- Department of Obstetrics and Gynecology, The Second Affiliated Hospital of Soochow University, Suzhou, Jiangsu, China
Background: Elevated inflammatory markers are commonly linked to poor prognoses in cancer patients, while optimal nutritional status correlates with improved survival outcomes. This study aimed to explore the interplay between nutritional and inflammatory markers and their impact on postoperative outcomes in ovarian cancer patients through a retrospective analysis.
Methods: Data were retrospectively retrieved from patients diagnosed with ovarian cancer who required surgical intervention at the Department of Obstetrics and Gynecology. Overall survival (OS) and cancer-specific survival (CSS) were monitored during follow-up. Kaplan-Meier survival curves were employed to assess OS and CSS across different patient cohorts, evaluating the prognostic significance of nutritional and inflammatory markers. Nomograms for predicting OS and CSS at one, three, and five years postoperatively were constructed, followed by external validation.
Results: The prognostic nutritional index (PNI) and Naples prognostic score (NPS) exhibited a significant correlation with OS and CSS in postoperative ovarian cancer patients (p < 0.05). Analysis indicated that patients with a PNI > 51.2 demonstrated the most favorable survival outcomes. Furthermore, those with a low-NPS (L-NPS) had notably better survival rates compared to their high-NPS (H-NPS) counterparts. Independent OS predictors included age, PNI, NPS, histological type, tumor size, targeted therapy, and diabetes. Similarly, the CSS prediction model incorporated age, NPS, tumor size, targeted therapy, and diabetes. The nomograms demonstrated robust predictive accuracy for three- and five-year survival, though one-year calibration curves showed limited agreement. Despite slightly reduced external validation performance compared to the initial sample, the model maintained strong predictive capability.
Conclusions: The nutritional inflammatory index serves as a key independent prognostic marker for OS and CSS in ovarian cancer patients. Nomograms based on PNI and NPS provide valuable prognostic insights for postoperative management. Incorporating these indices into clinical practice could improve patient stratification and guide personalized treatment plans.
1 Introduction
Ovarian cancer ranks among the three most prevalent gynecologic malignancies and remains a leading cause of mortality in this category. Its typically late-stage diagnosis, often resulting from the absence of distinct symptoms and the lack of early-stage screening, significantly compromises clinical outcomes (1, 2). Consequently, the efficacy of current treatments and the prognosis for ovarian cancer remain suboptimal. Modern therapeutic strategies must be informed by molecular profiling, as molecular biology plays a central role in shaping treatment paradigms (3). Increasing evidence underscores the complex interplay between nutrition, inflammation, and cancer prognosis, spurring ongoing investigations into these interactions across various malignancies, including ovarian cancer.
Several scoring systems that assess inflammation and nutritional status, including the lymphocyte-to-monocyte ratio (LMR), systemic inflammatory score (SIS), systemic immune-inflammatory index (SII), neutrophil-to-lymphocyte ratio (NLR), and COUNT, have demonstrated clinical relevance in gynecological cancers (4, 5). Among these, the prognostic nutritional index (PNI), Naples prognostic score (NPS), NLR, and LMR are particularly notable as nutritional-inflammation markers. PNI, initially introduced by Onodera (6), serves as a key indicator of both nutritional and inflammatory conditions, calculated from serum albumin levels and lymphocyte counts. Recent studies have revealed a strong correlation between PNI and prognosis in various cancers, as well as conditions like myocardial infarction and congenital heart disease (7, 8). Similarly, the NPS, developed in 2017 (9), integrates both inflammatory and nutritional biomarkers—albumin, total cholesterol (TC), NLR, and LMR—offering a comprehensive assessment of immune and nutritional status. The NPS has been validated as a reliable predictor of prognosis in multiple cancers, including colon, gallbladder, endometrial, and lung cancers (10–12). Emerging data emphasize the prognostic role of nutritional status in cancer patients, suggesting that markers like PNI and NPS may notably impact OS and cancer-specific survival (CSS) in ovarian cancer.
Elevated inflammatory markers often correlate with poor prognosis in cancer patients, while improved nutritional status is linked to enhanced survival outcomes. However, the specific influence of nutritional markers on ovarian cancer prognosis remains inadequately studied, revealing a notable research gap. This retrospective study seeks to explore the relationship between nutritional inflammatory markers and postoperative prognosis in ovarian cancer patients.
2 Methods
2.1 Study population
Between January 2022 and December 2023, this study enrolled 199 patients from the Department of Obstetrics and Gynecology at the Second People’s Hospital Affiliated to Suzhou University, along with 120 patients from the Department of Obstetrics and Gynecology at Binhai County People’s Hospital in Yancheng City, who were diagnosed with ovarian cancer and required surgical intervention. The study received approval and oversight from the Ethics Committee of the Second People’s Hospital Affiliated of Soochow University (Approval No. JD-LK-2022-079-01) and Binhai County People’s Hospital in Yancheng City (Approval No. 2024-BYKYLL-041). The study was conducted in accordance with the revised 2013 Helsinki Declaration. Written informed consent was obtained from each participant or their legal representative.
The inclusion criteria were as follows: (1) age ≥18 years; (2) a confirmed diagnosis of ovarian cancer requiring surgical intervention, as verified by a physician; and (3) provision of signed informed consent by the patient.
The exclusion criteria were as follows: (1) incomplete laboratory data; (2) a diagnosis of ovarian cancer without surgical treatment; and (3) refusal to provide informed consent.
2.2 Clinical data collection and follow-up
The study included a broad spectrum of variables: (1) Demographic data: age and body mass index (BMI); (2) Laboratory markers: TC, albumin, neutrophil, lymphocyte, and monocyte counts, carbohydrate antigen 125 (CA125), CA199, human epididymis secretory protein 4 (HE4), and carcinoembryonic antigen (CEA); (3) Comorbid conditions: hypertension and diabetes; (4) Tumor characteristics: tumor size, type of surgery (PDS or IDS), residual tumor post-surgery (yes or no), coronavirus disease 2019 (COVID-19) infection (yes or no), pathological type, tissue type, lymph node metastasis, International Federation of Gynecology and Obstetrics (FIGO) grade, differentiation status, history of chemotherapy, and targeted therapy; and (5) Length of hospital stay. Trained professionals collected laboratory markers and tumor characteristics. Biochemical analyses were conducted using a standardized automated system under the supervision of laboratory physicians, while pathologists determined tumor diagnoses and grading. Data on preexisting conditions were extracted from patients’ medical histories, and the hospital discharge system recorded the length of stay. Follow-up data were obtained through outpatient visits or telephone interviews. Survival outcomes were assessed at one, three, and five years post-surgery. The primary endpoints of the study were OS and CSS.
The study stratified variables according to criteria established by X-tile software (Figure 1). Age was categorized into three groups: ≤59 years, 60–69 years, and ≥70 years. Tumor size was classified as <8 cm, 8–12 cm, and >12 cm.
