- 1Division of Clinical Pharmacology, Department of Medicine, Indiana University School of Medicine, Indianapolis, IN, United States
- 2Division of Diagnostic Genetics and Genomics, Department of Medical and Molecular Genetics, Indiana University School of Medicine, Indianapolis, IN, United States
- 3Division of Hematology/Oncology, Department of Medicine, Indiana University School of Medicine, Indianapolis, IN, United States
- 4Center for Cancer Research, National Cancer Institute, National Institute of Health, Bethesda, MD, United States
- 5Department of Computer Science, University of Victoria, Victoria, BC, Canada
- 6LifeOmic Inc., Indianapolis, IN, United States
Background: Next-generation sequencing (NGS), including whole genome sequencing (WGS) and whole exome sequencing (WES), is increasingly being used for clinic care. While NGS data have the potential to be repurposed to support clinical pharmacogenomics (PGx), current computational approaches have not been widely validated using clinical data. In this study, we assessed the accuracy of the Aldy computational method to extract PGx genotypes from WGS and WES data for 14 and 13 major pharmacogenes, respectively.
Methods: Germline DNA was isolated from whole blood samples collected for 264 patients seen at our institutional molecular solid tumor board. DNA was used for panel-based genotyping within our institutional Clinical Laboratory Improvement Amendments- (CLIA-) certified PGx laboratory. DNA was also sent to other CLIA-certified commercial laboratories for clinical WGS or WES. Aldy v3.3 and v4.4 were used to extract PGx genotypes from these NGS data, and results were compared to the panel-based genotyping reference standard that contained 45 star allele-defining variants within CYP2B6, CYP2C8, CYP2C9, CYP2C19, CYP2D6, CYP3A4, CYP3A5, CYP4F2, DPYD, G6PD, NUDT15, SLCO1B1, TPMT, and VKORC1.
Results: Mean WGS read depth was >30x for all variant regions except for G6PD (average read depth was 29 reads), and mean WES read depth was >30x for all variant regions. For 94 patients with WGS, Aldy v3.3 diplotype calls were concordant with those from the genotyping reference standard in 99.5% of cases when excluding diplotypes with additional major star alleles not tested by targeted genotyping, ambiguous phasing, and CYP2D6 hybrid alleles. Aldy v3.3 identified 15 additional clinically actionable star alleles not covered by genotyping within CYP2B6, CYP2C19, DPYD, SLCO1B1, and NUDT15. Within the WGS cohort, Aldy v4.4 diplotype calls were concordant with those from genotyping in 99.7% of cases. When excluding patients with CYP2D6 copy number variation, all Aldy v4.4 diplotype calls except for one CYP3A4 diplotype call were concordant with genotyping for 161 patients in the WES cohort.
Conclusion: Aldy v3.3 and v4.4 called diplotypes for major pharmacogenes from clinical WES and WGS data with >99% accuracy. These findings support the use of Aldy to repurpose clinical NGS data to inform clinical PGx.
Introduction
Short-read next-generation sequencing (NGS) has emerged as a valuable tool to support clinical diagnosis and decision making in a variety of therapeutic disciplines (1–4). Clinical NGS frequently utilizes whole genome approaches (i.e., whole exome sequencing [WES] or whole genome sequencing [WGS]) that sequence gene regions across the genome. As a result, clinical NGS data contain a wealth of genetic information with relevance for pharmacogenetics (PGx), the practice of using genetic information to guide safe and effective medication use (5, 6).
Recently, computational methods have been developed to extract PGx-relevant genotypes, including star alleles for cytochrome P450 (CYP) enzymes, from NGS data without requiring additional genetic testing (7–13). These methods have primarily been validated using genetic reference material (e.g., cell lines from the Genetic Testing Materials Coordination Program [GeT-RM]) (14–16); however, in order to be suitable for clinical implementation, it is critical to verify the accuracy of computational genotype extraction methods using clinical NGS data derived from patient samples. Clinical NGS data can present numerous challenges that limit precise genotype determination, including low read depth, incomplete coverage of PGx-relevant loci, the inability to phase variants, and difficulty resolving large-scale structural variations (17). Computational genotyping methods have been iteratively developed towards the goal of overcoming these challenges, but their ability to accurately extract genotypes from clinical NGS data remains largely unestablished.
We previously assessed the performance of the computational genotyping software Aldy (version 3.3) using clinical WES (17). We found that Aldy v3.3 genotype calls were concordant with those from our validated genotyping reference standard for 13 major pharmacogenes; however, we noted important limitations of Aldy v3.3 that included the inability to determine CYP2D6 copy number or to phase clinically relevant, multi-variant alleles in CYP2B6, CYP2D6, and TPMT. To date, we are not aware of any study assessing the accuracy of computational methods for extracting pharmacogenotypes from clinical WGS data. Therefore, the primary objective of this study was to assess the accuracy of Aldy v.3.3 calls for 14 major pharmacogenes using WGS files from patients (n=100) seen at our institutional molecular solid tumor board. Since a new Aldy version (v4.4) was recently released to support improved copy number calling, variant calling, and phasing (18), we also assessed the performance of Aldy v4.4 on the WGS cohort and on the patient cohort (n=161) from our past WES validation.
Materials and methods
Patient enrollment
Written informed consent to participate in the study was obtained from patients with solid tumors treated at the Indiana University Precision Genomics Clinic (Indianapolis, IN) from September 2017 to March 2020. Patients consented to provide whole blood samples for genomic analyses, which consisted of (1) pharmacogenetics genotyping performed at the Indiana University Pharmacogenomics Laboratory and (2) WGS performed at NantOmics or WES performed at Ashion Analytics. The study enrolled a total of 100 patients with WGS and 164 patients with WES. The study protocol and the parent Indiana University Total Cancer Care Protocol were approved by the Indiana University Institutional Review Board. Patient demographic and clinical data, including age, sex, race/ethnicity, and date and type of first cancer diagnosis, were obtained from a Total Cancer Care Protocol study database.
