- 1Department of Hepatobiliary and Pancreatic Surgery, The Affiliated Hospital of North Sichuan Medical College, Nanchong, China
- 2Department of Clinical Medicine, North Sichuan Medical College, Nanchong, China
Background: Currently, there is no validated model for predicting the occurrence and prognosis of lung metastases (LM) in patients with pancreatic ductal adenocarcinoma (PDAC). We aimed to construct a nomogram for risk prediction and a prognostic model to guide clinical practice.
Methods: In total, 10,813 patients were enrolled from the Surveillance, Epidemiology, and End Results (SEER) database between 2010 and 2015 and divided into training and internal validation cohorts at a ratio of 7:3. Following nomogram construction, data of patients diagnosed with PDAC were retrospectively collected for external validation.
Results: Using multivariate logistic regression analysis, larger tumour size, primary tumour site in the body or tail of the pancreas, bone metastasis, and liver metastasis were associated with LM. Furthermore, through multivariate Cox analysis, we found that LM was associated with a poor prognosis in patients with PDAC. Patients who underwent surgery or chemotherapy had better prognoses.
Conclusion: The two nomograms showed excellent performance in the training and internal validation cohorts and a favourable performance in the external validation. The prognostic nomogram divided the patients into high- and low-risk groups based on mortality. The LM risk and prognostic prediction model in PDAC showed high accuracy and reliable clinical application.
Introduction
Globally, pancreatic ductal adenocarcinoma (PDAC) stands as the foremost reason for mortality associated with cancer, surpassing breast cancer as the third leading cause of cancer-related deaths in the United States (US) and is forcasted to be the second leading cause of cancer-related deaths in the US by 2030 (1, 2). In China, PDAC ranks ninth among the frequently encountered malignant tumours and the sixth prevalent cause of mortality (3). PDAC is characterised by a grim prognosis and high lethality, exemplified by a 5-year relative survival rate of roughly 10% in the US (4). Many factors affect the prognosis of patients with PDAC, of which distant metastasis is a vital factor. At the time of diagnosis, the majority of patients are found to have either locally advanced (30-35%) or metastatic (50-55%) stages of the disease (5).
In PDAC, the liver is the predominant site for distant metastasis, with the lung being the subsequent most frequent location (referred to as lung metastasis, or LM). The incidence of LM occurring after the initial diagnosis or recurrence of pancreatic cancer (PC) has been reported to be 4.76%–12.3% (6, 7). Three pathways are involved in developing LM: lymphatic metastasis, blood transport metastasis, and direct infiltration. Mechanistically, the metastatic process includes alterations in cancer cell surface adhesion molecules, transmembrane signal distortion, genetic changes, tumour immune microenvironment changes, and exosomes (8). The National Comprehensive Cancer Network (NCCN) guidelines define LM as stage IV, with an extremely poor prognosis. For patients diagnosed with isolated lung metastasis (LM), the reported median Overall Survival (OS) was 561 days, with a median Recurrence-Free Survival (RFS) of 748 days, and a median Progression-Free Survival (PFS) of 307 days (9). Compared with patients with localised tumours, the 5-year survival rate of patients with metastatic PDAC decreases significantly from 42% to 3% (10). Therefore, early diagnosis and detection of PDAC in patients with LM are essential.
Imaging examination is the preferred option for the preliminary assessment of metastasis in clinical scenarios, including computed tomography (CT), magnetic resonance imaging (MRI), and fluorodeoxyglucose positron emission tomography/computed tomography (FDG-PET/CT); however, each has its advantages, with no way to determine which has the best evaluation ability (11–15). The detection efficiency may be improved with a combination of these methods. Tissue biopsy is the gold standard for diagnosing metastatic carcinoma but is not routinely used for early diagnosis unless necessary. Liquid biopsy has attracted wide attention due to the minimal associated trauma and its ability to assess tumour heterogeneity; however, it has no standardised detection method and is costly; thus, it is not used in the clinic (16, 17). Accordingly, we urgently need to establish a new practical clinical diagnosis modality and improve the diagnostic efficiency of patients with PDAC and LM to improve prognosis.
Conventional clinical treatments for PDAC include surgery, chemotherapy, and radiotherapy. The PDAC diagnosis and treatment guidelines do not recommend surgical resection for patients with distant metastases. However, in a national multicentre study, Japanese scholars showed that surgery is feasible for PDAC with isolated pulmonary metastases and can be supplemented with gemcitabine (18). In the absence of contraindications, chemotherapy is the routine treatment after radical resection of PDAC, and the separate use of gemcitabine or fluorouracil is recommended (19). However, PDAC shows some resistance to chemotherapeutic drugs, especially gemcitabine. Studies have shown that PDAC cells are more resistant to gemcitabine than other chemotherapeutic drugs (20, 21). This greatly limits the use of gemcitabine and reduces the patient’s prognosis. Therefore, there is an urgent need for practical tools and useful clinical treatment options for PDAC with distant metastases.
The Surveillance, Epidemiology, and End Results (SEER) program gathers demographic, clinical, and outcome information for all cancer diagnoses within selected geographical areas and subgroups across the United States, covering approximately 48% of the total number of patients with cancer in the US population (22). As visual tools, nomograms are widely used in oncology research to build diagnostic and prognostic cancer models. One of the main advantages is the ability to individualise risk estimation based on patient and disease characteristics, which is superior to clinicians’ judgment regarding disease progression (23, 24). Furthermore, due to its significantly better clinical efficacy, the nomogram has replaced the tumour–lymph node–metastasis (TNM) staging system as a new standard for tumour diagnosis (25, 26). Nevertheless, no relevant nomogram has been established for the diagnostic prediction of PC with LM (PCLM). Therefore, the objective of this research was to develop and verify an effective nomogram for the clinical diagnostic prediction of PCLM.
Methods
Patient selection
We extracted data from patients newly diagnosed with PDAC between 2010 and 2015 from the SEER database. The following inclusion criteria were applied: (1) complete survival and follow-up data; (2) PC as the primary tumour (topographical codes from the International Classification of Diseases for Oncology ICD-O-3: C25.0-C25.3, C25.7-C25.9); (3) histological subtype PDAC (ICD-O: 8140, 8480, 8500); and (4) a clear pathological diagnosis of PC and an imaging diagnosis of LM.
The exclusion criteria were as follows: (1) incomplete baseline information, such as age, sex, race, grade, stage, treatment, and metastasis, and (2) patients with unknown primary tumours and metastatic status. Finally, our study included 10,813 patients diagnosed with PDAC, including 445 patients with LM.
In addition, we retrospectively collected data from patients with PDAC and LM at the Affiliated Hospital of North Sichuan Medical College between 2016 and 2023 as an external validation cohort. The inclusion and exclusion criteria for the external validation cohort were consistent with those of the internal cohort. The flow chart for patient selection is shown in Figures 1a, b. The research program was approved by the Institutional Review Committee of the Afemittee Hospital of North Sichuan Medical College (number: 2024ER568-1), and the requirement for informed consent was waived because it’s a retrospective study. Otherwise, all methods were performed in accordance with the relevant guidelines and regulations.
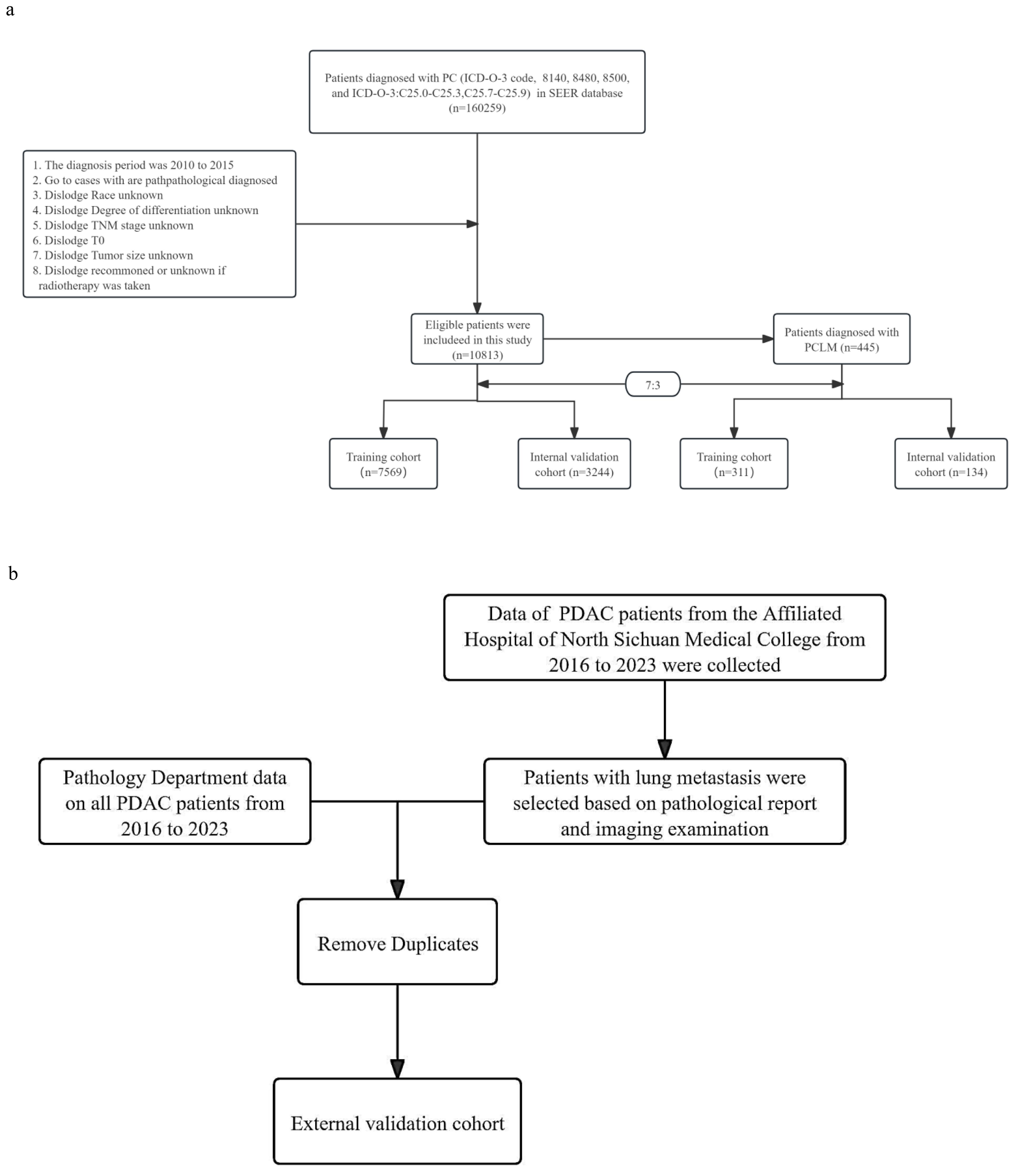
Figure 1. (a) Flow chart of patient inclusion and grouping. (b) Flow chart of patient selection in the external validation cohort.