2.3 Calculation of nutritional inflammatory markers
The nutritional inflammatory markers, NLR, LMR, PNI, and NPS, were calculated using the following formulas: (1) NLR as the ratio of neutrophil to lymphocyte counts; (2) LMR as the ratio of lymphocyte to monocyte counts; (3) PNI as albumin (g/L) plus five times the lymphocyte count; and (4) NPS, determined based on serum albumin, TC, NLR, and LMR levels following established methodologies. Using X-tile software, PNI was stratified into three categories: <46.1, 46.1–51.2, and >51.2 (Figure 1). NPS was assigned based on these criteria: (1) a score of 0 for serum albumin ≥40 g/L, TC >4.68 mmol/L, NLR <2.96, and LMR >4.44; (2) a score of 1 for serum albumin <40 g/L, TC ≤4.68 mmol/L, NLR ≥2.96, and LMR ≤4.44. The total NPS was calculated by summing the scores of these four parameters. Cumulative NPS values of 0–2 were categorized as low-NPS (L-NPS), while scores of 3–4 were defined as high-NPS (H-NPS).
2.4 Statistical analysis
The baseline characteristics of all enrolled patients were stratified according to survival outcomes. Non-normally distributed variables were expressed as quartile ranges and analyzed using the Wilcoxon rank-sum test. Categorical variables were presented as percentages and compared with chi-square tests. Univariate and multivariate Cox regression models were applied to calculate hazard ratios (HRs) and assess the independence of nutritional inflammatory indices as prognostic factors for OS and CSS in ovarian cancer patients. Kaplan-Meier curves were generated to illustrate OS and CSS across patient groups. To further examine the relationship between nutritional inflammatory markers and survival outcomes, restricted cubic spline (RCS) plots were constructed. A nomogram incorporating statistically significant markers from the multivariate Cox regression was developed. The nomogram’s diagnostic performance was evaluated using receiver operating characteristic (ROC) curves and calibration plots. ROC curves were also used to assess the prognostic value of different markers for ovarian cancer patients. External validation was performed concurrently. Finally, stratified analyses were conducted to explore the effects of various nutritional inflammatory indices on subpopulations of ovarian cancer patients. Statistical analyses were performed with R software (version 4.3.0) and STATA 17.0 (64-bit). A bilateral p-value <0.05 was considered statistically significant.
3 Results
3.1 Demographic and clinical characteristics of patients with ovarian cancer
A cohort of 199 ovarian cancer patients who underwent surgical treatment at the Second Affiliated Hospital of Suzhou University was analyzed. Of these, 141 patients (70.85%) survived, while 58 patients (29.15%) succumbed to the disease. The demographic and clinical characteristics of both groups were summarized in Table 1. Increased mortality risk was associated with advanced age, lower albumin levels, reduced lymphocyte counts, decreased PNI, elevated neutrophil counts, higher NLR, increased HE4, H-NPS, and postoperative tumor residue. Additional high-risk factors included high-grade histology, larger tumor size, FIGO stage IV, poor tumor differentiation, absence of targeted therapy, and a history of diabetes. Statistically significant differences between survivors and deceased patients were observed for all these variables (all p < 0.05). Furthermore, deceased patients had longer hospital stays compared to survivors. No significant differences were found regarding monocyte counts, CA125, CA199, CEA, tumor histological type, surgical procedure, COVID-19 infection, or lymphatic metastasis. The lack of statistical significance in postoperative survival and prognosis may be attributed to the small sample size of this study.
An analysis of 120 patients from Binhai County People’s Hospital (Table 1) yielded results consistent with those from the Second Affiliated Hospital to Suzhou University. Factors including advanced age, elevated BMI, low albumin levels, reduced lymphocyte count, increased NLR, elevated HE4, low PNI, H-NPS, lymphocyte metastasis, poor differentiation, absence of radiotherapy or targeted therapy, residual tumor post-surgery, and comorbid diabetes were all significantly associated with a higher risk of mortality (all p<0.05). In contrast, neutrophil levels did not exhibit statistical significance in the Binhai County cohort, which may be attributed to the smaller sample size in our study.
3.2 Identification of prognostic factors affecting OS and CSS in ovarian cancer postoperatively
Kaplan-Meier survival analysis revealed significant associations between PNI, NPS, and survival outcomes in ovarian cancer patients (p < 0.05), as depicted in Figure 2. Patients with PNI > 51.2 exhibited the best OS, whereas those with PNI < 46.1 had the poorest prognosis (Figures 2A-1). Likewise, survival outcomes were significantly better for patients with L-NPS compared to those with H-NPS (Figure 2A-2). These patterns were similarly observed for CSS, as shown in Figures 2A-3, 2A-4. Consistent results were obtained from the data of Binhai County People’s Hospital (Figures 2B-1–4).
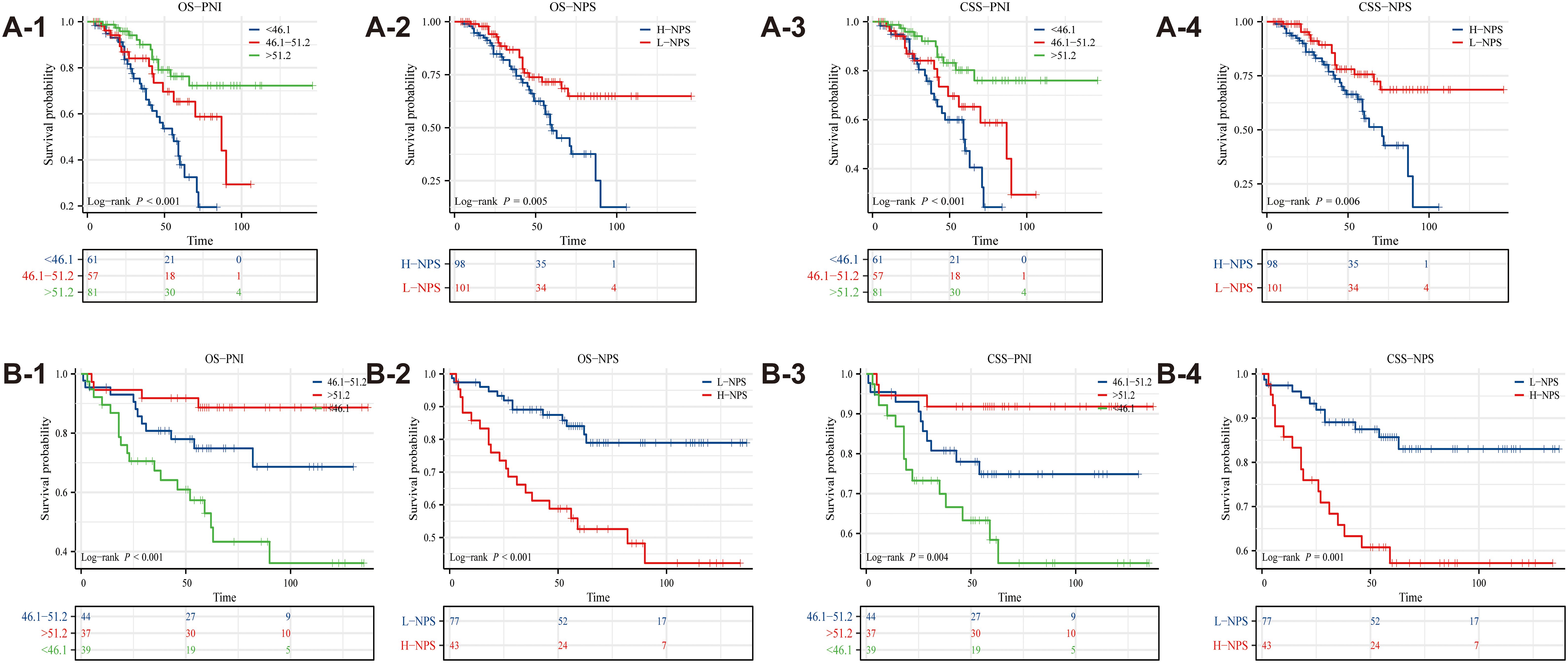
Figure 2. Kaplan-Meier survival curves evaluated the effects of PNI and NPS on survival outcomes (A1-A4: The Second People’s Hospital Affiliated of Soochow University; B1-B4: Binhai County People's Hospital).
