- Department of Nuclear Medicine, Zhuhai People’s Hospital (The Affiliated Hospital of Beijing Institute of Technology, Zhuhai Clinical Medical College of Jinan University), Zhuhai, China
Objective: To investigate the value of Fluorine-18 Fluorodeoxyglucose (18F-FDG) Positron Emission Tomography/Computed Tomography (PET/CT) combined with 3D quantitative technology and clinicopathological features in predicting the prognosis of non-small cell lung cancer (NSCLC).
Methods: A retrospective review was performed for patients who underwent PET/CT and curative resection of NSCLC between January 2016 and June 2019 in our hospital. PET/CT data, clinical features, and pathology results were collected. Gross tumor volume (GTV) was delineated on CT images by ITK-SNAP software. The prognosis was followed up, and the study endpoint was progression-free survival (PFS). Receiver operating characteristic curve (ROC) was used to initially assess the relationship between each parameter and PFS, and parameters were grouped accordingly. Cox proportional hazards regression was used to develop models based on clinicopathological features to predict prognosis of NSCLC patients. Kaplan–Meier method was used to draw the survival curves.
Results: A total of 128 patients were enrolled in the study with PFS of 8–96 months. Univariate analysis demonstrated that age, SUVindex (the ratio of SUVmax of lesion to SUVmax of liver), metabolic tumor volume (MTV), Dmax (the largest diameter), GTV, lymph node metastasis (LNM), and TNM staging are significantly related to recurrence (all p<0.05). The multivariate analysis showed that only age, SUVindex, and LNM were independent prognostic factor for PFS (all p < 0.05).
Conclusions: Although 18F-FDG PET/CT combined with 3D quantitative technique were helpful in predicting PFS in NSCLC, only age, SUVindex, and LNM were independent predictors for PFS.
Introduction
Lung cancer is the leading cause of cancer-related deaths worldwide with a 5-year survival rate of approximately 15% (1, 2), of which NSCLC is the most common type, accounting for more than 85% (3). With the continuous development of early diagnosis and adjuvant therapy in recent years, the prognosis of NSCLC has been improved, but the recurrence rate after treatment is still high. Although many studies have been conducted to find factors that could predict the prognosis, the best prognostic predictors available are still debated (4–6).
PET/CT has been used in lung cancer for more than 20 years. Many studies have confirmed that PET/CT plays a crucial role in the staging, efficacy evaluation, and detection of recurrence in NSCLC (7, 8). The maximum standard uptake value (SUVmax) is the most commonly used PET/CT parameter, but it is susceptible to a variety of factors and measured by different scanners varies widely. A study has shown that SUVindex is a simple and reliable predictor of prognosis in early-stage lung cancer (9). MTV represents the metabolic information of the whole tumor and can provide both metabolic activity and volume information. One study showed that MTV was significantly better than SUVmax in predicting lung cancer prognosis (10), but the threshold value used to measure MTV is still debated (11).
3D quantitative technology based on genomics has been a hot research topic in recent years, and ITK-SNAP software is commonly used in genomics at home and abroad to accurately extract 3D information of lesions (12). Previous studies have found that the assessment of intra-tumor features on CT is useful for predicting overall survival (OS) and PFS in NSCLC (13, 14).
In recent years, a study has also shown that systemic inflammation and nutritional status are closely related to malignant tumorigenesis, progression, and prognosis (15). Many inflammatory and nutritional markers based on serological indicators have been applied to predict the prognosis of NSCLC (16–18).
Therefore, we evaluated the value of PET/CT combined with 3D quantitative technique and clinicopathological features in predicting postoperative recurrence in NSCLC patients to look for new indicators that can accurately estimate the prognosis.
Materials and methods
Study design
All enrolled cases were NSCLC patients who underwent FDG PET/CT and radical resection within 2 weeks in our hospital from January 2016 to June 2019. A total of 68 patients underwent postoperative chemotherapy or targeted therapy. Pathological classification was performed according to the 2021 WHO classification of thoracic tumors and TNM staging according to the American Joint Committee on Cancer (AJCC) eighth edition. This study was approved by the Institutional Review Board of Zhuhai People’s Hospital in Guangdong Province, China. The requirement for informed consent was waived due to the retrospective nature of the study design.