Baseline characteristics
Baseline characteristic data were used to analyse the risk factors of LM in PDAC, including age at diagnosis, race, sex, grade, primary site, American Joint Committee on Cancer (AJCC) T stage, AJCC N stage, tumour size, and metastasis status. The following variables were collected to assess the prognosis of patients with PDAC with LM: age, sex, race, income, marital status, grade, tumour size, primary site, AJCC T stage, AJCC N stage, delayed treatment, surgery (performed or not performed), radiation (performed or not performed), chemotherapy (performed or not performed), and metastasis status. The optimal tumour size cut-off was determined by x-tiles software, and the subjects were divided into three groups according to the tumour size: ≤25 mm, 26–40 mm, and ≥41 mm (27). Finally, we used an electronic medical record system to collect the baseline characteristics of patients with PC in the external validation cohort.
Statistical analysis
First, patients were randomly divided into training and internal validation cohorts (7:3). Chi square and Fisher’s exact tests showed no significant differences in variables between cohorts (P > 0.05). Univariate logistic analysis was done first. Variables with P < 0.05 were selected for multivariable logistic analysis to determine LM risk factors in PDAC. Univariate Cox regression was used for prognostic factor analysis (P < 0.05), and these variables were included in multivariate Cox regression to determine independent prognostic factors for PDAC with LM. Odds ratios (ORs) and 95% confidence intervals (95% CIs) were calculated. Then, two nomograms based on risk and prognostic factors were established via the “rms” package in R. Receiver operating characteristic (ROC) curves were used to assess nomogram accuracy, and decision curve analysis (DCA) was used to test clinical utility (28, 29). Calibration plots were used to estimate prediction actual observation consistency. DCA was used to quantify net gain at different threshold probabilities to compare nomograms and other models (30). We verified the nomogram’s clinical utility by comparing ROC and DCA curve areas of the nomogram and TNM stages in training and validation cohorts. The prognostic nomogram’s predictive value was verified using high and low risk survival curves based on median risk score (31). In this study, overall survival (OS) from diagnosis to death (due to cancer) was the prognostic survival outcome. Finally, an external validation cohort was used to evaluate efficacy in Chinese patients. All data analyses were performed using R software (version 4.3.1).
Results
Clinical baseline characteristics of patients with PDAC
We included 10,813 patients with PDAC from the SEER database, among which 445 had LM. Meanwhile, 7,569 (70%) patients were assigned to the training cohort, and 3,244 (30%) patients were included in the internal validation cohort; there were no significant differences in the patient characteristics between these two cohorts (Table 1). The baseline clinical characteristics of the external validation group are shown in Table 2. A total of 132 patients with PDAC were included, 32 of whom developed LM.
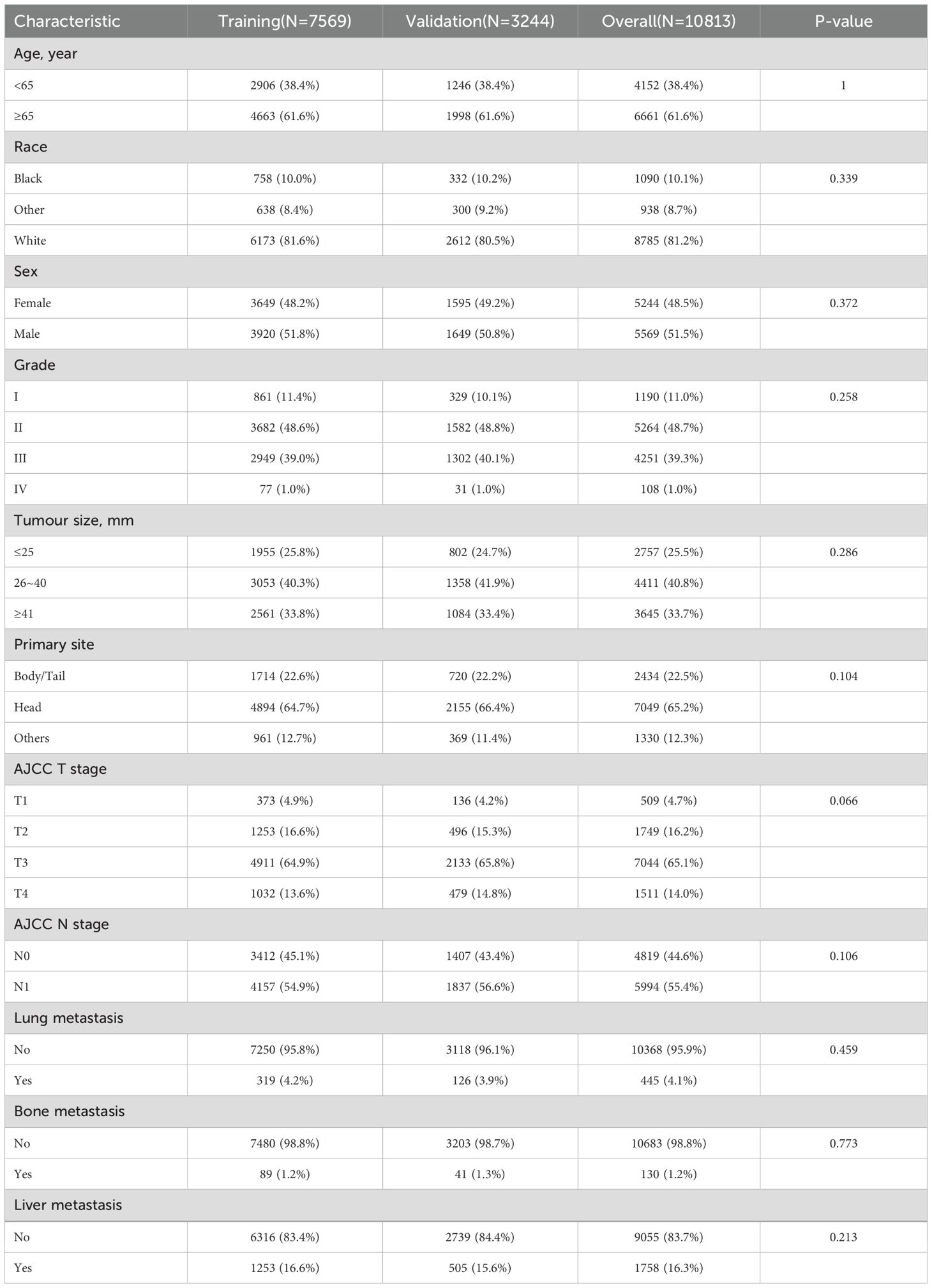
Table 1. Baseline characteristics of PDAC patients over training cohort and internal validation cohort.
Independent risk factors for LM in PDAC
As shown in Table 3, we performed univariate logistic regression analysis on the ten latent factors and then six LM-related variables, including grade, tumour size, primary site, AJCC T stage, bone metastasis, and liver metastasis. These variables were then subjected to a multivariate logistic regression analysis. The results showed that the independent predictors of LM in PDAC were the primary site, tumour size, bone metastasis, and liver metastasis.
Construction and validation of the diagnostic model
We constructed a risk prediction nomogram model for PCLM based on the primary site, tumour size, bone metastasis, and liver metastasis (Figure 2).
A validation curve was constructed in accordance with the model shown in the nomogram. The ROC analysis showed that the area under the curve (AUC) value of the nomogram was 0.776 in the training cohort and 0.858 in the internal cohort, indicating a strong discriminative power of the model (Figures 3a, d). The calibration curves were highly consistent with the predictions (Figures 3b, e). The DCA indicated the high validity of the nomogram model in clinical practice (Figures 3c, f).
