10.1080/21606544.2018.1515118
41. Ryan M, Kolstad J, Rockers P, Dolea C. How to Conduct a Discrete Choice Experiment for Health Workforce Recruitment and Retention in Remote and Rural Areas: A User Guide With Case Studies. World Health Organization & CapacityPlus: World Bank (2012), p. 94.
43. Appelbaum FR. Preparative Regimens and Ageism. Biol Blood Marrow Transplant (2011) 17:1419–20. doi: 10.1016/j.bbmt.2011.08.005
44. Landfeldt E, Eriksson J, Ireland S, Musingarimi P, Jackson C, Tweats E, et al. Patient, Physician, and General Population Preferences for Treatment Characteristics in Relapsed or Refractory Chronic Lymphocytic Leukemia: A Conjoint Analysis. Leuk Res (2016) 40:17–23. doi: 10.1016/j.leukres.2015.11.006
45. Byun JH, Kwon SH, Lee JE, Cheon JE, Jang EJ, Lee EK. Comparison of Benefit-Risk Preferences of Patients and Physicians Regarding Cyclooxygenase-2 Inhibitors Using Discrete Choice Experiments. Patient Prefer Adherence (2016) 10:641–50. doi: 10.2147/PPA.S98228
46. Van Houtven G, Johnson FR, Kilambi V, Hauber AB. Eliciting Benefit-Risk Preferences and Probability-Weighted Utility Using Choice-Format Conjoint Analysis. Med Decis Mak (2011) 31:469–80. doi: 10.1177/0272989X10386116
47. Hole AR, Kolstad JR. Mixed Logit Estimation of Willingness to Pay Distributions: A Comparison of Models in Preference and WTP Space Using Data From a Health-Related Choice Experiment. Empir Econ (2012) 42:445–69. doi: 10.1007/s00181-011-0500-1
48. de Bekker Grob EW, Ryan M, Gerard K. Discrete Choice Experiments in Health Economics: A Review of the Literature. Health Econ (2012) 21:145–72. doi: 10.1002/hec.1697
49. Arroyo R, Sempere AP, Ruiz-Beato E, Prefasi D, Carreño A, Roset M, et al. Conjoint Analysis to Understand Preferences of Patients With Multiple Sclerosis for Disease-Modifying Therapy Attributes in Spain: A Cross-Sectional Observational Study. BMJ Open (2017) 7:e014433. doi: 10.1136/bmjopen-2016-014433
50. Adams R. Improving Health Outcomes With Better Patient Understanding and Education. Risk Manag Healthc Policy (2010) 3:61–72. doi: 10.2147/RMHP.S7500
51. Institute of Medicine (IOM). Delivering High-Quality Cancer Care: Charting a New Course for a System in Crisis. Washington, DC: The National Academies Press (2013) doi: 10.17226/18359
52. Sitlinger A, Zafar SY. Health-Related Quality of Life: The Impact on Morbidity and Mortality. Surg Oncol Clin N Am (2018) 27:675–84. doi: 10.1016/j.soc.2018.05.008
53. Park M, Jo C, Bae EY, Lee E. A Comparison of Preferences of Targeted Therapy for Metastatic Renal Cell Carcinoma Between the Patient Group and Health Care Professional Group in South Korea. Value Heal (2012) 15:933–9. doi: 10.1016/j.jval.2012.05.008
54. Blinman PL, Davis ID, Martin A, Troon S, Sengupta S, Hovey E, et al. Patients’ Preferences for Adjuvant Sorafenib After Resection of Renal Cell Carcinoma in the SORCE Trial: What Makes it Worthwhile? Ann Oncol (2018) 29:370–6. doi: 10.1093/annonc/mdx715
55. Chan CMH, Blanch-Hartigan D, Taib NA, Wee LH, Krupat E, Meyer F. Preferences for Patient-Centered Care Among Cancer Survivors 5 Years Post-Diagnosis. Patient Educ Couns (2020) 103:1601–5. doi: 10.1016/j.pec.2020.02.033
56. Hagerty RG, Butow PN, Ellis PA, Lobb EA, Pendlebury S, Leighl N, et al. Cancer Patient Preferences for Communication of Prognosis in the Metastatic Setting. J Clin Oncol (2004) 22:1721–30. doi: 10.1200/JCO.2004.04.095
57. Garcia-Dominguez JM, Muñoz D, Comellas M, Gonzalbo I, Lizán L, Sánchez CP. Patient Preferences for Treatment of Multiple Sclerosis With Disease-Modifying Therapies: A Discrete Choice Experiment. Patient Prefer Adherence (2016) 10:1945–56. doi: 10.2147/PPA.S114619
58. Boqué C, Abad MR, Agustín MJ, García-Goñi M, Moreno C, Gabás-Rivera C, et al. Treatment Decision-Making in Chronic Lymphocytic Leukaemia: Key Factors for Healthcare Professionals. PRELIC Study. J Geriatr Oncol (2020) 11(1):24–30. doi: 10.1016/j.jgo.2019.03.010
Keywords: renal cell carcinoma (RCC), kidney cancer, advanced cancer, preferences, discrete-choice experiment (DCE)
Citation: Fernández O, Lázaro-Quintela M, Crespo G, Soto de Prado D, Pinto Á, Basterretxea L, Gómez de Liaño A, Etxaniz O, Blasco S, Gabás-Rivera C, Aceituno S, Palomar V and Polanco-Sánchez C (2022) Preferences for Renal Cell Carcinoma Pharmacological Treatment: A Discrete Choice Experiment in Patients and Oncologists. Front. Oncol. 11:773366. doi: 10.3389/fonc.2021.773366
Received: 09 September 2021; Accepted: 13 December 2021;
Published: 07 January 2022.
Edited by:
Sanja Štifter, Skejby Sygehus, DenmarkReviewed by:
Aleksandr Shulyak, National Academy of Medical Sciences of Ukraine, UkraineRafael Morales-Barrera, Vall d’Hebron University Hospital, Spain
Copyright © 2022 Fernández, Lázaro-Quintela, Crespo, Soto de Prado, Pinto, Basterretxea, Gómez de Liaño, Etxaniz, Blasco, Gabás-Rivera, Aceituno, Palomar and Polanco-Sánchez. This is an open-access article distributed under the terms of the Creative Commons Attribution License (CC BY). The use, distribution or reproduction in other forums is permitted, provided the original author(s) and the copyright owner(s) are credited and that the original publication in this journal is cited, in accordance with accepted academic practice. No use, distribution or reproduction is permitted which does not comply with these terms.
*Correspondence: Clara Gabás-Rivera, Y2dhYmFzQG91dGNvbWVzMTAuY29t