- 1Department of Breast Surgery, Harbin Medical University Cancer Hospital, Harbin, China
- 2Department of Pathology, Harbin Medical University Cancer Hospital, Harbin, China
- 3Department of Urology Xiang Ya Hospital, Central South University, Changsha, Hunan, China
Background: HER2 expression has a central role in breast cancer carcinogenesis and is associated with poor prognosis. Lately, identification of HER2-low breast cancer has been proposed to select patients for novel HER2-directed chemotherapy and includes cancers with immunohistochemistry (IHC) 1+or 2+with negative fluorescence in situ hybridization (FISH), encompassing approximately 55–60% of all breast carcinomas. Neoadjuvant chemotherapy(NAC) is an important therapeutic modality for HER2-low breast cancer (BC). Immune inflammatory biomarkers have been reportedly linked to the prognosis of some different breast cancer types, with varying results. In this study, we investigated the possible predictive roles of blood-based markers and clinicopathologic features in axillary pathologically complete response (pCR) after neoadjuvant treatment (NAT) in HER2-low BC.
Methods: HER2-low BC patients diagnosed and treated in the Harbin Medical University Cancer Hospital from January 2012 to December 2018 were included. Relevant clinical and pathological characteristics were included, and baseline and preoperative complete blood cell counts were evaluated to calculate four systemic immune-inflammatory markers: neutrophil-to-lymphocyte ratio (NLR), platelet-to-lymphocyte ratio (PLR), lymphocyte-to-monocyte ratio (LMR), and systemic immune-inflammation index (SII). The optimal cutoff values for these markers were determined using ROC curves and patients were classified into high-value and low-value groups based on these cutoff values. Univariate and multivariate logistic regression analyses were conducted to analyze factors influencing axillary pCR. The factors with independent predictive value were used to construct a forest map.
Results: A total of 998 patients were included in the study. 35.6% (355 of 998) of patients achieved axillary pCR after NAC. The result of multivariate logistic regression analysis showed that Estrogen receptor (ER) (OR=2.18; 95% CI 1.43-3.32; P<0.001),pathology type (OR=0.51; 95% CI 0.40-0.65; P<0.001),baseline platelet (OR=1.45; 95% CI 1.02-2.05; P=0.037),preoperative PLR (OR=1.63; 95% CI 1.01-2.64; P=0.046) were significant independent predictors of ypN0.
Conclusion: The forest map for predicting axillary pCR incorporates four variables, including ER, pathology type, platelet, platelet-to-lymphocyte ratio (PLR). In patients treated with NAC, a higher baseline platelet and a higher preoperative PLR was associated with a higher incidence of axillary pCR.
Introduction
Breast cancer is a malignant tumor with high heterogeneity, and it presents a significant threat to women’s health (1, 2). Human epidermal growth factor receptor 2 (HER2) is a member of the human tyrosine kinase receptor family and serves as a crucial molecular marker for the diagnosis and treatment of breast cancer (3, 4). HER2-low breast cancer is defined as tumors with a HER2 IHC score of 2+ and negative FISH or IHC 1+ (5) and is found in approximately 60% of hormone receptor-positive (HR+) tumors and 40% of triple-negative breast cancer (TNBC) cases (6).
Compared with conventional breast cancer treatment, HER2-low expression breast cancer still uses traditional surgical resection (7). Preoperative neoadjuvant chemotherapy (NAC) is an important treatment that can reduce the stage of the tumor, increasing the chance of surgery (8). pCR in breast tumors is associated with improved prognosis and is an independent predictor of survival (9). At present, several studies have found that the pCR rate of HER2-low breast cancer patients after neoadjuvant therapy is between 13% and 30% (6, 10, 11). However, these data lack hematologic parameters for studies of pCR after neoadjuvant therapy.
Systemic inflammation plays a key role in tumorigenesis and cancer progression by stimulating angiogenesis, influencing immune surveillance and therapeutic efficacy (12), and promoting a favorable tumor microenvironment for cancer cell growth and spread (13).Several inflammation-associated hematological parameters, including the neutrophil-to-lymphocyte ratio (NLR), platelet-to-lymphocyte ratio (PLR) and lymphocyte-to-monocyte ratio (LMR)and systemic immune-inflammation index (SII), have been investigated as effective markers for predicting immunotherapy efficacy and patient prognosis with advanced BC (14–18). However, the predictive roles of these routinely available peripheral blood markers in patients with HER2-low BC receiving neoadjuvant chemotherapy has not been reported.
This study aims to investigate the predictive role of neoadjuvant chemotherapy in patients with HER2-low BC clinicopathologic features, baseline and preoperative NLR,PLR,LMR and SII axillary pCR. We found that higher baseline platelet and higher preoperative PLR were associated with a higher incidence of axillary pCR in patients with low HER2 expression. At the same time, we analyzed the effects of baseline and preoperative NLR, LMR, SII, baseline PLR and baseline neutrophils on axillary pCR. However, the P-values of these indicators were greater than 0.05, so there was no correlation between these indicators and axillary PCR rate.
