- 1Department of Biochemistry and Molecular Biology and Zhejiang Key Laboratory of Pathophysiology, School of Basic Medical Sciences, Health Science Center, Ningbo University, Ningbo, China
- 2Department of Gastroenterology, The Affiliated No. 1 Hospital, Ningbo University, Ningbo, China
- 3Institute of Digestive Diseases of Ningbo University, Ningbo, China
Background: The transition from a healthy gastric mucosa to gastric cancer is a multi-step process. Early screening can significantly improve the survival rate of gastric cancer patients. A reliable liquid biopsy for gastric cancer prediction is urgently needed and since tRNA-derived fragments (tRFs) are abundant in various body fluids, tRFs are possible new biomarkers for gastric cancer.
Methods: A total of 438 plasma samples from patients with different gastric mucosal lesions as well as healthy individuals were collected. A specific reverse transcription primer, a forward primer, a reverse primer, and a TaqMan probe were designed. A standard curve was constructed and an absolute quantitation method was devised for detection of tRF-33-P4R8YP9LON4VDP in plasma samples of individuals with differing gastric mucosa lesions. Receiver operating characteristic curves were constructed to evaluate the diagnostic values of tRF-33-P4R8YP9LON4VDP for individual with differing gastric mucosa. A Kaplan–Meier curve was established to calculate the prognostic value of tRF-33-P4R8YP9LON4VDP for advanced gastric cancer patients. Finally, a multivariate Cox regression analysis was performed to assess the independent prognostic value of tRF-33-P4R8YP9LON4VDP for advanced gastric cancer patients.
Results: A detection method for plasma tRF-33-P4R8YP9LON4VDP was successfully established. Levels of plasma tRF-33-P4R8YP9LON4VDP were shown to reflect a gradient change from healthy individuals to gastritis patients to early and advanced gastric cancer patients. Significant differences were found among individuals with differing gastric mucosa, with reduced levels of tRF-33-P4R8YP9LON4VDP significantly related to a poor prognosis. tRF-33-P4R8YP9LON4VDP was found to be an independent predictor of an unfavorable survival outcome.
Conclusions: In this study, we developed a quantitative detection method for plasma tRF-33-P4R8YP9LON4VDP that exhibited hypersensitivity, convenience, and specificity. Detection of tRF-33-P4R8YP9LON4VDP was found to be a valuable means by which to monitor different gastric mucosa and to predict patient prognosis.
Introduction
Gastric cancer ranks fourth in lethality among cancer-related deaths (1). A lack of obvious discomfort at the early stages of the disease is the principal reason for the low-survival rate among patients with gastric cancer. If the tumor is diagnosed and treated at a localized stage, the prognosis is improved. Using reliable liquid biopsy analysis to detect cancer-related biomarkers is convenient and non-invasive for cancer screening. Therefore, a novel biomarker that is appropriate for discriminating gastric cancer patients from healthy individuals is clinically important.
Researchers have recognized that small RNAs are crucial players in a range of biological processes (2, 3). tRNA-derived fragments (tRFs) are fragments derived from mature tRNAs or pre-tRNAs (4). Based on the cleavage site of tRNAs, tRFs can be grouped into 3’ UtRF, 5’-tRFs, 3’-tRFs, i-tRFs, 5’-tRNA halves (5’-tRHs), and 3’-tRHs (5). tRFs are involved in the pathophysiology of various diseases. For example, tRFVal promotes gastric cancer progression via interaction with the chaperone, EEF1A1, which results in the degradation of P53 in a ubiquitin-dependent manner (2). Further, tRF3008A attenuates progression of colorectal cancer by destabilizing Forkhead Box K1 (FOXK1) in an Argonaute (AGO)-dependent manner (3).
Emerging studies have found tRFs to be abundant in various body fluids (6, 7) and dysregulated in various diseases (8–10), which suggests that tRFs may be promising biomarkers. Our team is interested in the evaluation of tRFs as biomarkers for gastric cancer. In a previous study, we found that relative to healthy individuals, gastric cancer patients had dysregulated tRF plasma profiles (11). Herein, one of these dysregulated tRFs, tRF-33-P4R8YP9LON4VDP (tRF-33), was assessed by an absolute quantification method, for its diagnostic potential as a plasma screening method for detection of patients with differing gastric mucosal lesions.
Materials and methods
Plasma samples
A total of 438 plasma samples were assessed, including 106 pairs of pre- and post-operative plasma samples from patients with advanced gastric cancer, 72 gastritis patients, 48 early gastric cancer patients, and 106 healthy individuals were obtained from September 2018 to October 2021. Peripheral venous blood samples were collected and centrifuged at 3000 rpm, with obtained plasma stored at -80°C before RNA extraction. Gastric mucosal lesions were identified by pathology.
The clinicopathologic data of advanced gastric cancer patients were collected. Participants with gastric cancer were not treated with antitumor agents, radiotherapy, or immuno-therapy. Moreover, patients with other malignant tumors, infectious diseases, or serious systemic diseases were excluded. All of the clinical diagnoses were confirmed by pathological examination. The study was approved by the Human Research Ethics Committee of Ningbo University (No. 2019022501). All of the participants enrolled provided signed written informed consent.
Isolation of total RNA and reverse transcription
The total RNA from 250 or 500 µL of plasma was first extracted with TRIzol LS (Invitrogen, Germany). Then, 8 µL of enzyme-free water was added to dissolve the extracted RNA. The concentration and purity of the total RNA were determined with a NanoDrop ultraviolet spectrophotometer (Thermo Fisher Scientific, Wilmington, DE, USA). A260/A280 ratio of 1.8 – 2.1 was considered acceptable.
For the reverse transcription of tRF-33, a specific stem-loop reverse transcription (RT) primer (5’-ACAGACGAGGGTACCTCCTCTCTTCTCTACTCGTGTCCTACCCTCGTCTGTCAGGCG-3’; Figure 1A) and cDNA synthesis kits (Tiosbio, Beijing, China) were used. The reaction system included an RT master mix with a double-stranded specific nuclease, RT primer, total RNA solution, and enzyme-free water. The reaction temperature was 37°C for 30 min and then 85°C for 5 min.
