- 1Beijing Hospital, National Center of Gerontology, Institute of the Geriatric Medicine, Chinese Academy of Medical Sciences & Peking Union Medical College, Beijing, China
- 2Beijing Hospital Continence Center, Beijing, China
Purpose: This study was designed to investigate the clinical value of a simplified five-item frailty index (sFI) for predicting short- and long-term outcomes in older patients with upper urinary tract urothelial carcinoma (UTUC) patients after radical nephroureterectomy (RNU).
Method: This retrospective study included 333 patients (aged ≥65 years) with UTUC. Patients were classified into five groups: 0, 1, 2, 3, and 3+, according to sFI score. The variable importance and minimum depth methods were used to screen for significant variables, and univariable and multivariable logistic regression models applied to investigated the relationships between significant variables and postoperative complications. Survival differences between groups were analyzed using Kaplan-Meier plots and log-rank tests. Cox proportional hazards regression was used to evaluate risk factors associated with overall survival (OS) and cancer-specific survival (CSS). Further, we developed a nomogram based on clinicopathological features and the sFI. The area under the curve (AUC), Harrel’s concordance index (C-index), calibration curve, and decision curve analysis (DCA) were used to evaluate the nomogram.
Result: Of 333 cases identified, 31.2% experienced a Clavien-Dindo grade of 2 or greater complication. Random forest–logistic regression modeling showed that sFI significantly influenced the incidence of postoperative complications in older patients (AUC= 0.756). Compared with patients with low sFI score, those with high sFI scores had significantly lower OS and CSS (p < 0.001). Across all patients, the random survival forest–Cox regression model revealed that sFI score was an independent prognostic factor for OS and CSS, with AUC values of 0.815 and 0.823 for predicting 3-year OS and CSS, respectively. The nomogram developed was clinically valuable and had good ability to discriminate abilities for high-risk patients. Further, we developed a survival risk classification system that divided all patients into high-, moderate-, and low-risk groups based on total nomogram points for each patient.
Conclusion: A simple five-item frailty index may be considered a prognostic factor for the prognosis and postoperative complications of UTUC following RNU. By using this predictive model, clinicians may increase their accuracy in predicting complications and prognosis and improve preoperative decision-making.
1 Introduction
Urinary tract urothelial carcinoma (UTUC), a transitional cell carcinoma of the renal pelvis and ureter, is a rare tumor with poor prognosis and an incidence of approximately 2 per 100,000 in developed countries (1–3). Due to lack of symptoms and delayed diagnosis, tumors usually develop muscle invasion or local progression, resulting in a worse prognosis than bladder cancer. For patients with T2/T3 and T4 stage tumors, the 5-year specific survival rates are less than 50% and 10%, respectively (1). Despite advances in systemic therapy and immunotherapy in recent years (4), radical nephroureterectomy (RNU) with bladder cuff resection remains the standard treatment for high-risk UTUC, regardless of tumor location. Factors related to prognosis after RNU mainly include clinicopathological features, such as lymphovascular invasion, pathological TNM stage, concomitant carcinoma in situ, and tumor multifocality, among others (1).
Population aging is among the most important trends worldwide, and the peak incidence of UTUC is in individuals aged 70–90 years, including high prevalence in people with frailty (1, 5). Frailty is a multidimensional physiological syndrome, typically involving loss of reserves (such as energy, physical capacity, cognition, and health) and increased vulnerability (6, 7). Consequently, frail older patients are more likely to have negative surgical outcome in the perioperative period. Frailty before surgery has been shown to objectively predict postoperative complications, mortality, and extended hospital stay (8–16). The Comprehensive Geriatric Assessment (CGA) is the gold standard for determining frailty (17), however, it requires specialist expertise and is time-consuming, making it less thanideal for use in routine clinical practice. Therefore, many scholars have attempted to use alternative methods to measure the degree of frailty. Fried and colleagues first proposed a standardized phenotype of frailty in older adults and demonstrated that frailty is associated with risk of adverse outcomes in such individuals (6). In 2005, the Canadian Study of Health and Aging developed a 70-item Frailty Index based on the presence and severity of current diseases, ability to perform activities of daily living, and physical and neurological signs from clinical examinations (7). In addition, several other indicators have also been shown to accurately assess frailty, such as the modified Frailty Index (mFI) (18–20) and the Memorial Sloan Kettering-Frailty Index (8). Although these indicators measurement indices represent improvements, their feasibility under the pressure of clinical work remains questionable. Therefore, the original modified frailty index (mFI-11) was modified to generate the mFI-5 by removing some variables, and the ability of mFI-5 to characterize frailty has been demonstrated in many studies (9, 21–27). Overall, frailty is an independent predictor of adverse outcomes after surgery, particular in older adults.
While many clinicopathological indicators may predict prognosis and morbidity in patients with UTUC, indicators that are simpler and easier to use in a clinical setting are also needed. Furthermore, preoperative risk and prognosis assessment tools currently used in older patients with UTUC do not include frailty. Therefore, in this study, we aimed to assess the prognostic significance of a simplified five-item frailty index on short-term (postoperative complications) and long-term (overall survival (OS) and cancer-specific survival (CSS)) outcomes after RNU for elderly patients with UTUC.
2 Patients and methods
2.1 Study population
Patients aged > 65 years undergoing RNU in the Department of Urology, Beijing Hospital, National Center of Gerontology, were retrospectively enrolled. The inclusion criteria were as follows: (1) patients aged ≥65 years; (2) patients undergoing RNU; (3) patients with histopathologically verified urothelial carcinoma; (4) patients without metastatic lesions; (5) patients with complete clinical and follow-up information. A total of, 333 elderly patients with UTUC (age ≥ 65 years) were included in the final study.
2.2 Data collection and definition of variables
Preoperative patient demographic and general clinical data were collected from patient electronic hospital records, including patient age, weight, height, body mass index (BMI), urine pathology, and presence of preoperative hydronephrosis. Patient comorbidities, including smoking status in the last year, chronic obstructive pulmonary disease, congestive cardiac failure, anti-hypertensive treatment, preoperative acute renal failure, diabetes mellitus, and functional status, were recorded. Pathological findings included cancer-related data, such as tumor stage, tumor grade, tumor site, tumor side, tumor size, lympho-vascular invasion (LVI), multifocality, concomitant CIS (carcinoma in situ), and surgical margin. Intraoperative and postoperative data, including American Society of Anesthesiologists (ASA) score, surgical treatment, blood transfusion, operation time, and postoperative complications, were obtained from anesthesia records, operative documents, and electronic medical record systems.
The simplified five-item frailty index (sFI) was calculated using five dichotomous comorbidity categories, as follows: history of diabetes mellitus, history of congestive heart failure, hypertension requiring medication, history of chronic obstructive pulmonary disease, and functional status, as previously reported (21–23, 28, 29). Functional status was defined as requiring assistance for any activities of daily living (ADLs), including bathing, feeding, dressing, and mobility. Each comorbidity was assigned one point, giving scores in the range,0 to 5. As a result of limitations in sample size, patients were divided into five groups based on sFI score, as follows: 0, 1, 2, 3, and 3+. In this study, OS was defined as the length of time from diagnosis to death or last follow-up visit and. CSS was calculated from the date of surgery to the date of death from cancer. Postoperative complications were graded according to the Clavien-Dindo classification system.
2.3 Features selection
Rapid advances in artificial intelligence have led to an explosion in the use of machine learning to develop prediction models for various diseases in recent years (30–33). Random forest is a classification algorithm comprising numerous many decision trees. Thus, random forests can better predict outcomes than individual classification trees, which can also automatically identify nonlinear effects between variables. A random survival forest model can be constructed by combining random forest and traditional survival analyses; random survival forest models are susceptible to outliers and should, therefore, be used in conjunction with, rather than as a complete replacement for, traditional survival analysis.Thus, we first collected data on clinical and pathological parameters. Then, variable screening was conducted using two methods, variable importance (VIMP) and minimal depth. A VIMP value less than 0 indicates that a variable reduces prediction accuracy, while a value greater than 0 indicates that a variable improves prediction accuracy. The minimum depth rule determines the importance of each variable to the final event by calculating the minimum depth when running to the last node; where variables with smaller values are more critical to the model. We use random forest’s VIMP and minimal depth method to select variables for predictive model construction.