Materials and methods
Patient selection and data collection
We retrospectively reviewed the clinical records in our institute from January 2012 to December 2018. The medical records of these patients were carefully reviewed to gain a comprehensive understanding of the effectiveness of breast cancer treatment and its associated factors. The inclusion criteria were as follows (1): Patients aged over 18 years. (2) Patients with HER2-0 or HER2-low (IHC 1+ or IHC 2+ and FISH-), breast cancer complete at least 4 cycles of anthracycline-taxeme-based NAC regimens.(3) Patients with a confirmed pathological diagnosis, complete clinical data, and peripheral blood indicators. (4) completed surgical treatment in our hospital with a pathological report. The exclusion criteria were as follows:(1) Patients who had distant metastasis at the time of diagnosis or bilateral breast cancer were excluded. (2) Patients who developed liver or kidney dysfunction during treatment and were unable to tolerate the treatment. (3) Patients with autoimmune diseases or other diseases that could affect peripheral blood indicators. A total of 998 patients were included in the study (Supplementary Table 1).
Data extraction and assessment
Peripheral blood samples were collected at baseline (defined the first blood collection time for patients just admitted to the hospital) and before surgery (defined a second blood collection after at least four cycles of neoadjuvant chemotherapy is performed before the patient has surgery). The NLR was defined as the absolute neutrophil count divided by the absolute lymphocyte count. The PLR was defined as the absolute platelet count divided by the absolute lymphocyte count and the LMR was defined as the absolute lymphocyte count divided by the absolute monocyte count. The SII is defined as absolute neutrophil count multiplied by absolute neutrophil count divided by absolute lymphocyte count. Data that may affect treatment efficacy were also collected including age, ER, PR, HER-2 expression, pN, pathological type, Histological Grade, Ki67% and Molecular subtype.
Breast tumor pCR was defined as the absence of invasive carcinoma residue. Axillary lymph node pCR was defined as the absence of cancer in all dissected axillary lymph nodes.
Chemotherapy regimens
Our study took anthracycline- (A-) based and/or taxane- (T-) based NAC regimens, repeat the cycle every three weeks for the selected regimens. All patients underwent a minimum of four cycles of NAC, antracycline and taxane regimens include: AC-T regimen: anthracyclines 100mg/m2, cyclophosphamide (C) 600mg/m2, followed by docetaxel 80-100mg/m2; TAC regimen: taxanes 75mg/m2, anthracyclines 50mg/m2, and cyclophosphamide 500mg/m2; AT regimen: taxanes 75mg/m2 and doxorubicin 60mg/m2.
Other regimens include: AC regimen: anthracyclines 90mg/m2 and cyclophosphamide 600mg/m2; TC regimen: docetaxel 80-100mg/m2 and cyclophosphamide 600mg/m2.
Grouping criteria
According to postoperative axillary lymph node pathologic response assessment, all included patients were dichotomized into two groups: pCR and non-pCR groups. The demographic,clinical and hematologic characteristics were compared between the two groups.
Statistical analysis
All statistical analyses were performed using IBM SPSS Statistics 26 software (https://www.ibm.com/cn-zh/spss). The data visualization was performed using R 4.3.0 (https://www.r-project.org/).
Categorical variables were expressed as numbers and percentages and were analyzed using the chi-square test. Normal distribution was assessed using the Shapiro-Wilk test. Normally distributed continuous variables were expressed as mean ± standard deviation (SD) and analyzed using the two-sample t-test, while non-normally distributed continuous variables were expressed as median and inter quartile range (IQR) and analyzed using the Mann-Whitney test.
A receiver operating characteristic (ROC) curve was constructed to estimate the optimal cut-off values for the baseline and preoperative NLR,PLR,MLR and SII, The cut-off values were selected based on the You-den index, which is calculated by subtracting 1 from the sum of sensitivity and specificity. Subsequently, the markers were further analyzed by dividing them into high and low values based on the optimal cut-off values. Logistic regression analysis was employed to conduct univariate analysis of the factors influencing pCR. The odds ratios (ORs) and 95% confidence intervals (CIs) were estimated for each variable. Subsequently, variables with a significance level of P<0.05 in the univariate analysis were included as potential predictors of pCR. P<0.05 is considered to indicate statistical significance for the difference.
Results
Patient and cancer characteristics
A total of 998 patients were included in the study. The mean age at initial diagnosis was 50 years (range 23-73). Table 1 presents the baseline characteristics of the patients. The majority of patients are ER receptor positive, PR receptor positive (72.7%, 62.5%), HER2-0 (53.1%), HER2-1+ (41.4%), HER2-2+/FISH- (5.5%) and nearly 65% of patients have positive axillary lymph nodes. 78% of patients had invasive ductal carcinoma, and another 2.2% had unknown pathological type. Histology II (61.6%) and III (10.4%) were the most common grade histology, with 23.8% of histological grades unknown. A larger proportion of patients (72.3%) had a Ki67 proliferation index of ≥14%. Nearly 30% of patient’s molecular subtype belongs Luminal A, TNBC and 40% belongs Luminal B. A total of 355(35.6%) patients achieved Axillary pCR after NAT.
Hematologic parameters
Peripheral blood samples were collected within 7 days before the first treatment and within 7 days before surgery Table 2, higher baseline neutrophil count (P = 0.017), higher baseline PLR count (P = 0.019), higher baseline and preoperative NLR (P = 0.005 and 0.028, respectively) and higher baseline and preoperative SII (P = 0.002 and 0.024, respectively) were observed in the non-pCR group than in the pCR group. However, no significant differences were observed between the two groups with respect to other hematological parameters.
