- 1Department of Biostatistics, School of Public Health, Fudan University, Shanghai, China
- 2Department of Neurosurgery, Huashan Hospital, Shanghai Medical College, Fudan University, Shanghai, China
- 3Neurosurgical Institute, Fudan University, Shanghai, China
- 4Shanghai Clinical Medical Center of Neurosurgery, Shanghai Municipal Health Commission, Shanghai, China
- 5Shanghai Key Laboratory of Brain Function and Restoration and Neural Regeneration, Science and Technology Commission of Shanghai Municipality, Shanghai, China
- 6National Clinical Research Centre for Aging and Medicine, Huashan Hospital, Fudan University, Shanghai, China
- 7Key Laboratory of Public Health Safety of Ministry of Education, Fudan University, Shanghai, China
Background and Objectives: Patients with primary malignant brain tumors may experience mental health disturbances that can significantly affect their daily life. This study aims to identify risk factors and generate predictive models for postoperative mental health disturbances (PMHDs) in adult glioma patients in accordance with different clinical periods; additionally, survival analyses will be performed.
Methods: This longitudinal cohort study included 2,243 adult patients (age at diagnosis ≥ 18 years) with nonrecurrent glioma who were pathologically diagnosed and had undergone initial surgical resection. Six indicators of distress, sadness, fear, irritability, mood and enjoyment of life, ranging from 0-10, were selected to assess PMHDs in glioma patients in the third month after surgery, mainly referring to the M.D. Anderson Symptom Inventory Brain Tumor Module (MDASI-BT). Factor analysis (FA) was applied on these indicators to divide participants into PMHD and control groups based on composite factor scores. Survival analyses were performed, and separate logistic regression models were formulated for preoperative and postoperative factors predicting PMHDs.
Results: A total of 2,243 adult glioma patients were included in this study. Based on factor analysis results, 300 glioma patients had PMHDs in the third postoperative month, and the remaining 1,943 were controls. Candidate predictors for PMHDs in the preoperative model were associated with age, clinical symptoms (intracranial space-occupying lesion, muscle weakness and memory deterioration), and tumor location (corpus callosum, basal ganglia and brainstem), whereas age, clinical symptoms (nausea and memory deterioration), tumor location (basal ganglia and brainstem), hospitalization days, WHO grade 4, postoperative chemotherapy or radiotherapy and postoperative Karnofsky Performance Scale (KPS) served as important factors in the postoperative model. In addition, the median overall survival (OS) time for glioma patients with PMHDs was 19 months, compared to 13 months for glioblastoma, IDH-wild type (GBM) patients with PMHDs.
Conclusion: The risk factors for PMHDs were identified. These findings may provide new insights into predicting the probability of PMHD occurrence in glioma patients in addition to aiding effective early intervention and improving prognosis based on different clinical stages.
1 Introduction
Gliomas are common infiltrative primary brain tumors (1) whose cellular origin remains controversial (2); they can occur in all age groups. The average annual age-adjusted incidence of glioma was 5.95/100,000, with 8.63%, 14.91%, and 76.46% of the population aged 0-14, 15-39, and 40 years or older, respectively (3). Mental disturbances are among the most common causes of death worldwide, accounting for approximately 14.30% of deaths (4), and they are prevalent in patients with glioma (5). Prolonging the survival of patients with gliomas through multiple therapeutic approaches is critical, but the impact of tumor diagnosis and disease progression on patients’ mood alterations and mental health warrants attention (6).
According to previous evidence, the invasive clinical nature of the glioma itself as well as other factors (e.g., treatment regimens) cause direct damage to brain function and may also contribute to neurocognitive changes (7–9) and psychological disorders (10). Approximately 19-80% of glioma patients undergoing oncologic surgery experience perioperative and peritumoral infarctions, which are correlated with postoperative neurologic dysfunction and impaired function (11). Mental health disturbances have a dramatic influence on life after cancer diagnosis, including cancer treatment and ongoing care, which poses substantial challenges and burdens for both patients and their family members who may supply them with supportive care services (12). Patients with glioma are more susceptible to moderate-to-severe depressive symptoms than cancer-free patients (13). The 6-month prevalence of clinical depression in brain tumor patients is approximately 20%, while up to 60% of patients may be affected by personality changes (14). The suicide incidence among cancer patients is 20% higher than that among the general population; therefore, the early period after disease diagnosis is a critical intervention phase for mental health issues (15).
Fewer papers than expected are currently available on mental health in glioma patients, and most available reports are limited by smaller sample sizes (16) in addition to focusing on single disorders such as depression (17). Explicit identification of potential risks for multiple indicators of mental health disturbances is urgently needed to further advance disease management and prevention. A study with a larger and more balanced population based on all pathological grades is encouraged. Although the etiological basis of glioma and the mechanisms underlying mental health disturbances remain obscure (18), the strategy of identifying clinical risk factors associated with their development to predict patient outcomes is feasible (19) and can be implemented to assist clinicians with earlier intervention, improve clinical diagnosis, and decrease the incidence of postoperative mental health disturbances (PMHDs).
Herein, we investigated the risk factors for PMHDs in glioma patients who had undergone tumor resection by collecting detailed demographic data and clinical information from the preoperative and postoperative stages to establish accurate prediction models for PMHDs and to compare the discrimination of survival between participants in the PMHD and control groups. The intent of predictive models is to identify target populations requiring priority attention, provide individualized early intervention and treatment strategies and support optimal evidence-based disease management, as well as better assess prognosis and improve the quality of patient survival.
2 Materials and methods
2.1 Patients
We recruited 2,825 participants who were pathologically diagnosed with glioma and had undergone maximal safe resection at Huashan Hospital from March 2010 to December 2018. The exclusion criteria included the following (1): aged <18 years old at diagnosis; (2) missing WHO grade; (3) recurrent glioma or multiple surgeries; and (4) no follow-up information of PMHDs in the third postoperative month. A total of 2,243 patients met all criteria for inclusion in the study (Figure 1). The study protocol was approved by the ethics review committee of the Huashan Hospital Affiliated to Fudan University (receiving ethics committee number KY2015-256). Written informed consent was signed by all participants of the Central Nervous System Disease Tissue Bank at Huashan Hospital.
2.2 Clinical data
Meatal health disturbance in the third postoperative month consisted of six indicators: distress, sadness, fear, irritability, mood, and enjoyment of life. Data for all indicators were collected at the third month postoperative follow-up. The selection of indicators referred to the M.D. Anderson Symptom Inventory Brain Tumor Module (MDASI-BT) (20) and the final list of symptoms based on content validity index (CVI) (21). The MDASI-BT is a patient-reported site-specific brain tumor module that evaluates the severity of symptoms experienced by cancer patients and the potential impact of those symptoms on daily life. The final list of symptoms was identified by the team that developed the MDASI-BT after conducting a CVI analysis of multiple symptoms prior to developing the scale. Each indicator refers to an eleven-point scale ranging from the lowest degree (0) to the highest degree (10) in one-point increments, with 0 being “not present” and 10 being “as bad as you can imagine” in the last 24 hours. Basic demographic information, clinical data and regular follow-up information of the participants were collected from the hospital’s electronic medical record system. The preoperative phase consisted of 40 variables related to demographic information, clinical symptoms, disease history, and tumor location. It should be mentioned that the tumor location variables are not independent of each other; for example, multifocal gliomas could involve multiple tumor locations simultaneously, whereas a single tumor may also involve different brain lobes. A series of 14 variables were incorporated in the postoperative phase, including WHO grade, molecular indicators and postoperative scale scores (Table 1).