Univariate and multivariate Cox regression analyses were conducted to identify factors influencing OS and CSS. For OS, independent risk factors included age, PNI, NPS, tissue type, tumor size, targeted therapy status, and diabetes (Table 2). Specifically, patients with PNI between 46.1 and 51.2 demonstrated improved survival outcomes compared to those with PNI <46.1 (HR = 0.461; 95% CI, 0.234–0.864; p = 0.036), while those with PNI >51.2 exhibited even more pronounced protective effects (HR = 0.357; 95% CI, 0.095–0.538; p = 0.025). In contrast, patients with H-NPS had significantly worse survival compared to those with L-NPS (HR = 1.576; 95% CI, 1.252–2.719; p = 0.013). For CSS, multivariate Cox regression analysis (Table 3) identified age, NPS, tumor size, targeted therapy status, and diabetes as independent risk factors. Consistent with OS, patients with H-NPS experienced poorer outcomes compared to those with L-NPS (HR = 1.654; 95% CI, 1.278–3.138; p = 0.031).
3.3 Relationship between nutritional inflammatory markers and survival prognosis of postoperative patients with ovarian cancer
An RCS diagram (Figure 3) was constructed to explore the relationship between nutritional inflammatory markers and survival prognosis in postoperative ovarian cancer patients. OS analysis indicated a negative correlation between PNI and LMR with patient prognosis, suggesting that higher values of these indices were associated with improved survival outcomes (Figures 3A, C). In contrast, NLR exhibited a positive correlation with prognosis, with elevated NLR values linked to poorer survival (Figure 3B). The CSS analysis mirrored the findings from OS, revealing a negative correlation between both PNI and LMR with prognosis (Figures 3D, F), while NLR remained positively correlated, indicating worse outcomes as its value increased (Figure 3E). Additional analyses included markers such as albumin, TC, CA125, CA199, CEA, and HE4 (Supplementary Figure S1).
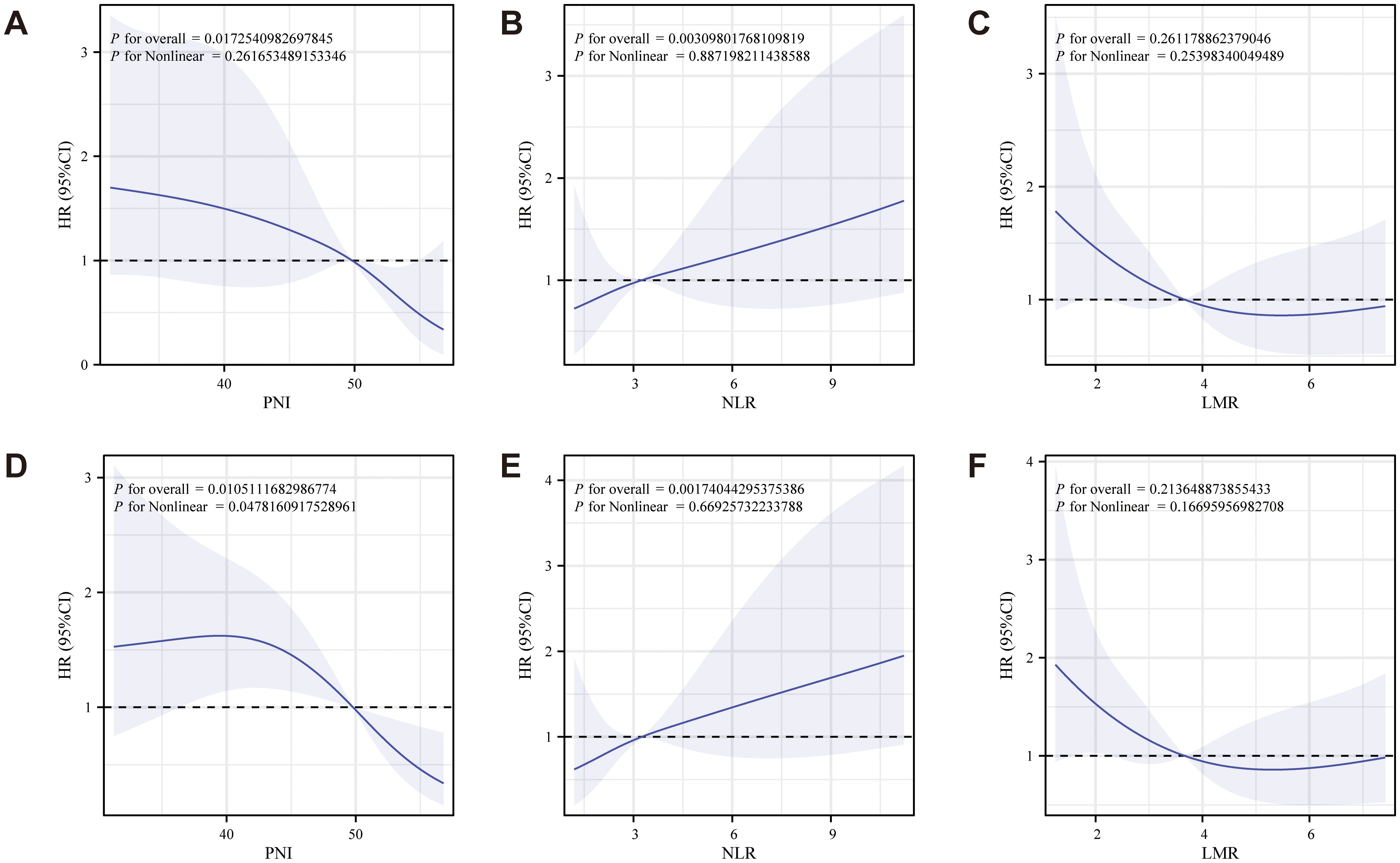
Figure 3. RCS diagram of the relationship between PNI, NLR, LMR and survival prognosis of patients with ovarian cancer after surgery (A-C: OS analysis showed that PNI and LMR were negatively correlated with prognosis, while NLR was positively correlated with prognosis; D-F: The CSS analysis showed that PNI and LMR were negatively associated with prognosis, while NLR remained positively associated).
3.4 Construction and evaluation of the nomogram
Univariate and multivariate Cox regression analyses identified seven independent predictors of OS: age, PNI, NPS, tissue type, tumor size, targeted therapy use, and diabetes presence (p < 0.05; Table 2). Similarly, five variables were determined to be independent predictors of CSS (p < 0.05; Table 3). Nomograms incorporating these predictors were developed to estimate one-, three-, and five-year OS and CSS (Figures 4A, D). Risk scores for each factor in the nomogram, shown in Figure 4, indicated that higher scores corresponded to increased mortality risk. The predictive accuracy of the nomograms was assessed, yielding a concordance (C-index) of 0.715 for the OS nomogram and 0.704 for the CSS nomogram. ROC curves for one-, three-, and five-year OS and CSS were plotted, with the area under the curve (AUC) values for OS being 0.722, 0.801, and 0.890, respectively (Figure 4B). For CSS, the AUC values were 0.708, 0.827, and 0.840, respectively (Figure 4E). Calibration curves for three- and five-year predictions closely aligned with the diagonal, indicating high consistency and reliable calibration, whereas the one-year calibration curves deviated, suggesting lower robustness in predicting one-year outcomes (Figures 4C, F).