Panel-based genotyping and orthogonal confirmation of additional variants identified by Aldy
We have previously described our panel-based genotyping method and CYP2D6 copy number testing in detail (17, 19). To summarize, genotyping was performed at the Clinical Laboratory Improvement Amendments (CLIA)-certified, College of American Pathologists (CAP)-accredited Indiana University Pharmacogenomics Laboratory using a validated, custom-designed OpenArray® platform (ThermoFisher, Waltham, MA). The genes included on the genotyping platform were CYP2B6, CYP2C8, CYP2C9, CYP2C19, CYP2D6 (including copy number), CYP3A4, CYP3A5, CYP4F2, DPYD, G6PD, SLCO1B1, TPMT, and VKORC1. The complete list of the 45 variants on the genotyping platform are shown in Supplemental Table 1. NUDT15 was added to the genotyping platform after genotyping was completed for the WGS cohort, necessitating orthogonal confirmation for any NUDT15 variant called by Aldy in the WGS cohort. Patients whose DNA sample passed clinical laboratory quality control (all 161 patients in the WES cohort and 94/100 patients in the WGS cohort) were genotyped.
Orthogonal confirmation was performed for any variant in the 14 assessed pharmacogenes that was detected by Aldy, not included in the genotyping platform, and had actionable recommendations in current Clinical Pharmacogenetics Implementation Consortium (CPIC) guidelines. Detailed descriptions of these orthogonal confirmation methods have been previously published (17). To summarize, these variants were confirmed with PCR-based genotyping using commercial TaqMan reagents, when available, and a positive genomic control from a Coriell cell line with known genotypes from 1000 Genomes for TaqMan allelic discrimination. All other variants were confirmed using Sanger sequencing methods, with primers for each variant being designed using the National Center for Biotechnology Information Primer-Blast tool (https://www.ncbi.nlm.nih.gov/tools/primer-blast) and synthesized by Integrated DNA Technologies, Inc. The assay identification numbers of the TaqMan reagents and the sequences of the Sanger sequencing primers are provided in Supplemental Table 2.
Next-generation sequencing
Whole blood samples were sent to the following CLIA-certified, CAP-accredited clinical laboratories for next-generation sequencing: NantOmics Laboratory (Culver City, CA) for WGS or Ashion Analytics Laboratory (Phoenix, AZ) for WES. Germline NGS was performed as part of each laboratory’s commercial paired normal/tumor sequencing workflow, available as GPS Cancer™ from NantOmics and as Genomic Enabled Medicine Exome Testing (GEM ExTra) from Ashion Analytics. NGS methods for GPS Cancer™ and GEM ExTra have been previously published (20, 21). Briefly, germline DNA was isolated from whole blood samples, and DNA sequencing libraries were prepared using a custom xGEN target capture kit (GEM ExTra) or a KAPA Hyper prep kit (GPS Cancer™). Libraries were sequenced using Illumina sequencing platforms, and sequencing data were analyzed using proprietary bioinformatics workflows. Sequencing reads were aligned to the Genome Reference Consortium Human Build 37 (GRCh37)/Human Genome version 19 (hg19). Binary alignment map (BAM) and variant calling files (VCFs) were returned from each laboratory and transferred into the Precision Health Cloud (LifeOmic, Indianapolis, IN) for analysis.
Analysis of next-generation sequencing data with Aldy and Cyrius
The Aldy and Cyrius genotype extraction methods have been described in detail in previous publications (9, 13, 18). Aldy v3.3 and v4.4 were downloaded via pypi packages (links available on the Aldy Github: https://github.com/0xTCG/aldy), and the Cyrius v1.1.1 source code was downloaded from the Cyrius Github (https://github.com/Illumina/Cyrius/releases/tag/v1.1.1). All callers were operationalized in the LifeOmic Precision Health Cloud using JupyterLab notebooks. Read depth was analyzed from NantOmics WGS BAM files for all variants included in the genotyping platform using Samtools and outputted in the.csv file format for analysis. The same method was previously used to analyze read depth from Ashion WES BAM files (17). Aldy performed genotype extraction for the major pharmacogenes contained on the genotyping platform, which included 14 genes from WGS BAM files and 13 genes from WES BAM files (VKORC1 was not assessed using WES BAMs since the rs9923231 promoter variant was not covered). The “WXS” and “Illumina” sequencing profiles were used for Aldy genotype extraction from WES data and WGS data, respectively. All other default Aldy parameters were used. Aldy output files, which were outputted in the.txt file format for analysis, included the list of variants detected and the assembled diplotype call. Cyrius was used to perform genotype extraction for the four BAM files that had discordant CYP2D6 genotype calls with Aldy v3.3 or v4.4 (or both) relative to the genotyping reference standard.
Analytical assessment of Aldy
The accuracy of Aldy calls was assessed at the variant and diplotype levels via comparison to clinical laboratory genotype results or results from orthogonal methods. Diplotypes called by Aldy with ambiguous phasing or with hybrid alleles calls containing CYP2D6 and CYP2D7 (herein referred to as “CYP2D6 hybrid alleles”) were excluded from the diplotype concordance analysis. Standard analytical parameters for diagnostic testing, including analytical sensitivity and specificity, intra- and inter-assay concordance, and overall accuracy, were assessed for each PGx-relevant variant in our cohort possessed by ≥1 study patient. Additional star alleles identified by Aldy were deemed “clinically actionable” if they had clinical function annotations other than “normal function” by the Pharmacogene Variation Consortium (PharmVar) and PharmGKB (22, 23).