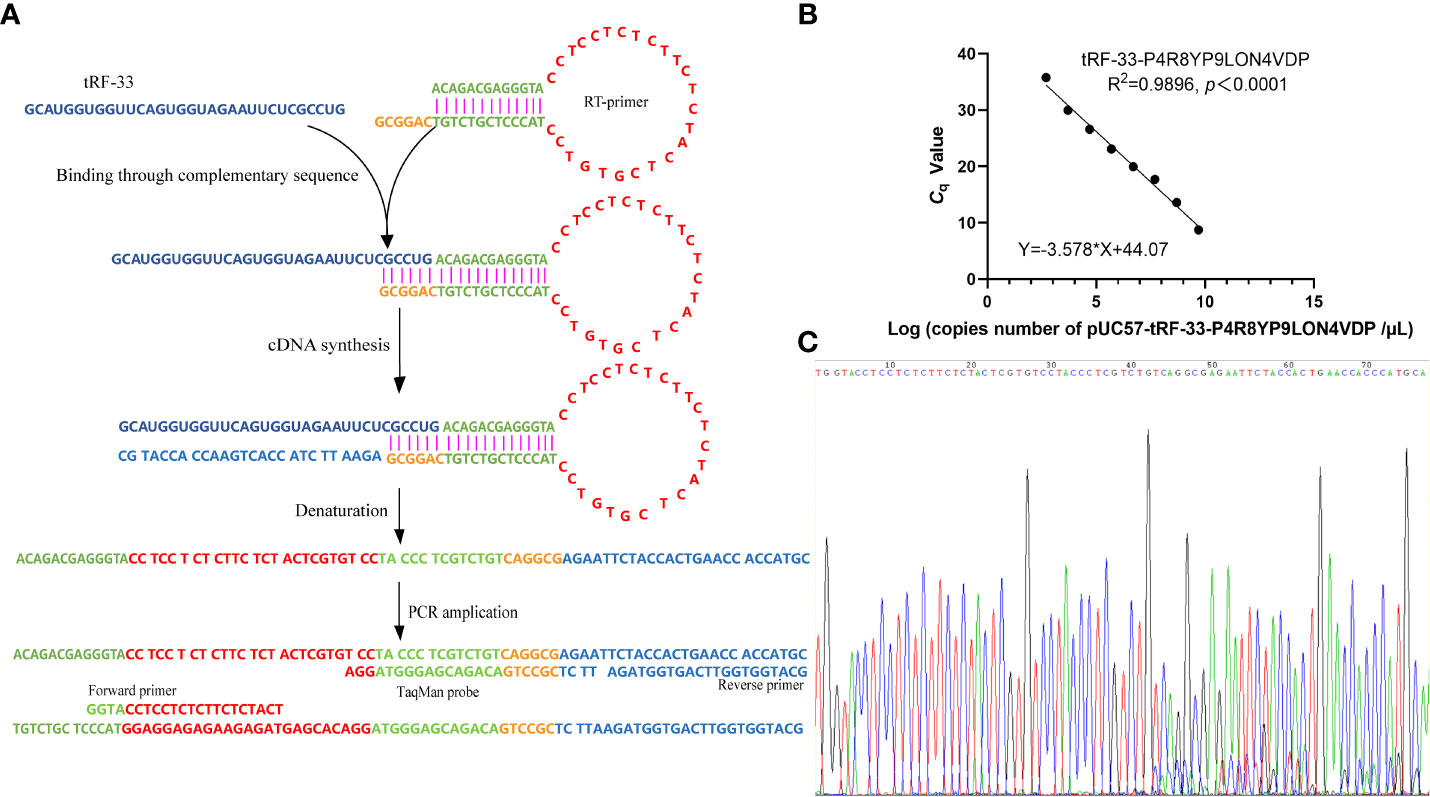
Figure 1 Establishment of the detection method for tRF-33-P4R8YP9LON4VDP in plasma. (A) Schematic representation of the method for detection of tRF-33. (B) The formula for the standard curve. (C) The T–A cloning sequencing results of the qRT-PCR product of tRF-33-P4R8YP9LON4VDP.
Quantitative PCR detection based on TaqMan probe
A quantitative real-time polymerase chain reaction (qPCR) was performed using a 5G qPCR Premix (Toroivd, Shanghai, China) containing dUTP, Mg2+, DNA polymerase, and PCR buffer. The reaction system contained 10 µL of the 5G qPCR Premix, 0.8 µL of the cDNA, 1.4 µL of the forward primer, 1.4 µL of the reverse primer, 0.8 µL of the TaqMan probe, and 5.6 µL of enzyme-free water. The reaction mixtures were then incubated in accordance with the instructions of the manufacturer. The primers are listed in Figure 1A and include: 5’-GGTACCTCCTCTCTTCTCTACT-3’ (Forward primer), 5’-GCATGGGTGGTTCAGTGGTAGA-3’ (Reverse primer), and 5’-FAM-TTCTCGCCTGACAGACGAGGGTAGGA-TAMRA-3’ (TaqMan probe).
Construction of the standard curve for the quantification of tRF-33-P4R8YP9LON4VDP (tRF-33)
To detect the tRF-33 amount in plasma, the qRT-PCR product of tRF-33 was first inserted into plasmid pUC57 (General Biology, Anhui, China), constructing the recombinant plasmid pUC57-tRF-33. Then, eight concentration gradients, based on the initial concentration of the plasmid DNA solution (150 ng/µL) were prepared. The number of copies of the diluted plasmid DNA were calculated based on the formula: 6.02×1023×concentration of plasmid DNA solution (ng/µL)/(660×2783). Then, the lg (number of copies) of the eight diluted plasmid DNA concentrations were determined by transformation. The Cq values of the eight diluted plasmid DNA concentrations were determined by qPCR. According to the lg (number of copies) and the corresponding Cq values, a regression curve was constructed.
