- 1Chemistry Department, University of Manitoba, Winnipeg, MB, Canada
- 2Chemistry Department, Brandon University, Brandon, MB, Canada
Glioblastoma multiforme (GBM) is a primary type of lethal brain tumor. Over the last two decades, temozolomide (TMZ) has remained the primary chemotherapy for GBM. However, TMZ resistance in GBM constitutes an underlying factor contributing to high rates of mortality. Despite intense efforts to understand the mechanisms of therapeutic resistance, there is currently a poor understanding of the molecular processes of drug resistance. For TMZ, several mechanisms linked to therapeutic resistance have been proposed. In the past decade, significant progress in the field of mass spectrometry-based proteomics has been made. This review article discusses the molecular drivers of GBM, within the context of TMZ resistance with a particular emphasis on the potential benefits and insights of using global proteomic techniques.
1 Introduction
Malignant tumors of the central nervous system (CNS) are difficult to treat and often result in poor overall patient survival (1). Glioblastoma multiforme (GBM) is a particularly aggressive tumor of the CNS. Its overall median survival of GBM patients is 12–18 months (4.6% survival rate at 5 years) (2). GBM cells are highly proliferative and infiltrative, which limits the possibility of complete tumor removal by surgical resection. In terms of clinical presentation, GBM patients experience persistent weakness, loss of vision, and alteration of speech (3). Around 14% of brain and CNS tumors belong to the category of GBM (4). Given the rare metastatic behavior of CNS tumors, the severity of the disease is graded from 1 to 4 by the World Health Organization classification of CNS tumors (5). Grade 4 glioma, also known as glioblastoma multiforme (GBM), is the most malignant type of primary CNS tumor (1).
The blood–brain barrier (BBB) involves astrocytes, neurons, and endothelial cells which regulate the transport of molecules into the brain. The BBB imposes the selective permeability of molecules. It is thought that lipophilic molecules with a molecular weight (MW) of less than 400 Da might have the possibility to pass through the BBB, whereas large and hydrophilic molecules are generally restricted (6). Hence, the BBB is an obstacle for transporting chemotherapeutics targeting various types of brain cancers including GBM (7). As a BBB-permeable drug, temozolomide (TMZ), commercially known as Temodar™, is the principal drug used to treat GBM, alongside radiation and surgical resection. Orally administered, TMZ is a DNA alkylating agent which breaks the DNA chains by attaching a methyl group to guanine at the oxygen-6 (O6), nitrogen-7 (N7), and adenine-3 (N3) positions. This generates the cytotoxic bases O6-methylguanine (O6-MG), N7-methylguanine (N7-MG), and N3-methyladenine (N3-MA) that manifest in a beneficial, clinical effect (8). During DNA replication, the mismatched base pairs induce cell-cycle arrest in the G2/M phase to induce death (2). In this regard, malignant tumors adapt. Here, a population of tumors may already possess “intrinsic” or “innate” resistance. Alternatively, tumor cells can also “acquire” or modify cellular networks to bypass the actions of the therapeutic agent. In both cases, continued exposure to these conditions applies selective pressures for cells to self-select and evolve into drug-resistant tumors (9, 10). Indeed, studies of TMZ-resistant GBMs demonstrate the activation of pathways responsible for DNA repair including 1) O6-methylguanine-DNA-methyltransferase (MGMT), 2) DNA mismatched repair (MMR) system, and 3) base excision repair (BER, the poly(ADP)-ribose polymerase (PARP) pathway) (11). Understanding of these networks and mechanisms linked to these processes may provide avenues for the development of new drugs and adjuvants.
Proteins are significant for their roles in pathophysiology, cellular biology, and molecular functions. The detailed study of the proteome is called proteomics, which investigates protein abundance, protein–protein interactions (PPI), cellular localization and functions, posttranslational modifications, and cellular signaling. Proteomics studies may be conducted under normal physiological conditions or under stress or pathological conditions (12). Conventional proteomics-based techniques such as two-dimensional polyacrylamide gel electrophoresis (2D-PAGE) and 2D differential in-gel electrophoresis (2D-DIGE) have been complemented with state-of-the-art high-throughput techniques such as liquid chromatography combined with high-resolution mass spectrometry (LC-MS). These improvements have been very useful in differentiating proteins and their expression levels in different biological systems (13). Protein identification and quantitation using these techniques can reveal differential expression of proteins and biomarkers (14).
Mass spectrometry-based proteomics can provide important insight into TMZ resistance phenotypes. The involvement of proteins in molecular function, signaling cascade, and protein–protein interactions can reveal crucial information (15). As GBM resistance to TMZ involves complex mechanisms, analyzing a large number of proteomic samples from different laboratories would provide a good chance to identify biomarkers with confidence to help in further efforts to understand TMZ resistance (16). The creation of an international LC-MS proteomics database of TMZ-resistant cells would be an important step in this direction, and this topic will be discussed at the end of this article.
2 Background
2.1 Brief details on glioblastoma multiforme and tumors of the CNS
As per the International Agency for Research on Cancer (IARC) database, brain and CNS cancers contribute to 3% of deaths among all other types of cancers (17). According to IARC, by 2040 there will be almost a double-fold increase (~46.5%) in deaths due to CNS tumors. In a more optimistic outlook, advances in immunohistology and molecular biology have revolutionized the understanding of CNS tumors, which is crucial for the development of therapies. The first edition of histological typing of CNS tumors was presented in 1979 to the WHO in Geneva (18). In 1993, the second edition revealed that histological typing of the tumors based on immunohistology was very useful (19). In 2000, a WHO working group classified CNS tumors based on histology and considered additional genetic background information in order to clarify disease diagnosis. The 2000 edition included data based on science, clinical signs and symptoms, imaging, and survival predictions (20). Further developments in molecular biology, mainly in genetics, provided characteristic molecular information on CNS tumors. Hence, in 2016 the fourth edition was released with considerable information on the latest pieces of evidence, combining histology with genetics, to understand various types of CNS tumors. A new classification of CNS tumors was published by WHO in 2016 (1). This revised classification incorporated molecular features and histogenesis of CNS tumors, whereas the previous WHO classification (2007) was based only on histological characteristics. The 2016 classification represents a paradigm shift in neuro-oncology and provides well-defined criteria for tumor definition, characterization, nomenclature, diagnosis, and treatment options. These drastic changes constituted major steps forward for strategic planning of patient treatments.
Anatomically, the CNS is made up of two types of cells: neurons and neuroglia. Neuroglia are non-neuronal cells representing half of the volume of the brain. Their function is to support, nourish, and protect the neurons, and they have the ability to divide and grow during their lifetime (Figure 1) (25). Neuroglia are also known as glia and are the most abundant cells in the CNS. There are four types of neuroglial cells: astrocytes, oligodendrocytes, microglia, and ependymal cells. Tumors arising from these cells are known as gliomas and are highly malignant in nature. A proportion of 29.1% of primary brain and other CNS tumors are malignant, and 70.9% are non-malignant. In the USA only, there was a projection of 88,970 new diagnoses of CNS tumors in 2022 (26). Also in the USA, an average of 16,606 deaths per year are reported, corresponding to 4.43 per 100,000 people (26). Glioblastoma multiforme (GBM) was the highest type of malignant tumor reported (14.3% of all tumors) with more frequency in men than in women. After diagnosis, the 5-year survival rate for patients with malignant brain and CNS tumors was 66.9% (26). Among all CNS tumors, GBM had the lowest median observed survival rate of only 8 months (26).
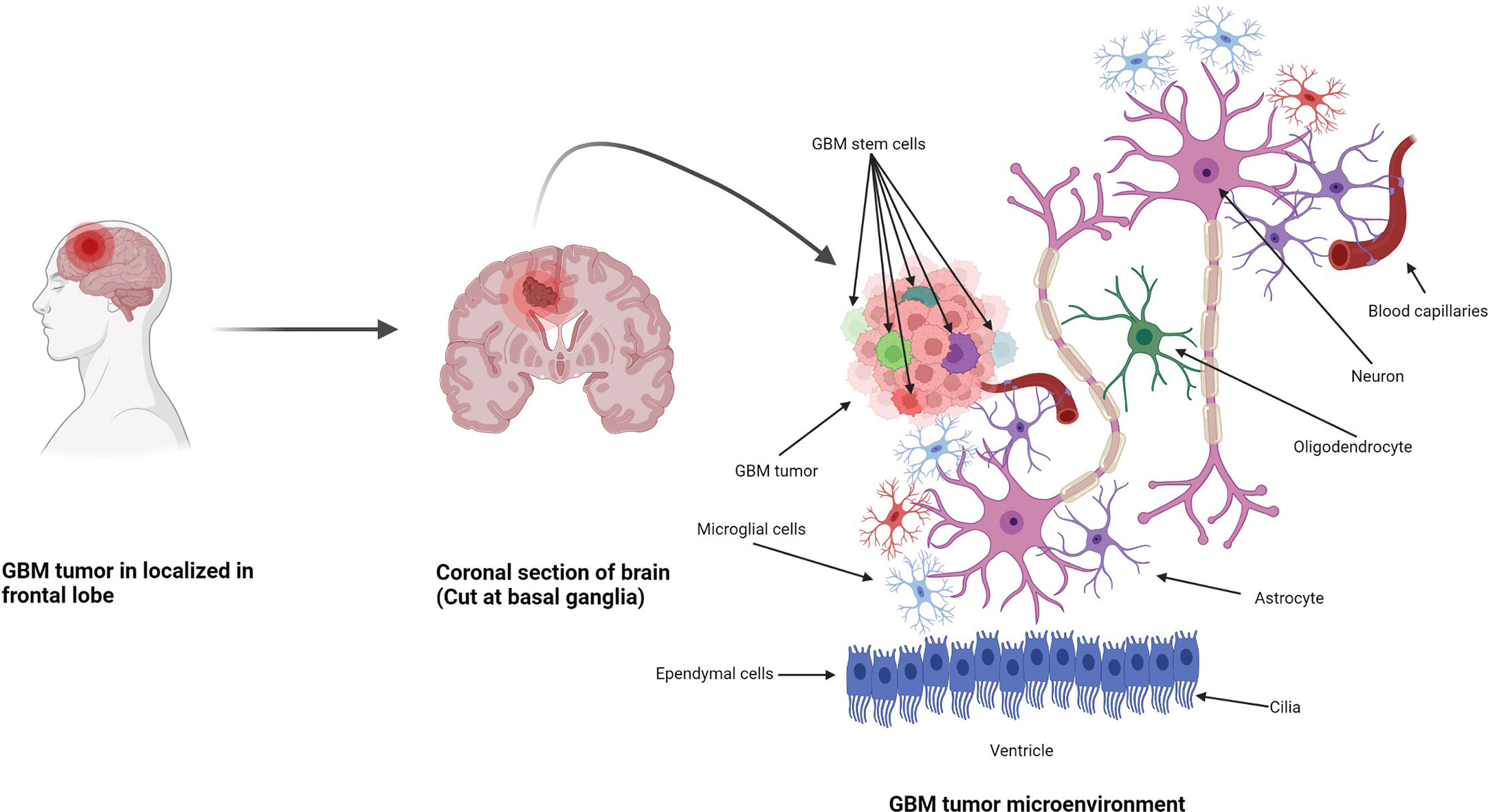
Figure 1 Formation of GBM tumors and their microenvironment. GBM tumors are often diagnosed in the frontal lobe, temporal lobe, and cerebellum (21). GBM tumor cells are composed of heterogenous cell populations (containing GMB stem cell markers (GSCs) in green, dark red, green dark green, purple, etc.) with properties of neural stem-like cells (NSCs): self-renewal, generation into differentiated GBM cells (22). The GBM microenvironment contains a variety of glial-type-associated cells including microglia and astrocytes, which are highly associated with glioma pathophysiology (23, 24).
The most common malignant tumors are due to GBM, representing 14.3% of all tumors arising in brain and CNS. In contrast, non-malignant tumors count for 38.3% of all diagnosed tumors (26). Gender-wise, GBM is more common in males, whereas meningioma is more common in females. The 5-year relative survival rate for malignant brain and CNS tumor was 35.6% versus 91.8% for non-malignant (26). Figure 2 shows the distribution of types of tumors and highlights the difference between survival rates of patients afflicted with non-malignant and malignant CNS/brain tumors (26).
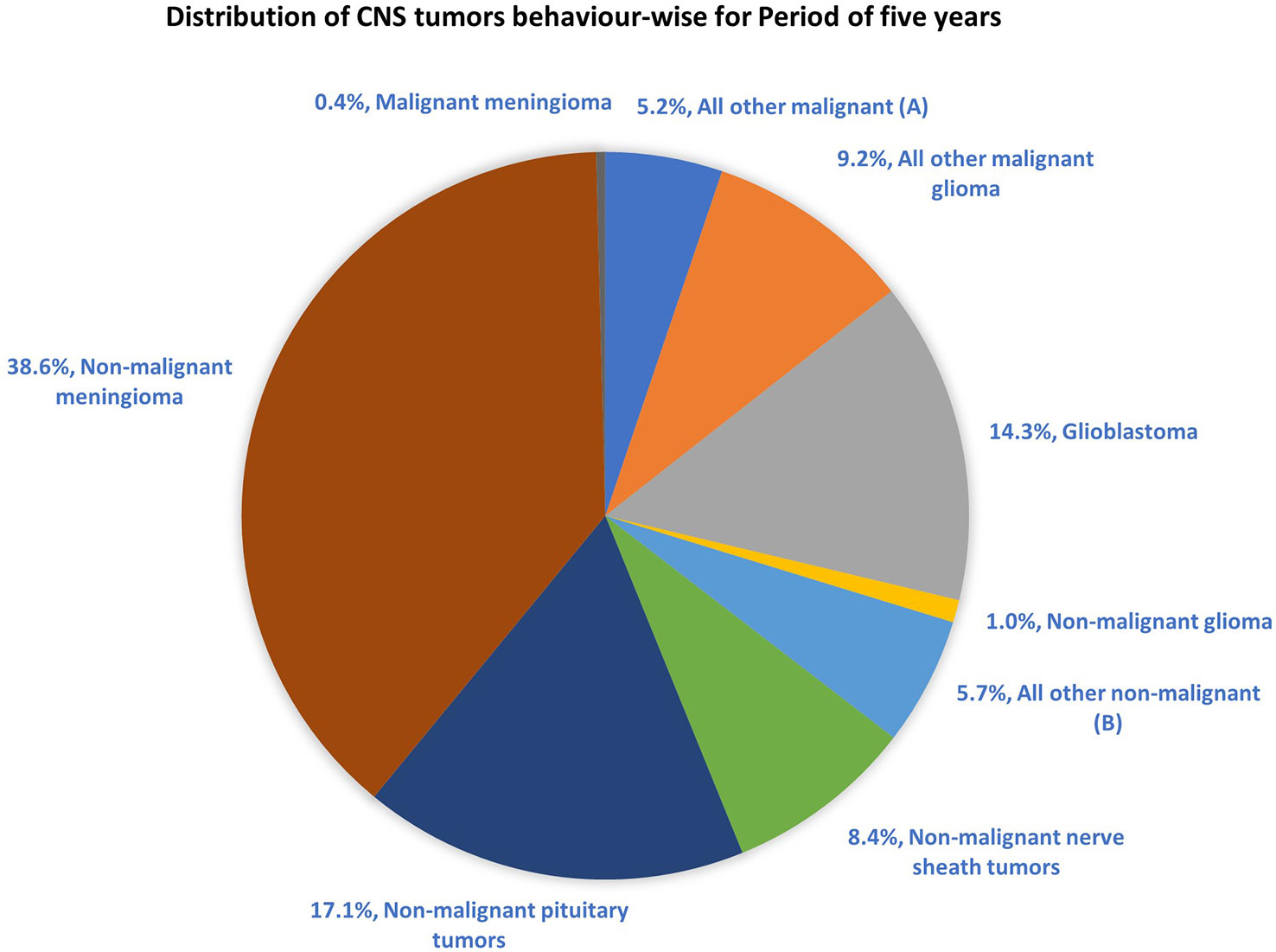
Figure 2 Distribution of primary brain and other CNS tumors by behavior (5-year total = 431,773 cases), CBTRUS statistical report (2014–2018): Malignant tumors are 29.1% and non-malignant 70.9% (26). Remark: The total percentage will not be 100, as rounding off was performed; (A, B) contain histologically different behaviors.
2.2 WHO CNS classification (2016, 2021) and isocitrate dehydrogenase profile in GBM: A major reform
In 2016, a WHO workgroup including neurologists, oncologists, histopathologists, and geneticists investigated brain and CNS tumors with the clear aim of classifying tumors based on the most advanced knowledge and on concerns expressed within workgroup members. Major key changes were incorporated into the first edition of classification based on histology in 1979, immunohistochemistry in 1993, genetics in 2000, and histopathology and genetics combined in 2007 (18, 19, 27). GBM tumors are well characterized clinically, and the 2016 CNS WHO classification is an example of how understanding the molecular biology of tumors helps to advance diagnostic and treatment trajectories (28). For GBM, incorporation of novel criteria for classification such as IHD1/IDH2 wild-type and IHD1/IDH2 (where IDH is isocitrate dehydrogenase) mutant genes led to newer grading for CNS neoplasm in GBM with improved diagnosis and focused treatment, providing superior prognosis with regard to clinical outcomes and patient survival rates (29).
3 Mechanism of action of temozolomide in GBM
Initially, Steven et al. synthesized the first-generation antineoplastic agent mitozolomide (8-carbamoyl-3-(2-chloroethyl)imidazo [5,1-d]-1,2,3,5-tetrazin-4(3H)-one. The effect of this agent was studied in L1210 mouse leukemia cells (30). Mitozolomide exerts DNA cross-linking through ethylene bridge formation. In the 1990s, the new second-generation imidazotetrazine-based chemotherapeutic prodrug, TMZ, emerged. The chemical designation of TMZ is 3-methyl-4-oxoimidaz[5,1-d][1,2,3,5]tetrazine-8-carboxamide. TMZ is part of a new class of alkylating agents with an imidazole ring (31). As a prodrug, TMZ itself is not active and does not require hepatic metabolism to create the active metabolite methyltriazen-1-yl imidazole-4-carboxamide (MTIC). The drug TMZ is absorbed efficiently after oral administration. It has time dependent antitumor activity and crosses the BBB. TMZ gets hydrolyzed at physiological pH (pH >7) into MTIC, which degrades and generates the reactive DNA methylating species methyl hydrazine (30, 32, 33). TMZ shows cytotoxicity only once it modifies its targets by addition of methyl groups at N7 (>70%) and O6 (6%) sites of guanine and N3 (9%) sites of adenine in genomic DNA (34) (Figure 3). Although occurring in a low proportion (7%), methylation of guanine at O6 (O6-MeG) is cytotoxic, mutagenic, and critical for TMZ-induced cytotoxicity (35). This methylation step damages DNA, and GBM cells will use different pathways to treat these modifications from TMZ.
