- Department of Oncology, Shengjing Hospital of China Medical University, Shenyang, China
Objective: To conduct an umbrella review of prospective meta-analyses and perform a causal relationship analysis to evaluate causal effects.
Methods: PubMed, Web of Science, Embase, and manual reference list searches were used from database inception to July 27, 2023. Meta-analyses of prospective studies on non-genetic risk factors for breast cancer incidence were included. Overlapping articles were assessed using corrected coverage area. We utilized the AMSTAR-2 criteria to evaluate methodological quality and graded each meta-analysis to assess the strength of evidence. This study is registered with PROSPERO (CRD42023470151). We further explored the causal impacts.
Results: Risk factors were classified into 11 categories. Among the 281 meta-analyses of cohort studies, five (1.8%) provided strong evidence, eight (2.8%) indicated highly suggestive evidence, and 23 (8.2%) and 55 (19.6%) showed suggestive and weak evidence, respectively. Breast density (2.89; 2.57-3.25), cardiac glycoside (1.39; 1.33-1.45), atrial fibrillation (1.18; 1.14-1.22), vegetable-fruit-soybean dietary pattern (0.87; 0.83-0.92), and postmenopausal women with BMI ≥25 (0.86; 0.81-0.91) were strongly associated with breast cancer incidence. For all associations graded as weak evidence or higher, further confirmed the causal relationship between BMI, fruit intake, calcium channel blockers, cheese intake, insulin like growth factor-1 levels, serum triglyceride levels causally
Discussion: Identifying primary risk factors is crucial for delineating high-risk populations among women, facilitating tailored prevention strategies and advancing investigations into underlying mechanisms.
Systematic review registration: https://www.crd.york.ac.uk/PROSPERO/, identifier CRD42023470151.
1 Introduction
By 2023, breast cancer (BC) accounted for 11.6% of all cancer diagnoses worldwide, with 2.3 million new cases representing 31% of diagnoses in women. It is the second most common cancer globally, following lung cancer, and the most common cancer among women (1, 2). Despite medical advances, BC incidence continues to rise (1). Identifying and mitigating risk factors is crucial to addressing the growing BC burden. Genetic factors such as BRCA gene mutations contribute to BC occurrence (3) but offer limited preventive value. Recent research has highlighted modifiable factors, such as environmental and lifestyle influences, that impact BC risk (4–8). Mitigating these non-genetic risk factors is crucial for lowering BC incidence. However, owing to the impracticality of studying these exposures through randomized controlled trials, these studies may introduce inherent biases, including selection bias (i.e., inappropriate participant selection) and information bias (i.e., inaccurate data collection), which could lead to either an overestimation or underestimation of the results. Furthermore, wide effect size ranges, and even conflicts often exhibited. For example, Kast et al. (9) reported that a higher BMI in young adults is associated with a reduced risk of BC. In contrast, Fakhri et al. (10) found that obese women have a higher risk of BC compared to those with a BMI below 30. In this context, meta-analysis is a useful tool to address studies with varying effect sizes and directions.
Nevertheless, recently, as original research continuously updates and more meta-analyses emerge, there are obvious discrepancies in findings, even within the same topic. For instance, two recent meta-analyses investigating the relationship between atrial fibrillation (AF) and breast cancer (BC) incidence reached entirely contradictory conclusions (11, 12). The umbrella review effectively evaluate these diverse results, combining systematic reviews and meta-analyses on a given topic, assessing sample size, association strength, heterogeneity, and bias.
However, establishing causality is challenging with observational research. Mendelian randomization (MR) uses genetic variation as a proxy for exposure, reducing confounding factors and enhancing causal inference (13, 14). Pearson-Stuttard et al. (15) explored the risk of developing multiple-site cancers with type 2 diabetes using an umbrella review and MR, exemplifying effective methodology.
Therefore, we aimed to conduct an umbrella review to explore BC risk factors and perform a MR analysis to evaluate the causal effects.
2 Materials and methods
2.1 Literature search and selection criteria
We conducted a comprehensive search using keywords across PubMed, Web of Science, and Embase databases. Our search encompassed meta-analyses that examined the association between non-genetic risk factors for BC from database inception to July 27, 2023. Supplementary Table 1 outlines the complete search strategy. We also manually reviewed reference lists of eligible studies.
This study followed the Preferred Reporting Items for Systematic Reviews and Meta-analyses (PRISMA) reporting guideline (16) and was registered at PROSPERO (https://www.crd.york.ac.uk/PROSPERO/) with CRD42023470151.
Two authors independently searched the databases, screened titles and abstracts, and identified meta-analyses meeting the inclusion criteria through full-text reading. A third author resolved any discrepancies. Inclusion criteria involved meta-analyses with: 1. observational cohort study designs; 2. non-genetic risk factors as the exposure of interest; 3. BC as the reported outcome; 4. available risk estimates between risk factors and BC (risk ratio, odds ratio, hazard ratio) with 95% confidence intervals (CIs), number of cases/controls, or total population size; 5. publication in English; and 6. study population comprising women. We excluded meta-analyses of genetic markers, systematic reviews without quantitative analyses, animal or laboratory studies, and reviews lacking study-specific data (risk ratio, odds ratio, hazard ratio) that could not be retrieved from the original studies. We also excluded studies with baseline populations already diagnosed with cancer.
2.2 Overlapping and outdated reviews
When two or more reviews examine the same exposure and outcome, overlapping associations may result in multiple primary studies being included in the reviews. Additionally, research indicates that half of published reviews become outdated within 5.5 years. We first identified meta-analyses with identical risk factors and outcomes to mitigate bias in interpreting such outcomes. Subsequently, we:
1. Selected the most recent literature for reviews with the same author.
2. Excluded outdated overlapping reviews published before 2018 with different authors.
3. Assessed the extent of overlap using a generated graphical cross-table (citation matrix) for reviews published before or after 2018 with different authors and used an index termed corrected coverage area (CCA) to quantify the degree of overlap, calculated as CCA = (N - r)/(r * c - r) * 100%, where N: the total number of primary studies across all reviews, r: rows, and c: columns, expressed as a percentage.
Overlap was categorized as follows: 1. very high: CCA >15%, 2. high: CCA=11-15%, 3. moderate: CCA=6-10%, and 4. slight: CCA=0-5% (17). Both reviews were retained for slight or moderate overlap. For high or very high overlap, the review with the highest quality based on the AMSTAR 2 tool was prioritized. The most recent review was included when quality ratings were identical (18, 19).
2.3 Data extraction
Two reviewers independently extracted data, including the first author’s name, journal name, publication year, study design, exposure factors, health outcomes, included studies, cases, total participants, and the estimated summary effects with 95% CIs, from eligible studies. Additionally, if available, information relevant to dose-response relationships was retrieved from meta-analyses. To ensure transparency, a third author reviewed the discrepancies between the two reviewers and resolved them by considering all relevant data and methodologies.
2.4 Evaluation of the quality of included meta-analyses
Two reviewers (WZ and XYQ) evaluated the methodological quality of the included studies using the AMSTAR-2 tool (20), assigning an overall score. In case of disagreement, a third author (GS) was consulted. AMSTAR-2 assesses 16 items, with 7 considered critical. Deficiencies in any critical item may affect the overall review quality. The key areas deemed crucial included: protocol registered before the commencement of the review; adequacy of the literature search; justification for excluding individual studies; risk of bias in the included studies; appropriateness of meta-analytical methods; considering bias risk when interpreting results; and assessment of the presence and likely impact of publication bias. The final ratings were classified into four levels ranging from “high” to “very low”: 1. high: no defects or one non-critical area with defects; 2. moderate: more than one non-critical area with defects; 3. low: one critical area with or without non-critical areas with defects and 4. very low: more than one critical area with or without non-critical areas with defects. AMSTAR-2 scoring results assess the quality of the included studies and account for potential biases and methodological limitations, providing a more reliable interpretation of results.
2.5 Evaluating the strength of evidence using grading criteria
BC risk factors were categorized into four evidence-based classes: class I (strong evidence), class II (highly suggestive evidence), class III (suggestive evidence), and class IV (weak evidence) (21, 22) (Table 1). This classification system offers an objective, standardized approach, consistent with other grading schemes in cancer epidemiology. This classification method enables the study to rank the strength of evidence for each risk factor. class I and II evidence correspond to factors with a high level of confidence, whereas class III and IV represent factors with lower confidence. Specifically, class IV (weak evidence) factors are associated with reduced confidence, which may be influenced by factors such as data heterogeneity, insufficient sample size, or methodological limitations.
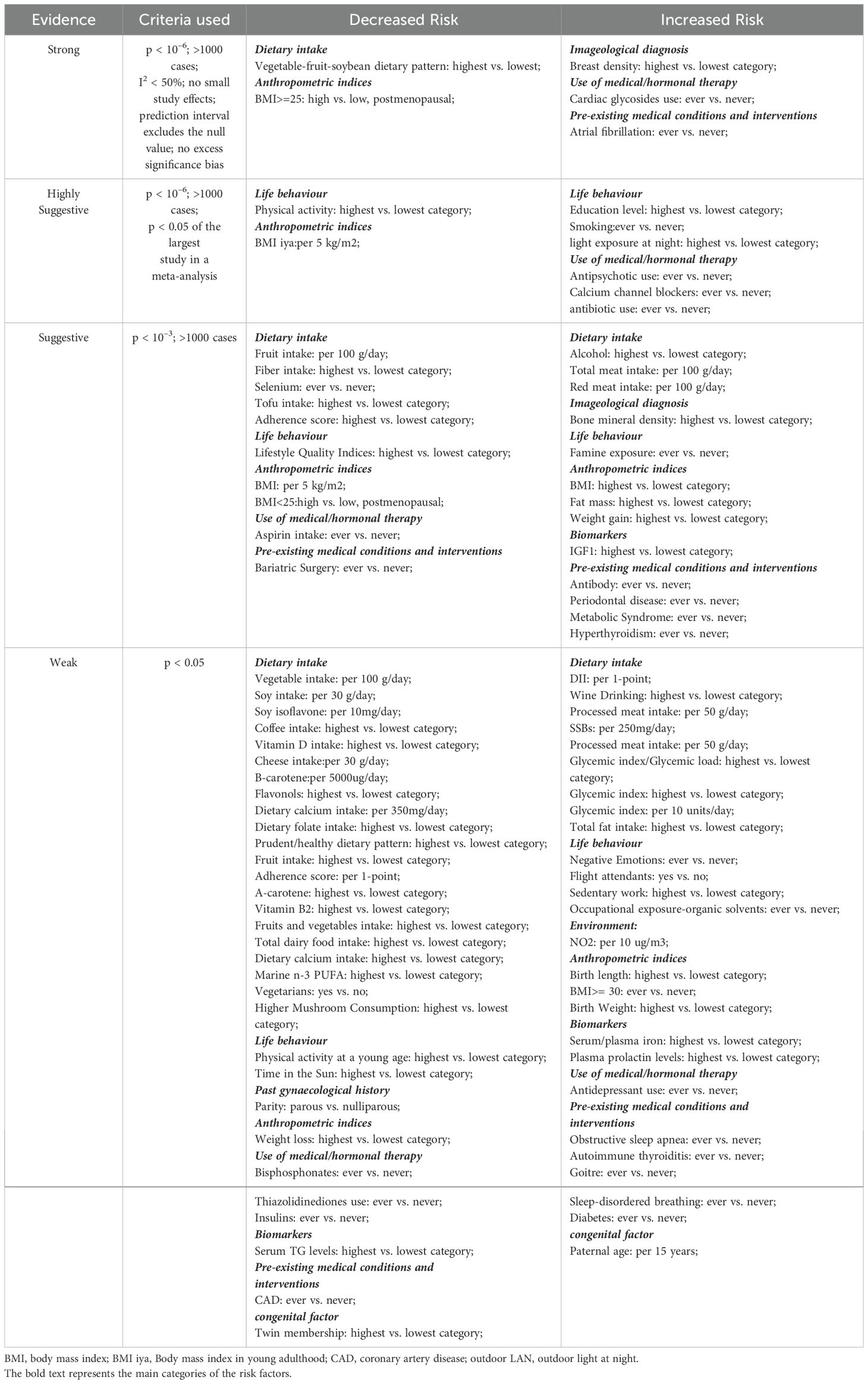
Table 1. Evidence grading for meta-analyses of risk factors associated with breast cancer incidence (cohort studies only).
2.6 Data analysis
We focused on cohort studies, recalculating summary effects and 95% CIs using random- or fixed-effects models (23). Heterogeneity was evaluated using the I² statistic and 95% predicted intervals (PIs) (24–26) to account for variability across studies and reduce its impact on the results. We evaluated the presence of publication bias by assessing small-study effects and excess significance bias. Egger’s regression asymmetry test (P<.10) and whether the summary estimate of random effects exceeded the point estimate of the largest study in the meta-analysis were used to assess small-study effects (27). Additionally, sensitivity analyses were conducted on all eligible cohort and case-control studies using the same criteria as those used in the primary analysis. R software version 4.3.0 was used for all statistical analyses (http://cran.r-project.org/).
2.7 MR
We performed a two-sample MR analysis using genetic variants as proxies for exposure to explore the causal impacts of non-genetic risk factors on BC (28) Genome-wide association study (GWAS) catalogs were queried for exposures rated as weak evidence or higher in the umbrella review to find relevant GWAS offering summary-level genetic information (29). We used data from previous GWAS to ascertain the association between single nucleotide polymorphisms (SNPs) and BC risk (30).
Given the necessity of MR analysis to validate the three core assumptions (31), we established stringent criteria for selecting the instrumental variables (IVs) to ensure the robustness and reliability of the results (32). First, we set a significance threshold of P<5e−08 to choose SNPs as IVs for each exposure. Second, we addressed linkage disequilibrium (LD) between SNPs by excluding those with strong LD (r2 = 0.001, κb=10,000 kb). The PhenoScanner database was used to mitigate the effects of confounding factors. Finally, we computed the F-statistic for all selected SNPs to evaluate weak instrument bias, excluding SNPs with an F-statistic <10, to ensure that all remaining SNPs were strongly associated with exposure (31).
Causal impacts were estimated using the inverse variation-weighted (IVW) MR as the primary analysis. In instances where exposure exhibited significant effects in the main IVW MR analysis, we applied various robust MR methods (such as the weighted median and MR-Egger) in the sensitivity analyses to address potential violations of the IV assumption (33). We systematically employed leave-one-out modeling to evaluate the potential influence of outliers and pleiotropic SNPs (34). We excluded one SNP at a time to determine whether individual SNPs affected the primary causal relationship. Considering the potential for genetic pleiotropy, interactions, and confounding effects among the different exposure factors, we conducted multivariable MR (MVMR) analyses (35) to assess the direct effects of various exposure factors on BC. To further investigate reverse causation, we performed a reverse MR analysis using BC-related SNPs as IVs (treating BC as the exposure and various risk factors as outcomes).