Association of baseline hematological parameters with pCR
Based on the outcome variable pCR, ROC analysis was conducted for hematological markers to select the optimal cutoff values.
Figure 1 shows the ROC curves for baseline hematological parameters. Figure 1A represents the ROC curve for baseline lymphocyte, with an AUC of 0.513 and an optimal cutoff of 1.895. Figure 1B represents the ROC curve for baseline neutrophil, with an AUC of 0.544 and an optimal cutoff of 2.875. Figure 1C represents the ROC curve for baseline monocyte, with an AUC of 0.491 and an optimal cutoff of 0.325. Figure 1D represents the ROC curve for baseline platelet, with an AUC of 0.536 and an optimal cutoff of 225.5. Figure 1E represents the ROC curve for baseline NLR, with an AUC of 0.546 and an optimal cutoff of 1.30. Figure 1F represents the ROC curve for baseline PLR, with an AUC of 0.535 and an optimal cutoff of 116.97. Figure 1G represents the ROC curve for baseline LMR, with an AUC of 0.529 and an optimal cutoff of 6.66. Figure 1H represents the ROC curve for baseline SII, with an AUC of 0.549 and an optimal cutoff of 468.09.
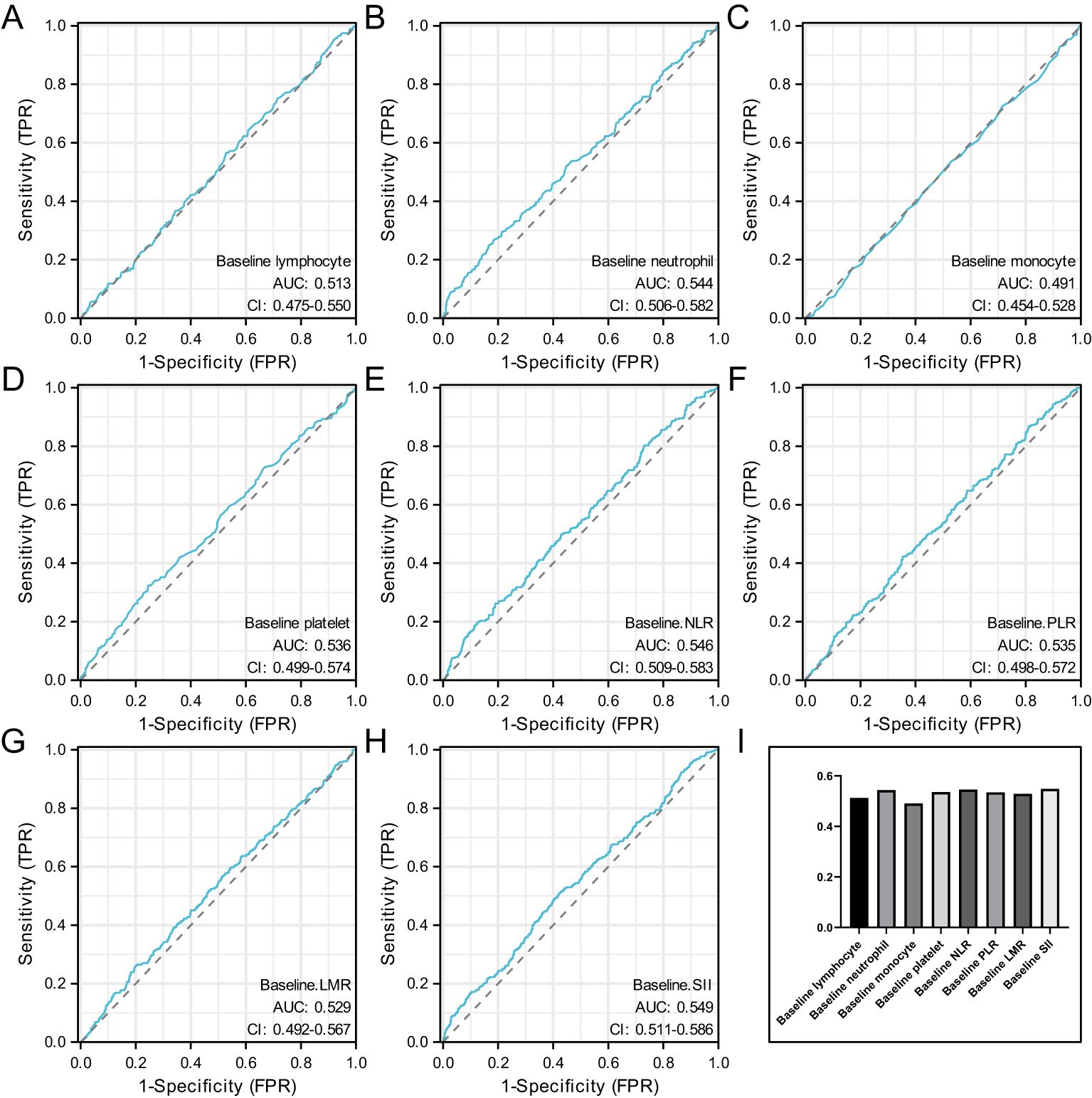
Figure 1. Represents the ROC curve for baseline lymphocyte (A), represents the ROC curve for baseline neutrophil (B), represents the ROC curve for baseline monocyte (C), represents the ROC curve for baseline platelet (D), represents the ROC curve for baseline NLR (E), represents the ROC curve for baseline PLR (F), represents the ROC curve for baseline LMR (G), represents the ROC curve for baseline SII (H) and the AUC value of baseline hematological parameters (I).