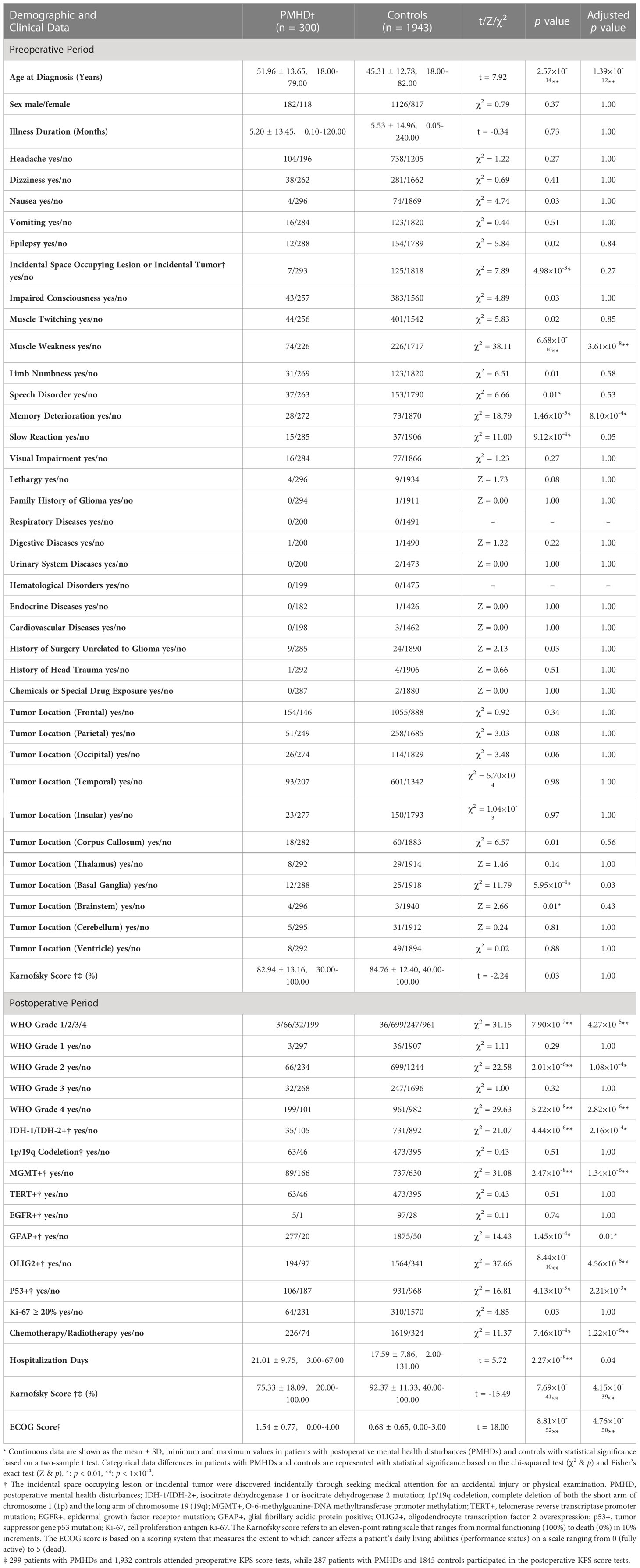
Table 1 Demographic and clinical data of patients with postoperative mental health disturbances and controls.
2.3 Statistical analysis
The Kaiser−Meyer−Olkin (KMO) test and Bartlett’s test of sphericity were conducted to investigate the sampling adequacy for utilizing factor analysis and the homogeneity of the data, respectively. A sample size with a KMO value greater than 0.5 is generally considered to be sufficient to perform factor analysis, and a p value of less than 0.05 in Bartlett’s test demonstrates data homogeneity. Principal component analysis and eigenvalues greater than 1.00 were used to extract the factors, and the maximum variance method was used as the rotation method. The least absolute shrinkage and selection operator (LASSO) was applied to sequence variables in different clinical periods and filter out the top 15 variables. The LASSO method, which was proposed by statistics professor Robert Tibshirani in 1996, is a high-dimensional data analysis method for performing regularization on data models and ranking the importance of variables (22). Continuous data are shown as the mean ± standard deviation and minimum - maximum values, and Student’s t test was used for statistical analysis to compare the two groups. The statistical significance of the differences in categorical data between PMHD patients and control subjects was determined by the chi-squared test and Fisher’s exact test. Prediction models were tested for multicollinearity using the variance inflation factor (VIF). Pearson’s correlation coefficient was used to measure linear correlation between continuous variables, while Cramer’s v was applied to measure the strength of association between categorical variables (23). Kaplan−Meier survival curves and log-rank tests were applied to compare the survival distributions between the two groups. Overall survival (OS) time was defined as the period elapsing between the date of the first glioma surgery and date of death. The sensitivity and specificity of the PMHD prediction models developed by implementing the logistic regression method were evaluated with receiver operating characteristic (ROC) curves. In addition, DeLong’s test was conducted to show whether the area under the ROC curves (AUCs) of the two models were significantly different. Statistical significance was denoted by p ≤ 0.05. SAS 9.4 and R version 4.2.1 were used for statistical analysis.
3 Results
3.1 Factor analysis
A total of 2,243 patients were enrolled in this study (Figure 1). The factor analysis of the six indicators that were assessed in glioma patients at the third postoperative month showed a KMO value of 0.69 and a Bartlett test p value of less than 0.05, indicating the feasibility of factor analysis. The two components with eigenvalues greater than 1.00 were finally extracted through factor analysis, which could explain 37.08% and 33.90% of the variance, respectively. The composite scores of patients’ psychiatric conditions were calculated based on these two components, and all patients were divided into two groups: 300 (13.37%) patients were assigned to the PMHD group, and the remaining 1,943 (86.63%) patients were assigned to the control group. The mean of standardized values of the six indexes between the two groups with significant differences were displayed in Figure 2, suggesting that patients in the control group performed better than those in the PMHD group in terms of postoperative mental health disturbances.