2.4 Statistical analysis
Statistical analyses were performed using R4.2.1 (R Core Team, Vienna, Austria). The Chi-square or Fisher’s exact tests were used to compare categorical variables. Continuous variables are described using mean with standard deviation (SD) and medians with interquartile range (IQR). The Kaplan–Meier method was applied to evaluate OS and CSS rates, and the log-rank test used to analyze significance. Univariable and multivariable Cox regression models were applied to assess risk factors associated with OS and CSS. Similarly, a multivariable logistic regression model was applied to assess relationships between variables and postoperative complications. All significant factors were incorporated into anomogram. The concordance index (C-index) and the area under the time-dependent receiver operating characteristic (ROC) curve (time-dependent AUC) were used to assess the discriminative ability of models. Calibration ability was evaluated using calibration plots. Decision Curve Analysis (DCA) was used to determine the clinical usefulness of the nomogram by calculating the net benefit at different threshold probabilities. Patients were divided into three groups according to total nomogram scores: low-, intermediate-, and high-risk. P<0.05 was considered statistically significant.
3 Results
3.1 Patient characteristics
The clinical characteristics of the 333 patients included in this study are shown in Table 1. Median follow-up time was 36.67 months (IQR: 21.83–67.93 months). Mean patients age was 74 ± 5.80 years, and mean operative time was 227 ± 71.6 minutes. Of patients, 264 were < 80 years old, and 69 were ≥ 80 years of age. Further, 148 (44.4%) patients were male, and 185 (55.6%) were female. Median BMI was 24.14 kg/m2 (IQR: 21.64–26.40 kg/m2). Pathological tumor stage was pT1, T2, T3, and T4 in 82 (24.6%), 103(30.9%), 132 (39.6%), and 16 (4.80%)patients, respectively. Primary tumors located in the renal pelvis accounted for 39.3%, while 60.7% were in the ureter. Approximately 12.6% of patients had multiple tumors at diagnosis, and the majority of patients (82.0%) had a high histologic grade. Patients were grouped according to sFI scores, as follows: sFI 0, 31 (9.31%); sFI 1, 87 (26.1%); sFI 2, 86 (25.8%); sFI 3, 88 (26.4%); and sFI 3+, 41 (12.3%). Within this population, 21 (6.31%) patients had a history of cerebral infarction, and 45 (13.5%) of renal failure.
3.2 Postoperative complications
At least one Clavien-Dindo postoperative complication grade ≥ 2 developed in 104 (31.2%) patients (Table 1). A breakdown of postoperative complications is presented in Table 2. A total of 138 postoperative complications were observed in this study: Clavien-Dindo grade 2, 95 (68.8%); Clavien-Dindo grade 3, 10 (7.2%); Clavien-Dindo grade 4, 31 (22.5%); and Clavien-Dindo grade 5, 2 (1.4%). The number of postoperative complications differs from the number of patients who experienced them, as some patients experienced more than one complication. Delirium was the most common complication (n=11, 9.6%), followed by Incision fat liquefaction (defined as necrosis of adipose tissue without infection) (n=11, 8.0%), requiring intensive care unit admission (n=10, 7.2%), pneumonia (n=9, 6.5%) and urinary tract infection (n=8, 5.8%). Overall, 3 deaths (sFI score 3+, 2 deaths; sFI score 3, 1 death) occurred during the initial 30 days after surgery (0.9% 30-day mortality rate). 90-day mortality was 2.1% (7 deaths: sFI score 3+: 3 deaths; sFI score 3: 2 deaths; sFI score 2: 2 deaths). Postoperative hospital length of stay and readmission data are summarized in Table S1.
Based on VIMP and the minimal depth analyses, major predictors of postoperative complications after surgery were history of cerebral infarction, sFI score, renal failure, smoking, ASA score, age, and sex (Supplementary Figure 1). Moreover, we performed logistic regression analysis to determine which factors were associated with Clavien-Dindo grade ≥3 postoperative complications (Table 3),and found that the history of cerebral infarction, sFI, renal failure, smoking, ASA score, and age were significantly associated with postoperative complications. Multivariable analysis revealed that history of cerebral infarction (odds ratio (OR)=4.587, p=0.003), sFI (sFI=2~3 vs. sFI=0~1, OR=3.121, p = 0.031; sFI=4~5 vs. sFI=0~1, OR=6.666, p= 0.002), renal failure (OR=2.617, p=0.020), smoking (OR=2.284, p=0.024), and age (OR=2.174, p=0.035) were significant independent predictors of complications graded as Dindo-Clavien 3 or more. The C-Index of the model was 0.756 (95% confidence interval (CI),0.703-0.809).
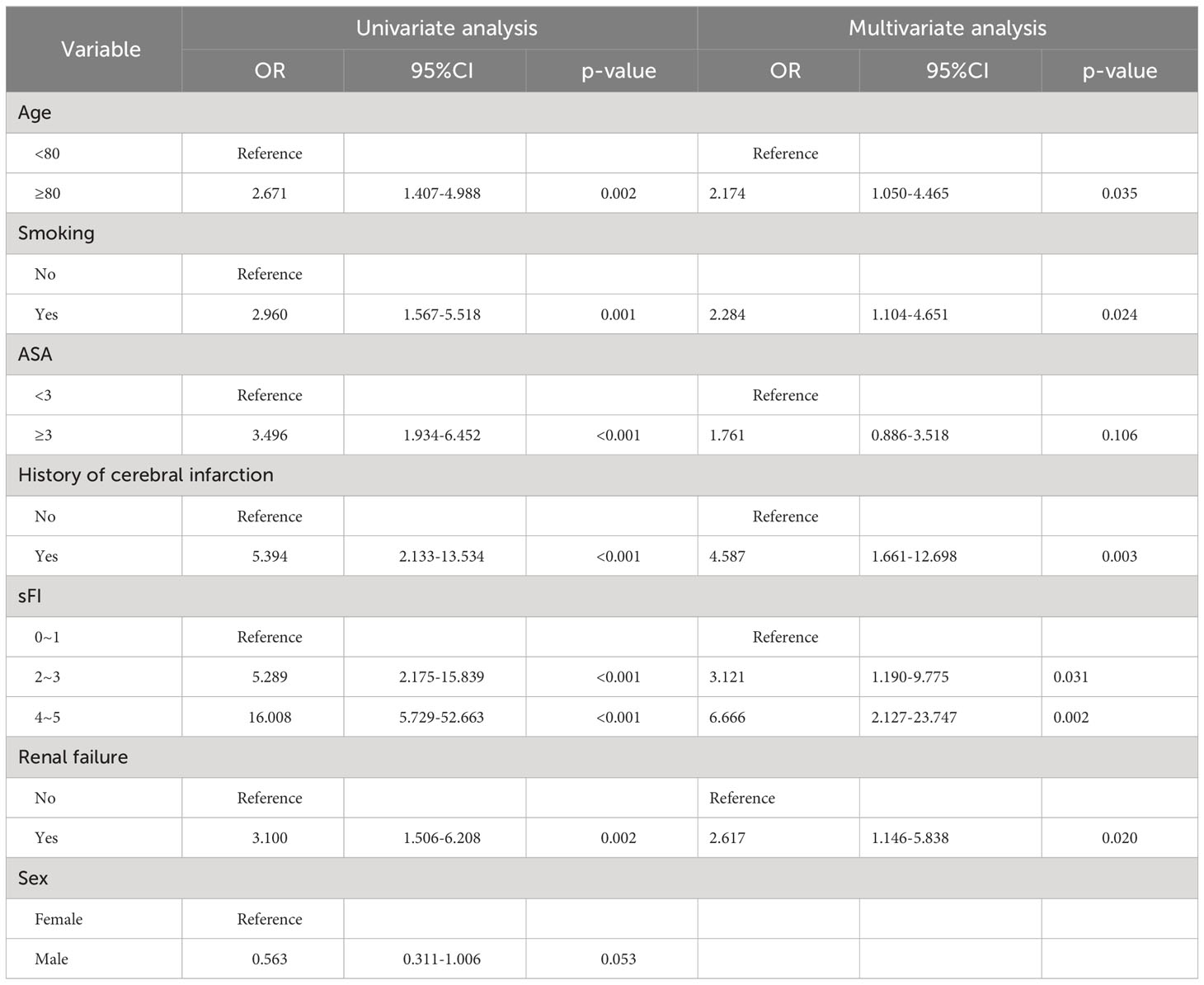
Table 3 Univariate and multivariate logistic regression model assessing predictors of postoperative complications.