When the AUC value of baseline hematological parameters was greater than 0.05, indicating that these indicators were more closely related to axillary PCR before neoadjuvant therapy. Figure 1I.
Association of preoperative hematological parameters with pCR
Figure 2 shows the ROC curves for preoperative hematological parameters. Figure 2A represents the ROC curve for preoperative lymphocyte, with an AUC of 0.51 and an optimal cutoff of 1.635. Figure 2B represents the ROC curve for preoperative neutrophil, with an AUC of 0.534 and an optimal cutoff of 4.035. Figure 2C represents the ROC curve for preoperative monocyte, with an AUC of 0.522 and an optimal cutoff of 0.655. Figure 2D represents the ROC curve for preoperative platelet, with an AUC of 0.499 and an optimal cutoff of 349.5. Figure 2E preoperative the ROC curve for baseline NLR, with an AUC of 0.531 and an optimal cutoff of 4.00. Figure 2F represents the ROC curve for preoperative PLR, with an AUC of 0.508 and an optimal cutoff of 117.07. Figure 2G represents the ROC curve for preoperative LMR, with an AUC of 0.532 and an optimal cutoff of 2.07. Figure 2H represents the ROC curve for preoperative SII, with an AUC of 0.527 and an optimal cutoff of 835.04.
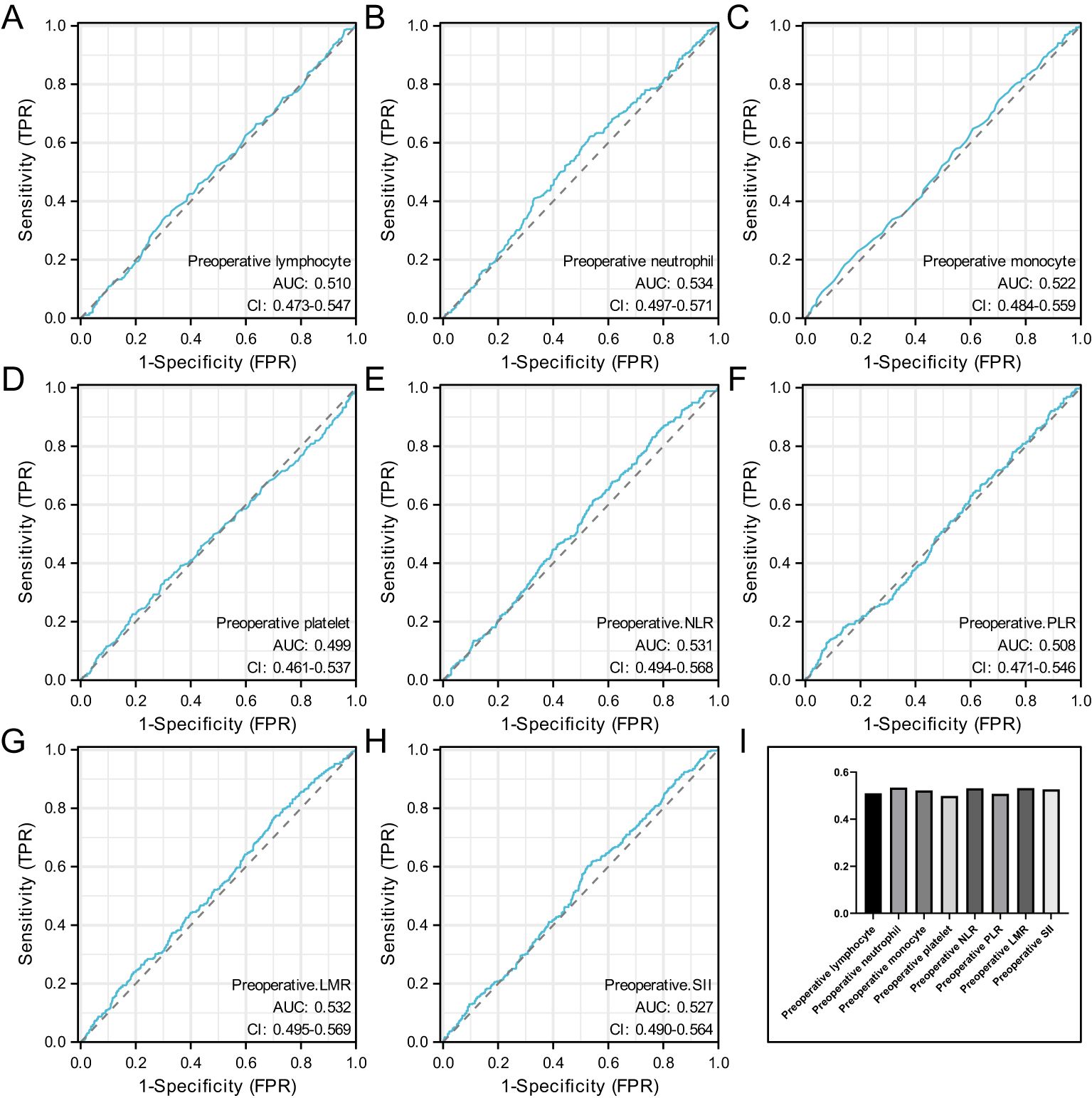
Figure 2. Represents the ROC curve for preoperative lymphocyte (A), represents the ROC curve for preoperative neutrophil (B), represents the ROC curve for preoperative monocyte (C), represents the ROC curve for preoperative platelet (D), preoperative the ROC curve for baseline NLR (E), represents the ROC curve for preoperative PLR (F), represents the ROC curve for preoperative LMR (G), represents the ROC curve for preoperative SII (H) and the AUC value of preoperative hematological parameters (I).