For the detection of plasma samples, the Cq value of each sample was achieved by absolute quantification. The number of plasma copies of tRF-33 were calculated based on the standard curve (Figure 1B) and the concentration of plasma tRF-33 (copies/mL) on the volume of total RNA.
Statistical analysis
Data analyses were performed with GraphPad Prism v8.0 (San Diego, CA, USA) and SPSS Statistics Version 18.0 (Chicago, IL, USA). Simple linear regression was used to construct the standard curve with lg (number of copies) and the corresponding Cq values. The data of tRF-33 levels, expressed as median with interquartile, were inconsistent and fitted abnormal distribution. Tamhane′s T2 and Dunnett′s T3 were used for the analysis of inconsistent data. Diagnostic values were analyzed by constructing receiver operating characteristic (ROC) curves. A Kaplan–Meier curve was used to evaluate prognostic performance, followed by univariate and multivariate Cox proportional regression analyses.
Results
Detection method for tRF-33-P4R8YP9LON4VDP amount in plasma
Since tRF-33 is a short RNA of 33 nt, a hairpin RT primer was designed. The loop part of the RT primer was designed to form a discontinuous complementary sequence of 5’-ACAGACGAGGGTA-3’ and 5’-TACCCTCGTCTGT-3’ to form a stem-loop, which was connected to tRF-33 by a hydrogen bond through its 3’-sticky end (5’-CAGGCG-3’) during transcription (Figure 1A). Then, forward and reverse primers as well as the TaqMan probe were added (Figure 1A). The sequencing results of the qRT-PCR product confirmed the specificity of the primers (Figure 1C).
To detect the absolute amount of tRF-33 in plasma, a standard curve (Y = −3.578 × X + 44.07) was constructed based on the eight diluted plasmid DNA concentrations and the corresponding Cq values (Figure 1B). The correlation coefficient was 0.9896, indicating a good linear relationship for the range of plasmid DNA concentrations.
To investigate the diagnostic significance of tRF-33 in gastric cancer, we detected the tRF-33 abundance in the plasma samples from patients and healthy individuals. The amount of tRF-33 in the plasma of patients with advanced gastric cancer was lower than that of healthy individuals (p < 0.001) or patients with gastritis (p < 0.05) (Figure 2A). Moreover, tRF-33 also showed lower level in early gastric cancer patients than that of healthy individuals (p < 0.001) or patients with gastritis (p < 0.05). The results suggest the potential value of tRF-33 for identification of different gastric pathologic states. However, no statistical significance was observed between the early and advanced gastric cancer patients, or healthy controls and gastritis patients. To assess the usefulness of tRF-33 for monitoring the post-operative condition of advanced gastric cancer patients, we assessed the amount of tRF-33 in paired pre-and post-operative plasma samples, however, no statistical significance was found in the paired pre-and post-operative plasma samples (Figure 2A). The reason might be due to small sample size and over-dispersed data distribution.
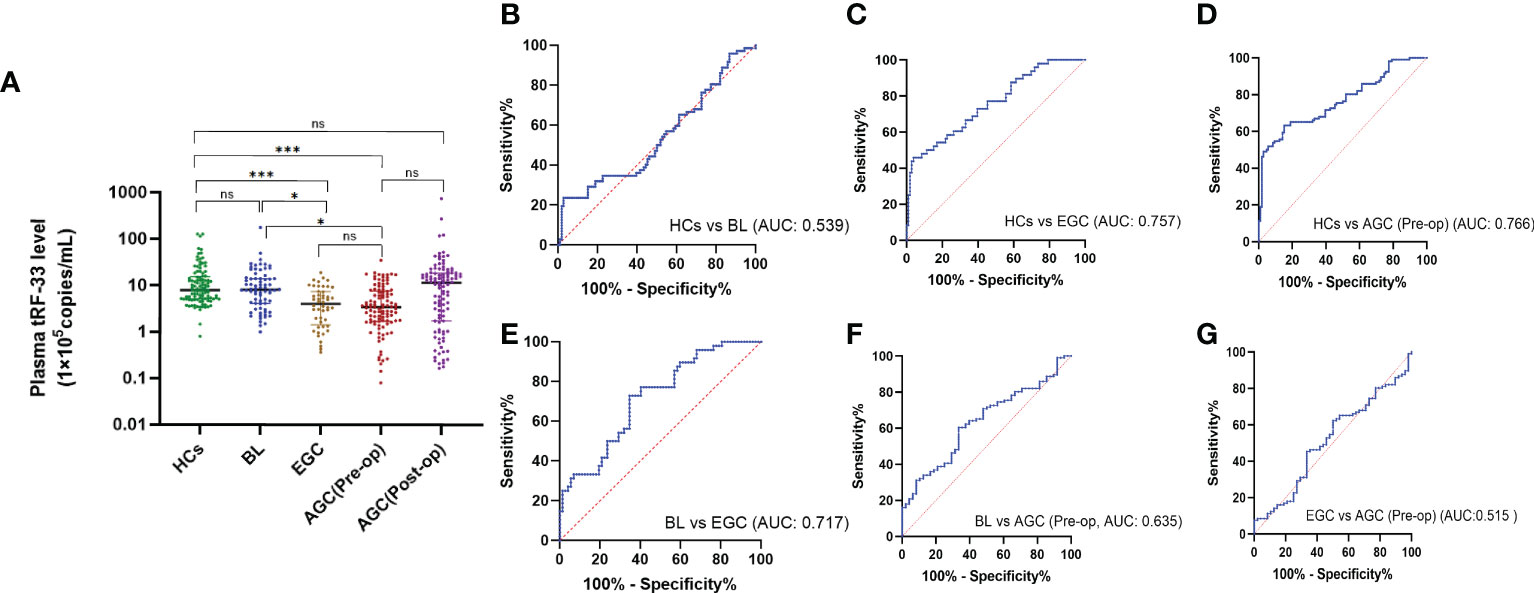
Figure 2 Expression levels and diagnostic values of tRF-33-P4R8YP9LON4VDP in different cohorts. (A) The amount of tRF-33 in the plasma showed a gradient change in healthy individuals (HC, n = 106), benign lesion (BL, n = 72), early gastric cancer (EGC, n = 48), pre-operative advanced gastric cancer [AGC (Pre-op), n = 106), and the paired post-operative plasma of advanced gastric cancer patients [AGC (Post-op), n = 106]. *p < 0.05, ***p < 0.001, ns, no significance. Tamhane′s T2 and Dunnett′s T3 were used. (B) Receiver operating characteristic (ROC) curve analysis of tRF-33 for healthy individuals and patients with benign lesions. (C) ROC curve analysis of tRF-33 for healthy individuals and early gastric cancer patients. (D) ROC curve analysis of tRF-33 for healthy individuals and advanced gastric cancer patients. (E) ROC curve analysis of tRF-33 for patients with benign lesion and early gastric cancer patients. (F) ROC curve analysis of tRF-33 for patients with benign lesion and advanced gastric cancer patients. (G) ROC curve analysis of tRF-33 for early and advanced gastric cancer patients. AUC, area under ROC curve.