Based on the results of multivariable analysis, five factors were combined to establish a nomogram (Supplementary Figure 2). AUC analysis of ROC curves was used to evaluate the discrimination performance of the nomogram. As shown in Supplementary Figure 3, the AUC value, calibration plot, and DCA curves for the model suggested acceptable performance and discrimination, calibrating ability, and clinical usefulness.
3.3 Overall survival and cancer-specific survival according to sFI
As shown in Figure 1, we analyzed OS and CSS curves according to sFI. There were significant survival differences in OS and CSS (p<0.001) among the five groups of patients classified by sFI. Eight variables selected by VIMP and minimal depth methods were chosen for subsequent modeling (variables below the horizontal dotted line) (Figure 2). Based on univariate analysis, age, smoking, hydronephrosis, LVI, T stage, N stage, margin, and sFI score were significantly associated with worse survival rate. Those eight variables were included in multivariate Cox regression analysis, which showed that age (hazard ratio (HR)=1.055, p=0.003), hydronephrosis (HR=1.779, p=0.019), LVI (HR=2.087, p=0.003), T stage (T2 vs. T1, HR=1.798, p = 0.037; T3 vs. T1, HR=2.219, p = 0.003; T4 vs. T1, HR=3.476, p = 0.002), N stage (HR=3.170, p<0.001), margin (HR=2.517, p=0.015), and sFI score (sFI=2 vs. sFI=0, HR=3.025, p = 0.037; sFI=3 vs. sFI=0, HR=3.407, p = 0.023; sFI=3+ vs. sFI=0, HR=3.965, p = 0.016) were all significant prognostic factors for OS (Table 4).
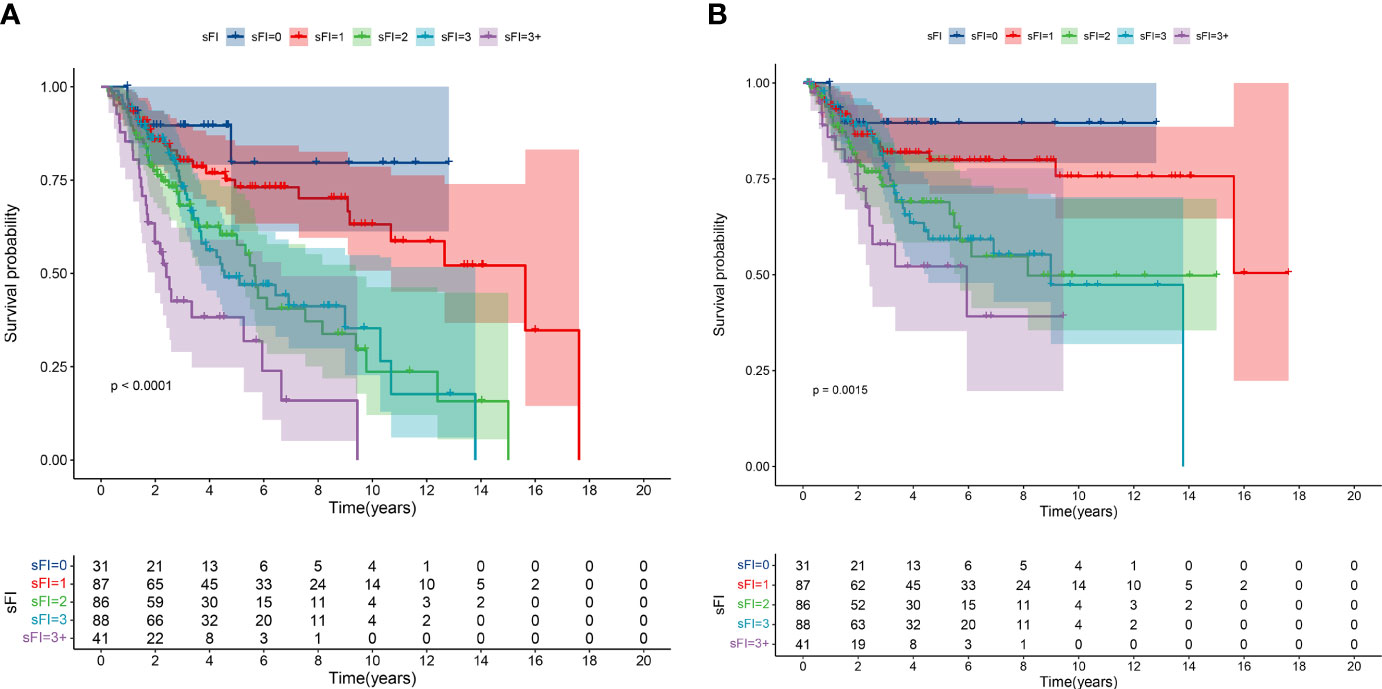
Figure 1 Kaplan–Meier curves for overall survival (OS) (A) and cancer-specific survival (CSS) (B) in patients with UTUC according to sFI.
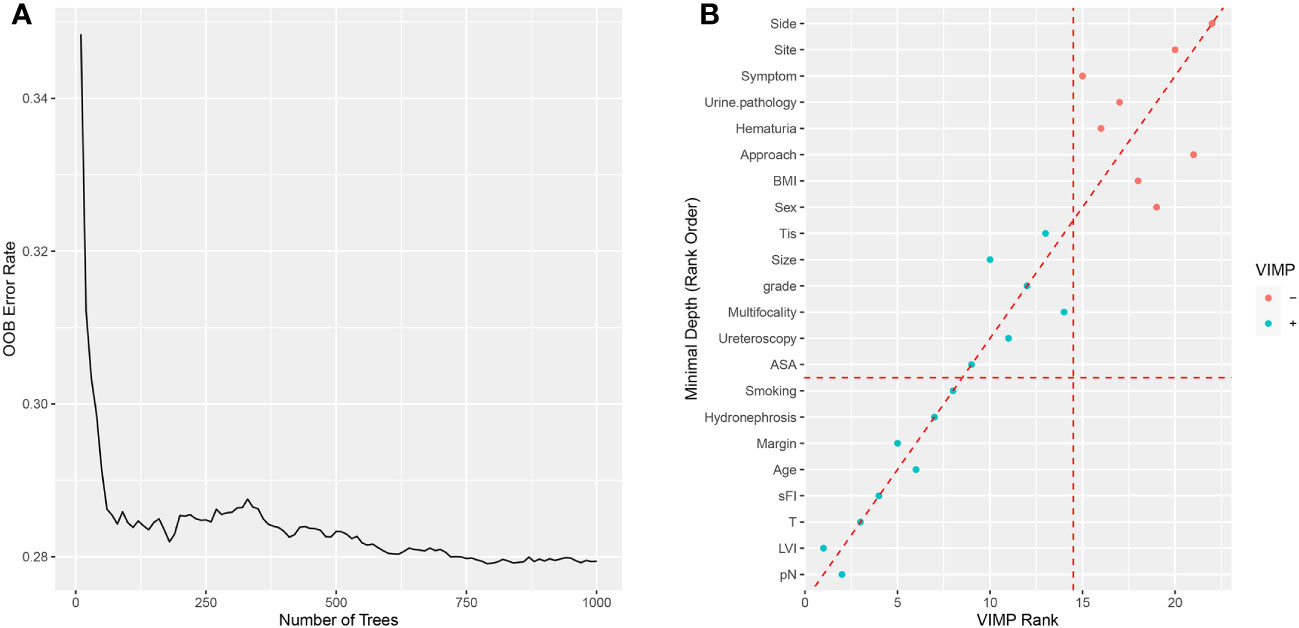
Figure 2 Random survival forest for OS. (A) The prediction error rate for random survival forests of 1000 trees. (B) Variables selected by VIMP and minimal depth.