Inclusion and exclusion criteria
Inclusion criteria were as follows: (I) complete data were available; (II) patients did not undergo any anti-tumor therapy or had no history of malignancy before PET/CT examination; (III) all patients underwent curative resection and confirmed to be NSCLC; and (IV) all patients underwent standardized postoperative treatment.
Exclusion criteria were as follows: (I) poor image quality or undistinguished lesion boundary or (II) without standardize treatment or without review after surgery.
Imaging equipment and methods
The Philips Gemini TF PET/CT scanner (Philips Medical Systems, Netherlands) was applied to conduct the PET/CT imaging. Patients fasted for more than 4 h, and fasting blood glucose was measured before intravenous injection of 18F-FDG (Atom High-tech Isotope Pharmaceutical Co., Ltd., Guangzhou, China; radiochemical purity > 95%, 4.07–5.18 MBq/kg). Patients were allowed to rest for 60 min in a quiet, warm, dark condition before PET/CT examination.
First low-dose CT scan was performed for attenuation correction and anatomical location with a tube voltage of 80 kV, a tube current of 150 mAs, and a layer thickness of 2 mm. PET acquisition was performed in three-dimensional mode with the scope of acquisition from the upper thigh to the cranial roof, and the scanning time was 70 s/bed.
Image analysis and segmentation
The EBW system (Philips, Netherlands) was used for image processing. The following parameters including MTV of primary foci with the threshold of 40%SUVmax, Dmax (the largest diameter of primary foci), SUVmax of the primary foci, and normal liver parenchyma were measured. SUVindex = SUVmax of the primary foci/SUVmax of normal liver parenchyma (19).
ITK-SNAP (Version 3.8) was used for image segmentation. Two experienced nuclear physicians outlined the CT axial images layer by layer until the entire primary lesion was outlined, and the GTV was automatically calculated by the software. When the boundary was not clear, the PET images were referred to. The internal tumor cavity was avoided while outlining. The average of the measurements were recorded.
Follow-up
Patients were followed up at 3-month intervals for the first 3 years after the operation, at 6-month intervals for the next 2 years, and annually thereafter. Patients were followed up via the HIS system and telephone. The beginning time was the date of surgery, and the termination was tumor recurrence or deadline of 31 December 2023. The recurrence was confirmed by imaging or pathology. PFS was defined as the time from initial treatment to tumor progression, death, or deadline.
Statistical analysis
GraphPad Prism (Version 9.2), R Language, and SPSS (Version 25.0, IBM) were used for data analysis. Normally distributed data were expressed as mean ± standard deviation, and non-normal data were expressed as the median (interquartile range). Comparisons between two groups of normally distributed data were analyzed using a Student’s t-tests, and comparisons between groups of non-normal data were analyzed using the Mann–Whitney U test. The prognostic value of each parameter for PFS was initially assessed using the receiver operating characteristic (ROC) curve. Univariate and multivariate analyses were performed with the COX proportional risk regression model to obtain the independent predictors of PFS. Survival curves were plotted using the Kaplan–Meier method, and the log-rank test was used to analyze the survival differences between groups. A p-value<0.05 was considered statistically significant.
Results
In total, 128 patients were analyzed in the present study, including 79 cases in stage I, 18 cases in stage II, and 31 cases in stage III. A total of 34 patients experienced recurrence with an overall median PFS of 48 months (Table 1), and three patients experienced new cancers beyond 5 years.
Univariate analysis of progression-free survival
Univariate analysis revealed that the age, SUVindex, MTV, GTV, LNM, stage, and Dmax were correlated with PFS (all p <0.05). The hazard ratio (HR) was 2.161 (1.003–4.655), 3.541 (1.749–7.170), 2.433 (1.178–5.028), 2.256 (1.091–4.666), 4.057 (2.002–8.223), 3.658 (1.793–7.461), 2.288 (1.085–4.826), respectively. The gender, pathological type, and adjuvant therapy were not correlated with PFS (all p> 0.05) (Table 1).