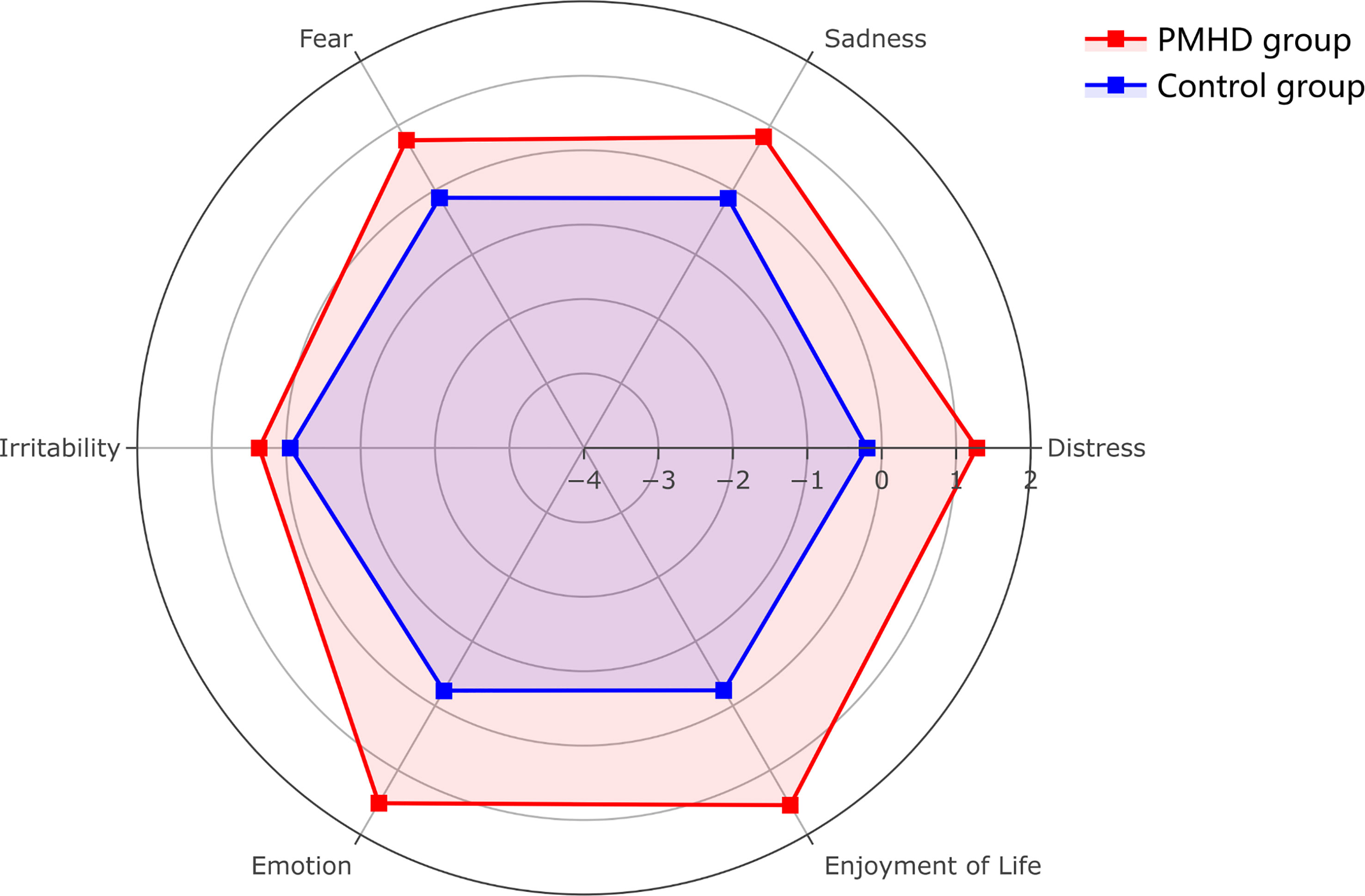
Figure 2 Radar plot of six indicators of postoperative mental health disturbances in the PMHD and control groups. The figure shows the mean values of the six indicators after standardization (patients with PMHDs in red, controls in blue).
3.2 Patient characteristics
A total of 2,243 adult glioma patients were predominantly male (1,308/2,243; 58.31%), with a mean age at diagnosis of 46.20 years (standard deviation = 13.09). In accordance with the composite score of the factor analysis, 300 (13.37%) of these patients suffered from mental health disturbances at the third month postoperative follow-up, while the remaining 1943 (86.63%) cases served as a control group with relatively favorable mental status. Patients in the PMHD group were significantly older than those in the control group (mean [SD] age, 51.96 [13.65] vs. 45.31 [12.78] years, t = 7.92, p < 0.001). Patients with gliomas located in specific positions, such as the corpus callosum (18/300 [6.00%] vs. 60/1943 [3.09%], χ2 = 6.57, p = 0.01), basal ganglia (12/300 [4.00%] vs. 25/1943 [1.29%], χ2 = 11.79, p < 0.001), and brainstem (4/300 [1.33%] vs. 3/1943 [0.15%], Z = 2.66, p = 0.01), were more prone to PMHDs. In addition, 66.33% (199/300) and 49.46% (961/1943) patients had WHO grade 4 gliomas in the PMHD and control groups, respectively. The detailed clinical variables and the respective statistical results of the univariate analysis for the preoperative and postoperative phases are tabulated in Table 1.
3.3 Prediction models and survival analysis
Multiple variables were found to be significantly different between the PMHD and control groups by a univariate analysis which included all variables. Therefore, the importance of the variables was ranked separately based on different study populations using the LASSO method, and the top 15 variables were selected to construct different models for predicting PMHDs. Both models in Table 2 are for all glioma patients, whereas the two postoperative models in Table 3 are for glioblastoma, IDH-wildtype (GBM) patients and diffuse astrocytoma, IDH-mutant (DA) patients, respectively. To improve the generalizability of the models, molecular indicators were not included in the postoperative model as well as in the predictive model for DA. In addition, there was a strong correlation between Karnofsky Performance Scale (KPS) score and Eastern Cooperative Oncology Group (ECOG) score, with a Pearson’s correlation coefficient of -0.81, and the variable ECOG was excluded from the screening of variables.
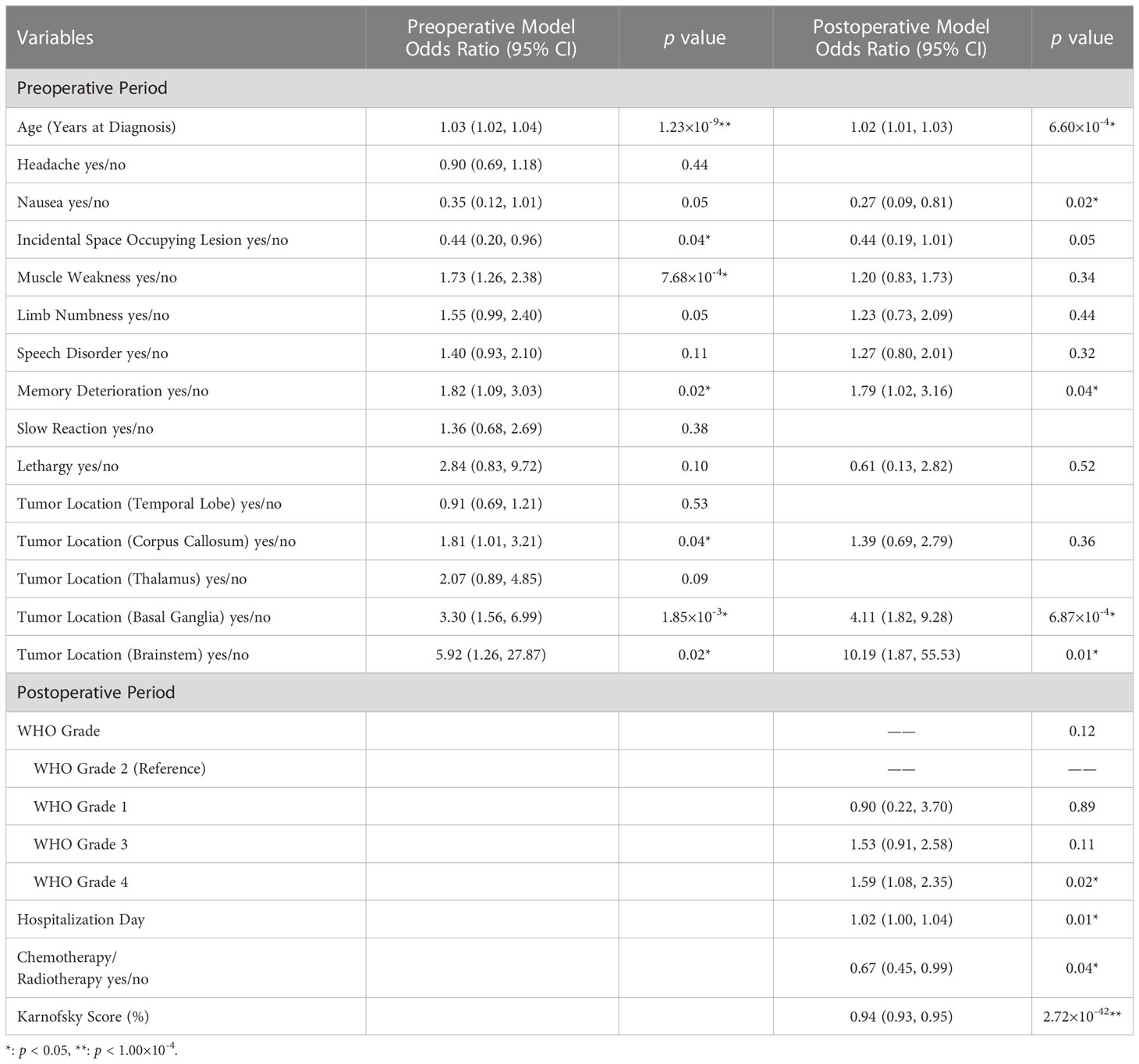
Table 2 Multivariable logistic regression model for predicting postoperative mental health disorders in glioma patients.
