- 1Department of Breast Oncology, Tianjin Medical University Cancer Institute and Hospital, Tianjin, China
- 2Operations Department, Shanghai OrigiMed Co., Ltd., Shanghai, China
Background: Clinical characteristics including estrogen receptor (ER), progesterone receptor (PR), and human epidermal growth factor 2 (HER2) are important biomarkers in the treatment of breast cancer, but how genomic mutations affect their status is rarely studied. This study aimed at finding genomic mutations associated with these clinical characteristics.
Methods: There were 160 patients with breast cancer enrolled in this study. Samples from those patients were used for next-generation sequencing, targeting a panel of 624 pan-cancer genes. Short nucleotide mutations, copy number variations, and gene fusions were identified for each sample. Fisher’s exact test compared each pair of genes. A similarity score was constructed with the resulting P-values. Genes were clustered with the similarity scores. The identified gene clusters were compared to the status of clinical characteristics including ER, PR, HER2, and a family history of cancer (FH) in terms of the mutations in patients.
Results: Gene-by-gene analysis found that CCND1 mutations were positively correlated with ER status while ERBB2 and CDK12 mutations were positively correlated with HER2 status. Mutation-based clustering identified four gene clusters. Gene cluster 1 (ADGRA2, ZNF703, FGFR1, KAT6A, and POLB) was significantly associated with PR status; gene cluster 2 (COL1A1, AXIN2, ZNF217, GNAS, and BRIP1) and gene cluster 3 (FGF3, FGF4, FGF19, and CCND1) were significantly associated with ER status; gene cluster 2 was also negatively associated with a family history of cancer; and gene cluster 4 was significantly negatively associated with age. Patients were classified into four corresponding groups. Patient groups 1, 2, 3, and 4 had 24.1%, 36.5%, 38.7%, and 41.3% of patients with an FDA-recognized biomarker predictive of response to an FDA-approved drug, respectively.
Conclusion: This study identified genomic mutations positively associated with ER and PR status. These findings not only revealed candidate genes in ER and PR status maintenance but also provided potential treatment targets for patients with endocrine therapy resistance.
Introduction
Breast cancer is the most common cancer among women. The age-standardized incidence of breast cancer even surpassed lung cancer worldwide (1, 2). Although the treatment of breast cancer has achieved great success, there are still 18%–46% of patients whose cancer would eventually develop into late-stage breast cancer (3, 4).
For patients with late-stage breast cancer or patients with unresectable tumors, traditional endocrine therapy and chemotherapy are used concerning the status of several important biomarkers including estrogen receptor (ER), progesterone receptor (PR), and human epidermal growth factor receptor 2 (HER2). With the development of precision medicine, genomic mutations are utilized as additional biomarkers in endocrine therapy and chemotherapy. For hormone-positive patients, inactivating NF1 mutations could be used as the prognostic biomarker of endocrine therapy resistance (5); for patients with triple-negative breast cancer, germline BRCA1/2 mutations could be employed to choose the appropriate medicine. For patients with triple-negative breast cancer with germline BRCA1/2 mutations, carboplatin chemotherapy brought about more clinical benefits than standard docetaxel chemotherapy (6). The objective response rate of carboplatin chemotherapy was two times higher than that of docetaxel chemotherapy.
The above phenomenon drove us to rethink the background mechanism of different ER, PR, and HER2 statuses. As we know, genomic mutations play a significant role in cancer development. They could also take part in the maintenance of ER, PR, and HER2 status. Evidence shows that breast cancer patients with amplification of CCND1 tended to be ER-positive and associated with worse 15-year survival (7, 8). Patients with CCND1 amplification would need long-term treatment. Identifying genomic mutations associated with ER, PR, and HER2 status could help to develop new biomarkers and treatment. In this work, we enrolled 160 Chinese patients at identifying somatic mutations in a Chinese population associated with a family history of cancer (FH).
In this work, we enrolled 160 patients with breast cancer. Samples from the 160 patients were used for next-generation sequencing. Short nucleotide mutations, copy number variations, and gene fusions were identified for each sample. The association between gene mutation clusters and the status of ER, PR, and HER2 were studied. Further, the enrichment of genomic mutations in the Kyoto Encyclopedia of Genes and Genomes (KEGG) pathway and actionability for each patient group were inferred.
Methods
Patients and Samples
This study was approved by the Ethics Committee of Tianjin Medical University Cancer Institute and Hospital (ID: bc2021063). Patients included in this study were those with breast cancer, with written informed consent, and with successful sequencing results. There are 160 Chinese patients included. Patients were staged according to the TNM system. Samples from these patients were obtained from surgery and fixed in 4% neutral-buffered formalin at 4°C for 24 h. The processed samples were embedded in paraffin wax for storage.
Immunohistochemistry and Fluorescence In Situ Hybridization
PR, ER, and HER2 expressions were first detected with immunohistochemistry (IHC). Briefly, a 3–5-μm slice of the wax sample was deparaffinized on the glass slide in a 60°C oven for 1 h and washed with xylene for 15 min. The sample was rehydrated with gradient concentrations (100%, 95%, 85%, and 75%) of alcohol. Four minutes of pressure cooking and 10 min of cold-water bath were used to induce antigen retrieval. Hydrogen peroxide (3%) was used to quench endogenous peroxidase activity for 30 min. The slides were blocked with goat serum (10%) for 30 min and stained with rabbit antibodies for ER, PR, and HER2. A secondary antibody biotinylated with horseradish peroxidase (HRP) was further stained for 25 min (K8002 kit, Dako, Santa Clara, CA). The slides were processed with diaminobenzidine for 5 min (K8002 kit, Dako, Santa Clara, CA). After washing with deionized water, hematoxylin was used to restain the slides. ER- and PR-positive rates of >1% were defined as ER+ and PR+, respectively. HER2 score = 3+ and HER2 score = 0/1+ were defined as HER2+ and HER2-, respectively. HER2 score = 2+ was further verified by fluorescence in situ hybridization (FISH). It was performed according to the manual of the PathVysion HER-2 DNA Probe Kit (Abbott Molecular Inc., IL, USA). The HER2 FISH result was interpreted according to the American Society of Clinical Oncology (ASCO)/College of American Pathologists (CAP) guidelines (9).
The tumors were classified into three hormonal subtypes according to ER, PR, and HER2 status. The three hormonal subtypes are the luminal subtype (ER+ or PR+), HER2-enriched subtype (ER- and PR-, HER2+), and TNBC subtype (ER-, PR-, and HER2-).
Library Construction, Sequencing, and Mutation Calling
The library construction and sequencing were performed in the Clinical Laboratory Improvement Amendments (CLIA)/CAP-compliant Molecular Diagnostics Service Laboratory of Shanghai OrigiMed Co., Ltd. Methods were similar to our previous work (10, 11). Briefly, the formalin-fixed paraffin-embedded (FFPE) tissues were deparaffinized by heating in a 60°C oven for 1 h. As a control, white blood cells from the paired whole blood samples were separated by centrifugation.
DNA was extracted using KAPA (Kapa Biosystems, Wilmington, MA, USA) HyperPrep Kit (Illumina, San Diego, CA, USA). Samples with at least 50 ng of double-stranded DNA were applied in further library construction for both tumor tissues and paired white blood cells. A panel of 624 pan-cancer genes was targeted for amplification. To reduce errors from amplification, molecular identifiers (MIDs) were added to DNA segment ends. Barcodes were also added for multiplex sequencing. The prepared DNA libraries were sequenced on an Illumina NovaSeq 6000 sequencer (Illumina, San Diego, CA). Around both ends of the reads, 151 base pairs were read. The average sequencing depth was about 1,000×.