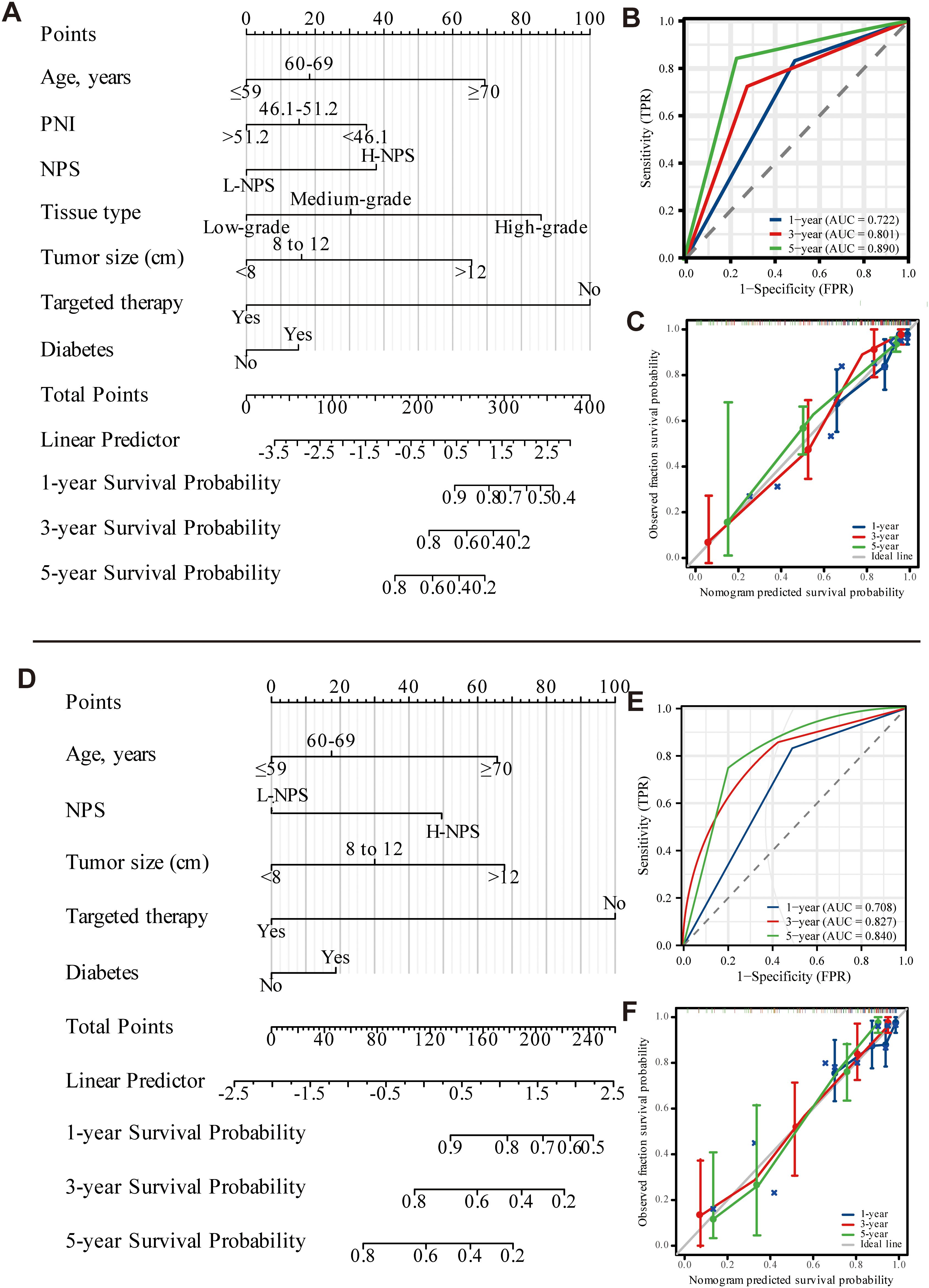
Figure 4. Nomogram of OS and CSS 1, 3, and 5 years after ovarian cancer surgery (A-C: OS; D-F: CSS).
External validation was performed using data from 120 patients at Binhai County People’s Hospital, applying the same characteristic variables to construct a nomogram for predicting 1-, 3-, and 5-year OS and CSS (Figures 5A, B). The nomogram’s risk scores for each factor were presented in Figure 5, where higher scores correlated with an increased risk of mortality. Predictive accuracy was assessed by evaluating the C-index, which was 0.735 for the OS nomogram and 0.714 for the CSS nomogram. ROC curves for 1-year, 3-year, and 5-year OS and CSS were also generated. For OS, AUC values were 0.753, 0.782, and 0.724, respectively (Figure 5A-2), while for CSS, AUC values were 0.743, 0.766, and 0.708 (Figure 5B-2). Calibration curves for 3- and 5-year predictions closely approximated the diagonal, reflecting strong consistency and reliable calibration. In contrast, the 1-year calibration curve showed notable deviation from the diagonal, indicating reduced predictive accuracy for the 1-year outcome (Figures 5A-3, 5B-3). A comparison with data from the Second People’s Hospital of Suzhou University revealed that while model performance at Binhai County People’s Hospital was somewhat lower, the evaluation metrics still confirmed the model’s overall effectiveness.