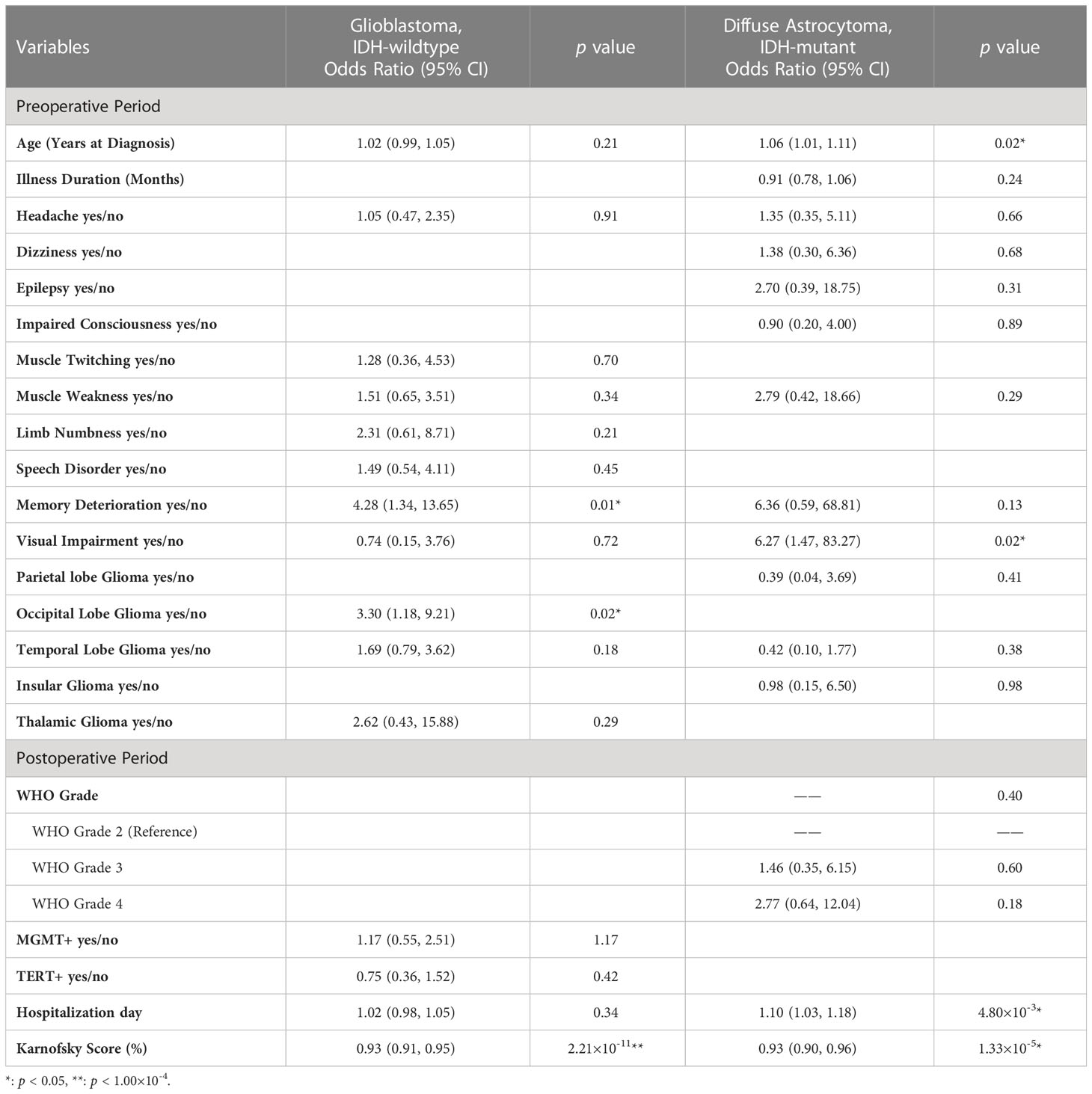
Table 3 Multivariable logistic regression model for predicting postoperative mental health disorders in different subgroups of glioma patients.
Further logistic regression analysis revealed that the age at diagnosis (preoperative model: odds ratio [OR], 1.03; 95% confidence interval [CI], 1.02-1.04; p < 0.001; postoperative model: OR, 1.02; 95% CI, 1.00-1.03; p < 0.001) was significantly different in the two models (Table 2). Additionally, clinical symptoms of incidental findings of intracranial space occupying lesion (OR, 0.44; 95% CI, 0.20-0.96; p = 0.04), muscle weakness (OR, 1.73; 95% CI, 1.26-2.38; p < 0.001) and memory deterioration (OR, 1.82; 95% CI, 1.09-3.03; p = 0.02) were critical influencing factors in the preoperative model. Tumors growing in specific locations, such as the corpus callosum (OR, 1.81; 95% CI, 1.01-3.21; p = 0.04), basal ganglia (OR, 3.30; 95% CI, 1.56-6.99; p = 1.85×10-3), and brainstem (OR, 5.92; 95% CI, 1.26-27.87; p = 0.02), were also significantly associated with PMHDs. In the multivariate postoperative model, nausea (OR, 0.27; 95%CI, 0.09-0.81; p = 0.02), memory deterioration (OR, 1.79; 95% CI, 1.02-3.16; p = 0.04), basal ganglia glioma (OR, 4.11; 95% CI, 1.82-9.28; p < 0.001), brainstem glioma (OR, 10.19; 95% CI, 1.87-55.53; p = 0.01), WHO grade 4 (OR, 1.59; 95% CI, 1.08-2.35; p = 0.02), hospitalization day (OR, 1.02; 95% CI, 1.00-1.04; p = 0.01), and postoperative Karnofsky Performance Scale (KPS) score (OR, 0.94; 95% CI, 0.93-0.95; p < 0.001) were regarded as pivotal predictors of PMHDs.
Predictive models for GBM and DA were specifically investigated (Table 3). ROC curve analysis was utilized to validate the performance of the PMHD prediction models which were stratified by different clinical periods or subgroups (Figure 3). The AUCs of the multivariate prediction models varied from 0.69 to 0.93, with the highest AUC being for DA. Nevertheless, the postoperative model outperformed the model constructed by preoperative variables only, with a p value of less than 0.05 for the two curves by DeLong’s test indicating a significant difference. VIF was used to detect the severity of multicollinearity in the multivariate logistic prediction models, and the VIFs of the variables were all less than 1.80 (eTable 1). A simplified version of the prediction model with an AUC of 0.82 was also constructed for the purpose of model generalization (eTable 2).