Regarding CSS, seven variables were included in the univariate analysis (Supplementary Figure 4; Table 5). Six factors(hydronephrosis, LVI, T stage, N stage, margin, and sFI score)had significant effects on CSS and were identified as prognostic factors (p < 0.05) (Table 5).
3.4 Development and validation of a novel prognostic nomogram
Based on the results of multivariable analysis, a nomogram for OS and CSS prediction was constructed (Figure 3; Supplementary Figure 5). C-index values of the nomogram for OS and CSS prediction were 0.750 (95% CI, 0.704–0.796) and 0.781 (95% CI, 0.732–0.830), respectively. In addition, the time-dependent AUC values for predicting OS and CSS within 5 years were both > 0.7 for predicting OS and CSS within 5 years (Figure 4; Supplementary Figure 6), indicating good discrimination by the nomogram. The 1-, 3-, and 5-year AUC values for OS were 0.697, 0.815, and 0.862, respectively (Figure 4), while for CSS, the 1-, 3-, and 5-year AUC values were 0.772, 0.823, and 0.843, respectively (Supplementary Figure 6). Calibration curves showed good agreement between the predicted and observed risks for both OS and CSS (Figure 5; Supplementary Figure 7). As shown in Figure 6 and Supplementary Figure 8, DCA suggested that the nomogram demonstrated a higher net benefit than pathology factors for predicting 1-, 3-, and 5-year OS and CSS.
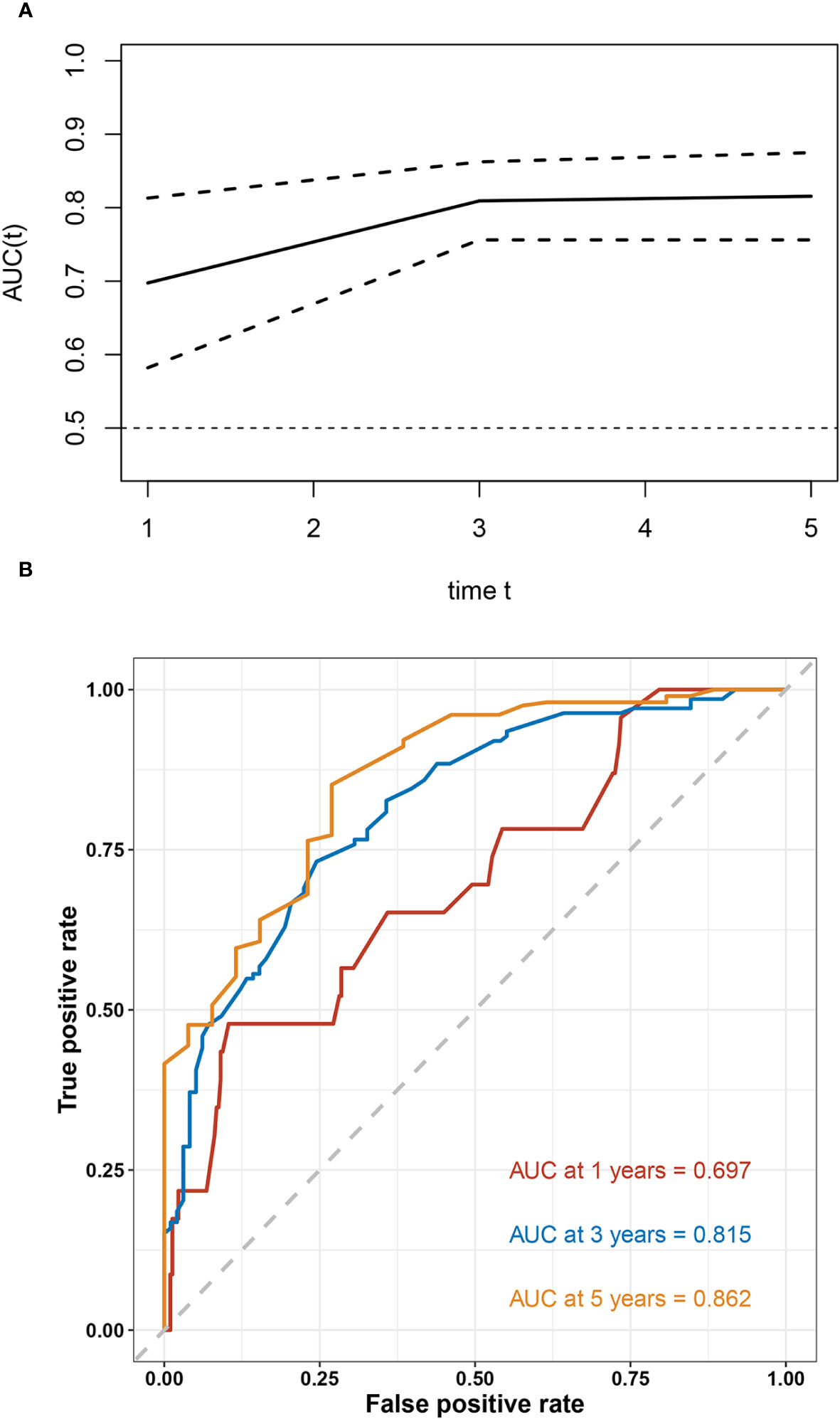
Figure 4 Evaluation of the discriminative ability of the OS nomogram. The time-independent AUC (A) and ROC curves (B) for the nomogram.
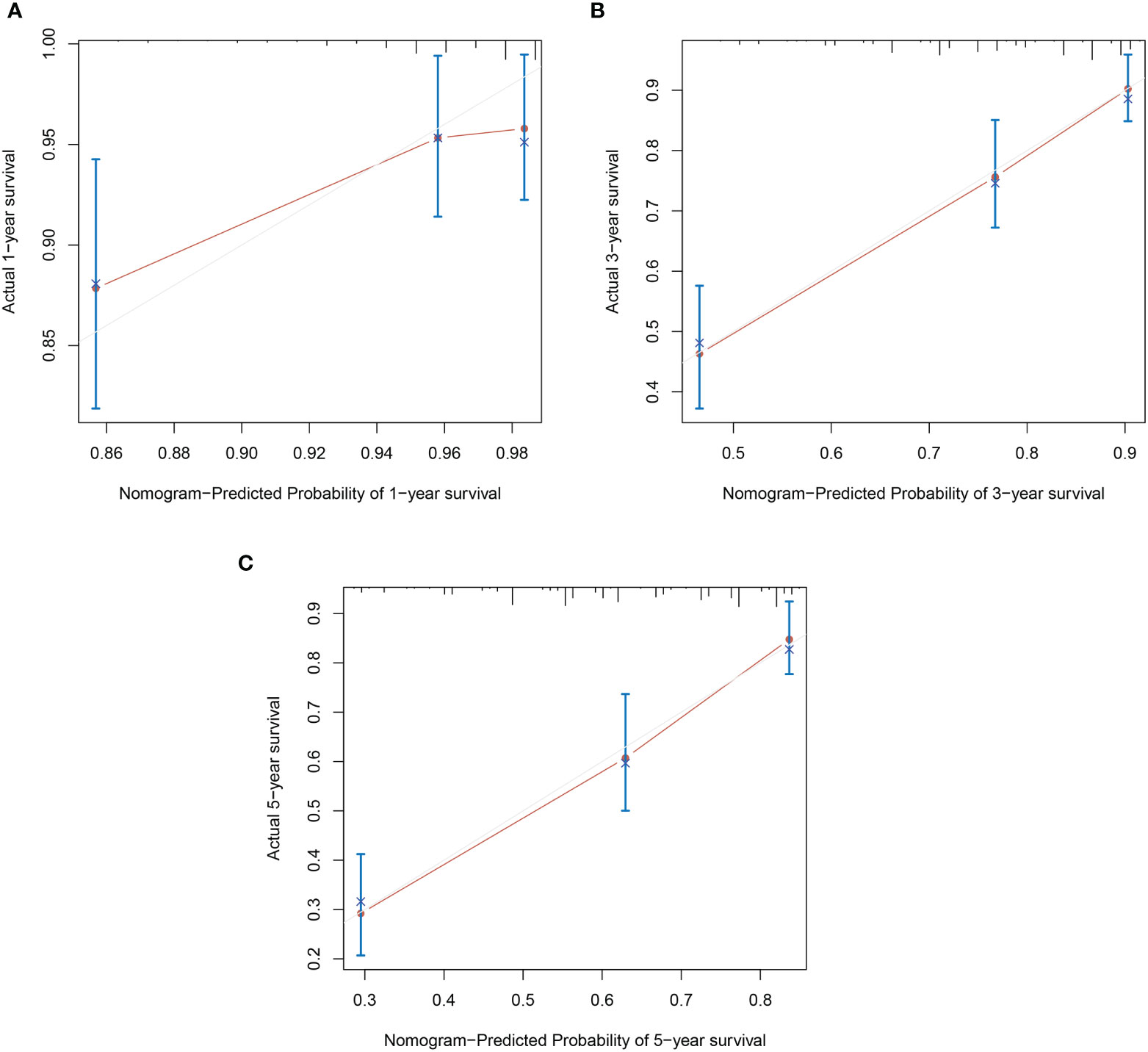
Figure 5 Calibration plots of OS nomogram model. (A) 1-year calibration plot of OS; (B) 3-year calibration plot of OS; (C) 5-year calibration plot of OS.