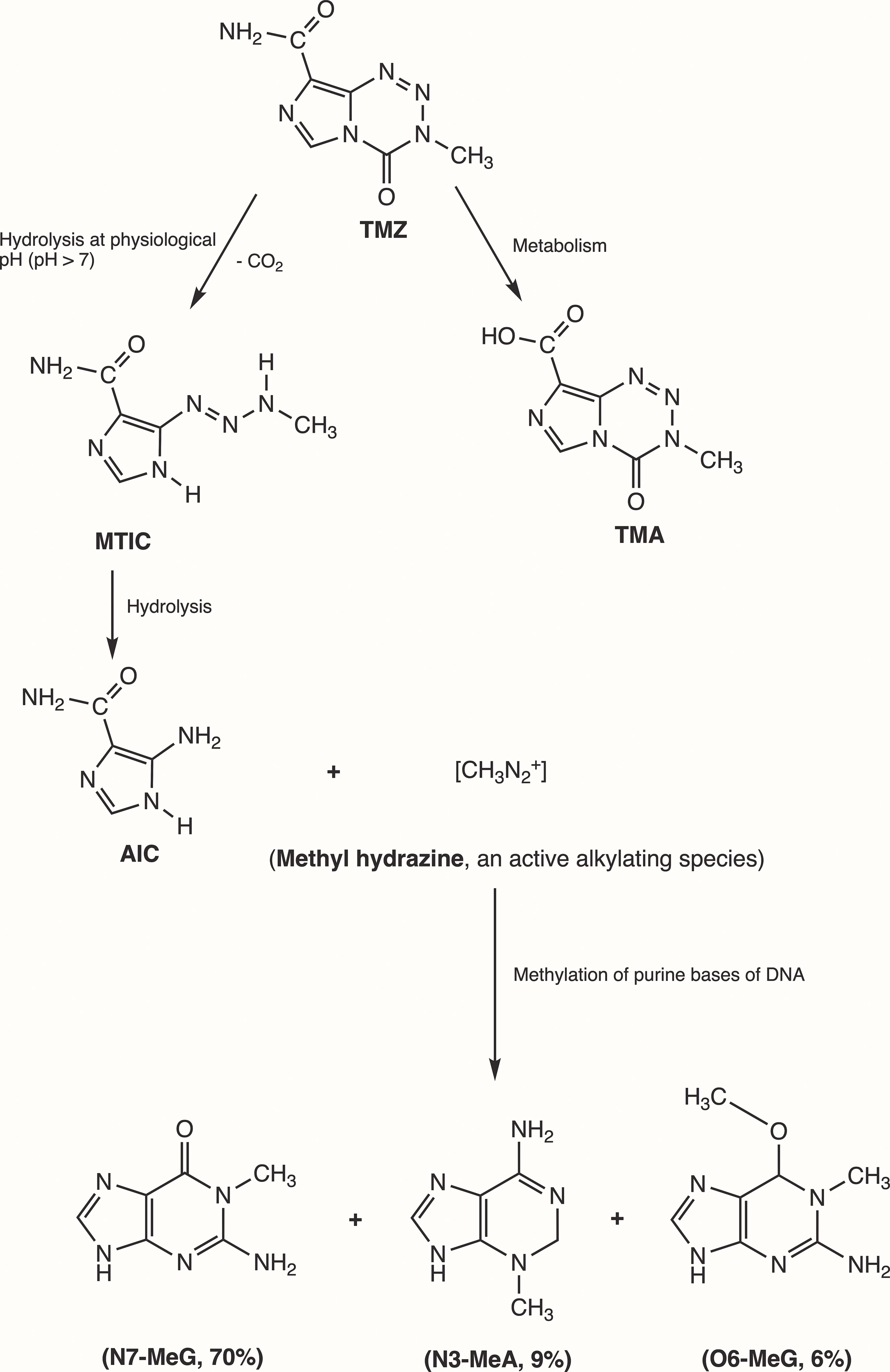
Figure 3 Mechanism of TMZ after oral administration. Activation of prodrug (TMZ) occurs at physiological pH (>7) after oral intake. TMZ is stable in an acidic environment (pH<7) and degrades rapidly by hydrolysis at basic pH. TMZ hydrolyzation results in MTIC via decarboxylation and further hydrolysis produces AIC and methyl hydrazine (DNA methylating species). The pH of brain tumors is alkaline compared with healthy surrounding tissues, resulting in increased activation of TMZ within the tumor environment (35). (TMZ, temozolomide (3-methyl-4-oxo-3H,4H-imidazo[4,3-d][1,2,3,5]tetrazine-8-carboxamide); TMA, temozolomide acid; MTIC, 5-(3-methyltriazen-1-yl)-imidazole-4-carboxamide; AIC, 5-aminoimidazole-4-carboxamide; N7-MeG, methylation at N7 position of guanine; N3-MeA, methylation at N3 position of adanine; O6-MeG; methylation at O6 position of guanine).
TMZ modification of GBM cells at the O6 site of guanine leads to DNA replication errors due to mismatched bases. Instantly, the mismatch repair (MMR) system will interrupt the replication process. When MMR enzymes recognize the mispaired thymine on the daughter strand, they will excise the mispaired fragment, whereas O6-MeG remains in the template strand. After the MMR’s attempt to remove the O6-MeG adduct, single- and double-stranded breaks in the DNA are produced, triggering cell death by apoptosis (31). Methylation of the N7 site on guanine accounts for 70% of TMZ modification in cells, but no corresponding cytotoxicity has been found. In 9% of TMZ treatment cases, N3-methyladenine is extremely toxic for cells and can block the progression of DNA replication or cause chromosome aberration, thus killing GBM cells (36). Cytotoxicity of TMZ depends on the normal MMR mechanism, and GBM cells are sensitized by TMZ when the DNA-MMR mechanism is triggered, causing double-strand DNA to break and leading to programmed cell death (37).
4 Temozolomide resistance in GBM
TMZ chemotherapy administered with radiotherapy offers significantly superior prognosis for GBM patients, and hence it is widely considered as a first-line chemotherapy regimen to treat GBM. However, two major types of resistance against the chemo- and radiotherapies, (1) intrinsic and (2) acquired, remain persistent challenges across all cancers including GBM (Figure 4) (38, 41).
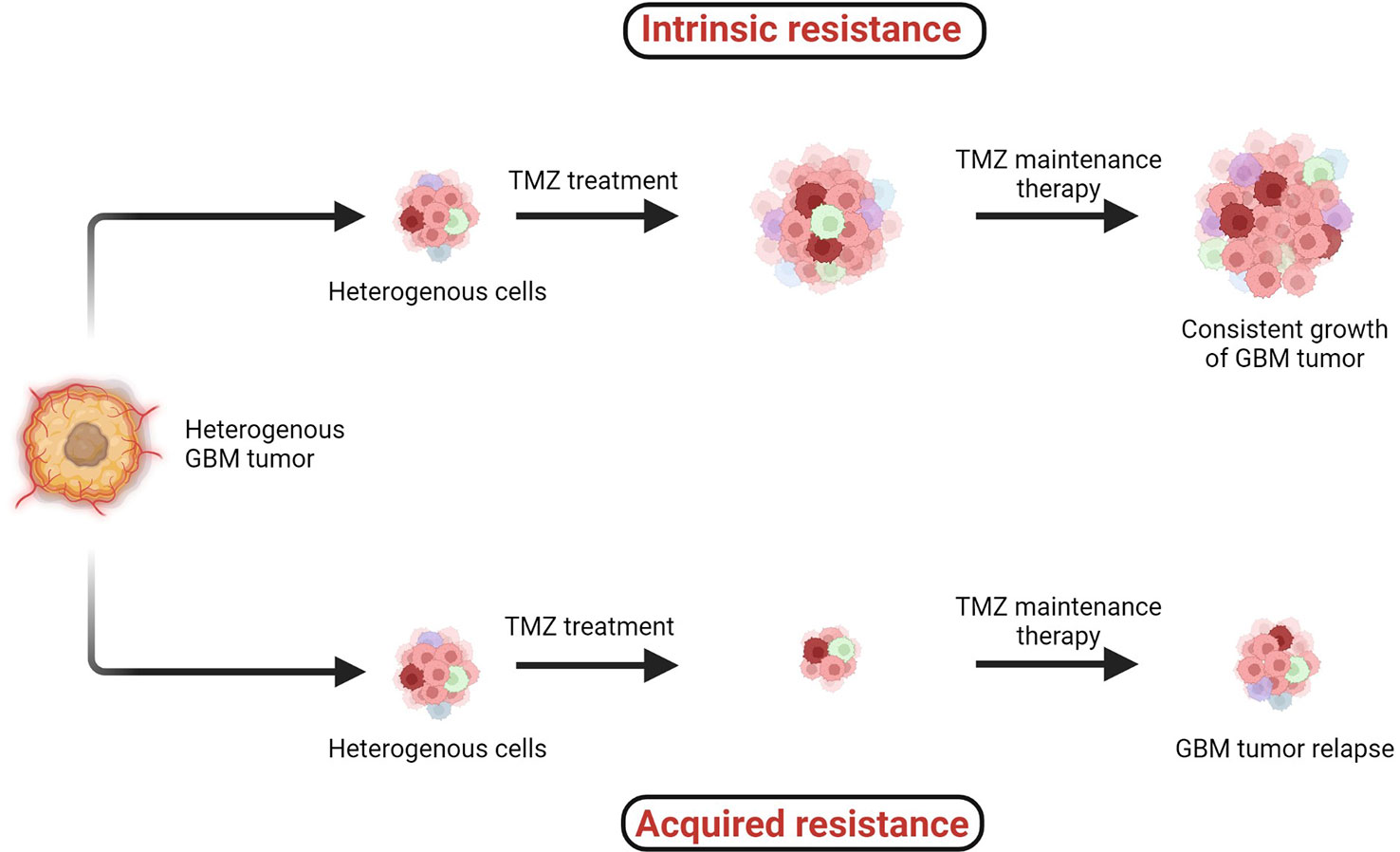
Figure 4 Two major types of resistance development: (1) intrinsic and (2) acquired resistance. Intrinsic resistant tumors contain very small amounts of or no antitumor T cells, creating natural resistance against therapies. Acquired resistance is resistance development against the therapy after clinical treatments are given (38–41).
When the specific tumorigenic subpopulation of GBM cells is intrinsically resistant, these cells maintain uncontrolled cellular proliferation before, during, and after therapeutic treatment and hence the tumor mass keeps growing. In the case of acquired resistance, a rare population of cells acquires resistance against the therapies (including radio- and chemo-) via genetic mutations or abnormal alterations in cell signaling pathways. Generally in the acquired resistance phenotype, clinical benefits of treatment are observed initially and the tumor mass is reduced; however, after a certain period of treatment due to acquisition of resistance, the tumor relapses (39).
Several cell lines have been studied extensively to understand the molecular mechanisms causing TMZ resistance in GBM. This phenomenon remains insufficiently understood as multiple molecular mechanisms are involved and need to be investigated (42). Several theories of TMZ resistance have been published; however, gaps in further detailed understanding still remain (2, 43, 44).
TMZ has been used as a frontline DNA methylating agent for GBM since its approval by USFDA in 2005. In terms of cytotoxicity, no other drugs have been found as efficient as TMZ. However, GBM cells involving high extents of DNA repair can reverse the methylation efficiency of TMZ, in which case GBM cells can become resistant against TMZ therapy. A challenge still persists, as for 50% of patients who receive TMZ as primary chemotherapy, poor survival rates are observed due to TMZ resistance in GBM (42).
Three major types of DNA repair mechanisms are responsible for TMZ resistance: MGMT, MMR, and base excision repair (BER, the poly (ADP)-ribose polymerase (PARP) pathway) (11). The primary resistance mechanism against TMZ is directly linked with high MGMT expression, whereas a secondary mechanism is linked to the MMR system in cells lacking MGMT (45–47).,, The third mechanism is the PARP pathway, which mainly involves the removal of N7-methylguanine and N3-methyladenine adducts (42). This BER pathway has minimal effects compared with MGMT and MMR. This is because the removal of N7- and N3-methyl adducts does not cause DNA double-strand breaks. When MGMT, alkylpurine-DNA-glycosylase (APNG), and BER protein are expressed, GBM cells are resistant to TMZ (42).
4.1 O6-Methylguanine-DNA methyltransferase
In GBM, MGMT is a significant role player in developing resistance against chemotherapies, including TMZ. MGMT plays a suicidal role in repairing methylation of O6-MeG lesions, resulting in the decreased cytotoxic effect of TMZ. MGMT reverses the mechanism of TMZ by removing the methyl group O6-MeG and restores DNA into its original state (48). TMZ induces DNA methylation by generating O6-methylguanine, which triggers cytotoxicity and apoptosis (30, 32). The MGMT gene is located on chromosome 10q26 and encodes a DNA-repair protein, which eliminates methyl groups from the O6 position of guanine, thus avoiding gene mutation, cell death, and tumorigenesis caused by alkylating agents (46). The epigenetic regulation of specific sites of MGMT CpG islands influences MGMT transcription (49). CpG islands are composed of short stretches of DNA with a high cytosine 5′ to guanine content, separated by a phosphodiester bond. Chemotherapy and radiotherapy may modulate the methylation level of the MGMT gene as well as protein expression. Overexpression of MGMT is an important mechanism of TMZ resistance.
MGMT can reverse the methylation of TMZ on the O6-MeG position by transferring the methyl group to an internal cysteine residue, which leads to no cytotoxicity from TMZ, thus preventing cells from destruction (TMZ resistance) (Figure 5A) (53) (54)., Monica et al. and Rodrigo et al. have discovered that if the MGMT promoter responsible for MGMT expression is methylated, then patients have higher chances of survival (55) (46)., Studies have shown that the MGMT signaling pathway also plays a role in the TMZ resistance of glioma tumor cells (53). A combination of interferon-α (IFN-α) and antiepileptic drug levetiracetam (LEV) along with TMZ has shown to cause a potential decrease in the proliferation of glioma cells as per the in vivo study of subcutaneous xenografts and orthotopic xenografts in a mouse model (56).
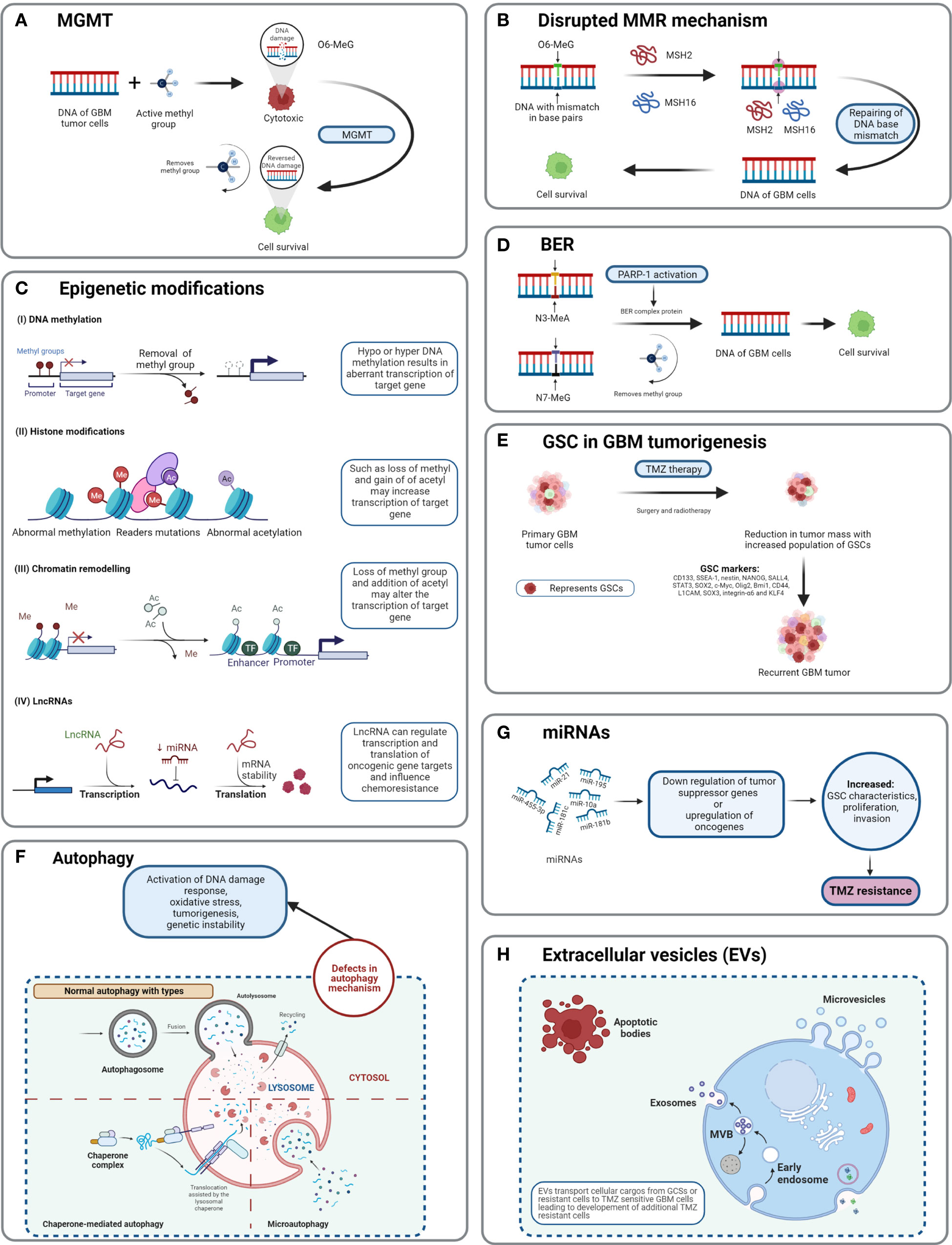
Figure 5 Detailed TMZ resistance mechanism in GBM. (A) Enzyme MGMT reverses the methylation O6-MeG by removing a methyl group, leading to increased cellular survival of GBM cells (50). (B) Disruption in the MMR mechanism repairs the mismatch between DNA bases (51). (C) Major epigenetic alterations include DNA methylation, histone modifications, chromatin remodeling, and lncRNAs (52). (D) The BER system removes or repairs DNA nucleotides. N3-MeA and N7-MeG are repaired by the BER mechanism (2). (E) GCSs are diverse and lead to generation of TMZ-resistant cells with self-renewal capabilities (52). (F) Defects in normal autophagy contribute to resistance development against TMZ (52). (G) The expression levels of miRNAs alter gene regulation, causing TMZ resistance (52). (H) Extracellular vesicles (EVs) are capable of regulating TMZ resistance related to biological material and cellular cargo (52).
4.2 Disrupted mismatch repair pathway
The cytotoxicity of TMZ is mediated mainly by a DNA-MMR mechanism on the O6-MeG base. MMR proteins are active role players in determining the efficacy of TMZ, as their deficiency or a mutation in the MMR pathway can create TMZ resistance during treatment through interruptions in the breaking of DNA-mispaired chains (11). During the MMR mechanism, thymine attempts to bind O6-MeG through the “futile DNA repair cycle” in order to fix the mismatch repair, leading to cell-cycle arrest and apoptosis (Figure 5B). The presence of MMR-deficient cells in the tumor promotes the development of resistance against standard chemotherapeutic agents such as TMZ, procarbazine, and cisplatin. Low-abundance MMR proteins such as MSH2 and MSH6 are correlated with increased TMZ resistance and tumor progression (57, 58).