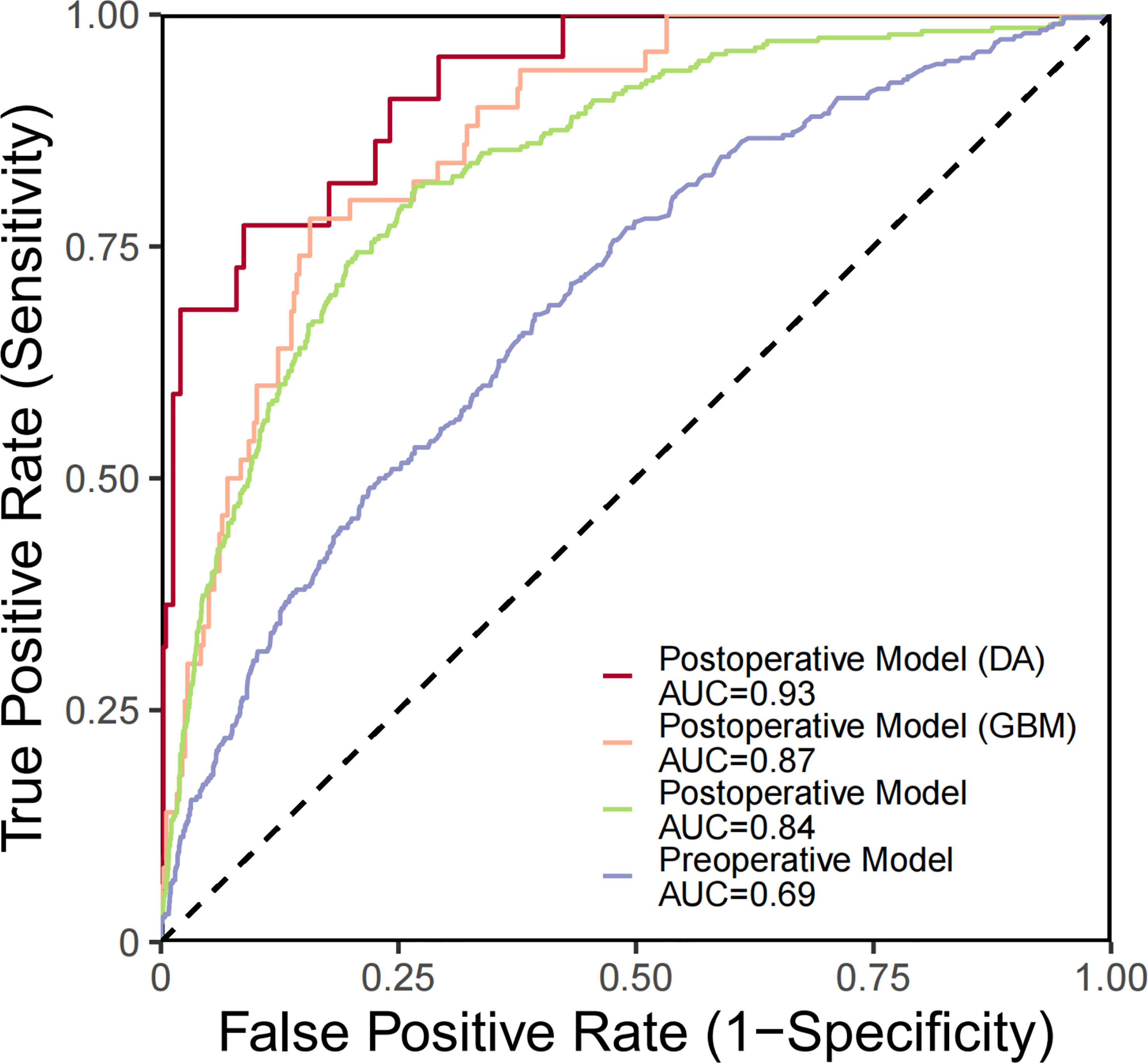
Figure 3 ROC curves generated for PMHD prediction with different clinical periods and subgroups. DA, diffuse astrocytoma, IDH-mutant; GBM, glioblastoma, IDH-wildtype.
Among all glioma patients, the median OS was 19 and 73 months in the PMHD and control groups, with the 5-year survival rates of 24.88% and 53.51%, respectively (Figure 4). While in GBM patients, the median OS was 13 and 23 months and the 5-year survival rates were 9.61% and 25.36% in the PMHD and control groups, respectively. Additionally, in IDH-mutated WHO grade 4 diffuse astrogliomas, the median OS was significantly shorter in patients with PMHDs than in controls (14 vs. 46 months, P < 0.001). In low-grade gliomas (LGG) containing WHO grades 1 and 2, the 5-year survival rate for patients with PMHDs was found to be 73.96%, lower than the 86.33% for controls.
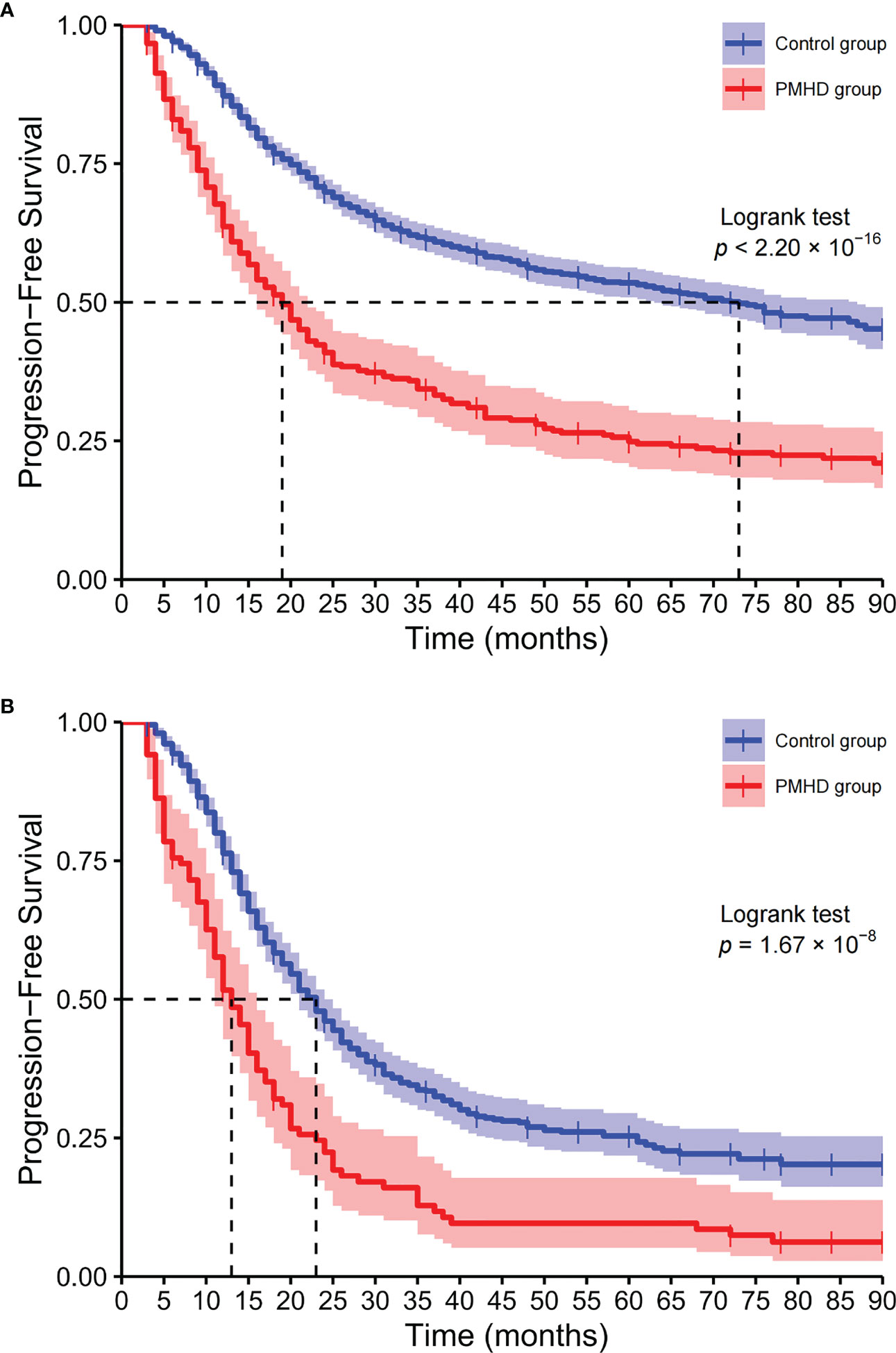
Figure 4 Kaplan−Meier curves of overall survival. Kaplan-Meier plots of overall survival for all glioma patients (A) and glioblastoma, IDH-wildtype (B) patients (PMHD group in red, control group in blue).