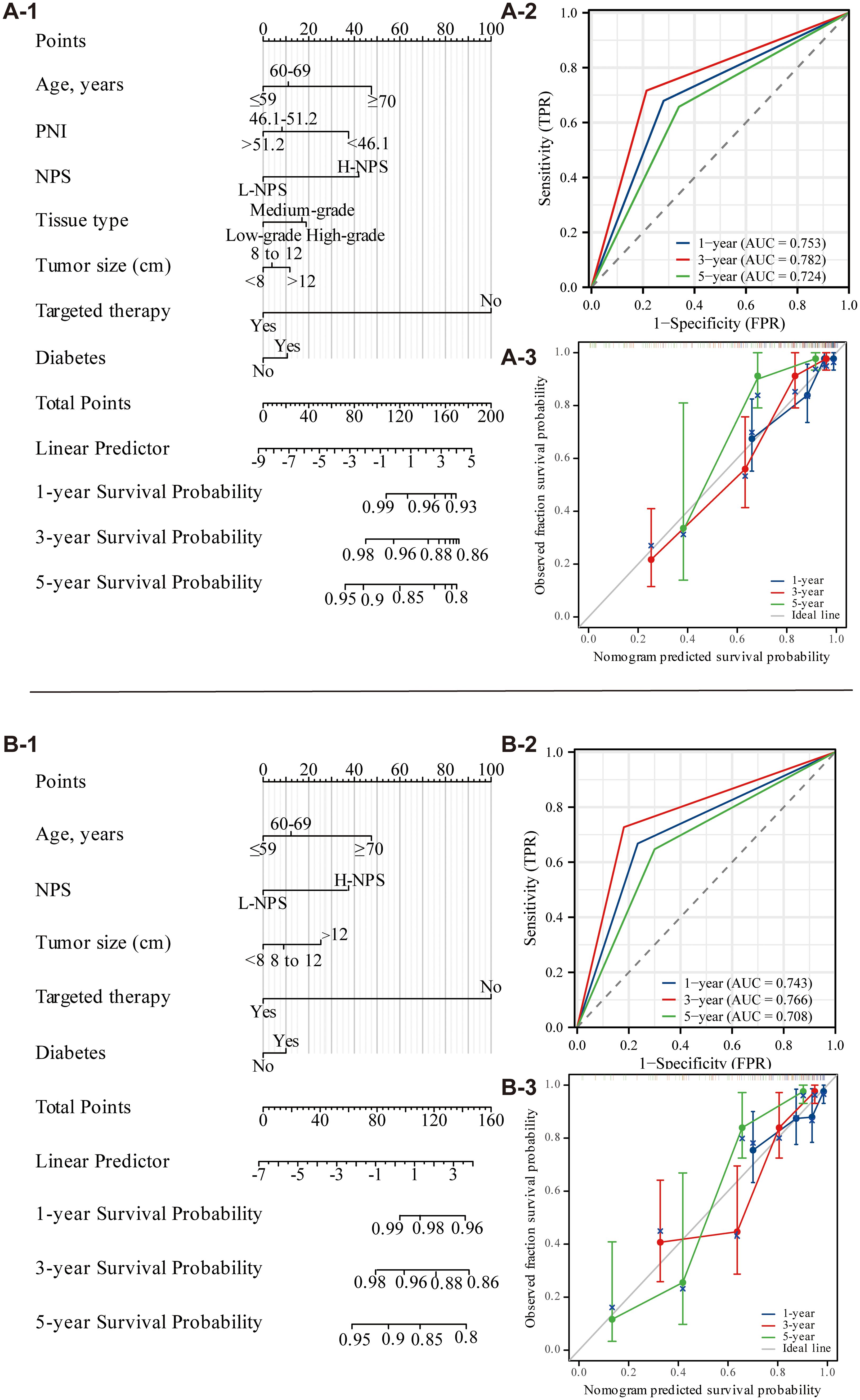
Figure 5. Nomogram of OS and CSS 1, 3, and 5 years after ovarian cancer surgery (external validation; A: OS; B: CSS).
3.5 Predictive value of nutritional inflammatory markers for survival and prognosis of postoperative patients with ovarian cancer
Multivariate Cox regression analysis was used to develop a diagnostic nomogram, with correlated variables plotted alongside the ROC curve to evaluate its predictive accuracy for survival and prognosis in postoperative ovarian cancer patients (Figure 6). For OS, the model exhibited the highest predictive value, yielding an AUC of 0.910 (Figure 6B-1). In comparison, the AUCs for individual variables were 0.678 for PNI, 0.627 for NPS, 0.424 for tissue type, 0.743 for tumor size, and 0.703 for targeted therapy (Figure 6A-1). In the CSS analysis, the model again demonstrated the highest predictive value, achieving an AUC of 0.844 (Figure 6B-2). The AUCs for other variables were 0.612 for NPS, 0.722 for tumor size, and 0.685 for targeted therapy (Figure 6A-2). These results confirm the nomogram’s significant diagnostic utility, highlighting its capacity to accurately predict survival and prognosis in postoperative ovarian cancer patients.
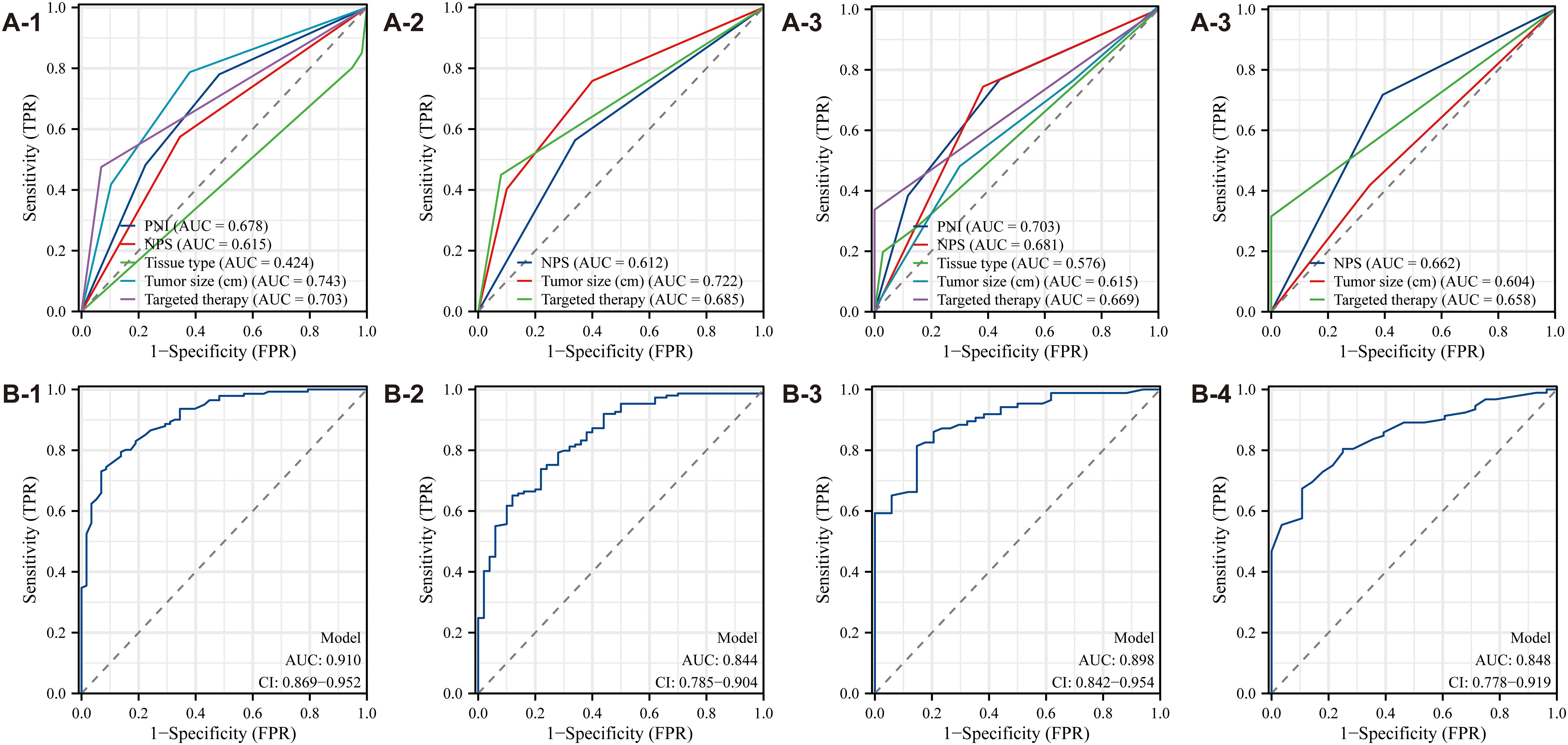
Figure 6. ROC curve of the diagnostic nomogram model (A1-A4: The Second People’s Hospital Affiliated of Soochow University; B1-B4: Binhai County People's Hospital).