4.3 Base excision repair
Base excision repair is a prevalent system to repair DNA in mammalian cells. It plays a significant role in the maintenance of genome integrity as it eliminates nucleobases with small modifications from the cell cycle. The BER mechanism recruits glycosylase, endonuclease, polymerase, and DNA ligase to repair single-nucleotide modifications such as methylation, oxidation, deamination, and single-strand breaks (SSBs) (52). Methylation adducts formed at N3-MeA and N7-MeG are very abundant (>90%); however, their cytotoxic effects are very limited owing to rapid detection and repair by BER mechanisms (59). When GBM cells treated by TMZ are marked by methylation at N3-MeA and N7-MeG, BER will detect the wrong paired nucleosides and fix the DNA chain using a lesion-specific DNA glycosylase during replication cycles (Figure 5D) (60). After the repair process, even though GBM cells were treated with TMZ, they lost N3-MeA and N7-MeG so the MMR system cannot detect any mistake in the DNA chain and cells will stay alive. Poly (ADP-ribose) (PAR) polymerase (PARP) catalyzes the synthesis of PAR. PARP-1 and PARP-2 recognize SSB produced by alkylating agents, oxidative stress, radiation, etc. However, PARP-1 is linked with the BER mechanism as a part of DNA damage response (DDR) (61, 62). Hence, several studies and clinical trials have been conducted to investigate the efficiency of PARP-1 inhibitors. However, due to the limitations of the blood–brain barrier (BBB) and heterogenous tumor response, this remains a clinical challenge (63).
4.4 GBM stem cell markers
Morphologically, GBM tumor masses possess heterogenous cells and contain subcell populations with the capacity of self-renewal and tumorigenesis known as tumor-initiating cells (TICs) or GBM stem cells (GSCs) (64). GSCs are abnormal neural stem-like cells (NSCs) which contribute to the development of pathological heterogeneity in astroglial tumors. GSCs are involved in pathological gliomagenesis (65). GBMs composed of GSCs are capable of creating spheres known as “neurospheres” which can regenerate new spheres and contribute to tumorigenesis (22). In addition to causing abnormal cellular proliferation and tumorigenesis, the GSC subpopulation is also involved in resistance developed against chemo- and radiotherapies (66).
As TMZ is still a standard key chemotherapy for GBM treatment, TMZ resistance makes GBM non-curable. This calls for the study of signature molecules to explore alternative options for adjuvant therapies (67). Reported GSC markers are CD133, SSEA-1, nestin, NANOG, SALL4, STAT3, SOX2, c-Myc, Olig2, Bmi1, CD44, L1CAM, SOX3, integrin-α6, and KLF4 (Figure 5E) (68–71),,, Fundamental research on GSCs and associated molecular markers demonstrates that GSCs are capable of tumorigenesis, self-renewal, differentiation, and resistance to chemo- and radiotherapies (2, 69, 72–74). In vitro studies indicate the potential of GSCs in forming tumor spheroids. In addition, in vivo xenotransplantation of GSCs into immunocompromised mice via subcutaneous cell injection reforms tumors with identical histological characteristics (75). GCSs treated with various common antineoplastic agents (etoposide, camptothecin, cisplatin, TMZ, doxorubicin, vincristine, etc.) exhibited significant resistance against chemotherapies and in some cases recovery followed by cell survival and proliferation (75, 76).
4.5 Autophagy
Autophagy is an intracellular degradation process, which disintegrates the cytoplasmic components using lysosomal machineries (77). In other words, a cell can self-digest its own cellular components in the lysosome (78). Autophagy becomes important during starvation and cellular stress whereby organelles, cytoplasm, and cellular proteins are engulfed, consumed, and recycled to maintain normal physiological activity in the body (79). Based on physiological functions and modes of transportation of cytoplasmic components to lysosomes, three forms of autophagy are defined: chaperone-mediated autophagy, microautophagy, and macroautophagy (normally known as autophagy) (Figure 5F) (78). Autophagy is generally seen as important in cancer prevention. Controversially, it has been suggested that under neoplastic conditions autophagy can promote cell survival (80). Defects in the autophagy mechanism can result in cancer and be associated with oxidative stress, activation of DNA damage response, tumorigenesis, and genome instability (81). Normal physiological autophagy events exert a cytoprotective effect by degrading misfolded proteins, damaged organelles, and reactive oxygen species. This results in regulation of aberrant mutations and ultimately cancer (82). Defects in autophagy genes or autophagy mechanisms can lead to neoplastic conditions such as allelic loss of the beclin1 tumor-suppressor gene in epithelial ovarian carcinoma (83). Autophagy keeps a paradoxal double role as it can both suppress and promote tumors (82, 84).
4.6 Epigenetic modifications
Epigenetic alteration is one of the several mechanisms of cancer drug resistance. The “epigenetic” term was coined by Conrad Waddington and defined as “the branch of biology which studies the causal interactions between genes and their products, which bring the phenotype into being” (85). With further research and more detailed understanding of the epigenetic mechanism, the definition of epigenetics has evolved. Recently, it has been defined as “heritable changes in gene expression without changing the DNA sequence” (86). The main epigenetic modifications are DNA methylation of cytosine, acetylation/deacetylation of histone proteins, and nucleosome positioning. In DNA methylation, the methyl group is attached to the 5′ position of cytosine on the CpG island, whereas the histone modification involves methylation, acetylation, reader mutations, and phosphorylation of histone proteins (87). Understanding the molecular mechanism of TMZ resistance at the epigenetic level can provide a novel approach to identify new targets that can restore the efficiency of TMZ. A recent study on long non-coding RNAs (lncRNAs) indicates that LncRNA SOX2OT (sex determining region Y-box 2 overlapping transcript) elevates SOX2 expression and is associated with tumor growth and poor prognosis (88). Aberrant expression of lncRNAs is associated with therapy-resistant glioma or GBM. Oncogenic lncRNAs such as MALAT1, NEAT1, H19, MIAT, UCA, HIF1A-AS2, XIST, and HOTAIR are significantly influenced by chemotherapeutic agent TMZ (89, 90). Developing inhibitors against such epigenetic alterations could be beneficial in terms of improving the clinical advantages provided by standard therapies. Histone acetylation and deacetylation are two major events involved in histone-related epigenetic alterations. Histone acetyl transferase (HAT) adds an acetyl group to histone, whereas histone deacetylase (HDAC) removes an acetyl group (91). Such epigenetic modifications support the acquisition of adaptive TMZ resistance during treatment. Specific genes emerge to extend cell survival and proliferation (92). Epigenetic modifications such as DNA methylation, histone acetylation/deacetylation, chromatic remodeling, and lncRNAs have been explained in GBM (Figure 5C) (93). To obtain clinical benefits from a TMZ chemotherapeutic regime, many clinical studies are in development to test and develop histone deacetylation inhibitors (93). To target epigenetic mechanisms, inhibitors have been designed and are presently under clinical trial investigation (phase I/II/II). Their use would be in a combined therapy along with TMZ as the primary treatment (93).
4.7 MicroRNAs
MicroRNAs (miRNAs) are single-stranded, non-coding regulatory RNAs that contain 22–25 nucleotide bases (94). They are well studied for their involvement in neoplasticity of GBM tumors (95). MicroRNAs are thought to act as posttranscriptional regulators in gene expression and in cell proliferation, angiogenesis, and generation of CSCs (96). MicroRNAs are not only capable of driving the neoplastic behavior of GBM but also key in the acquisition of TMZ resistance. For example, miR-21, miR-195, miR-455-3p, miR-10a, miR-181b, and miR-181c are reported to be heavily involved in TMZ resistance (Figure 5G) (97). Targeting miRNAs using inhibitors can restore the cytotoxicity of TMZ, such as by transfecting overexpressing miR-21 GBM cells with miR-21hibitors, which reveals resensitization of TMZ-resistant cells. MicroRNAs are receiving attention as biomarkers and as potential therapeutics that target GBM (97).
4.8 Extracellular vesicles
Extracellular vesicles are heterogeneous, vesicular, bilayer lipid structures of varying sizes (50 to 1000 nm) released by all cells. From those cells, they carry a molecular cargo such as lipids, proteins, DNA, mRNA, and miRNAs (67). Common extracellular vesicles (EVs) such as exosomes, microvesicles, oncosomes, and microparticles are involved in various cancers including breast, prostate, GBM, gastric, and colorectal (Figure 5H). Proteomics analysis of EVs shows that proteins involved in the cell adhesion pathway are involved during TMZ treatment and responsible for drug resistance (98). The mediator role of EVs in intercellular communication in tumor microenvironments affects chemotherapy treatments. Hypoxia is associated with tumorigenesis and induces chemoresistance in GBM. Particularly, GCS-derived EVs have shown to increase TMZ resistance significantly through miR-30b-3p (99). The capability of EVs to transfer lipids and proteins to tumor microenvironments promotes invasion, angiogenesis, and resistance against anticancer drugs (100). Despite limited knowledge of the mechanisms of secretion and anticancer promotion by EVs, additional research work is needed to clearly understand the cargo capacity of EVs.
5 Molecular bases for CNS tumors, GBM classification, and TMZ resistance
One topic of interest is the genetic background of GBM and TMZ resistance. The 2016 classification of CNS tumors is based on an integrated approach for diagnosis to achieve reproducibility, clinicopathological prediction, and treatment planning (29). According to this integrated method, the disease should be diagnosed based on histology and molecular information to justify the types or specific subtypes of tumors, including GBM. Mutations in markers either are early indicators of tumorigenesis or represent the specific progress of cancer such as in the case of high- or low-grade glioma tumors (29). The identification and assessment of such markers determine the clinical outcomes and specifically if the first line of treatment of TMZ would be beneficial to the patients or not and if additional adjuvant therapies are needed. Characteristic molecular markers for CNS tumors and GBM are described below in correlation with TMZ treatment outcomes.
5.1 Isocitrate dehydrogenases
IDH, metabolic enzymes, are categorized as IDH1, IDH2, and IDH3. The enzyme IDH1 is localized in the cytoplasm and in the peroxisomes (101). It is involved in lipid metabolism and glucose sensing. Enzymes IDH2 and IDH3 are present in mitochondria and involved in the Krebs cycle (102). As key functions, all IDHs catalyze oxidative decarboxylation of isocitrate and transform it into α-ketoglutarate (αKG) in the citric acid cycle (103). Enzymes IDH1 and IDH2 are associated with unique tumor-cell metabolism. For the first time, Parsons et al. discovered mutations in IDH in human GBM (104, 105). As per the 2016 WHO CNS classification, GBMs are of two types based on IDH status: (1) GBM-IDH-wt (wild type) and (2) GBM-IDH-mut (mutant) (28). Details about the mechanism, characteristics, and impact on median overall survival (OS) for GBM-IDH-wt and GBM-IDH-mut are shown in Figure 6. GBM patients with IDH-wt represent poor OS (around 15 months) in comparison with those with IDH-mut (around 36 months) (108). In another study, the same was reported, in that glioma patients with IDH1 or IDH2 mutations had a better outcome compared with patients with IDH-wt where no IDH mutation was observed (105). IDH1 and IDH2 mutations are commonly found in low-grade glioma (70% of WHO grade 2 and 3 astrocytomas and oligodendrogliomas) and secondary GBM (which is developed from lower-grade gliomas). Primary GBMs rarely show the IDH mutations. IDH1 mutation is correlated with MGMT promoter methylation (109), and patients with the hypermethylated MGMT promoter are sensitized to TMZ treatment (109).
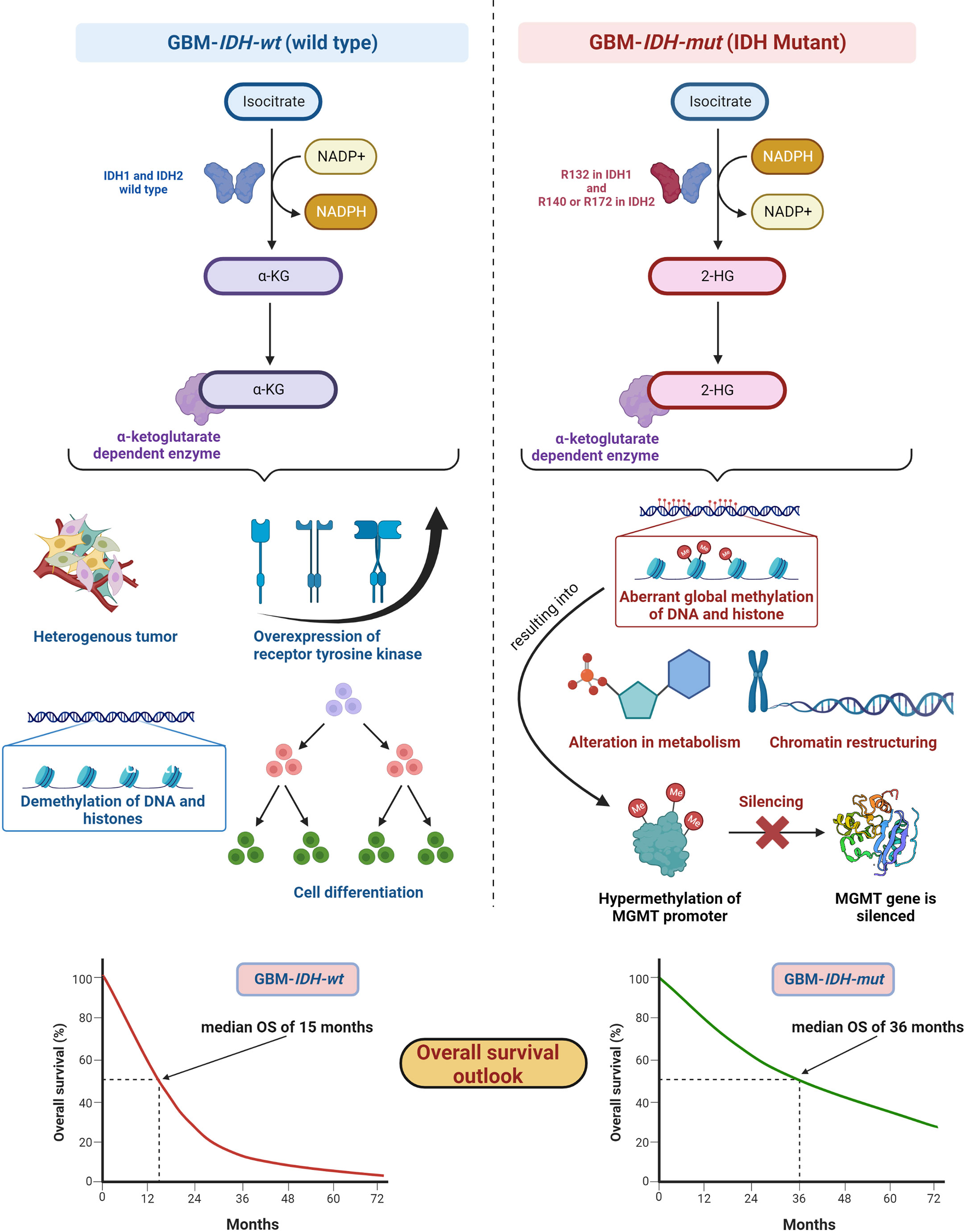
Figure 6 IDH status in GBM (1) GBM-IDH-wt and (2) GBM-IDH-mut: IDH-1 is localized in cytoplasm whereas IDH-2 is mitochondrial. In normal conditions, IDH1 and IDH2 catalyze substrate isocitrate to α-KG. Mutation on arginine on 132 to histidine (R132H) is most frequent mutation of IDH-1 in GBM; however, R140 or R172 is a common mutation in IDH-2 (106). IDH-mutation generates 2-HG as an oncometabolite. 2-HG create intense effect on cellular system via alteration in metabolism, chromatin restructuring, and aberrant global methylation of DNA and histone, which further hypermethylates MGMT promoter and consequently silences MGMT gene resulting into extended OS compared with IDH wt-type. IDH-wt results into heterogeneous tumor formation, alteration in receptor tyrosine kinases (EGFR and PDGFRA), demethylation of DNA and histones, and cell differentiation (107). Median OS for IDH-GBM-mut is 36 months however it is only 15 months in case of GBM-IDH-wt.
GBM-IDH-wt represents the characteristic of interpatient differences, heterogeneous tumors, overexpression of receptor tyrosine kinase, normal methylation of DNA and histones, and significant cell differentiation (107). Due to the larger proliferative and invasive capacity of IDH-wt type tumors than IDH-mut, the median OS of GBM-IDH-wt is much shorter compared with GBM-IDH-mut. IDH-mut decreases the normal catalytic activity and produces lower amounts of α-ketoglutarate (α-KG) and NADPH. Lowering the amount of α-KG results in the generation of 2-hydroxyglutarate (2-HG), an oncometabolite, using NADPH (109, 110). The accumulation of 2-HG increases the local and genome-wide methylation pattern. Hence, hypermethylation of the MGMT promoter silences the overall MGMT expression in patients, leading to increased toxicity of TMZ (105, 111). Additionally, IDH1 or IDH2 mutations were found in colorectal cancer, prostate cancer, thyroid carcinoma, melanoma, and acute myeloid leukemia (112).
Fundamentally, the detection of IDH mutations in GBM denotes an early episode of gliomagenesis and the presence or absence of such mutations determines the disease progression and therapeutic outcomes (113, 114). Considering these facts, IDH mutants or wild-type profiles have become reliable diagnostic and prognostic markers in GBM (115). The GBM-IDH-wt status indicates the shorter median OS of around 15 months. The 2016 CNS WHO classification, which incorporated molecular markers as part of layered information for an integrated diagnostic approach, was a vital step forward. Detection of IDH status in GBM with the other markers became an essential part of standard diagnosis of CNS tumors (29).
5.2 The biomarker O6-methylguanine-DNA methyltransferase
Higher MGMT expression levels correspond to poor clinical outcome for patients; hence, MGMT levels are an important factor in determining the therapeutic benefits of TMZ. MGMT protein levels vary according to organs, with highest levels (liver) and relatively low levels (brain). Usually, MGMT levels in tumors are higher than in the healthy tissue of origin. The CpG islands of MGMT genes are not methylated in normal tissues (46). However, methyl-CpG-binding proteins alter the chromatin structures and prevent the binding of transcription factors, resulting in silencing of the MGMT gene. It has been shown that TMZ chemotherapy is more efficient when lower levels of MGMT protein are present. In such cases, patients have longer OS rates and progression-free survival (PSF) rates (116). Glioma patients with MGMT CpG promoter methylation have shown prolonged PFS and OS (117, 118). In the MGMT gene, methylation of the CpG island inhibits transcription of the gene, and cell lines with the methylated promoter of MGMT cannot repair alkylation in O6-methylguanine.