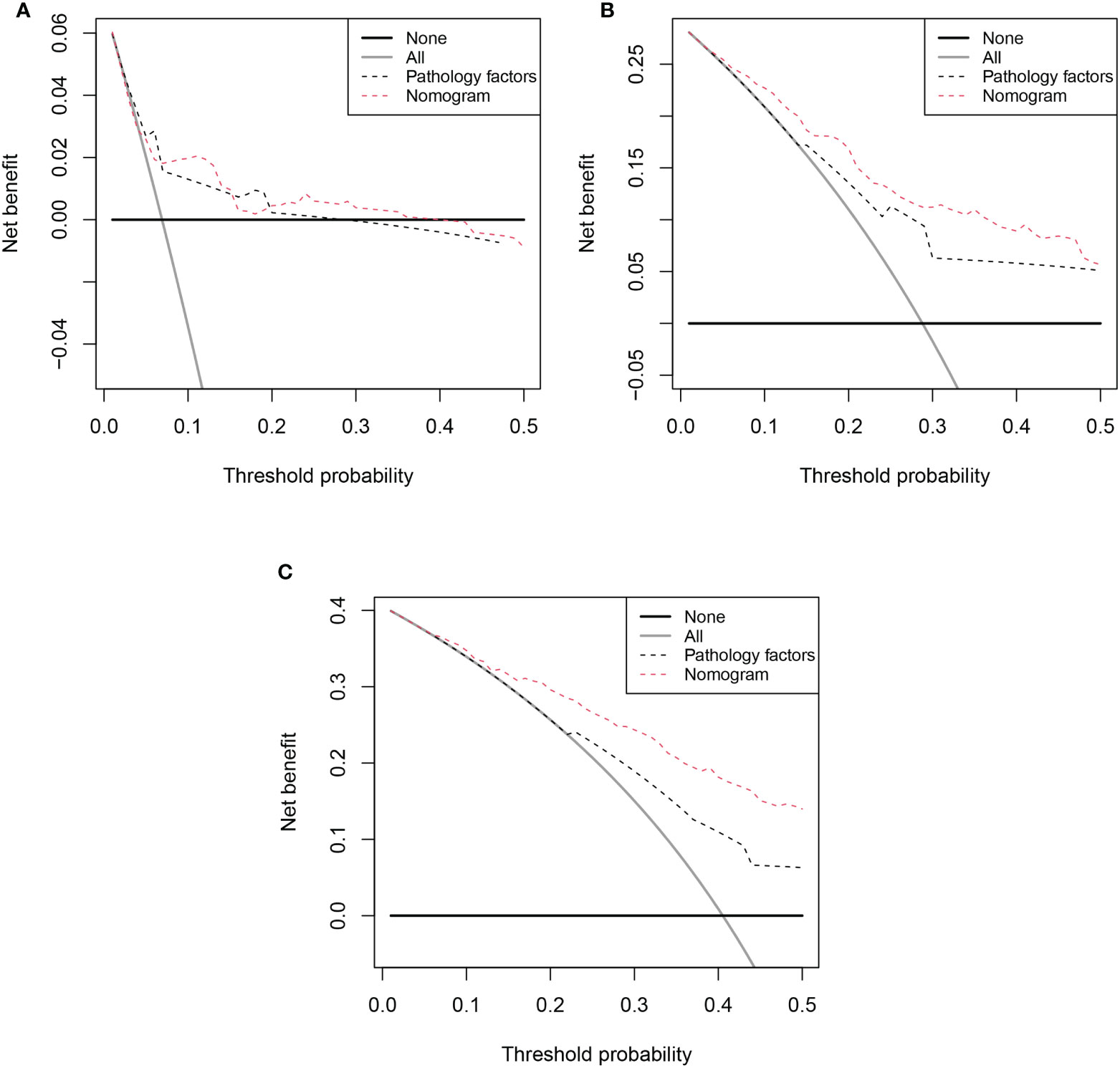
Figure 6 Decision curve analysis (DCA) of OS nomogram. (A) 1-year DCA of nomogram; (B) 3-year DCA of nomogram; (C) 5-year DCA of nomogram;.
3.5 Risk stratification based on the nomogram
Finally, we constructed a risk classification systembased on the patient total nomogram scores. Patients were divided into three groups: low-risk (score < 95), intermediate-risk (95 ≤ score < 175), and high-risk (score ≥ 175). Significant differences were detected among the Kaplan-Meier curves for OS and CSS of the three groups (Figure 7; Supplementary Figure 9).
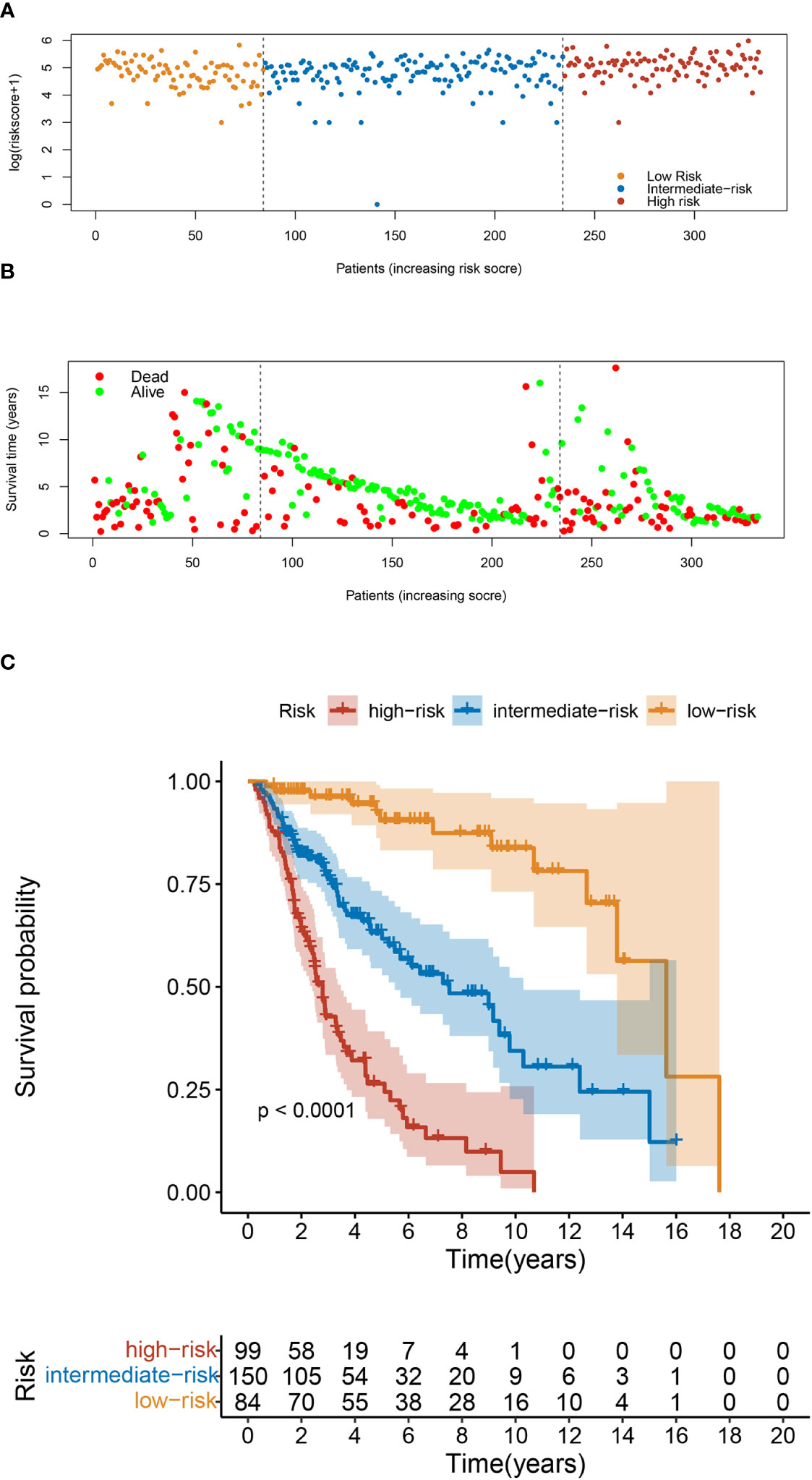
Figure 7 Risk Classification based on OS nomogram. (A) Survival status map. (B) Risk heatmap. (C) Kaplan–Meier overall survival curves of patients with UTUC with different risks stratified by the nomogram.