3 Results
3.1 Literature retrieval and selection
Figure 1 depicts the literature retrieval and selection process. Up to July 2023, we retrieved 58,242 articles. After screening and following the exclusion of duplicate meta-analyses based on assessed exposure and outcome (36–51) (Supplementary Table 7), 218 articles, including 427 meta-analyses remained (52–269).
3.2 Characteristics of meta-analyses
Among the 427 included meta-analyses, the estimated values from studies ranged from 2 to 69 (median, 10). The median number of cases and individuals for each meta-analysis were 14,055 and 3,328,403, respectively. The minimum and maximum numbers of cases in meta-analyses were 138 and 591,297, respectively. The smallest total population was 1,083, whereas the largest was 18,281,388. Furthermore, 403 of the 427 meta-analyses included >1,000 patients with BC.
From the pool of 427 meta-analyses, 281 consisted exclusively of cohort studies, including at least two that evaluated 202 risk factors and categorized into 10 major groups. (Figure 2 and Supplementary Table 2).
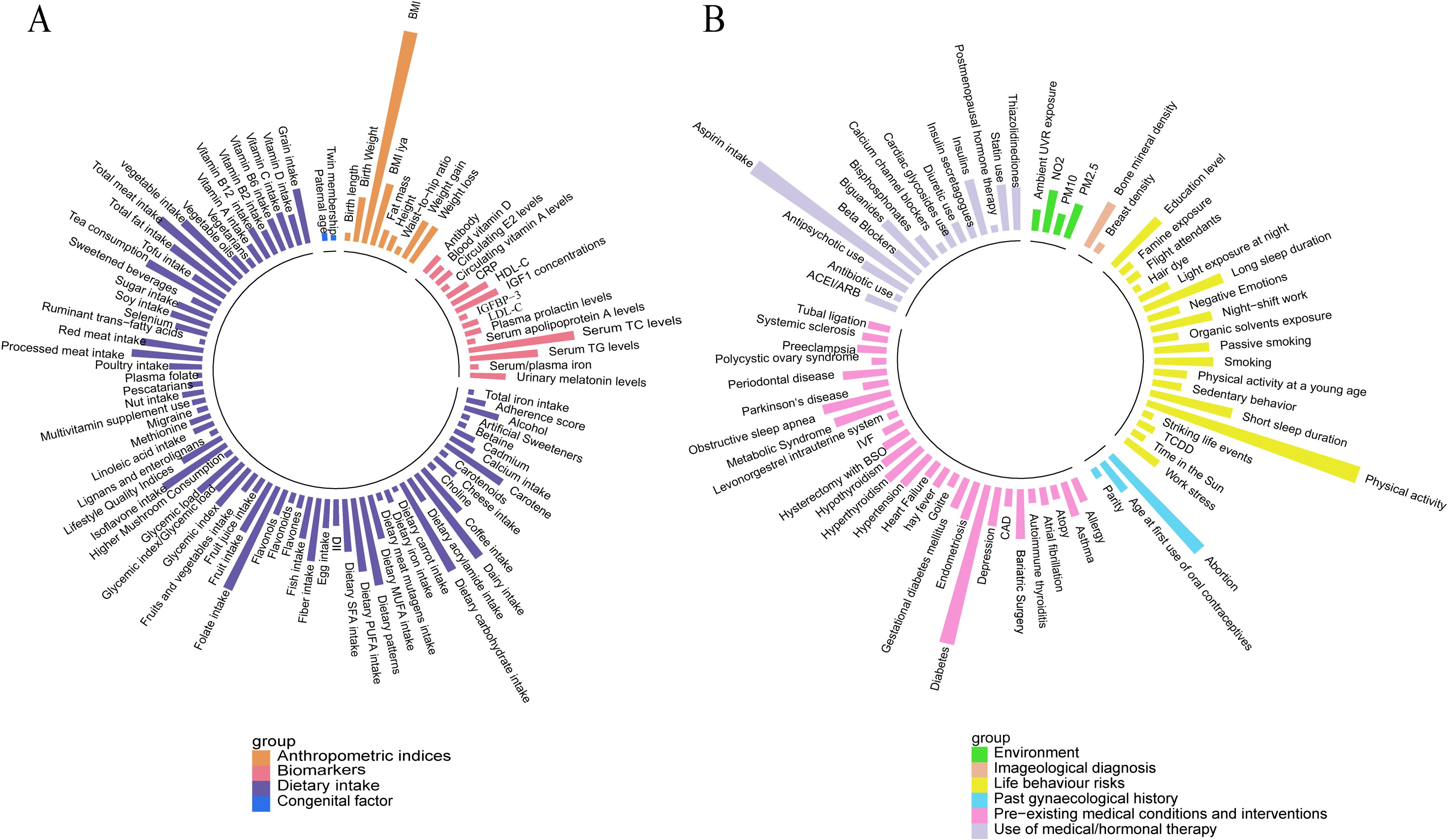
Figure 2. Overall presentation of associations with the risk of breast cancer (cohort studies only) (A) Anthropometric indices, biomarkers, congenital factors, and dietary intake. (B) Environment, imageological diagnosis, lifestyle behavior, gynecological history, pre-existing medical conditions and interventions, and medical/hormonal therapy use.
3.3 Quality assessment
We evaluated the methodological quality of 218 studies, which included meta-analyses of 427 observational studies, using the AMSTAR-2 tool (Supplementary Table 4). Overall, most meta-analyses exhibited low to very low quality owing to various factors such as study design limitations and reduced methodological rigor (such as potential publication bias, indirectness, and inconsistency). Specifically, only a small proportion was rated as having “high” or “moderate” quality.
3.4 Main analysis
3.4.1 Summary effect size
When using P<.05 as the threshold for statistical significance, among the 281 studies solely comprising cohort studies, 150 (53%) and 115 (41%) meta-analyses presented significant summary fixed- and random-effects estimates, respectively (Supplementary Table 2). Applying a threshold of P<.001, 78 (28%) and 40 (14%) studies produced significant findings using summary fixed- and random-effects models, respectively. In case of P<10-6, 49 (17%) and 21 (7%) studies provided significant summary fixed- and random-effects estimates, respectively. In meta-analyses where the random-effects estimate had P<10-6, 13 reported different risk factors associated with an increased BC incidence. These thresholds were selected in alignment with the evidence grading system used in this study, which allow for a clearer distinction of the strength and reliability of the findings at each level of evidence.
3.4.2 Heterogeneity between studies
We reanalyzed 281 meta-analyses using random or fixed-effects models and found that 91 (32%) exhibited significant heterogeneity (I² = 50–75%). Notably, 33 (12%) of the meta-analyses showed substantial heterogeneity (I² > 75%) (Supplementary Table 3). The heterogeneity observed in most outcomes can be attributed to several factors, including study design, sample size, study quality, environmental influences, and population characteristics. When calculating the 95% PIs, the null hypothesis was excluded in 22 studies, including alcohol, smoking, glycemic index, glycemic index/glycemic load, coffee intake, breast density, IGF-1 concentrations, serum triglyceride (TG) levels and the like.
3.4.3 Grading the evidence
Evidence from 46 meta-analyses suggested the presence of small-study effects. Moreover, evidence of excessive significant bias (P<.10) was observed in 65 meta-analyses with different exposures, including BMI, total meat intake, vegetable intake, metabolic syndrome, dietary calcium intake, and PA. For further details, please refer to Supplementary Table 3.
3.4.4 Grading the evidence
We classified the strength of evidence for each risk factor associated with BC (Table 1). Moreover, Supplementary Table 5 presents information on elaborate explanations of the assessment criteria (specifically for cohort studies), whereas Supplementary Table 6 details the outcomes for all studies.
Among the 281 meta-analyses included in the primary analysis, only five (1.8%) met the criteria for strong evidence. Including breast density (2.89; 95% CI: 2.57-3.25), cardiac glycosides use (1.39; 95% CI: 1.33-1.45), AF history (1.18; 95% CI: 1.14-1.22), adherence to a vegetable-fruit-soybean dietary pattern (0.87; 95% CI: 0.83-0.92) and Postmenopausal women with a BMI ≥25 (0.86high vs. low; 95% CI: 0.81-0.91) (Table 1, Supplementary Table 5). Eight analyses (2.8%) provided highly suggestive evidence (Table 1, Figure 3). Twenty-three analyses (8.2%) provided suggestive evidence, 55 (19.6%) provided weak evidence, and the remaining showed no significant association.
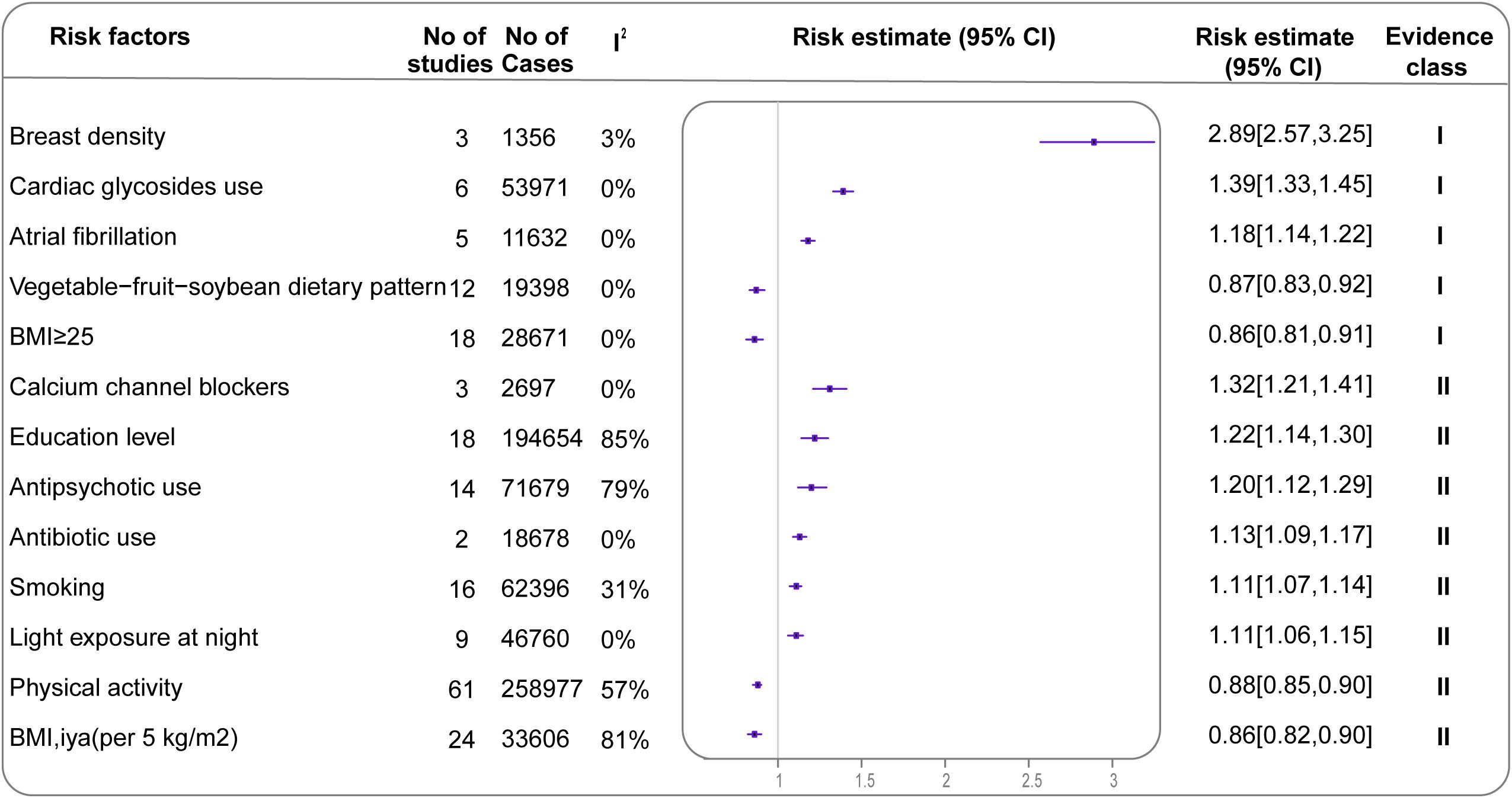
Figure 3. Forest plot of effect estimates and 95% CIs for all exposures associated with BC in the main analysis (summary random effects for cohort studies only) Evidence is graded as strong or highly suggestive (n=13). CI, confidence interval; BC, breast cancer.
3.5 Sensitivity analyses
When cohort and case-control studies were included (Supplementary Table 6), additional four exposure factors associated with increased BC incidence provided strong evidence: autoimmune thyroiditis (2.71; 95% CI: 2.13-3.43), weight gain (1.55; 95% CI: 1.40-1.71), oral progestogen (1.28; 95% CI: 1.19-1.39), and light exposure at night (1.13; 95% CI: 1.09-1.16). Strong evidence for two exposures reducing BC incidence was also found: number of births (0.79; 95% CI: 0.75-0.83) and sex hormone-binding globulin (0.65; 95% CI: 0.58-0.73); both associations were only included in case-control studies and were not evaluated in the main analysis. Conversely, when case-control studies were included, the strong correlations between breast density, BMI ≥25 (high vs. low, postmenopausal), and BC risk were downgraded to highly suggestive evidence (2.89; 95% CI: 2.57-3.25) and not significant (0.86; 95% CI: 0.81-0.91), whereas the remaining three strong associations remained strong.
3.6 MR
In the MR analysis, 22 risk factors had available genetic instruments (Supplementary Table 8). The genetically predicted IGF-1 concentrations demonstrated a correlation with BC (1.08; 95% CI: 1.02-1.14) (Figure 4, Supplementary Table 9). Additionally, higher fruit intake (0.64; 95% CI: 0.46-0.90), cheese intake (0.82; 95% CI: 0.69-0.98), higher serum TG levels (0.91; 95% CI: 0.86-0.97) and higher BMI category (0.82; 95% CI: 0.71-0.95) were found to have protective effects against BC. The sensitivity analysis yielded directions consistent with the main analysis, supporting potential causal effects (Supplementary Figures 1, 2, Supplementary Table 10). Reverse MR-IVW analysis (Supplementary Figure 3) indicated a potential bidirectional relationship between calcium channel blockers (CCBs) and BC. No other exposures demonstrated a similar reverse causal association with BC incidence.