When the AUC value of preoperative hematological parameters was greater than 0.05, indicating that these indicators were more closely related to axillary PCR after neoadjuvant therapy. Figure 2I.
Univariate analysis of axillary pCR
The proportions of patients achieving pCR based on different clinical and tumor characteristics are presented in Table 3. In univariate logistic regression, patients with ER≥1%, PR≥1%, Ki67>14% were more likely to achieve pCR compared to those with ER<1%(P <0.001), PR<1% (P <0.001), Ki67 ≤ 14% (P = 0.006). pCR is more readily available to patients with invasive ductal carcinoma (P = 0.000), patients with higher values of baseline neutrophil, baseline platelet, baseline NLR, baseline PLR, preoperative PLR and preoperative LMR were more likely to achieve pCR compared to patients with lower values (P = 0.002; P = 0.01; P = 0.004; P = 0.036; P = 0.012; P = 0.03 respectively). On the other hand, patients with lower values of baseline LMR, baseline SII, preoperative neutrophil, preoperative NLR, preoperative SII were more likely to achieve pCR compared to patients with higher values (P = 0.031; P = 0.009; P = 0.009; P = 0.012; P = 0.022 respectively).
Multivariate analysis of axillary pCR
In the multivariate analysis, after including variables with P values<0.05 from the univariate analysis, two clinical and tumor characteristics and two hematologic markers still maintained their independent predictive roles. These factors include: ER at biopsy (OR=2.18; 95% CI 1.43–3.32; P <0.001), pathological type at biopsy (OR=0.51; 95% CI 0.40–0.65; P <0.001), baseline platelet (OR=1.45; 95% CI 1.02–2.05; P =0.037), preoperative PLR (OR=1.63; 95% CI 1.0–2.64; P =0.046). Incorporate the above factors as shown in Figure 3.
Univariate analysis of ER status
The proportion of ER status patients with different clinical and hematological parameters is shown in Table 4. In univariate logistic regression, among ER-negative patients, 93% had PR < 1% (P <0.001),71% had zero HER2 status (P <0.001),64.3% had invasive ductal carcinoma (P <0.001) and 78% had Ki67 > 14% (P <0.001). The proportion of LuminalA 279patients reached 94% (P <0.001) and nearly 53% achieved pCR (P <0.001). However, there was no statistical difference between ER status in terms of age, baseline and preoperative hematologic parameters (P >0.05).
Discussion
To our knowledge, this is the first study demonstrating hematological parameters prognostic significance in HER2-low breast cancer patients treated with NACT. This study first showed that baseline platelet, preoperative PLR was an independent predictor of axillary pCR. whereas the patient’s axillary pCR was not substantially correlated with the NLR, MLR and SII.
Several studies have investigated the pCR-predictive roles of systemic immune-infammatory markers (SIMs), but there was no consensus on the optimal indicator. As BC is a heterogeneous disease, different subtypes are amenable to different therapies (18). This study focused on patients with HER-low BC treated with neoadjuvant chemotherapy to avoid the bias among subtlety and find the optimal predictors in this subgroup. For early-stage breast cancer patients who are eligible for surgery, NAT has become the standard treatment method. However, evaluating treatment efficacy relies on pathological assessment of surgical specimens, and this opportunity for evaluation is only available once. Therefore, it is of great significance to search for indicators that can infer pathological response without the need for surgery (19).
Based on the traditional evaluation of neoadjuvant therapy effect, pathological and imaging methods, which rely on the limitation of the patient’s chemotherapy cycle time, we consider whether the efficacy of the patient’s neoadjuvant chemotherapy cycle can be predicted in advance through the indicators commonly used in clinical cases to supplement the pathological diagnosis.
The clinicopathological characteristics of cancer patients and SIMs derived from the quantification of immune and inflammatory cells in peripheral blood have the potential to serve as predictors for pathological treatment response. In the present study, ER status, clinical Pathology Type. The level of platelets before neoadjuvant chemotherapy and the ratio of platelets to lymphocytes before surgery were independent predictors of pCR in patients with HER2-low BC treated with NAT.
Qijun Zheng MD et al. (8) study included 953 and 267 patients from Peking University Cancer Hospital and Peking University First Hospital, respectively. In the construction cohort, 39.7% (238 of 600) of patients achieved axillary pCR after NAC. The result of multivariate logistic regression analysis showed that tumor grade, NAC regimen, and tumor biologic subtype were significant independent predictors of ypN0 (P < 0.05). This study found that the pCR rate reached 35% similar to our results, however in terms of tumor grade, and tumor biologic subtype, we didn’t find any of these factors were significant independent predictors of pCR, we considered this to be related to HER2 status, sample size, and selection bias, and statistical confirmation was needed at a later stage. Many previous studies have investigated systemic immune-inflammatory markers before NAT. Some studies have also suggested that a combination of systemic immune-inflammatory markers may better predict pCR in breast cancer patients after NAT (20, 21). Rulan Ma et al. (22) study found that white blood cell (WBC) platelet (PLT), PLR were independent predictors of pCR after NAC. There is also study found that PLR is an independent predictor of pCR after NAT in HER2-low breast cancer (23), This is consistent with our conclusions.