Efforts have been made to improve the antitumor effects of TMZ including development of pseudosubstrates, interfering RNA (RNAi), viral proteins, and many other agents. It has been revealed that cell exposure to alkylating agents induces nuclear factor-kappa B (NF-κB) activation, which increases MGMT expression.
5.3 BRAF
The v-raf murine sarcoma viral oncogene homolog B1 (BRAF) is associated with RAF serine/threonine protein kinases. The three RAF family proteins are RAF1/CRAF, BRAF, and ARAF. BRAF mutations were identified in various cancers including 59% in melanomas, 18% in colorectal cancers, 11% in gliomas, and 14% in liver cancers (119). With regard to primary brain tumors, V600E mutations and KIAA1549–BRAF fusions are the most commonly observed type of mutations. Firstly, BRAF-V600E mutations (valine substituted with glutamic acid at position 600) are present in gliomas including pleomorphic xanthoastrocytoma, ganglioglioma, pilocytic astrocytoma, low-grade gliomas and pediatric GBM. Secondly, KIAA1549-BRAF fusion is the most common BRAF alteration in pilocytic astrocytoma. This is also commonly found in malignant melanomas, papillary thyroid carcinomas, and colorectal carcinomas (120).
The BRAF protein participates in the cascade of the Ras-Raf-MEK-extracellular signal-regulated kinase (ERK) or mitogen-activated protein kinase (MAPK)/ERK signaling pathway, which affects cell division and cellular differentiation. Gliomas along with BRAF mutations also show additional alterations in tumor protein P53 (TP53), TERTp, CDKN2A/B, and PTEN and favor the response to BRAF and/or MEK inhibitors (121). Various RAF inhibitors (Vemurafenib™, Dabrafenib™, Encorafenib™) and MEK inhibitors (Cobimetinib™, Trametinib™, Binimetinib™) have been approved to improve clinical output in the treatment of various cancers. However, if CNS tumors show heterogeneity, resistance development against inhibitors does not provide guaranteed therapeutic benefits from the treatments (120).
5.4 EGFR and PTEN
The epidermal growth factor receptor (EGFR) is a cell surface receptor and tyrosine kinase, which shows amplification and/or mutation in several types of cancers. EGFR has been identified as a contributor to tumor growth (122). Four members of the EGFR family (ErbB1 (EGFR), ErbB2, ErbB3, ErbB4) are known to be actively involved in cell division, differentiation, and apoptosis (123). EGFR overexpression and gene alteration are regularly observed in rapidly developing primary GBM and do not signify the existence of less malignant precursor lesions. It has been proven that EGFR signaling is often interrupted by frequent events related to EGFR gene amplification (protein overexpression) and mutation in the neoplasm (124). Tumorigenic cancer stem cells (CSCs) are well recognized for their role in aggressive disease progression and recurrence (74). The heterogeneity of CNS tumors is due to various factors including the generation of CSC as the disease progresses. CSC are capable of self-renewal, constant spreading in surrounding areas including healthy cells or tissues, and tumorigenesis upon secondary transplantation (74, 125). It has been revealed that tumorigenic glioma stem cells populations show chemo- and radio-resistance after treatment in glioma (74, 126, 127). Alterations in EGFR oncogenes are observed in half of the primary GBMs and result in resistance against chemo- and radiation therapies. Recently, efforts have been made to fight resistance by targeting EGFR and DNA using “combi-molecules” (designed to aim at two targets in cancer cells) (66). Various therapeutics such as monoclonal antibodies (Cetuximab™, Nimotuzumab™), inhibitors (Gefitinib™, Erlotinib™) are known to trigger different EGFR signaling pathways to enhance the survival of cancer cells and to develop resistance against therapeutic agents (128). Cellular stress introduced by chemotherapy has shown similar effects. Specifically, the EGFR variant III (EGFRvIII) mutation is the most common in GBM and is associated with tumorigenicity and poor prognosis in GBM patients (129). The exact mechanism for generating EGFRvIII is still unclear; however, its presence increases cell proliferation (123). For increased overall survival of patients, various inhibitors and adjuvants have been developed, although with limited success. Several small-molecule- and antibody-based therapies have been implemented, but development of drug resistance followed by secondary resistance against these inhibitors has resulted in poor clinical outcomes (130, 131).
The deletion of phosphatase and tensin homologs on chromosome 10 (PTEN) results in a tumor-suppressor gene, which regulates cellular growth, proliferation, survival, apoptosis, metabolism, and cell migration and also carries functional roles in the nervous system. PTEN mutations have been observed in older over-survived patients; however, there is no strong connection with over-survival (132).
From a clinical standpoint, there are two types of GBM: primary and secondary. Primary GBM occurs more often (~90% of cases) with tumors that develop de novo, often in older patients and without any history of less malignant forms of precursor lesions. Secondary GBM develops gradually from lower-grade diffuse astrocytoma or anaplastic astrocytoma in younger patients at diagnosis. From a genetics perspective, primary GBM manifests EGFR amplification, PTEN mutation, and the entire loss of chromosome 10, whereas secondary GBM shows frequent mutations in the TP53 gene (encodes tumor-suppressor protein p53) with longer overall survival (133). However, recent studies indicate that TP53 mutations are crucial roles players in developing radio-resistance and are associated with poor survival in specific CNS-related neoplasms (134, 135).
5.5 Alpha thalassemia X-linked intellectual disability and tumor protein P53
Alpha thalassemia X-linked intellectual disability (ATRX) belongs to the SWI/SNF2 (SWItch/sucrose non-fermentable) family of chromatin remodeling proteins. The ATRX gene was first found in patients with the alpha thalassemia X-linked intellectual disability syndrome (136). A mutation in ATRX leads to the “alternative lengthening of telomeres” (ALT) phenotype and to genomic destabilization (137).
The wild-type tumor protein TP53 plays an inhibitory role against cellular growth when DNA damage occurs. A mutation in the TP53 gene changes the protein function, leading to failure in preventing cellular replication and promoting proliferation of neoplastic astrocytoma (138).
Four molecular features that differentiate diffuse astrocytoma and oligodendroglioma are IDH wild type/mutants, TP53 mutations, ATRX loss, and 1p19q codeletion. Diffuse astrocytomas are either IDH mutants with ATRX loss and TP53 mutations, or IDH wild type. IDH mutants with 1p/19q codeletion are characterized as oligodendrogliomas (139). The ATRX mutation shows rapid progression of glioma tumors vs. wild type and hence has therapeutic potential (140).
6 LC-MS-based proteomics studies in human GBM and TMZ resistance
Several TMZ resistance mechanisms have been discussed in the literature. Still, the TMZ-resistance hurdle has proven to be a significant challenge due to the complex nature of GBM (Figure 7). The TMZ resistance mechanism is very complex, and studying cell signaling pathways and protein–protein interactions (PPI) can provide crucial information on the matter. Finding early signature biomarkers can also be key in adjusting the treatment strategy (144). OMICS research with high-throughput technologies such as next-generation DNA sequencing, proteomics by liquid chromatography-mass spectrometry (LC-MS/MS), metabolomics, and genomics has revealed its potential for helping to understand various forms of cancers at the molecular level (145). In particular, LC-MS/MS-based proteomics has widely served the OMICS purpose in cancer cell biology and drug resistance mechanisms.
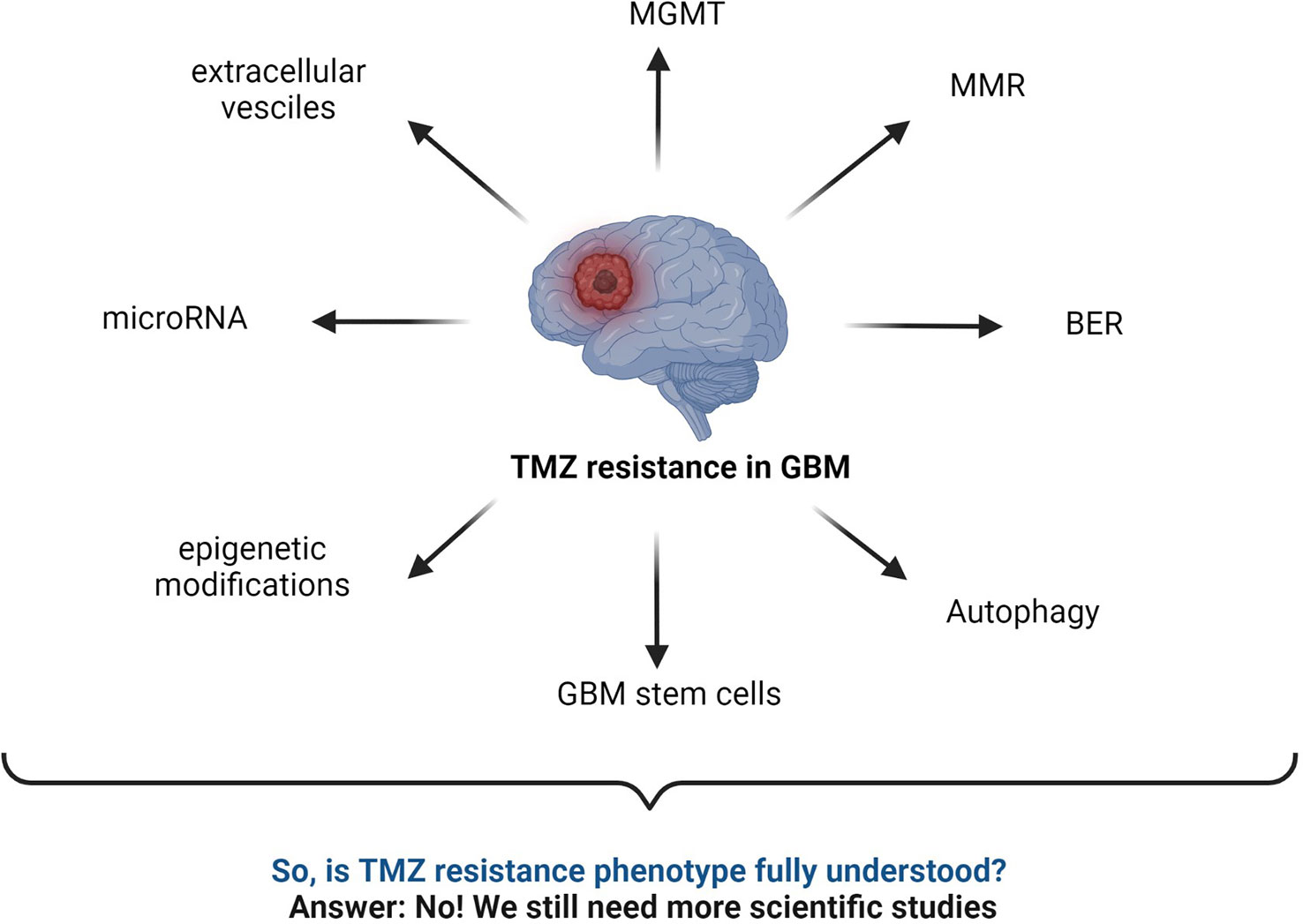
Figure 7 Summary of commonly known mechanisms for TMZ resistance in GBM. MGMT status, BER mechanism, interrupted MMR mechanism, autophagy, microRNA, GBM stem cells, epigenetic modifications, extracellular vesicles, etc., are major responsible factors reported for TMZ resistance (2, 43, 52, 59, 141–143).
Despite remarkable advances in the molecular biology towards understanding neoplastic phenotypes, the therapeutic success rate is still low for many types of cancers. The limitations of clinical benefits from chemotherapies in the treatment of GBM indicate that there is still a considerable amount of research necessary to gain more understanding of drug resistance mechanisms. Mutations are not fully understood yet, and alternative pathways of chemotherapeutic resistance are activated when specific proteins are targeted during treatment. Proteomic studies offer insightful approaches to better understand the downstream effects of cancer-related genotypes (146).
This section discusses possible LC-MS-based workflows to study cellular proteomics with the goal of better understanding resistance phenotypes in GBM. For years, proteomics has gained a very important role in glioma research, both for the investigation of disease pathobiology and for the development of efficient therapeutics against GBM and other forms of tumors. In addition, glioma proteomics can potentially identify biomarkers, which is very helpful for diagnosis, treatment decisions, prognosis, and assessment of treatment response (147). Not only the primary (amino acid sequence) and secondary structures can account for the function of proteins, but posttranslational modifications such as glycosylation, acetylation, and phosphorylation can directly affect how proteins function. Advanced high-throughput LC-MS-based proteomics is one of the indispensable techniques in cancer biomarker discovery. Recent advances in chromatography and MS technology have revealed the remarkable capabilities of high sensitivity and high-resolution multiplexed quantitation. LC-MS/MS-based proteomics is used to identify differences in protein expression and lends itself to pathway analysis in various cancerous tumors with radio- and chemoresistance phenotypes (148).
A typical LC-MS proteomics workflow includes selection of sample type (cancerous cells, tumor, tissue, and secreted media), extraction of proteins, LC-MS/MS analysis, database search, and interpretation (Figure 8-top). Accurate and efficient quantification of proteins using the labeling of peptides is a very popular approach in LC-MS-based proteomics, e.g., label-free (Figure 8-bottom), tandem mass tag (TMT) labeling, and iTRAQ (isobaric tagging for relative and absolute quantification) (Figure 9). These methods are used to perform multiplexed and simultaneous quantification of proteins and further identification.
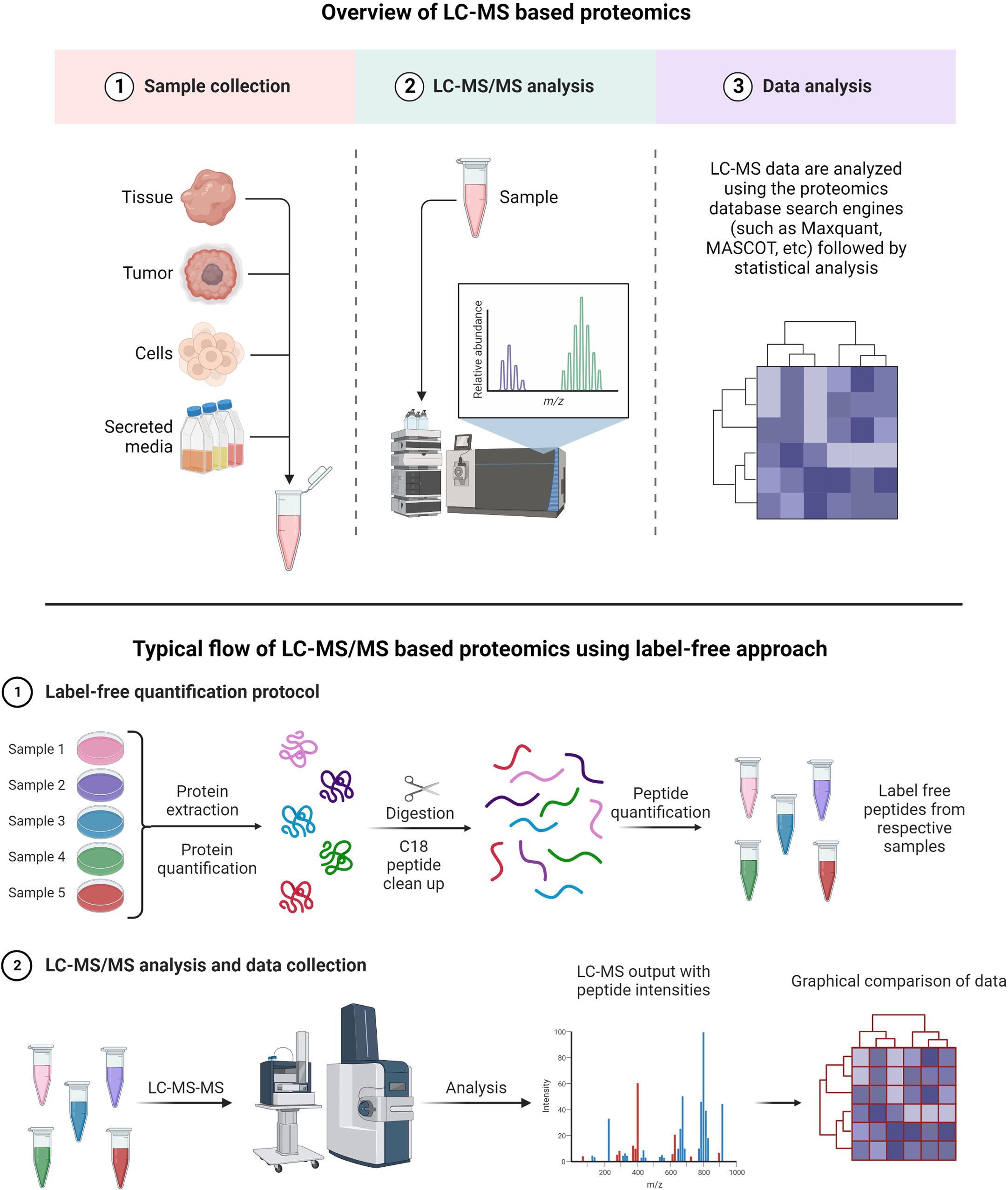
Figure 8 Example of proteomics workflows. Top figure: Biological samples such as tumors, cells, tissues, and secreted media are processed to extract the proteins. Extracted proteins are digested and analyzed by LC-MS/MS. Raw files generated from LC-MS/MS are used in a proteomics database search engine for protein identification and quantitation. Bottom figure: tandem mass tag (TMT) labeling is used to perform multiplex analysis for simultaneous relative quantification of proteins and for identification (149, 150).