Raw reads were first trimmed for adaptors by Cutadapt (version 1.18). Reads were de-duplicated according to MID labels. The software Burrows–Wheeler alignment with maximal exact matches (BWA-MEM, version 0.7.9a) (12) was used to map all the reads onto University of California, Santa Cruz (UCSC) hg19 reference sequences. The alignment was further recalibrated by BaseRecalibrator of GATK (version 3.8). Short nucleotide mutations were called with Mutect2 (13) and varscan (14). Germline mutations were defined as those in the paired white blood cells but not in the known single-nucleotide polymorphism database (ESP6500, 1000 Genomes, gnomAD, and ExAC). Somatic mutations were retrieved by filtering out possible germline mutations. Germline mutations were obtained from each patient’s white blood sequencing and the single-nucleotide polymorphism databases. Further, copy number variations were called with CNVkit (15), and gene fusions were identified with an in-house pipeline (16).
Tumor mutational burden (TMB) was defined as the number of mutations per million effective coverage length of the genome (1.795717 million bases).
Significantly Mutated Genes
Mutations could be preferentially present in specific genes because of gene length, genomic regions, and other patient characteristics. To reduce mutation bias, MutSigCV (17) was used to identify the significantly mutated genes. The calculation was performed using the service on the GenePattern website (18). Default parameters were used. The resulting P-values were adjusted by the Benjamin and Hochberg method for the correction of multiple testing errors.
Actionable Annotation of Mutations
A mutation actionable database, OncoKB (19), was used to query the available drugs and their evidence level for each mutation. A python script released by OncoKB, MafAnnotator.py, was used to automate the query. The disease type was set to “BRCA.” According to the evidence level of confidence, actionable mutations were classified into six, namely, “Level_1”, “Level_2A”, “Level_2B”, “Level_3A”, “Level_3B”, and “Level_4”. Level_1 is the treatment with an FDA-recognized biomarker predictive of response to an FDA-approved drug; Level_2A is the treatment with standard-care biomarker predictive of response to an FDA-approved drug. Level_ 2B is the treatment with standard-care biomarker predictive of response to an FDA-approved drug in another indication but not the standard care in this indication. Level_3A is the treatment with compelling clinical evidence that supports the biomarker as being predictive of response to a drug in this indication. Level_3B is the treatment with compelling clinical evidence that supports the biomarker as being predictive of response to a drug in another indication. Level_4 is the treatment with compelling biological evidence that supports the biomarker as being predictive of response to a drug.
Public Datasets
A muskoskeletal memorial sloan kettering (MSK) cohort (20) was also used to contrast the results in this study. This cohort included 1,756 patients with breast cancer. Data were downloaded from the cBioPortal (www.cbioportal.org).
Statistical Analysis
Comparisons between categorical variables were performed using Fisher’s exact test. Continuous variables were compared by the Mann–Whitney U test. Multiple-testing comparisons were corrected with the Benjamin and Hochberg method. P-values below 0.05 were considered statistically significant. The similarity score of two factors was obtained by transforming P-values to “-log10(P-value)”. When analyzing the association between clinical characteristics and genomic mutations, the cases were omitted if missing records in the current analysis.
Results
Clinical Characteristics of the Patients
Between 2016 and 2018, 160 patients diagnosed with breast cancer were enrolled in this study (Table 1). The patients were about 50 years old, and 32.5% of them were at late-stage. About 50% of patients were either ER-positive or PR-positive and 28.8% of them were HER2-positive. Samples were harvested from 95 primary tumors (59.4%) and 63 metastatic tumors (39.4%). A family history of cancer (FH) was present in 31.3% of patients. The distribution of FH is shown in Supplementary Figure 1A. Lung cancer, liver cancer, breast cancer, endometrial cancer, and colorectal cancer were diagnosed mostly in family members of patients with breast cancer (Supplementary Figure 1B), which accounted for 34.2% of patients with FH.
Mutation Landscape of Breast Cancer
he median percentage of effective coverage (depth >100) is 99.63% (standard error = 1.56), and the lowest is 90.3%. Short nucleotide genomic mutations in the tumors were called with Mutec2 (Figure 1). Mutations with allele frequency below 0.1% were filtered out for accuracy. The top-10 mutated genes were TP53, PIK3CA, ERBB2, CDK12, PTEN, CCND1, FGF19, FGF3, RB1, and BRCA1 (Figure 2). Only six patients had germline mutations in genes including BRCA1, BRCA2, ATM, and RAD51D. For the top-mutated genes, the association between mutations and clinical characteristics including ER, PR, HER2, and FH is displayed in Figures 2A–D, respectively. CCND1 was found significantly associated with ER status (Figure 2A) while ERBB2 and CDK12 were with HER2 status (Figure 2C). No significantly associated mutations were found significantly associated with PR (Figure 2B) and FH (Figure 2D). The association between gene mutations and breast cancer subtypes is shown in Supplementary Figures 2A–C. The HER-enriched subtype had a higher proportion of ERBB2 and CDK12 (Supplementary Figure 2B), and the TNBC subtype had a higher proportion of Kirsten Rat Sarcoma Viral Proto-Oncogene (KRAS) and a lower proportion of ERBB2 (Supplementary Figure 2C).
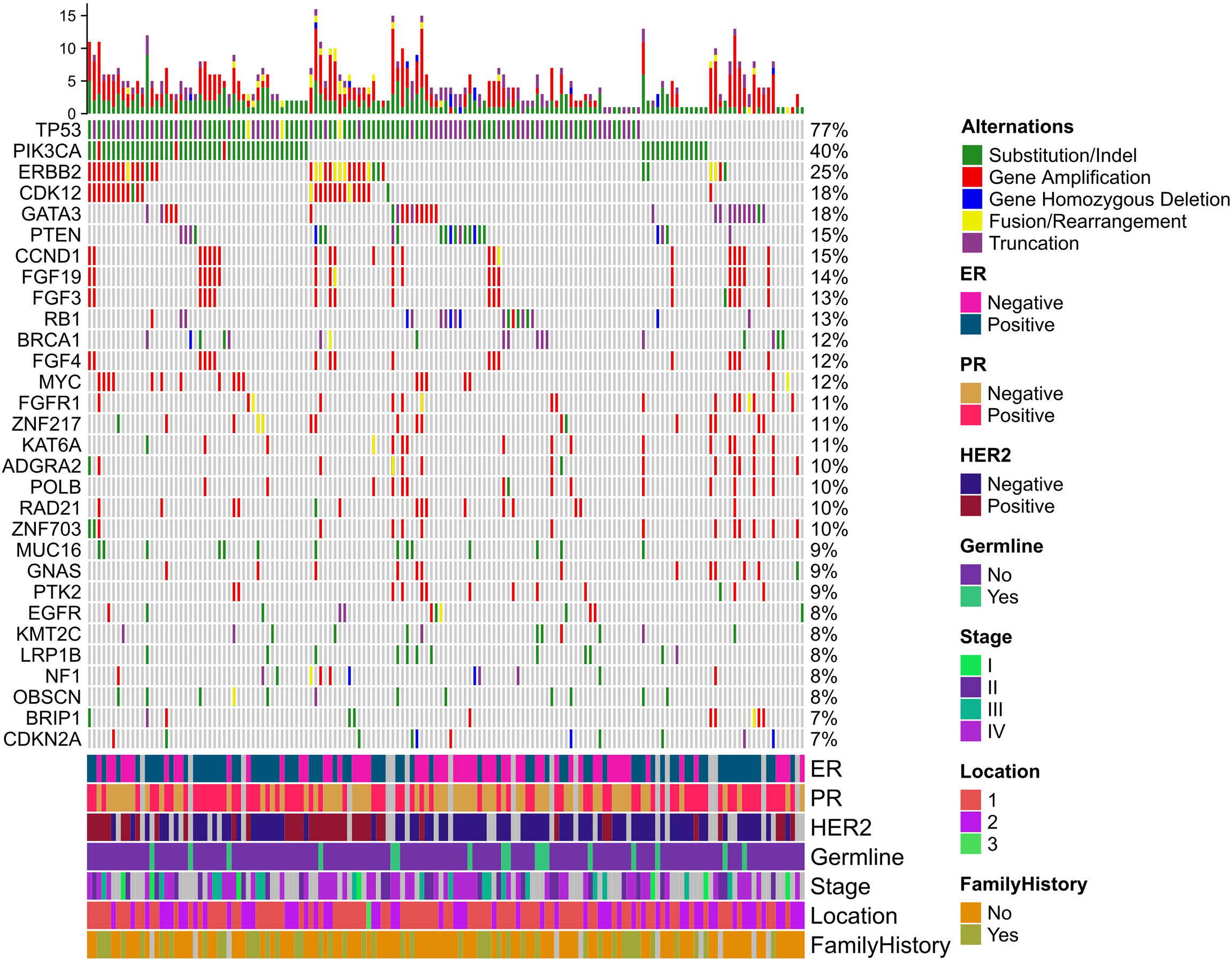
Figure 1 Mutational landscape of breast cancer. Five types of mutations are indicated with different colors in the heatmap plot. The top bar plot summarizes the mutation type proportion in each patient. The below color bar indicates the clinical characteristics of each patient. The heatmap shows the mutations at each gene in each patient.