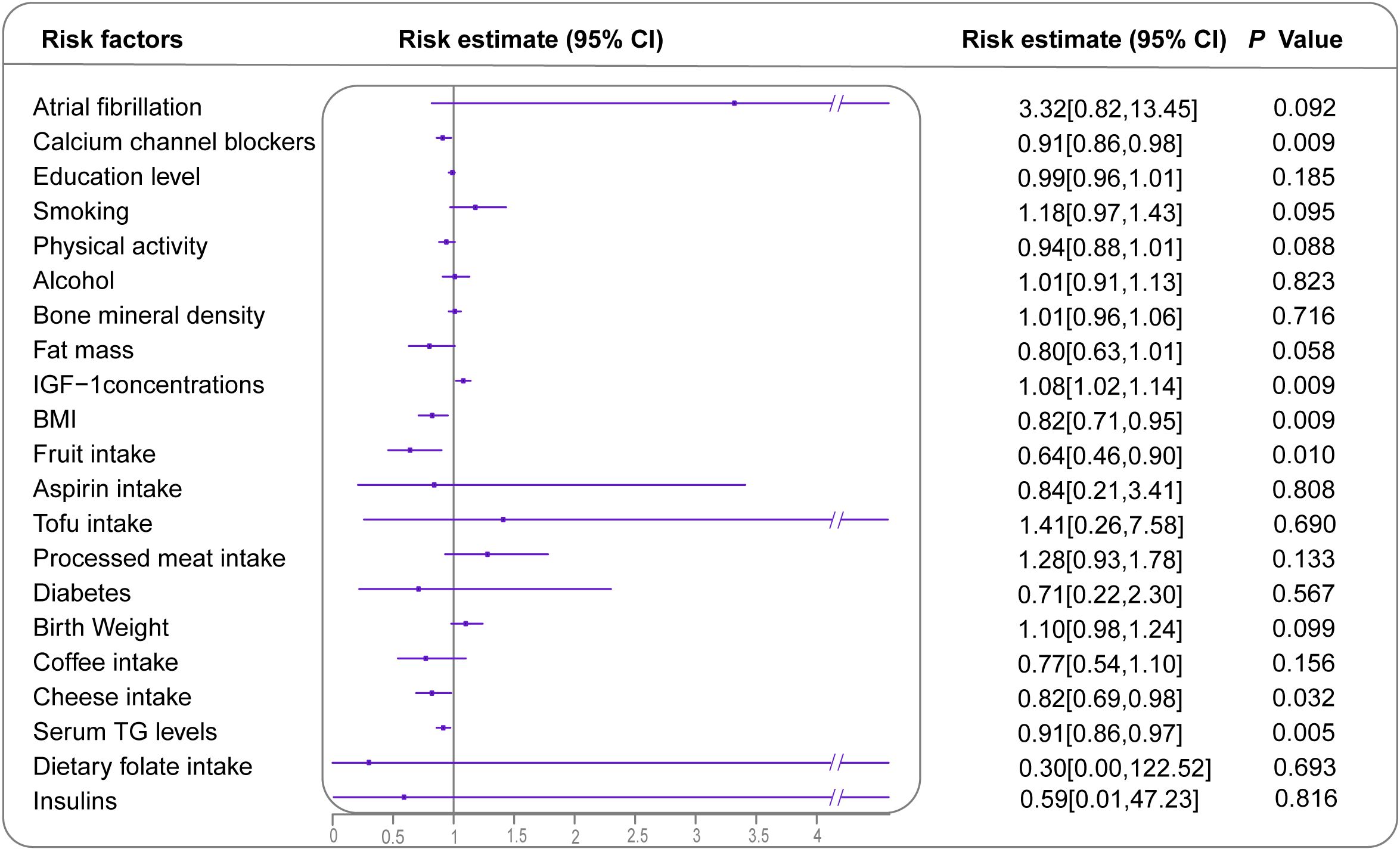
Figure 4. Forest plot demonstrating inverse variance weighted MR results for all identified risk or protective factors for BC with available GWAS. Effect sizes are presented as ORs with 95% CIs. However, the OR for goiter is 28.76 [0.00, 509785.17], which is significantly higher than other values, and is, therefore, not displayed. MR, Mendelian randomization; BC, breast cancer; GWAS, genome-wide association studies; OR, odds ratio; CI, confidence interval.
In the MVMR analysis, genetic predictions of CCBs and IGF-1 concentrations showed independent associations with BC after adjusting for BMI and serum TG levels. Similarly, the genetic predictions of fruit and cheese intake were independently linked to a decreased risk of BC (Supplementary Table 11).
4 Discussion
This umbrella review analyzed data from 427 meta-analyses, with 281 including at least two cohort studies. In the primary analysis, only five meta-analyses provided strong evidence regarding BC, showing significant strength and no bias. Increased breast density, AF history, and cardiac glycoside use were linked to an elevated BC risk. Conversely, adherence to a vegetable-fruit-soybean dietary pattern was associated with a decreased BC incidence. Furthermore, BMI inversely correlated with BC risk among postmenopausal women with a BMI ≥25. Using MR analysis, we identified causal effects between six risk factors and BC: BMI, CCBs, fruit intake, cheese intake, IGF-1 levels, and serum TG levels. CCBs exhibited bidirectional effects on BC.
A meta-analysis examining the relationship between AF history and cardiac glycoside use in BC incidence has garnered substantial evidence. Prior investigations into whether AF increases BC incidence have produced conflicting findings, with this link absent in the WCRF CUP report (270). Hence, our evidence grading system offers valuable supplementary insights. Some studies have proposed that the increased BC risk might stem from shared risk factors such as obesity, diabetes, and unhealthy lifestyles. Nonetheless, these assertions require further validation using clinical data. Another suggested mechanism linking AF to BC involves a systemic inflammatory response (271, 272); however, large-scale epidemiological studies have not confirmed this link. Additionally, cardiac glycosides, particularly digoxin, emerged as potent BC risk factors in our study, possibly because of their estrogenic properties and binding to estrogen receptors (273). In a sizable prospective study involving postmenopausal women (274), adjustment for multiple variables revealed a heightened risk of incident BC associated with AF in women; however, further adjustment for cardiac glycosides mitigated this risk, indicating their potential intermediary role.
Our meta-analysis revealed strong evidence supporting an inverse relationship between BMI and BC incidence, irrespective of menopausal status. Our results are consistent with those of the 2018 WCRF CUP report. Several mechanisms may explain the inverse correlation between BMI and BC risk. First, studies have indicated a negative correlation between breast density and BMI (275, 276), with women who are obese or overweight exhibiting lower breast density and, thus, a reduced BC risk. Second, Among obesity-related protein biomarkers, lower adiponectin levels and higher leptin and IGF-1 levels were associated with an increased BC risk. Adiponectin levels were negatively correlated with BMI and leptin concentration in women (277). Reduced adiponectin levels may enhance insulin signaling (278), which is associated with tumor growth. Another adipokine, leptin, is a key molecular mediator of the relationship between obesity and BC and is overexpressed in individuals who are obese or overweight. Finally, IGF-1, which is mediated by the IGF-1 receptor, is implicated in BC development and progression by regulating proliferation and survival genes via multiple signaling pathways (279). Our comprehensive review, supported by the MR analysis, confirmed the causal relationship between increased IGF-1 concentrations and elevated BC risk, further strengthening our findings.
Substantial suggestive evidence indicates an association between CCB use and an increased BC risk, as confirmed through the MR analysis. Prior studies, such as that by Thakur et al. (174), have suggested an elevated risk of BC with CCB use, whereas Wright et al. (280) indicated no significant association. These differing conclusions likely stem from the high heterogeneity in study design, population, and follow-up duration (281). However, the mechanisms underlying the effect of CCBs on BC risk remain unclear (282). In vitro CCB treatment has been shown to upregulate pathways related to breast cell proliferation and migration (283), whereas calcium-dependent processes exhibit tumor-suppressive effects in BC (284). Moreover, the MR reverse causation analysis suggested that BC influences the use of CCBs. This finding reveals a complex bidirectional relationship, highlighting the need for additional research on its mechanisms and clinical implications. Given the uncertainty surrounding the underlying mechanisms, this area presents a novel and important avenue for future studies, particularly in investigating causality pathways and their potential roles in BC risk.
Our study has several strengths. First, we prioritized prospective cohort studies as the main analysis to avoid the influence of epidemiological bias as much as possible. At the same time, in order to make the results comprehensive, all observational studies including case-control studies were further included in the sensitivity analysis, and the differences were discussed. Overlapping articles were assessed using CCA, and the highest quality or most recent reviews of overlapping articles were included to avoid duplicate inclusion. We employed comprehensive and robust methodologies, ensuring the rigor and reliability of our findings. Moreover, the large sample size further enhanced the credibility and precision of our results. Finally, given the inherent limitations of inferring causation from observational studies, this pioneering effort amalgamated umbrella reviews with bidirectional two-sample MR studies in the BC risk domain, providing novel insights into the potential and reverse causal relationships among risk exposures.
However, this study has some limitations. First, it relies on literature retrieval by the original authors and findings from past meta-analyses, potentially leading to some studies being overlooked. Second, we found significant heterogeneity across studies, likely attributable to differences in study design, sample characteristics, and measurement methods. Although random effects models and sensitivity analyses were employed to account for heterogeneity, its impact could not be completely eliminated. Furthermore, the AMSTAR-2 assessment indicated that most of the included studies were of low quality, suggesting a potential risk of bias. Despite conducting bias tests, the exact sources of bias could not be definitively identified. Future research should implement more consistent study designs and enhance methodological quality to minimize both heterogeneity and bias. Third, studies that separately reported the results for pre- and postmenopausal women were limited. Consequently, stratified analyses based on menopausal status were not conducted in this study, potentially overlooking the differential effects of certain exposures owing to differences in menopausal status. Fourth, the umbrella review identified numerous significant exposures. However, the MR analysis has limitations owing to genetic instrument constraints and sample size issues, resulting in fewer established causal effects. This limitation does not imply that the exposures not identified in this analysis lack causal effects, nor does it confirm the absolute accuracy of the conclusions drawn from the MR analysis.
This umbrella review synthesized meta-analyses focusing on the risk factors associated with BC. The MR analysis elucidated the causal relationships between specific risk factors and BC incidence. Identifying these factors facilitates the development of targeted preventive strategies for high-risk populations and investigations into their underlying mechanisms.
Data availability statement
The original contributions presented in the study are included in the article/Supplementary Material. Further inquiries can be directed to the corresponding authors.
Author contributions
ZW: Conceptualization, Data curation, Formal Analysis, Methodology, Software, Writing – original draft, Writing – review & editing. LF: Investigation, Methodology, Validation, Visualization, Writing – original draft. YX: Data curation, Software, Validation, Visualization, Writing – original draft. ZZ: Data curation, Writing – original draft. LW: Investigation, Project administration, Resources, Supervision, Writing – review & editing. SG: Conceptualization, Funding acquisition, Project administration, Supervision, Writing – review & editing.
Funding
The author(s) declare that financial support was received for the research and/or publication of this article. This study was supported in part by Natural Science Foundation of Liaoning Province. (No. 2023JH2/101700135).
Acknowledgments
The authors would like to thank all the participants in this study, and those all who provide help to this study.
Conflict of interest
The authors declare that the research was conducted in the absence of any commercial or financial relationships that could be construed as a potential conflict of interest.
Generative AI statement
The author(s) declare that no Generative AI was used in the creation of this manuscript.
Publisher’s note
All claims expressed in this article are solely those of the authors and do not necessarily represent those of their affiliated organizations, or those of the publisher, the editors and the reviewers. Any product that may be evaluated in this article, or claim that may be made by its manufacturer, is not guaranteed or endorsed by the publisher.