We evaluated relationships among plasma tRF-33 level and clinicopathologic parameters of advanced gastric cancer patients. First, based on cut-off value, the patients were grouped into a tRF-33 low-level group (n = 52) and a high-level group (n = 54). As shown in Table 1, low plasma levels of tRF-33 were related to poor tumor differentiation grade, bigger tumor size, and higher carbohydrate antigen 125 (CA125) levels in advanced gastric cancer patients. There were no relationships with other parameters such as patient age, gender, carcinoembryonic antigen (CEA), α-fetoprotein (AFP), CA199 levels, tumor-node-metastasis (TNM) stage, tumor invasion degree, or distant metastasis (Table 1).
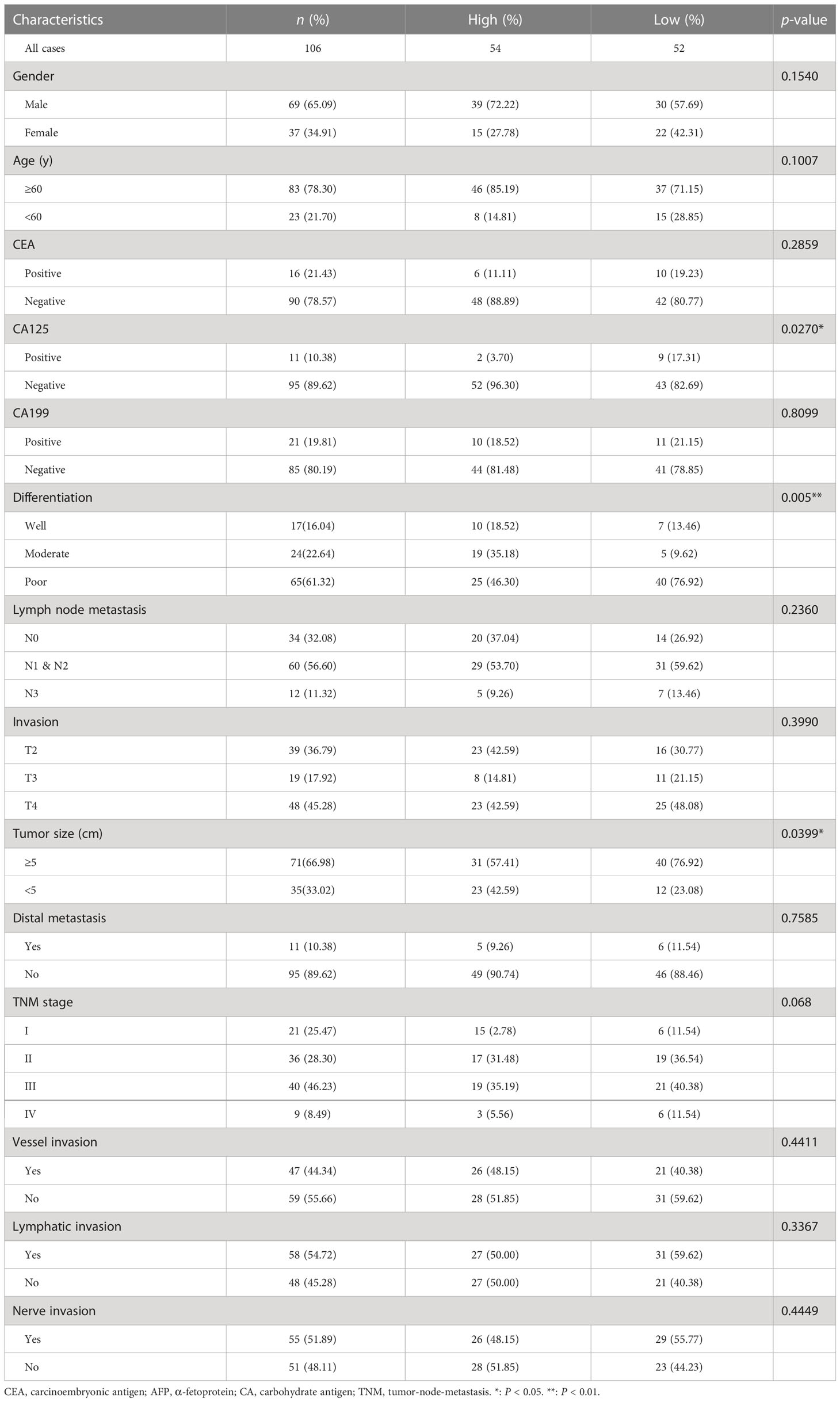
Table 1 The correlation between the amount of tRF-33-P4R8YP9LON4VDP in plasma and clinicopathological features of gastric cancer patients.