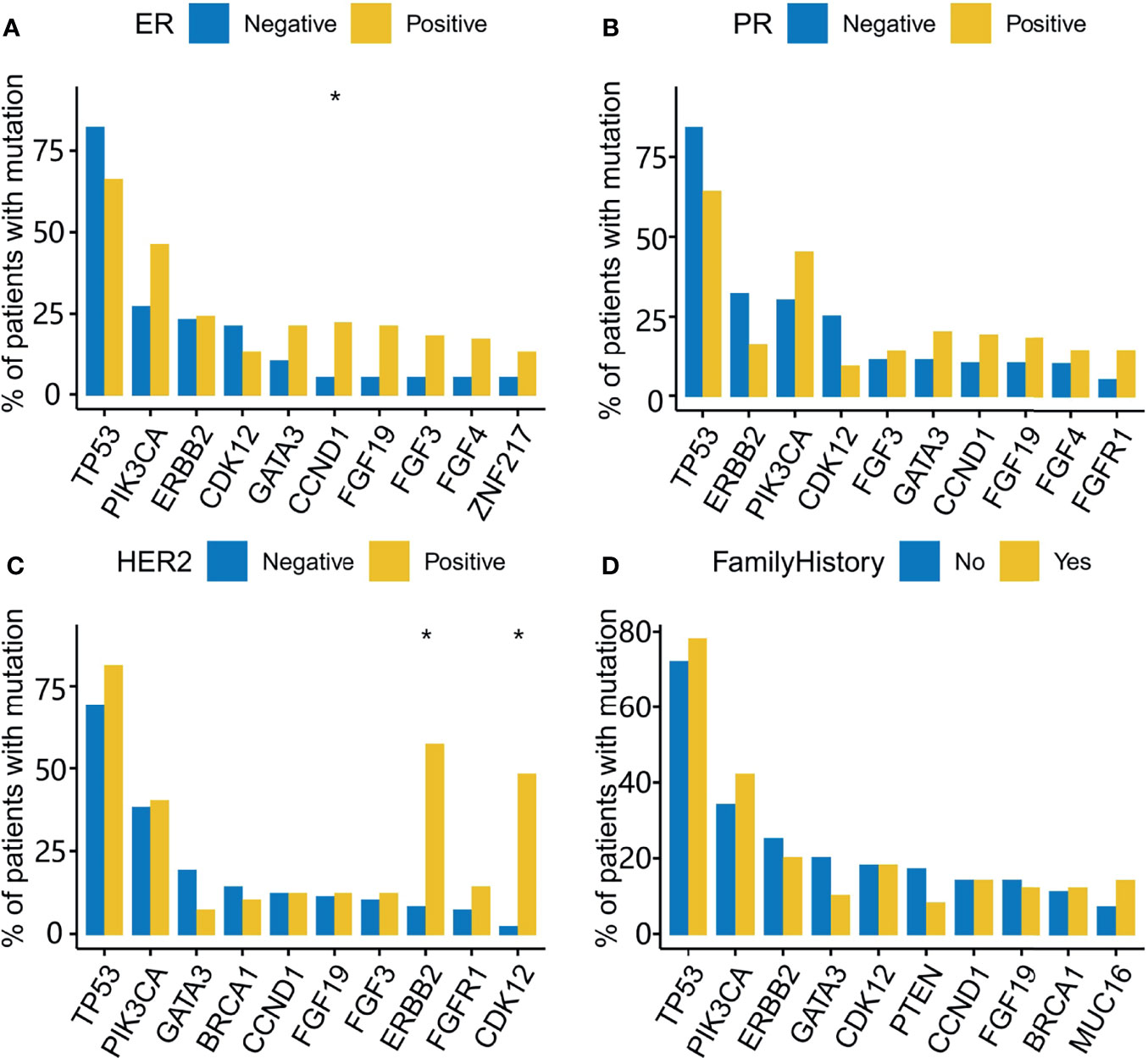
Figure 2 Gene mutations associated with ER, PR, HER2, and a family history of cancer (FH). The top 10 mutated genes are used in the analysis of mutational association with ER (A), PR (B), HER2 (C), and FH (D). The Y-axis indicates the percentage of patients with the mutated genes across the X-axis for receptor-positive and -negative groups. *, adjusted P-value<0.05.
The results of this study were contrasted with those of the MSK cohort (20). In the MSK cohort, ER+ and PR+ patients had a higher proportion of PIK3CA, CDH1, MAP3K1, AKT1, ESR1, and GATA3 mutations and a lower proportion of TP53 mutations (Supplementary Figures 2D, E); HER2+ patients had a higher proportion of TP53, NF1, and MLL2 and a lower proportion of CDH1, GATA3, and MAP3K1 (Supplementary Figure 2F). The luminal subtype had a higher proportion of PIK3CA, CDH1, GATA3, and MAP3K1 mutations and a lower proportion of TP53 mutations (Supplementary Figure 2G), and the HER2-enriched subtype had a higher proportion of TP53 and NF1 mutations and a lower proportion of GATA3 mutations (Supplementary Figure 2H). No significant mutations were found in the TNBC subtype (Supplementary Figure 2I).
To reduce mutational bias from gene length, genomic region, and patient characteristics, etc., MutSigCV was used to identify the significantly mutated genes. Five genes (TP53, PIK3CA, AKT1, PTEN, and GATA3) were found significantly mutated with an adjusted P-value less than 0.05. None of these genes was significantly associated with ER, PR, HER2, and FH.
Clustering Mutations
Testing each gene's mutation frequency by Fisher's exact test had a low power to find out significant genes because of multiple testing problem. To improve statistical power, a clustering method was employed in advance. The clustering procedure included three steps. First, the association between two mutations was calculated with Fisher’s exact test. Second, the resulting P-values were transformed into a similarity score between two genes as “-log10(P-value)”. Third, the similarity scores were used to hierarchically cluster genes with Euclidean distance and complete linkage (Figure 3). From the heatmap result, four clusters having a high association within them were marked with rectangles. Patients were classified into four corresponding groups if there is a gene mutation in each gene cluster. Mutations within each group of patients were annotated with KEGG pathways. A hypergeometrical test was used to infer the significance of enrichment (Figure 4). Interestingly, group 1 was enriched with signaling pathways regulating pluripotency of stem cells (21) (Figure 4A); group 2 was enriched with protein digestion and absorption pathway and extracellular matrix (ECM)–receptor interaction pathway (22) (Figure 4B); group 3 was enriched with hippo signaling pathway (23) (Figure 4C); and group 4 was enriched with VEGF signaling pathway (24) (Figure 4D).