Some studies showed how lymphopenia can be a predictor of poor outcome in BC patients with increased risk of disease progression and worse long-term survival, this is associated with a weaker anti-tumor response and a lower number of tumor-infiltrating lymphocytes (TILs) (24, 25). The circulating lymphocyte count and lymphocytes characteristics, especially T-cell receptor diversity, have been investigated, either alone or in combination, as prognostic factors at diagnosis in BC patients (26). However, our study found no correlation between baseline peripheral blood lymphocyte count and preoperative peripheral blood lymphocyte count and the incidence of axillary pCR. Considering that this difference may be characteristic of the HER2-low BC subtype, the molecular mechanism of HER2-low BC and lymphocyte infiltration can be further investigated.
At the same time, we evaluated the influencing factors of axillary pCR in ER-positive and ER-negative patients, and found that pCR was more easily achieved in ER-negative patients, which was similar to the result of He et al. (27). However such patients tended to have poor prognosis. In addition, we found no correlation between ER status and hematological parameters.
However, the predictive roles of these markers in patients with HER2-low BC receiving NAT remain unclear. This study retrospectively analyzed the registered data of patients with HER2-low BC who were treated with NAC This is the first study to comprehensively assess the roles of clinicopathological characteristics, hematologic markers, NLR, PLR, LMR and SII in predicting axillary pCR in patients with HER2-low BC treated with NAC. Other data that may affect the treatment efficacy were also analyzed. Our results showed higher baseline PLT, and preoperative PLR was associated with a higher incidence of pCR. Some studies have suggested that the number of circulating platelets is associated with the level of serum VEGF-A and that platelet release promotes tumor growth and angiogenesis via VEGF integrins cooperative signaling in animal models of breast cancer (28, 29). In addition, it has been shown that platelets promote tumor proliferation via adenosine diphosphate receptors or metalloproteinase-9 and that their inhibitors reduce platelet-enhanced cancer cell proliferation (30, 31). Based on these results, it can be hypothesized that as the baseline PLT and preoperative PLR increases, the oncological outcomes of patients with cancer better.
This study has several limitations. First, this was a single-institute retrospective study, thus, selection bias may have occurred. Second, the patients included in this study was relatively Prognostic information is missing. Third, Pathology Type Histological Grade Partial deletion is present, and Invasive ductal carcinoma (IDC) accounts for almost 80% of patients. Future prospective large-scale studies, including a more varied population with a longer follow-up period are warranted to verify the outcomes of this study.
Conclusion
In conclusion, our study found that ER, pathology type, baseline PLT and preoperative PLR have independent predictive value for pCR after NAT in HER2-low breast cancer. However, future prospective studies are warranted to verify the predictive value of Those factors.
Data availability statement
The original contributions presented in the study are included in the article/Supplementary Material. Further inquiries can be directed to the corresponding authors.
Ethics statement
This study was reviewed and approved by The Ethics Committee of Harbin Medical University Cancer Hospital. The patients/participants provided their written informed consent to participate in this study.
Author contributions
SY: Conceptualization, Data curation, Investigation, Methodology, Software, Visualization, Writing – original draft, Writing – review & editing. GL: Conceptualization, Investigation, Methodology, Software, Visualization, Writing – original draft. JS: Conceptualization, Investigation, Methodology, Software, Visualization, Writing – original draft. LY: Conceptualization, Investigation, Methodology, Software, Visualization, Writing – original draft. ZF: Conceptualization, Investigation, Software, Visualization, Writing – original draft. WS: Conceptualization, Investigation, Methodology, Software, Visualization, Writing – original draft. BW: Conceptualization, Investigation, Methodology, Software, Visualization, Writing – original draft. AN: Data curation, Writing – review & editing. QW: Writing – review & editing. SX: Writing – review & editing.
Funding
The author(s) declare that financial support was received for the research and/or publication of this article. The National Natural Science Foundation of China (Grant No. 82072904 82103325); Climbing Program of Harbin Medical University Cancer Hospital (Grant No. PDYS2024-04); Key R&D Program of Heilongjiang Province (2022ZX06C15); Haiyan Fund Project of Harbin Medical University Cancer Hospital (JJMS 2022-17, JJYQ2024-06).
Conflict of interest
The authors declare that the research was conducted in the absence of any commercial or financial relationships that could be construed as a potential conflict of interest.
Publisher’s note
All claims expressed in this article are solely those of the authors and do not necessarily represent those of their affiliated organizations, or those of the publisher, the editors and the reviewers. Any product that may be evaluated in this article, or claim that may be made by its manufacturer, is not guaranteed or endorsed by the publisher.