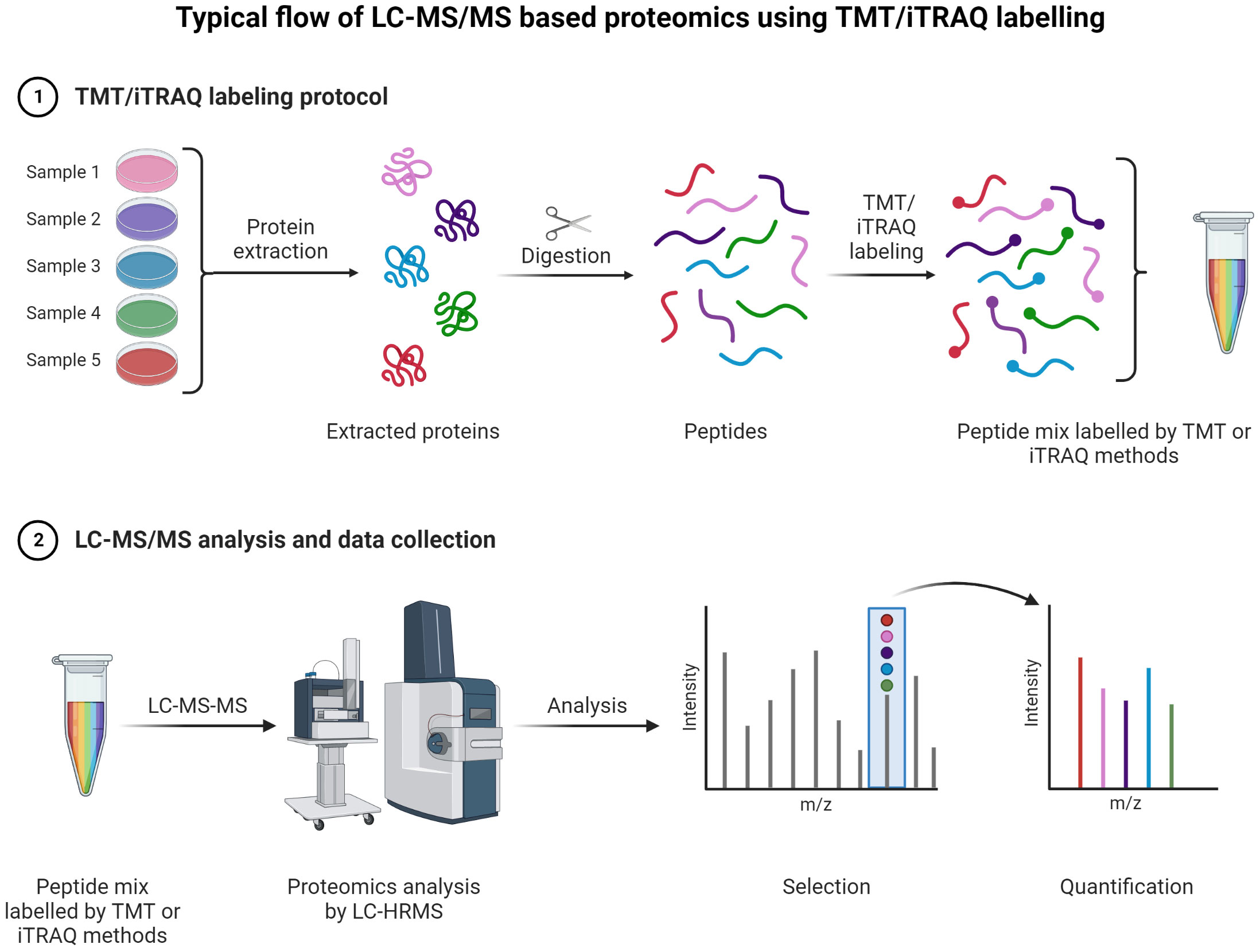
Figure 9 TMT or iTRAQ labeling is used to perform multiplexed (up to 18-plex) analysis for simultaneous quantification of proteins and identification (149, 150) (151, 152). Proteins are extracted and digested with respective enzyme(s). The peptides are labeled with TMT or iTRAQ multiplexed (up to 18-plex) reagents and mixed pool of TMT or iTRAQ labeled peptides are analyzed LC-MS (153–155).
Label-free quantification has gained popularity in large-scale proteomics and biomarker discovery, as there is no need for isotopic labeling and thus low costs are associated with such experiments (156). The key advantage of the label-free method is a wide-range proteome span for identification and quantification of proteins in high and low abundance (157, 158).
TMT and iTRAQ are labeling techniques that allow MS/MS quantification along with enhanced sensitivity for MS. They are very useful for relative and absolute quantification (159). The key advantage of TMT is allowing for multiple tagging and thus reducing overall LC-MS/MS analysis time as multiplex mixed samples can be analyzed simultaneously (160). Peptides are labeled with multiplex reagents (up to 16-plex) that all yield the same molecular ion m/z value for a particular peptide. Upon MS/MS dissociation, fragment ion masses are unique with respect to the original label. Relative abundances can be determined with ease using this method for particular peptides from proteins with different levels of expression. With iTRAQ, the principle is the same with the possibility of analyzing 4-plex or 8-plex samples.
There are many cancer-related questions that cannot be answered using genomics data alone. Hence, The Cancer Genome Atlas (TCGA) aims to get full insight into cancer at the protein level (161). The capabilities of MS technology are continuously evolving, with improved protein identification at lower detection limits for complex matrix systems such as tissues, cells, and various forms of biological fluids (serum, plasma, urine, etc.). MS is also capable of determining post-translational modification with great accuracy and of quantifying proteins in a robust and reliable manner. Proteomics is a tool of choice to establish links between genotype and protein function. The Clinical Proteomic Technology Assessment for Cancer (CPTAC) aims to understand the molecular aspects of cancer by studying proteins resulting from alterations found in genomics data of various cancers (162). PTAC utilizes two key methods: “Targeting Genome to Proteome” (Targeting G2P) and “Mapping Proteome to Genome” (Mapping P2G) to advance further understanding of various types of cancer. The site of cBioPortal provides open-source multidimensional genomics data for various cancers (163). In order to create high-quality cancer proteomics datasets, a global collaboration is needed among clinical oncologists and scientists of all relevant fields. CPTAC has extensive data sets related to breast, colon, and ovarian cancers from TCGA. A recently published article summarizes an integrative CPTAC study on GBM using proteogenomics and metabolomics data generated using 10 multidimensional types of analyses: whole-genome sequencing (WGS), whole-exome sequencing (WES), RNA sequencing (RNA-seq), microRNA-seq (miRNA-seq), single-nucleus RNA sequencing (snRNA-seq), DNA methylation arrays, proteomics, phosphoproteomics, acetylomics, lipidomics, and metabolomics (164). This informative study suggests to conduct further research to reveal the complexity of GBM in order to provide stratification of various tumor types for efficient clinical management. These complexities of GBM are attributed to the heterogenous nature of CNS tumors.
Despite rigorous research on molecular characterization of GBM, the key challenge in understanding the development of drug resistance remains unchanged, whether it concerns intrinsic or acquired drug resistance. During treatment, multidrug resistance (MDR), defined as resistance from neoplastic cells against a wide variety of chemotherapeutic agents, remains an unresolved problem in GBM (13). Some accepted MDR events include for instance increased ability to repair DNA, genetic factors, enhanced efflux of drugs, growth factors, and metabolism of xenobiotics (165). The complexity of MDR extends well beyond these mechanisms, and understanding the exact processes taking place is necessary for the development of strategies for personalized treatments.
Over the last two decades, genomics has contributed significantly to reveal genetic alterations and signaling pathways associated with various cancers (161, 166). Proteomics, or the study of proteins from specific biological systems, has the potential to provide insight into the expression levels of proteins, their regulatory functions, de-/activation, PPI, cellular signaling, and PTMs.
Cellular heterogeneity in tumors is a main feature in GBM. Several studies have been conducted to understand the driving force behind such complex tumor microenvironments, and LC-MS-based proteomics has been the most effective approach. Proteomic approaches other than LC-MS have been utilized to investigate the biology of GBM cell lines, tumors, plasma, and serum samples. These methods involve 2D gel electrophoresis, matrix-assisted laser desorption/ionization mass spectrometry (MALDI-MS), and electrospray ionization-MS, among other techniques. Table 1 gives examples of recent literature exploring analysis of human GBM specimens of the types mentioned above.
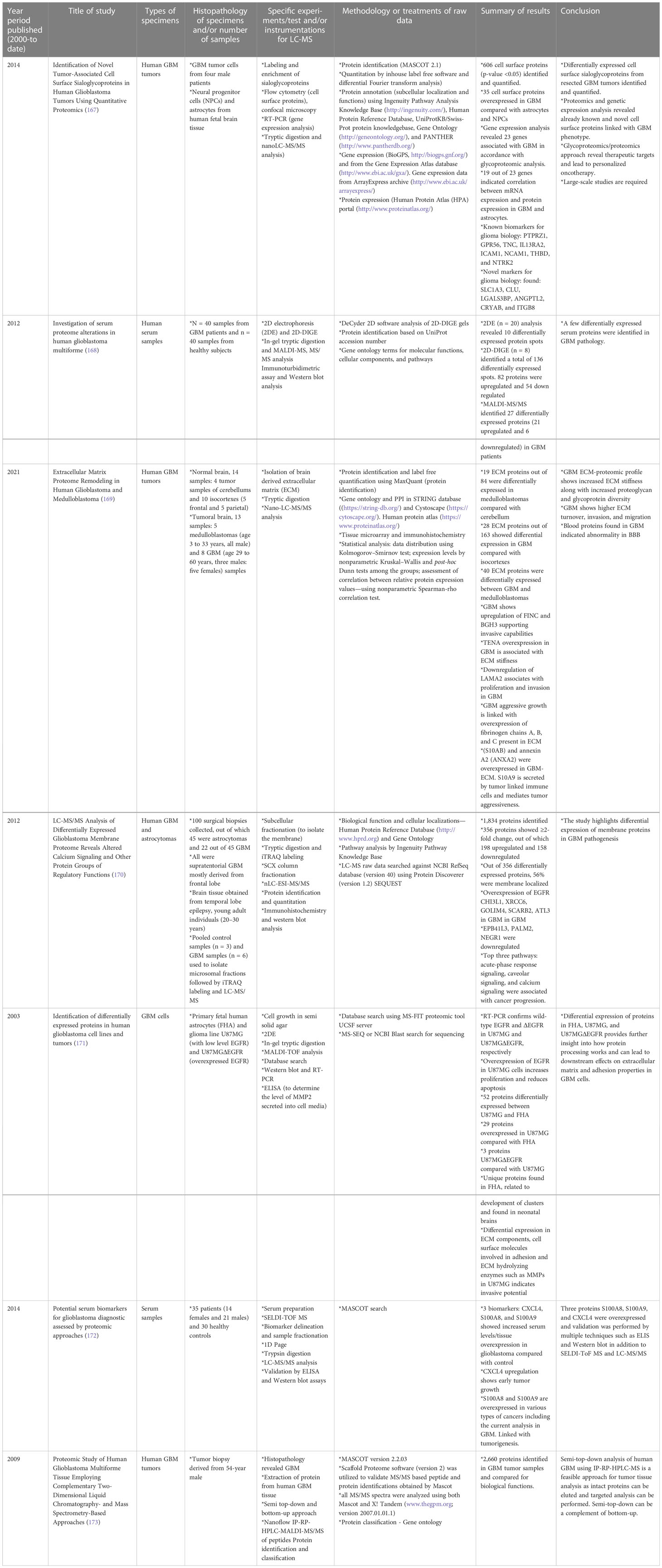
Table 1 Selective list of proteomics studies conducted (from year 2000 to date) on human GBM specimens to understand pathophysiology and cellular biology. .
Moving on to methods specifically using LC-MS-based proteomics, Table 2 summarizes selected studies that were primarily focused on finding or understanding the root causes of the TMZ resistance development. This table was compiled from the Google Scholar Search engine using the following terms: “Proteomics of temozolomide resistance glioma,” “Proteomics of TMZ resistant glioma,” and “Proteomics of temozolomide resistance in GBM.” Most experiments in these studies are focused on comparing tumors from specific cell lines or from patients. Many different research groups have utilized subcellular proteomics as an avenue to find the functional role of proteins from specific subcellular components such as EVs, nucleus, plasma membrane, cytoplasm apart from just GCSs, whole-cell lysate, and tumors. Table 1 also provides a list of experiments undertaken to test the activity and viability or various cell types. The “results” column summarizes the outcomes of selected experiments in terms of protein action to undermine the TMZ resistance phenotype in different types of samples types including cells, tissues, and tumors. Each experiment outlined in this table is unique in terms of supporting a hypothesis with different pieces of evidence. The table also highlights that instead of only comparing tumors of different subjects or cell lines, studying the intratumoral proteome of GMB is a necessary and complementary approach for understanding the protein diversity at different tumor stages with specific time span (184).
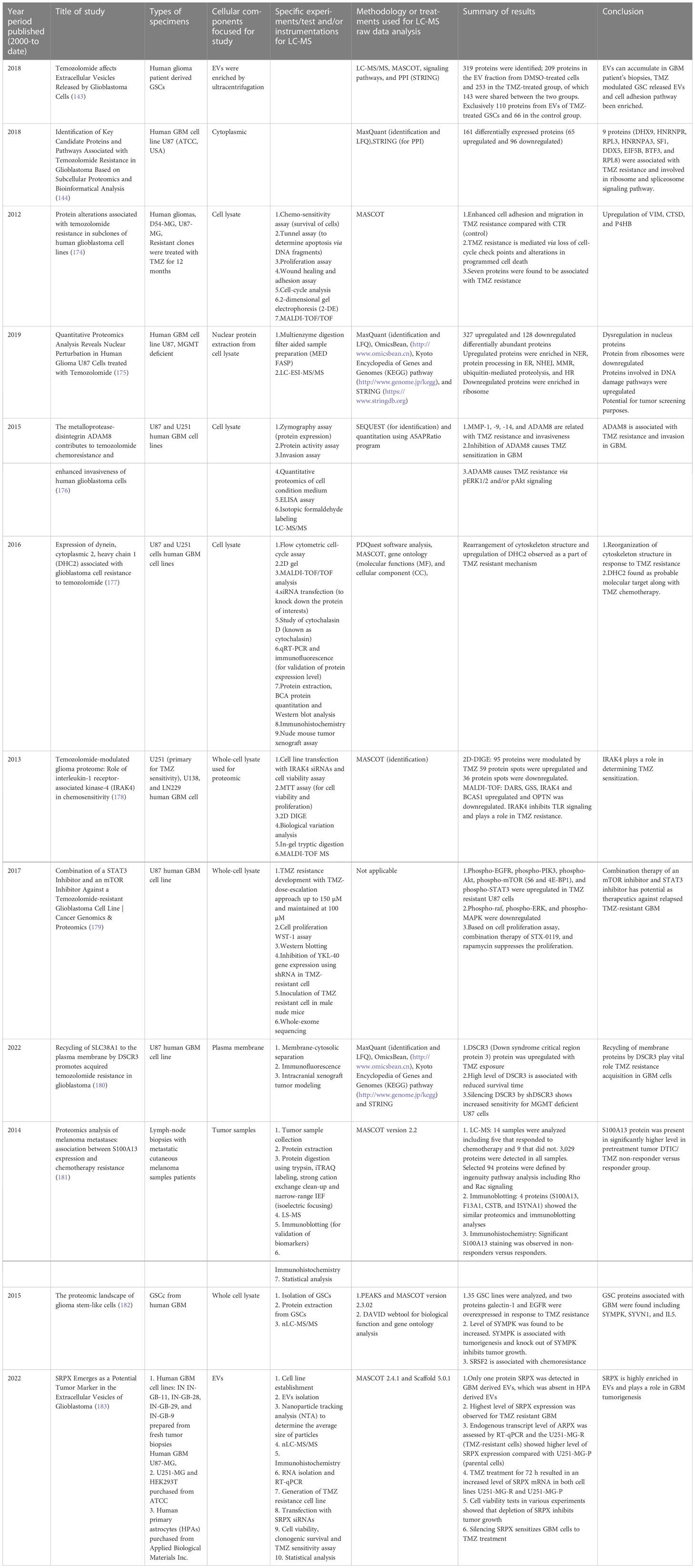
Table 2 List of selected studies conducted (from year 2000 to date) to understand the TMZ resistance in human GBM and other types of cancers (TMZ treatment) using LC-MS-based proteomics approach.
Even with significant advances in cancer diagnosis and treatment options, oncologists still fail to provide maximum clinical benefits to patients due to the development of resistance against various therapies. Tumor cells are capable of choosing alternate mechanisms to sustain resistance against therapies. Consequently, generalized and standard clinical approaches are not useful for better prognosis and enhanced survival. No single chemotherapeutic or concomitant medication can work for all types of GBM tumors. To treat GBM in a timely and effective manner, it becomes essential to inhibit and reverse the resistance mechanism.
Considering this, more vast and in-depth proteomics studies would be beneficial to identify all possible pathways triggered by TMZ treatment to kick off resistance. Initiating a global consortium to work jointly on this topic will be a necessary step to create an expansive proteomics database primarily focused on therapy-resistant cells/tumors. The key advantage provided by proteomics data is to give insight into the molecular features and signature biomarker proteins or metabolites active in relevant pathways. This knowledge is essential for the design of future therapies or to restore the efficacy of currently proven drugs such as TMZ in GBM.
7 Concept of Global Proteomics Consortium on Cancer Therapy Resistance
It is indispensable to identify as much as possible the mechanisms that activate resistance against cytotoxic drugs. The functional roles of protein molecules are key in understanding these mechanisms. The Clinical Proteomic Technology Assessment for Cancer (CPTAC) organization has been performing a wide array of proteomics studies, also focused on transcriptomics and genomics, for specific tumor samples (185). However, more global collaborations are needed to support this noble action of creating a global library. Proteomics of therapeutic resistance in cancer has the potential to identify relevant drug-resistant biomarkers with the highest specificity.
In the last section of this review article, it is proposed to create a Global Proteomics Consortium on Cancer Therapy Resistance (GPCCTR). GPCCTR would involve collaborative research to link specific drug-resistant cancer proteomics LC-MS data with multi-dimensional studies on genomics, proteomics, and histology, in correlation with clinical conditions of patients with a timeline.
Some important factors to consider and match between studies would be the cancer phenotype and variations in the administered treatment. Other necessary information would include the time of tumor diagnosis, the initial treatment regime, and the length of various treatments.
The foundational work for establishing this consortium could be initiated with a focus on one cancer type such as GBM, and gradual expansion could take place. The main role of GPCCTR would be to consider proteomics data sets for therapy-resistant (TR) tumors with the following main objectives: 1) collection and classification of the TR-GBM tumors based on clinical information; 2) genomic profiling of TR-GBM tumors; and 3) proteomic profiling of TR-GBM tumors that were resistant against radiation, specific chemotherapies, and other molecular therapies. The proposed workflow for GPCCTR is shown in Figure 10.