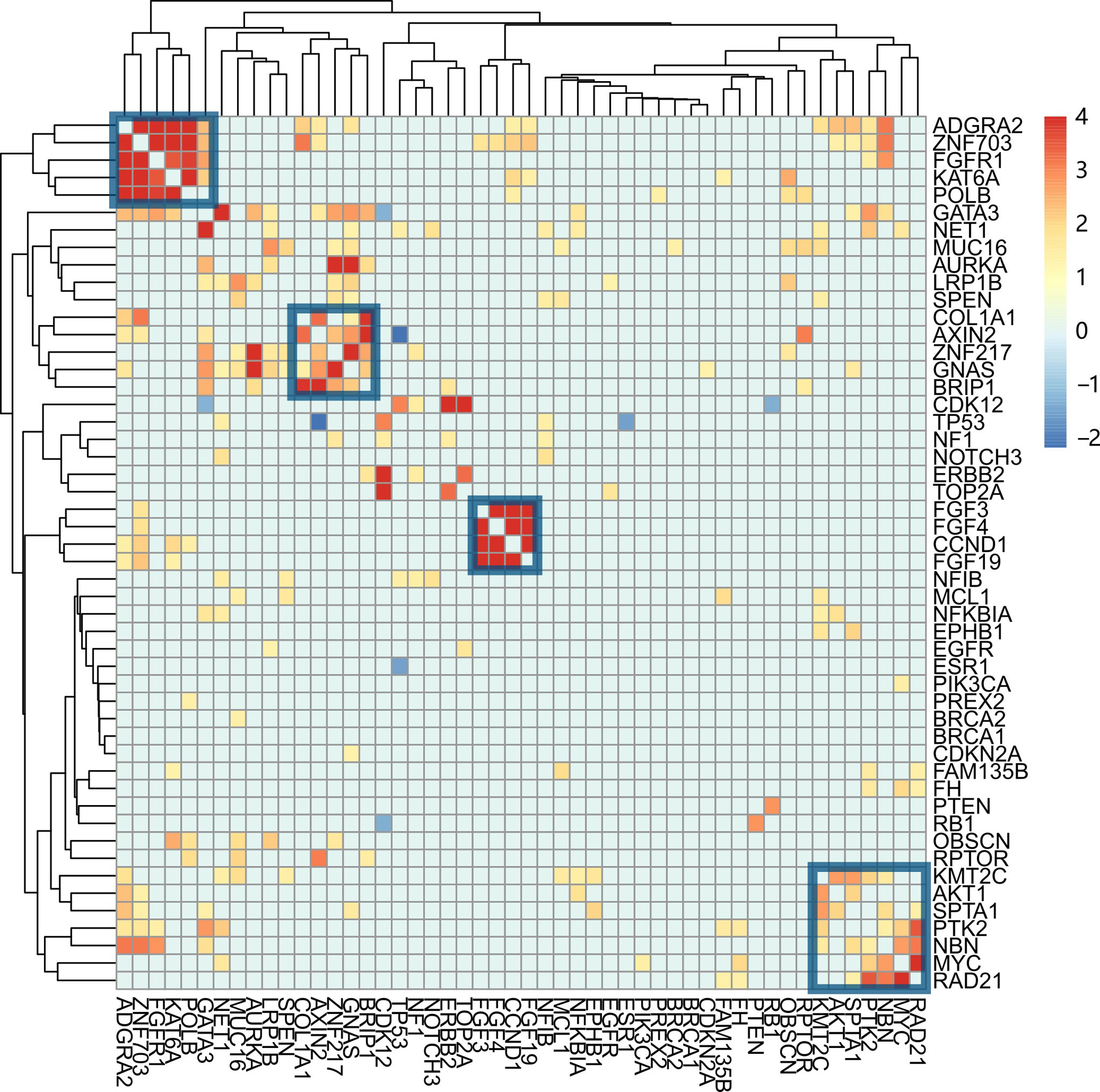
Figure 3 Co-mutation between genes and their association with clinical characteristics. The co-mutation association between each pair of mutations is calculated with Fisher’s exact test. The resulting P-value was transformed into a similarity score, -log10(P-value). Genes were hierarchically clustered with Euclidean distance and complete linkage. The four gene clusters are indicated with blue squares. From left to right, they are named clusters 1, 2, 3, and 4.
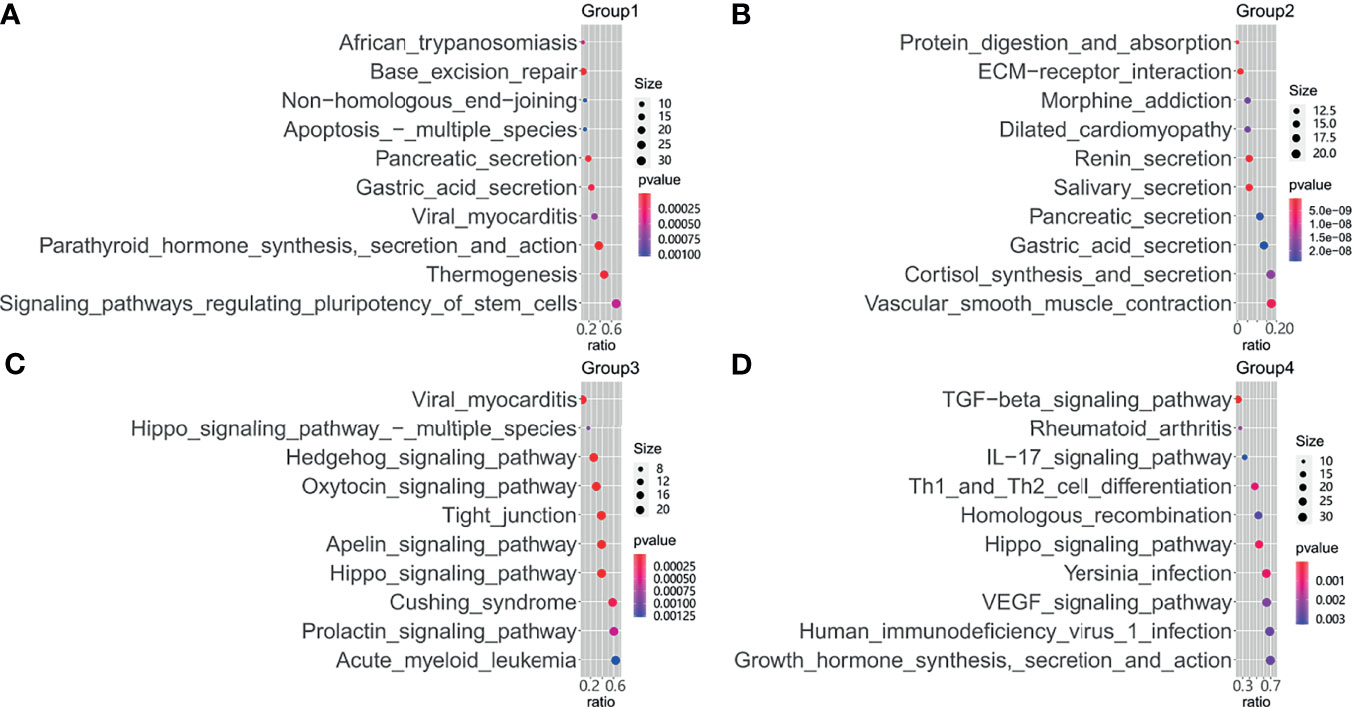
Figure 4 Functional enrichment of mutational genes. Enriched Kyoto Encyclopedia of Genes and Genomes (KEGG) pathways for mutated genes are plotted against the odds ratio in patient groups 1 (A), group 2 (B), group 3 (C), and group 4 (D).