Supplementary material
The Supplementary Material for this article can be found online at: https://www.frontiersin.org/articles/10.3389/fonc.2025.1437677/full#supplementary-material
References
1. Siegel RL, Miller KD, Fuchs HE, Jemal A. Cancer statistics, 2022. CA Cancer J Clin. (2022) 72:7–33. doi: 10.3322/caac.21708
2. Jiang YZ, Ma D, Suo C, Shi J, Xue M, Hu X, et al. Genomic and transcriptomic landscape of triple-negative breast cancers: subtypes and treatment strategies. Cancer Cell. (2019) 35:428–40.e5. doi: 10.1016/j.ccell.2019.02.001
3. Harbeck N, Gnant M. Breast cancer. Lancet. (2017) 389:1134–50. doi: 10.1016/S0140-6736(16)31891-8
4. Li YW, Dai LJ, Wu XR, Zhao S, Xu YZ, Jin X, et al. Molecular characterization and classification of HER2-positive breast cancer inform tailored therapeutic strategies. Cancer Res. (2024) 84(21):3669–83. doi: 10.1158/0008-5472.c.7522987
5. Tarantino P, Hamilton E, Tolaney SM, Cortes J, Morganti S, Ferraro E, et al. HER2-low breast cancer: pathological and clinical landscape. J Clin Oncol. (2020) 38:1951–62. doi: 10.1200/JCO.19.02488
6. Tarantino P, Jin Q, Tayob N, Jeselsohn RM, Schnitt SJ, Vincuilla J, et al. Prognostic and biologic significance of ERBB2-low expression in early-stage breast cancer. JAMA Oncol. (2022) 8:1177–83. doi: 10.1001/jamaoncol.2022.2286
7. Tang L, Li Z, Jiang L, Shu X, Xu Y, Liu S. Efficacy evaluation of neoadjuvant chemotherapy in patients with HER2-low expression breast cancer: A real-world retrospective study. Front Oncol. (2022) 12:999716. doi: 10.3389/fonc.2022.999716
8. Zheng Q, Yan H, He Y, Wang J, Zhang N, Huo L, et al. An ultrasound-based nomogram for predicting axillary node pathologic complete response after neoadjuvant chemotherapy in breast cancer: Modeling and external validation. Cancer. (2024) 130:1513–23. doi: 10.1002/cncr.v130.S8
9. Mougalian SS, Hernandez M, Lei X, Lynch S, Kuerer HM, Symmans WF, et al. Ten-year outcomes of patients with breast cancer with cytologically confirmed axillary lymph node metastases and pathologic complete response after primary systemic chemotherapy. JAMA Oncol. (2016) 2:508–16. doi: 10.1001/jamaoncol.2015.4935
10. Denkert C, Seither F, Schneeweiss A, Link T, Blohmer JU, Just M, et al. Clinical and molecular characteristics of HER2-low-positive breast cancer: pooled analysis of individual patient data from four prospective, neoadjuvant clinical trials. Lancet Oncol. (2021) 22:1151–61. doi: 10.1016/S1470-2045(21)00301-6
11. Alves FR, Gil L, Vasconcelos de Matos L, Baleiras A, Vasques C, Neves MT, et al. Impact of human epidermal growth factor receptor 2 (HER2) low status in response to neoadjuvant chemotherapy in early breast cancer. Cureus. (2022) 14:e22330. doi: 10.7759/cureus.22330
12. Crusz SM, Balkwill FR. Inflammation and cancer: advances and new agents. Nat Rev Clin Oncol. (2015) 12:584–96. doi: 10.1038/nrclinonc.2015.105
13. Hanahan D, Weinberg RA. Hallmarks of cancer: the next generation. Cell. (2011) 144:646–574. doi: 10.1016/j.cell.2011.02.013
14. Yamamoto T, Kawada K, Obama K. Inflammation-related biomarkers for the prediction of prognosis in colorectal cancer patients. Int J Mol Sci. (2021) 22(15):8002. doi: 10.3390/ijms22158002
15. Zhang CL, Jiang XC, Li Y, Pan X, Gao MQ, Chen Y, et al. Independent predictive value of blood inflammatory composite markers in ovarian cancer: recent clinical evidence and perspective focusing on NLR and PLR. J Ovarian Res. (2023) 16:36. doi: 10.1186/s13048-023-01116-2
16. Giannetta E, La Salvia A, Rizza L, Muscogiuri G, Campione S, Pozza C, et al. Are markers of systemic inflammatory response useful in the management of patients with neuroendocrine neoplasms? Front Endocrinol (Lausanne). (2021) 12:672499. doi: 10.3389/fendo.2021.672499
17. Hu X, Tian T, Sun Q, Jiang W. Prognostic value of the neutrophil-to-lymphocyte ratio and platelet-to-lymphocyte ratio in laryngeal cancer: What should we expect from a meta-analysis? Front Oncol. (2022) 12:945820. doi: 10.3389/fonc.2022.945820
18. Wu HY, Lin CY, Tzeng YD, Hung CC, Liu SI, Yin CH, et al. Preoperative systemic inflammation response index: Clinicopathologic predictor of pathological complete response in HER2-positive breast cancer patients receiving neoadjuvant systemic therapy. J Chin Med Assoc. (2024) 87:226–35. doi: 10.1097/JCMA.0000000000001034