4 Discussion
Among all types of cancer, upper tract urothelial carcinoma is one of the most aggressive. RNU with bladder cuff resection is the gold-standard treatment for high-risk UTUC. However, surgery has considerable risks, despite its excellent oncological outcomes. It was reported that 20.5% of patients experienced morbidity, and 1.5% died within 30 days after surgery (34). Moreover, the growing geriatric population and advance in minimally invasive procedures have boosted the demand for radical procedures, such as RNU, in the elderly. But a lower physiological reserve mean that risks are higher for older adults. Therefore, it is very important to assess the risk of complications and prognosis in the elderly. It was reported recently that frailty is strongly associated with postoperative morbidity and in-hospital mortality in older patients (20, 35); however, the association between frailty and outcomes in UTUC is unknown.
To our best knowledge, this was the first study to validate the effectiveness of the sFI in older patients with UTUC undergoing RNU. One strength and a novelty feature of the present study is the application of machine learning methods. By employing a random forest model, we determined the importance of each variable using the VIMP and minimal depth methods. We found that sFI was associated with postoperative complications, consistent with previous studies. Further, we constructed a nomogram based on five variables with a good predictive value for postoperative complications in elderly patients with UTUC. Moreover, we assessed the relationship between sFI and prognosis, and found that high sFI scores were significantly associated with worse OS (p<0.001) and CSS(p=0.0015). High sFI score was identified as an independent risk factor for OS and CSS. We also integrated sFI and clinical variables into a nomogram to predict OS and CSS, where nomograms have shown excellent ability to individualize risk stratification.
Frailty is characterized by multifactorial deterioration in energy metabolism, strength, endurance, and function beyond what is expected for a person of a given age (6, 7, 36, 37). Meta-analysis showed that the prevalence of frailty among older adults undergoing general surgery ranged from 8% to 77.8% (5). Recently, frailty has been increasingly recognized as a common feature affecting surgical outcomes, such as increased risk of postoperative complications, mortality, and prolonged hospital stay. The mFI-11 index is used to evaluate frailty and predict postoperative mortality and morbidity (18, 38); for example, Dicpinigaitis and colleagues reported that higher mFI-11 score is associated with development of severe complications, but not with in-hospital mortality or extended length of stay (39). In addition, Heimann et al. found that preoperative frailty independently predicts OS in elderly patients with brain metastases requiring surgery (40). Banaszek et al. also concluded that mFI-11 is an independent predictor of adverse events, acute length of stay, and in-hospital mortality in patients after traumatic spinal cord injury (41); however, there remains a lack of consensus regarding the best way to assess frailty, in terms of feasibility and reliability in daily clinical practice.
Consequently, the reorganized 5-item modified frailty index (mFI-5) was developed based on five routinely collected patient comorbidity factors. According to the present study, patients with higher mFI-5 score had higher levels of perioperative complication and worse survival rates, consistent with previous findings (9). Additionally, Subramaniam et al. found that the mFI-5 was a significant independent predictor of mortality and postoperative complications (42). Likewise, in a retrospective National Surgical Quality Improvement Program study of 336,556 patients undergoing primary hip and knee arthroplasty, Traven et al. found that frailty was a significant independent predictor of postoperative complications, including life-threatening medical complications, surgical site infections, readmission, and mortality within 30 days (21). Similarly, in a multicenter retrospective study, Yamashita et al. showed that high preoperative modified 5-item frailty index score was a significant independent predictor of poor OS (23). These studies support our conclusion that the 5-item modified frailty index (mFI) is a proven and reliable method to characterize frailty and as associated with postoperative complications and poor prognosis. Therefore, surgical and prognostic risk stratification based on mFI-5 score can help surgeons assess surgical risk and predict patient prognosis, as well as guiding post-surgery recovery plans and assisting in the prevention of complications in high-risk patients.
Our study indicated that sFI has significant impact on CSS. However, Other studies suggest that frailty is associated with perioperative and short-term outcomes but not cancer-specific outcomes (43). This may be attributed to various factors. Firstly, these studies assessed frailty differently, which may lead to heterogeneity. Further, the follow-up period is short, which may have affected survival outcomes. Secondly, elevated levels of some inflammatory cytokines have been reported in frail patients (44), suggesting that chronic inflammation may play a role. Chronic inflammation is closely related to the occurrence and progression of tumors. In addition, frail patients cannot tolerate the trauma and side effects of treatment, which will also affect the patient’s prognosis. Although the underlying mechanisms between frailty and poor prognosis are not well understood, these reasons may explain the poorer prognosis of frail patients. We also found that smoking was a risk factor for major complications but not for long-term outcomes. Many previous studies have shown that smoking is a risk factor for postoperative complications (45, 46). However, there have been few studies on the relationship between smoking and postoperative complications of UTUC. Therefore, our results can provide guidance for the perioperative period, and indicate that smoking cessation is necessary for patients undergoing surgery for UTUC. However, in terms of long-term prognosis, smoking is not associated with non-negligible differences in overall mortality and cancer specific mortality rates. This finding is inconsistent with those of previous studies (46, 47), possibly because the population included in our study was older, potentially resulting in selection bias. Further, our follow-up period was short, which may have affected survival outcomes.
This study had several limitations. First, selection bias may have occurred because the study was conducted in a single center. Our data show that the prevalence of frailty was 90%; the main reason for this is that our hospital is a national geriatric center, and most patients admitted to the hospital have multiple comorbidities, leading to a greater proportion of patients with high frailty scores. Second, although we internally validated the predictive value of sFI, our findings were not externally validated using an independent dataset. In addition, this study is limited by the limitations of the recording variables. Finally, physical and environmental factors can affect the prognosis of elderly patients with cancer. Therefore, it is essential to account for additional confounding factors that may influence patient outcomes.
5 Conclusion
Overall, our data demonstrate an association between frailty and RNU outcomes. sFI represents a potential predictor of procedure-related complications and prognosis in older patients with UTUC patients. Moreover, the nomogram developed in this study incorporates sFI and clinical risk factors to effectively predict OS and CSS in elderly patients with UTUC following RNU.
Data availability statement
The original contributions presented in the study are included in the article/Supplementary Material. Further inquiries can be directed to the corresponding authors.