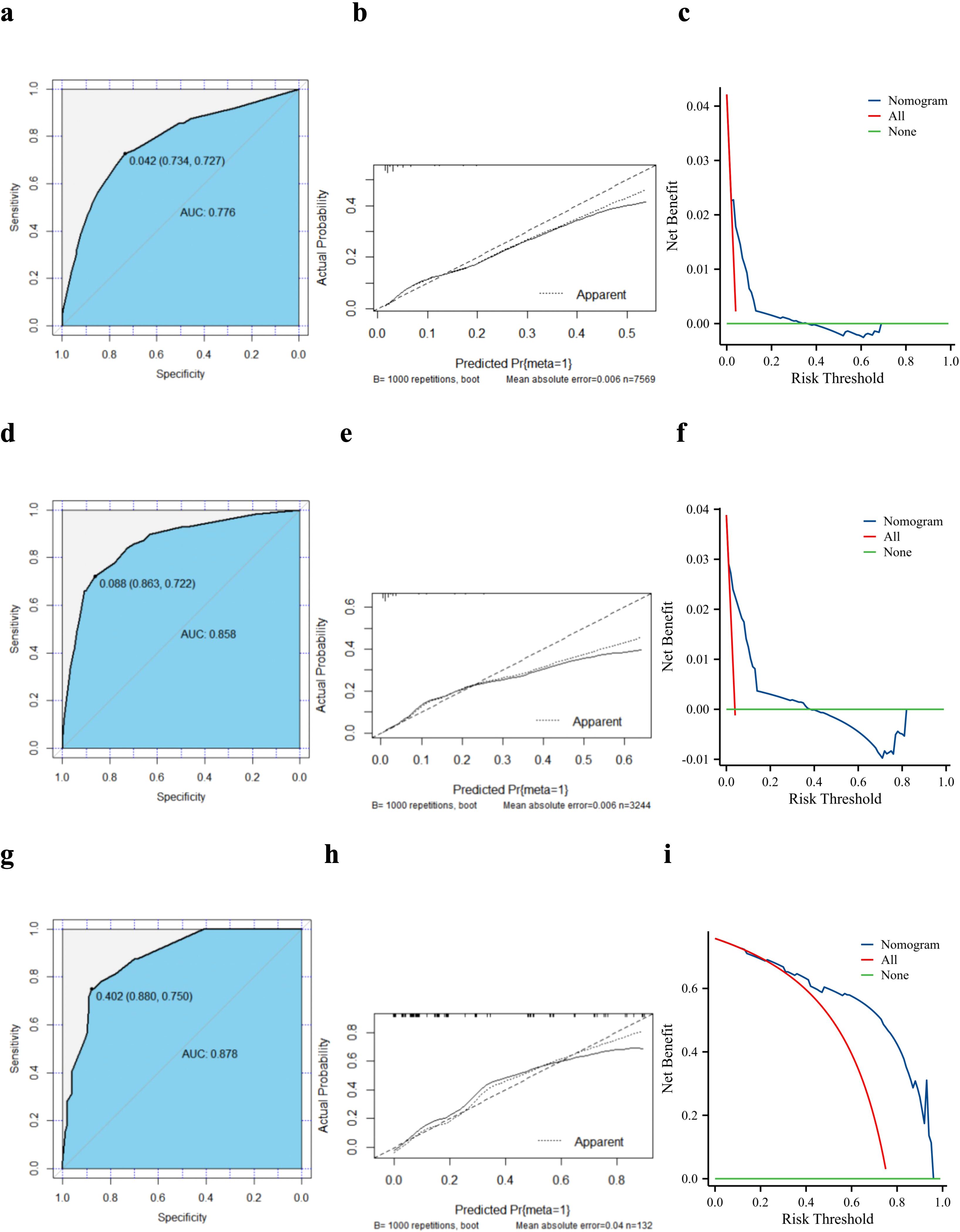
Figure 3. Validation of the diagnostic model. Receiver operating characteristic curves, calibration plots, and decision curve analysis of the nomogram for the risk of lung metastasis in pancreatic ductal adenocarcinoma based on the training cohort (a–c), internal validation cohort (d–f), and external validation cohort (g–i). AUC, area under the curve.
We created an external validation cohort to evaluate the model further using the same analysis. The ROC analysis showed that the AUC value of the nomogram was 0.878, which indicated that the model also had a good discriminatory ability in Chinese patients with PC (Figure 3g). The calibration curve showed good agreement between the nomogram predictions and actual observations, and the external validation cohort was almost identical to the training cohort (Figure 3h). Furthermore, the DCA showed clinical effectiveness similar to that in the training cohort (Figure 3i).
The TNM system is an internationally accepted criterion for cancer staging commonly used to predict the clinical behaviour of malignant tumours and guide clinical decision-making. We compared the predictive effects of both methods by plotting traditional TNM staging against the ROC curves of the nomogram and DCA. The analysis showed that the nomogram had better discrimination than TNM staging in both the modelled and external validation cohorts (Figures 4a–f).
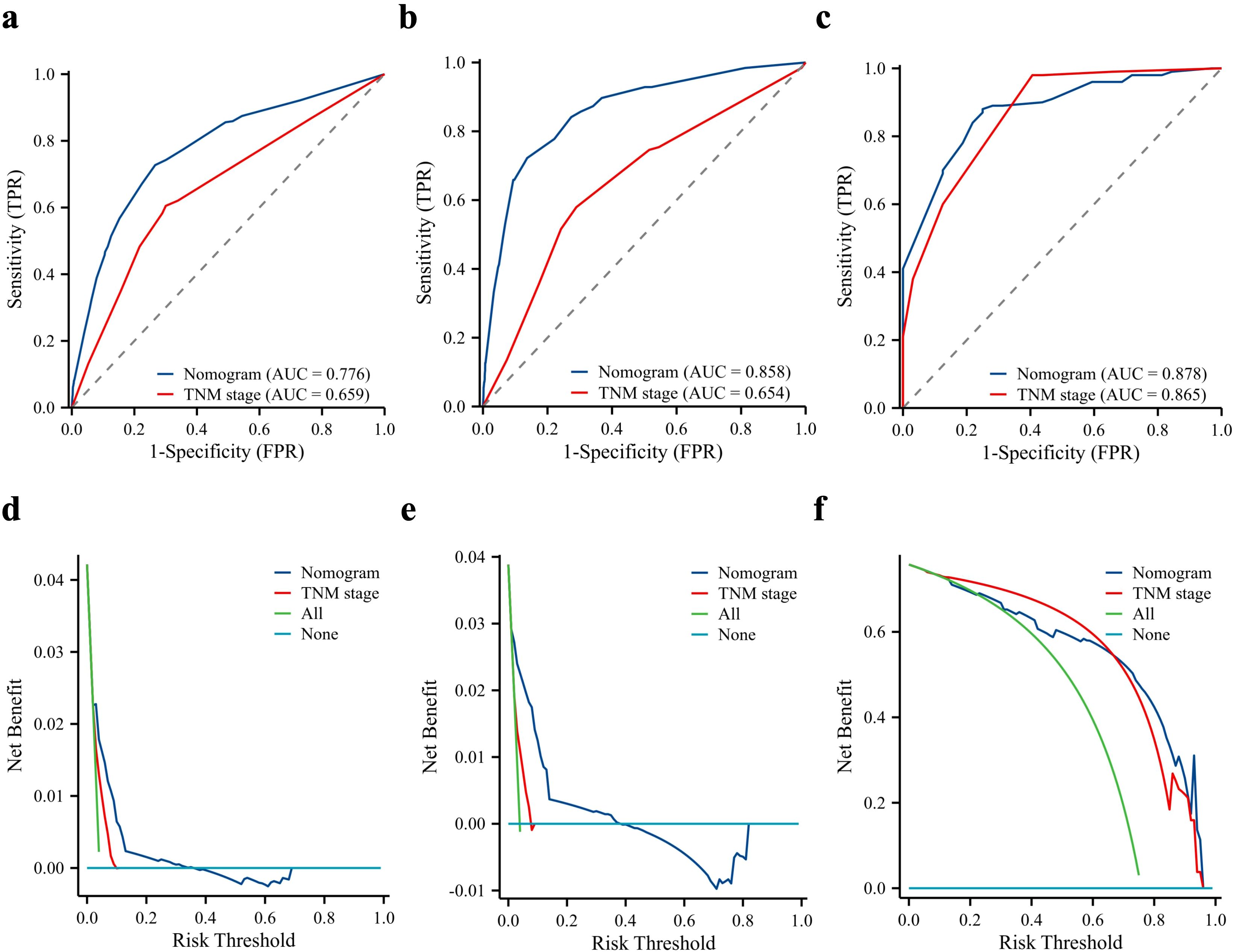
Figure 4. Predictive performance of the nomogram compared to the TNM stage. Comparison of receiver operating characteristic curve and decision curve analysis between nomogram and TNM stage in the training cohort (a, d), internal validation cohort (b, e), and external validation cohort (c, f). AUC, area under the curve.