19. Heil J, Kuerer HM, Pfob A, Rauch G, Sinn HP, Golatta M, et al. Eliminating the breast cancer surgery paradigm after neoadjuvant systemic therapy: current evidence and future challenges. Ann Oncol. (2020) 31:61–71. doi: 10.1016/j.annonc.2019.10.012
20. Jin X, Wang K, Shao X, Huang J. Prognostic implications of the peripheral platelet-to-lymphocyte ratio and neutrophil-to-lymphocyte ratio in predicting pathologic complete response after neoadjuvant chemotherapy in breast cancer patients. Gland Surg. (2022) 11:1057–66. doi: 10.21037/gs-22-244
21. Kim HY, Kim TH, Yoon HK, Lee A. The role of neutrophil-lymphocyte ratio and platelet-lymphocyte ratio in predicting neoadjuvant chemotherapy response in breast cancer. J Breast Cancer. (2019) 22:425–38. doi: 10.4048/jbc.2019.22.e41
22. Ma R, Wei W, Ye H, Dang C, Li K, Yuan D. A nomogram based on platelet-to-lymphocyte ratio for predicting pathological complete response of breast cancer after neoadjuvant chemotherapy. BMC Cancer. (2023) 23:245. doi: 10.1186/s12885-023-10703-x
23. Hu Y, Wang S, Ding N, Li N, Huang J, Xiao Z. Platelet/lymphocyte ratio is superior to neutrophil/lymphocyte ratio as a predictor of chemotherapy response and disease-free survival in luminal B-like (HER2(-)) breast cancer. Clin Breast Cancer. (2020) 20:e403–9. doi: 10.1016/j.clbc.2020.01.008
24. Che YQ, Zhang Y, Wang D, Liu HY, Shen D, Luo Y. Baseline lymphopenia: A predictor of poor outcomes in HER2 positive metastatic breast cancer treated with trastuzumab. Drug Des Devel Ther. (2019) 13:3727–34. doi: 10.2147/DDDT.S212610
25. Trédan O, Manuel M, Clapisson G, Bachelot T, Chabaud S, Bardin-dit-Courageot C, et al. Patients with metastatic breast cancer leading to CD4+ T cell lymphopaenia have poor outcome. Eur J Cancer. (2013) 49:1673–82. doi: 10.1016/j.ejca.2012.11.028
26. Manuel M, Tredan O, Bachelot T, Clapisson G, Courtier A, Parmentier G, et al. Lymphopenia combined with low TCR diversity (divpenia) predicts poor overall survival in metastatic breast cancer patients. Oncoimmunology. (2012) 1:432–40. doi: 10.4161/onci.19545
27. Dou H, Li F, Wang Y, Chen X, Yu P, Jia S, et al. Estrogen receptor-negative/progesterone receptor-positive breast cancer has distinct characteristics and pathologic complete response rate after neoadjuvant chemotherapy. Diagn Pathol. (2024) 19:5. doi: 10.1186/s13000-023-01433-6
28. Jiang L, Luan Y, Miao X, Sun C, Li K, Huang Z, et al. Platelet releasate promotes breast cancer growth and angiogenesis via VEGF-integrin cooperative signalling. Br J Cancer. (2017) 117:695–703. doi: 10.1038/bjc.2017.214
29. Salgado R, Benoy I, Dirix L, Vermeulen P, Van Marck E. Importance of platelets in VEGF-mediated angiogenesis in tumors. FEBS Lett. (2002) 520:182. doi: 10.1016/S0014-5793(02)02801-6
30. Cho MS, Noh K, Haemmerle M, Li D, Park H, Hu Q, et al. Role of ADP receptors on platelets in the growth of ovarian cancer. Blood. (2017) 130:1235–42. doi: 10.1182/blood-2017-02-769893
Keywords: breast cancer, HER2-low, axillary node, neoadjuvant chemotherapy, pathologic complete response
Citation: Yang S, Liang G, Sun J, Yang L, Fu Z, Sun W, Wei B, Nanding A, Wang Q and Xu S (2025) Higher baseline platelet and preoperative platelets to lymphocytes ratio was associated with a higher incidence of axillary node pathologic complete response after neoadjuvant chemotherapy in HER2-low breast cancer: a retrospective cohort study. Front. Oncol. 15:1437677. doi: 10.3389/fonc.2025.1437677
Received: 24 May 2024; Accepted: 20 January 2025;
Published: 14 March 2025.
Edited by:
Sharon R. Pine, University of Colorado Anschutz Medical Campus, United StatesReviewed by:
Sungchan Gwark, Ewha Womans University Seoul Hospital, Republic of KoreaFrancesca Piccotti, Scientific Clinical Institute Maugeri (ICS Maugeri), Italy
Copyright © 2025 Yang, Liang, Sun, Yang, Fu, Sun, Wei, Nanding, Wang and Xu. This is an open-access article distributed under the terms of the Creative Commons Attribution License (CC BY). The use, distribution or reproduction in other forums is permitted, provided the original author(s) and the copyright owner(s) are credited and that the original publication in this journal is cited, in accordance with accepted academic practice. No use, distribution or reproduction is permitted which does not comply with these terms.
*Correspondence: Abiyasi Nanding, YWJpeWFzaTAwMUAxNjMuY29t; Qin Wang, d2FuZ3FpbjA5MjNAMTYzLmNvbQ==; Shouping Xu, c2hvdXBpbmd4dUBocmJtdS5lZHUuY24=
†These authors have contributed equally to this work