Association of Mutational Clusters With Clinical Characteristics
The transformed P-value was calculated after Fisher’s exact test between the status of clinical characteristics and each group of patients (Figure 5A). Group 1 was significantly associated with PR status; groups 2 and 3 were significantly associated with ER status. Fisher’s exact test was also used to compare the gene cluster and FH status, which indicated that cluster 2 and cluster 4 were found negatively associated with a family history of cancer and age, respectively (Figure 5B). The association of breast cancer subtypes and TNM staging with mutation clusters is shown in Supplementary Figures 3A, B. No significant associations were found between them. We also analyzed the association between gene mutations and clinical characteristics in the MSK cohort. Cluster 2 showed a significant association with PR status.
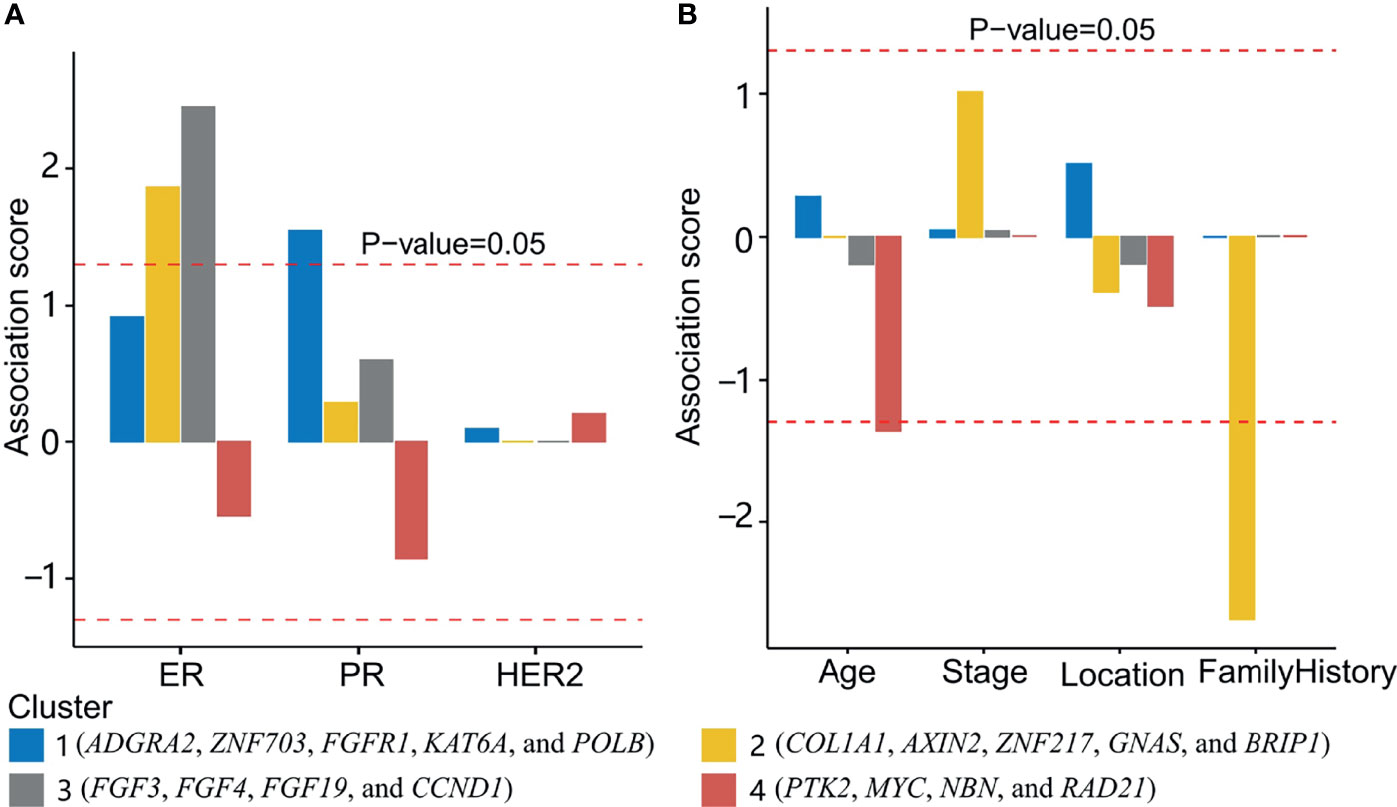
Figure 5 Association between gene clusters and clinical characteristics. (A) The association between gene clusters and three biomarkers (ER, PR, and HER2 status). Above the upper and below the lower red dashed lines indicated a positive and negative association with P-value <0.05, respectively. (B) The association of clusters 1, 2, 3, and 4 with age, stage, location (primary or metastatic), and a family history of cancer was shown. Cluster 2 and cluster 4 were found negatively associated with a family history of cancer and age, respectively.
Actionability of Mutational Gene Clusters
Mutations in the tumors of the four groups of patients were annotated with the OncoKB database (19). Actionability was classified into six levels according to the confidence in the evidence (Figure 6). Level_1 was of the highest evidence, and Level_4 was of the lowest evidence. The highest evidence level, Level_1, was the treatments with an FDA-recognized biomarker predictive of response to an FDA-approved drug, and the lowest evidence level, Level_4, was the treatments with compelling biological evidence that supports the biomarker as being predictive of response to a drug. Patient groups 1, 2, 3, and 4 had 24.1%, 36.5%, 38.7%, and 41.3% of patients with an FDA-recognized biomarker predictive of response to an FDA-approved drug, respectively (Figure 6). ERBB2 and PIK3CA were the most enriched actionable genes in groups 2, 3, and 4 with a high evidence level. Although the percentage of actionable mutations in FGFR1 was high in group 1, they had a low evidence level for majority of the patients.
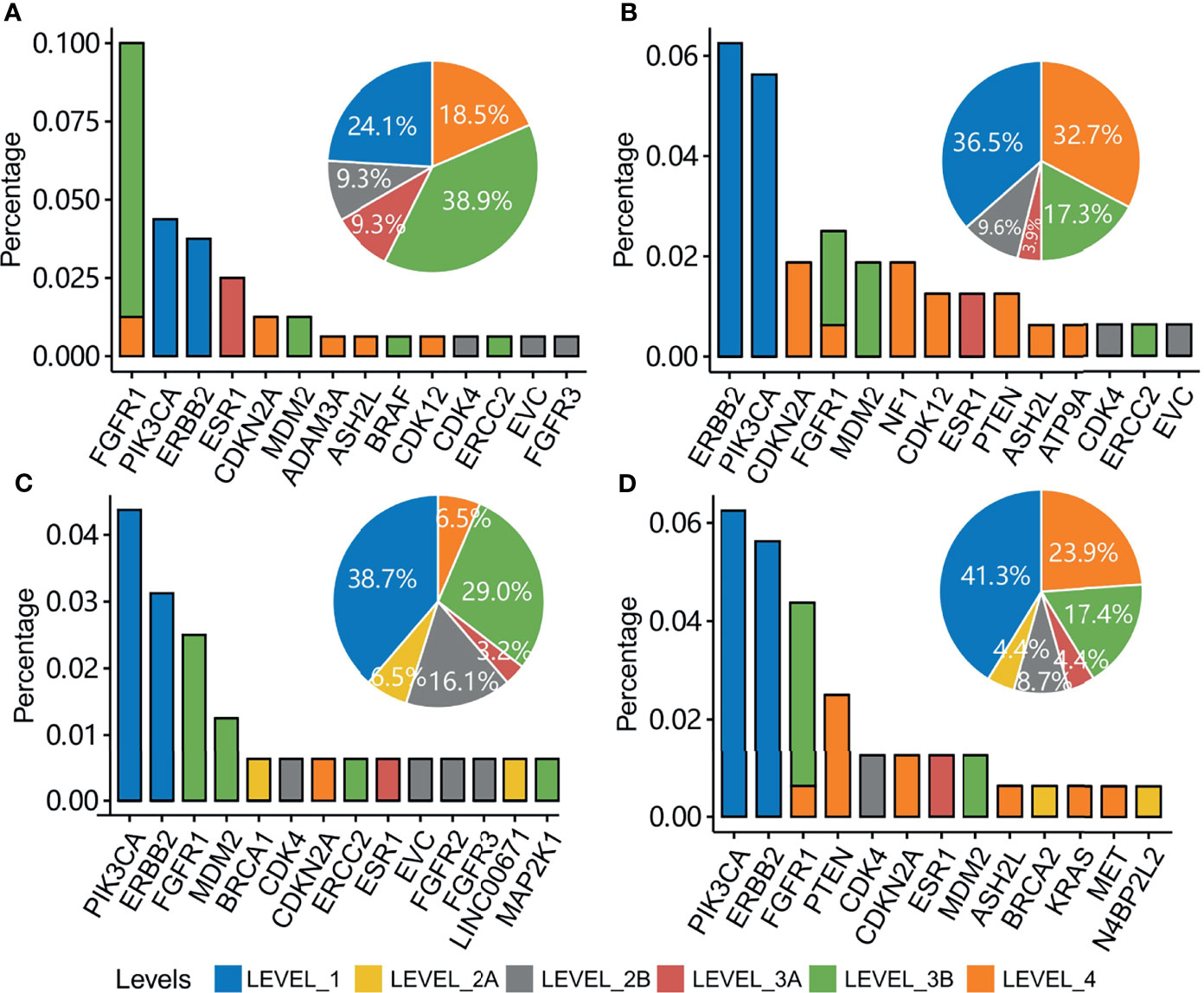
Figure 6 Clinical actionability of somatic alterations for patients with mutations in each patient group. Percentage of patients with actionable genes are shown in patient groups 1 (A), 2 (B), 3 (C), and 4 (D). Bin sizes in the pie plot indicate the related percentage of actionable levels in each patient group.