Clinical diagnostic significance of tRF-33-P4R8YP9LON4VDP
To assess the diagnostic monitoring potential of tRF-33 for different gastric pathologic states, we plotted receiver operating characteristic (ROC) curves for different groups of patients. As shown in (Figure 2) and Table 2, the area under the ROC curve (AUC) was 0.757 (p < 0.0001), differentiating between early gastric cancer patients and healthy individuals (Figure 2C). At the cut-off value of 334748, tRF-33 demonstrated 45.8% sensitivity, 96.2% specificity, 92.4% positive predictive value (PPV), and a 64.0% negative predictive value (NPV) (Table 2). We also found that tRF-33 was effective in distinguishing advanced gastric cancer patients from healthy individuals (AUC: 0.766, p < 0.0001) (Figure 2D; Table 2). Further, tRF-33 also differentiated early or advanced gastric cancer patients from gastritis patients (AUC: 0.717, 0.635, respectively; Figures 2E, F; Table 2). However, tRF-33 did not significantly differentiate advanced gastric cancer patients from early gastric cancer patients, or gastritis patients from healthy controls (Figures 2B, G). These results demonstrate tRF-33 to be efficient at differentiation of gastric cancer patients from healthy individuals and gastritis patients.
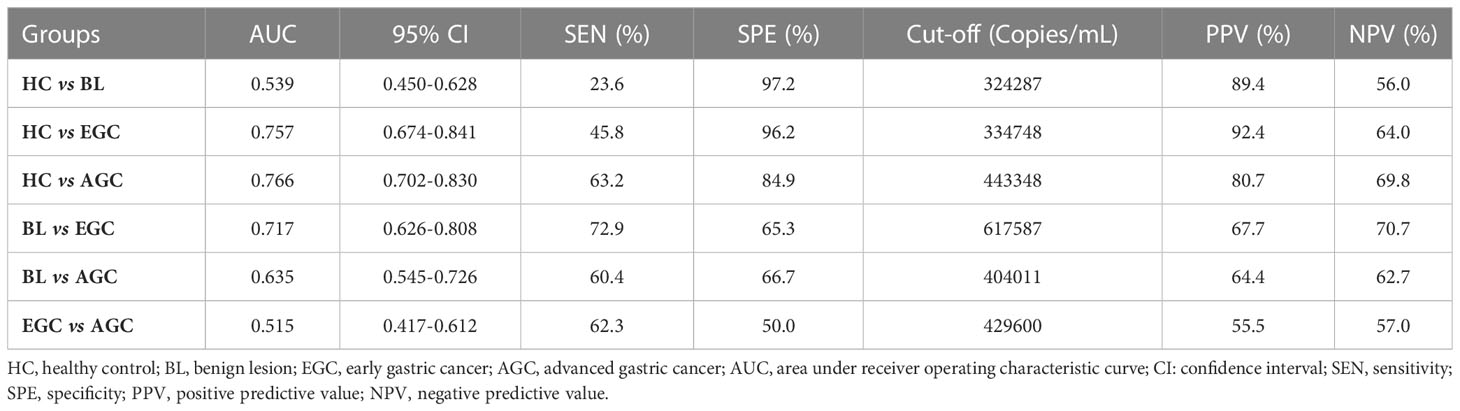
Table 2 The diagnostic value of tRF-33-P4R8YP9LON4VDP in monitoring gastric mucosa of different pathological states.
Relationship of reduced levels of tRF-33-P4R8YP9LON4VDP with an unfavorable prognosis
Data for survival and follow-up were available for most of the study participants, however two patients who were lost to follow-up. Patient death was used as the clinical endpoint event for calculation of overall survival (OS), with an average approximating 21 months. By comparison of Kaplan–Meier curves, patients with reduced levels of tRF-33 had poorer survival expectancy than those with higher tRF-33 levels (p<0.001; Figure 3A). Univariate Cox regression analysis demonstrated a relationship between plasma tRF-33 level and poor survival (HR: 0.367, 95% CI: 0.189–0.713, p< 0.01; Figure 3B). Moreover, multivariate Cox analysis demonstrated tRF-33 down-regulation to predict an unfavorable prognosis for advanced gastric cancer patients (HR: 0.371, 95% CI: 0.139–0.996; p<0.05; Figure 3C), independent of other tumor markers such as; differentiation degree, tumor size, TNM stage, tumor invasion degree, or distant metastasis.
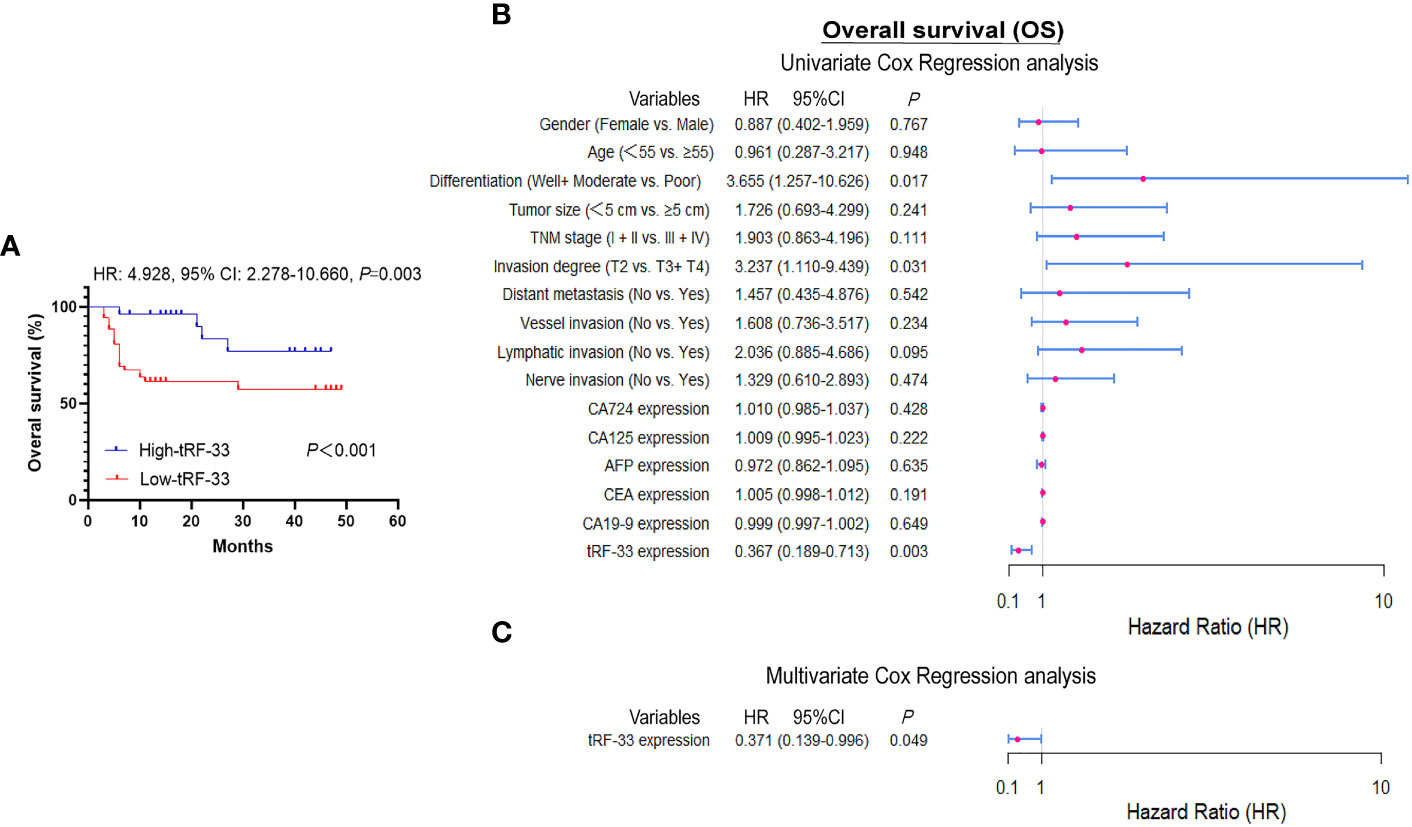
Figure 3 Diagnostic values of tRF-33-P4R8YP9LON4VDP. (A) Kaplan–Meier survival curve based on tRF-33 levels and overall survival (OS) of advanced gastric cancer (AGC) patients. (B, C) Forest plots of the univariate and multivariate Cox proportional regression analysis for OS in the AGC cohort.
Discussion
More than 76 million gastric cancer-related deaths have been reported and more than one million newly diagnosed gastric cancer have been reported on a yearly basis (1). According to recent GLOBOCAN statistics, the occurrence of gastric cancer varies widely in different regions. East Asians have the highest incidence, with 32.5 new cases per 10 million male residents and 13.2 new cases per 10 million female residents (1). Because of the silent symptoms of early gastric cancer, most patients are identified at an advanced stage (12). Identification of asymptomatic individuals suffering from gastric cancer is dependent upon the method of screening. Upper gastrointestinal endoscopy followed by pathologic assessment is the gold standard for gastric cancer discrimination. However, the risks for hemorrhage and perforation make this approach less desirable. With poor sensitivity and specificity for the commonly used tumor markers for gastric cancer prediction (13), there is an urgent need to develop novel gastric cancer biomarkers that improve early gastric cancer detection.