Determination of clinical impact of additional star alleles identified by Aldy
For patients with additional clinically actionable star alleles identified by Aldy (that were able to be unambiguously phased), medication data was obtained since each patient’s respective date of first cancer diagnosis via query of the Indiana Health Information Exchange, a statewide electronic health record data repository, as previously described (19). Patient pharmacogene phenotypes were categorized based on current diplotype to phenotype translation tables curated by PharmGKB and CPIC (22). Patient medications were considered actionable if current CPIC guidelines provide “strong” or “moderate” recommendations to alter therapy with the medication (i.e., prescribe an alternative medication, adjust the dose, increase monitoring, or perform additional functional testing) based on each patient’s Aldy-predicted phenotype (relative to their phenotype as predicted by the genotyping reference standard) (24). Medications that were considered actionable for each patient are summarized in Supplemental Table 3.
Results
Patient population
For comparison to the genotyping reference standard, Aldy calls were extracted from clinical NGS data in a cohort of 264 patients, which consisted of 100 patients with clinical WGS and 164 patients with clinical WES. The demographic and clinical characteristics of the overall study population are presented in Table 1, with characteristics for the WGS cohort provided in Supplemental Table 4. Demographic and clinical characteristics for the WES cohort have been previously published (17). Within the overall study population, the median age at first cancer diagnosis was 56 years old (interquartile range: 16). Our population was 53% male, 85% white race, and 99% non-Hispanic ethnicity. The most frequent primary cancer diagnoses in our population were breast (15%), colorectal (12%), pancreatic (11%), prostate (10%), and soft tissue sarcoma (7%).
Coverage of pharmacogenetic variants by next-generation sequencing
Read depth analysis was performed to confirm that the chromosomal positions for the 45 variants included on our genotyping panel were sufficiently covered within our NGS data. The average read depth at each position for the WGS cohort is shown in Supplemental Table 5, and the average read depth for the WES cohort has been previously published (17). Within the WGS cohort, all PGx-relevant loci had an average read depth above the minimum recommended WGS coverage threshold of 30 reads except for the G6PD “A” and c.202G>A “A-” alleles, which had an average read depth of 29 ± 15 (mean ± standard deviation) reads (25). Within the WES cohort, all PGx loci had an average read depth above 100 reads except for CYP3A4*22, which had a read depth of 44 ± 12 reads. These findings confirmed that all PGx loci were adequately covered in the NGS data to proceed with the analytical assessment of the Aldy method.
Analytical assessment of Aldy v3.3 using whole genome sequencing
An analysis validating Aldy v3.3 genotyping calls within the WES cohort has been previously published (17). For 94 patients in the WGS cohort, Aldy v3.3 calls were compared to the genotyping reference standard at both the variant and diplotype levels, with results shown in Tables 2, 3, respectively. Aldy v3.3 calls were concordant with the reference standard for all assessed variants within CYP2C8, CYP2C9, CYP2C19, CYP3A5, CYP4F2, DPYD, G6PD, NUDT15, SLCO1B1, TPMT, and VKORC1, as reflected by point estimates of 100% for analytical sensitivity, analytical specificity, and overall accuracy. The following discordant Aldy v3.3 calls were observed: one CYP2B6*6 (c.516G>T) call; two CYP2D6*2 (c.886C>T) calls; two CYP2D6*2 (c.1457G>C) calls; and one CYP3A4*22 (c.522-191C>T) call. Interrogation of the WGS data revealed that five of these heterozygous variants had variant allele frequencies just outside of the 25-75% threshold used by Aldy v3.3 to classify variants as heterozygous in diploid genotype calls, which explains the discordant results. Aldy v3.3’s intra- and inter-assay concordance, defined as the ability to reproduce the same call for the same sequencing file within the same and subsequent runs, respectively, were confirmed to be 100% for all assessed variants. Stemming from the variant level results, five diplotype calls (WGS16 in CYP2B6, WGS5, WGS35, and WGS55 in CYP2D6, and WGS73 in CYP3A4) were discordant. Aldy v3.3 identified additional alleles not covered by the genotyping reference standard for 196 diplotype calls, which included 15 clinically actionable alleles within CYP2B6 (1), CYP2C19 (3), DPYD (8), SLCO1B1 (2), and NUDT15 (1); these additional clinically actionable alleles were confirmed by orthogonal genotyping methods. All other 1,005 diplotype calls were concordant across the 14 assessed genes.
Analytical assessment of Aldy v4.4 using whole exome and whole genome sequencing
Aldy v4.4 diplotype calls were extracted from WES and WGS sequencing files. For three of the WES files, Aldy v4.4 returned a warning that “the average coverage was too low” and did not produce output. Table 4 summarizes Aldy v4.4 diplotype calls compared to the genotyping reference standard for the remaining 161 patients in the WES cohort across the 13 assessed pharmacogenes. With the exception of 23 CYP2D6 diplotypes that were excluded from analysis due to containing copy number variation, all Aldy v4.4 calls were concordant with the reference standard except for one CYP3A4 diplotype call (WES55) in one patient in which WES read depth at the CYP3A4*22 locus was <30. Aldy v4.4 identified additional alleles not covered by the genotyping standard for 385 diplotypes, which included 18 clinically actionable star alleles within CYP2B6 (2), CYP2C19 (1), CYP2D6 (6), DPYD (5), G6PD (1), NUDT15 (1), and SLCO1B1 (2). All additional clinically actionable alleles were orthogonally confirmed during this analysis or during our previous WES validation (17).