4 Discussion
This longitudinal cohort study enrolled 2,243 glioma patients at the Huashan Hospital over an 8-year period, providing demographic information and clinical data on a sizeable sample involving all WHO grades. In our study, separate preoperative and postoperative predictive models were constructed to pinpoint the potential clinical risk factors for mental health disturbances in glioma patients after surgical resection. Postoperative predictive models specifically targeting the GBM and DA subgroups were also performed. Survival analysis of multiple datasets revealed that patients with mental health disturbances had a worse prognosis and shorter survival times than did controls; Glioma patients with PMHDs had a median survival time of 19 months, whereas the median survival time was only 13 months for GBM patients with PMHDs. The current study aimed to accurately predict the probability of mental health disturbances in the short term (within three months) postoperatively from different clinical stages, to clearly identify special populations that need focused attention and to deliver promising clinical reference values for evidence-based early intervention and cancer risk management.
Mental health disturbances were common neuropsychiatric complications and comorbidities in patients with brain tumors (24, 25). The incidence of mental health disturbances in this study was relatively underestimated at only 13.37%; this was probably attributable to our appraisement of glioma patients’ mental status in the third postoperative month. Patients who failed to complete the MDASI-BT in the third postoperative month due to missed visits or death were excluded from the study. Moreover, some patients experienced partial progress in physical function and relative improvement in mental status after enduring the risks inherent in brain tumor surgery and prolonged postoperative rehabilitation. The stigma associated with mental health disorders, influenced by cultural and contextual value systems (26), was also a potential reason for the relatively low reporting rate. Additionally, it should be noted that mental health disturbances are not equivalent to psychiatric disorders. Therefore, the incidence rate of PHMDs in this study should not be directly compared to other statistics in the literature. Glioma patients generally suffer from the mental stress of a poor prognosis, considerable financial pressure, the symptom burden of the disease and decreased workforce participation (27) throughout the disease trajectory. The tumor and its treatment options may affect the brain with far-reaching mental health consequences. As the mental symptoms progress, the need for care support among glioma patients gradually increases, imposing a heavy burden on caregivers, families, the healthcare system and society (28). Therefore, identifying risk factors that contribute to mental health disturbances and providing early and effective interventions are critical for improving the quality of life of glioma patients (29, 30).
The incidence of glioma varies with age (31), and advanced age is broadly recognized as a predictor of poor prognosis in glioma patients (32, 33); this was consistent with the relevant findings in our study. Several studies (34, 35) have reported that glioma patients with global cerebral manifestations, focal neurologic deficits and neurocognitive dysfunction will endure unfavorable life effects; these results support our research findings that glioma patients with clinical symptoms of nausea, muscle weakness, memory deterioration and visual impairment are more prone to experiencing mental health disturbances. Visual impairment can be caused by lesions in brain regions involved in the visual pathway, such as the occipital, parietal and temporal lobes, as well as factors such as high intra-cranial pressure. In addition, it was noteworthy that the vast majority of glioma patients experienced partial relief of their neuropsychiatric symptoms after maximal safe tumor resection (36, 37). Thus, preoperative neurological deficits may be potentially valuable for early detection of disease, but they were not equivalent to the patient’s postoperative mental status and could not directly influence PMHDs. Intracranial space occupying lesion (ICSOL) or intracranial tumor, discovered incidentally through accidental injury seeking medical attention or routine physical examination, was a potential protective factor for the occurrence of PMHD in glioma patients. Incidental findings of brain abnormalities were not relevant to the purpose of this examination (38). The symptoms associated with glioma were less evident in these patients at the time of brain imaging, demonstrating the value of early detection of latent disease and facilitating early intervention.
The anatomic location of gliomas considerably influences prognosis and therapeutic options. Gliomas growing in specific regions undoubtedly impose immense challenges for surgical resection and carry potential risks and complications that may induce neurological impairments. Lesions of the corpus callosum, the largest bundle of white matter fibers that extensively connect the left and right hemispheres of the brain, may affect the exchange of information between the bilateral hemispheres and lead to mental health disturbances and cognitive deficits (39). The basal ganglia, located beneath the cerebral cortex, are primarily involved in motor control, and their lesions can present with psychiatric symptoms as well as differing degrees of cognitive dysfunction. Brainstem glioma is a very rare brain tumor that typically occurs in children and is infrequent in adults, representing only 1% to 2% of adult gliomas. The prognosis of brainstem gliomas is highly heterogeneous, with median survival times varying from 11 to 84 months (40), compared with the median survival time of 54 months in this study.
Glioma classification continues to evolve, and a new classification system based on key molecular parameters is of critical importance in providing diagnostic and prognostic information and optimizing tumor management. Generally, the higher the WHO grade of glioma is, the worse the prognosis. GBM is one of the most malignant central nervous system tumors and is classified as WHO grade 4. Common complications of GBM are depression and other mental diseases that may occur in conjunction with GBM; these are partly attributable to the negative prospects expected after a GBM diagnosis but may be due to the partially overlapping molecular mechanisms of the two diseases (10). Key molecular markers are indispensable and precise tools for improving diagnostic accuracy, determining prognosis and optimizing oncology risk management (41). However, some predictive models in our study did not include molecular indicators for the sake of model generalization. Enhanced detection of critical molecular parameters may help to construct models with satisfactory performance for supplying early and effective interventions and facilitating personalized treatment.
KPS is widely recognized as an effective and reliable tool for evaluating patients’ functional status (42) and has potential utility in predicting survival, assessing prognosis (43) and serving as an early predictor of glioma. Lower KPS is a risk factor for the incidence of PMHDs in glioma patients and negatively affects the survival time of patients (44). In this study, it was observed that the median survival time of the higher KPS group (KPS > 70) was longer than that of the lower KPS group (KPS ≤ 70), with significant statistical difference (22 vs. 66 months, p < 0.001). In addition, it should be recognized that there are varying degrees of correlation between variables. The presence of postoperative KPS may affect the significance of other variables in the prediction models, such as preoperative neurological deficits. Glioma patients with PMHDs often have longer hospitalization days, which may be related to their physical condition and severity of the disease. Furthermore, longer hospitalization days also mean increased costs and prolonged care support, resulting in greater financial and psychological burdens for patients and their families. Compelling evidence suggests that mental health disturbances carry substantial weight in the survival outcomes of glioma patients, as the log-rank test indicated that the survival curves are significantly different between the PMHD and control groups. Among all glioma patients, the median OS and 5-year survival rate were 19 months and 24.88% in the PMHD group, compared with 73 months and 53.51% in the control group, respectively. In GBM and DA patients with both WHO grade 4, the median OS was 13 and 14 months in the PMHD group and 23 and 46 months in the control group, respectively. In the PMHD group, the 5-year survival rate was 73.96% in LGG patients and 9.61% in GBM patients.
Nevertheless, several limitations of our study should be acknowledged. First, we mainly relied on simple indicators from the MDASI-BT to evaluate mental health disturbances in glioma patients without adopting international general scales, such as the Hospital Anxiety and Depression Scale (HADS), the Hamilton Anxiety Rating Scale (HAM-A) and the Positive and Negative Syndrome Scale (PANSS). In subsequent cohort studies, the application of generic scales and more extensive validation in the preoperative and postoperative follow-up were contemplated to evaluate patients’ mental health disturbances. Second, all patients were registered from only one hospital, and the establishment of a multicenter study with a larger sample size should be considered to minimize selection bias. Finally, this cohort study spanned over 8 years, and histological samples from patients with a relatively long history of glioma-related surgery could not be supplemented, making it impossible to retest some important molecular indicators. Moving forward, future studies should explore the comprehensive and detailed data of recent years based on the new WHO classification criteria to overcome this limitation.