Supplementary material
The Supplementary Material for this article can be found online at: https://www.frontiersin.org/articles/10.3389/fonc.2025.1541233/full#supplementary-material
References
1. Siegel RL, Miller KD, Wagle NS, Jemal A. Cancer statistics, 2023. CA Cancer J Clin. (2023) 73:17–48. doi: 10.3322/caac.21763
2. Bray F, Laversanne M, Sung H, Ferlay J, Siegel RL, Soerjomataram I, et al. Global cancer statistics 2022: GLOBOCAN estimates of incidence and mortality worldwide for 36 cancers in 185 countries. CA Cancer J Clin. (2024) 74:229–63. doi: 10.3322/caac.21834
3. Clusan L, Ferriere F, Flouriot G, Pakdel F. A basic review on estrogen receptor signaling pathways in breast cancer. Int J Mol Sci. (2023) 24(7):6834. doi: 10.3390/ijms24076834
4. Slepicka PF, Cyrill SL, Dos Santos CO. Pregnancy and breast cancer: pathways to understand risk and prevention. Trends Mol Med. (2019) 25:866–81. doi: 10.1016/j.molmed.2019.06.003
5. Winters S, Martin C, Murphy D, Shokar NK. Breast cancer epidemiology, prevention, and screening. Prog Mol Biol Transl Sci. (2017) 151:1–32. doi: 10.1016/bs.pmbts.2017.07.002
6. Sun YS, Zhao Z, Yang ZN, Xu F, Lu HJ, Zhu. ZY, et al. Risk factors and preventions of breast cancer. Int J Biol Sci. (2017) 13:1387–97. doi: 10.7150/ijbs.21635
7. Loibl S, Poortmans P, Morrow M, Denkert C, Curigliano G. Breast cancer. Lancet. (2021) 397:1750–69. doi: 10.1016/S0140-6736(20)32381-3
8. Hong R, Xu B. Breast cancer: an up-to-date review and future perspectives. Cancer Commun (Lond). (2022) 42:913–36. doi: 10.1002/cac2.12358
9. Kast K, John EM, Hopper JH, Andrieu N, Noguès C, Mouret-Fourme E, et al. Associations of height, body mass index, and weight gain with breast cancer risk in carriers of a pathogenic variant in BRCA1 or BRCA2: the BRCA1 and BRCA2 Cohort Consortium. Breast Cancer Res. (2023) 25:72. doi: 10.1186/s13058-023-01673-w
10. Fakhri N, Chad MA, Lahkim M, Houari A, Dehbi H, Belmouden A, et al. Risk factors for breast cancer in women: an update review. Med Oncol. (2022) 39:197. doi: 10.1007/s12032-022-01804-x
11. Zhang M, Li LL, Zhao QQ, Peng XD, Wu K, Li X, et al. The association of new-onset atrial fibrillation and risk of cancer: A systematic review and meta-analysis. Cardiol Res Pract. (2020) 2020:2372067. doi: 10.1155/2020/2372067
12. Bao Y, Lee J, Thakur U, Ramkumar S, Marwick TH. Atrial fibrillation in cancer survivors - a systematic review and meta-analysis. Cardiooncology. (2023) 9:29. doi: 10.1186/s40959-023-00180-3
13. Sekula P, Del Greco MF, Pattaro C, Kottgen A. Mendelian randomization as an approach to assess causality using observational data. J Am Soc Nephrol. (2016) 27:3253–65. doi: 10.1681/ASN.2016010098
14. Bowden SJ, Bodinier B, Kalliala I, Zuber V, Vuckovic D, Doulgeraki T, et al. Genetic variation in cervical preinvasive and invasive disease: a genome-wide association study. Lancet Oncol. (2021) 22:548–57. doi: 10.1016/s1470-2045(21)00028-0
15. Pearson-Stuttard J, Papadimitriou N, Markozannes G, Cividini S, Kakourou A, Gill D, et al. Type 2 diabetes and cancer: an umbrella review of observational and mendelian randomization studies. Cancer Epidemiol Biomarkers Prev. (2021) 30:1218–28. doi: 10.1158/1055-9965.EPI-20-1245
16. Gates M, Gates A, Pieper D, Fernandes RM, Tricco AC, Moher D, et al. Reporting guideline for overviews of reviews of healthcare interventions: development of the PRIOR statement. BMJ. (2022) 9:e070849. doi: 10.1136/bmj-2022-070849
17. Pieper D, Antoine SL, Mathes T, Neugebauer EA, Eikermann M. Systematic review finds overlapping reviews were not mentioned in every other overview. J Clin Epidemiol. (2014) 67:368–75. doi: 10.1016/j.jclinepi.2013.11.007
18. Okoth K, Chandan JS, Marshall T, Thangaratinam S, Thomas GN, Nirantharakumar K, et al. Association between the reproductive health of young women and cardiovascular disease in later life: umbrella review. BMJ. (2020) 371:m3502. doi: 10.1136/bmj.m3502
19. Pollock M, Fernandes RM, Newton AS, Scott SD, Hartling L. A decision tool to help researchers make decisions about including systematic reviews in overviews of reviews of healthcare interventions. Syst Rev. (2019) 8:29. doi: 10.1186/s13643-018-0768-8
20. Shea BJ, Reeves BC, Wells G, Thuku M, Hamel C, Moran J, et al. AMSTAR 2: a critical appraisal tool for systematic reviews that include randomised or non-randomised studies of healthcare interventions, or both. BMJ. (2017) 358:j4008. doi: 10.1136/bmj.j4008
21. Kalliala I, Markozannes G, Gunter MJ, Paraskevaidis E, Gabra H, Mitra A, et al. Obesity and gynaecological and obstetric conditions: umbrella review of the literature. BMJ. (2017) 359:j4511. doi: 10.1136/bmj.j4511
22. Kyrgiou M, Kalliala I, Markozannes G, Gunter MJ, Paraskevaidis E, Gabra H, et al. Adiposity and cancer at major anatomical sites: umbrella review of the literature. BMJ. (2017) 356:j477. doi: 10.1136/bmj.j477
23. DerSimonian R, Laird N. Meta-analysis in clinical trials. Control Clin Trials. (1986) 7:3. doi: 10.1016/0197-2456(86)90046-2
24. Higgins. JPT, Thompson. SG, Deeks. JJ, Altman DG. Measuring inconsistency in meta-analyses. BMJ Open. (2003) 327:557–60. doi: 10.1136/bmj.327.7414.557
25. IntHout J, Ioannidis JP, Rovers MM, Goeman JJ. Plea for routinely presenting prediction intervals in meta-analysis. BMJ Open. (2016) 6:e010247. doi: 10.1136/bmjopen-2015-010247
26. Riley RD, Higgins JPT, Deeks JJ. Interpretation of random effects meta-analyses. BMJ. (2011) 10:d549. doi: 10.1136/bmj.d549
27. Egger M, Smith GD, Schneider M, Minder C. Bias in meta-analysis detected by a simple, graphical test. BMJ. (1997) 13:629–34. doi: 10.1136/bmj.315.7109.629
28. Burgess S, Foley CN, Zuber V. Inferring causal relationships between risk factors and outcomes from genome-wide association study data. Annu Rev Genomics Hum Genet. (2018) 19:303–27. doi: 10.1146/annurev-genom-083117-021731
29. Sollis E, Mosaku A, Abid A, Buniello A, Cerezo M, Gil L, et al. The NHGRI-EBI GWAS Catalog: knowledgebase and deposition resource. Nucleic Acids Res. (2023) 51:D977–85. doi: 10.1093/nar/gkac1010
30. Escala-Garcia M, Morra A, Canisius S, Chang-Claude J, Kar S, Zheng W, et al. Breast cancer risk factors and their effects on survival: a Mendelian randomisation study. BMC Med. (2020) 18:327. doi: 10.1186/s12916-020-01797-2
31. Hartwig FP, Borges MC, Horta BL, Bowden J, Davey Smith G. Inflammatory biomarkers and risk of schizophrenia: A 2-sample mendelian randomization study. JAMA Psychiatry. (2017) 74:1226–33. doi: 10.1001/jamapsychiatry.2017.3191
32. Tang P, Guo X, Chong L, Li R. Mendelian randomization study shows a causal effect of asthma on epilepsy risk. Front Immunol. (2023) 14:1071580. doi: 10.3389/fimmu.2023.1071580
33. Burgess S, Butterworth A, Thompson SG. Mendelian randomization analysis with multiple genetic variants using summarized data. Genet Epidemiol. (2013) 37:658–65. doi: 10.1002/gepi.21758
34. Wu F, Huang Y, Hu J, Shao Z. Mendelian randomization study of inflammatory bowel disease and bone mineral density. BMC Med. (2020) 18:312. doi: 10.1186/s12916-020-01778-5
35. Carter AR, Sanderson E, Hammerton G, Richmond RC, Davey Smith G, Heron J, et al. Mendelian randomisation for mediation analysis: current methods and challenges for implementation. Eur J Epidemiol. (2021) 36:465–78. doi: 10.1007/s10654-021-00757-1
36. Wu D, Zhao Z, Chen C, Lu G, Wang C, Gao S, et al. Impact of obstructive sleep apnea on cancer risk: a systematic review and meta-analysis. Sleep Breath. (2023) 27:843–52. doi: 10.1007/s11325-022-02695-y
37. Wei F, Chen W, Lin X. Night-shift work, breast cancer incidence, and all-cause mortality: an updated meta-analysis of prospective cohort studies. Sleep Breath. (2021) 26:1509–26. doi: 10.1007/s11325-021-02523-9
38. Santucci C, Gallus S, Martinetti M, La Vecchia C, Bosetti C. Aspirin and the risk of nondigestive tract cancers: An updated meta-analysis to 2019. Int J Cancer. (2021) 148:1372–82. doi: 10.1002/ijc.33311
39. Li Y, Ma L. The association between coffee intake and breast cancer risk: a meta-analysis and dose-response analysis using recent evidence. Ann Palliat Med. (2021) 10:3804–16. doi: 10.21037/apm-20-1962
40. Chen S, Wu F, Hai R, You Q, Xie L, Shu L, et al. Thyroid disease is associated with an increased risk of breast cancer: a systematic review and meta-analysis. Gland Surg. (2021) 10:336–46. doi: 10.21037/gs-20-878
41. Wei J, Shi Z, Na R, Wang CH, Resurreccion WK, Zheng SL, et al. Germline HOXB13 G84E mutation carriers and risk to twenty common types of cancer: results from the UK Biobank. Br J Cancer. (2020) 123:1356–9. doi: 10.1038/s41416-020-01036-8
42. Ishihara BP, Farah D, Fonseca MCM, Nazario A. The risk of developing breast, ovarian, and endometrial cancer in obese women submitted to bariatric surgery: a meta-analysis. Surg Obes Relat Dis. (2020) 16:1596–602. doi: 10.1016/j.soard.2020.06.008
43. Farvid MS, Spence ND, Holmes MD, Barnett JB. Fiber consumption and breast cancer incidence: A systematic review and meta-analysis of prospective studies. Cancer. (2020) 126:3061–75. doi: 10.1002/cncr.32816
44. Farahmand M, Monavari SH, Shoja Z, Ghaffari H, Tavakoli M, Tavakoli A. Epstein-Barr virus and risk of breast cancer: a systematic review and meta-analysis. Future Oncol. (2019) 15:2873–85. doi: 10.2217/fon-2019-0232
45. Xiao Y, Ke Y, Wu S, Huang S, Li S, Lv Z, et al. Association between whole grain intake and breast cancer risk: a systematic review and meta-analysis of observational studies. Nutr J. (2018) 17:87. doi: 10.1186/s12937-018-0394-2
46. Anderson JJ, Darwis NDM, Mackay DF, Celis-Morales CA, Lyall DM, Sattar N, et al. Red and processed meat consumption and breast cancer: UK Biobank cohort study and meta-analysis. Eur J Cancer. (2018) 90:73–82. doi: 10.1016/j.ejca.2017.11.022
47. Chen Y, Tan F, Wei L, Li X, Lyu Z, Feng X, et al. Sleep duration and the risk of cancer: a systematic review and meta-analysis including dose-response relationship. BMC Cancer. (2018) 18(1):1149. doi: 10.1186/s12885-018-5025-y
48. Turati F, Galeone C, Gandini S, Augustin LS, Jenkins DJ, Pelucchi C, et al. High glycemic index and glycemic load are associated with moderately increased cancer risk. Mol Nutr Food Res. (2015) 59:1384–94. doi: 10.1002/mnfr.201400594
49. Chen Q, Lang L, Wu W, Xu G, Zhang X, Li T, et al. A meta-analysis on the relationship between exposure to ELF-EMFs and the risk of female breast cancer. PloS One. (2013) 8:e69272. doi: 10.1371/journal.pone.0069272
50. Turner LB. A meta-analysis of fat intake, reproduction, and breast cancer risk: an evolutionary perspective. Am J Hum Biol. (2011) 23:601–8. doi: 10.1002/ajhb.21176
51. González-Pérez A, García Rodríguez LA, López-Ridaura R. Effects of non-steroidal anti-inflammatory drugs on cancer sites other than the colon and rectum: a meta-analysis. BMC Cancer. (2003) 3:28. doi: 10.1186/1471-2407-3-28
52. Zhou J, Dai Y, Zuo Z, Liu T, Li S. Famine exposure during early life and risk of cancer in adulthood: A systematic review and meta-analysis. J Nutr Health Aging. (2023) 27(7):550–8. doi: 10.1007/s12603-023-1947-4
53. Ye X, Zhang Y, He Y, Sheng M, Huang J, Lou W. Association between consumption of artificial sweeteners and breast cancer risk: A systematic review and meta-analysis of observational studies. Nutr Cancer. (2023) 75:795–804. doi: 10.1080/01635581.2023.2178957
54. Yao X, Hu Q, Liu X, Ling Q, Leng Y, Zhao H, et al. Atrial fibrillation and breast cancer-Vicious twins? A systematic review and meta-analysis. Front Cardiovasc Med. (2023) 10:1113231. doi: 10.3389/fcvm.2023.1113231
55. Yang J, Zhang S, Jiang W. Impact of beta blockers on breast cancer incidence and prognosis. Clin Breast Cancer. (2023) 23(6):664–71.e21. doi: 10.1016/j.clbc.2023.05.014
56. Yang J, Shen H, Mi M, Qin Y. Isoflavone consumption and risk of breast cancer: an updated systematic review with meta-analysis of observational studies. Nutrients. (2023) 15(10):2402. doi: 10.3390/nu15102402
57. Xiong F, Dai Q, Zhang S, Bent S, Tahir P, Van Blarigan EL, et al. Diabetes and incidence of breast cancer and its molecular subtypes: A systematic review and meta-analysis. (2023) 40(1):e3709. doi: 10.1101/2023.05.13.23289893
58. Wilson RB, Lathigara D, Kaushal D. Systematic review and meta-analysis of the impact of bariatric surgery on future cancer risk. Int J Mol Sci. (2023) 24(7):6192. doi: 10.3390/ijms24076192
59. Wang YT, Hu KR, Zhao J, Ai FL, Shi YL, Wang XW, et al. The association between exposure to second-hand smoke and disease in the chinese population: A systematic review and meta-analysis. BioMed Environ Sci. (2023) 36:24–37. doi: 10.3967/bes2023.003
60. Van Puyvelde H, Dimou N, Katsikari A, Indave Ruiz BI, Godderis L, Huybrechts I, et al. The association between dietary intakes of methionine, choline and betaine and breast cancer risk: A systematic review and meta-analysis. Cancer Epidemiol. (2023) 83:102322. doi: 10.1016/j.canep.2023.102322
61. Shin S, Fu J, Shin WK, Huang D, Min S, Kang D. Association of food groups and dietary pattern with breast cancer risk: A systematic review and meta-analysis. Clin Nutr. (2023) 42:282–97. doi: 10.1016/j.clnu.2023.01.003
62. Armenta-Guirado BI, González-Rocha A, Mérida-Ortega Á, López-Carrillo L, Denova-Gutiérrez E. Lifestyle quality indices and female breast cancer risk: A systematic review and meta-analysis. Adv Nutr. (2023) 14:685–709. doi: 10.1016/j.advnut.2023.04.007
63. Praud D, Deygas F, Amadou A, Bouilly M, Turati F, Bravi F, et al. Traffic-related air pollution and breast cancer risk: A systematic review and meta-analysis of observational studies. Cancers (Basel). (2023) 15(3):927. doi: 10.3390/cancers15030927
64. Peng C, Wu K, Chen X, Lang H, Li C, He L, et al. Migraine and risk of breast cancer: A systematic review and meta-analysis. Clin Breast Cancer. (2023) 23:e122–30. doi: 10.1016/j.clbc.2022.12.011