As shown in Table 5, Aldy v4.4 diplotype calls from WGS data were concordant across all genes except for one CYP2C9 call (WGS48) and two CYP2D6 calls (WGS53 and 55). The CYP2C9 discordant call is puzzling since Aldy v3.3 produced the correct call, and the WGS variant allele frequency was 71% (Aldy v4.4 called the variant homozygous). Notably, two (WGS5 and 35) of the three discordant CYP2D6 calls from Aldy v3.3 were corrected by v4.4. However, one additional CYP2D6 discordant call (WGS53) by Aldy v4.4 was identified, which consisted of a copy number discrepancy. Cyrius was run on the four BAMs (WGS5, WGS35, WGS53, and WGS55) with discordant CYP2D6 Aldy v3.3 or v4.4 (or both calls), generating an accurate genotype call in all four cases (results shown in Supplemental Table 6). Aldy v4.4 did correct the CYP2B6 call (WGS16) that was miscalled by v3.3. Aldy v4.4 identified 232 additional alleles not covered by targeted genotyping in the WGS cohort, including the same 15 clinically actionable alleles that were previously identified by v3.3. Differences between additional major star alleles identified by Aldv v3.3 and v4.4 are due to new updates to Aldy’s gene databases from PharmVar with Aldy v4.4. The intra- and inter-assay concordance of Aldy v4.4 for all assessed variants was confirmed to be 100% using both WES and WGS data. All diplotype calls from Aldy v4.4, Aldy v3.3, and the genotyping reference standard for the WES and WGS cohorts are provided in Supplemental File 1.
Clinical impact of additional star alleles identified by Aldy
Thirty patients (11.5% of the study population), consisting of 13 and 17 patients from the WGS and WES cohorts, respectively, had additional clinically actionable star alleles conclusively identified by Aldy (two subjects [WGS69 and WES33] had additional clinically actionable CYP2B6 alleles that were unable to be unambiguously phased). Genotype-predicted phenotypes for these patients, as predicted by Aldy results and by results from the genotyping reference standard, are shown in Table 6; in addition, Table 6 summarizes prescriptions for actionable medications (i.e., those with different CPIC recommendations based on Aldy-predicted and genotyping-predicted phenotypes) since each patients’ respective date of first cancer diagnosis. Twenty-one of the 30 patients had medication data available, which included 289 ± 460 (median ± interquartile range) unique prescriptions per patient during the 7.3 ± 7.5 year follow-up period, defined as each patient’s date of first cancer diagnosis until the end of the data collection period. Of these 21 patients, 5 (23.8%) were prescribed a medication which had a different CPIC recommendation based on their Aldy-predicted pharmacogene phenotype.
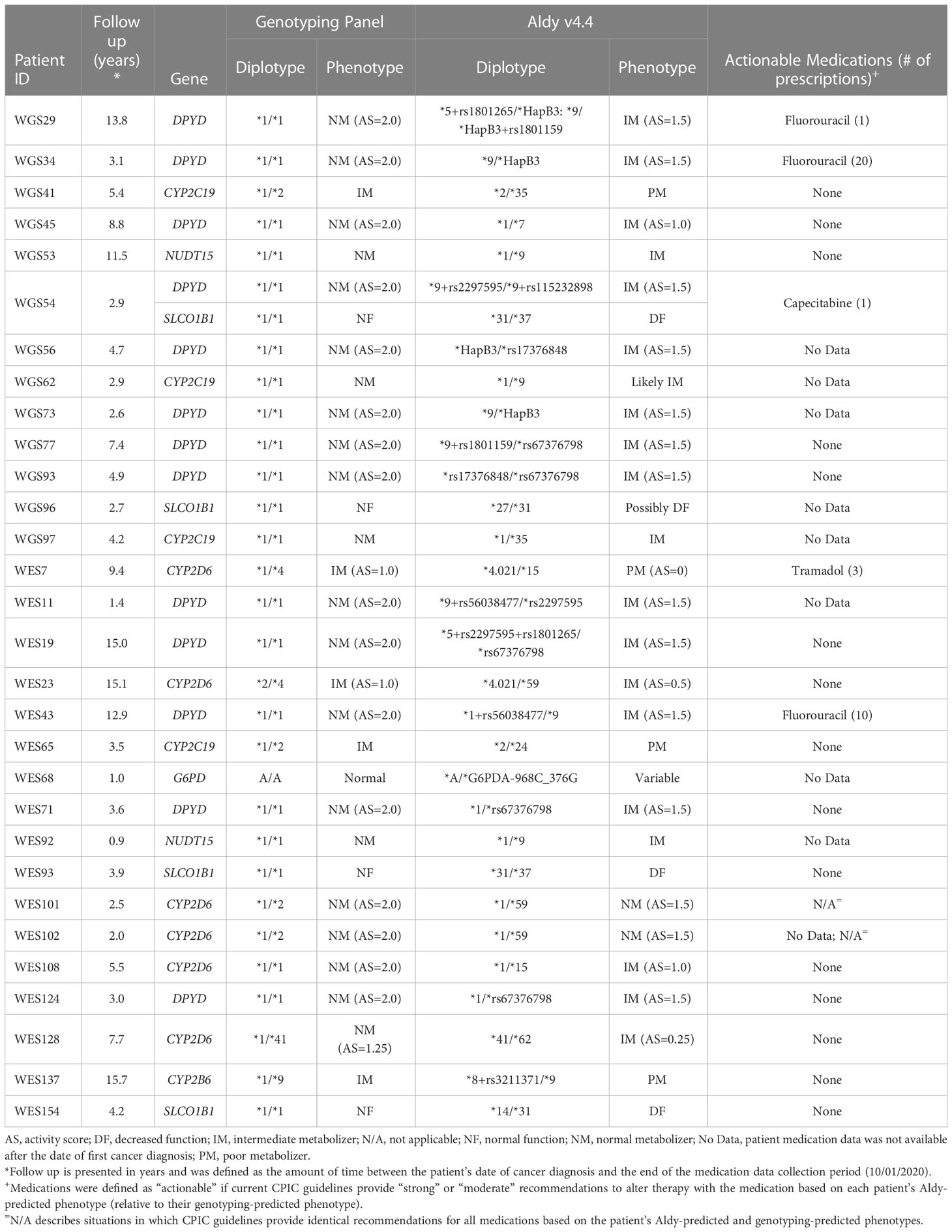
Table 6 Genotype-predicted phenotypes based on genotyping reference standard and Aldy v4.4 results, including whether patients were prescribed actionable medications since their respective dates of first cancer diagnosis.