Multivariate analysis with respect to PFS and survival curves
Combinatorial effects and interactions between Age, SUVindex, MTV, GTV, Stage, LNM, and Dmax were examined using Cox proportional hazard models. It was found that only Age, SUVindex, and LNM remained independent predictors of PFS (all p < 0.05), with HR of 3.456 (1.483–8.053), 3.606 (1.510–8.612), and 3.123 (1.067–9.139), respectively (Table 2).
Our study also found that patients with age >60 years were 2.135 times more likely to experience recurrence than those with age ≤60 years (Figure 1A). Patients with an SUVindex of >2.36 were 3.508 times more likely to experience recurrence than those with a SUVindex of ≤2.36 (Figure 1B). Patients with LNM were 3.681 times more likely to experience recurrence than those without LNM (Figure 1C).
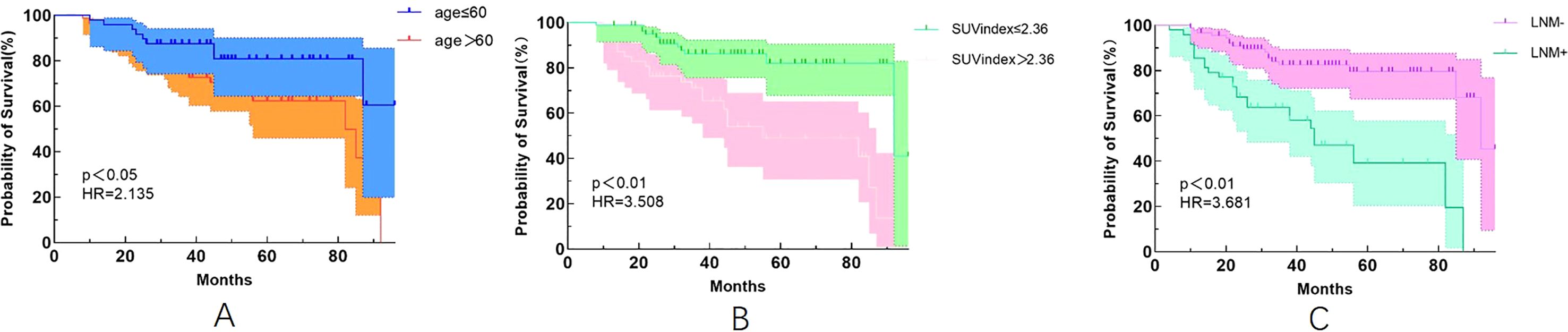
Figure 1. Kaplan-Meier survival curves for different variables. (A) Age (p<0.05); (B) SUVindex (p<0.05); (C) LNM (p<0.05).
Survival ROC curves parameters for predicting PFS
The survival ROC curve analysis for different time points of Age, SUVindex, LNM, and the Combined showed that the AUC value of the Combined was higher than the other parameters (Figure 2).
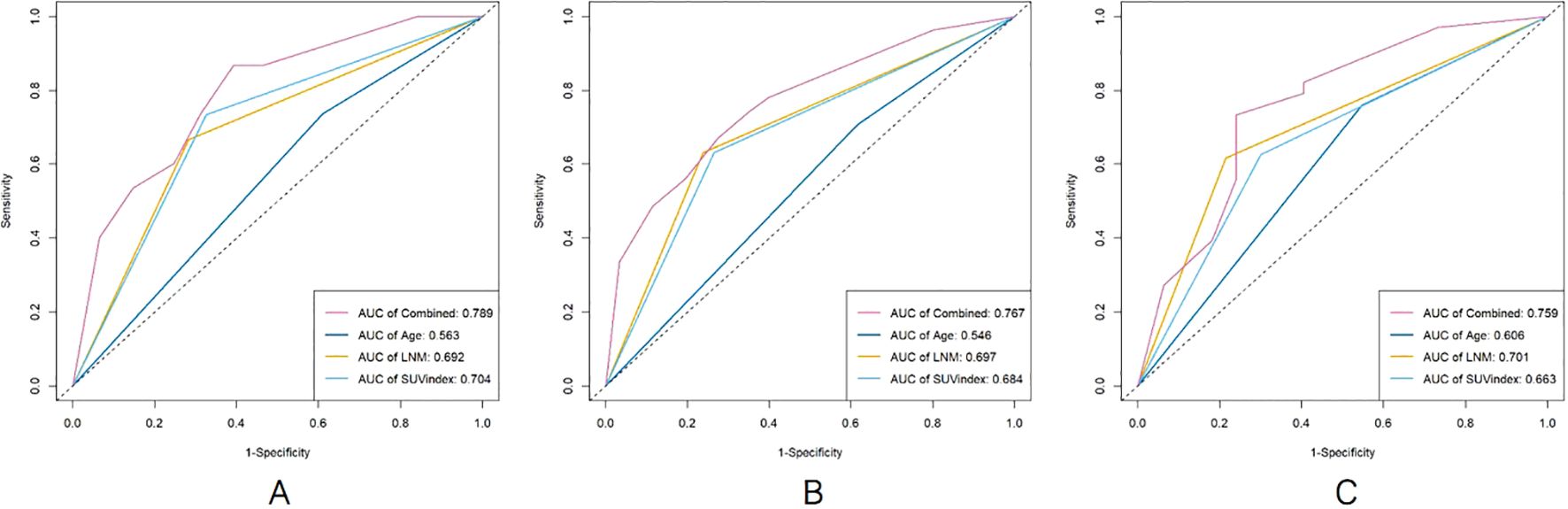
Figure 2. The comparison of survival ROC curves for predicting PFS [(A), 2 years; (B), 4 years; (C), 6 years].