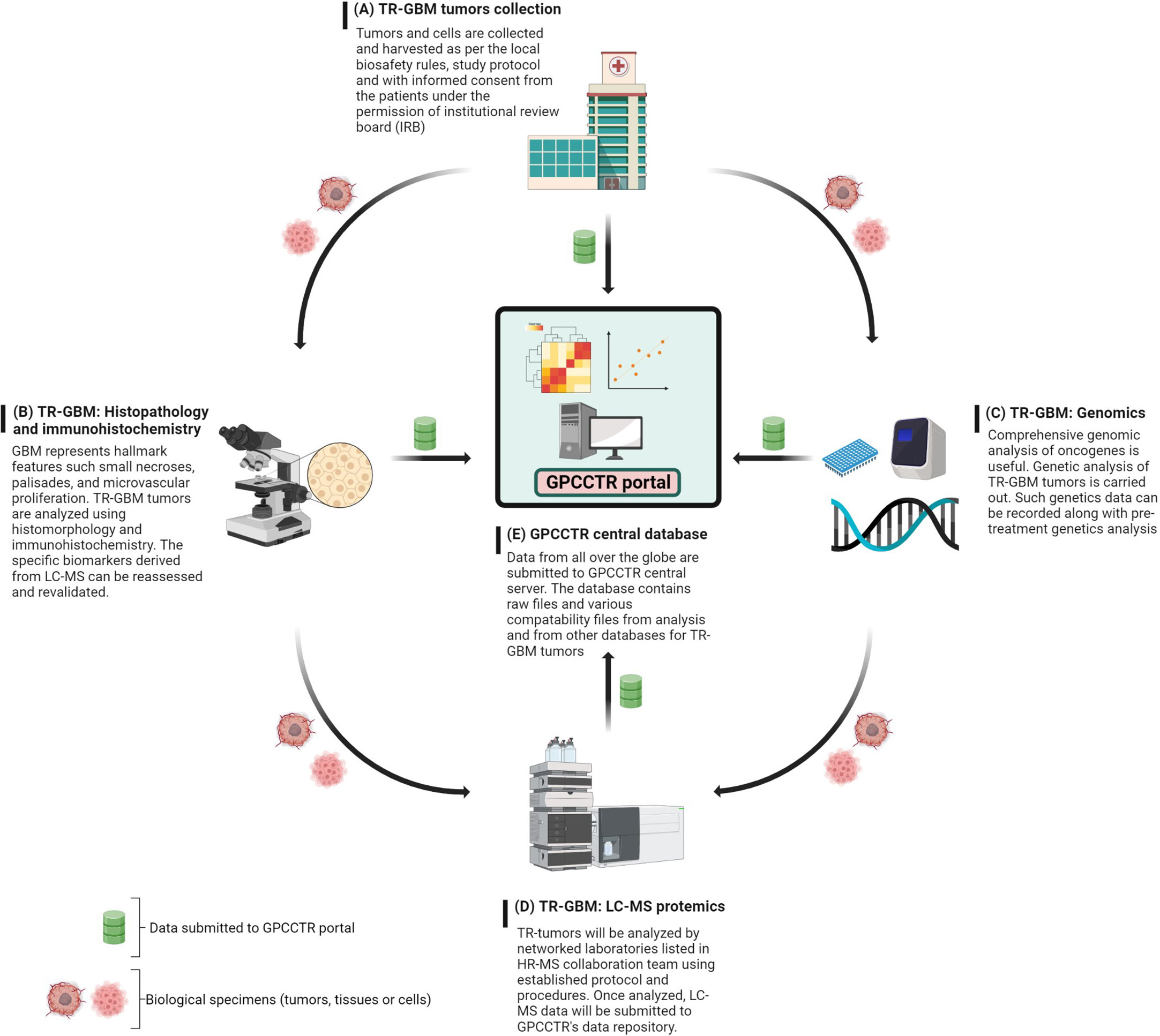
Figure 10 GPCCTR involves five important steps: (A) TR-GBM tumor collection: TR tumors from patients during and after treatment will be collected, labeled, and stored as per the scientific protocol. (B) TR-GBM histology: TR tumors will be tested for resistant specific markers. (C) Genomic analysis of TR-GBM tumors: oncogenic alterations related to TMZ resistance will be analyzed. (D) LC-MS proteomic analysis of TR-GBM tumors: TR tumors will be sent to an LC-MS proteomics laboratory where the analysis is performed with established standard methodology. (E) GPCCTR central database: all of data from (A–D) will be submitted onto the portal. Data analysis and correlation will be performed on a cloud platform. All info and data would be open source and downloadable by registered users.
The text below explains steps A through E of the GPCCTR workflow.
A. For the collection of TR-GBM tumors, as per the 2016 WHO classification, these should be classified and named as relevant clinically. It is also critical to mention the duration of treatment patients have undergone. Accurate records of clinical history are beneficial in assessing correlations with proteomics analyses later, where statistics must validate the correlations.
B. Histopathology and immunohistochemistry of TR-GBM tumors: These tumors feature heterogenous histopathology, as well as aggressive proliferation and invasion. Histomorphological patterns are useful in determining the types of secondary structures of GBM cells. Correlations between histopathology and molecular mechanisms can be important indicators on how therapeutic resistance could be overcome (186). The discovery of novel immunohistochemistry-based biomarkers for TR-GBM will then be useful for prediction of the prognosis and optimizing the treatments (187). Data along with the summary of results and a report should be stored in the GPCCTR portal.
C. For the genomic analysis of TR-GBM tumors, information on genomic alteration determines the severity of subgroups of tumors and oncogenic drivers in the development of resistance. Resistance-specific genomic alterations are key to determining the downstream effects on cellular physiology and on the proteome. Genomic analysis after the course of treatment docks additional values for revalidation of LC-MS data and determination of significant changes in genomic-based biomarkers (188). Advances in molecular genetics have equipped clinicians with accurate and detailed understanding of genetic and epigenetic alterations (189).
D. For LC-MS proteomic analysis of TR-GBM, tumors categorized as per sections A and B should be analyzed by LC-MS according to predefined protocols with standard operating procedures (SOPs) in terms of sample preparation and labeling of peptides or amino acids. For labeling, isobaric tags can be used for relative and absolute quantitation: isobaric tags for relative and absolute quantitation (iTRAQ), stable isotope labeling by amino acids in cell culture (SILAC), and TMT (149, 150).
E. The central repository of GPCCTR is used to upload all data collected by the consortium scientists. Data should contain details on clinical history including the treatments or therapies undertaken by the patients, reports on genetic profiling of tumors, and raw/processed data from LC-MS proteomic analyses. The quality of data is critical, and hence, all collaborators are required to follow SOPs to determine the criteria for data to be uploaded into the portal.
8 Conclusion
Temozolomide has remained a first-line chemotherapy treatment for GBM for almost two decades. However, intrinsic or acquired resistance development against TMZ diminishes the therapeutic benefits of treatment, resulting in poor prognosis. Various combinations of treatments are utilized with continuous development of new therapies, but the overall prognosis remains poor. Extensive research studies have been conducted to try and elucidate the mechanism(s) of TMZ resistance development and for the development of alternative therapies. Studies show that TMZ resistance development can be driven by multiple mechanisms involving various genes, proteins, and enzymes.
The use of advanced LC-MS-based proteomics to help understand TMZ resistance holds significant promises, as these methods can delve into the molecular understanding of resistance mechanisms, which is necessary to developing personalized treatment and new therapeutics. However, the invasive and heterogenous nature of GBM tumors adds other challenges for neurooncologists in the development of universal treatment plans. A global effort is needed to conduct a multidimensional proteomic study by laboratories around the world, in order to acquire a profound understanding of TMZ resistance. The proposed GPCCTR global database would include all clinical variables. Vast proteomics datasets can provide a deep understanding of specific proteins and pathways involved in TMZ resistance. Studying an extensive number of TMZ-resistant tumors using LC-MS-based proteomics can reveal the potential mechanisms or pathways by which the resistance phenotype is developed. The GPCCTR database is a unique concept to link global experts in proteomics and oncology and put together their contributions into an expansive data library. This would be highly beneficial for the identification of new targets and for the design of new drugs, including personalized medicine.
Author contributions
MT: concept, extensive search for literatures, creation of figures (from Figures 1–10) and drafted this manuscript; VC: critical review of the manuscript, inputs and editing; HP: critical review of the manuscript, inputs and editing as subject expert including correctness and grammar and final approval. All authors contributed to the article and approved the submitted version.
Funding
NSERC (Grant # RGPIN 05502-17) has provided funding to HP and stipend to MT to conduct the research on the relevant project for his PhD thesis.
Acknowledgments
Jason Nguyen performed some initial search on topic of ‘Proteomics and TMZ resistance in glioma or GBM)’.
Conflict of interest
The authors declare that the research was conducted in the absence of any commercial or financial relationships that could be construed as a potential conflict of interest.
Publisher’s note
All claims expressed in this article are solely those of the authors and do not necessarily represent those of their affiliated organizations, or those of the publisher, the editors and the reviewers. Any product that may be evaluated in this article, or claim that may be made by its manufacturer, is not guaranteed or endorsed by the publisher.
References
1. Stupp R, Mason WP, van den Bent MJ, Weller M, Fisher B, Taphoorn, et al. Radiotherapy plus concomitant and adjuvant temozolomide for glioblastoma. N Engl J Med (2005) 352:987–96. doi: 10.1056/NEJMoa043330
2. Jiapaer S, Furuta T, Tanaka S, Kitabayashi T, Nakada M. Potential strategies overcoming the temozolomide resistance for glioblastoma. Neurol Med Chir (Tokyo) (2018) 58:405–21. doi: 10.2176/nmc.ra.2018-0141
3. Alexander BM, Cloughesy TF. Adult glioblastoma. J Clin Oncol (2017) 35:2402–9. doi: 10.1200/JCO.2017.73.0119
4. Ostrom QT, Patil N, Cioffi G, Waite K, Kruchko C, Barnholtz-Sloan JS. CBTRUS statistical report: Primary brain and other central nervous system tumors diagnosed in the united states in 2013–2017. Neuro-Oncol (2020) 22:iv1–iv96. doi: 10.1093/neuonc/noaa200
5. Weller M, Wick W, Aldape K, Brada M, Berger M, Pfister SM, et al. Glioma. Nat Rev Dis Primer (2015) 1. doi: 10.1038/nrdp.2015.17
6. Pandey PK, Sharma AK, Gupta U. Blood brain barrier: An overview on strategies in drug delivery, realistic in vitro modeling and in vivo live tracking. Tissue Barriers (2016) 4:e1129476.
7. Haumann R, Videira JC, Kaspers GJL, van Vuurden DG, Hulleman E. Overview of current drug delivery methods across the blood–brain barrier for the treatment of primary brain tumors. CNS Drugs (2020) 34:1121–31. doi: 10.1007/s40263-020-00766-w
8. Vengoji R, Macha MA, Batra SK, Shonka NA. Natural products: a hope for glioblastoma patients. Oncotarget (2018) 9:22194–219. doi: 10.18632/oncotarget.25175
9. Luqmani YA. Mechanisms of drug resistance in cancer chemotherapy. Med Princ Pract (2005) 14(suppl 1):35–48. doi: 10.1159/000086183
10. Wijdeven RH, Pang B, Assaraf YG, Neefjes J. Old drugs, novel ways out: Drug resistance toward cytotoxic chemotherapeutics. Drug Resist Updat (2016) 28:65–81. doi: 10.1016/j.drup.2016.07.001
11. Zhang J, Stevens MFG, Bradshaw TD. Temozolomide: Mechanisms of action, repair and resistance. Curr Mol Pharmacol (2012) 5(1):102–14.
13. Cao Y, Li Z, Mao L, Cao H, Kong J, Yu B, et al. The use of proteomic technologies to study molecular mechanisms of multidrug resistance in cancer. Eur J Med Chem (2019) 162:423–34. doi: 10.1016/j.ejmech.2018.10.001
14. Shen B, Dong X, Yuan B, Zhang Z. Molecular markers of MDR of chemotherapy for HSCC: Proteomic screening with high-throughput liquid chromatography-tandem mass spectrometry. Front Oncol (2021) 11. doi: 10.3389/fonc.2021.687320
15. Sahni S, Nahm C, Krisp C, Molloy MP, Mehta S, Maloney S, et al. Identification of novel biomarkers in pancreatic tumor tissue to predict response to neoadjuvant chemotherapy. Front Oncol (2020) 10. doi: 10.3389/fonc.2020.00237
16. Proteomic patterns associated with response to breast cancer neoadjuvant treatment. Mol Syst Biol (2020) 16:e9443.
17. IARC. Home. Available at: https://www.iarc.who.int/.
18. Zülch KJ. Histological typing of tumours of the central nervous system. Geneva: World Health Organization (1979).
19. Kleihues P, Burger PC, Scheithauer BW. Histological typing of tumours of the central nervous system. In: World health organization international histological classiWcation of tumours. Heidelberg: Springer (1993).
20. Descriptive epidemiology of CNS tumors in France: results from the gironde registry for the period 2000–2007. Neuro-Oncology (2011) 13(12):1370–8.
21. Zada G, Bond AE, Wang Y-P, Giannotta SL, Deapen D. Incidence trends in the anatomic location of primary malignant brain tumors in the united states: 1992–2006. World Neurosurg (2012) 77:518–24. doi: 10.1016/j.wneu.2011.05.051
22. Yuan X, Belugali Nataraj N, Perry G, Loayza Puch F, Nagel R, Marin I, et al. Isolation of cancer stem cells from adult glioblastoma multiforme. Oncogene (2004) 23:9392–400. doi: 10.1038/sj.onc.1208311
23. Oliveira AI, Anjo SI, Vieira de Castro J, Serra SC, Salgado AJ, Manadas B, et al. Crosstalk between glial and glioblastoma cells triggers the “go-or-grow” phenotype of tumor cells. Cell Commun Signal (2017) 15:37. doi: 10.1186/s12964-017-0194-x
24. Charles NA, Holland EC, Gilbertson R, Glass R, Kettenmann H. The brain tumor microenvironment. Glia (2012) 60:502–14. doi: 10.1002/glia.21264
25. Verkhratsky A, Ho MS, Zorec R, Parpura V. The concept of neuroglia. In: Verkhratsky A, Ho MS, Zorec R, Parpura V, editors. Neuroglia in neurodegenerative diseases. Singapore: Springer (2019). p. 1–13. doi: 10.1007/978-981-13-9913-8_1
26. Ostrom QT, Cioffi G, Waite K, Kruchko C, Barnholtz-Sloan JS. CBTRUS statistical report: Primary brain and other central nervous system tumors diagnosed in the united states in 2014–2018. Neuro-Oncol (2021) 23:iii1–iii105. doi: 10.1093/neuonc/noab200
27. Kleihues P, Cavenee WK. World health organization ClassiWcation of tumours. pathology and genetics of tumours of the nervous system. Lyon: IARC Press (2000).
28. Louis DN, Perry A, Reifenberger G, von Deimling A, Figarella-Branger D, Cavenee WK, et al. The 2016 world health organization classification of tumors of the central nervous system: a summary. Acta Neuropathol (Berl.) (2016) 131:803–20.
29. Louis DN, Ohgaki H, Wiestler OD, Caveene WK. World health organization histological classification of tumours of the central nervous system. France: International Agency for Research on Cancer (2016).
30. Stevens MFG, Hickman JA, Stone R, Gibson NW, Baig GU, Lunt E, et al. Antitumour imidazotetrazines. 1. synthesis and chemistry of 8-carbamoyl-3-(2-chloroethyl)imidazo[5,1-d]-1,2,3,5-tetrazin-4(3H)-one, a novel broad-spectrum antitumor agent. J Med Chem (1984) 27:196–201. doi: 10.1021/jm00368a016
31. Koumarianou A, Kaltsas G, Kulke MH, Oberg K, Strosberg JR, Spada F, et al. Temozolomide in advanced neuroendocrine neoplasms: Pharmacological and clinical aspects. Neuroendocrinology (2015) 101:274–88. doi: 10.1159/000430816
32. Stevens MFG, Hickman JA, Langdon SP, Chubb D, Vickers L, Stone R, et al. Antitumor activity and pharmacokinetics in mice of 8-Carbamoyl-3-methyl-imidazo[5,1-d]-1,2,3,5-tetrazin-4(3H)-one (CCRG 81045; m & b 39831), a novel drug with potential as an alternative to dacarbazine. Cancer Res (1987) 47:5846.
33. Newlands E, Blackledge G, Slack J, Rustin G, Smith D, Stuart N, et al. Phase I trial of temozolomide (CCRG 81045: M&B 39831: NSC 362856). Br J Cancer (1992) 65:287–91. doi: 10.1038/bjc.1992.57
34. Tentori L, Vernole P, Lacal PM, Madaio R, Portarena I, Levati L, et al. Cytotoxic and clastogenic effects of a DNA minor groove binding methyl sulfonate ester in mismatch repair deficient leukemic cells. Leukemia (2000) 14:1451–9. doi: 10.1038/sj.leu.2401842
35. Newlands ES, Stevens MFG, Wedge SR, Wheelhouse RT, Brock C. Temozolomide: a review of its discovery, chemical properties, pre-clinical development and clinical trials. Cancer Treat Rev (1997) 23:35–61. doi: 10.1016/S0305-7372(97)90019-0
36. Lawley PD, Phillips DH. DNA Adducts from chemotherapeutic agents. Mutat Res (1996) 355:13–40. doi: 10.1016/0027-5107(96)00020-6
37. Friedman HS, Kerby T, Calvert H. Temozolomide and treatment of malignant glioma. Clin Cancer Res (2000) 6(7):2585–97.
38. Bianco R, Troiani T, Tortora G, Ciardiello F. Intrinsic and acquired resistance to EGFR inhibitors in human cancer therapy. Endocr.-Relat Cancer Endocr Relat Cancer (2005) 12:S159–71. doi: 10.1677/erc.1.00999
39. Li X, Lewis MT, Huang J, Gutierrez C, Osborne CK, Wu M-F, et al. Intrinsic resistance of tumorigenic breast cancer cells to chemotherapy. JNCI J Natl Cancer Inst (2008) 100:672–9. doi: 10.1093/jnci/djn123
40. Lippert TH, Ruoff H-J, Volm M. Intrinsic and acquired drug resistance in malignant tumors. Arzneimittelforschung (2008) 58:261–4.
41. Beier D, Schulz JB, Beier CP. Chemoresistance of glioblastoma cancer stem cells - much more complex than expected. Mol Cancer (2011) 10:128. doi: 10.1186/1476-4598-10-128
42. Lee SY. Temozolomide resistance in glioblastoma multiforme. Genes Dis (2016) 3:198–210. doi: 10.1016/j.gendis.2016.04.007
43. Chen X, Zhang M, Gan H, Wang H, Lee J-H, Fang D, et al. A novel enhancer regulates MGMT expression and promotes temozolomide resistance in glioblastoma. Nat Commun (2018) 9:2949. doi: 10.1038/s41467-018-05373-4
44. Kitange GJ, Carlson BL, Schroeder MA, Grogan PT, Lamont JD, Decker PA, et al. Induction of MGMT expression is associated with temozolomide resistance in glioblastoma xenografts. Neuro-Oncol. (2009) 11:281–91. doi: 10.1215/15228517-2008-090
45. Chumakova A, Lathia JD. Outlining involvement of stem cell program in regulation of O6-methylguanine DNA methyltransferase and development of temozolomide resistance in glioblastoma: An editorial highlight for ‘Transcriptional control of O 6 -methylguanine DNA methyltransferase. J Neurochem (2018) 144:688–90. doi: 10.1111/jnc.14280
46. Hegi ME, Diserens A-C, Gorlia T, Hamou M-F, de Tribolet N, Weller M, et al. MGMT gene silencing and benefit from temozolomide in glioblastoma. N Engl J Med (2005) 352:997–1003. doi: 10.1056/NEJMoa043331
47. Perazzoli G, Prados J, Ortiz R, Caba O, Cabeza L, Berdasco M, et al. Temozolomide resistance in glioblastoma cell lines: Implication of MGMT, MMR, p-glycoprotein and CD133 expression. PloS One (2015) 10:e0140131. doi: 10.1371/journal.pone.0140131
48. Lang F, Liu Y, Chou F-J, Yang C. Genotoxic therapy and resistance mechanism in gliomas. Pharmacol Ther (2021) 228:107922. doi: 10.1016/j.pharmthera.2021.107922
49. Hegi ME, Liu L, Herman JG, Stupp R, Wick W, Weller, et al. Correlation of O6-methylguanine methyltransferase (MGMT) promoter methylation with clinical outcomes in glioblastoma and clinical strategies to modulate MGMT activity. J Clin Oncol (2008) 26:4189–99. doi: 10.1200/JCO.2007.11.5964
50. Kohsaka S, Wang L, Yachi K, Mahabir R, Narita T, Itoh T, et al. STAT3 inhibition overcomes temozolomide resistance in glioblastoma by downregulating MGMT expression. Mol Cancer Ther (2012) 11:1289–99. doi: 10.1158/1535-7163.MCT-11-0801
51. Stritzelberger J, Distel L, Buslei R, Fietkau R, Putz F. Acquired temozolomide resistance in human glioblastoma cell line U251 is caused by mismatch repair deficiency and can be overcome by lomustine. Clin Transl Oncol (2018) 20:508–16. doi: 10.1007/s12094-017-1743-x
52. Singh N, Miner A, Hennis L, Mittal S. Mechanisms of temozolomide resistance in glioblastoma - a comprehensive review. Cancer Drug Resist (2021) 4:17–43.