Discussion
In this investigation, we assessed the performance of the two most recent major versions of the Aldy computational tool (v3.3, released on 07/17/21; v4.4, released on 12/14/22) (26) in extracting PGx genotypes from 255 clinically obtained NGS files for 13-14 major pharmacogenes. Our findings demonstrate that Aldy v3.3 diplotype calls from WGS data were concordant with the genotyping reference standard for 1005 of 1010 calls, yielding an overall accuracy of 99.5%. In a previous investigation, we found that, when excluding genotypes with CYP2D6 copy number variation, Aldy v3.3 diplotype calls from WES data were perfectly concordant with the genotyping reference standard for 736 diplotypes in the validation cohort (17). When testing Aldy v4.4 on these same NGS files, we observed a diplotype call overall accuracy rate of 975/978 (99.7%) in the WGS cohort and 1487/1488 (99.9%) in the WES cohort. In addition, Aldy v3.3 identified additional star alleles not covered by genotyping for 196 diplotype calls, in which 15 diplotypes contained alleles with actionable CPIC recommendations. Within the same WGS cohort, Aldy v4.4 identified additional star alleles for 232 diplotype calls, including the same 15 diplotypes with actionable CPIC recommendations identified by Aldy v3.3. This study demonstrates the benefits of using sequencing data with Aldy-based genotype extraction to identify additional clinically actionable variants not covered by standard genotyping assays, as demonstrated by our findings that 23.8% of patients with additional actionable alleles were prescribed a medication where CPIC guidelines provide different recommendations. However, it is important to also consider potential challenges when identifying and interpreting additional alleles from sequencing data that may not have clinical relevance or defined function.
Past studies have compared the analytical performance of computational genotype extraction tools, including Aldy, Astrolabe, Cyrius, Stargazer, and StellarPGx (12, 13, 18, 27) Although results vary among these analyses, Aldy, Astrolabe, Cyrius and StellarPGx consistently demonstrate >80% accuracy for genotype extraction from WGS for complex CYP2D6 reference samples that include full gene deletions, full gene duplications, and hybrid rearrangements containing CYP2D6 and CYP2D7. Recently, Aldy v4.0 was shown to have an accuracy of >98% across all tested short-read sequencing platforms, including an accuracy of >99% for Illumina WGS (18). Our results expand on these findings to demonstrate Aldy v4.4’s ability to produce >99% accuracy when using clinically obtained NGS data. In comparison to most other platforms, Aldy also offers additional functionalities with clinical utility, including efficient adaptation for use with WES data and the ability to genotype non-CYP enzyme genes with CPIC recommendations, including CFTR, DPYD, G6PD, IFNL3, NUDT15, SLCO1B1, TPMT, UGT1A1, and VKORC1 (24).
No diagnostic test is perfectly accurate. Based on our experience testing Aldy’s performance using clinical NGS data, we observed that the primary source of inaccuracy stemmed from the inherent variability in NGS data, which included modest but technically significant variability in coverage and read depth at PGx-relevant loci and the rare potential for apparent sequencing errors to influence Aldy calls. An essential consideration before running Aldy is to thoroughly characterize the coverage and read depth of the sequencing input. In our analyses, we found that mean NGS coverage >30x for all assessed PGx variants was sufficient for Aldy to perform accurate genotype determination. When using Aldy on WES data, it is critical to determine whether clinically relevant non-coding variants (e.g., CYP2C19*17) are covered by the platform’s target capture method. Given the non-uniform coverage patterns, Aldy cannot determine CYP2D6 copy number from WES data, making it unable to perform CYP2D6 genotyping in accordance with the minimum recommended variants to test by the Association for Molecular Pathology (28). Relative to experimental methods that involve physical reagents prone to degradation or batching imperfections, computational methods offer a theoretical advantage in terms of reproducibility; indeed, the reproducibility of Aldy genotype extraction from WES and WGS was demonstrated to be perfect within our intra- and inter-assay concordance analyses.
Given their efficient workflows, adaptability to multiple genetic data sources, and high accuracy, computational genotyping platforms are emerging as useful tools to enable PGx research (29–32). To our knowledge, these approaches have not yet been widely implemented to support clinical care. When devising strategies to clinically implement Aldy-based NGS genotype extraction at a major academic medical center, we encountered the following two challenges (1): the need to perform orthogonal confirmation for every new clinically actionable variant detected by Aldy and (2) the inability to truly validate Aldy unless the sequencing and bioinformatics workflows are controlled as part of the process. With plans to establish an institutional clinical sequencing laboratory, we ultimately envision clinically validating Aldy to allow us to embed extracted genotypes into the electronic health record to interact with existing clinical decision support modalities (e.g., best practice alerts) and thereby promote genotype-guided prescribing. However, based on this investigation and our previous WES validation (17), we raise a number of considerations to ensure accurate Aldy genotyping when using clinical sequencing data.