Relationship between different stages and recurrence
Since univariate analysis showed that staging was associated with PFS, we further compared survival outcomes across different stages. We found that stage III patients are more prone to experience recurrence compared to stage I and II patients (Figures 3A, B). However, the difference in survival between stage II and stage III patients was not statistically significant (Figure 3).
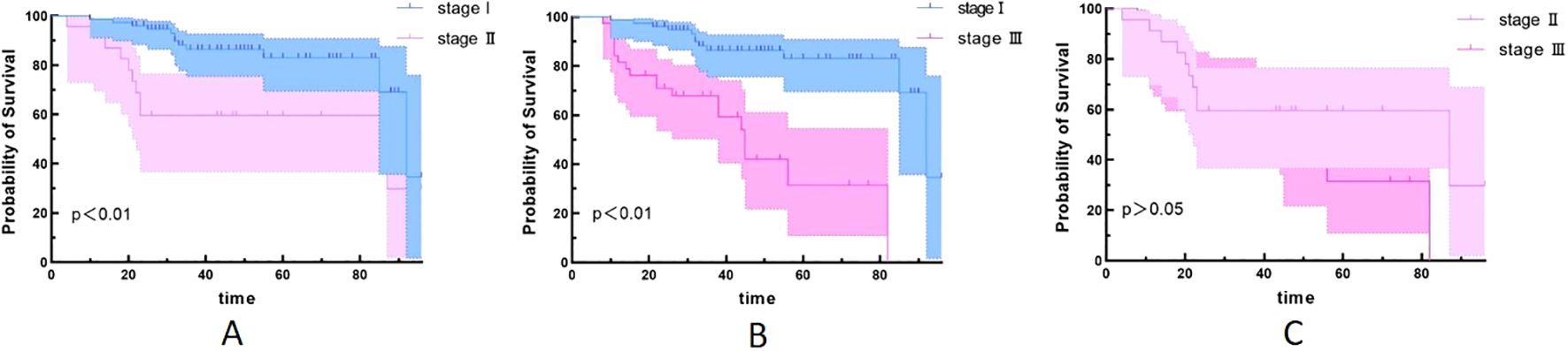
Figure 3. Kaplan–Meier survival curves for different stages. (A) stage I and stage II (p<0.05); (B) stage I and stage III (p<0.05); (C) stage II and stage III (p>0.05).
Discussion
This study explored the value of 18F-FDG PET/CT combined with 3D quantitative technique and clinicopathological features in predicting the postoperative risk of recurrence in NSCLC, in order to identify new reliable and sensitive prognostic indicators, stratify patients according to prognosis, and thus develop appropriate treatment strategies.
In this study, univariate analysis indicated that Age, MTV, GTV, SUVindex, LNM, and Stage were predictive factors of tumor recurrence, and patients were divided into two prognostic subgroups. In the multivariate analysis, Age, SUVindex, and LNM were found to be independent predictors of disease recurrence, thus establishing a model to predict PFS of NSCLC.