Clinical baseline characteristics of patients with PDAC and LM
A total of 445 patients with LM were used to identify the prognostic factors. All patients were randomly categorised at the ratio of 7:3 into training (n = 311) and validation (n = 134) cohorts. No variables were significantly different between the two cohorts (Table 4).
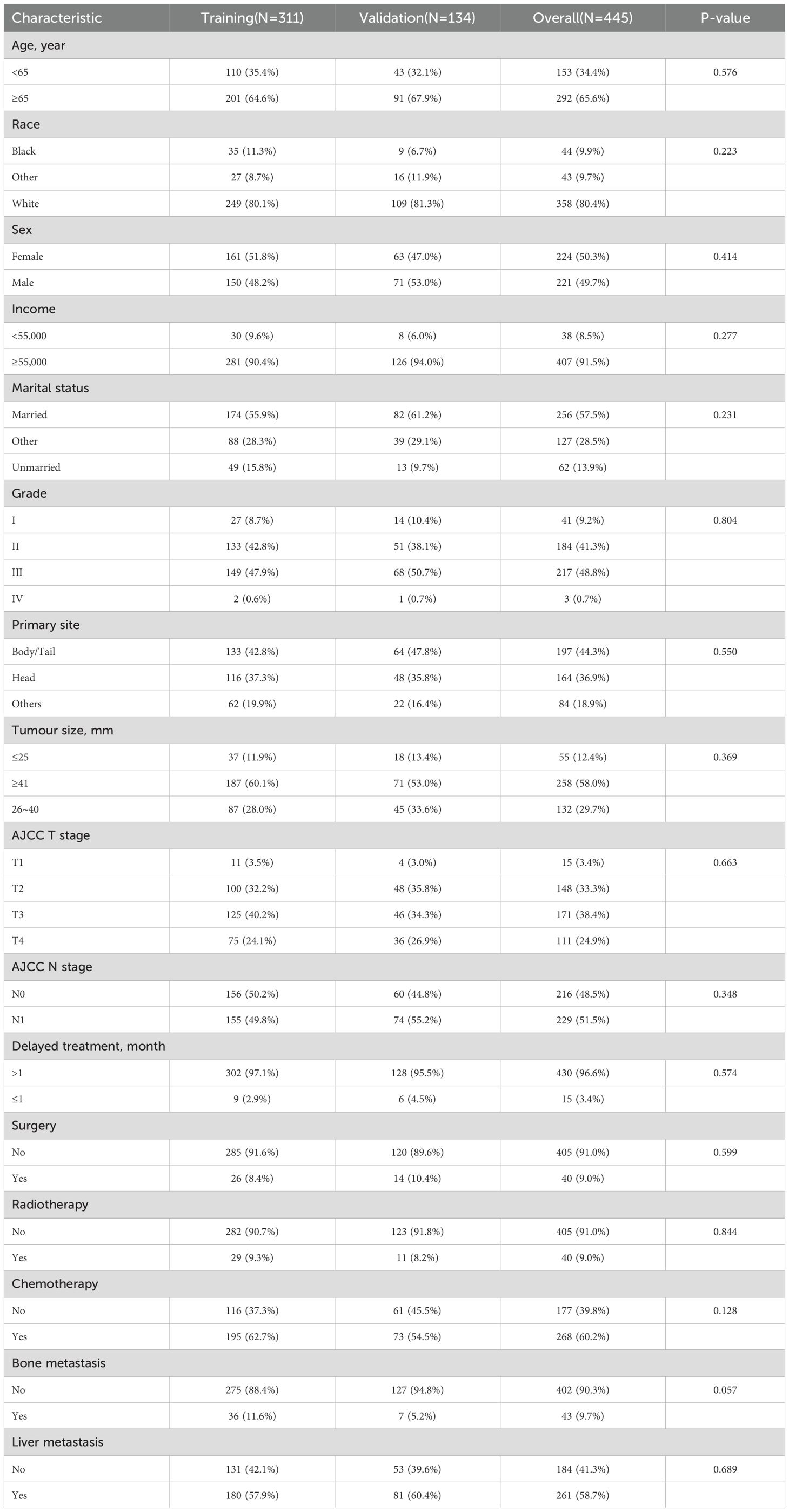
Table 4. Baseline characteristics of PDAC patients with LM among training cohort and internal validation cohort.
Construction and validation of the prognostic model
Six variables were related to the prognosis of patients with PDAC and LM: race, primary site, surgery, chemotherapy, bone metastasis, and liver metastasis. Independent prognostic factors, including the primary site, liver metastasis, surgery, and chemotherapy, were identified using multivariate Cox regression analysis (Table 5).
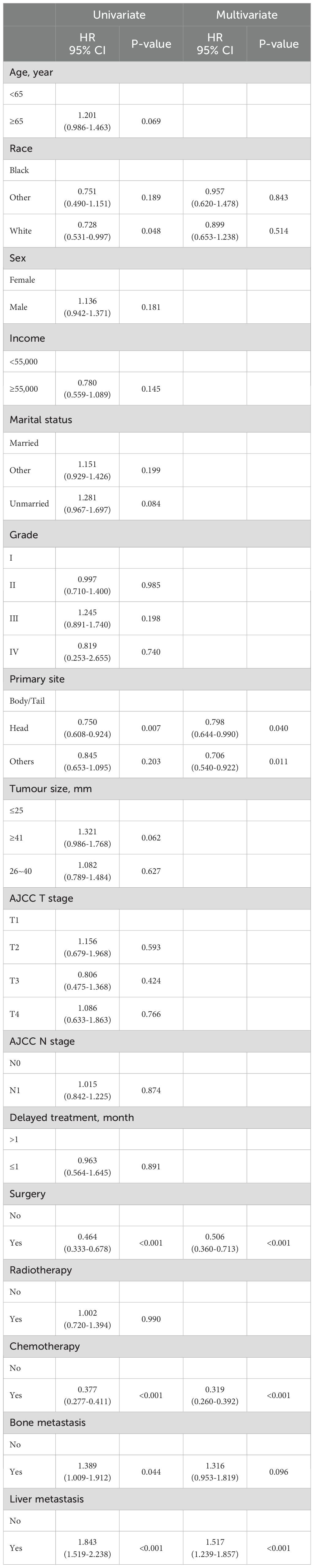
Table 5. Univariate and multivariate COX regression analysis of prognostic factors for PDAC patients with LM.
We constructed a prognosis prediction nomogram model for PDAC with LM based on the primary site, liver metastasis, surgery, and chemotherapy (Figure 5).
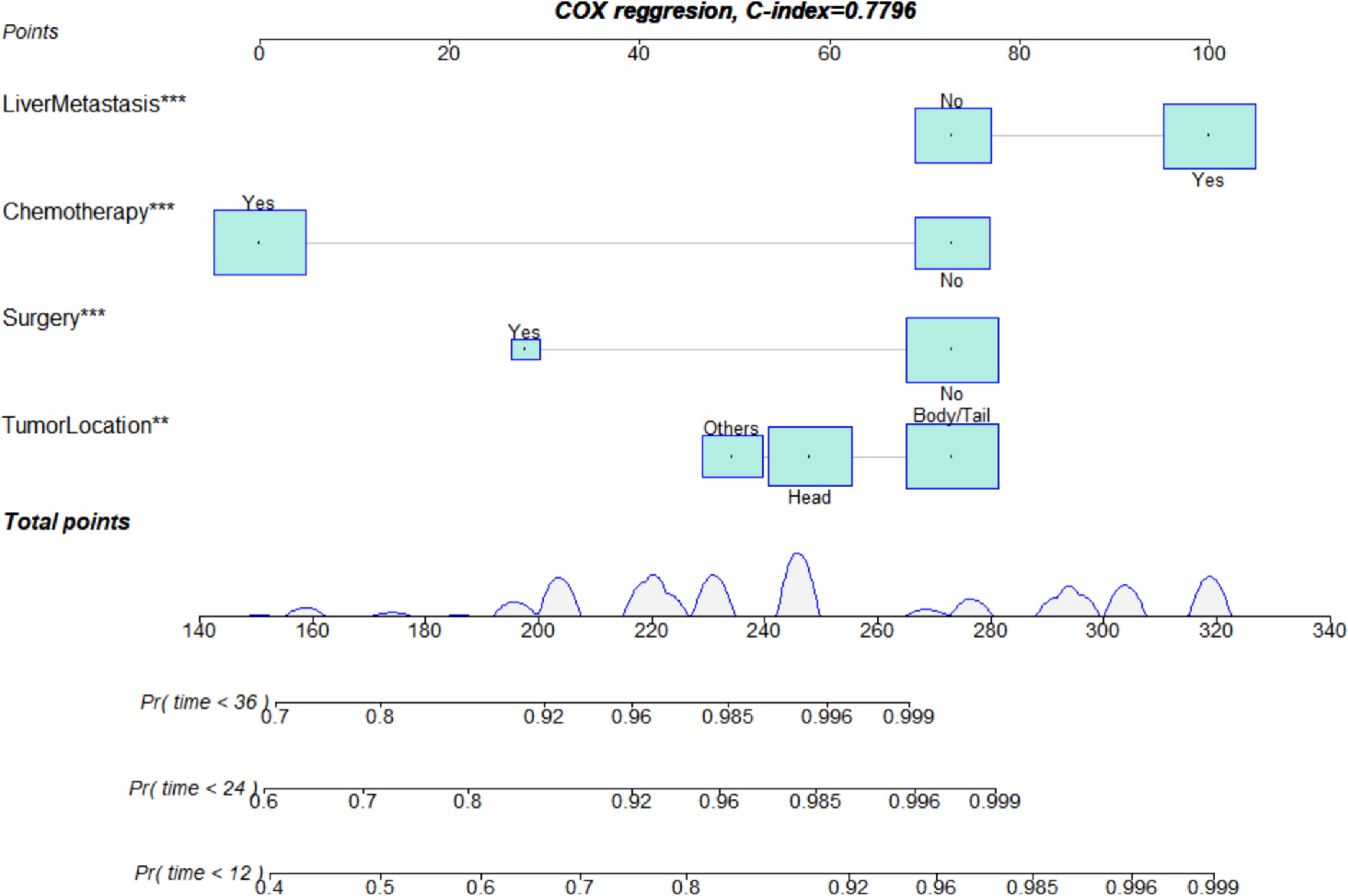
Figure 5. Nomogram for assessing the prognosis of lung metastasis in pancreatic ductal adenocarcinoma. **p<0.01, ***p<0.001.