Discussion
The role of ER in the treatment of breast cancer can be traced back to Beatson’s work in 1896 (25), which found that ovariectomy could cause regression of metastatic breast cancer. Gradually, antiestrogen therapy became one of the cornerstones of breast cancer treatment. Patients with breast cancer were subtyped according to ER, PR, and HER2 status. With the recent development of genomics, mutations were found to play an important role in cancer development. For example, GATA3 mutation causes ESR1 ligand activation and leads to endocrine therapy resistance (26). However, research had been rare on the role of genomic mutations in the maintenance of ER, PR, and HER2 status. Moreover, familial history could also share some unknown gene mutations. In this study, we made an association study to find the possible candidate mutational genes associated with ER, PR, HER2, and FH status.
We first used gene-by-gene comparison methods to infer the associated genes. Only the top 10 genes were compared between different ER, PR, and HER2 statuses because only a small number of patients had mutated for the other genes. Three significantly mutated genes (CCND1, ERBB2, and CDK12) were found after adjusting multiple-comparison errors with the Benjamin and Hochberg method. MutSigCV did find five significantly mutated genes (TP53, PIK3CA, AKT1, PTEN, and GATA3). However, neither of them was associated with ER, PR, HER2, and FH status. The statistical power was low because of multiple-comparison errors. For example, discriminating FGFR1 between the PR+ and PR- groups will need about 900 patients per group. To reduce multiple-comparison times, genes were clustered first. Gene clustering was performed according to gene mutation status across samples. Fisher’s exact test was used to compare each pair of genes. A similarity score was inferred using the resulting P-value. Genes were clustered with similarity scores. Four clusters of genes with high within-cluster similarity were identified.
Cluster 1 was significantly associated with PR status and contained five genes (ADGRA2, ZNF703, FGFR1, KAT6A, and POLB). ZNF703 was found a common luminal B breast cancer oncogene (27). FGFR1 amplification was positively associated with luminal B breast cancer (28). In this work, FGFR1 mutations were either amplification (83.3%) or gene fusion (16.7%). POLB mutations were reported to be positively associated with PR expression in gastric cancer (29). However, no report was found about breast cancer. This cluster could be related to the maintenance of PR status.
Cluster 2 was significantly associated with ER status. Cluster 2 had five genes (COL1A1, AXIN2, ZNF217, GNAS, and BRIP1). A study showed that COL1A1 was positively associated with ER and PR expression (30). In this work, COL1A1 mutations were mostly gene amplification (72.7%) and gene fusion (9.0%). In ER+ breast cancer, ZNF217 worked as a positive enhancer of ER (31). In this work, 85% of ZNF217 mutations were gene amplification and gene fusion, which could bring about a higher expression of ZNF217.
Cluster 3 was positively associated with ER status. Four genes (FGF3, FGF4, FGF19, and CCND1) were included in this cluster. The co-amplification of FGF3, FGF4, FGF19, and CCND1was also observed in another pan-cancer study from the United States (32). The co-amplification of these genes was caused by large segmental duplication in chromosome 11q13. The coincidence of CCND1 with ER-positive breast cancer was also observed previously (7, 8).
Cluster 4 was not significantly associated with ER, PR, HER2, or FH status. This cluster consisted of four genes (PTK2, MYC, NBN, and RAD21). Most mutations in these genes were amplification. The amplification of PTK2, MYC, NBN, and RAD21 found prognostic biomarkers independent of breast cancer subtype (33). Such a phenomenon prompted the invalidity of ER, PR, and HER2 on the subtyping of these patients with breast cancer.
Family history could increase the risk of breast cancer. Indeed, the distribution of the cancers diagnosed in the family members of the 160 patients was not random. Lung cancer, liver cancer, breast cancer, endometrial cancer, and colorectal cancer were present in most of their family members. As far as is known, breast cancer, endometrial cancer, and colorectal cancer in any close family member could be risk factors for breast cancer (34–36). A higher percentage of breast cancer, endometrial cancer, and colorectal cancer in family members can be expected. To our surprise, the percentage of liver cancer was significantly higher than that of breast cancer. It may imply that liver cancer in any close family member could also be a risk factor for breast cancer. Lung cancer itself in the population was higher than other cancers. The high proportion of lung cancer in family members of patients with breast cancer could come from either the high background incidence rate or the association with breast cancer, which cannot be discriminated against using our current data.
Considering the high percentage of family history-related cancer, we were curious about the somatic gene mutations associated with FH. The rationale behind this hypothesis is that the similar genomic polymorphism and environment in the family members could cause a subtype of breast cancer associated with FH. However, no positively associated gene mutations or mutational clusters were found. There was only one negatively associated gene cluster (cluster 2). Although the significance of this cluster was still unclear, it shed light on the pathology of these Chinese patients with breast cancer.
The underlying etiology was predicted with mutational signature analysis using an R package “deconstructSigs” (37). The mutational spectrum in the tumors of the four patient groups was compared against COSMIC (Catalogue Of Somatic Mutations In Cancer) signatures (v2.0). For mutations in the tumors, patient groups 1 (Supplementary Figure 4A) and 2 (Supplementary Figure 4B) were more affected by signature 13 (most common in cervical and bladder cancers, attributed to the activity of the AID/APOBEC family), patient group 3 by signature 1 (endogenous mutational process initiated by spontaneous deamination of 5-methylcytosine) (Supplementary Figure 4C), and patient group 4 by signature 6 (defective DNA mismatch repair) (Supplementary Figure 4D).
In comparison to the MSK cohort, this cohort showed a different gene mutation spectrum when considering the breast cancer subtypes and ER, PR, and HER2 statuses (Supplementary Figure 5). It suggested that there were different mechanisms in the two populations. For example, this cohort had higher proportions of ERBB2 mutations in the HER2-enriched subtype but the MSK cohort had a similar mutation frequency between the HER2-enriched subtype and the other patients’ clinical characteristics. Such difference would lead to different treatment strategies in different populations.
Conclusion
Through the association study, we revealed that some genomic mutations and mutational clusters were significantly associated with ER and PR status. The identification of these genomic mutations could help to improve endocrine therapy.
Data Availability Statement
The original contributions presented in the study are included in the article/Supplementary Material. Further inquiries can be directed to the corresponding authors.