Important considerations to ensure accurate Aldy-based genotype extraction from clinical sequencing data:
1. The need to confirm that pharmacogenomic loci of interest are adequately covered (i.e., read depth ≥ 30x), which should include variants defined by the Association for Molecular Pathology as the minimum set to include in PGx genotyping assays for CYP2C9 (33), CYP2C19 (34), CYP2D6 (28), NUDT15, TPMT (35), and VKORC1 (36)
2. Aldy cannot phase potentially ambiguous haplotypes (e.g., TPMT *1/*3A vs. *3B/*3C), which require additional testing, such as phased genetic testing or activity assays, to conclusively resolve
3. Aldy will only call variants included in its gene databases (available online at: https://github.com/0xTCG/aldy/tree/master/aldy/resources/genes) and will not identify novel variants
4. Aldy cannot determine CYP2D6 copy number from WES, which requires additional genetic testing
5. Aldy’s ability to accurately call hybrid alleles containing CYP2D6 and CYP2D7 from clinical sequencing data has not been confirmed and requires validation using an appropriate orthogonal platform (e.g., long-read sequencing)
We acknowledge the following limitations of our investigation. For variants not included within the panel-based genotyping platform, orthogonal confirmation methods were only performed for samples identified by Aldy as having the relevant variants. As a result, we were only able to assess the analytical sensitivity of Aldy in detecting these variants and not the analytical specificity. Additionally, our assessments were confined to the variants that were detected in our moderately sized, predominantly White study population. Therefore, clinically actionable pharmacogene variants that are relatively rare or are found in other racial/ethnic groups were not able to be assessed. We also did not confirm Aldy’s performance in calling hybrid alleles containing CYP2D6 and CYP2D7 from WGS, which would require extensive characterization using multiple testing platforms including additional sequencing and CYP2D6 copy number testing. However, consideration of Aldy for clinical CYP2D6 testing from WGS would require an extensive validation of Aldy’s ability to accurately call complex CYP2D6 structural variation and confirmation using appropriate reference materials and patient samples. Finally, we did not attempt to assess UGT1A1, which is supported by Aldy and included in CPIC recommendations to guide atazanavir therapy (37), because it is not included on our genotyping panel due to atazanavir not being widely prescribed at our institution.
In conclusion, our findings demonstrate Aldy’s ability to extract pharmacogenotypes from clinical WES and WGS data with high accuracy. Future work is needed to further optimize Aldy-based genotyping and determine optimal strategies to implement Aldy-based genotype extraction from clinical NGS data to inform genotype-guided prescribing.
Data availability statement
The genomic sequencing data presented in this article cannot be deposited into a public database due to ethical approval restrictions on sharing the data publicly. Requests to access the datasets should be directed to TCS (tskaar@iu.edu).
Ethics statement
The studies involving human participants were reviewed and approved by Indiana University Institutional Review Board. The patients/participants provided their written informed consent to participate in this study.
Author contributions
TS, RL, WO, ER, CG, TL, EM, and RR analyzed the data. TS, RL, JH, AB, BPS, SS, IN, BAS, SB, RR, and TCS contributed to the conception and design of the study. TS and RL wrote the manuscript. All authors contributed to the article and approved the submitted version.
Funding
This work was funded by the Indiana University Grand Challenge to support the institutional Precision Health Initiative (TS, RL, WO, ER, BPS, and TCS), K23GM147805 (TS), NSERC Discovery RGPIN-04973 (IN), Canada Research Chairs Program (IN) and R35GM131812 (TCS).
Conflict of interest
Authors JH and AB are laboratory directors of The Indiana University School of Medicine Pharmacogenomics Laboratory, a fee-for-service laboratory that offers clinical pharmacogenetic testing. Authors SS and IN have a patent US 20200168298A1 for the Aldy genotyping platform. Authors RR, BAS, and SB are current or former employees of the company LifeOmic Inc., a commercial entity that supports management and analysis of genetic data.
The remaining authors declare that the research was conducted in the absence of any commercial or financial relationships that could be construed as a potential conflict of interest.
Publisher’s note
All claims expressed in this article are solely those of the authors and do not necessarily represent those of their affiliated organizations, or those of the publisher, the editors and the reviewers. Any product that may be evaluated in this article, or claim that may be made by its manufacturer, is not guaranteed or endorsed by the publisher.