The ROC analysis of the prognostic nomogram showed that the AUC values for 1-year and 2-year OS in the training cohort were 0.771 and 0.849, respectively (Figure 6a). We performed ROC analysis using internal and external validation cohorts to validate the nomogram further. The AUC values in the internal and external validation cohort for 1-year and 2-year OS were 0.850 and 0.799 (Figure 6b) and 0.766 and 0.767 (Figure 6c), respectively. Thus, the prognosis nomogram performed better in predicting OS at 1 and 2 years. The calibration curves of the prognostic nomogram also showed strong agreement between the predicted OS and the actual values (Figure 7).
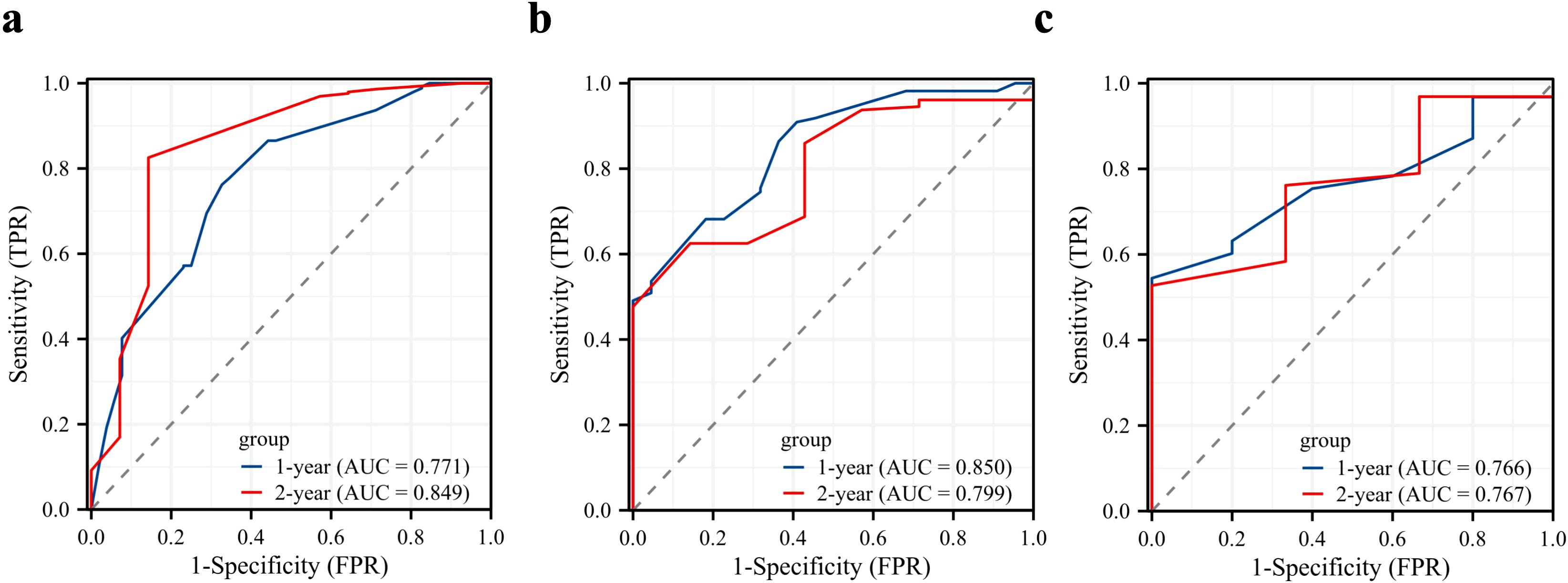
Figure 6. Validation of the prognostic model. Receiver operating characteristic curves for the prognostic nomogram in predicting the 1- and 2-year overall survival in the training cohort (a), internal validation cohort (b), and external validation cohort (c).
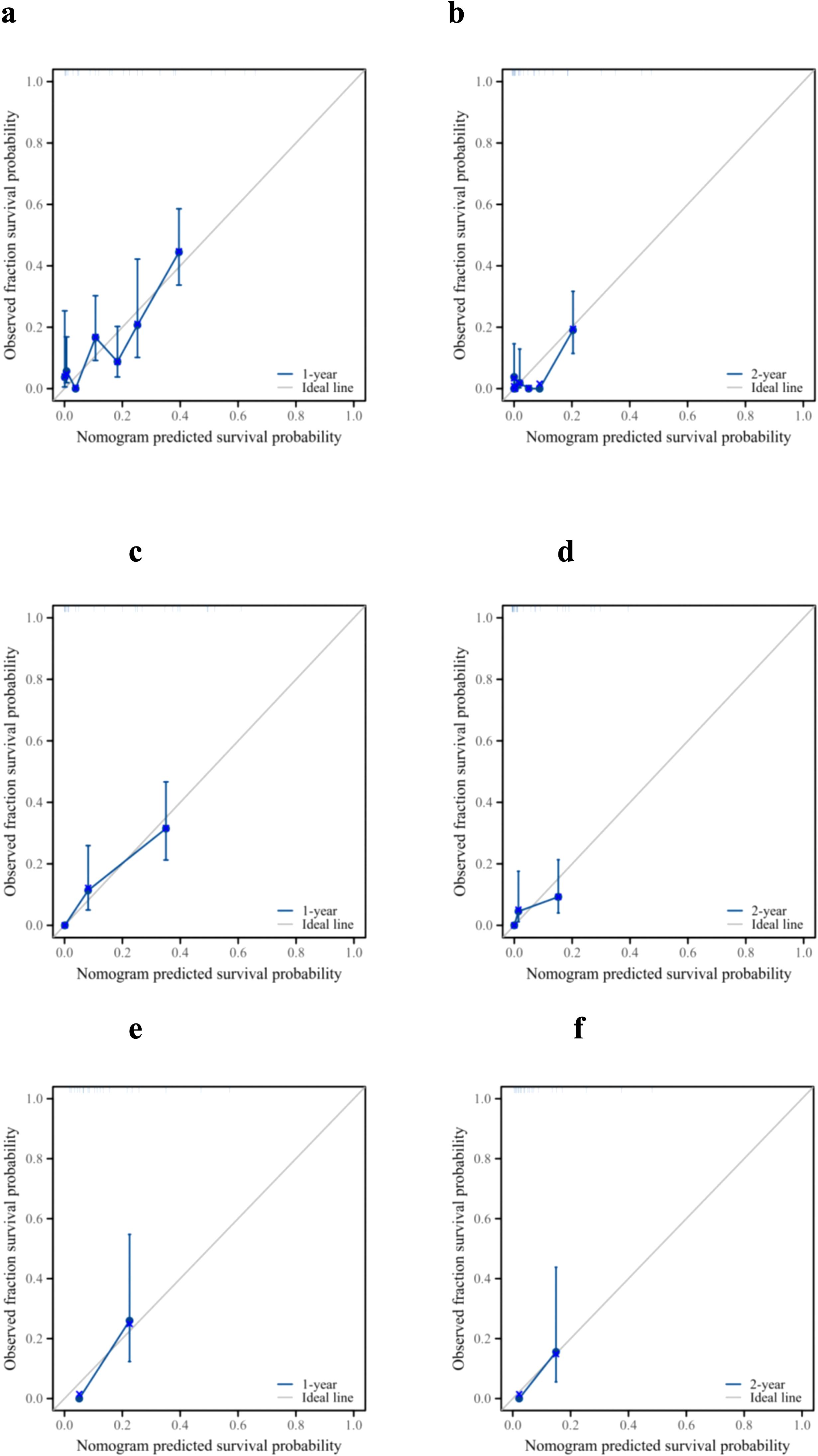
Figure 7. Prognostic performance of the model. Calibration curves for the 1- and 2-year overall survival of patients with pancreatic ductal adenocarcinoma and lung metastasis in the training cohort (a, b), internal validation cohort (c, d), and external validation cohort (e, f).