65. Pan B, Lai H, Ma N, Li D, Deng X, Wang X, et al. Association of soft drinks and 100% fruit juice consumption with risk of cancer: a systematic review and dose-response meta-analysis of prospective cohort studies. Int J Behav Nutr Phys Act. (2023) 20:58. doi: 10.1186/s12966-023-01459-5
66. Tran TV, Kitahara CM, Leenhardt L, de Vathaire F, Boutron-Ruault MC, Journy N. The effect of thyroid dysfunction on breast cancer risk: an updated meta-analysis. Endocr Relat Cancer. (2023) 30(1):e220155. doi: 10.1530/erc-22-0155
67. Malcomson FC, Wiggins C, Parra-Soto S, Ho FK, Celis-Morales C, Sharp L, et al. Adherence to the 2018 World Cancer Research Fund (WCRF)/American Institute for Cancer Research (AICR) Cancer Prevention Recommendations and cancer risk: A systematic review and meta-analysis. Cancer. (2023) 21(1):407. doi: 10.1002/cncr.34842
68. Lou MWC, Drummond AE, Swain CTV, Milne RL, English DR, Brown KA, et al. Linking physical activity to breast cancer via inflammation, part 2: the effect of inflammation on breast cancer risk. Cancer Epidemiol Biomarkers Prev. (2023) 32:597–605. doi: 10.1158/1055-9965.Epi-22-0929
69. Lian Y, Wang GP, Chen GQ, Chen HN, Zhang GY. Association between ultra-processed foods and risk of cancer: a systematic review and meta-analysis. Front Nutr. (2023) 10:1175994. doi: 10.3389/fnut.2023.1175994
70. Heting M, Wenping L, Yanan W, Dongni Z, Xiaoqing W, Zhli Z. Levonorgestrel intrauterine system and breast cancer risk: An updated systematic review and meta-analysis of observational studies. Heliyon. (2023) 9:e14733. doi: 10.1016/j.heliyon.2023.e14733
71. Hassan H, Allen I, Sofianopoulou E, Walburga Y, Turnbull C, Eccles DM, et al. Long- term outcomes of hysterectomy with bilateral salpingo-oophorectomy: a systematic review and meta-analysis. Am J Obstet Gynecol. (2023) 230(1):44–57. doi: 10.1016/j.ajog.2023.06.043
72. Ghoreishy SM, Bagheri A, Nejad MM, Larijani B, Esmaillzadeh A. Association between calcium intake and risk of breast cancer: An updated systematic review and dose–response meta-analysis of cohort studies. Clin Nutr ESPEN. (2023) 55:251–9. doi: 10.1016/j.clnesp.2023.03.026
73. Florez-Garcia VA, Guevara-Romero EC, Hawkins MM, Bautista LE, Jenson TE, Yu J, et al. Cadmium exposure and risk of breast cancer: A meta-analysis. Environ Res. (2023) 219:115109. doi: 10.1016/j.envres.2022.115109
74. Fitzpatrick D, Pirie K, Reeves G, Green J, Beral V. Combined and progestagen-only hormonal contraceptives and breast cancer risk: A UK nested case–control study and meta-analysis. PloS Med. (2023) 20(3):e1004188. doi: 10.1371/journal.pmed.1004188
75. Dehesh T, Fadaghi S, Seyedi M, Abolhadi E, Ilaghi M, Shams P, et al. The relation between obesity and breast cancer risk in women by considering menstruation status and geographical variations: a systematic review and meta-analysis. BMC Womens Health. (2023) 23:392. doi: 10.1186/s12905-023-02543-5
76. de Oliveira VA, Oliveira IKF, Pereira IC, Mendes LKF, Carneiro da Silva FC, Torres-Leal FL, et al. Consumption and supplementation of vitamin E in breast cancer risk, treatment, and outcomes: A systematic review with meta-analysis. Clin Nutr ESPEN. (2023) 54:215–26. doi: 10.1016/j.clnesp.2023.01.032
77. Cong X, Liu Q, Li W, Wang L, Feng Y, Liu C, et al. Systematic review and meta-analysis of breast cancer risks in relation to 2,3,7,8-tetrachlorodibenzo-p-dioxin and per- and polyfluoroalkyl substances. Environ Sci Pollut Res Int. (2023) 30(37):86540–55. doi: 10.1007/s11356-023-28592-9
78. Chen Y, Mushashi F, Son S, Bhatti P, Dummer T, Murphy RA. Diabetes medications and cancer risk associations: a systematic review and meta-analysis of evidence over the past 10 years. Sci Rep. (2023) 13:11844. doi: 10.1038/s41598-023-38431-z
79. Zhou W, Veliz PT, Smith EML, Chen W, Reddy RM, Larson JL. Comparison of pre-diagnosis physical activity and its correlates between lung and other cancer patients: accelerometer data from the UK biobank prospective cohort. Int J Environ Res Public Health. (2023) 20(2):1001. doi: 10.3390/ijerph20021001
80. Bakierzynska M, Cullinane MC, Redmond HP, Corrigan M. Prophylactic aspirin intake and breast cancer risk; A systematic review and meta-analysis of observational cohort studies. Eur J Surg Oncol. (2023) 49(10):106940. doi: 10.1016/j.ejso.2023.05.015
81. Li Z, Wang YH, Wang LL, Hu DT, Teng Y, Zhang TY, et al. Polycystic ovary syndrome and the risk of endometrial, ovarian and breast cancer: An updated meta-analysis. Scott Med J. (2022) 67:109–20. doi: 10.1177/00369330221107099
82. Zhuang Y, Pang X, Qi Y, Zhang T, Cao G, Xue H, et al. The incidence risk of breast and gynecological cancer by antidepressant use: A systematic review and dose-response meta-analysis of epidemiological studies involving 160,727 patients. Front Oncol. (2022) 12:939636. doi: 10.3389/fonc.2022.939636
83. Zheng X, Li N, Zhang Y, Zhao J. Is heart failure a new risk factor for incident cancer? Front Cardiovasc Med. (2022) 9:828290. doi: 10.3389/fcvm.2022.828290
84. Zhang J, Yang J. Allium vegetables intake and risk of breast cancer: A meta-analysis. Iran J Public Health. (2022) 51:746–57. doi: 10.18502/ijph.v51i4.9235
85. Ye J, Peng H, Huang X, Qi X. The association between endometriosis and risk of endometrial cancer and breast cancer: a meta-analysis. BMC Womens Health. (2022) 22:455. doi: 10.1186/s12905-022-02028-x
86. Yap DWT, Tan NKW, Tan BKJ, Teo YH, Tan VKM, See A, et al. The association of obstructive sleep apnea with breast cancer incidence and mortality: A systematic review and meta-analysis. J Breast Cancer. (2022) 25:149–63. doi: 10.4048/jbc.2022.25.e11
87. Xu C, Ganesan K, Liu X, Ye Q, Cheung Y, Liu D, et al. Prognostic value of negative emotions on the incidence of breast cancer: A systematic review and meta-analysis of 129,621 patients with breast cancer. Cancers (Basel). (2022) 14(3):475. doi: 10.3390/cancers14030475
88. Xiao W, Huang J, Wang J, Chen Y, Hu N, Cao S. Occupational exposure to organic solvents and breast cancer risk: a systematic review and meta-analysis. Environ Sci Pollut Res Int. (2022) 29:1605–18. doi: 10.1007/s11356-021-17100-6
89. van Weers S, Hrzic R, Elands RJJ. Oral contraceptive use and breast cancer risk according to molecular subtypes status: A meta-analysis. Eur J Public Health. (2022) 14(3):574. doi: 10.3390/cancers14030574
90. Reng Q, Zhu LL, Feng L, Li YJ, Zhu YX, Wang TT, et al. Dietary meat mutagens intake and cancer risk: A systematic review and meta-analysis. Front Nutr. (2022) 9:962688. doi: 10.3389/fnut.2022.962688
91. Weinmann S, Tanaka LF, Schauberger G, Osmani V, Klug SJ. Breast cancer among female flight attendants and the role of the occupational exposures: A systematic review and meta-analysis. J Occup Environ Med. (2022) 64:822–30. doi: 10.1097/jom.0000000000002606
92. Parra-Soto S, Ahumada D, Petermann-Rocha F, Boonpoor J, Gallegos JL, Anderson J, et al. Association of meat, vegetarian, pescatarian and fish-poultry diets with risk of 19 cancer sites and all cancer: findings from the UK Biobank prospective cohort study and meta-analysis. BMC Med. (2022) 20:79. doi: 10.1186/s12916-022-02257-9
93. Nouri M, Mohsenpour MA, Katsiki N, Ghobadi S, Jafari A, Faghih S, et al. Effect of serum lipid profile on the risk of breast cancer: systematic review and meta-analysis of 1,628,871 women. J Clin Med. (2022) 11(15):4503. doi: 10.3390/jcm11154503
94. Najdi N, Esmailzadeh A, Shokrpour M, Nikfar S, Razavi SZ, Sepidarkish M, et al. A systematic review and meta-analysis on tubal ligation and breast cancer risk. Syst Rev. (2022) 11:126. doi: 10.1186/s13643-022-02000-8
95. Manouchehri E, Taghipour A, Ghavami V, Shandiz FH, Ebadi A, Roudsari RL. Menstrual and reproductive factors and risk of breast cancer in Iranian female population: A systematic review and meta-analysis. Int J Prev Med. (2022) 13:26. doi: 10.4103/ijpvm.IJPVM_646_20
96. Markellos C, Ourailidou ME, Gavriatopoulou M, Halvatsiotis P, Sergentanis TN, Psaltopoulou T. Olive oil intake and cancer risk: A systematic review and meta-analysis. PloS One. (2022) 17:e0261649. doi: 10.1371/journal.pone.0261649
97. Long T, Liu K, Long J, Li J, Cheng L. Dietary glycemic index, glycemic load and cancer risk: a meta-analysis of prospective cohort studies. Eur J Nutr. (2022) 61:2115–27. doi: 10.1007/s00394-022-02797-z
98. Wu S, Yuan C, Liu S, Zhang Q, Yang Z, Sun F, et al. Irritable bowel syndrome and long-term risk of cancer: A prospective cohort study among 0.5 million adults in UK biobank. Am J Gastroenterol. (2022) 117:785–93. doi: 10.14309/ajg.0000000000001674
99. Liu F, Peng Y, Qiao Y, Huang Y, Song F, Zhang M, et al. Consumption of flavonoids and risk of hormone-related cancers: a systematic review and meta-analysis of observational studies. Nutr J. (2022) 21:27. doi: 10.1186/s12937-022-00778-w
100. Li N, Guo X, Sun C, Lowe S, Su W, Song Q, et al. Dietary carbohydrate intake is associated with a lower risk of breast cancer: A meta-analysis of cohort studies. Nutr Res. (2022) 100:70–92. doi: 10.1016/j.nutres.2022.01.004
101. Khoramdad M, Solaymani-Dodaran M, Kabir A, Ghahremanzadeh N, Hashemi E-o, Fahimfar N, et al. Breast cancer risk factors in Iranian women: a systematic review and meta-analysis of matched case-control studies. Eur J Med Res. (2022) 27(1):311. doi: 10.1186/s40001-022-00952-0
102. Kacimi SEO, Elgenidy A, Cheema HA, Ould Setti M, Khosla AA, Benmelouka AY, et al. Prior tonsillectomy and the risk of breast cancer in females: A systematic review and meta-analysis. Front Oncol. (2022) 12:925596. doi: 10.3389/fonc.2022.925596
103. Islam MA, Sathi NJ, Abdullah HM, Tabassum T. A meta-analysis of induced abortion, alcohol consumption, and smoking triggering breast cancer risk among women from developed and least developed countries. Int J Clin Pract. (2022) 2022:6700688. doi: 10.1155/2022/6700688
104. Han X, Zhao R, Wang Y, Ma H, Yu M, Chen X, et al. Dietary vitamin A intake and circulating vitamin A concentrations and the risk of three common cancers in women: A meta-analysis. Oxid Med Cell Longev. (2022) 2022:7686405. doi: 10.1155/2022/7686405
105. Han M, Wang Y, Jin Y, Zhao X, Cui H, Wang G, et al. Benign thyroid disease and the risk of breast cancer: An updated systematic review and meta-analysis. Front Endocrinol (Lausanne). (2022) 13:984593. doi: 10.3389/fendo.2022.984593
106. Gao Z, Xi Y, Shi H, Ni J, Xu W, Zhang K. Antipsychotic exposure is an independent risk factor for breast cancer: A systematic review of epidemiological evidence. Ca-a Cancer J Clinicians. (2022) 12:993367. doi: 10.3389/fonc.2022.993367
107. Bodewes FTH, van Asselt AA, Dorrius MD, Greuter MJW, de Bock GH. Mammographic breast density and the risk of breast cancer: A systematic review and meta-analysis. Breast. (2022) 66:62–8. doi: 10.1016/j.breast.2022.09.007
108. Gamboa-Loira B, Lopez-Carrillo L, Mar-Sanchez Y, Stern D, Cebrian ME. Epidemiologic evidence of exposure to polycyclic aromatic hydrocarbons and breast cancer: A systematic review and meta-analysis. Chemosphere. (2022) 290:133237. doi: 10.1016/j.chemosphere.2021.133237
109. Byun D, Hong S, Ryu S, Nam Y, Jang H, Cho Y, et al. Early-life body mass index and risks of breast, endometrial, and ovarian cancers: a dose-response meta-analysis of prospective studies. Br J Cancer. (2022) 126:664–72. doi: 10.1038/s41416-021-01625-1
110. Amerizadeh A, Vaseghi G, Farajzadegan Z, Asgary S. An updated systematic review and meta-analysis on association of serum lipid profile with risk of breast cancer incidence. Int J Prev Med. (2022) 13:142. doi: 10.4103/ijpvm.IJPVM_285_20
111. Zhang H, Guo L, Tao W, Zhang J, Zhu Y, Abdelrahim MEA, et al. Possible breast cancer risk related to background parenchymal enhancement at breast MRI: A meta-analysis study. Nutr Cancer. (2021) 73:1371–7. doi: 10.1080/01635581.2020.1795211
112. Wong ATY, Heath AK, Tong TYN, Reeves GK, Floud S, Beral V, et al. Sleep duration and breast cancer incidence: results from the Million Women Study and meta-analysis of published prospective studies. Sleep. (2021) 44(2):zsaa166. doi: 10.1093/sleep/zsaa166
113. Wei W, Wu BJ, Wu Y, Tong ZT, Zhong F, Hu CY. Association between long-term ambient air pollution exposure and the risk of breast cancer: a systematic review and meta-analysis. Environ Sci Pollut Res Int. (2021) 28:63278–96. doi: 10.1007/s11356-021-14903-5
114. Wei L, Han N, Sun S, Ma X, Zhang Y. Sleep-disordered breathing and risk of the breast cancer: A meta-analysis of cohort studies. Int J Clin Pract. (2021) 75:e14793. doi: 10.1111/ijcp.14793
115. Urbano T, Vinceti M, Wise LA, Filippini T. Light at night and risk of breast cancer: a systematic review and dose-response meta-analysis. Int J Health Geogr. (2021) 20:44. doi: 10.1186/s12942-021-00297-7
116. Wong ATY, Fensom GK, Key TJ, Charlotte Onland-Moret N, Tong TYN, Travis RC. Urinary melatonin in relation to breast cancer risk: Nested case–Control analysis in the DOM study and meta-analysis of prospective studies. Cancer Epidemiol Biomarkers Prevention. (2021) 30:97–103. doi: 10.1158/1055-9965.EPI-20-0822
117. Van NTH, Hoang T, Myung SK. Night shift work and breast cancer risk: a meta-analysis of observational epidemiological studies. Carcinogenesis. (2021) 42:1260–9. doi: 10.1093/carcin/bgab074
118. Nourmohammadi H, Shoushtari SY, Joybari L, Ghasemi M, Ghaysouri A, Keshavarz K. Human papillomavirus infection and risk of breast cancer in Iran: A meta-analysis. Iranian Red Crescent Med J. (2021) 23(7):e646. doi: 10.32592/ircmj.2021.23.7.646
119. Michels N, Specht IO, Heitmann BL, Chajès V, Huybrechts I. Dietary trans-fatty acid intake in relation to cancer risk: a systematic review and meta-analysis. Nutr Rev. (2021) 79:758–76. doi: 10.1093/nutrit/nuaa061
120. Naghshi S, Sadeghian M, Nasiri M, Mobarak S, Asadi M, Sadeghi O. Association of total nut, tree nut, peanut, and peanut butter consumption with cancer incidence and mortality: A comprehensive systematic review and dose-response meta-analysis of observational studies. Adv Nutrition. (2021) 12:793–808. doi: 10.1093/advances/nmaa152