tRFs were first discovered in the1970s in the urine of cancer patients (14). In recent decades, tRFs have been identified as functional non-coding RNAs (ncRNAs) (4). Many studies have demonstrated tRFs to be ubiquitous in all domains of organisms (15–17) and to participate in various biological processes by interaction with diverse proteins or RNAs, regulating gene expression (2, 18). Moreover, accumulating evidence has shown differential tRF profiles in tumor tissues and body fluids from cancer patients (3, 19–21). Furthermore, several studies have shown that some tRFs are tissue-specific (22, 23). For example, Torres et al. demonstrated tRNAArgCCT derived 5’-tRFArgCCT to be specifically detected in the brain but not in other tissue such as testis, ovary, heart, skeletal muscle, or liver. In contrast, tRNAGlnCTG derived 3’-tRF GlnCTG was found in heart, skeletal muscle, and liver but not in the brain (23). These results suggest the possibility that tRFs may be markers or molecular targets for specific cancers. Abnormal tRF expression has been associated with several diseases including cancers (24), autoimmune diseases (21), and neurodegenerative diseases (8).
Recent studies have found expression of diverse tRFs in different biological samples. For example, Xue et al. detected serum tRFs by the SYBR Green RT-PCR method (25). Wang et al. detected plasma tRF levels by relative quantification (26). In order to assess plasma tRF levels in reduced volumes, we combined the methods of specific reverse transcription and absolute quantification (Figure 1). This combination has been rarely reported. To detect tRF-33 in the low-volume plasma, we designed a specific hairpin structure RT primer, which effectively extended the first strand during cDNA synthesis (Figure 1A). The advantages of this method are as follows. First, the connection between RT primer 3’ sticky end (5’-CAGGC-3’) and the end of tRF-33 had complete sequence complementarity, which improved the efficiency and specificity of the reverse transcription (Figure 1A). Second, the hairpin structure of the RT primer that extended the first strand during the cDNA synthesis was beneficial for the design of amplification primers.
As there is no universally acceptable control for plasma RNA quantitation (27), we constructed a standard curve (Y = −3.578 × X + 44.07) for the purpose of absolute quantification (Figure 1B), which effectively improved the detection efficiency and reduced detection costs. During the amplification process, we designed a specific probe complementary to the first strand to increase the binding efficiency and subsequent amplification (Figure 1A). The developed detection method allowed us to achieve accurate and reliable quantification of tRF-33 in low-volume plasma.
As the development of gastric cancer is a gradual process, we were curious about whether tRF-33 was associated with dynamic changes in the gastric mucosa. We analyzed the tRF-33 levels in the plasma samples of individuals with differing gastric mucosa. Strikingly, tRF-33 showed a gradient change from healthy individuals to patients with gastritis, early gastric cancer, and advanced gastric cancer (Figure 2A), indicating the potential of tRF-33 for dynamic monitoring of the progression of different gastric mucosal lesions. Further, based on patient clinicopathologic data, we found that reduced tRF-33 was related to poor tumor differentiation, bigger tumor size, and a higher level of CA125 (Table 1), which prompted us to evaluate its diagnostic and prognostic value in gastric cancer.