DCA was used to evaluate the clinical value of the nomogram. As shown in Figure 8, the prognostic nomogram showed significant positive gains when predicting the 1- and 2-year mortality risks of patients in the training cohort (Figures 8a, b). The 1-year mortality risk of the patients in the internal validation cohort also showed remarkable positive gains (Figure 8c). Furthermore, the nomogram showed positive gains within a range when predicting the 2-year mortality risk of patients in the internal validation cohort and the 1- and 2-year mortality risks of patients in the external validation cohort (Figure 8). Therefore, the nomogram has important clinical utility in predicting the OS of patients with PDAC and LM.
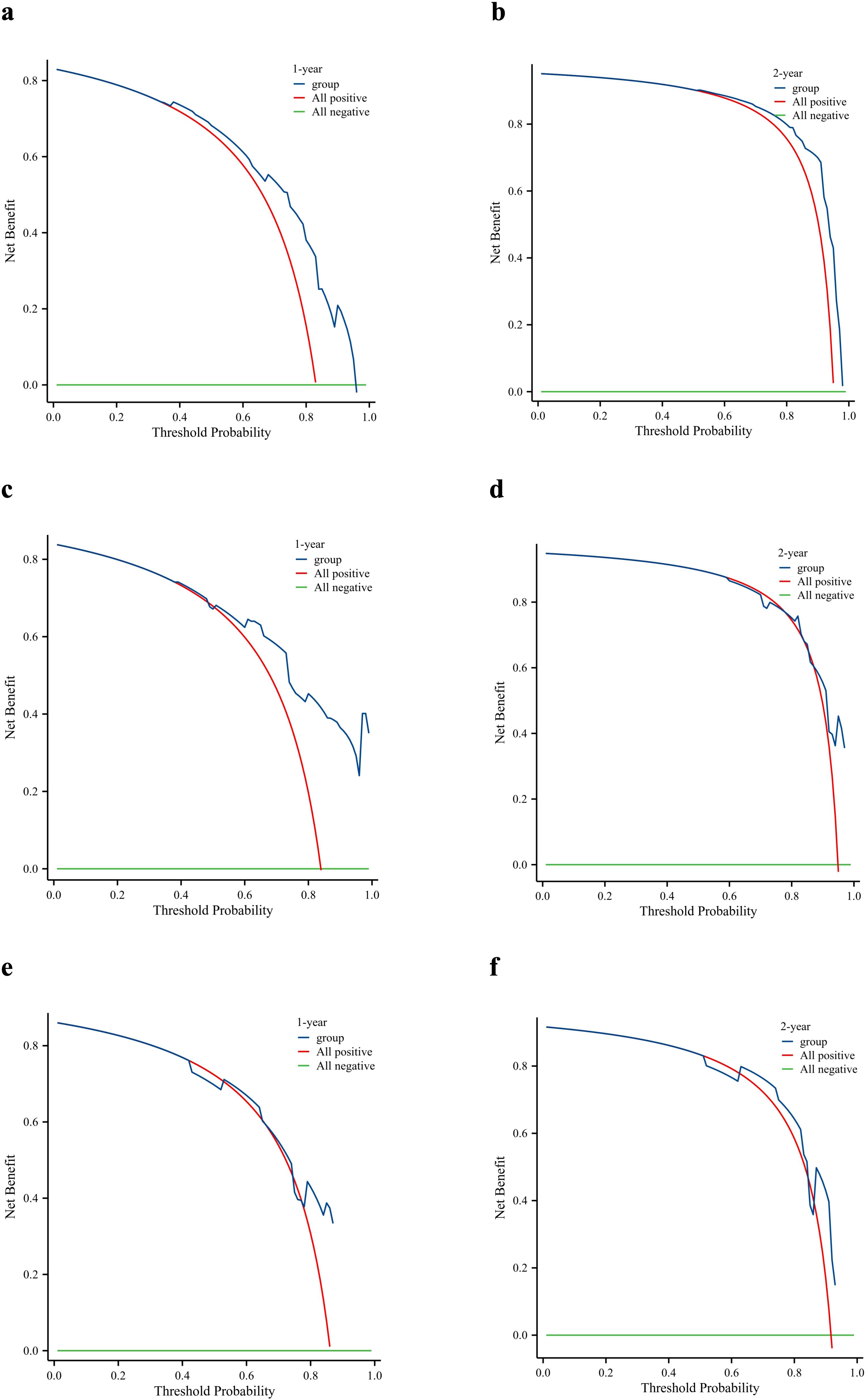
Figure 8. Clinical utility of the nomogram. Decision curve analysis of the 1- and 2-year survival benefits in the training cohort (a, b), internal validation cohort (c, d), and external validation cohort (e, f).
Finally, we divided the patients into the training, internal validation, and external validation cohorts into high- and low-risk groups according to the median risk score. Kaplan–Meier survival curves showed that all high-risk groups in these three cohorts had significantly lower prognoses than the low-risk groups (Figure 9).
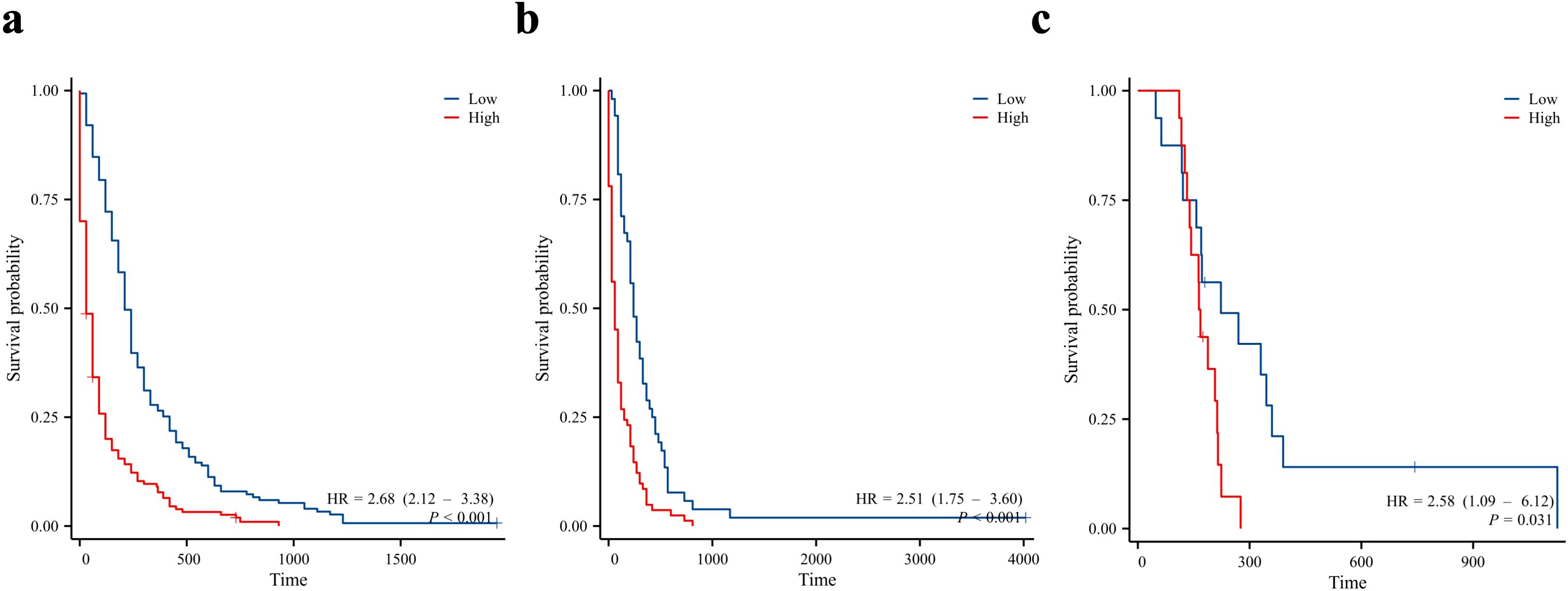
Figure 9. Kaplan-Meier curves for overall survival in patients in the low and high-risk groups. Training cohort (a), internal validation cohort (b), and external validation cohort (c).
Discussion
PDAC is the leading cause of cancer-related death worldwide with an extremely lower 5-year overall survival (32). Since most patients present atypical symptoms at early stage (33), they are often diagnosed with locally advanced (30–35%) or metastatic (50–55%) disease (5). The lung is the second most common site of metastasis, besides the liver, with the incidence of LM reported to be 4.76–12.3% (6, 7). Most patients with PDAC and LM cannot undergo surgical treatment, which greatly increases mortality. Unlike primary lung cancer, PDAC with LM showed no obvious symptoms, such as hemoptysis or cough. Therefore, timely diagnosis of LM in patients with PDAC is particularly important. In the present study, models were established using the SEER database and validated using our single-center data to help clinical diagnosis and treatment.
Our research has revealed that primary tumours in the pancreatic body and tail are more prone to develop into liver metastases (LM) compared with tumours in the pancreatic head. This may be attributed to the fact that they are frequently detected at a late stage due to the absence of obstructive jaundice. Moreover, tumours in the pancreatic body or tail are generally larger at the time of initial diagnosis and more susceptible to metastases (34). Consequently, these patients are at a higher risk of pulmonary metastasis, which is in line with the results of previous studies. Patients with larger tumours at the primary site are more likely to develop distant metastases. In combination with the findings of previous studies, this conclusion might only be applicable to patients in the early stages of pancreatic cancer (PC). When PC exhibits local or distant spread, it is not suitable for predicting lung metastasis; however, at this stage, the tumour size is large and the potential for lung metastasis is increased (35). Among the data extracted from the SEER database, 13.46% of patients with liver metastasis had LM, and 29.94% of those with bone metastases had LM. This finding indicates a high probability of LM in the presence of multiple distant metastases.