Previous studies (20–22) have shown that the prognosis of lung cancer is related to many factors, such as age, clinical stage, cell type, treatment modality, primary tumor size, and SUVmax, but the conclusions are different. A study (23) showed that age, positive lymph node staging, and complete resection were important prognostic factors for long-term survival after lung cancer surgery. Some studies (24, 25) also showed that age was not a risk factor for recurrence. Our study showed that age was associated with postoperative recurrence in NSCLC patients, older patients were more likely to relapse, and the risk of recurrence in patients over 60 years was 2.135 times higher than under 60 years.
In the absence of distant metastasis, whether metastasis involves the ipsilateral mediastinal lymph node (N2) is the most determinant of treatment and survival in patients with lung cancer. Stage N2 is considered to be a more advanced stage of lung cancer, and patients with that stage are more likely to recur than patients with stage N1 (26). In a study of 39,731 patients based on SEER database, Dai et al. (27) found that LNM was an independent factor of prognosis in NSCLC patients. Our study showed that patients in the LNM group were more likely to have recurrence after surgery, and the probability of recurrence was 3.681 times higher than that in the negative group, and the sensitivity for predicting recurrence was the highest among all predictive factors, which was consistent with the conclusions of previous studies.
Chansky et al. (28) studied 9,137 surgically treated NSCLC patients and found that stage was the most important prognostic factor, while cell type was less important. Wang et al. (29) reviewed the clinicopathological characteristics and prognostic factors of 33,919 lung cancer patients and found that TNM stage, gender, age, tumor site, cell type, and differentiation were all independent factors affecting prognosis. Our results found no statistically significant difference in PFS between adenocarcinoma and non-adenocarcinoma patients. Univariate analysis and chi-square test showed that TNM stage was associated with postoperative recurrence, but it was not included in the multivariate analysis. Further analysis revealed that stage III patients are more prone to experience recurrence compared to stage I and II patients. However, the difference in survival between stage II and stage III was not statistically significant. This may be due to the small number of stage II (18 cases) and stage III (31 cases), which can be further analyzed and verified by large sample data in the future.
Melloni et al. (9) found that SUVindex, MTV, and TLG were significantly associated with risk of recurrence, but only TLG seemed to be the most accurate prognostic factor for postoperative recurrence of NSCLC. Laura et al. (30) has found that the diagnostic accuracy of PET/CT for solitary pulmonary nodules (SPNs) is improved by using the ratio between SUVmax in SPN and SUVmean in mediastinal blood pool and between SUVmax in SPN and SUVmean in the liver. In our study, the results of univariate analysis were consistent with it, but only SUVindex could predict postoperative recurrence in multivariate analysis, which may be related to the different cases included in the two studies. Melloni’s study only included stage I patients, while our study included patients who underwent radical resection from stage I to stage III.
Our study also found that there was no statistically significant difference in PFS between patients with adjuvant therapy and without adjuvant therapy.
The heterogeneity and aggressiveness of tumor increased with increasing tumor size (31); for this reason, we also analyzed the effect of GTV and Dmax, which represents the anatomical size of tumor, on PFS. Although the univariate analysis showed that tumor GTV (26.1 cm3) and maximum diameter (41.5 mm) were associated with PFS, the results of multivariate analysis were not statistically significant. This also indicates that the larger the tumor, the worse the prognosis, and also suggests that the role of 3D quantitative technique in predicting postoperative recurrence of NSCLC is not particularly prominent.
Although some studies have shown that serum albumin and lymphocyte count have some value in assessing the prognosis of NSCLC (32, 33). We analyzed the results of preoperative serum albumin and lymphocyte in all patients and found that serum albumin and lymphocyte levels were not associated with postoperative recurrence. This may be due to the inclusion of different cases.
There are some limitations in the present study. First, this study is a single-center retrospective study, which may have selective bias and needs to be verified by large sample, multi-center, and prospective studies. Second, only stage I–III NSCLC patients who underwent radical resection and standardized postoperative therapy were included in this study, and patients with more treatment modalities were planned in the next step with lateral and longitudinal prognosis analysis.