In conclusion, potential predictors of PMHDs were identified, and the predictive models provide a new means of accurately assessing the underlying risks of PMHDs. These results may be used to assist clinicians with early intervention of possible mental health problems in glioma patients and to provide social and family support services targeted towards populations needing priority attention, thereby enhancing risk management, optimizing treatment strategies and improving prognosis. Elucidating the etiology and pathogenesis of PMHD, as well as avoiding exposure its risk factors, merits future endeavors.
Data availability statement
The raw data supporting the conclusions of this article will be made available by the authors, without undue reservation.
Ethics statement
The study protocol was approved by the ethics review committee of the Huashan Hospital Affiliated to Fudan University (receiving ethics committee number KY2015-256). Written informed consent was signed by all participants of the Central Nervous System Disease Tissue Bank at Huashan Hospital. The patients/participants provided their written informed consent to participate in this study.
Author contributions
YW and JZ contributed equally to this work. Drafting of the manuscript: YW, JZ, and YY. Statistical analysis: YW and YY. Supervision: GQ and JW. Correspondence to: YY and JW. All authors contributed to the article and approved the submitted version.
Funding
This study was funded by Shanghai Shenkang Hospital Development Center (No. SHDC2020CR5004).
Conflict of interest
The authors declare that the research was conducted in the absence of any commercial or financial relationships that could be construed as a potential conflict of interest.
Publisher’s note
All claims expressed in this article are solely those of the authors and do not necessarily represent those of their affiliated organizations, or those of the publisher, the editors and the reviewers. Any product that may be evaluated in this article, or claim that may be made by its manufacturer, is not guaranteed or endorsed by the publisher.
Supplementary material
The Supplementary Material for this article can be found online at: https://www.frontiersin.org/articles/10.3389/fonc.2023.1153455/full#supplementary-material
Abbreviations
PMHDs, postoperative mental health disturbances; FA, factor analysis; GBM, glioblastoma IDH-wildtype; DA, diffuse astrocytoma IDH-mutant.
References
1. Miller JJ, Shih HA, Andronesi OC, Cahill DP. Isocitrate dehydrogenase-mutant glioma: evolving clinical and therapeutic implications. Cancer-Am Cancer Soc (2017) 123(23):4535–46. doi: 10.1002/cncr.31039
2. Alcantara Llaguno SR, Parada LF. Cell of origin of glioma: biological and clinical implications. Br J Cancer (2016) 115(12):1445–50. doi: 10.1038/bjc.2016.354
3. Low JT, Ostrom QT, Cioffi G, Neff C, Waite KA, Kruchko C, et al. Primary brain and other central nervous system tumors in the united states (2014-2018): a summary of the CBTRUS statistical report for clinicians. Neurooncol Pract May (2022) 9(3):165–82. doi: 10.1093/nop/npac015
4. Walker ER, McGee RE, Druss BG. Mortality in mental disorders and global disease burden implications: a systematic review and meta-analysis. JAMA Psychiat (2015) 72(4):334–41. doi: 10.1001/jamapsychiatry.2014.2502
5. Acquaye AA, Vera-Bolanos E, Armstrong TS, Gilbert MR, Lin L. Mood disturbance in glioma patients. J Neurooncol (2013) 113(3):505–12. doi: 10.1007/s11060-013-1143-1
6. Bhanja D, Ba D, Tuohy K, Wilding H, Trifoi M, Padmanaban V, et al. Association of low-grade glioma diagnosis and management approach with mental health disorders: a MarketScan analysis 2005-2014. Cancers (Basel) (2022) 14(6). doi: 10.3390/cancers14061376
7. van Kessel E, Snijders TJ, Baumfalk AE, et al. Neurocognitive changes after awake surgery in glioma patients: a retrospective cohort study. J Neuro-Oncol (2020) 146(1):97–109. doi: 10.1007/s11060-019-03341-6
8. Lee J, Hall RCW. The impact of gliomas on cognition and capacity. J Am Acad Psychiatry (2019) 47(3):350–9. doi: 10.29158/JAAPL.003841-19
9. Aldape K, Amin SB, Ashley DM, Barnholtz-Sloan JS, Bates AJ, Beroukhim R, et al. Glioma through the looking GLASS: molecular evolution of diffuse gliomas and the glioma longitudinal analysis consortium. Neuro-Oncology (2018) 20(7):873–84. doi: 10.1093/neuonc/noy020
10. Mugge L, Mansour TR, Crippen M, Alam Y, Schroeder J. Depression and glioblastoma, complicated concomitant diseases: a systemic review of published literature. Neurosurg Rev (2020) 43(2):497–511. doi: 10.1007/s10143-018-1017-2
11. Strand PS, Berntsen EM, Fyllingen EH, Sagberg LM, Reinertsen I, Gulati S, et al. Brain infarctions after glioma surgery: prevalence, radiological characteristics and risk factors. Acta Neurochir (Wien) (2021) 163(11):3097–108. doi: 10.1007/s00701-021-04914-z
12. Souza ALR, Guimaraes RA, de Araujo Vilela D, de Assis RM, de Almeida Cavalcante Oliveira LM, Souza MR, et al. Factors associated with the burden of family caregivers of patients with mental disorders: a cross-sectional study. BMC Psychiatry (2017) 17(1):353. doi: 10.1186/s12888-017-1501-1
13. Loppenthin K, Johansen C, Larsen MB, Forchhammer BH, Brennum J, Piil K, et al. Depressive symptoms in Danish patients with glioma and a cancer-free comparison group. J Natl Compr Canc Netw (2020) 18(9):1222–9. doi: 10.6004/jnccn.2020.7570
14. Pace A, Dirven L, Koekkoek JAF, Golla H., Fleming J., Ruda R., et al. European Association for neuro-oncology (EANO) guidelines for palliative care in adults with glioma. Lancet Oncol (2017) 18(6):e330–40. doi: 10.1016/S1470-2045(17)30345-5
15. Chang WH, Lai AG. Cumulative burden of psychiatric disorders and self-harm across 26 adult cancers. Nat Med (2022) 28(4):860–70. doi: 10.1038/s41591-022-01740-3
16. Singer S, Roick J, Danker H, Kortmann RD, Papsdorf K, Taubenheim S, et al. Psychiatric co-morbidity, distress, and use of psycho-social services in adult glioma patients-a prospective study. Acta Neurochir (Wien) (2018) 160(6):1187–94. doi: 10.1007/s00701-018-3527-7
17. Shi C, Lamba N, Zheng LJ, Cote D, Regestein QR, Liu CM, et al. Depression and survival of glioma patients: a systematic review and meta-analysis. Clin Neurol Neurosurg (2018) 172:8–19. doi: 10.1016/j.clineuro.2018.06.016
18. Saunders CN, Cornish AJ, Kinnersley B, Law PJ, Houlston RS, Claus EB, et al. Searching for causal relationships of glioma: a phenome-wide mendelian randomisation study. Brit J Cancer (2021) 124(2):447–54. doi: 10.1038/s41416-020-01083-1
19. Feng Y, Wang J, Tan DZ, Cheng P, Wu AH. Relationship between circulating inflammatory factors and glioma risk and prognosis: a meta-analysis. Cancer Med-Us (2019) 8(17):7454–68. doi: 10.1002/cam4.2585