Surgery represents the sole curative treatment for pancreatic ductal adenocarcinoma (PDAC). Nevertheless, the PDAC diagnosis and treatment guidelines do not advocate surgical resection in patients with distant metastases. Chemotherapy is routinely carried out following the radical resection of PDAC, and the separate application of gemcitabine or fluorouracil is recommended (19). However, PDAC exhibits certain resistance to chemotherapeutic drugs, particularly gemcitabine. Studies have demonstrated that PDAC cells are more resistant to gemcitabine compared with other chemotherapeutic drugs (20, 21). This significantly restricts the use of gemcitabine and deteriorates the patient’s prognosis. Consequently, there is an urgent necessity for practical tools and concise clinical treatment options. In this respect, identifying the prognostic factors for patients with PDAC and liver metastases (LM) and developing a new tool for clinicians could enable personalised treatment options for each patient.
This study revealed that the primary site, liver metastasis, surgery, and chemotherapy were independent prognostic factors for patients with pancreatic ductal adenocarcinoma (PDAC) and liver metastasis (LM). The median overall survival (OS) of patients with isolated liver metastasis (IL) was merely 561 days (9). Early diagnosis and prompt treatment can enhance the prognosis of patients. As in previous studies (36–38), we found that the prognosis of patients who underwent surgery and chemotherapy was significantly improved. Additionally, in patients who are unable to undergo surgery, combining gemcitabine with nab paclitaxel (GnP) can substantially improve the feasibility and efficacy of surgery (39). Liver metastasis is the predominant site of distant metastasis in PDAC; thus, patients with PDAC and LM often have liver metastasis concurrently, and the prognosis of patients with multiple distant metastases is undoubtedly very poor (6). We also found that the prognosis of primary tumours in the pancreatic head was far superior to that of tumours in other sites. This might be related to the tendency of patients with pancreatic head cancer to develop jaundice during early diagnosis and treatment. It has also been demonstrated that PDACs located in the pancreatic body and tail are rich in genetic programs involved in tumour invasion and epithelial to mesenchymal transition, as well as having a poor antitumour immune response, resulting in a better prognosis of head PDAC than body and tail PDACs (40, 41).
Predictive and prognostic nomograms were constructed based on these factors. The ROC AUCs of the internal validation and external validation cohorts were high. Meanwhile, the calibration plots and DCA of the internal and external validation cohorts showed good discriminative power and clinical applicability. Therefore, these two nomograms can predict the risk and prognosis of LM in PDAC and help make individualised clinical decisions. The two prediction models derived from this study will become convenient and effective practical tools in clinical practice.
This study has some limitations. Although external validation was performed in this study, the amount of data in the external validation cohort was small; therefore, the results were not convincing. Next, neither the internal validation data from the SEER database nor the external data considered potential factors that may influence the diagnosis, such as body mass index, alcohol consumption, smoking, tumour biomarkers, and routine blood tests. Furthermore, we can add genetic testing as one of the predictive factors for diagnosis and prognosis on the basis of the clinical characteristics to enhance the reliability of the prediction (42). Despite these limitations, the nomogram remains practical, and we expect that prediction model validation will be further improved in the near future.
Conclusion
In summary, we identified risk and prognostic factors for PDAC with LM based on univariate, multivariate logistic, and Cox regression analyses. Risk factors include the primary site, tumour size, bone metastasis, and liver metastasis. Prognostic factors included the primary site, liver metastasis, surgery, and chemotherapy. We established two nomograms for the diagnosis and prognosis of PDAC with LM using R software, which can help clinicians effectively identify high-risk patients and target them individually.
Data availability statement
The raw data supporting the conclusions of this article will be made available by the authors, without undue reservation.
Ethics statement
The research program was approved by the Institutional Review Committee of the Affiliated Hospital of North Sichuan Medical College (number: 2024ER568-1). The studies were conducted in accordance with the local legislation and institutional requirements. The requirement for informed consent was waived because it’s a retrospective study. The manuscript presents research on animals that do not require ethical approval for their study.
Author contributions
YC: Data curation, Formal analysis, Investigation, Methodology, Software, Validation, Writing – original draft. XL: Data curation, Formal analysis, Software, Writing – original draft. JY: Data curation, Formal analysis, Methodology, Writing – original draft. JSL: Conceptualization, Investigation, Resources, Writing – original draft. JDL: Resources, Writing – original draft. PY: Resources, Writing – original draft. BW: Resources, Writing – original draft. GZ: Resources, Writing – original draft. DD: Resources, Writing – original draft. YL: Funding acquisition, Resources, Writing – original draft. SY: Methodology, Resources, Writing – original draft. LM: Funding acquisition, Resources, Writing – original draft. CL: Conceptualization, Data curation, Project administration, Writing – review & editing, Funding acquisition, Supervision.
Funding
The author(s) declare that financial support was received for the research and/or publication of this article. This work was supported by the Nanchong City Science and Technology Bureau and School Strategic Cooperation Project (grant no. 22SXQT0057) and the Youth Project of the University-level Scientific Research Development Fund of North Sichuan Medical College (grant no. CBY22-QNA07).
Conflict of interest
The authors declare that the research was conducted in the absence of any commercial or financial relationships that could be construed as a potential conflict of interest.
Generative AI statement
The author(s) declare that no Generative AI was used in the creation of this manuscript.
Publisher’s note
All claims expressed in this article are solely those of the authors and do not necessarily represent those of their affiliated organizations, or those of the publisher, the editors and the reviewers. Any product that may be evaluated in this article, or claim that may be made by its manufacturer, is not guaranteed or endorsed by the publisher.
References
1. Halbrook CJ, Lyssiotis CA, Pasca di Magliano M, and Maitra A. Pancreatic cancer: Advances and challenges. Cell. (2023) 186(8):1729–54. doi: 10.1016/j.cell.2023.02.014
2. Wood LD, Canto MI, Jaffee EM, and Simeone DM. Pancreatic cancer: pathogenesis, screening, diagnosis, and treatment. Gastroenterology. (2022) 163(2):386–402.e1. doi: 10.1053/j.gastro.2022.03.056
3. Chen X, Yi B, Liu Z, Zou H, Zhou J, Zhang Z, et al. Global, regional and national burden of pancreatic cancer, 1990 to 2017: Results from the Global Burden of Disease Study 2017. Pancreatology. (2020) 20(3):462–9. doi: 10.1016/j.pan.2020.02.011
4. Mercanti L, Sindaco M, Mazzone M, Di Marcantonio MC, Piscione M, Muraro R, et al. PDAC, the influencer cancer: cross-talk with tumor microenvironment and connected potential therapy strategies. Cancers (Basel). (2023) 15(11):2923. doi: 10.3390/cancers15112923
5. Park W, Chawla A, and O’Reilly EM. Pancreatic cancer: A review. Jama. (2021) 326(9):851–62. doi: 10.1001/jama.2021.13027
6. Chapa-Azuela Ó, Corona-Torres MJ, Ayala-Moreno EA, Arroyo-Paredes PS, Pérez-Torres E, Rodríguez-Báez A, et al. Current status of pancreatic cancer with oligometastasis. Cir Cir. (2023) 91(2):262–7. doi: 10.24875/ciru.22000364
7. Adekolujo OS, Wahab A, Akanbi MO, Oyasiji T, Hrinczenko B, and Alese OB. Isolated pulmonary metastases in pancreatic ductal adenocarcinoma: a review of current evidence. Cancer Biol Ther. (2023) 24(1):2198479. doi: 10.1080/15384047.2023.2198479