In conclusion, 18F-FDG PET/CT combined with 3D quantitative techniques, and clinicopathological features were helpful in predicting PFS in NSCLC patients, but only age, SUVindex, and stage were independent predictors.
Data availability statement
The raw data supporting the conclusions of this article will be made available by the authors, without undue reservation.
Ethics statement
The studies involving humans were approved by the Institutional Review Board of Zhuhai People’s Hospital in Guangdong Province, China. The studies were conducted in accordance with the local legislation and institutional requirements. Written informed consent for participation was not required from the participants or the participants’ legal guardians/next of kin in accordance with the national legislation and institutional requirements.
Author contributions
YS: Data curation, Funding acquisition, Methodology, Validation, Writing – original draft, Writing – review & editing. SQ: Data curation, Investigation, Software, Writing – original draft, Writing – review & editing. JW: Conceptualization, Formal Analysis, Investigation, Resources, Supervision, Validation, Visualization, Writing – original draft, Writing – review & editing.
Funding
The author(s) declare that financial support was received for the research and/or publication of this article. This work was supported by the Zhuhai Science and Technology Program Project, Guang Dong Province, China (No. 2320004000038).
Acknowledgments
Thanks to the colleagues and friends who provided help in this study.
Conflict of interest
The authors declare that the research was conducted in the absence of any commercial or financial relationships that could be construed as a potential conflict of interest.
Generative AI statement
The author(s) declare that no Generative AI was used in the creation of this manuscript.
Publisher’s note
All claims expressed in this article are solely those of the authors and do not necessarily represent those of their affiliated organizations, or those of the publisher, the editors and the reviewers. Any product that may be evaluated in this article, or claim that may be made by its manufacturer, is not guaranteed or endorsed by the publisher.
References
1. Siegel RL, Miller KD, Fuchs HE, Jemal A. Cancer statistics, 2022. CA Cancer J Clin. (2022) 72:7–33. doi: 10.3322/caac.21708
2. Yoshida Y, Murayama T, Sato Y, Suzuki Y, Saito H, Nomura Y. Gender differences in long-term survival after surgery for non-small cell lung cancer. Thorac Cardiovasc Surg. (2016) 64:507–14. doi: 10.1055/s-0035-1558995
3. Ettinger DS, Wood DE, Aisner DL, Akerley W, Bauman J, Chirieac LR, et al. Non-small cell lung cancer, version 5.2017, NCCN clinical practice guidelines in oncology. J Natl Compr Canc Netw. (2017) 15:504–35. doi: 10.6004/jnccn.2017.0050
4. Li S, Wang H, Yang Z, Zhao L, Lv W, Du H, et al. Naples Prognostic Score as a novel prognostic prediction tool in video-assisted thoracoscopic surgery for early-stage lung cancer: a propensity score matching study. Surg Endosc. (2021) 35:3679–97. doi: 10.1007/s00464-020-07851-7
5. Galata C, Messerschmidt A, Kostic M, Karampinis I, Roessner E, El Beyrouti H, et al. Prognostic factors for long-term survival following complete resection by lobectomy in stage I non-small cell lung cancer. Thorac Cancer. (2022) 13:2861–6. doi: 10.1111/1759-7714.14630
6. Han Z, Hu Z, Zhao Q, Xue W, Duan G. The advanced lung cancer inflammation index predicts outcomes of patients with non-small cell lung cancer following video-assisted thoracic surgery. J Int Med Res. (2021) 49:3000605211062442. doi: 10.1177/03000605211062442
7. Kandathil A, Kay FU, Butt YM, Wachsmann JW, Subramaniam RM. Role of FDG PET/CT in the eighth edition of TNM staging of non-small cell lung cancer. Radiographics. (2018) 38:2134–49. doi: 10.1148/rg.2018180060