Supplementary material
The Supplementary Material for this article can be found online at: https://www.frontiersin.org/articles/10.3389/fonc.2023.1199741/full#supplementary-material
References
1. Seidelmann SB, Smith E, Subrahmanyan L, Dykas D, Abou Ziki MD, Azari B, et al. Application of whole exome sequencing in the clinical diagnosis and management of inherited cardiovascular diseases in adults. Circ Cardiovasc Genet (2017) 10(1). doi: 10.1161/circgenetics.116.001573
2. Groopman EE, Marasa M, Cameron-Christie S, Petrovski S, Aggarwal VS, Milo-Rasouly H, et al. Diagnostic utility of exome sequencing for kidney disease. New Engl J Med (2019) 380(2):142–51. doi: 10.1056/NEJMoa1806891
3. Yang Y, Muzny DM, Reid JG, Bainbridge MN, Willis A, Ward PA, et al. Clinical whole-exome sequencing for the diagnosis of mendelian disorders. New Engl J Med (2013) 369(16):1502–11. doi: 10.1056/NEJMoa1306555
4. Huang KL, Mashl RJ, Wu Y, Ritter DI, Wang J, Oh C, et al. Pathogenic germline variants in 10,389 adult cancers. Cell (2018) 173(2):355–370.e14. doi: 10.1016/j.cell.2018.03.039
5. Tafazoli A, Guchelaar HJ, Miltyk W, Kretowski AJ, Swen JJ. Applying next-generation sequencing platforms for pharmacogenomic testing in clinical practice. Front Pharmacol (2021) 12:693453. doi: 10.3389/fphar.2021.693453
6. Relling MV, Klein TE. CPIC: clinical pharmacogenetics implementation consortium of the pharmacogenomics research network. Clin Pharmacol Ther (2011) 89(3):464–7. doi: 10.1038/clpt.2010.279
7. Sangkuhl K, Whirl-Carrillo M, Whaley RM, Woon M, Lavertu A, Lavertu RB, et al. Pharmacogenomics clinical annotation tool (PharmCAT). Clin Pharmacol Ther (2020) 107(1):203–10. doi: 10.1002/cpt.1568
8. Klanderman BJ, Koch C, Machini K, Parpattedar SS, Bandyadka S, Lin CF, et al. Automated pharmacogenomic reports for clinical genome sequencing. J Mol Diagn (2022) 24(3):205–18. doi: 10.1016/j.jmoldx.2021.12.001
9. Numanagić I, Malikić S, Ford M, Qin X, Toji L, Radovich M, et al. Allelic decomposition and exact genotyping of highly polymorphic and structurally variant genes. Nat Commun (2018) 9(1):828. doi: 10.1038/s41467-018-03273-1
10. Lee SB, Wheeler MM, Patterson K, McGee S, Dalton R, Woodahl EL, et al. Stargazer: a software tool for calling star alleles from next-generation sequencing data using CYP2D6 as a model. Genet med: Off J Am Coll Med Genet (2019) 21(2):361–72. doi: 10.1038/s41436-018-0054-0
11. Twist GP, Gaedigk A, Miller NA, Farrow EG, Willig LK, Dinwiddie DL, et al. Constellation: a tool for rapid, automated phenotype assignment of a highly polymorphic pharmacogene, CYP2D6, from whole-genome sequences. NPJ Genom Med (2016) 1:15007. doi: 10.1038/npjgenmed.2015.7
12. Twesigomwe D, Drögemöller BI, Wright GEB, Siddiqui A, da Rocha J, Lombard Z, et al. StellarPGx: a nextflow pipeline for calling star alleles in cytochrome P450 genes. Clin Pharmacol Ther (2021) 110(3):741–9. doi: 10.1002/cpt.2173
13. Chen X, Shen F, Gonzaludo N, Malhotra A, Rogert C, Taft RJ, et al. Cyrius: accurate CYP2D6 genotyping using whole-genome sequencing data. Pharmacogenom J (2021) 21(2):251–61. doi: 10.1038/s41397-020-00205-5
14. Gaedigk A, Boone EC, Scherer SE, Lee SB, Numanagić I, Sahinalp C, et al. CYP2C8, CYP2C9, and CYP2C19 characterization using next-generation sequencing and haplotype analysis: a GeT-RM collaborative project. J Mol Diagn (2022) 24(4):337–50. doi: 10.1016/j.jmoldx.2021.12.011
15. Gaedigk A, Turner A, Everts RE, Scott SA, Aggarwal P, Broeckel U, et al. Characterization of reference materials for genetic testing of CYP2D6 alleles: a GeT-RM collaborative project. J Mol Diagn (2019) 21(6):1034–52. doi: 10.1016/j.jmoldx.2019.06.007
16. Pratt VM, Everts RE, Aggarwal P, Beyer BN, Broeckel U, Epstein-Baak R, et al. Characterization of 137 genomic DNA reference materials for 28 pharmacogenetic genes: a GeT-RM collaborative project. J Mol Diagn (2016) 18(1):109–23. doi: 10.1016/j.jmoldx.2015.08.005
17. Ly RC, Shugg T, Ratcliff R, Osei W, Lynnes TC, Pratt VM, et al. Analytical validation of a computational method for pharmacogenetic genotyping from clinical whole exome sequencing. J Mol Diagn (2022) 24(6):576–85. doi: 10.1016/j.jmoldx.2022.03.008
18. Hari A, Zhou Q, Gonzaludo N, Harting J, Scott SA, Qin X, et al. An efficient genotyper and star-allele caller for pharmacogenomics. Genome Res (2023) 33(1):61-70. doi: 10.1101/gr.277075.122
19. Shugg T, Ly RC, Rowe EJ, Philips S, Hyder MA, Radovich M, et al. Clinical opportunities for germline pharmacogenetics and management of drug-drug interactions in patients with advanced solid cancers. JCO Precis Oncol (2022) 6:e2100312. doi: 10.1200/po.21.00312
20. Rabizadeh S, Garner C, Sanborn JZ, Benz SC, Reddy S, Soon-Shiong P. Comprehensive genomic transcriptomic tumor-normal gene panel analysis for enhanced precision in patients with lung cancer. Oncotarget (2018) 9(27):19223–32. doi: 10.18632/oncotarget.24973
21. White T, Szelinger S, LoBello J, King A, Aldrich J, Garinger N, et al. Analytic validation and clinical utilization of the comprehensive genomic profiling test, GEM ExTra(®). Oncotarget (2021) 12(8):726–39. doi: 10.18632/oncotarget.27945