20. Armstrong TS, Mendoza T, Gning I, Coco C, Cohen MZ, Eriksen L, et al. Validation of the M.D. Anderson symptom inventory brain tumor module (MDASI-BT). J Neurooncol (2006) 80(1):27–35. doi: 10.1007/s11060-006-9135-z
21. Armstrong TS, Cohen MZ, Eriksen L, Cleeland C. Content validity of self-report measurement instruments: an illustration from the development of the brain tumor module of the M.D. Anderson symptom inventory. Oncol Nurs Forum. May 10 (2005) 32(3):669–76. doi: 10.1188/05.ONF.669-676
22. Tibshirani R. Regression shrinkage and selection via the lasso: a retrospective. J R Stat Soc B (2011) 73:273–82. doi: 10.1111/j.1467-9868.2011.00771.x
23. Akoglu H. User's guide to correlation coefficients. Turk J Emerg Med Sep (2018) 18(3):91–3. doi: 10.1016/j.tjem.2018.08.001
24. Farzaneh R, Malek A, Mirzaei F, Amiri S, Salehpour F, Meshkini A, et al. Evaluation of comorbid psychiatric disorders in patients with primary brain tumors before and after surgery. Neurol Psychiatry Brain Res (2020) 36:8–13. doi: 10.1016/j.npbr.2020.02.003
25. Bunevicius A, Deltuva VP, Tamasauskas A. Association of pre-operative depressive and anxiety symptoms with five-year survival of glioma and meningioma patients: a prospective cohort study. Oncotarget (2017) 8(34):57543–51. doi: 10.18632/oncotarget.15743
26. Subu MA, Wati DF, Netrida N, Priscilla V, Dias JM, Abraham MS, et al. Types of stigma experienced by patients with mental illness and mental health nurses in Indonesia: a qualitative content analysis. Int J Ment Health Sy (2021) 15(1). doi: 10.1186/s13033-021-00502-x
27. Haider SA, Asmaro K, Kalkanis SN, Lee IY, Bazydlo M, Nerenz DR, et al. The economic impact of glioma survivorship: the cost of care from a patient perspective. Neurology (2020) 95(11):e1575–81. doi: 10.1212/WNL.0000000000010263
28. Flechl B, Ackerl M, Sax C, Oberndorfer S, Calabek B, Sizoo E, et al. The caregivers' perspective on the end-of-life phase of glioblastoma patients. J Neurooncol (2013) 112(3):403–11. doi: 10.1007/s11060-013-1069-7
29. Madhusoodanan S, Ting MB, Farah T, Ugur U. Psychiatric aspects of brain tumors: a review. World J Psychiatry (2015) 5(3):273–85. doi: 10.5498/wjp.v5.i3.273
30. Halcomb EJ, McInnes S, Patterson C, Moxham L. Nurse-delivered interventions for mental health in primary care: a systematic review of randomized controlled trials. Fam Pract (2019) 36(1):64–71. doi: 10.1093/fampra/cmy101
31. Ostrom QT, Cote DJ, Ascha M, Kruchko C, Barnholtz-Sloan JS. Adult glioma incidence and survival by race or ethnicity in the united states from 2000 to 2014. JAMA Oncol (2018) 4(9):1254–62. doi: 10.1001/jamaoncol.2018.1789
32. Li GZ, Zhai Y, Wang Z, Wang ZL, Huang RY, Jiang HY, et al. Postoperative standard chemoradiotherapy benefits primary glioblastoma patients of all ages. Cancer Med-Us (2020) 9(6):1955–65. doi: 10.1002/cam4.2754
33. Corell A, Carstam L, Smits A, Henriksson R, Jakola AS. Age and surgical outcome of low-grade glioma in Sweden. Acta Neurol Scand (2018) 138(4):359–68. doi: 10.1111/ane.12973
34. Loppenthin K, Johansen C, Larsen MB, Forchhammer BH, Brennum J, Piil K, et al. Depressive symptoms in Danish patients with glioma and a cancer-free comprison group. J Natl Compr Canc Ne (2020) 18(9):1222. doi: 10.6004/jnccn.2020.7570
35. IJzerman-Korevaar M, Snijders TJ, de Graeff A, Teunissen SCCM, de Vos FYF. Prevalence of symptoms in glioma patients throughout the disease trajectory: a systematic review. J Neuro-Oncol Dec (2018) 140(3):485–96. doi: 10.1007/s11060-018-03015-9
36. Simon M, Schramm J. Surgical management of intracranial gliomas. Recent Results Cancer Res (2009) 171:105–24. doi: 10.1007/978-3-540-31206-2_6
37. Aghi MK, Nahed BV, Sloan AE, Ryken TC, Kalkanis SN, Olson JJ. The role of surgery in the management of patients with diffuse low grade glioma: a systematic review and evidence-based clinical practice guideline. J Neurooncol (2015) 125(3):503–30. doi: 10.1007/s11060-015-1867-1
38. Illes J, Kirschen MP, Edwards E, Stanford LR, Bandettini P, Cho MK, et al. Ethics. incidental findings in brain imaging research. Science (2006) 311(5762):783–4. doi: 10.1126/science.1124665
39. Popoola O, Olayinka O, Azizi H, Ojimba C, Khan T, Kallikkadan J, et al. Neuropsychiatric manifestations of partial agenesis of the corpus callosum: a case report and literature review. Case Rep Psychiatry (2019) 2019:5925191. doi: 10.1155/2019/5925191
40. Leibetseder A, Leitner J, Mair MJ, Meckel S, Hainfellner JA, Aichholzer M, et al. Prognostic factors in adult brainstem glioma: a tertiary care center analysis and review of the literature. J Neurol Mar (2022) 269(3):1574–90. doi: 10.1007/s00415-021-10725-0
41. Sledzinska P, Bebyn MG, Furtak J, Kowalewski J, Lewandowska MA. Prognostic and predictive biomarkers in gliomas. Int J Mol Sci (2021) 22(19). doi: 10.3390/ijms221910373
42. Azam F, Latif MF, Farooq A, Tirmazy SH, AlShahrani S, Bashir S, et al. Performance status assessment by using ECOG (Eastern cooperative oncology group) score for cancer patients by oncology healthcare professionals. Case Rep Oncol (2019) 12(3):728–36. doi: 10.1159/000503095
43. Peus D, Newcomb N, Hofer S. Appraisal of the karnofsky performance status and proposal of a simple algorithmic system for its evaluation. BMC Med Inform Decis (2013) 13. doi: 10.1186/1472-6947-13-72
Keywords: postoperative mental health disturbances, factor analysis, risk factors, glioma, predictive models
Citation: Wang Y, Zhang J, Luo C, Yao Y, Qin G and Wu J (2023) Predictive models and survival analysis of postoperative mental health disturbances in adult glioma patients. Front. Oncol. 13:1153455. doi: 10.3389/fonc.2023.1153455
Received: 29 January 2023; Accepted: 05 April 2023;
Published: 21 April 2023.
Edited by:
Teresa Somma, Federico II University Hospital, ItalyReviewed by:
Shervin Taslimi, Queen’s University, CanadaAntonio Silvani, IRCCS Carlo Besta Neurological Institute Foundation, Italy
Andrea Botturi, IRCCS Carlo Besta Neurological Institute Foundation, Italy
Copyright © 2023 Wang, Zhang, Luo, Yao, Qin and Wu. This is an open-access article distributed under the terms of the Creative Commons Attribution License (CC BY). The use, distribution or reproduction in other forums is permitted, provided the original author(s) and the copyright owner(s) are credited and that the original publication in this journal is cited, in accordance with accepted academic practice. No use, distribution or reproduction is permitted which does not comply with these terms.
*Correspondence: Ye Yao, eXlhb0BmdWRhbi5lZHUuY24=; Jinsong Wu, d3VqaW5zb25nQGh1YXNoYW4ub3JnLmNu
†These authors have contributed equally to this work
‡ORCID: Chen Lou, orcid.org/0000-0002-0344-1624