121. Li C, Fan Z, Lin X, Cao M, Song F, Song F. Parity and risk of developing breast cancer according to tumor subtype: A systematic review and meta-analysis. Cancer Epidemiol. (2021) 75:102050. doi: 10.1016/j.canep.2021.102050
122. Lee J, Lee J, Lee DW, Kim HR, Kang MY. Sedentary work and breast cancer risk: A systematic review and meta-analysis. J Occup Health. (2021) 63:e12239. doi: 10.1002/1348-9585.12239
123. Keefe D. Fertility treatment and cancers-the eternal conundrum: A systematic review and meta-analysis. Obstetrical Gynecological Survey. (2021) 76:343–4. doi: 10.1097/01.ogx.0000754400.96078.d7
124. Kazemi A, Barati-Boldaji R, Soltani S, Mohammadipoor N, Esmaeilinezhad Z, Clark CCT, et al. Intake of various food groups and risk of breast cancer: A systematic review and dose-response meta-analysis of prospective studies. Adv Nutr. (2021) 12:809–49. doi: 10.1093/advances/nmaa147
125. Hao Y, Jiang M, Miao Y, Li X, Hou C, Zhang X, et al. Effect of long-term weight gain on the risk of breast cancer across women's whole adulthood as well as hormone-changed menopause stages: A systematic review and dose–response meta-analysis. Obesity Res Clin Practice. (2021) 15:439–48. doi: 10.1016/j.orcp.2021.08.004
126. Farvid MS, Sidahmed E, Spence ND, Mante Angua K, Rosner BA, Barnett JB. Consumption of red meat and processed meat and cancer incidence: a systematic review and meta-analysis of prospective studies. Eur J Epidemiol. (2021) 36:937–51. doi: 10.1007/s10654-021-00741-9
127. Chong F, Wang Y, Song M, Sun Q, Xie W, Song C. Sedentary behavior and risk of breast cancer: a dose–response meta-analysis from prospective studies. Breast Cancer. (2021) 28:48–59. doi: 10.1007/s12282-020-01126-8
128. Chen H, Gao Y, Wei N, Du K, Jia Q. Strong association between the dietary inflammatory index(DII) and breast cancer: a systematic review and meta-analysis. Aging (Albany NY). (2021) 13:13039–47. doi: 10.18632/aging.202985
129. Barańska A, Błaszczuk A, Kanadys W, Malm M, Drop K, Polz-Dacewicz M. Oral contraceptive use and breast cancer risk assessment: A systematic review and meta-analysis of case-control studies, 2009–2020. Cancers. (2021) 13(22):5654. doi: 10.3390/cancers13225654
130. Ba DM, Ssentongo P, Beelman RB, Muscat J, Gao X, Richie JP. Higher mushroom consumption is associated with lower risk of cancer: A systematic review and meta-analysis of observational studies. Adv Nutr. (2021) 12:1691–704. doi: 10.1093/advances/nmab015
131. Arafat HM, Omar J, Muhamad R, Al-Astani TAD, Shafii N, Al Laham NA, et al. Breast cancer risk from modifiable and non-modifiable risk factors among palestinian women: A systematic review and meta-analysis. Asian Pac J Cancer Prev. (2021) 22:1987–95. doi: 10.31557/apjcp.2021.22.7.1987
132. Zhou W, Chen X, Huang H, Liu S, Xie A, Lan L. Birth weight and incidence of breast cancer: dose-response meta-analysis of prospective studies. Clin Breast Cancer. (2020) 20:e555–68. doi: 10.1016/j.clbc.2020.04.011
133. Zhang D, Xu P, Li Y, Wei B, Yang S, Zheng Y, et al. Association of vitamin C intake with breast cancer risk and mortality: a meta-analysis of observational studies. Aging (Albany NY). (2020) 12:18415–35. doi: 10.18632/aging.103769
134. Zeng J, Gu Y, Fu H, Liu C, Zou Y, Chang H. Association between one-carbon metabolism-related vitamins and risk of breast cancer: A systematic review and meta-analysis of prospective studies. Clin Breast Cancer. (2020) 20:e469–80. doi: 10.1016/j.clbc.2020.02.012
135. Wu Y, Wang M, Sun W, Li S, Wang W, Zhang D. Age at last birth and risk of developing breast cancer: a meta-analysis. Eur J Cancer Prev. (2020) 29:424–32. doi: 10.1097/cej.0000000000000560
136. Wei Y, Lv J, Guo Y, Bian Z, Gao M, Du H, et al. Soy intake and breast cancer risk: a prospective study of 300,000 Chinese women and a dose-response meta-analysis. Eur J Epidemiol. (2020) 35:567–78. doi: 10.1007/s10654-019-00585-4
137. Wang Y, Zhao Y, Chong F, Song M, Sun Q, Li T, et al. A dose-response meta-analysis of green tea consumption and breast cancer risk. Int J Food Sci Nutr. (2020) 71:656–67. doi: 10.1080/09637486.2020.1715353
138. Wang Y, Yan P, Fu T, Yuan J, Yang G, Liu Y, et al. The association between gestational diabetes mellitus and cancer in women: A systematic review and meta-analysis of observational studies. Diabetes Metab. (2020) 46:461–71. doi: 10.1016/j.diabet.2020.02.003
139. Wang Q, Liu X, Ren S. Tofu intake is inversely associated with risk of breast cancer: A meta-analysis of observational studies. PloS One. (2020) 15:e0226745. doi: 10.1371/journal.pone.0226745
140. Song HJ, Jeon N, Squires P. The association between acid-suppressive agent use and the risk of cancer: a systematic review and meta-analysis. Eur J Clin Pharmacol. (2020) 76:1437–56. doi: 10.1007/s00228-020-02927-8
141. Simin J, Tamimi RM, Engstrand L, Callens S, Brusselaers N. Antibiotic use and the risk of breast cancer: A systematic review and dose-response meta-analysis. Pharmacol Res. (2020) 160:105072. doi: 10.1016/j.phrs.2020.105072
142. Ren X, Xu P, Zhang D, Liu K, Song D, Zheng Y, et al. Association of folate intake and plasma folate level with the risk of breast cancer: a dose-response meta-analysis of observational studies. Aging (Albany NY). (2020) 12:21355–75. doi: 10.18632/aging.103881
143. Ramalho NM, Carneiro VCG. Education level and breast cancer incidence: a meta-analysis of cohort studies. Menopause-the J North Am Menopause Society. (2020) 27:119–9. doi: 10.1097/GME.0000000000001464
144. Peng R, Liang X, Zhang G, Yao Y, Chen Z, Pan X, et al. Association use of bisphosphonates with risk of breast cancer: A meta-analysis. BioMed Res Int. (2020) 2020:5606573. doi: 10.1155/2020/5606573
145. Okekunle AP, Gao J, Wu X, Feng R, Sun C. Higher dietary soy intake appears inversely related to breast cancer risk independent of estrogen receptor breast cancer phenotypes. Heliyon. (2020) 6:e04228. doi: 10.1016/j.heliyon.2020.e04228
146. Li M, Han M, Chen Z, Tang Y, Ma J, Zhang Z, et al. Does marital status correlate with the female breast cancer risk? A systematic review and meta-analysis of observational studies. PloS One. (2020) 15:e0229899. doi: 10.1371/journal.pone.0229899
147. Kim Y, Kim J. N-6 polyunsaturated fatty acids and risk of cancer: accumulating evidence from prospective studies. Nutrients. (2020) 12(9):2523. doi: 10.3390/nu12092523
148. Khatami A, Pormohammad A, Farzi R, Saadati H, Mehrabi M, Kiani SJ, et al. Bovine Leukemia virus (BLV) and risk of breast cancer: a systematic review and meta-analysis of case-control studies. Infect Agent Cancer. (2020) 15:48. doi: 10.1186/s13027-020-00314-7
149. Jin Q, Su J, Yan D, Wu S. Epstein-barr virus infection and increased sporadic breast carcinoma risk: A meta-analysis. Med Princ Pract. (2020) 29:195–200. doi: 10.1159/000502131
150. Hiller TWR, O'Sullivan DE, Brenner DR, Peters CE, King WD. Solar ultraviolet radiation and breast cancer risk: A systematic review and meta-analysis. Environ Health Perspect. (2020) 128:16002. doi: 10.1289/ehp4861
151. Hidayat K, Zhou HJ, Shi BM. Influence of physical activity at a young age and lifetime physical activity on the risks of 3 obesity-related cancers: systematic review and meta-analysis of observational studies. Nutr Rev. (2020) 78:1–18. doi: 10.1093/nutrit/nuz024
152. Adani G, Filippini T, Wise LA, Halldorsson TI, Blaha L, Vinceti M. Dietary intake of acrylamide and risk of breast, endometrial, and ovarian cancers: A systematic review and dose-response meta-analysis. Cancer Epidemiol Biomarkers Prev. (2020) 29:1095–106. doi: 10.1158/1055-9965.Epi-19-1628
153. Zhao TT, Jin F, Li JG, Xu YY, Dong HT, Liu Q, et al. Dietary isoflavones or isoflavone-rich food intake and breast cancer risk: A meta-analysis of prospective cohort studies. Clin Nutr. (2019) 38:136–45. doi: 10.1016/j.clnu.2017.12.006
154. Zhang L, Huang S, Cao L, Ge M, Li Y, Shao J. Vegetable-fruit-soybean dietary pattern and breast cancer: A meta-analysis of observational studies. J Nutr Sci Vitaminol (Tokyo). (2019) 65:375–82. doi: 10.3177/jnsv.65.375
155. Yu S, Zhu L, Wang K, Yan Y, He J, Ren Y. Green tea consumption and risk of breast cancer: A systematic review and updated meta-analysis of case-control studies. Med (Baltimore). (2019) 98:e16147. doi: 10.1097/md.0000000000016147
156. Xiping Z, Shuai Z, Feijiang Y, Bo C, Shifeng Y, Qihui C. Meta-analysis of the correlation between schizophrenia and breast cancer. Clin Breast Cancer. (2019) 19:e172–85. doi: 10.1016/j.clbc.2018.10.012
157. Vishwakarma G, Ndetan H, Das DN, Gupta G, Suryavanshi M, Mehta A, et al. Reproductive factors and breast cancer risk: A meta-analysis of case-control studies in Indian women. South Asian J Cancer. (2019) 8:80–4. doi: 10.4103/sajc.sajc_317_18
158. Yoon YS, Kwon AR, Lee YK, Oh SW. Circulating adipokines and risk of obesity related cancers: A systematic review and meta-analysis. Obes Res Clin Pract. (2019) 13:329–39. doi: 10.1016/j.orcp.2019.03.006
159. Song D, Deng Y, Liu K, Zhou L, Li N, Zheng Y, et al. Vitamin D intake, blood vitamin D levels, and the risk of breast cancer: a dose-response meta-analysis of observational studies. Aging (Albany NY). (2019) 11:12708–32. doi: 10.18632/aging.102597
160. Seretis A, Cividini S, Markozannes G, Tseretopoulou X, Lopez DS, Ntzani EE, et al. Association between blood pressure and risk of cancer development: a systematic review and meta-analysis of observational studies. Sci Rep. (2019) 9:8565. doi: 10.1038/s41598-019-45014-4
161. Ren C, Zeng K, Wu C, Mu L, Huang J, Wang M. Human papillomavirus infection increases the risk of breast carcinoma: a large-scale systemic review and meta-analysis of case-control studies. Gland Surg. (2019) 8:486–500. doi: 10.21037/gs.2019.09.04
162. Nindrea RD, Aryandono T, Lazuardi L, Dwiprahasto I. Association of dietary intake ratio of n-3/n-6 polyunsaturated fatty acids with breast cancer risk in western and asian countries: A meta-analysis. Asian Pac J Cancer Prev. (2019) 20:1321–7. doi: 10.31557/apjcp.2019.20.5.1321
163. Nindrea RD, Aryandono T, Lazuardi L, Dwiprahasto I. Association of overweight and obesity with breast cancer during premenopausal period in asia: A meta-analysis. Int J Prev Med. (2019) 10:192. doi: 10.4103/ijpvm.IJPVM_372_18
164. Nindrea RD, Anwar SL, Harahap WA, Lazuardi L, Dwiprahasto I, Aryandono T. Oral contraceptive used more than 5 years is associated with increased risk of breast cancer: A meta-analysis of 28,776 South east Asian women. Systematic Rev Pharmacy. (2019) 10:137–48. doi: 10.5530/srp.2019.2.22
165. Mao J, Shi J, He S, Li N, Shi J, Yang F. Reproductive factors and risk of breast cancer: a pooled meta-analysis of 55 case-control studies based on different source populations. Int J Clin Exp Med. (2019) 12:6453–68.
166. Namazi N, Irandoost P, Heshmati J, Larijani B, Azadbakht L. The association between fat mass and the risk of breast cancer: A systematic review and meta-analysis. Clin Nutr. (2019) 38:1496–503. doi: 10.1016/j.clnu.2018.09.013
167. Ji LW, Jing CX, Zhuang SL, Pan WC, Hu XP. Effect of age at first use of oral contraceptives on breast cancer risk: An updated meta-analysis. Med (Baltimore). (2019) 98:e15719. doi: 10.1097/md.0000000000015719
168. Hossain S, Beydoun MA, Beydoun HA, Chen X, Zonderman AB, Wood RJ. Vitamin D and breast cancer: A systematic review and meta-analysis of observational studies. Clin Nutr ESPEN. (2019) 30:170–84. doi: 10.1016/j.clnesp.2018.12.085
169. Guo M, Liu T, Li P, Wang T, Zeng C, Yang M, et al. Association between metabolic syndrome and breast cancer risk: an updated meta-analysis of follow-up studies. Front Oncol. (2019) 9:1290. doi: 10.3389/fonc.2019.01290
170. Chen X, Wang Q, Zhang Y, Xie Q, Tan X. Physical activity and risk of breast cancer: A meta-analysis of 38 cohort studies in 45 study reports. Value Health. (2019) 22:104–28. doi: 10.1016/j.jval.2018.06.020
171. Chen JH, Yuan Q, Ma YN, Zhen SH, Wen DL. Relationship between bone mineral density and the risk of breast cancer: A systematic review and dose–response meta-analysis of ten cohort studies. Cancer Manage Res. (2019) 11:1453–64. doi: 10.2147/CMAR.S188251
172. Chang VC, Cotterchio M, Khoo E. Iron intake, body iron status, and risk of breast cancer: a systematic review and meta-analysis. BMC Cancer. (2019) 19:543. doi: 10.1186/s12885-019-5642-0
173. Chan DSM, Abar L, Cariolou M, Nanu N, Greenwood DC, Bandera EV, et al. World Cancer Research Fund International: Continuous Update Project—systematic literature review and meta-analysis of observational cohort studies on physical activity, sedentary behavior, adiposity, and weight change and breast cancer risk. Cancer Causes Control. (2019) 30:1183–200. doi: 10.1007/s10552-019-01223-w
174. Thakur AA, Wang X, Garcia-Betancourt MM, Forse RA. Calcium channel blockers and the incidence of breast and prostate cancer: A meta-analysis. J Clin Pharm Ther. (2018) 43:519–29. doi: 10.1111/jcpt.12673
175. Tang GH, Satkunam M, Pond GR, Steinberg GR, Blandino G, Schünemann HJ, et al. Association of metformin with breast cancer incidence and mortality in patients with type II diabetes: A GRADE-assessed systematic review and meta-analysis. Cancer Epidemiol Biomarkers Prev. (2018) 27:627–35. doi: 10.1158/1055-9965.Epi-17-0936
176. Sun M, Fan Y, Hou Y, Fan Y. Preeclampsia and maternal risk of breast cancer: a meta-analysis of cohort studies. J Matern Fetal Neonatal Med. (2018) 31:2484–91. doi: 10.1080/14767058.2017.1342806
177. Shao J, Wu L, Leng WD, Fang C, Zhu YJ, Jin YH, et al. Periodontal disease and breast cancer: A meta-analysis of 1,73,162 participants. Front Oncol. (2018) 8:601. doi: 10.3389/fonc.2018.00601
178. Rezaianzadeh A, Ghorbani M, Rezaeian S, Kassani A. Red meat consumption and breast cancer risk in premenopausal women: A systematic review and meta-analysis. Middle East J Cancer. (2018) 9:5–12.