53. Nie E, Miao F, Jin X, Wu W, Zhou X, Zeng A, et al. Fstl1/DIP2A/MGMT signaling pathway plays important roles in temozolomide resistance in glioblastoma. Oncogene (2019) 38:2706–21. doi: 10.1038/s41388-018-0596-2
54. Bobola MS, Kolstoe DD, Blank A, Chamberlain MC, Silber JR. Repair of 3-methyladenine and abasic sites by base excision repair mediates glioblastoma resistance to temozolomide. Front Oncol (2012) 2:176. doi: 10.3389/fonc.2012.00176
55. Gil Del Alcazar CR, Todorova PK, Habib AA, Mukherjee B, Burma S. Augmented HR repair mediates acquired temozolomide resistance in glioblastoma. Mol Cancer Res MCR (2016) 14:928–40. doi: 10.1158/1541-7786.MCR-16-0125
56. Ni X-R, Guo C-C, Yu Y-J, Yu Z-H, Cai H-P, Wu W-C, et al. Combination of levetiracetam and IFN-α increased temozolomide efficacy in MGMT-positive glioma. Cancer Chemother Pharmacol (2020) 86:773–82. doi: 10.1007/s00280-020-04169-y
57. Cahill DP, Levine KK, Betensky RA, Codd PJ, Romany CA, Reavie LB, et al. Loss of the mismatch repair protein MSH6 in human glioblastomas is associated with tumor progression during temozolomide treatment. Clin Cancer Res (2007) 13:2038. doi: 10.1158/1078-0432.CCR-06-2149
58. McFaline-Figueroa JL, Braun CJ, Stanciu M, Nagel ZD, Mazzucato P, Sangaraju D, et al. Minor changes in expression of the mismatch repair protein MSH2 exert a major impact on glioblastoma response to temozolomide. Cancer Res (2015) 75:3127–38. doi: 10.1158/0008-5472.CAN-14-3616
59. Yoshimoto K, Mizoguchi M, Hata N, Murata H, Hatae R, Amano T, et al. Complex DNA repair pathways as possible therapeutic targets to overcome temozolomide resistance in glioblastoma. Front Oncol (2012) 2:186. doi: 10.3389/fonc.2012.00186
60. Trivedi RN, Almeida KH, Fornsaglio JL, Schamus S, Sobol RW. The role of base excision repair in the sensitivity and resistance to temozolomide-mediated cell death. Cancer Res (2005) 65:6394–400. doi: 10.1158/0008-5472.CAN-05-0715
61. Higuchi F, Nagashima H, Ning J, Koerner MVA, Wakimoto H, Cahill DP. Restoration of temozolomide sensitivity by PARP inhibitors in mismatch repair deficient glioblastoma is independent of base excision repair. Clin Cancer Res (2020) 26:1690–9. doi: 10.1158/1078-0432.CCR-19-2000
62. Liu X, Shi Y, Guan R, Donawho C, Luo Y, Palma J. Potentiation of temozolomide cytotoxicity by Poly(ADP)Ribose polymerase inhibitor ABT-888 requires a conversion of single-stranded DNA damages to double-stranded DNA breaks. Mol Cancer Res (2008) 6:1621–9. doi: 10.1158/1541-7786.MCR-08-0240
63. Gupta SK, Smith EJ, Mladek AC, Tian S, Decker PA, Kizilbash SH, et al. PARP inhibitors for sensitization of alkylation chemotherapy in glioblastoma: Impact of blood-brain barrier and molecular heterogeneity. Front Oncol (2019) 8:670. doi: 10.3389/fonc.2018.00670
64. Surawicz TS, Davis F, Freels S, Laws ER, Menck HR. Brain tumor survival: results from the national cancer data base. J Neurooncol (1998) 40:151–60. doi: 10.1023/A:1006091608586
65. Ignatova TN, Kukekov VG, Laywell ED, Suslov ON, Vrionis FD, Steindler DA. Human cortical glial tumors contain neural stem-like cells expressing astroglial and neuronal markers in vitro. Glia (2002) 39:193–206. doi: 10.1002/glia.10094
66. Sharifi Z, Abdulkarim B, Meehan B, Rak J, Daniel P, Schmitt J, et al. Mechanisms and antitumor activity of a binary EGFR/DNA–targeting strategy overcomes resistance of glioblastoma stem cells to temozolomide. Clin Cancer Res (2019) 25:7594. doi: 10.1158/1078-0432.CCR-19-0955
67. Garnier D, Meehan B, Kislinger T, Daniel P, Sinha A, Abdulkarim B, et al. Divergent evolution of temozolomide resistance in glioblastoma stem cells is reflected in extracellular vesicles and coupled with radiosensitization. Neuro-Oncol. (2018) 20:236–48. doi: 10.1093/neuonc/nox142
68. Son MJ, Woolard K, Nam D-H, Lee J, Fine HA. SSEA-1 is an enrichment marker for tumor-initiating cells in human glioblastoma. Cell Stem Cell (2009) 4:440–52. doi: 10.1016/j.stem.2009.03.003
69. Bradshaw A, Wickremsekera A, Tan ST, Peng L, Davis PF, Itinteang T. Cancer stem cell hierarchy in glioblastoma multiforme. Front Surg (2016) 3:21. doi: 10.3389/fsurg.2016.00021
70. Lathia JD, Gallagher J, Heddleston JM, Wang J, Eyler CE, MacSwords J, et al. Integrin alpha 6 regulates glioblastoma stem cells. Cell Stem Cell (2010) 6:421–32. doi: 10.1016/j.stem.2010.02.018
71. Vicentic JM, Drakulic D, Garcia I, Vukovic V, Aldaz P, Puskas N, et al. SOX3 can promote the malignant behavior of glioblastoma cells. Cell Oncol (2019) 42:41–54. doi: 10.1007/s13402-018-0405-5
72. Najafi M, Mortezaee K, Majidpoor J. Cancer stem cell (CSC) resistance drivers. Life Sci (2019) 234:116781. doi: 10.1016/j.lfs.2019.116781
73. Eun K, Ham SW, Kim H. Cancer stem cell heterogeneity: origin and new perspectives on CSC targeting. BMB Rep (2017) 50:117. doi: 10.5483/BMBRep.2017.50.3.222
74. Lathia JD, Mack SC, Mulkearns-Hubert EE, Valentim CL, Rich JN. Cancer stem cells in glioblastoma. Genes Dev (2015) 29:1203–17. doi: 10.1101/gad.261982.115
75. Eramo A, Ricci-Vitiani L, Zeuner A, Pallini R, Lotti F, Sette G, et al. Chemotherapy resistance of glioblastoma stem cells. Cell Death Differ (2006) 13:1238–41. doi: 10.1038/sj.cdd.4401872
76. Garros-Regulez L, Aldaz P, Arrizabalaga O, Moncho-Amor V, Carrasco-Garcia E, Manterola L, et al. mTOR inhibition decreases SOX2-SOX9 mediated glioma stem cell activity and temozolomide resistance. Expert Opin Ther Targets (2016) 20:393–405. doi: 10.1517/14728222.2016.1151002
77. Mizushima N. Autophagy: process and function. Genes Dev (2007) 21:2861–73. doi: 10.1101/gad.1599207
78. Levine B, Kroemer G. Autophagy in the pathogenesis of disease. Cell (2008) 132:27–42. doi: 10.1016/j.cell.2007.12.018
79. Rabinowitz Joshua D, White E. Autophagy and metabolism. Science (2010) 330:1344–8. doi: 10.1126/science.1193497
80. Mathew R, Karantza-Wadsworth V, White E. Role of autophagy in cancer. Nat Rev Cancer (2007) 7:961–7. doi: 10.1038/nrc2254
82. Singh SS, Vats S, Chia AY-Q, Tan TZ, Deng S, Ong MS, et al. Dual role of autophagy in hallmarks of cancer. Oncogene (2018) 37:1142–58. doi: 10.1038/s41388-017-0046-6
83. Shen Y, Li D-D, Wang L-L, Deng R, Zhu X-F. Decreased expression of autophagy-related proteins in malignant epithelial ovarian cancer. Autophagy (2008) 4:1067–8. doi: 10.4161/auto.6827
84. Guo JY, Xia B, White E. Autophagy-mediated tumor promotion. Cell (2013) 155:1216–9. doi: 10.1016/j.cell.2013.11.019
85. Goldberg AD, Allis CD, Bernstein E. Epigenetics: A landscape takes shape. Cell (2007) 128:635–8. doi: 10.1016/j.cell.2007.02.006
86. Chuang JC, Jones PA. Epigenetics and MicroRNAs. Pediatr Res (2007) 61:24–9. doi: 10.1203/pdr.0b013e3180457684
87. Kelly TK, De Carvalho DD, Jones PA. Epigenetic modifications as therapeutic targets. Nat Biotechnol (2010) 28:1069–78. doi: 10.1038/nbt.1678
88. Liu B, Zhou J, Wang C, Chi Y, Wei Q, Fu Z, et al. LncRNA SOX2OT promotes temozolomide resistance by elevating SOX2 expression via ALKBH5-mediated epigenetic regulation in glioblastoma. Cell Death Dis (2020) 11:1–18. doi: 10.1038/s41419-020-2540-y
89. Rezaei O, Tamizkar KH, Sharifi G, Taheri M, Ghafouri-Fard S. Emerging role of long non-coding RNAs in the pathobiology of glioblastoma. Front Oncol (2021) 10. doi: 10.3389/fonc.2020.625884
90. Ghafouri-Fard S, Agabalazadeh A, Abak A, Shoorei H, Hassanzadeh Taheri MM, et al. Role of long non-coding RNAs in conferring resistance in tumors of the nervous system. Front Oncol (2021) 11. doi: 10.3389/fonc.2021.670917
91. Kitange GJ, Mladek AC, Carlson BL, Schroeder MA, Pokorny JL, Cen L, et al. Inhibition of histone deacetylation potentiates the evolution of acquired temozolomide resistance linked to MGMT upregulation in glioblastoma xenografts. Clin Cancer Res (2012) 18:4070–9. doi: 10.1158/1078-0432.CCR-12-0560
92. Rabé M, Dumont S, Álvarez-Arenas A, Janati H, Belmonte-Beitia J, Calvo GF, et al. Identification of a transient state during the acquisition of temozolomide resistance in glioblastoma. Cell Death Dis (2020) 11:1–14.
93. Wu Q, Berglund AE, Etame AB. The impact of epigenetic modifications on adaptive resistance evolution in glioblastoma. Int J Mol Sci (2021) 22(15):8324. doi: 10.3390/ijms22158324
94. Buruiană A, Florian Ștefan I, Florian AI, Timiș T-L, Mihu CM, Miclăuș M, et al. The roles of miRNA in glioblastoma tumor cell communication: Diplomatic and aggressive negotiations. Int J Mol Sci (2020) 21:1950. doi: 10.3390/ijms21061950
95. Mizoguchi M, Guan Y, Yoshimoto K, Hata N, Amano T, Nakamizo A, et al. Clinical implications of microRNAs in human glioblastoma. Front Oncol (2013) 3. doi: 10.3389/fonc.2013.00019
96. Luo J, Wang X, Yang Y, Mao Q. Role of micro-RNA (miRNA) in pathogenesis of glioblastoma. Eur Rev Med Pharmacol Sci (2015) 19:1630–9.
97. Wong STS, Zhang X-Q, Zhuang JT-F, Chan H-L, Li C-H, Leung GKK. MicroRNA-21 inhibition enhances in vitro chemosensitivity of temozolomide-resistant glioblastoma cells. Anticancer Res (2012) 32:2835–41.
98. Namee NM, O’Driscoll L. Extracellular vesicles and anti-cancer drug resistance. Biochim Biophys Acta BBA - Rev Cancer (2018) 1870:123–36. doi: 10.1016/j.bbcan.2018.07.003
99. Yin J, Ge X, Shi Z, Yu C, Lu C, Wei Y, et al. Extracellular vesicles derived from hypoxic glioma stem-like cells confer temozolomide resistance on glioblastoma by delivering miR-30b-3p. Theranostics (2021) 11:1763–79. doi: 10.7150/thno.47057
100. Simon T, Jackson E, Giamas G. Breaking through the glioblastoma micro-environment via extracellular vesicles. Oncogene (2020) 39:4477–90. doi: 10.1038/s41388-020-1308-2
101. Bleeker FE, Atai NA, Lamba S, Jonker A, Rijkeboer D, Bosch KS, et al. The prognostic IDH1R132mutation is associated with reduced NADP+-dependent IDH activity in glioblastoma. Acta Neuropathol (Berl.) (2010) 119:487–94.
102. Yen KE, Bittinger MA, Su SM, Fantin VR. Cancer-associated IDH mutations: biomarker and therapeutic opportunities. Oncogene (2010) 29:6409–17. doi: 10.1038/onc.2010.444
103. Waitkus MS, Diplas BH, Yan H. Isocitrate dehydrogenase mutations in gliomas. Neuro-Oncol. (2015) 18:16–26. doi: 10.1093/neuonc/nov136
104. Parsons DW, Jones S, Zhang X, Lin JC-H, Leary RJ, Angenendt P, et al. An integrated genomic analysis of human glioblastoma multiforme. science (2008) 321:1807–12. doi: 10.1126/science.1164382
105. Yan H, Parsons DW, Jin G, McLendon R, Rasheed BA, Yuan W, et al. IDH1 and IDH2 mutations in gliomas. N Engl J Med (2009) 360:765–73. doi: 10.1056/NEJMoa0808710
106. Han S, Liu Y, Cai SJ, Qian M, Ding J, Larion M, et al. IDH mutation in glioma: molecular mechanisms and potential therapeutic targets. Br J Cancer (2020) 122:1580–9. doi: 10.1038/s41416-020-0814-x
107. White K, Connor K, Clerkin J, Murphy BM, Salvucci M, O’Farrell AC, et al. New hints towards a precision medicine strategy for IDH wild-type glioblastoma. Ann Oncol (2020) 31:1679–92. doi: 10.1016/j.annonc.2020.08.2336
108. Hartmann C, Hentschel B, Wick W, Capper D, Felsberg J, Simon M, et al. Patients with IDH1 wild type anaplastic astrocytomas exhibit worse prognosis than IDH1-mutated glioblastomas, and IDH1 mutation status accounts for the unfavorable prognostic effect of higher age: implications for classification of gliomas. Acta Neuropathol (Berl.) (2010) 120:707–18. doi: 10.1007/s00401-010-0781-z
109. Wick W, Meisner C, Hentschel B, Platten M, Schilling A, Wiestler B, et al. Prognostic or predictive value of MGMT promoter methylation in gliomas depends on IDH1 mutation. Neurology (2013) 81:1515–22. doi: 10.1212/WNL.0b013e3182a95680