8. Konert T, van de Kamer JB, Sonke JJ, Vogel WV. The developing role of FDG PET imaging for prognostication and radiotherapy target volume delineation in non-small cell lung cancer. J Thorac Dis. (2018) 10:S2508–21. doi: 10.21037/jtd.2018.07.101
9. Melloni G, Gajate AM, Sestini S, Gallivanone F, Bandiera A, Landoni C, et al. New positron emission tomography derived parameters as predictive factors for recurrence in resected stage I non-small cell lung cancer. Eur J Surg Oncol. (2013) 39:1254–61. doi: 10.1016/j.ejso.2013.07.092
10. Pellegrino S, Fonti R, Mazziotti E, Piccin L, Mozzillo E, Damiano V, et al. Total metabolic tumor volume by 18F-FDG PET/CT for the prediction of outcome in patients with non-small cell lung cancer. Ann Nucl Med. (2019) 33:937–44. doi: 10.1007/s12149-019-01407-z
11. Krak NC, Boellaard R, Hoekstra OS, Twisk JW, Hoekstra CJ, Lammertsma AA. Effects of ROI definition and reconstruction method on quantitative outcome and applicability in a response monitoring trial[J. Eur J Nucl Med Mol Imaging. (2005) 32:294–301. doi: 10.1007/s00259-004-1566-1
12. Foy JP, Durdux C, Giraud P, Bibault JE. RE: the rise of radiomics and implications for oncologic management. J Natl Cancer Inst. (2018) 110:1275–6. doi: 10.1093/jnci/djy037
13. Tominaga M, Yamazaki M, Umezu H, Sugino H, Fuzawa Y, Yagi T, et al. Prognostic value and pathological correlation of peritumoral radiomics in surgically resected non-small cell lung cancer. Acad Radiol. (2024) 31(9):3801–10. doi: 10.1016/j.acra.2024.01.033
14. Yang L, Yang J, Zhou X, Huang L, Zhao W, Wang T, et al. Development of a radiomics nomogram based on the 2D and 3D CT features to predict the survival of non-small cell lung cancer patients. Eur Radiol. (2019) 29:2196–206. doi: 10.1007/s00330-018-5770-y
15. Singh N, Baby D, Rajguru JP, Patil PB, Thakkannavar SS, Pujari VB. Inflammation and cancer. Ann Afr Med. (2019) 18:121–6. doi: 10.4103/aam.aam_56_18
16. Wang Y, Li Y, Chen P, Xu W, Wu Y, Che G. Prognostic value of the pretreatment systemic immune-inflammation index (SII) in patients with nonsmall cell lung cancer: A meta-analysis. Ann Transl Med. (2019) 7:433. doi: 10.21037/atm.2019.08.116
17. Gunsel-Yildirim G, Ceylan KC, Dikmen D. The effect of perioperative immunonutritional support on nutritional and inflammatory status in patients undergoing lung cancer surgery: A prospective, randomized controlled study. Support Care Cancer. (2023) 31:365. doi: 10.1007/s00520-023-07838-9
18. Olmez OF, Bilici A, Gursoy P, Cubukcu E, Sakin A, Korkmaz T, et al. Impact of systemic inflammatory markers in patients with ALK-positive non-small cell lung cancer treated with crizotinib. Pulmonology. (2023) 29:478–85. doi: 10.1016/j.pulmoe.2022.11.006
19. Shiono S, Abiko M, Okazaki T, Chiba M, Yabuki H, Sato T. Positron emission tomography for predicting recurrence in stage I lung adenocarcinoma: standardized uptake value corrected by mean liver standardized uptake value. Eur J Cardiothorac Surg. (2011) 40:1165–9. doi: 10.1016/j.ejcts.2011.02.041
20. Kim HC, Ji W, Lee JC, Kim HR, Song SY, Choi CM, et al. Prognostic factor and clinical outcome in stage III non-small cell lung cancer: A study based on real-world clinical data in the Korean population. Cancer Res Treat. (2021) 53:1033–41. doi: 10.4143/crt.2020.1350
21. Liu J, Dong M, Sun X, Li W, Xing L, Yu J. Prognostic value of 18F-FDG PET/CT in surgical non-small cell lung cancer: A meta-analysis. PLoS One. (2016) 11:e0146195. doi: 10.1371/journal.pone.0146195
22. Borst GR, Belderbos JS, Boellaard R, Comans EF, De Jaeger K, Lammertsma AA, et al. Standardised FDG uptake: a prognostic factor for inoperable non-small cell lung cancer. Eur J Cancer. (2005) 41:1533–41. doi: 10.1016/j.ejca.2005.03.026