Data analysis from Binhai County People’s Hospital revealed that the OS model exhibited the highest predictive value, with an AUC of 0.898 (Figure 6B-3). In contrast, the AUC values for other variables were as follows: PNI, 0.703; NPS, 0.681; tissue type, 0.576; tumor size, 0.618; and targeted therapy, 0.669 (Figure 6A-3). Likewise, for CSS, the model again demonstrated the highest predictive value, with an AUC of 0.848 (Figure 6B-4). The AUC values for additional variables were: NPS, 0.662; tumor size, 0.604; and targeted therapy, 0.658 (Figure 6A-4). These results further substantiate the diagnostic utility of the nomogram, reinforcing its robust predictive capability for survival and prognosis in postoperative ovarian cancer patients.
3.6 Subgroup analysis
Subgroup analysis of OS and CSS in postoperative ovarian cancer patients was performed based on factors such as age, tumor size, histological type, and tissue characteristics to assess survival prognosis. OS analysis using the NPS (Figures 7A–C) revealed that patients aged 60–69 years with H-NPS faced a significantly higher mortality risk than those with L-NPS (HR = 3.534; 95% CI, 1.230–10.151; p = 0.019). Similar patterns were observed in patients with tumors >12 cm, endometrioid adenocarcinoma, FIGO grade I, as well as those undergoing surgery and chemotherapy (all p < 0.05). Additionally, among patients aged 60–69 years, those with tumors >12 cm, serous adenocarcinoma, low-grade histology, no lymphatic metastasis, absence of targeted therapy, and without hypertension or diabetes, demonstrated the lowest mortality when PNI >51.2 (p < 0.05) (Figures 7D–F). In terms of CSS, the NPS analysis yielded comparable results (Figures 7G–I), with higher mortality rates in patients with tumors >12 cm and endometrioid adenocarcinoma, regardless of hypertension, surgical procedure, or residual tumor presence (all p < 0.05). Further PNI analysis for CSS (Figures 7J–L) indicated that patients aged 60–69 years with tumors >12 cm, serous adenocarcinoma, high-grade histology, absence of lymphatic metastasis, receiving chemotherapy, without targeted therapy, and regardless of hypertension or diabetes, surgery type, or residual tumor showed the lowest mortality rates when PNI >51.2 (all p < 0.05).
4 Discussion
Ovarian cancer ranks among the three most prevalent gynecologic malignancies and poses a significant public health threat. As the deadliest of all gynecologic cancers, it is characterized by a notably high mortality rate (13). This poor prognosis is largely due to late-stage diagnoses, as most patients present with advanced disease at the time of detection (1). Key factors, including age, tumor size, histological subtype, and comorbidities, significantly impact survival outcomes (14). The advent of laparoscopy represents a notable advancement in the treatment of advanced ovarian cancer (15). Additionally, biological and molecular characteristics play a critical role in selecting the most precise and personalized therapeutic strategies for patients (16). By evaluating prognostic factors that influence postoperative outcomes, clinicians can adopt targeted treatments to address the underlying causes of poor prognosis. Early detection and timely intervention remain essential for improving patients’ quality of life and extending survival. This study emphasizes the growing relevance of nutritional and inflammatory markers as significant predictors of postoperative prognosis in ovarian cancer patients. A deeper understanding of how these markers correlate with survival outcomes offers valuable insights for patient management and could inform the development of innovative therapeutic strategies.
An imbalance between inflammatory and anti-inflammatory processes can lead to cell necrosis, apoptosis, coagulation abnormalities, and immune suppression (17). NLR serves as a well-established marker of systemic inflammatory response, reflecting both the activation and regulation of inflammation within the body (18). Neutrophils play a pivotal role in this process by secreting pro-inflammatory cytokines, regulatory cytokines, and chemokines, which initiate an inflammatory cascade during infection. In contrast, lymphocytes, integral to the immune system, counteract inflammation by releasing anti-inflammatory factors such as interleukin-10 (IL-10) (19). Monocytes contribute significantly to the innate immune response, continuously secreting pro-inflammatory cytokines, enzymes, and growth factors upon recruitment, thus amplifying the inflammatory response. Clinical data analysis indicates that elevated NLR is associated with an increased risk of mortality. The RCS diagram further reveals that a higher LMR correlates with improved OS and CSS in postoperative ovarian cancer patients, whereas elevated NLR is linked to reduced OS and CSS.
Although NLR and LMR reflect the systemic inflammatory response resulting from immune activation, they fail to capture the immune-nutritional status, a critical factor influencing ovarian cancer prognosis. Immune and nutritional disturbances are integral to this prognosis. PNI, which assesses the nutritional and inflammatory state, is derived from serum albumin levels and lymphocyte counts. In contrast, NPS is a novel prognostic tool that combines NLR, LMR, albumin, and TC. Both PNI and NPS are simple to calculate and accurately represent the systemic nutritional, immune, and inflammatory status of patients (6, 9). PNI has been extensively used as a prognostic marker in various cancers, including colon, liver, and esophageal cancers. Furthermore, its clinical relevance has been demonstrated in non-cancerous conditions such as COVID-19, cardiovascular diseases, Crohn’s disease, and sepsis (20–22). However, the prognostic value of PNI in ovarian cancer remains inconsistent (23). For example, Yoshikawa et al. identified a PNI threshold of 46.5 for patients with ovarian clear cell carcinoma, showing that those in the high-PNI group had significantly better OS compared to the low-PNI group (24). This study identified significant associations between both PNI and NPS with OS and CSS in postoperative ovarian cancer patients. A PNI >51.2 was found to be a protective factor, correlating with improved survival outcomes. Patients with L-NPS demonstrated significantly better prognoses than those with H-NPS. RCS analysis further corroborated the relationship between higher PNI and extended OS and CSS. Subgroup analysis reinforced these findings, indicating that PNI is also linked to muscle loss during ovarian cancer treatment. Specifically, low PNI and reduced skeletal muscle mass independently predicted increased all-cause mortality (25). A separate investigation into preoperative PNI and OS in ovarian cancer patients reported significantly longer OS in the high-PNI group compared to the low-PNI group (26).
The role of nutritional inflammatory markers in postoperative prognosis for ovarian cancer is an important area of study, as demonstrated in this research. Prior studies established a scoring system—termed the peripheral blood score (PBS)—based on various peripheral blood parameters, including neutrophil count, lymphocyte count, monocyte count, albumin level, TC, and fibrinogen. This model revealed that lower PBS values were associated with better outcomes, while higher values were linked to poorer prognosis. Furthermore, PBS, along with FIGO grade and residual lesions, was identified as an independent predictor of OS and progression-free survival (PFS) in patients with epithelial ovarian cancer (EOC) (27). Our findings reinforce the notion that nutritional inflammatory markers are independent prognostic factors for OS and CSS in postoperative ovarian cancer patients. These results are consistent with previous studies (28), which highlight the significant influence of systemic inflammation and nutritional status on cancer prognosis (29). By analyzing a comprehensive cohort of clinical characteristics, laboratory parameters, and treatment modalities, this research addresses a notable gap in the current literature by establishing a direct link between nutritional inflammatory markers and survival outcomes in ovarian cancer patients. This study represents the first integration of nutritional inflammatory markers into predictive models for postoperative OS and CSS in ovarian cancer. Independent predictors for OS included age, PNI, NPS, tissue type, tumor size, targeted therapy, and diabetes, while those for CSS comprised age, NPS, tumor size, targeted therapy, and diabetes. Using these variables, nomograms were constructed that exhibited robust predictive accuracy for 3- and 5-year survival. However, calibration curves for 1-year survival revealed some discrepancies. The OS model demonstrated the highest predictive accuracy, with an AUC of 0.910, while the CSS model achieved an AUC of 0.844. External validation yielded AUC values of 0.898 for OS and 0.848 for CSS. This validation confirms the model’s generalizability, reliability, and clinical applicability. Although the external validation results showed slightly reduced efficacy compared to the initial cohort, the model still displayed strong predictive performance. The observed differences may be due to the smaller sample size in the external validation cohort and the population heterogeneity between the two hospitals. In conclusion, the prognostic nomogram incorporating PNI and NPS offers considerable diagnostic value for postoperative ovarian cancer patients.