179. Qiao Y, Yang T, Gan Y, Li W, Wang C, Gong Y, et al. Associations between aspirin use and the risk of cancers: a meta-analysis of observational studies. BMC Cancer. (2018) 18:288. doi: 10.1186/s12885-018-4156-5
180. Liu K, Zhang W, Dai Z, Wang M, Tian T, Liu X, et al. Association between body mass index and breast cancer risk: Evidence based on a dose–response meta-analysis. Cancer Manage Res. (2018) 10:143–51. doi: 10.2147/CMAR.S144619
181. Li D, Hao X, Li J, Wu Z, Chen S, Lin J, et al. Dose-response relation between dietary inflammatory index and human cancer risk: evidence from 44 epidemiologic studies involving 1,082,092 participants. Am J Clin Nutrition. (2018) 107:371–88. doi: 10.1093/ajcn/nqx064
182. Lafranconi A, Micek A, Paoli PD, Bimonte S, Rossi P, Quagliariello V, et al. Coffee intake decreases risk of postmenopausal breast cancer: A dose-response meta-analysis on prospective cohort studies. Nutrients. (2018) 10(2):112. doi: 10.3390/nu10020112
183. Kim AS, Ko HJ, Kwon JH, Lee JM. Exposure to secondhand smoke and risk of cancer in never smokers: A meta-analysis of epidemiologic studies. Int J Environ Res Public Health. (2018) 15(9):1981. doi: 10.3390/ijerph15091981
184. Hidayat K, Yang CM, Shi BM. Body fatness at a young age, body fatness gain and risk of breast cancer: systematic review and meta-analysis of cohort studies. Obes Rev. (2018) 19:254–68. doi: 10.1111/obr.12627
185. Du R, Lin L, Cheng D, Xu Y, Xu M, Chen Y, et al. Thiazolidinedione therapy and breast cancer risk in diabetic women: A systematic review and meta-analysis. Diabetes Metab Res Rev. (2018) 34(2). doi: 10.1002/dmrr.2961
186. Deng Y, Xu H, Zeng X. Induced abortion and breast cancer: An updated meta-analysis. Med (Baltimore). (2018) 97:e9613. doi: 10.1097/md.0000000000009613
187. Cordina-Duverger E, Menegaux F, Popa A, Rabstein S, Harth V, Pesch B, et al. Night shift work and breast cancer: a pooled analysis of population-based case-control studies with complete work history. Eur J Epidemiol. (2018) 33:369–79. doi: 10.1007/s10654-018-0368-x
188. Chen H, Shao F, Zhang F, Miao Q. Association between dietary carrot intake and breast cancer: A meta-analysis. Med (Baltimore). (2018) 97:e12164. doi: 10.1097/md.0000000000012164
189. Xu J, Huang L, Sun GP. Urinary 6-sulfatoxymelatonin level and breast cancer risk: systematic review and meta-analysis. Sci Rep. (2017) 7:5353. doi: 10.1038/s41598-017-05752-9
190. Schlesinger S, Chan DSM, Vingeliene S, Vieira AR, Abar L, Polemiti E, et al. Carbohydrates, glycemic index, glycemic load, and breast cancer risk: a systematic review and dose-response meta-analysis of prospective studies. Nutr Rev. (2017) 75:420–41. doi: 10.1093/nutrit/nux010
191. Nindrea RD, Aryandono T, Lazuardi L. Breast cancer risk from modifiable and non-modifiable risk factors among women in southeast asia: A meta-analysis. Asian Pac J Cancer Prev. (2017) 18:3201–6. doi: 10.22034/apjcp.2017.18.12.3201
192. Ni H, Rui Q, Zhu X, Yu Z, Gao R, Liu H. Antihypertensive drug use and breast cancer risk: a meta-analysis of observational studies. Oncotarget. (2017) 8:62545–60. doi: 10.18632/oncotarget.19117
193. Nagel G, Peter RS, Klotz E, Brozek W, Concin H. Bone mineral density and breast cancer risk: Results from the Vorarlberg Health Monitoring & Prevention Program and meta-analysis. Bone Rep. (2017) 7:83–9. doi: 10.1016/j.bonr.2017.09.004
194. Kolahdouz Mohammadi R, Bagheri M, Kolahdouz Mohammadi M, Shidfar F. Ruminant trans-fatty acids and risk of breast cancer: a systematic review and meta-analysis of observational studies. Minerva Endocrinol. (2017) 42:385–96. doi: 10.23736/s0391-1977.16.02514-1
195. Karasneh RA, Murray LJ, Cardwell CR. Cardiac glycosides and breast cancer risk: A systematic review and meta-analysis of observational studies. Int J Cancer. (2017) 140:1035–41. doi: 10.1002/ijc.30520
196. Jia Y, Li F, Liu YF, Zhao JP, Leng MM, Chen L. Depression and cancer risk: a systematic review and meta-analysis. Public Health. (2017) 149:138–48. doi: 10.1016/j.puhe.2017.04.026
197. Chen Y, Liu L, Zhou Q, Imam MU, Cai J, Wang Y, et al. Body mass index had different effects on premenopausal and postmenopausal breast cancer risks: a dose-response meta-analysis with 3,318,796 subjects from 31 cohort studies. BMC Public Health. (2017) 17:936. doi: 10.1186/s12889-017-4953-9
198. Neil-Sztramko SE, Boyle T, Milosevic E, Nugent SF, Gotay CC, Campbell KL. Does obesity modify the relationship between physical activity and breast cancer risk? Breast Cancer Res Treat. (2017) 166:367–81. doi: 10.1007/s10549-017-4449-4
199. Zhou Y, Wang T, Zhai S, Li W, Meng Q. Linoleic acid and breast cancer risk: a meta-analysis. Public Health Nutr. (2016) 19:1457–63. doi: 10.1017/s136898001500289x
200. Wang M, Wu X, Chai F, Zhang Y, Jiang J. Plasma prolactin and breast cancer risk: a meta- analysis. Sci Rep. (2016) 6:25998. doi: 10.1038/srep25998
201. Sun S, Li X, Ren A, Du M, Du H, Shu Y, et al. Choline and betaine consumption lowers cancer risk: a meta-analysis of epidemiologic studies. Sci Rep. (2016) 6:35547. doi: 10.1038/srep35547
202. Neilson HK, Farris MS, Stone CR, Vaska MM, Brenner DR, Friedenreich CM. Moderate-vigorous recreational physical activity and breast cancer risk, stratified by menopause status: A systematic review and meta-analysis. Menopause. (2016) 24:322–44. doi: 10.1097/GME.0000000000000745
203. Mullie P, Koechlin A, Boniol M, Autier P, Boyle P. Relation between breast cancer and high glycemic index or glycemic load: A meta-analysis of prospective cohort studies. Crit Rev Food Sci Nutr. (2016) 56:152–9. doi: 10.1080/10408398.2012.718723
204. Li C, Yang L, Zhang D, Jiang W. Systematic review and meta-analysis suggest that dietary cholesterol intake increases risk of breast cancer. Nutr Res. (2016) 36:627–35. doi: 10.1016/j.nutres.2016.04.009
205. Lei L, Yang Y, He H, Chen E, Du L, Dong J, et al. Flavan-3-ols consumption and cancer risk: A meta-analysis of epidemiologic studies. Oncotarget. (2016) 7:73573–92. doi: 10.18632/oncotarget.12017
206. Gong W-J, Zheng W, Xiao L, Tan L-M, Song J, Li X-P, et al. Circulating resistin levels and obesity-related cancer risk: a meta-analysis. Oncotarget. (2016) 7:57694–704. doi: 10.18632/oncotarget.11034
207. Fabiani R, Minelli L, Rosignoli P. Apple intake and cancer risk: a systematic review and meta-analysis of observational studies. Public Health Nutr. (2016) 19:2603–17. doi: 10.1017/s136898001600032x
208. Chen J-Y, Zhu H-C, Guo Q, Shu Z, Bao X-H, Sun F, et al. Dose-dependent associations between wine drinking and breast cancer risk - meta-analysis findings. Asian Pacific J Cancer prevention: APJCP. (2016) 17:1221–33. doi: 10.7314/APJCP.2016.17.3.1221
209. Cao Y, Hou L, Wang W. Dietary total fat and fatty acids intake, serum fatty acids and risk of breast cancer: A meta-analysis of prospective cohort studies. Int J Cancer. (2016) 138:1894–904. doi: 10.1002/ijc.29938
210. Cai X, Wang C, Yu W, Fan W, Wang S, Shen N, et al. Selenium exposure and cancer risk: an updated meta-analysis and meta-regression. Sci Rep. (2016) 6:19213. doi: 10.1038/srep19213
211. Bae JM, Kim EH. Human papillomavirus infection and risk of breast cancer: a meta-analysis of case-control studies. Infect Agent Cancer. (2016) 11:14. doi: 10.1186/s13027-016-0058-9
212. Zhang J, Huang Y, Wang X, Lin K, Wu K. Environmental polychlorinated biphenyl exposure and breast cancer risk: A meta-analysis of observational studies. PloS One. (2015) 10:e0142513. doi: 10.1371/journal.pone.0142513
213. Xin Y, Li XY, Sun SR, Wang LX, Huang T. Vegetable oil intake and breast cancer risk: a meta-analysis. Asian Pac J Cancer Prev. (2015) 16:5125–35. doi: 10.7314/apjcp.2015.16.12.5125
214. Wu YC, Zheng D, Sun JJ, Zou ZK, Ma ZL. Meta-analysis of studies on breast cancer risk and diet in Chinese women. Int J Clin Exp Med. (2015) 8:73–85.
215. Wang T. The link between Parkinson's disease and breast and prostate cancers: A meta-analysis. Int J Neurosci. (2015) 125:895–903. doi: 10.3109/00207454.2014.986265
216. Touvier M, Fassier P, His M, Norat T, Chan DS, Blacher J, et al. Cholesterol and breast cancer risk: a systematic review and meta-analysis of prospective studies. Br J Nutr. (2015) 114:347–57. doi: 10.1017/s000711451500183x
217. Shi Y, Li T, Wang Y, Zhou L, Qin Q, Yin J, et al. Household physical activity and cancer risk: a systematic review and dose-response meta-analysis of epidemiological studies. Sci Rep. (2015) 5:14901. doi: 10.1038/srep14901
218. Larsson SC, Orsini N, Wolk A. Urinary cadmium concentration and risk of breast cancer: a systematic review and dose-response meta-analysis. Am J Epidemiol. (2015) 182:375–80. doi: 10.1093/aje/kwv085
219. Hu F, Wu Z, Li G, Teng C, Liu Y, Wang F, et al. The plasma level of retinol, vitamins A, C and α-tocopherol could reduce breast cancer risk? A meta-analysis and meta-regression. J Cancer Res Clin Oncol. (2015) 141:601–14. doi: 10.1007/s00432-014-1852-7
220. He XY, Liao YD, Yu S, Zhang Y, Wang R. Sex hormone binding globulin and risk of breast cancer in postmenopausal women: a meta-analysis of prospective studies. Horm Metab Res. (2015) 47:485–90. doi: 10.1055/s-0034-1395606
221. Guo J, Huang Y, Yang L, Xie Z, Song S, Yin J, et al. Association between abortion and breast cancer: an updated systematic review and meta-analysis based on prospective studies. Cancer Causes Control. (2015) 26:811–9. doi: 10.1007/s10552-015-0536-1
222. de Pedro M, Baeza S, Escudero MT, Dierssen-Sotos T, Gómez-Acebo I, Pollán M, et al. Effect of COX-2 inhibitors and other non-steroidal inflammatory drugs on breast cancer risk: a meta-analysis. Breast Cancer Res Treat. (2015) 149:525–36. doi: 10.1007/s10549-015-3267-9
223. Chen Z, Shao J, Gao X, Li X. Effect of passive smoking on female breast cancer in China: a meta-analysis. Asia Pac J Public Health. (2015) 27:Np58–64. doi: 10.1177/1010539513481493
224. Bae JM, Kim EH. Hormone replacement therapy and risk of breast cancer in korean women: A quantitative systematic review. J Prev Med Public Health. (2015) 48:225–30. doi: 10.3961/jpmph.15.046
225. Zhao G, Lin X, Zhou M, Zhao J. Relationship between exposure to extremely low-frequency electromagnetic fields and breast cancer risk: a meta-analysis. Eur J Gynaecol Oncol. (2014) 35:264–9.