Ethics Statement
The studies involving human participants were reviewed and approved by the Research Ethics Committee of Tianjin Medical University Cancer Institute and Hospital. The patients/participants provided their written informed consent to participate in this study.
Author Contributions
ZT, YZ, and LL designed the study. CH, CW, NL, WZ, SL, LZ, WM, SW, and YZ performed the data collection and analysis. CH, ZT, YZ, and LL prepared the manuscript. All authors contributed to the article and approved the submitted version.
Funding
This work is supported by Tianjin Key Medical Discipline (Specialty) Construction Project and Tianjin Medical University Cancer Hospital "14th Five-Year" Peak Discipline Support Program Project. The funders have no role in study design, data collection and analysis, decision to publish, or preparation of the manuscript.
Conflict of Interest
YZ and LL are employees of Shanghai OrigiMed Co., Ltd.
The remaining authors declare that the research was conducted in the absence of any commercial or financial relationships that could be construed as a potential conflict of interest.
Publisher’s Note
All claims expressed in this article are solely those of the authors and do not necessarily represent those of their affiliated organizations, or those of the publisher, the editors and the reviewers. Any product that may be evaluated in this article, or claim that may be made by its manufacturer, is not guaranteed or endorsed by the publisher.
Supplementary Material
The Supplementary Material for this article can be found online at: https://www.frontiersin.org/articles/10.3389/fonc.2022.778511/full#supplementary-material
Supplementary Figure 1 | Cancer distribution in the family members of patients with breast cancer. (A) Relative proportion of patients with breast cancer whose family members have any type of cancer is indicated with the bin sizes. (B) The percentage of patients with breast cancer whose family members have any type of cancer is sorted along the x-axis.
Supplementary Figure 2 | Comparison of gene mutations with MSK cohort. Top mutated genes are compared between the patients with the subtypes of Luminal (A), HER2_enriched (B), and TNBC (C) in this study. In the MSK cohort, the difference between ER (D), PR (E), and HER2 (F) statuses are also shown. And top mutated genes are compared between the patients with the subtypes of Luminal (G), HER2_enriched (H), and TNBC (I). *, adjusted P-value <0.05; **, adjusted P-value <0.01; ***, adjusted P>-value <0.001.
Supplementary Figure 3 | The association between patient groups and breast cancer subtypes (Luminal, HER2, and TNBC). (A) The association between gene clusters and breast cancer subtypes is indicated with association scores. Association scores above the upper and below the lower red dashed lines indicate a positive and negative association with P-value<0.05, respectively. (B) Association between gene clusters and breast cancer TNM staging is indicated with association scores.
Supplementary Figure 4 | Deconstruct the signatures in the four groups of patients. The weights of COSMIC (Catalogue Of Somatic Mutations In Cancer) signatures are deconstructed from somatic mutations in the four patient groups using an R package “deconstructSigs”. There are 30 COSMIC signatures (v2.0). The relative importance of signatures is shown with a pie plot for the patient group 1 (A), 2 (B), 3 (C), and 4 (D). A larger area indicates higher importance.
Supplementary Figure 5 | The association between patient groups and clinical characteristics in the MSK cohort. The association between gene clusters and clinical characteristics is indicated with association scores in the MSK cohort. Association scores above the upper and below the lower red dashed lines indicate a positive and negative association with P-value<0.05, respectively.
Supplementary Table 1 | The gene list of sequencing panel.
References
1. Feng R-M, Zong Y-N, Cao S-M, Xu R-H. Current Cancer Situation in China: Good or Bad News From the 2018 Global Cancer Statistics? Cancer Commun (2019) 39(1):22. doi: 10.1186/s40880-019-0368-6
2. Sung H, Ferlay J, Siegel RL, Laversanne M, Soerjomataram I, Jemal A, et al. Global Cancer Statistics 2020: GLOBOCAN Estimates of Incidence and Mortality Worldwide for 36 Cancers in 185 Countries. CA A Cancer J Clin (2021) 71(3):209–49. doi: 10.3322/caac.21660
3. Williams F, Thompson E. Disparities in Breast Cancer Stage at Diagnosis: Importance of Race, Poverty, and Age. J Health Dispar Res Pract (2017) 10(3):34–45.
4. Seung SJ, Traore AN, Pourmirza B, Fathers KE, Coombes M, Jerzak KJ. A Population-Based Analysis of Breast Cancer Incidence and Survival by Subtype in Ontario Women. Curr Oncol (2020) 27(2):e191–8. doi: 10.3747/co.27.5769
5. Pearson A, Proszek P, Pascual J, Fribbens C, Shamsher MK, Kingston B, et al. Inactivating NF1 Mutations Are Enriched in Advanced Breast Cancer and Contribute to Endocrine Therapy Resistance. Clin Cancer Res (2020) 26(3):608–22. doi: 10.1158/1078-0432.CCR-18-4044
6. Tutt A, Tovey H, Cheang MCU, Kernaghan S, Kilburn L, Gazinska P, et al. Carboplatin in BRCA1/2-Mutated and Triple-Negative Breast Cancer BRCAness Subgroups: The TNT Trial. Nat Med (2018) 24(5):628–37. doi: 10.1038/s41591-018-0009-7
7. Lundberg A, Lindström LS, Li J, Harrell JC, Darai-Ramqvist E, Sifakis EG, et al. The Long-Term Prognostic and Predictive Capacity of Cyclin D1 Gene Amplification in 2305 Breast Tumours. Breast Cancer Res (2019) 21(1):34. doi: 10.1186/s13058-019-1121-4
8. Elsheikh S, Green AR, Aleskandarany MA, Grainge M, Paish CE, Lambros MBK, et al. CCND1 Amplification and Cyclin D1 Expression in Breast Cancer and Their Relation With Proteomic Subgroups and Patient Outcome. Breast Cancer Res Treat (2008) 109(2):325–35. doi: 10.1007/s10549-007-9659-8
9. Wolff AC, Hammond MEH, Hicks DG, Dowsett M, McShane LM, Allison KH, et al. Recommendations for Human Epidermal Growth Factor Receptor 2 Testing in Breast Cancer: American Society of Clinical Oncology/College of American Pathologists Clinical Practice Guideline Update. J Clin Oncol (2013) 31(31):3997–4013. doi: 10.1200/JCO.2013.50.9984
10. Chen X, Bu Q, Yan X, Li Y, Yu Q, Zheng H, et al. Genomic Mutations of Primary and Metastatic Lung Adenocarcinoma in Chinese Patients. J Oncol (2020) 2020:e6615575. doi: 10.1155/2020/6615575
11. Xu S, Guo Y, Zeng Y, Song Z, Zhu X, Fan N, et al. Clinically Significant Genomic Alterations in the Chinese and Western Patients With Intrahepatic Cholangiocarcinoma. BMC Cancer (2021) 21(1):152. doi: 10.1186/s12885-021-07792-x
12. Li H, Durbin R. Fast and Accurate Short Read Alignment With Burrows–Wheeler Transform. Bioinformatics (2009) 25(14):1754–60. doi: 10.1093/bioinformatics/btp324