110. Nørøxe DS, Poulsen HS, Lassen U. Hallmarks of glioblastoma: a systematic review. ESMO Open (2016) 1:e000144.
111. Dang L, Jin S, Su SM. IDH mutations in glioma and acute myeloid leukemia. Trends Mol Med (2010) 16:387–97. doi: 10.1016/j.molmed.2010.07.002
112. Yang H, Ye D, Guan K-L, Xiong Y. IDH1 and IDH2 mutations in tumorigenesis: mechanistic insights and clinical perspectives. Clin Cancer Res (2012) 18:5562–71. doi: 10.1158/1078-0432.CCR-12-1773
113. Juratli TA, Kirsch M, Robel K, Soucek S, Geiger K, von Kummer R, et al. IDH mutations as an early and consistent marker in low-grade astrocytomas WHO grade II and their consecutive secondary high-grade gliomas. J Neurooncol (2012) 108:403–10. doi: 10.1007/s11060-012-0844-1
114. Juratli TA, Kirsch M, Robel K, Soucek S, Geiger K, von Kummer R, et al. The prognostic value of IDH mutations and MGMT promoter status in secondary high-grade gliomas. J Neurooncol (2012) 110:325–33. doi: 10.1007/s11060-012-0977-2
115. Guo C, Pirozzi CJ, Lopez GY, Yan H. Isocitrate dehydrogenase mutations in gliomas: mechanisms, biomarkers and therapeutic target. Curr Opin Neurol (2011) 24:648–52. doi: 10.1097/WCO.0b013e32834cd415
116. Friedman HS, McLendon RE, Kerby T, Dugan M, Bigner SH, Henry AJ, et al. DNA Mismatch repair and o^ 6-alkylguanine. DNA alkyltransferase analysis and response to temodal in newly diagnosed malignant glioma. J Clin Oncol (1998) 16:3851–7. doi: 10.1200/JCO.1998.16.12.3851
117. Wick W, Hartmann C, Engel C, Stoffels M, Felsberg J, Stockhammer F, et al. NOA-04 randomized phase III trial of sequential radiochemotherapy of anaplastic glioma with procarbazine, lomustine, and vincristine or temozolomide. J Clin Oncol (2009) 27:5874. doi: 10.1200/JCO.2009.23.6497
118. van den Bent MJ, Dubbink HJ, Sanson M, van der Lee-Haarloo CR, Hegi M, Jeuken JW, et al. MGMT promoter methylation is prognostic but not predictive for outcome to adjuvant PCV chemotherapy in anaplastic oligodendroglial tumors: a report from EORTC brain tumor group study 26951. J Clin Oncol (2009) 27:5881. doi: 10.1200/JCO.2009.24.1034
119. Davies H, Bignell GR, Cox C, Stephens P, Edkins S, Clegg S, et al. Mutations of the BRAF gene in human cancer. Nature (2002) 417:949–54. doi: 10.1038/nature00766
120. Schreck KC, Grossman SA, Pratilas CA. BRAF mutations and the utility of RAF and MEK inhibitors in primary brain tumors. Cancers (2019) 11:1262. doi: 10.3390/cancers11091262
121. Dono A, Vu J, Anapolsky M, Hines G, Takayasu T, Yan Y, et al. Additional genetic alterations in BRAF-mutant gliomas correlate with histologic diagnoses. J Neurooncol (2020) 149:463–72. doi: 10.1007/s11060-020-03634-1
122. Mellinghoff IK, Wang MY, Vivanco I, Haas-Kogan DA, Zhu S, Dia EQ, et al. Molecular determinants of the response of glioblastomas to EGFR kinase inhibitors. N Engl J Med (2005) 353:2012–24. doi: 10.1056/NEJMoa051918
123. Huang PH, Xu AM, White FM. Oncogenic EGFR signaling networks in glioma. Sci Signal (2009) 2:re6. doi: 10.1126/scisignal.287re6
124. Sigismund S, Avanzato D, Lanzetti L. Emerging functions of the EGFR in cancer. Mol Oncol (2018) 12:3–20. doi: 10.1002/1878-0261.12155
125. Silver DJ, Lathia JD. Revealing the glioma cancer stem cell interactome, one niche at a time. J Pathol (2018) 244:260–4. doi: 10.1002/path.5024
126. Beier D, Schulz JB, Beier CP. Chemoresistance of glioblastoma cancer stem cells-much more complex than expected. Mol Cancer (2011) 10:1–11. doi: 10.1186/1476-4598-10-128
127. Bao S, Wu Q, McLendon RE, Hao Y, Shi Q, Hjelmeland AB, et al. Glioma stem cells promote radioresistance by preferential activation of the DNA damage response. Nature (2006) 444:756–60. doi: 10.1038/nature05236
128. Tan X, Lambert PF, Rapraeger AC, Anderson RA. Stress-induced EGFR trafficking: Mechanisms, functions, and therapeutic implications. Trends Cell Biol (2016) 26:352–66. doi: 10.1016/j.tcb.2015.12.006
129. Nishikawa R, Ji XD, Harmon RC, Lazar CS, Gill GN, Cavenee WK, et al. A mutant epidermal growth factor receptor common in human glioma confers enhanced tumorigenicity. Proc Natl Acad Sci U S A (1994) 91:7727–31. doi: 10.1073/pnas.91.16.7727
130. Thorne AH, Zanca C, Furnari F. Epidermal growth factor receptor targeting and challenges in glioblastoma. Neuro-Oncol. (2016) 18:914–8. doi: 10.1093/neuonc/nov319
131. Lynch TJ, Bell DW, Sordella R, Gurubhagavatula S, Okimoto RA, Brannigan BW, et al. Activating mutations in the epidermal growth factor receptor underlying responsiveness of non-small-cell lung cancer to gefitinib. N Engl J Med (2004) 350:2129–39. doi: 10.1056/NEJMoa040938
132. Endersby R, Baker SJ. PTEN signaling in brain: neuropathology and tumorigenesis. Oncogene (2008) 27:5416–30. doi: 10.1038/onc.2008.239
133. Ohgaki H, Kleihues P. The definition of primary and secondary glioblastoma. Clin Cancer Res (2013) 19:764. doi: 10.1158/1078-0432.CCR-12-3002
134. Werbrouck C, Evangelista CCS, Lobón-Iglesias M-J, Barret E, Le Teuff G, Merlevede J, et al. TP53 pathway alterations drive radioresistance in diffuse intrinsic pontine gliomas (DIPG). Clin Cancer Res (2019) 25:6788. doi: 10.1158/1078-0432.CCR-19-0126
135. Cho S-Y, Park C, Na D, Han JY, Lee J, Park O-K, et al. High prevalence of TP53 mutations is associated with poor survival and an EMT signature in gliosarcoma patients. Exp Mol Med (2017) 49:e317–7. doi: 10.1038/emm.2017.9
136. Kannan K, Inagaki A, Silber J, Gorovets D, Zhang J, Kastenhuber ER, et al. Whole-exome sequencing identifies ATRX mutation as a key molecular determinant in lower-grade glioma. Oncotarget (2012) 3:1194–203. doi: 10.18632/oncotarget.689
137. Heaphy CM, de Wilde RF, Jiao Y, Klein AP, Edil BH, Shi C, et al. Altered telomeres in tumors with ATRX and DAXX mutations. Science (2011) 333:425–5. doi: 10.1126/science.1207313
138. Rasheed BKA, McLendon RE, Herndon JE, Friedman HS, Friedman AH, Bigner DD, et al. Alterations of the TP53 gene in human gliomas. Cancer Res (1994) 54:1324.
139. Lapointe S, Perry A, Butowski NA. Primary brain tumours in adults. Lancet (2018) 392:432–46. doi: 10.1016/S0140-6736(18)30990-5
140. Haase S, Garcia-Fabiani MB, Carney S, Altshuler D, Núñez FJ, Méndez FM, et al. Mutant ATRX: uncovering a new therapeutic target for glioma. Expert Opin Ther Targets (2018) 22:599–613. doi: 10.1080/14728222.2018.1487953
141. Yun E-J, Kim S, Hsieh J-T, Baek ST. Wnt/β-catenin signaling pathway induces autophagy-mediated temozolomide-resistance in human glioblastoma. Cell Death Dis (2020) 11:771. doi: 10.1038/s41419-020-02988-8
142. Ujifuku K, Mitsutake N, Takakura S, Matsuse M, Saenko V, Suzuki K, et al. miR-195, miR-455-3p and miR-10a∗ are implicated in acquired temozolomide resistance in glioblastoma multiforme cells. Cancer Lett (2010) 296:241–8. doi: 10.1016/j.canlet.2010.04.013
143. André-Grégoire G, Bidère N, Gavard J. Temozolomide affects extracellular vesicles released by glioblastoma cells. Cell Secretome Pers Regen Med (2018) 155:11–5.
144. Yi G, Xiang W, Feng W, Chen Z, Li Y, Deng S, et al. Identification of key candidate proteins and pathways associated with temozolomide resistance in glioblastoma based on subcellular proteomics and bioinformatical analysis. BioMed Res Int (2018) 2018:5238760. doi: 10.1155/2018/5238760
145. Chakraborty S, Hosen M, Ahmed M, Shekhar HU. Onco-Multi-OMICS approach: A new frontier in cancer research. BioMed Res Int (2018) 2018:9836256. doi: 10.1155/2018/9836256
146. Ellis MJ, Gillette M, Carr SA, Paulovich AG, Smith RD, Rodland KK, et al. Connecting genomic alterations to cancer biology with proteomics: The NCI clinical proteomic tumor analysis consortium. Cancer Discovery (2013) 3:1108–12. doi: 10.1158/2159-8290.CD-13-0219
147. Niclou SP, Fack F, Rajcevic U. Glioma proteomics: status and perspectives. Journal of Proteomics (2010) 73(10):1823–28. doi: 10.1016/j.jprot.2010.03.007
148. Chang L, Ni J, Beretov J, Wasinger VC, Hao J, Bucci J, et al. Identification of protein biomarkers and signaling pathways associated with prostate cancer radioresistance using label-free LC-MS/MS proteomic approach. Sci Rep (2017) 7:41834. doi: 10.1038/srep41834
149. Altelaar AFM, Frese CK, Preisinger C, Hennrich ML, Schram AW, Timmers HTM, et al. Benchmarking stable isotope labeling based quantitative proteomics. J Proteomics (2013) 88:14–26. doi: 10.1016/j.jprot.2012.10.009
150. Chahrour O, Cobice D, Malone J. Stable isotope labelling methods in mass spectrometry-based quantitative proteomics. J Pharm Biomed Anal (2015) 113:2–20. doi: 10.1016/j.jpba.2015.04.013
151. Boylan KL, Andersen JD, Anderson LB, Higgins L, Skubitz AP. Quantitative proteomic analysis by iTRAQ® for the identification of candidate biomarkers in ovarian cancer serum. Proteome Sci (2010) 8:31. doi: 10.1186/1477-5956-8-31
152. Calderón-González KG, et al. Determination of the protein expression profiles of breast cancer cell lines by quantitative proteomics using iTRAQ labelling and tandem mass spectrometry. J Proteomics (2015) 124:50–78. doi: 10.1016/j.jprot.2015.04.018
153. Moulder R, Bhosale SD, Goodlett DR, Lahesmaa R. Analysis of the plasma proteome using iTRAQ and TMT-based isobaric labeling. Mass Spectrom Rev (2018) 37:583–606. doi: 10.1002/mas.21550
154. Xu D, Zhu X, Ren J, Huang S, Xiao Z, Jiang H, et al. Quantitative proteomic analysis of cervical cancer based on TMT-labeled quantitative proteomics. J Proteomics (2022) 252:104453. doi: 10.1016/j.jprot.2021.104453
155. Kumar V, Ray S, Ghantasala S, Srivastava S. An integrated quantitative proteomics workflow for cancer biomarker discovery and validation in plasma. Front Oncol (2020) 10. doi: 10.3389/fonc.2020.543997
156. Dai P, Wang Q, Wang W, Jing R, Wang W, Wang F, et al. Unraveling molecular differences of gastric cancer by label-free quantitative proteomics analysis. Int J Mol Sci (2016) 17:69. doi: 10.3390/ijms17010069
157. Raimondo S, Saieva L, Cristaldi M, Monteleone F, Fontana S, Alessandro R. Label-free quantitative proteomic profiling of colon cancer cells identifies acetyl-CoA carboxylase alpha as antitumor target of citrus limon-derived nanovesicles. J Proteomics (2018) 173:1–11. doi: 10.1016/j.jprot.2017.11.017
158. Chokchaichamnankit D, Watcharatanyatip K, Subhasitanont P, Weeraphan C, Keeratichamroen S, Sritana N, et al. Urinary biomarkers for the diagnosis of cervical cancer by quantitative label−free mass spectrometry analysis. Oncol Lett (2019) 17:5453–68.
159. Macklin A, Khan S, Kislinger T. Recent advances in mass spectrometry based clinical proteomics: applications to cancer research. Clin Proteomics (2020) 17:17. doi: 10.1186/s12014-020-09283-w
160. Friedrich C, Schallenberg S, Kirchner M, Ziehm M, Niquet S, Haji M, et al. Comprehensive micro-scaled proteome and phosphoproteome characterization of archived retrospective cancer repositories. Nat Commun (2021) 12:3576. doi: 10.1038/s41467-021-23855-w
161. Sanchez-Vega F, Mina M, Armenia J, Chatila WK, Luna A, La KC, et al. Oncogenic signaling pathways in the cancer genome atlas. Cell (2018) 173:321–337.e10. doi: 10.1016/j.cell.2018.03.035
162. Objectives. Office of Cancer Clinical Proteomics Research - CPTAC (2022). Available at: https://proteomics.cancer.gov/programs/cptac/about/objectives.
163. cBioPortal for cancer genomics . Available at: https://www.cbioportal.org/.
164. Wang L-B, Karpova A, Gritsenko MA, Kyle JE, Cao S, Li Y, et al. Proteogenomic and metabolomic characterization of human glioblastoma. Cancer Cell (2021) 39:509–528.e20. doi: 10.1016/j.ccell.2021.01.006
165. Bukowski K, Kciuk M, Kontek R. Mechanisms of multidrug resistance in cancer chemotherapy. Int J Mol Sci (2020) 21:3233. doi: 10.3390/ijms21093233
166. Wheeler DA, Wang L. From human genome to cancer genome: the first decade. Genome Res (2013) 23:1054–62. doi: 10.1101/gr.157602.113
167. Autelitano F, Loyaux D, Roudières S, Déon C, Guette F, Fabre P, et al. Identification of novel tumor-associated cell surface sialoglycoproteins in human glioblastoma tumors using quantitative proteomics. PloS One (2014) 9:e110316. doi: 10.1371/journal.pone.0110316
168. Gollapalli K, Ray S, Srivastava R, Renu D, Singh P, Dhali S, et alInvestigation of serum proteome alterations in human glioblastoma multiforme. PROTEOMICS (2012) 12(14):2378–90. doi: 10.1002/pmic.201200002
169. Trombetta-Lima M, Rosa-Fernandes L, Angeli CB, Moretti IF, Franco YM, Mousessian AS, et al. Extracellular matrix proteome remodeling in human glioblastoma and medulloblastoma. J Proteome Res (2021) 20:4693–707. doi: 10.1021/acs.jproteome.1c00251
170. Polisetty RV, Gautam P, Sharma R, Harsha HC, Nair SC, Gupta MK, et al. LC-MS/MS analysis of differentially expressed glioblastoma membrane proteome reveals altered calcium signaling and other protein groups of regulatory functions*. Mol Cell Proteomics (2012) 11:M111.013565. doi: 10.1074/mcp.M111.013565
171. Zhang R, Tremblay T-L, Mcdermid A, Thibault P, Stanimirovic D. Identification of differentially expressed proteins in human glioblastoma cell lines and tumors. Glia (2003) 42:194–208. doi: 10.1002/glia.10222
172. Popescu ID, Codrici E, Albulescu L, Mihai S, Enciu A-M, Albulescu R, et al. Potential serum biomarkers for glioblastoma diagnostic assessed by proteomic approaches. Proteome Sci (2014) 12:47. doi: 10.1186/s12953-014-0047-0
173. Melchior K, Tholey A, Heisel S, Keller A, Lenhof H-P, Meese E, et al. Proteomic study of human glioblastoma multiforme tissue employing complementary two-dimensional liquid chromatography- and mass spectrometry-based approaches. J Proteome Res (2009) 8:4604–14. doi: 10.1021/pr900420b
174. Sun S, Wong TS, Zhang XQ, Pu JKS, Lee NP, Day PJR, et al. Protein alterations associated with temozolomide resistance in subclones of human glioblastoma cell lines. J Neurooncol (2012) 107:89–100. doi: 10.1007/s11060-011-0729-8
175. Guo J, Yi G, Liu Z, Sun X, Yang R, Guo M, et al. Quantitative proteomics analysis reveals nuclear perturbation in human glioma U87 cells treated with temozolomide. Cell Biochem Funct (2020) 38:185–94. doi: 10.1002/cbf.3459
176. Dong F, Eibach M, Bartsch JW, Dolga AM, Schlomann U, Conrad C, et al. The metalloprotease-disintegrin ADAM8 contributes to temozolomide chemoresistance and enhanced invasiveness of human glioblastoma cells. Neuro-Oncol. (2015) 17:1474–85. doi: 10.1093/neuonc/nov042
177. Wang H, Feng W, Lu Y, Li H, Xiang W, Chen Z, et al. Expression of dynein, cytoplasmic 2, heavy chain 1 (DHC2) associated with glioblastoma cell resistance to temozolomide. Sci Rep (2016) 6:28948. doi: 10.1038/srep28948
178. Kumar DM, Patil V, Ramachandran B, Nila MV, Dharmalingam K, Somasundaram K., et al. Temozolomide-modulated glioma proteome: Role of interleukin-1 receptor-associated kinase-4 (IRAK4) in chemosensitivity. PROTEOMICS (2013) 13:2113–24. doi: 10.1002/pmic.201200261
179. Miyata H, Ashizawa T, Iizuka A, Kondou R, Nonomura C, Sugino T, et alCombination of a STAT3 inhibitor and an mTOR inhibitor against a temozolomide-resistant glioblastoma cell line. Cancer Genomics Proteomics (2017) 14(1):83–91. doi: 10.21873/cgp.20021
180. Lin R, Xu Y, Xie S, Zhang Y, Wang H, Yi G-Z, et al. Recycling of SLC38A1 to the plasma membrane by DSCR3 promotes acquired temozolomide resistance in glioblastoma. J Neurooncol (2022) 157:15–26. doi: 10.1007/s11060-022-03964-2
181. Azimi A, Pernemalm M, Frostvik Stolt M, Hansson J, Lehtiö J, Egyházi Brage S, et al. Proteomics analysis of melanoma metastases: association between S100A13 expression and chemotherapy resistance. Br J Cancer (2014) 110:2489–95. doi: 10.1038/bjc.2014.169
182. Lichti CF, Wildburger NC, Shavkunov AS, Mostovenko E, Liu H, Sulman EP, et al. The proteomic landscape of glioma stem-like cells. EuPA Open Proteomics (2015) 8:85–93. doi: 10.1016/j.euprot.2015.06.008
183. Ampudia-Mesias E, El-Hadad S, Cameron CS, Wöhrer A, Ströbel T, Saydam N, et al. SRPX emerges as a potential tumor marker in the extracellular vesicles of glioblastoma. Cancers (2022) 14:1984. doi: 10.3390/cancers14081984
184. de Aquino PF, Carvalho PC, Nogueira FCS, da Fonseca CO, de Souza Silva JCT, Carvalho da da M GC, et al. A time-based and intratumoral proteomic assessment of a recurrent glioblastoma multiforme. Front Oncol (2016) 6. doi: 10.3389/fonc.2016.00183
185. Edwards NJ, Oberti M, Thangudu RR, Cai S, McGarvey PB, Jacob S, et al. The CPTAC data portal: A resource for cancer proteomics research. J Proteome Res (2015) 14:2707–13. doi: 10.1021/pr501254j
186. Hara A, Kanayama T, Noguchi K, Niwa A, Miyai M, Kawaguchi M, et al. Treatment strategies based on histological targets against invasive and resistant glioblastoma. J Oncol (2019) 2019:e2964783. doi: 10.1155/2019/2964783
187. Becker AP, Sells BE, Haque SJ, Chakravarti A. Tumor heterogeneity in glioblastomas: From light microscopy to molecular pathology. Cancers (2021) 13:761. doi: 10.3390/cancers13040761
188. Sasmita AO, Wong YP, Ling APK. Biomarkers and therapeutic advances in glioblastoma multiforme. Asia Pac J Clin Oncol (2018) 14:40–51. doi: 10.1111/ajco.12756
Keywords: cellular proteomics, glioblastoma multiforme (GBM), temozolomide resistance (TMZ resistance), IDH, chemoresistance, MGMT, LC-MS
Citation: Teraiya M, Perreault H and Chen VC (2023) An overview of glioblastoma multiforme and temozolomide resistance: can LC-MS-based proteomics reveal the fundamental mechanism of temozolomide resistance? Front. Oncol. 13:1166207. doi: 10.3389/fonc.2023.1166207
Received: 14 February 2023; Accepted: 23 March 2023;
Published: 26 April 2023.
Edited by:
Tsung-I Hsu, Taipei Medical University, TaiwanReviewed by:
Hong-Yi Lin, Taipei Medical University, TaiwanAmrita Sule, Broad Institute, United States
Shao-Ming Wang, China Medical University, Taiwan
Copyright © 2023 Teraiya, Perreault and Chen. This is an open-access article distributed under the terms of the Creative Commons Attribution License (CC BY). The use, distribution or reproduction in other forums is permitted, provided the original author(s) and the copyright owner(s) are credited and that the original publication in this journal is cited, in accordance with accepted academic practice. No use, distribution or reproduction is permitted which does not comply with these terms.
*Correspondence: Milan Teraiya, teraiyam@myumanitoba.ca; Helene Perreault, Helene.Perreault@umanitoba.ca