Recent studies have confirmed the value of tRFs as biomarkers for the detection of various types of cancers. For example, Xue et al. identified the clinical significance of tRFMetCAT and tRFValTAC in pancreatic ductal adenocarcinoma (PDAC) diagnosis with AUCs of 0.687 and 0.793, respectively (25). Wang et al. demonstrated the diagnostic values of tRFArgCCT-017, tRFGlyCCC-001, and tiRNAPheGAA-003 in breast cancer by construction of joint ROC curves (26). In this study, the clinical significance of tRF-33 for monitoring different gastric mucosal lesions was assessed by ROC curves between different cohorts. In this manner, tRF-33 levels distinguished early gastric cancer patients from the healthy individuals, with sensitivity and specificity of 45.8% and 96.2%, respectively. Advanced gastric cancer patients were distinguished from the healthy individuals, with sensitivity and specificity of 63.2% and 84.9%, respectively (Figure 2 and Table 2). Further, tRF-33 also showed clinical value for distinguishing early gastric cancer patients and advanced gastric cancer from gastritis patients, with sensitivities of 72.9%, 60.4%, respectively, and specificities of 65.3%, 66.7%, respectively (Figure 2 and Table 2). Moreover, survival analysis of advanced gastric cancer patients demonstrated that downregulated of tRF-33 related to an unfavorable survival (Figure 3).
Conclusion
We developed a method for quantitative detection of plasma tRF-33 by use of a hairpin RT primer and absolute quantification. tRF-33 was found to be a promising biomarker for monitoring gastric cancer patient progression. Results demonstrated, by multivariate prediction models, that tRF-33 is an independent variable for prediction of unfavorable survival outcomes for patients with gastric cancer.
Data availability statement
The raw data supporting the conclusions of this article will be made available by the authors, without undue reservation.
Ethics statement
The studies involving human participants were reviewed and approved by Human Research Ethics Committee of Ningbo University. The patients/participants provided their written informed consent to participate in this study.
Author contributions
JuG designed the study. SZ and JuG wrote the manuscript. SZ, YX, XY, and JiG collected clinical information. GY reviewed the pathologic diagnoses. All authors contributed to the article and approved the submitted version.
Funding
This study was supported by grants from Zhejiang Provincial Natural Science Foundation of China (no. LGF21H200004), National Natural Science Foundation of China (no. 81974316), Ningbo Municipal Bureau of Science and Technology (no. 2021Z133, 2022Z130), Nature Science Foundation of Ningbo (no.2021J253); Zhejiang Province Public Welfare Technology Application Research Project of China (no. LGF22H160039), and the K.C. Wong Magna Fund in Ningbo University.
Acknowledgments
The authors thank the technical support of the Core Facilities, Health Science Center, Ningbo University.
Conflict of interest
The authors declare that the research was conducted in the absence of any commercial or financial relationships that could be construed as a potential conflict of interest.
Publisher’s note
All claims expressed in this article are solely those of the authors and do not necessarily represent those of their affiliated organizations, or those of the publisher, the editors and the reviewers. Any product that may be evaluated in this article, or claim that may be made by its manufacturer, is not guaranteed or endorsed by the publisher.
References
1. Sung H, Ferlay J, Siegel RL, Laversanne M, Soerjomataram I, Jemal A, et al. Global cancer statistics 2020: GLOBOCAN estimates of incidence and mortality worldwide for 36 cancers in 185 countries. CA Cancer J Clin (2021) 71(3):209–49. doi: 10.3322/caac.21660
2. Cui H, Li H, Wu H, Du F, Xie X, Zeng S, et al. A novel 3'tRNA-derived fragment tRF-Val promotes proliferation and inhibits apoptosis by targeting EEF1A1 in gastric cancer. Cell Death Dis (2022) 13(5):471. doi: 10.1038/s41419-022-04930-6
3. Han Y, Peng Y, Liu S, Wang X, Cai C, Guo C, et al. tRF3008A suppresses the progression and metastasis of colorectal cancer by destabilizing FOXK1 in an AGO-dependent manner. J Exp Clin Cancer Res (2022) 41(1):32. doi: 10.1186/s13046-021-02190-4
4. Zhu L, Ge J, Li T, Shen Y, Guo J. tRNA-derived fragments and tRNA halves: the new players in cancers. Cancer Lett (2019) 452:31–7. doi: 10.1016/j.canlet.2019.03.012
5. Xie Y, Yao L, Yu X, Ruan Y, Li Z, Guo J. Action mechanisms and research methods of tRNA-derived small RNAs. Signal Transduct Target Ther (2020) 5(1):109. doi: 10.1038/s41392-020-00217-4
6. Weng Q, Wang Y, Xie Y, Yu X, Zhang S, Ge J, et al. Extracellular vesicles-associated tRNA-derived fragments (tRFs): biogenesis, biological functions, and their role as potential biomarkers in human diseases. J Mol Med (Berl). (2022) 100(5):679–95. doi: 10.1007/s00109-022-02189-0
7. Guo Y, Bai D, Liu W, Liu Y, Zhang Y, Kou X, et al. Altered sperm tsRNAs in aged male contribute to anxiety-like behavior in offspring. Aging Cell (2021) 20(9):e13466. doi: 10.1111/acel.13466
8. Hogg MC, Rayner M, Susdalzew S, Monsefi N, Crivello M, Woods I, et al. 5'ValCAC tRNA fragment generated as part of a protective angiogenin response provides prognostic value in amyotrophic lateral sclerosis. Brain Commun (2020) 2(2):fcaa138. doi: 10.1093/braincomms/fcaa138