8. Giovannetti E, van der Borden CL, Frampton AE, Ali A, Firuzi O, and Peters GJ. Never let it go: Stopping key mechanisms underlying metastasis to fight pancreatic cancer. Semin Cancer Biol. (2017) 44:43–59. doi: 10.1016/j.semcancer.2017.04.006
9. Ebrahimi A, Cham J, Puglisi L, De Shadarevian M, Hermel DJ, Spierling Bagsic SR, et al. Do patients with metastatic pancreatic adenocarcinoma to the lung have improved survival? Cancer Med. (2023) 12(9):10243–53. doi: 10.1002/cam4.5751
10. Siegel RL, Miller KD, Wagle NS, and Jemal A. Cancer statistics. CA Cancer J Clin. (2023) 73(1):17–48. doi: 10.3322/caac.21763
11. Zins M, Matos C, and Cassinotto C. Pancreatic adenocarcinoma staging in the era of preoperative chemotherapy and radiation therapy. Radiology. (2018) 287(2):374–90. doi: 10.1148/radiol.2018171670
12. Bae JS, Kim JH, Joo I, Chang W, and Han JK. MDCT findings predicting post-operative residual tumor and survival in patients with pancreatic cancer. Eur Radiol. (2019) 29(7):3714–24. doi: 10.1007/s00330-019-06140-9
13. Chen FM, Ni JM, Zhang ZY, Zhang L, Li B, and Jiang CJ. Presurgical evaluation of pancreatic cancer: A comprehensive imaging comparison of CT versus MRI. AJR Am J Roentgenol. (2016) 206(3):526–35. doi: 10.2214/ajr.15.15236
14. Hu S, Zhang J, Zuo C, Cheng C, Liu Q, and Sun G. (18)F-FDG-PET/CT findings in pancreatic metastasis. Radiol Med. (2015) 120(10):887–98. doi: 10.1007/s11547-014-0473-1
15. Bugazia D, Al-Najjar E, Esmail A, Abdelrahim S, Abboud K, Abdelrahim A, et al. Pancreatic ductal adenocarcinoma: the latest on diagnosis, molecular profiling, and systemic treatments. Front Oncol. (2024) 14:1386699. doi: 10.3389/fonc.2024.1386699
16. Kamyabi N and Bernard V and Maitra A. Liquid biopsies in pancreatic cancer. Expert Rev Anticancer Ther. (2019) 19(10):869–78. doi: 10.1080/14737140.2019.1670063
17. Abdullah E, Mohamed B, and Maen A. Pancreatic Tumorigenesis: Precursors, Genetic Risk Factors and Screening. In: Emad Hamdy G, editor. Pancreatic Cancer. IntechOpen, Rijeka (2023) 29(11):8693–719. doi: 10.5772/intechopen.110887
18. Homma Y, Endo I, Matsuyama R, Sho M, Mizuno S, Seyama Y, et al. Outcomes of lung metastasis from pancreatic cancer: A nationwide multicenter analysis. J Hepatobil Pancreat Sci. (2022) 29(5):552–61. doi: 10.1002/jhbp.1127
19. Jiang S, Fagman JB, Ma Y, Liu J, Vihav C, Engstrom C, et al. A comprehensive review of pancreatic cancer and its therapeutic challenges. Aging (Albany NY). (2022) 14(18):7635–49. doi: 10.18632/aging.204310
20. Zeng S, Pöttler M, Lan B, Grützmann R, and Pilarsky C and Yang H. Chemoresistance in pancreatic cancer. Int J Mol Sci. (2019) 20(18):4504. doi: 10.3390/ijms20184504
21. Gautam SK, Basu S, Aithal A, Dwivedi NV, Gulati M, and Jain M. Regulation of pancreatic cancer therapy resistance by chemokines. Semin Cancer Biol. (2022) 86:69–80. doi: 10.1016/j.semcancer.2022.08.010
22. Che WQ, Li YJ, Tsang CK, Wang YJ, Chen Z, Wang XY, et al. How to use the Surveillance, Epidemiology, and End Results (SEER) data: research design and methodology. Mil Med Res. (2023) 10(1):50. doi: 10.1186/s40779-023-00488-2
23. Balachandran VP, Gonen M, Smith JJ, and DeMatteo RP. Nomograms in oncology: more than meets the eye. Lancet Oncol. (2015) 16(4):e173–80. doi: 10.1016/s1470-2045(14)71116-7
24. Ohori Tatsuo G, Riu Hamada M, Gondo T, and Hamada R. Nomogram as predictive model in clinical practice. Gan To Kagaku Ryoho. (2009) 36(6):901–6.
25. Wang X, Lu J, Song Z, Zhou Y, Liu T, and Zhang D. From past to future: Bibliometric analysis of global research productivity on nomogram (2000-2021). Front Public Health. (2022) 10:997713. doi: 10.3389/fpubh.2022.997713
26. Sternberg CN. Are nomograms better than currently available stage groupings for bladder cancer? J Clin Oncol. (2006) 24(24):3819–20. doi: 10.1200/jco.2006.07.1290
27. Camp RL, Dolled-Filhart M, and Rimm DL. X-tile: a new bio-informatics tool for biomarker assessment and outcome-based cut-point optimization. Clin Cancer Res. (2004) 10(21):7252–9. doi: 10.1158/1078-0432.Ccr-04-0713
28. Martínez Pérez JA and Pérez Martin PS. ROC curve. Semergen. (2023) 49(1):101821. doi: 10.1016/j.semerg.2022.101821
29. Kerr KF, Brown MD, Zhu K, and Janes H. Assessing the clinical impact of risk prediction models with decision curves: guidance for correct interpretation and appropriate use. J Clin Oncol. (2016) 34(21):2534–40. doi: 10.1200/jco.2015.65.5654
30. Fitzgerald M, Saville B, and Lewis R. Decision curve analysis. JAMA. (2015) 313(4):409–10. doi: 10.1001/jama.2015.37
31. Fenlon C, O’Grady L, Doherty ML, and Dunnion J. A discussion of calibration techniques for evaluating binary and categorical predictive models. Prev Vet Med. (2018) 149:107–14. doi: 10.1016/j.prevetmed.2017.11.018
32. Zhu J, Shi Y, Lan S, Wang J, Jiang F, Tang C, et al. Dissection of pyroptosis-related prognostic signature and CASP6-mediated regulation in pancreatic adenocarcinoma: new sights to clinical decision-making. Apoptosis. (2023) 28(5-6):769–82. doi: 10.1007/s10495-023-01823-7
33. Franck C, Müller C, Rosania R, Croner RS, Pech M, and Venerito M. Advanced pancreatic ductal adenocarcinoma: moving forward. Cancers (Basel). (2020) 12(7):1955. doi: 10.3390/cancers12071955
34. Mackay TM, van Erning FN, van der Geest LGM, de Groot JWB, Haj Mohammad N, Lemmens VE, et al. Association between primary origin (head, body and tail) of metastasised pancreatic ductal adenocarcinoma and oncologic outcome: A population-based analysis. Eur J Cancer. (2019) 106:99–105. doi: 10.1016/j.ejca.2018.10.008
35. Ansari D, Bauden M, Bergström S, Rylance R, Marko-Varga G, and Andersson R. Relationship between tumour size and outcome in pancreatic ductal adenocarcinoma. Br J Surg. (2017) 104(5):600–7. doi: 10.1002/bjs.10471
36. Yun WG, Kwon W, Han Y, Sohn HJ, Kim HS, Lee M, et al. Can surgical resection of metastatic lesions be beneficial to pancreatic ductal adenocarcinoma patients with isolated lung metastasis? Cancers (Basel). (2022) 14(9):2067. doi: 10.3390/cancers14092067
37. Mashiko T, Nakano A, Masuoka Y, Yamamoto S, Ozawa S, and Nakagohri T. Significance of pulmonary resection in patients with metachronous pulmonary metastasis from pancreatic ductal adenocarcinoma: a retrospective cohort study. BMC Surg. (2021) 21(1):237. doi: 10.1186/s12893-021-01236-w
38. Wahler IL, Damanakis A, Große Hokamp N, Bruns C, and Schmidt T. Therapy of locally advanced and oligometastatic pancreatic adenocarcinoma. Cancers (Basel). (2023) 15(24):5881. doi: 10.3390/cancers15245881
39. Igarashi T, Yamada S, Hoshino Y, Murotani K, Baba H, Takami H, et al. Prognostic factors in conversion surgery following nab-paclitaxel with gemcitabine and subsequent chemoradiotherapy for unresectable locally advanced pancreatic cancer: Results of a dual-center study. Ann Gastroenterol Surg. (2023) 7(1):157–66. doi: 10.1002/ags3.12613
40. Dreyer SB, Jamieson NB, Upstill-Goddard R, Bailey PJ, McKay CJ, Biankin AV, et al. Defining the molecular pathology of pancreatic body and tail adenocarcinoma. Br J Surg. (2018) 105(2):e183–91. doi: 10.1002/bjs.10772
41. Abdelrahim M, Esmail A, Kasi A, Esnaola NF, Xiu J, Baca Y, et al. Comparative molecular profiling of pancreatic ductal adenocarcinoma of the head versus body and tail. NPJ Precis Oncol. (2024) 8(1):85. doi: 10.1038/s41698-024-00571-4
Keywords: pancreatic ductal adenocarcinoma, lung metastasis, predictors, Surveillance Epidemiology and End Results (SEER) database, logistic regression, nomogram
Citation: Chen Y, Liu X, Yu J, Li J, Li J, Yi P, Wu B, Zhang G, Deng D, Li Y, Yan S, Ma L and Lan C (2025) Risk and prognostic factors of survival for patients with pancreatic ductal adenocarcinoma metastasis to lung: a cohort study. Front. Oncol. 15:1521616. doi: 10.3389/fonc.2025.1521616
Received: 02 November 2024; Accepted: 25 April 2025;
Published: 21 May 2025.
Edited by:
Liang Qiao, The University of Sydney, AustraliaReviewed by:
Yao Kong, Sun Yat-sen Memorial Hospital, ChinaYewei Zhang, Nanjing Medical University, China
Copyright © 2025 Chen, Liu, Yu, Li, Li, Yi, Wu, Zhang, Deng, Li, Yan, Ma and Lan. This is an open-access article distributed under the terms of the Creative Commons Attribution License (CC BY). The use, distribution or reproduction in other forums is permitted, provided the original author(s) and the copyright owner(s) are credited and that the original publication in this journal is cited, in accordance with accepted academic practice. No use, distribution or reproduction is permitted which does not comply with these terms.
*Correspondence: Chuan Lan, aHhsYW5jaHVhbkAxNjMuY29t