13. Cibulskis K, Lawrence MS, Carter SL, Sivachenko A, Jaffe D, Sougnez C, et al. Sensitive Detection of Somatic Point Mutations in Impure and Heterogeneous Cancer Samples. Nat Biotechnol (2013) 31(3):213–9. doi: 10.1038/nbt.2514
14. Koboldt DC, Chen K, Wylie T, Larson DE, McLellan MD, Mardis ER, et al. VarScan: Variant Detection in Massively Parallel Sequencing of Individual and Pooled Samples. Bioinformatics (2009) 25(17):2283–5. doi: 10.1093/bioinformatics/btp373
15. Talevich E, Shain AH, Botton T, Bastian BC. CNVkit: Genome-Wide Copy Number Detection and Visualization From Targeted DNA Sequencing. PloS Comput Biol (2016) 12(4):e1004873. doi: 10.1371/journal.pcbi.1004873
16. Cao J, Chen L, Li H, Chen H, Yao J, Mu S, et al. An Accurate and Comprehensive Clinical Sequencing Assay for Cancer Targeted and Immunotherapies. Oncologist (2019) 24(12):e1294–302. doi: 10.1634/theoncologist.2019-0236
17. Lawrence MS, Stojanov P, Polak P, Kryukov GV, Cibulskis K, Sivachenko A, et al. Mutational Heterogeneity in Cancer and the Search for New Cancer-Associated Genes. Nature (2013) 499(7457):214–8. doi: 10.1038/nature12213
18. Reich M, Liefeld T, Gould J, Lerner J, Tamayo P, Mesirov JP. GenePattern 2.0. Nat Genet (2006) 38(5):500–1. doi: 10.1038/ng0506-500
19. Chakravarty D, Gao J, Phillips S, Kundra R, Zhang H, Wang J, et al. OncoKB: A Precision Oncology Knowledge Base. JCO Precis Oncol (2017) 1):1–16. doi: 10.1200/PO.17.00011
20. Razavi P, Chang MT, Xu G, Bandlamudi C, Ross DS, Vasan N, et al. The Genomic Landscape of Endocrine-Resistant Advanced Breast Cancers. Cancer Cell (2018) 34(3):427–438.e6. doi: 10.1016/j.ccell.2018.08.008
21. Koury J, Zhong L, Hao J. Targeting Signaling Pathways in Cancer Stem Cells for Cancer Treatment. Stem Cells Int (2017) 2017:2925869. doi: 10.1155/2017/2925869
22. Parker AL, Cox TR. The Role of the ECM in Lung Cancer Dormancy and Outgrowth. Front Oncol (2020) 10:1766. doi: 10.3389/fonc.2020.01766
23. Teoh SL, Das S. The Emerging Role of the Hippo Pathway in Lung Cancers: Clinical Implications. Curr Drug Targ (2017) 18(16):1880–92. doi: 10.2174/1389450117666160907153338
24. Frezzetti D, Gallo M, Maiello MR, D’Alessio A, Esposito C, Chicchinelli N, et al. VEGF as a Potential Target in Lung Cancer. Expert Opin Ther Targ (2017) 21(10):959–66. doi: 10.1080/14728222.2017.1371137
25. Beatson TG. Meeting IX.—May 20, 1896: On the Treatment of Inoperable Cases of Carcinoma of the Mamma: Suggestions for a New Method of Treatment, With Illustrative Cases (1896). Available at: https://www.ncbi.nlm.nih.gov/pmc/articles/PMC5518378/ (Accessed cited 2021 Aug 16).
26. Theodorou V, Stark R, Menon S, Carroll JS. GATA3 Acts Upstream of FOXA1 in Mediating ESR1 Binding by Shaping Enhancer Accessibility. Genome Res (2013) 23(1):12–22. doi: 10.1101/gr.139469.112
27. Holland DG, Burleigh A, Git A, Goldgraben MA, Perez-Mancera PA, Chin S-F, et al. ZNF703 Is a Common Luminal B Breast Cancer Oncogene That Differentially Regulates Luminal and Basal Progenitors in Human Mammary Epithelium. EMBO Mol Med (2011) 3(3):167–80. doi: 10.1002/emmm.201100122
28. Erber R, Rübner M, Davenport S, Hauke S, Beckmann MW, Hartmann A, et al. Impact of Fibroblast Growth Factor Receptor 1 (FGFR1) Amplification on the Prognosis of Breast Cancer Patients. Breast Cancer Res Treat (2020) 184(2):311–24. doi: 10.1007/s10549-020-05865-2
29. Tan X, Wu X, Ren S, Wang H, Li Z, Alshenawy W, et al. A Point Mutation in DNA Polymerase β (POLB) Gene Is Associated With Increased Progesterone Receptor (PR) Expression and Intraperitoneal Metastasis in Gastric Cancer. J Cancer (2016) 7(11):1472–80. doi: 10.7150/jca.14844
30. Liu J, Shen J-X, Wu H-T, Li X-L, Wen X-F, Du C-W, et al. Collagen 1a1 (COL1A1) Promotes Metastasis of Breast Cancer and Is a Potential Therapeutic Target. Discov Med (2018) 25(139):211–23.
31. Nguyen NT, Vendrell JA, Poulard C, Győrffy B, Goddard-Léon S, Bièche I, et al. A Functional Interplay Between ZNF217 and Estrogen Receptor Alpha Exists in Luminal Breast Cancers. Mol Oncol (2014) 8(8):1441–57. doi: 10.1016/j.molonc.2014.05.013
32. Schrock AB, Miller VA, Alexander BM, Ross JS, Chung J, Ali SM. Pan-Cancer Genomic Landscape of the Cyclin D1/FGF3,4,19 (11q13) Amplicon Including Associations With HPV Status, and ESR1 and AR Alterations. Ann Oncol (2019) 30:v29. doi: 10.1093/annonc/mdz239.012
33. Garrido-Castro AC, Spurr LF, Hughes ME, Li YY, Cherniack AD, Kumari P, et al. Genomic Characterization of De Novo Metastatic Breast Cancer. Clin Cancer Res (2021) 27(4):1105–18. doi: 10.1158/1078-0432.CCR-20-1720
34. Shiyanbola OO, Arao RF, Miglioretti DL, Sprague BL, Hampton JM, Stout NK, et al. Emerging Trends in Family History of Breast Cancer and Associated Risk. Cancer Epidemiol Biomarkers Prev (2017) 26(12):1753–60. doi: 10.1158/1055-9965.EPI-17-0531
35. Turati F, Edefonti V, Bosetti C, Ferraroni M, Malvezzi M, Franceschi S, et al. Family History of Cancer and the Risk of Cancer: A Network of Case–Control Studies. Ann Oncol (2013) 24(10):2651–6. doi: 10.1093/annonc/mdt280
36. Teerlink CC, Albright FS, Lins L, Cannon-Albright LA. A Comprehensive Survey of Cancer Risks in Extended Families. Genet Med (2012) 14(1):107–14. doi: 10.1038/gim.2011.2
Keywords: estrogen receptor, progesterone receptors, human epidermal growth factor receptor 2, genomic mutations, a family history of cancer
Citation: Hao C, Wang C, Lu N, Zhao W, Li S, Zhang L, Meng W, Wang S, Tong Z, Zeng Y and Lu L (2022) Gene Mutations Associated With Clinical Characteristics in the Tumors of Patients With Breast Cancer. Front. Oncol. 12:778511. doi: 10.3389/fonc.2022.778511
Received: 17 September 2021; Accepted: 14 March 2022;
Published: 14 April 2022.
Edited by:
Luis E. Arias-Romero, National Autonomous University of Mexico, MexicoReviewed by:
Sandhya Annamaneni, Osmania University, IndiaGabriela Figueroa González, Universidad Nacional Autónoma de México, Mexico
Ernesto Rojas, Dana Farber Cancer Institute, United States
Claudia Fabiola Mendez-Catala, UNAM, Mexico
Copyright © 2022 Hao, Wang, Lu, Zhao, Li, Zhang, Meng, Wang, Tong, Zeng and Lu. This is an open-access article distributed under the terms of the Creative Commons Attribution License (CC BY). The use, distribution or reproduction in other forums is permitted, provided the original author(s) and the copyright owner(s) are credited and that the original publication in this journal is cited, in accordance with accepted academic practice. No use, distribution or reproduction is permitted which does not comply with these terms.
*Correspondence: Zhongsheng Tong, dG9uZ3pob25nc2hlbmdAdGptdWNoLmNvbQ==; Yanwu Zeng, emVuZ3l3QG9yaWdpbWVkLmNvbQ==; Leilei Lu, bHVsbEBvcmlnaW1lZC5jb20=