9. Zhu L, Li Z, Yu X, Ruan Y, Shen Y, Shao Y, et al. The tRNA-derived fragment 5026a inhibits the proliferation of gastric cancer cells by regulating the PTEN/PI3K/AKT signaling pathway. Stem Cell Res Ther (2021) 12(1):418. doi: 10.1186/s13287-021-02497-1
10. Zhu P, Lu J, Zhi X, Zhou Y, Wang X, Wang C, et al. tRNA-derived fragment tRFLys-CTT-010 promotes triple-negative breast cancer progression by regulating glucose metabolism via G6PC. Carcinogenesis. (2021) 42(9):1196–207. doi: 10.1093/carcin/bgab058
11. Shen Y, Yu X, Ruan Y, Li Z, Xie Y, Yan Z, et al. Global profile of tRNA-derived small RNAs in gastric cancer patient plasma and identification of tRF-33-P4R8YP9LON4VDP as a new tumor suppressor. Int J Med Sci (2021) 18(7):1570–79. doi: 10.7150/ijms.53220
12. Wang P, Sun Z, Wang W, Deng J, Wang Z, Liang H, et al. Conditional survival of patients with gastric cancer who undergo curative resection: a multi-institutional analysis in China. Cancer. (2018) 124(5):916–24. doi: 10.1002/cncr.31160
13. Feng F, Tian Y, Xu G, Liu Z, Liu S, Zheng G, et al. Diagnostic and prognostic value of CEA, CA19-9, AFP and CA125 for early gastric cancer. BMC Cancer. (2017) 17(1):737. doi: 10.1186/s12885-017-3738-y
14. Speer J, Gehrke CW, Kuo KC, Waalkes TP, Borek E. tRNA breakdown products as markers for cancer. Cancer. (1979) 44(6):2120–3. doi: 10.1002/1097-0142(197912)44:6<2120::aid-cncr2820440623>3.0.co;2-6
15. Li Y, Luo J, Zhou H, Liao JY, Ma LM, Chen YQ, et al. Stress-induced tRNA-derived RNAs: a novel class of small RNAs in the primitive eukaryote giardia lamblia. Nucleic Acids Res (2008) 36(19):6048–55. doi: 10.1093/nar/gkn596
16. Wang Y, Weng Q, Ge J, Zhang X, Guo J, Ye G. tRNA-derived small RNAs: Mechanisms and potential roles in cancers. Genes Dis (2022) 9(6):1431–42. doi: 10.1016/j.gendis.2021.12.009
17. Thompson DM, Parker R. The RNase Rny1p cleaves tRNAs and promotes cell death during oxidative stress in saccharomyces cerevisiae. J Cell Biol (2009) 185(1):43–50. doi: 10.1083/jcb.200811119
18. Mo D, He F, Zheng J, Chen H, Tang L, Yan F. tRNA-derived fragment trf-17-79mp9pp attenuates cell invasion and migration via THBS1/TGF-β1/Smad3 axis in breast cancer. Front Oncol (2021) 11:656078. doi: 10.3389/fonc.2021.656078
19. Wu Y, Yang X, Jiang G, Zhang H, Ge L, Chen F, et al. 5'-tRF-GlyGCC: a tRNA-derived small RNA as a novel biomarker for colorectal cancer diagnosis. Genome Med (2021) 13(1):20. doi: 10.1186/s13073-021-00833-x
20. Gu X, Ma S, Liang B, Ju S. Serum hsa_tsr016141 as a kind of tRNA-derived fragments is a novel biomarker in gastric cancer. Front Oncol (2021) 11:679366. doi: 10.3389/fonc.2021.679366
21. Yang P, Zhang X, Chen S, Tao Y, Ning M, Zhu Y, et al. A novel serum tsrna for diagnosis and prediction of nephritis in SLE. Front Immunol (2021) 12:735105. doi: 10.3389/fimmu.2021.735105
22. Sharma U, Conine CC, Shea JM, Boskovic A, Derr AG, Bing XY, et al. Biogenesis and function of tRNA fragments during sperm maturation and fertilization in mammals. Science. (2016) 351(6271):391–96. doi: 10.1126/science.aad6780
23. Torres AG, Reina O, Stephan-Otto Attolini C, Ribas de Pouplana L. Differential expression of human tRNA genes drives the abundance of tRNA-derived fragments. Proc Natl Acad Sci U S A. (2019) 116(17):8451–56. doi: 10.1073/pnas.1821120116
24. Yu X, Song X, Xie Y, Zhang S, Guo J. Establishment of an absolute quantitative method to detect a plasma tRNA-derived fragment and its application in the non-invasive diagnosis of gastric cancer. Int J Mol Sci (2023) 24(1):322. doi: 10.3390/ijms24010322
25. Xue M, Shi M, Xie J, Zhang J, Jiang L, Deng X, et al. Serum tRNA-derived small RNAs as potential novel diagnostic biomarkers for pancreatic ductal adenocarcinoma. Am J Cancer Res (2021) 11(3):837–48. doi: 10.21203/rs.3.rs-63978/v1
26. Wang J, Ma G, Ge H, Han X, Mao X, Wang X, et al. Circulating tRNA-derived small RNAs (tsRNAs) signature for the diagnosis and prognosis of breast cancer. NPJ Breast Cancer. (2021) 7(1):4. doi: 10.1038/s41523-020-00211-7
Keywords: tRNA-derived fragment, absolute quantification, gastric cancer, diagnostic value, prognostic value, tRF-33-P4R8YP9LON4VDP
Citation: Zhang S, Xie Y, Yu X, Ge J, Ye G and Guo J (2023) Absolute quantification of a plasma tRNA-derived fragment for the diagnosis and prognosis of gastric cancer. Front. Oncol. 13:1106997. doi: 10.3389/fonc.2023.1106997
Received: 24 November 2022; Accepted: 28 March 2023;
Published: 17 April 2023.
Edited by:
Zequn Li, The Affiliated Hospital of Qingdao University, ChinaReviewed by:
Cuicui Li, Capital Medical University, ChinaRakesh Ramjiawan, Harvard Medical School, United States
Copyright © 2023 Zhang, Xie, Yu, Ge, Ye and Guo. This is an open-access article distributed under the terms of the Creative Commons Attribution License (CC BY). The use, distribution or reproduction in other forums is permitted, provided the original author(s) and the copyright owner(s) are credited and that the original publication in this journal is cited, in accordance with accepted academic practice. No use, distribution or reproduction is permitted which does not comply with these terms.
*Correspondence: Junming Guo, Z3VvanVubWluZ0BuYnUuZWR1LmNu