Author contributions
JYL: Project development, Data collection, Data analysis, Manuscript writing. HRW: Project development, Data collection, Data analysis, Manuscript writing. PJW: Data collection, Data analysis, Manuscript editing. JWW: Data collection, Data analysis. JYW: Data analysis, Manuscript editing. HMH: Project development, Data analysis, Manuscript editing. JLW: Project development, Data analysis, Manuscript editing. YGZ: Project development, Data analysis, Manuscript editing. All authors contributed to the article and approved the submitted version.
Funding
The authors declare financial support was received for the research, authorship, and/or publication of this article. The study was funded by National Key R&D Program of China (Grant Numbers: 2018YFC2002202), the Chinese Academy of Medical Sciences (Grant Numbers: BJ-2022-237), and National High Level Hospital Clinical Research Funding (Grant Numbers: BJ-2022-143).
Conflict of interest
The authors declare that the research was conducted in the absence of any commercial or financial relationships that could be construed as a potential conflict of interest.
Publisher’s note
All claims expressed in this article are solely those of the authors and do not necessarily represent those of their affiliated organizations, or those of the publisher, the editors and the reviewers. Any product that may be evaluated in this article, or claim that may be made by its manufacturer, is not guaranteed or endorsed by the publisher.
Supplementary material
The Supplementary Material for this article can be found online at: https://www.frontiersin.org/articles/10.3389/fonc.2023.1187677/full#supplementary-material
Supplementary Table 1 | Length of Stay and readmission by groups of sFIFigure captions.
References
1. Rouprêt M, Babjuk M, Burger M, Capoun O, Cohen D, Compérat EM, et al. European association of urology guidelines on upper urinary tract urothelial carcinoma: 2020 update. Eur Urol (2021) 79(1):62–79. doi: 10.1016/j.eururo.2020.05.042
2. Soria F, Shariat SF, Lerner SP, Fritsche H-M, Rink M, Kassouf W, et al. Epidemiology, diagnosis, preoperative evaluation and prognostic assessment of upper-tract urothelial carcinoma (UTUC). World J Urol (2017) 35(3):379–87. doi: 10.1007/s00345-016-1928-x
3. Siegel RL, Miller KD, Wagle NS, Jemal A. Cancer statistics, 2023. CA: Cancer J For Clin (2023) 73(1):17–48. doi: 10.3322/caac.21763
4. Birtle A, Johnson M, Chester J, Jones R, Dolling D, Bryan RT, et al. Adjuvant chemotherapy in upper tract urothelial carcinoma (the POUT tr ial): a phase 3, open-label, randomised controlled trial. Lancet (2020) 395(10232):1268–77. doi: 10.1016/S0140-6736(20)30415-3
5. Aucoin SD, Hao M, Sohi R, Shaw J, Bentov I, Walker D, et al. Accuracy and Feasibility of Clinically Applied Frailty Instruments bef ore Surgery: A Systematic Review and Meta-analysis. Anesthesiology (2020) 133(1):78–95. doi: 10.1097/ALN.0000000000003257
6. Fried LP, Tangen CM, Walston J, Newman AB, Hirsch C, Gottdiener J, et al. Frailty in older adults: evidence for a phenotype. J Gerontol A Biol Sci Med Sci (2001) 56(3):M146–56. doi: 10.1093/gerona/56.3.M146
7. Rockwood K, Song X, MacKnight C, Bergman H, Hogan DB, McDowell I, et al. A global clinical measure of fitness and frailty in elderly people. CMAJ (2005) 173(5):489–95. doi: 10.1503/cmaj.050051
8. Shahrokni A, Tin A, Alexander K, Sarraf S, Afonso A, Filippova O, et al. Development and evaluation of a new frailty index for older surgical patients with cancer. JAMA Network Open (2019) 2(5):e193545. doi: 10.1001/jamanetworkopen.2019.3545
9. Sathianathen NJ, Jarosek S, Lawrentschuk N, Bolton D, Konety BR. A simplified frailty index to predict outcomes after radical cystectomy. Eur Urol Focus (2019) 5(4):658–63. doi: 10.1016/j.euf.2017.12.011
10. Rothenberg KA, Stern JR, George EL, Trickey AW, Morris AM, Hall DE, et al. Association of frailty and postoperative complications with unplanned readmissions after elective outpatient surgery. JAMA Network Open (2019) 2(5). doi: 10.1001/jamanetworkopen.2019.4330
11. Wang Y, Zheng Y, Wen Z, Zhou Y, Wang Y, Huang Z. Effects of frailty on patients undergoing head and neck cancer surgery with flap reconstruction: a retrospective analysis. BMJ Open (2022) 12(12). doi: 10.1136/bmjopen-2022-062047
12. Han B, Li Q, Chen X. Frailty and postoperative complications in older Chinese adults undergoing major thoracic and abdominal surgery. Clin Interventions Aging (2019) 14:947–57. doi: 10.2147/CIA.S201062
13. Goldstein DP, Sklar MC, Almeida JR, Gilbert R, Gullane P, Irish J, et al. Frailty as a predictor of outcomes in patients undergoing head and neck cancer surgery. Laryngoscope (2019) 130(5):E340–5. doi: 10.1002/lary.28222
14. Lal S, Gray A, Kim E, Bunton RW, Davis P, Galvin IF, et al. Frailty in elderly patients undergoing cardiac surgery increases hospital stay and 12-month readmission rate. Heart Lung Circ (2020) 29(8):1187–94. doi: 10.1016/j.hlc.2019.10.007
15. Gu C, Lu A, Lei C, Wu Q, Zhang X, Wei M, et al. Frailty index is useful for predicting postoperative morbidity in older patients undergoing gastrointestinal surgery: a prospective cohort study. BMC Surg (2022) 22(1). doi: 10.1186/s12893-022-01471-9
16. Kundi H, Wadhera RK, Strom JB, Valsdottir LR, Shen C, Kazi DS, et al. Association of frailty with 30-day outcomes for acute myocardial infarction, heart failure, and pneumonia among elderly adults. JAMA Cardiol (2019) 4(11):1084–91. doi: 10.1001/jamacardio.2019.3511
17. Solomon DH. Geriatric assessment: methods for clinical decision making. JAMA (1988) 259(16):2450–2. doi: 10.1001/jama.1988.03720160070033
18. Velanovich V, Antoine H, Swartz A, Peters D, Rubinfeld I. Accumulating deficits model of frailty and postoperative mortality and morbidity: its application to a national database. J Surg Res (2013) 183(1):104–10. doi: 10.1016/j.jss.2013.01.021
19. Obeid NM, Azuh O, Reddy S, Webb S, Reickert C, Velanovich V, et al. Predictors of critical care-related complications in colectomy patients using the National Surgical Quality Improvement Program: exploring frailty and aggressive laparoscopic approaches. J Trauma Acute Care Surg (2012) 72(4):878–83. doi: 10.1097/TA.0b013e31824d0f70
20. Pizzonia M, Giannotti C, Carmisciano L, Signori A, Rosa G, Santolini F, et al. Frailty assessment, hip fracture and long-term clinical outcomes in older adults. Eur J Clin Invest (2021) 51(4):e13445. doi: 10.1111/eci.13445
21. Traven SA, Reeves RA, Sekar MG, Slone HS, Walton ZJ. New 5-factor modified frailty index predicts morbidity and mortality in primary hip and knee arthroplasty. J Arthroplasty (2019) 34(1):140–4. doi: 10.1016/j.arth.2018.09.040
22. Pierce KE, Naessig S, Kummer N, Larsen K, Ahmad W, Passfall L, et al. The five-item modified frailty index is predictive of 30-day postoperative complications in patients undergoing spine surgery. Spine (Phila Pa 1976) (2021) 46(14):939–43. doi: 1097/BRS.0000000000003936
23. Yamashita S, Mashima N, Higuchi M, Matsumura N, Hagino K, Kikkawa K, et al. Modified 5-item frailty index score as prognostic marker after radical cystectomy in bladder cancer. Clin Genitourin Cancer (2022) 20(3):e210–6. doi: 10.1016/j.clgc.2021.12.016