The findings carry important implications for clinical practice. Identifying nutritional inflammatory markers as reliable prognostic indicators provides healthcare providers with a robust tool for stratifying patients according to their postoperative risk. This approach could inform more personalized treatment strategies, such as enhanced postoperative monitoring or targeted nutritional interventions to optimize inflammatory status both pre- and postoperatively. Additionally, the results support incorporating nutritional inflammatory markers into routine clinical assessments, which may improve the predictive accuracy of survival outcomes, thereby enabling more informed decision-making and enhancing the overall management of ovarian cancer patients.
This study has several limitations that warrant consideration. The retrospective design may have introduced bias, and the relatively small sample size could limit the generalizability of the results. Additionally, the research methodology is somewhat restricted due to the small sample size and the limited statistical approaches employed, which diminish methodological diversity. Despite data collection from two hospitals, the lack of broader diversity remains a concern. Future research should prioritize validating these results in larger, multicenter cohorts and exploring the underlying mechanisms by which nutritional and inflammatory status influence cancer progression and treatment response.
5 Conclusion
In summary, the nutritional inflammatory index serves as a significant independent prognostic factor for OS and CSS in ovarian cancer patients. Its integration into clinical practice may improve patient stratification and enable more tailored therapeutic strategies. The development of a nomogram based on key predictors highlights its potential value in clinical decision-making. Nevertheless, additional research is required to validate these results and investigate the mechanisms connecting nutritional inflammation to tumor dynamics and patient outcomes. A more comprehensive understanding of these interactions could improve prognostic models and optimize management approaches for ovarian cancer patients.
Data availability statement
The raw data supporting the conclusions of this article will be made available by the authors, without undue reservation.
Ethics statement
The studies involving humans were approved by Ethics Committee of the Second People's Hospital Affiliated to Soochow University (Approval No. JD-LK-2022-079-01). The studies were conducted in accordance with the local legislation and institutional requirements. The participants provided their written informed consent to participate in this study. Written informed consent was obtained from the individual(s) for the publication of any potentially identifiable images or data included in this article.
Author contributions
YZ: Conceptualization, Data curation, Investigation, Methodology, Writing – original draft, Writing – review & editing. WX: Formal analysis, Methodology, Project administration, Writing – original draft. XL: Funding acquisition, Methodology, Software, Supervision, Validation, Writing – original draft. ZY: Data curation, Formal analysis, Writing – original draft. YM: Data curation, Investigation, Methodology, Writing – original draft. YC: Conceptualization, Formal analysis, Methodology, Writing – original draft, Writing – review & editing. WZ: Conceptualization, Data curation, Formal analysis, Funding acquisition, Investigation, Methodology, Project administration, Resources, Software, Supervision, Validation, Visualization, Writing – original draft, Writing – review & editing.
Funding
The author(s) declare that financial support was received for the research, authorship, and/or publication of this article. This work is funded by the 23rd batch of the Science and Technology Development Plan of Suzhou in 2023 (Capacity Improvement of Clinical Trial Institutions, SLT2023033) and Guangzhou Xinuowang Information Technology Co., LTD (Application of Visual Accurate Electrophysiological Diagnosis and Treatment in Pain Diagnosis and Treatment and Related Basic Research, H221112).
Acknowledgments
The authors express their gratitude to all collaborators for their contributions to data analysis and manuscript preparation.
Conflict of interest
The authors declare that the research was conducted in the absence of any commercial or financial relationships that could be construed as a potential conflict of interest.
The authors declare that this study received funding from Guangzhou Xinuowang Information Technology Co., LTD. The funder had the following involvement in the study: participate in research design and data analysis.
Generative AI statement
The author(s) declare that no Generative AI was used in the creation of this manuscript.
Publisher’s note
All claims expressed in this article are solely those of the authors and do not necessarily represent those of their affiliated organizations, or those of the publisher, the editors and the reviewers. Any product that may be evaluated in this article, or claim that may be made by its manufacturer, is not guaranteed or endorsed by the publisher.
Supplementary material
The Supplementary Material for this article can be found online at: https://www.frontiersin.org/articles/10.3389/fonc.2025.1531987/full#supplementary-material
Supplementary Figure 1 | RCS diagram of the relationship between albumin, total cholesterol, CA125, CA199, CEA and HE4 and survival prognosis in patients with ovarian cancer after surgery.
References
1. Liu L, Cai L, Liu C, Yu S, Li B, Pan L, et al. Construction and validation of a novel glycometabolism-related gene signature predicting survival in patients with ovarian cancer. Front Genet. (2020) 11:585259. doi: 10.3389/fgene.2020.585259
2. Li Y, Zhu X, Liu C, Han Q, Chen X, Liu Y, et al. Low FOXJ2 expression is associated with unfavorable postoperative prognosis of patients with epithelial ovarian cancer. Med (Baltimore). (2021) 100:e24759. doi: 10.1097/MD.0000000000024759
3. Arcieri M, Tius V, Andreetta C, Restaino S, Biasioli A, Poletto E, et al. How BRCA and homologous recombination deficiency change therapeutic strategies in ovarian cancer: a review of literature. Front Oncol. (2024) 14:1335196. doi: 10.3389/fonc.2024.1335196
4. Xie H, Ruan G, Wei L, Zhang H, Ge Y, Zhang Q, et al. Comprehensive comparison of the prognostic value of systemic inflammation biomarkers for cancer cachexia: a multicenter prospective study. Inflammation Res. (2022) 71:1305–13. doi: 10.1007/s00011-022-01626-7
5. Li Y, Zhang C, Ji R, Lu H, Zhang W, Li LL, et al. Prognostic significance of the controlling nutritional status (CONUT) score in epithelial ovarian cancer. Int J Gynecol Cancer. (2020) 30:74–82. doi: 10.1136/ijgc-2019-000865