23. Wada H, Fukuse T, Hitomi S. Long-term survival of surgical cases of lung cancer. Lung Cancer. (1995) 13:269–74. doi: 10.1016/0169-5002(95)00499-8
24. Yang YH, Kim HE, Park BJ, Lee S, Park SY, Lee CY, et al. Positive nodal status is still a risk factor for long-term survivors of non-small cell lung cancer 5 years after complete resection. J Thorac Dis. (2021) 13:5826–34. doi: 10.21037/jtd-21-854
25. Landreneau RJ, Normolle DP, Christie NA, Awais O, Wizorek JJ, Abbas G, et al. Recurrence and survival outcomes after anatomic segmentectomy versus lobectomy for clinical stage I nonsmall-cell lung cancer: a propensity-matched analysis. J Clin Oncol. (2014) 32:2449–55. doi: 10.1200/JCO.2013.50.8762
26. Izbicki JR, Passlick B, Pantel K, Pichlmeier U, Hosch SB, Karg O, et al. Effectiveness of radical systematic mediastinal lymphadenectomy in patients with resectable non-small cell lung cancer: results of a prospective randomized trial. Ann Surg. (1998) 227:138–44. doi: 10.1097/00000658-199801000-00020
27. Dai C, Ren Y, Xie D, Zheng H, She Y, Fei K, et al. Does lymph node metastasis have a negative prognostic impact in patients with NSCLC and M1a disease? J Thorac Oncol. (2016) 11:1745–54. doi: 10.1016/j.jtho.2016.06.030
28. Chansky K, Sculier JP, Crowley JJ, Giroux D, Van Meerbeeck J, Goldstraw P, et al. , International Staging Committee and Participating Institutions. The International Association for the Study of Lung Cancer Staging Project: prognostic factors and pathologic TNM stage in surgically managed non-small cell lung cancer. J Thorac Oncol. (2009) 4:792–801. doi: 10.1097/JTO.0b013e3181a7716e
29. Wang BY, Huang JY, Cheng CY, Lin CH, Ko J, Liaw YP. Lung cancer and prognosis in Taiwan: a population-based cancer registry. J Thorac Oncol. (2013) 8:1128–35. doi: 10.1097/JTO.0b013e31829ceba4
30. Evangelista L, Cuocolo A, Pace L, Mansi L, Del Vecchio S, Miletto P, et al. Performance of FDG-PET/CT in solitary pulmonary nodule based on pre-test likelihood of Malignancy: results from the ITALIAN retrospective multicenter trial. Eur J Nucl Med Mol Imaging. (2018) 45:1898–907. doi: 10.1007/s00259-018-4016-1
31. Galera A. The impact of lamarck’s theory of evolution before darwin’s theory. J Hist Biol. (2016) 50:1–18. doi: 10.1007/s10739-015-9432-5
32. Zhang CL, Gao MQ, Jiang XC, Pan X, Zhang XY, Li Y, et al. Research progress and value of albumin-related inflammatory markers in the prognosis of nonsmall cell lung cancer: A review of clinical evidence. Ann Med. (2023) 55:1294–307. doi: 10.1080/07853890.2023.2192047
Keywords: lung cancer, PET/CT, prognosis, 3D quantitative technology, NSCLC
Citation: Su Y, Qiu S and Wang J (2025) The value of 18F-FDG PET/CT combined with 3D quantitative technology and clinicopathological features in predicting prognosis of NSCLC. Front. Oncol. 15:1533569. doi: 10.3389/fonc.2025.1533569
Received: 24 November 2024; Accepted: 17 March 2025;
Published: 08 April 2025.
Edited by:
Daniela Gabbia, University of Padova, ItalyReviewed by:
Salvatore Annunziata, Fondazione Policlinico Universitario A. Gemelli IRCCS, ItalyShenhai Wei, First Hospital of Tsinghua University, China
Copyright © 2025 Su, Qiu and Wang. This is an open-access article distributed under the terms of the Creative Commons Attribution License (CC BY). The use, distribution or reproduction in other forums is permitted, provided the original author(s) and the copyright owner(s) are credited and that the original publication in this journal is cited, in accordance with accepted academic practice. No use, distribution or reproduction is permitted which does not comply with these terms.
*Correspondence: Yuling Su, eGN6MjAxMzA4QDE2My5jb20=