24. Mah SJ, Anpalagan T, Marcucci M, Eiriksson L, Reade CJ, Jimenez W, et al. The five-factor modified frailty index predicts adverse postoperative and chemotherapy outcomes in gynecologic oncology. Gynecol Oncol (2022) 166(1):154–61. doi: 10.1016/j.ygyno.2022.05.012
25. Magno-Pardon DA, Luo J, Carter GC, Agarwal JP, Kwok AC. An analysis of the modified five-item frailty index for predicting complications following free flap breast reconstruction. Plast Reconstr Surg (2022) 149(1):41–7. doi: 10.1097/PRS.0000000000008634
26. Balasundaram N, Kanake S, Thaghalli Sunil Kumar V, Chandra I, Schlesselman C, Vogel TR. Modified frailty index as an indicator for outcomes after lower extremity endovascular revascularization. Surgery (2023) 173(3):837–45. doi: 10.1016/j.surg.2022.09.018
27. Liu H, Akhavan A, Ibelli T, Alerte E, Etigunta S, Kuruvilla A, et al. Using the modified frailty index to predict complications in breast reduction: A national surgical quality improvement program study of 14,160 cases. Aesthet Surg J (2022) 42(8):890–9. doi: 10.1093/asj/sjac059
28. Wilson JM, Schwartz AM, Farley KX, Bradbury TL, Guild GN. Combined malnutrition and frailty significantly increases complications and mortality in patients undergoing elective total hip arthroplasty. J Arthroplasty (2020) 35(9):2488–94. doi: 10.1016/j.arth.2020.04.028
29. Vu CCL, Runner RP, Reisman WM, Schenker ML. The frail fail: Increased mortality and post-operative complications in orthopaedic trauma patients. Injury (2017) 48(11):2443–50. doi: 10.1016/j.injury.2017.08.026
30. Yang H, Tian J, Meng B, Wang K, Zheng C, Liu Y, et al. Application of extreme learning machine in the survival analysis of chronic heart failure patients with high percentage of censored survival time. Front Cardiovasc Med (2021) 8:726516. doi: 10.3389/fcvm.2021.726516
31. Kwak S, Everett RJ, Treibel TA, Yang S, Hwang D, Ko T, et al. Markers of myocardial damage predict mortality in patients with aortic stenosis. J Am Coll Cardiol (2021) 78(6):545–58. doi: 10.1016/j.jacc.2021.05.047
32. Kong J, Ha D, Lee J, Kim I, Park M, Im S-H, et al. Network-based machine learning approach to predict immunotherapy response in cancer patients. Nat Commun (2022) 13(1):3703. doi: 10.1038/s41467-022-31535-6
33. Sammut S-J, Crispin-Ortuzar M, Chin S-F, Provenzano E, Bardwell HA, Ma W, et al. Multi-omic machine learning predictor of breast cancer therapy response. Nature (2022) 601(7894):623–9. doi: 10.1038/s41586-021-04278-5
34. Katz M, Wollin DA, Donin NM, Meeks W, Gulig S, Zhao LC, et al. Effect of malnutrition on radical nephroureterectomy morbidity and mortality: opportunity for preoperative optimization. Clin Genitourin Cancer (2018) 16(4):e807–15. doi: 10.1016/j.clgc.2018.02.012
35. Liu Z, Wu H, Liufu N, Cheng S, Huang H, Hu C, et al. Development and validation of a nomogram incorporating selected systemic inflammation-based prognostic marker for complication prediction after vascularized fibula flap reconstruction. Oral Oncol (2019) 99:104467. doi: 10.1016/j.oraloncology.2019.104467
36. Fedarko NS. The biology of aging and frailty. Clinics Geriatric Med (2011) 27(1):27–37. doi: 10.1016/j.cger.2010.08.006
37. Morley JE, Vellas B, Abellan van Kan G, Anker SD, Bauer JM, Bernabei R, et al. Frailty consensus: A call to action. J Am Med Directors Assoc (2013) 14(6):392–7. doi: 10.1016/j.jamda.2013.03.022
38. Tsiouris A, Hammoud ZT, Velanovich V, Hodari A, Borgi J, Rubinfeld I. A modified frailty index to assess morbidity and mortality after lobectomy. J Surg Res (2013) 183(1):40–6. doi: 10.1016/j.jss.2012.11.059
39. Dicpinigaitis AJ, Al-Mufti F, Bempong PO, Kazim SF, Cooper JB, Dominguez JF, et al. Prognostic significance of baseline frailty status in traumatic spinal cord injury. Neurosurgery (2022) 91(4):575–82. doi: 10.1227/neu.0000000000002088
40. Heimann M, Schäfer N, Bode C, Borger V, Eichhorn L, Giordano FA, et al. Outcome of elderly patients with surgically treated brain metastases. Front Oncol (2021) 11:713965. doi: 10.3389/fonc.2021.713965
41. Banaszek D, Inglis T, Marion TE, Charest-Morin R, Moskven E, Rivers CS, et al. Effect of frailty on outcome after traumatic spinal cord injury. J Neurotrauma (2020) 37(6):839–45. doi: 10.1089/neu.2019.6581
42. Subramaniam S, Aalberg JJ, Soriano RP, Divino CM. New 5-factor modified frailty index using american college of surgeons NSQIP data. J Am Coll Surg (2018) 226(2):173–81. doi: 10.1016/j.jamcollsurg.2017.11.005
43. Abdelfatah E, Ramos-Santillan V, Cherkassky L, Cianchetti K, Mann G. High risk, high reward: frailty in colorectal cancer surgery is associated with worse postoperative outcomes but equivalent long-term oncologic outcomes. Ann Surg Oncol (2023) 30(4):2035–45. doi: 10.1245/s10434-022-12970-7
44. Velissaris D, Pantzaris N, Koniari I, Koutsogiannis N, Karamouzos V, Kotroni I, et al. C-reactive protein and frailty in the elderly: A literature review. J Clin Med Res (2017) 9(6):461–5. doi: 10.14740/jocmr2959w
45. Yamamichi T, Ichinose J, Iwamoto N, Omura K, Ozawa H, Kondo Y, et al. Correlation between smoking status and short-term outcome of thoracoscopic surgery for lung cancer. Ann Thorac Surg (2022) 113(2):459–65. doi: 10.1016/j.athoracsur.2021.01.063
46. Tellini R, Mari A, Muto G, Cacciamani GE, Ferro M, Stangl-Kremser J, et al. Impact of smoking habit on perioperative morbidity in patients treated with radical cystectomy for urothelial bladder cancer: A systematic review and meta-analysis. Eur Urol Oncol (2021) 4(4):580–93. doi: 10.1016/j.euo.2020.10.006
Keywords: upper urinary tract urothelial carcinoma, frailty, postoperative complication, prognosis, risk stratification, random forest
Citation: Liu J, Wang H, Wu P, Wang J, Wang J, Hou H, Wang J and Zhang Y (2023) A simplified frailty index and nomogram to predict the postoperative complications and survival in older patients with upper urinary tract urothelial carcinoma. Front. Oncol. 13:1187677. doi: 10.3389/fonc.2023.1187677
Received: 17 March 2023; Accepted: 07 September 2023;
Published: 11 October 2023.
Edited by:
Kenneth L. Seldeen, University at Buffalo, United StatesReviewed by:
Riccardo Tellini, Careggi University Hospital, ItalyEihab Abdelfatah, New York University, United States
Copyright © 2023 Liu, Wang, Wu, Wang, Wang, Hou, Wang and Zhang. This is an open-access article distributed under the terms of the Creative Commons Attribution License (CC BY). The use, distribution or reproduction in other forums is permitted, provided the original author(s) and the copyright owner(s) are credited and that the original publication in this journal is cited, in accordance with accepted academic practice. No use, distribution or reproduction is permitted which does not comply with these terms.
*Correspondence: Huimin Hou, aG91aHVpbWluMDMwNUAxNjMuY29t; Jianlong Wang, d2psc3BwbGFhYUBzaW5hLmNvbQ==; Yaoguang Zhang, emhhbmd5YW9ndWFuZzMyNDdAYmpobW9oLmNu
†These authors have contributed equally to this work and share the first authorship
‡These authors have contributed equally to this work and share the last authorship