22. PharmGKB. PGx gene-specific information tables. Available at: https://www.pharmgkb.org/page/pgxGeneRef.
23. Pharmacogene variation consortium. Genes. Available at: https://www.pharmvar.org/genes.
24. Clinical pharmacogenetics implementation consortium. Guidelines. Available at: https://cpicpgx.org/guidelines/.
25. Sun Y, Liu F, Fan C, Wang Y, Song L, Fang Z, et al. Characterizing sensitivity and coverage of clinical WGS as a diagnostic test for genetic disorders. BMC Med Genomics (2021) 14(1):102. doi: 10.1186/s12920-021-00948-5
26. Numanagić I. Aldy release history. Available at: https://pypi.org/project/aldy/4.4/#history.
27. Twesigomwe D, Wright GEB, Drögemöller BI, da Rocha J, Lombard Z, Hazelhurst S. A systematic comparison of pharmacogene star allele calling bioinformatics algorithms: a focus on CYP2D6 genotyping. NPJ Genom Med (2020) 5:30. doi: 10.1038/s41525-020-0135-2
28. Pratt VM, Cavallari LH, Del Tredici AL, Gaedigk A, Hachad H, Ji Y, et al. Recommendations for clinical CYP2D6 genotyping allele selection: a joint consensus recommendation of the association for molecular pathology, college of American pathologists, Dutch pharmacogenetics working group of the royal Dutch pharmacists association, and the European society for pharmacogenomics and personalized therapy. J Mol Diagn (2021) 23(9):1047–64. doi: 10.1016/j.jmoldx.2021.05.013
29. Pan A, Scodellaro S, Khan T, Ushcatz I, Wu W, Curtis M, et al. Pharmacogenetic profiling via genome sequencing in children with medical complexity. Pediatr Res (2022) 93(4):905–10. doi: 10.1038/s41390-022-02313-3
30. Powell NR, Shugg T, Ly RC, Albany C, Radovich M, Schneider BP, et al. Life-threatening docetaxel toxicity in a patient with reduced-function CYP3A variants: a case report. Front Oncol (2021) 11:809527. doi: 10.3389/fonc.2021.809527
31. Verma SS, Keat K, Li B, Hoffecker G, Risman M, Sangkuhl K, et al. Evaluating the frequency and the impact of pharmacogenetic alleles in an ancestrally diverse biobank population. J Transl Med (2022) 20(1):550. doi: 10.1186/s12967-022-03745-5
32. Aruldhas BW, Quinney SK, Overholser BR, Heathman MA, Masters AR, Ly RC, et al. Pharmacokinetic modeling of r and s-methadone and their metabolites to study the effects of various covariates in post-operative children. CPT Pharmacomet Syst Pharmacol (2021) 10(10):1183–94. doi: 10.1002/psp4.12687
33. Pratt VM, Cavallari LH, Del Tredici AL, Hachad H, Ji Y, Moyer AM, et al. Recommendations for clinical CYP2C9 genotyping allele selection: a joint recommendation of the association for molecular pathology and college of American pathologists. J Mol Diagn (2019) 21(5):746–55. doi: 10.1016/j.jmoldx.2019.04.003
34. Pratt VM, Del Tredici AL, Hachad H, Ji Y, Kalman LV, Scott SA, et al. Recommendations for clinical CYP2C19 genotyping allele selection: a report of the association for molecular pathology. J Mol Diagn (2018) 20(3):269–76. doi: 10.1016/j.jmoldx.2018.01.011
35. Pratt VM, Cavallari LH, Fulmer ML, Gaedigk A, Hachad H, Ji Y, et al. TPMT and NUDT15 genotyping recommendations: a joint consensus recommendation of the association for molecular pathology, clinical pharmacogenetics implementation consortium, college of American pathologists, Dutch pharmacogenetics working group of the royal Dutch pharmacists association, European society for pharmacogenomics and personalized therapy, and pharmacogenomics knowledgebase. J Mol Diagn (2022) 24(10):1051–63. doi: 10.1016/j.jmoldx.2022.06.007
36. Pratt VM, Cavallari LH, Del Tredici AL, Hachad H, Ji Y, Kalman LV, et al. Recommendations for clinical warfarin genotyping allele selection: a report of the association for molecular pathology and the college of American pathologists. J Mol Diagn (2020) 22(7):847–59. doi: 10.1016/j.jmoldx.2020.04.204
Keywords: pharmacogenetic algorithm, next-generating sequencing, whole genome sequencing (WGS), whole exome sequencing (WES), pharmacogenomics (PGx), pharmacogenetics (PGx)
Citation: Shugg T, Ly RC, Osei W, Rowe EJ, Granfield CA, Lynnes TC, Medeiros EB, Hodge JC, Breman AM, Schneider BP, Sahinalp SC, Numanagić I, Salisbury BA, Bray SM, Ratcliff R and Skaar TC (2023) Computational pharmacogenotype extraction from clinical next-generation sequencing. Front. Oncol. 13:1199741. doi: 10.3389/fonc.2023.1199741
Received: 03 April 2023; Accepted: 22 May 2023;
Published: 04 July 2023.
Edited by:
Elisa Frullanti, University of Siena, ItalyReviewed by:
Numrah Fadra, Mayo Clinic, United StatesBritt Drögemöller, University of British Columbia, Canada
Copyright © 2023 Shugg, Ly, Osei, Rowe, Granfield, Lynnes, Medeiros, Hodge, Breman, Schneider, Sahinalp, Numanagić, Salisbury, Bray, Ratcliff and Skaar. This is an open-access article distributed under the terms of the Creative Commons Attribution License (CC BY). The use, distribution or reproduction in other forums is permitted, provided the original author(s) and the copyright owner(s) are credited and that the original publication in this journal is cited, in accordance with accepted academic practice. No use, distribution or reproduction is permitted which does not comply with these terms.
*Correspondence: Todd C. Skaar, dHNrYWFyQGl1LmVkdQ==
†These authors have contributed equally to this work and share first authorship