6. Liu CC, Liu PH, Chen HT, Chen JY, Lee CW, Cheng WJ, et al. Association of preoperative prognostic nutritional index with risk of postoperative acute kidney injury: A meta-analysis of observational studies. Nutrients. (2023) 15(13):2929. doi: 10.3390/nu15132929
7. Wakita M, Fukatsu A, Amagai T. Nutrition assessment as a predictor of clinical outcomes for infants with cardiac surgery: using the prognostic nutritional index. Nutr Clin Pract. (2011) 26:192–8. doi: 10.1177/0884533611399922
8. Li M, Cai J, Jiang K, Li Y, Li S, Wang Q, et al. Prognostic nutritional index during hospitalization correlates with adverse outcomes in elderly patients with acute myocardial infarction: a single-center retrospective cohort study. Aging Clin Exp Res. (2024) 36:56. doi: 10.1007/s40520-024-02702-0
9. Galizia G, Lieto E, Auricchio A, Cardella F, Mabilia A, Podzemny V, et al. Naples prognostic score, based on nutritional and inflammatory status, is an independent predictor of long-term outcome in patients undergoing surgery for colorectal cancer. Dis Colon Rectum. (2017) 60:1273–84. doi: 10.1097/DCR.0000000000000961
10. Li Q, Cong R, Wang Y, Kong F, Ma J, Wu Q, et al. Naples prognostic score is an independent prognostic factor in patients with operable endometrial cancer: Results from a retrospective cohort study. Gynecol Oncol. (2021) 160:91–8. doi: 10.1016/j.ygyno.2020.10.013
11. Gu J, Deng S, Jiang Z, Mao F, Xue Y, Qin L, et al. Modified Naples prognostic score for evaluating the prognosis of patients with obstructive colorectal cancer. BMC Cancer. (2023) 23:941. doi: 10.1186/s12885-023-11435-8
12. Feng JF, Zhao JM, Chen S, Chen QX. Naples prognostic score: A novel prognostic score in predicting cancer-specific survival in patients with resected esophageal squamous cell carcinoma. Front Oncol. (2021) 11:652537. doi: 10.3389/fonc.2021.652537
13. Tortorella L, Vizzielli G, Fusco D, Cho WC, Bernabei R, Scambia G, et al. Ovarian cancer management in the oldest old: improving outcomes and tailoring treatments. Aging Dis. (2017) 8:677–84. doi: 10.14336/AD.2017.0607
14. Cheng CY, Hsu HC, Tai YJ, Chiang YC, Chen YL, Cheng WF. Outcome and prognostic factors of unexpected ovarian carcinomas. Cancer Med. (2023) 12:6466–76. doi: 10.1002/cam4.v12.6
15. Salvatore GA, Vito AC, Andrea R, Ilaria DB, Stefano C, Giuseppe V, et al. Laparoscopy vs. laparotomy for advanced ovarian cancer: a systematic review of the literature. Minerva Med. (2019) 110:341–57. doi: 10.23736/S0026-4806.19.06132-9
16. Arcieri M, Andreetta C, Tius V, Zapelloni G, Titone F, Restaino S, et al. Molecular biology as a driver in therapeutic choices for ovarian cancer. Int J Gynecol Cancer. (2024). doi: 10.1136/ijgc-2024-005700
17. Li T, Dong G, Zhang M, Xu Z, Hu Y, Xie B, et al. Association of neutrophil-lymphocyte ratio and the presence of neonatal sepsis. J Immunol Res. (2020) 2020:7650713. doi: 10.1155/2020/7650713
18. Dragoescu AN, Padureanu V, Stanculescu AD, Chiutu LC, Tomescu P, Geormaneanu C, et al. Neutrophil to lymphocyte ratio (NLR)-A useful tool for the prognosis of sepsis in the ICU. Biomedicines. (2021) 10(1):75. doi: 10.3390/biomedicines10010075
19. Huang Z, Fu Z, Huang W, Huang K. Prognostic value of neutrophil-to-lymphocyte ratio in sepsis: A meta-analysis. Am J Emerg Med. (2020) 38:641–7. doi: 10.1016/j.ajem.2019.10.023
20. Wang ZH, Lin YW, Wei XB, Li F, Liao XL, Yuan HQ, et al. Predictive value of prognostic nutritional index on COVID-19 severity. Front Nutr. (2020) 7:582736. doi: 10.3389/fnut.2020.582736
21. Li T, Qi M, Dong G, Li X, Xu Z, Wei Y, et al. Clinical value of prognostic nutritional index in prediction of the presence and severity of neonatal sepsis. J Inflammation Res. (2021) 14:7181–90. doi: 10.2147/JIR.S343992
22. Hayashi J, Uchida T, Ri S, Hamasaki A, Kuroda Y, Yamashita A, et al. Clinical significance of the prognostic nutritional index in patients undergoing cardiovascular surgery. Gen Thorac Cardiovasc Surg. (2020) 68:774–9. doi: 10.1007/s11748-020-01300-x
23. Miao Y, Li S, Yan Q, Li B, Feng Y. Prognostic significance of preoperative prognostic nutritional index in epithelial ovarian cancer patients treated with platinum-based chemotherapy. Oncol Res Treat. (2016) 39:712–9. doi: 10.1159/000452263
24. Yoshikawa N, Yoshida K, Tamauchi S, Ikeda Y, Nishino K, Niimi K, et al. The preoperative prognostic nutritional index for the prediction of outcomes in patients with early-stage ovarian clear cell carcinoma. Sci Rep. (2020) 10:7135. doi: 10.1038/s41598-020-64171-5
25. Lee J, Weng CS, Chang CL, Hsu WH, Jan YT, Wu KP. Association of prognostic nutritional index with muscle loss and survival in patients with ovarian cancer treated with primary debulking surgery and chemotherapy. Support Care Cancer. (2023) 31:267. doi: 10.1007/s00520-023-07719-1
26. Karakaş S, Demirayak G, Önder AB, Özdemir İA, Comba C, Çaypınar SS, et al. The association between the preoperative prognostic nutritional index and the controlling nutritional status score on tumor stage, chemotherapeutic response and overall survival in ovarian cancer. Nutr Cancer. (2022) 74:1770–9. doi: 10.1080/01635581.2021.2022170
27. Bai G, Zhou Y, Rong Q, Qiao S, Mao H, Liu P. Development of nomogram models based on peripheral blood score and clinicopathological parameters to predict preoperative advanced stage and prognosis for epithelial ovarian cancer patients. J Inflammation Res. (2023) 16:1227–41. doi: 10.2147/JIR.S401451
28. Feng Z, Wen H, Ju X, Bi R, Chen X, Yang W, et al. The preoperative prognostic nutritional index is a predictive and prognostic factor of high-grade serous ovarian cancer. BMC Cancer. (2018) 18:883. doi: 10.1186/s12885-018-4732-8
Keywords: nutritional inflammatory markers, PNI, NPS, ovarian cancer, prognosis
Citation: Zhang Y, Xing W, Liang X, Yang Z, Ma Y, Chen Y and Zhu W (2025) Relationship between nutritional-inflammatory markers and postoperative outcomes in ovarian cancer: a retrospective study. Front. Oncol. 15:1531987. doi: 10.3389/fonc.2025.1531987
Received: 21 November 2024; Accepted: 10 February 2025;
Published: 11 March 2025.
Edited by:
Giuseppe Vizzielli, University of Udine, ItalyReviewed by:
Martina Arcieri, Ospedale Santa Maria della Misericordia di Udine, ItalySara Pregnolato, University of Udine, Italy
Copyright © 2025 Zhang, Xing, Liang, Yang, Ma, Chen and Zhu. This is an open-access article distributed under the terms of the Creative Commons Attribution License (CC BY). The use, distribution or reproduction in other forums is permitted, provided the original author(s) and the copyright owner(s) are credited and that the original publication in this journal is cited, in accordance with accepted academic practice. No use, distribution or reproduction is permitted which does not comply with these terms.
*Correspondence: Weipei Zhu, endwMzMzeHhAMTYzLmNvbQ==; Ying Chen, Y3k2NjM4NUAxNjMuY29t
†These authors have contributed equally to this work