226. Yu F, Jin Z, Jiang H, Xiang C, Tang J, Li T, et al. Tea consumption and the risk of five major cancers: a dose-response meta-analysis of prospective studies. BMC Cancer. (2014) 14:197. doi: 10.1186/1471-2407-14-197
227. Yang W-S, Deng Q, Fan W-Y, Wang W-Y, Wang X. Light exposure at night, sleep duration, melatonin, and breast cancer: a dose-response analysis of observational studies. Eur J Cancer Prevention. (2014) 23:269–76. doi: 10.1097/cej.0000000000000030
228. Sergentanis TN, Diamantaras AA, Perlepe C, Kanavidis P, Skalkidou A, Petridou ET. IVF and breast cancer: a systematic review and meta-analysis. Hum Reprod Update. (2014) 20:106–23. doi: 10.1093/humupd/dmt034
229. Park JH, Cha ES, Ko Y, Hwang MS, Hong JH, Lee WJ. Exposure to dichlorodiphenyltrichloroethane and the risk of breast cancer: A systematic review and meta-analysis. Osong Public Health Res Perspect. (2014) 5:77–84. doi: 10.1016/j.phrp.2014.02.001
230. Nie XC, Dong DS, Bai Y, Xia P. Meta-analysis of black tea consumption and breast cancer risk: update 2013. Nutr Cancer. (2014) 66:1009–14. doi: 10.1080/01635581.2014.936947
231. Lv M, Zhu X, Zhong S, Chen W, Hu Q, Ma T, et al. Radial scars and subsequent breast cancer risk: a meta-analysis. PloS One. (2014) 9:e102503. doi: 10.1371/journal.pone.0102503
232. Liu XO, Huang YB, Gao Y, Chen C, Yan Y, Dai HJ, et al. Association between dietary factors and breast cancer risk among Chinese females: systematic review and meta-analysis. Asian Pac J Cancer Prev. (2014) 15:1291–8. doi: 10.7314/apjcp.2014.15.3.1291
233. Chen C, Huang YB, Liu XO, Gao Y, Dai HJ, Song FJ, et al. Active and passive smoking with breast cancer risk for Chinese females: a systematic review and meta-analysis. Chin J Cancer. (2014) 33:306–16. doi: 10.5732/cjc.013.10248
234. Zheng JS, Hu XJ, Zhao YM, Yang J, Li D. Intake of fish and marine n-3 polyunsaturated fatty acids and risk of breast cancer: meta-analysis of data from 21 independent prospective cohort studies. Bmj. (2013) 346:f3706. doi: 10.1136/bmj.f3706
235. Yang Y, Zhang F, Skrip L, Wang Y, Liu S. Lack of an association between passive smoking and incidence of female breast cancer in non-smokers: evidence from 10 prospective cohort studies. PloS One. (2013) 8:e77029. doi: 10.1371/journal.pone.0077029
236. Warren GW, Gritz ER. Active smoking and breast cancer risk: Original cohort data and meta-analysis: Gaudet MM, Gapstur SM, Sun J, et al. (American Cancer Society, Atlanta, GA) J Natl Cancer Inst 105:515-525, 2013. Breast Diseases. (2013) 24:319–20. doi: 10.1016/j.breastdis.2013.10.029
237. Wu W, Kang S, Zhang D. Association of vitamin B6, vitamin B12 and methionine with risk of breast cancer: a dose-response meta-analysis. Br J Cancer. (2013) 109:1926–44. doi: 10.1038/bjc.2013.438
238. Song JK, Bae JM. Citrus fruit intake and breast cancer risk: a quantitative systematic review. J Breast Cancer. (2013) 16:72–6. doi: 10.4048/jbc.2013.16.1.72
239. Qu X, Zhang X, Qin A, Liu G, Zhai Z, Hao Y, et al. Bone mineral density and risk of breast cancer in postmenopausal women. Breast Cancer Res Treat. (2013) 138:261–71. doi: 10.1007/s10549-013-2431-3
240. Liu X, Lv K. Cruciferous vegetables intake is inversely associated with risk of breast cancer: a meta-analysis. Breast. (2013) 22:309–13. doi: 10.1016/j.breast.2012.07.013
241. Lin Y, Wang C, Zhong Y, Huang X, Peng L, Shan G, et al. Striking life events associated with primary breast cancer susceptibility in women: a meta-analysis study. J Exp Clin Cancer Res. (2013) 32:53. doi: 10.1186/1756-9966-32-53
242. Kim JS, Kang EJ, Woo OH, Park KH, Woo SU, Yang DS, et al. The relationship between preeclampsia, pregnancy-induced hypertension and maternal risk of breast cancer: a meta-analysis. Acta Oncol. (2013) 52:1643–8. doi: 10.3109/0284186x.2012.750033
243. Kamdar BB, Tergas AI, Mateen FJ, Bhayani NH, Oh J. Night-shift work and risk of breast cancer: a systematic review and meta-analysis. Breast Cancer Res Treat. (2013) 138:291–301. doi: 10.1007/s10549-013-2433-1
244. Heikkilä K, Nyberg ST, Theorell T, Fransson EI, Alfredsson L, Bjorner JB, et al. Work stress and risk of cancer: meta-analysis of 5700 incident cancer events in 116,000 European men and women. Bmj. (2013) 346:f165. doi: 10.1136/bmj.f165
245. Hui C, Qi X, Qianyong Z, Xiaoli P, Jundong Z, Mantian M. Flavonoids, flavonoid subclasses and breast cancer risk: a meta-analysis of epidemiologic studies. PloS One. (2013) 8:e54318. doi: 10.1371/journal.pone.0054318
246. Gao Y, Huang YB, Liu XO, Chen C, Dai HJ, Song FJ, et al. Tea consumption, alcohol drinking and physical activity associations with breast cancer risk among Chinese females: a systematic review and meta-analysis. Asian Pac J Cancer Prev. (2013) 14:7543–50. doi: 10.7314/apjcp.2013.14.12.7543
247. Bonifazi M, Tramacere I, Pomponio G, Gabrielli B, Avvedimento EV, La Vecchia C, et al. Systemic sclerosis (scleroderma) and cancer risk: systematic review and meta-analysis of observational studies. Rheumatol (Oxford). (2013) 52:143–54. doi: 10.1093/rheumatology/kes303
248. Amadou A, Ferrari P, Muwonge R, Moskal A, Biessy C, Romieu I, et al. Overweight, obesity and risk of premenopausal breast cancer according to ethnicity: a systematic review and dose-response meta-analysis. Obes Rev. (2013) 14:665–78. doi: 10.1111/obr.12028
249. Undela K, Srikanth V, Bansal D. Statin use and risk of breast cancer: a meta-analysis of observational studies. Breast Cancer Res Treat. (2012) 135:261–9. doi: 10.1007/s10549-012-2154-x
250. Ni XJ, Xia TS, Zhao YC, Ma JJ, Zhao J, Liu XA, et al. Postmenopausal hormone therapy is associated with in situ breast cancer risk. Asian Pac J Cancer Prev. (2012) 13:3917–25. doi: 10.7314/apjcp.2012.13.8.3917
251. Hu F, Wang Yi B, Zhang W, Liang J, Lin C, Li D, et al. Carotenoids and breast cancer risk: a meta-analysis and meta-regression. Breast Cancer Res Treat. (2012) 131:239–53. doi: 10.1007/s10549-011-1723-8
252. Du X, Zhang R, Xue Y, Li D, Cai J, Zhou S, et al. Insulin glargine and risk of cancer: a meta-analysis. Int J Biol Markers. (2012) 27:e241–6. doi: 10.5301/jbm.2012.9349
253. Aune D, Chan DS, Vieira AR, Rosenblatt DA, Vieira R, Greenwood DC, et al. Fruits, vegetables and breast cancer risk: a systematic review and meta-analysis of prospective studies. Breast Cancer Res Treat. (2012) 134:479–93. doi: 10.1007/s10549-012-2118-1
254. Aune D, Chan DS, Vieira AR, Navarro Rosenblatt DA, Vieira R, Greenwood DC, et al. Dietary compared with blood concentrations of carotenoids and breast cancer risk: a systematic review and meta-analysis of prospective studies. Am J Clin Nutr. (2012) 96:356–73. doi: 10.3945/ajcn.112.034165
255. Angelousi AG, Anagnostou VK, Stamatakos MK, Georgiopoulos GA, Kontzoglou KC. Primary HT and risk for breast cancer: a systematic review and meta-analysis. Eur J Endocrinol. (2012) 166:373–81. doi: 10.1530/eje-11-0838
256. Walker K, Bratton DJ, Frost C. Premenopausal endogenous oestrogen levels and breast cancer risk: a meta-analysis. Br J Cancer. (2011) 105:1451–7. doi: 10.1038/bjc.2011.358
257. Dong JY, Zhang L, He K, Qin LQ. Dairy consumption and risk of breast cancer: a meta-analysis of prospective cohort studies. Breast Cancer Res Treat. (2011) 127:23–31. doi: 10.1007/s10549-011-1467-5
258. Chan AL, Leung HW, Wang SF. Multivitamin supplement use and risk of breast cancer: a meta-analysis. Ann Pharmacother. (2011) 45:476–84. doi: 10.1345/aph.1P445
259. Key TJ, Appleby PN, Reeves GK, Roddam AW. Insulin-like growth factor 1 (IGF1), IGF binding protein 3 (IGFBP3), and breast cancer risk: pooled individual data analysis of 17 prospective studies. Lancet Oncol. (2010) 11:530–42. doi: 10.1016/s1470-2045(10)70095-4
260. Buck K, Zaineddin AK, Vrieling A, Linseisen J, Chang-Claude J. Meta-analyses of lignans and enterolignans in relation to breast cancer risk. Am J Clin Nutr. (2010) 92:141–53. doi: 10.3945/ajcn.2009.28573
261. Xu X, Dailey AB, Peoples-Sheps M, Talbott EO, Li N, Roth J. Birth weight as a risk factor for breast cancer: a meta-analysis of 18 epidemiological studies. J Womens Health (Larchmt). (2009) 18:1169–78. doi: 10.1089/jwh.2008.1034
262. Vojtechova P, Martin RM. The association of atopic diseases with breast, prostate, and colorectal cancers: a meta-analysis. Cancer Causes Control. (2009) 20:1091–105. doi: 10.1007/s10552-009-9334-y
263. Sadri G, Mahjub H. Passive or active smoking, which is more relevant to breast cancer. Saudi Med J. (2007) 28:254–8.
264. Xue F, Michels KB. Intrauterine factors and risk of breast cancer: a systematic review and meta-analysis of current evidence. Lancet Oncol. (2007) 8:1088–100. doi: 10.1016/s1470-2045(07)70377-7
265. Takkouche B, Etminan M, Montes-Martínez A. Personal use of hair dyes and risk of cancer: a meta-analysis. Jama. (2005) 293:2516–25. doi: 10.1001/jama.293.20.2516
266. Renehan AG, Zwahlen M, Minder C, O'Dwyer ST, Shalet SM, Egger M. Insulin-like growth factor (IGF)-I, IGF binding protein-3, and cancer risk: systematic review and meta-regression analysis. Lancet. (2004) 363:1346–53. doi: 10.1016/s0140-6736(04)16044-3
267. Boyd NF, Stone J, Vogt KN, Connelly BS, Martin LJ, Minkin S. Dietary fat and breast cancer risk revisited: a meta-analysis of the published literature. Br J Cancer. (2003) 89:1672–85. doi: 10.1038/sj.bjc.6601314
268. Khuder SA, Simon VJ Jr. Is there an association between passive smoking and breast cancer? Eur J Epidemiol. (2000) 16:1117–21. doi: 10.1023/a:1010967513957
269. Brennan SF, Cantwell MM, Cardwell CR, Velentzis LS, Woodside JV. Dietary patterns and breast cancer risk: a systematic review and meta-analysis. Am J Clin Nutr. (2010) 91:1294–302. doi: 10.3945/ajcn.2009.28796
270. Harris HR, Tamimi RM, Willett WC, Hankinson SE, Michels KB. Body size across the life course, mammographic density, and risk of breast cancer. Am J Epidemiol. (2011) 174:909–18. doi: 10.1093/aje/kwr225
271. Danforth DN. The role of chronic inflammation in the development of breast cancer. Cancers (Basel). (2021) 13(15):3918. doi: 10.3390/cancers13153918
272. Tan R, Cong T, Xu G, Hao Z, Liao J, Xie Y, et al. Anthracycline-induced atrial structural and electrical remodeling characterizes early cardiotoxicity and contributes to atrial conductive instability and dysfunction. Antioxid Redox Signal. (2022) 37:19–39. doi: 10.1089/ars.2021.0002
273. Biggar RJ, Wohlfahrt J, Oudin A, Hjuler T, Melbye M. Digoxin use and the risk of breast cancer in women. J Clin Oncol. (2011) 29:2165–70. doi: 10.1200/JCO.2010.32.8146
274. Wassertheil-Smoller S, McGinn AP, Martin L, Rodriguez BL, Stefanick ML, Perez M. The associations of atrial fibrillation with the risks of incident invasive breast and colorectal cancer. Am J Epidemiol. (2017) 185:372–84. doi: 10.1093/aje/kww185
275. Vachon CM, Sasano H, Ghosh K, Brandt KR, Watson DA, Reynolds C, et al. Aromatase immunoreactivity is increased in mammographically dense regions of the breast. Breast Cancer Res Treat. (2011) 125:243–52. doi: 10.1007/s10549-010-0944-6
276. Garcia-Estevez L, Cortes J, Perez S, Calvo I, Gallegos I, Moreno-Bueno G. Obesity and breast cancer: A paradoxical and controversial relationship influenced by menopausal status. Front Oncol. (2021) 11:705911. doi: 10.3389/fonc.2021.705911
277. Ryan AS, Berman DM, Nicklas BJ. Plasma adiponectin and leptin levels, body composition, and glucose utilization in adult women with wide ranges of age and obesity. Diabetes Care. (2003) 26:2383–8. doi: 10.2337/diacare.26.8.2383
278. Kim KY, Baek A, Hwang JE, Choi YA, Jeong J, Lee MS, et al. Adiponectin-activated AMPK stimulates dephosphorylation of AKT through protein phosphatase 2A activation. Cancer Res. (2009) 69:4018–26. doi: 10.1158/0008-5472.CAN-08-2641
279. Zhong W, Wang X, Wang Y, Sun G, Zhang J, Li Z. Obesity and endocrine-related cancer: The important role of IGF-1. Front Endocrinol (Lausanne). (2023) 14:1093257. doi: 10.3389/fendo.2023.1093257
280. Wright. CM, Moorin. RE, Chowdhury. EK, Stricker. BH, Reid. CM, Saunders. CM, et al. Calcium channel blockers and breast cancer incidence: An updated systematic review and meta-analysis of the evidence. Cancer Epidemiol. (2017) 50:Pt A. doi: 10.1016/j.canep.2017.08.012
281. Ho C, Ha NT, Youens D, Abhayaratna WP, Bulsara MK, Hughes JD, et al. Association between long-term use of calcium channel blockers (CCB) and the risk of breast cancer: a retrospective longitudinal observational study protocol. BMJ Open. (2024) 14:e080982. doi: 10.1136/bmjopen-2023-080982
282. Lin SY, Huang HY, Chiang LT, Huang LY, Wang CC. Use of calcium channel blockers and risk of breast cancer among women aged 55 years and older: a nationwide population-based cohort study. Hypertens Res. (2023) 46:2272–9. doi: 10.1038/s41440-023-01321-y
283. Raebel MA, Zeng C, Cheetham TC, Smith DH, Feigelson HS, Carroll NM, et al. Risk of breast cancer with long-term use of calcium channel blockers or angiotensin-converting enzyme inhibitors among older women. Am J Epidemiol. (2017) 185:264–73. doi: 10.1093/aje/kww217
Keywords: breast cancer, etiology, cohort studies, umbrella review, meta-analysis
Citation: Wang Z, Feng L, Xia Y, Zhu Z, Wu L and Gao S (2025) Risk factors for breast cancer: an umbrella review of observational cohort studies and causal relationship analysis. Front. Oncol. 15:1541233. doi: 10.3389/fonc.2025.1541233
Received: 07 December 2024; Accepted: 11 April 2025;
Published: 02 May 2025.
Edited by:
Tewodros Eshete, St. Paul’s Hospital Millennium Medical College, EthiopiaReviewed by:
Maryam Khayamzadeh, Academy of Medical Sciences, IranNiyat Essayas Tekie, Addis Ababa University, Ethiopia
Copyright © 2025 Wang, Feng, Xia, Zhu, Wu and Gao. This is an open-access article distributed under the terms of the Creative Commons Attribution License (CC BY). The use, distribution or reproduction in other forums is permitted, provided the original author(s) and the copyright owner(s) are credited and that the original publication in this journal is cited, in accordance with accepted academic practice. No use, distribution or reproduction is permitted which does not comply with these terms.
*Correspondence: Lina Wu, d3VsbjFAc2otaG9zcGl0YWwub3Jn; Song Gao, Z2FvZ2FvMDIyOUBob3RtYWlsLmNvbQ==