- 1School of Pharmacy, Tianjin Medical University, Tianjin, China
- 2Department of Pharmacy, Chu Hisen-I Memorial Hospital, Tianjin Medical University, Tianjin, China
- 3Tianjin Neurological Institute, Tianjin Medical University, Tianjin, China
- 4Tang Center for Herbal Medicine Research, University of Chicago, Chicago, IL, United States
- 5Department of Pathogen Biology, School of Basic Medical Sciences, Tianjin Medical University, Tianjin, China
American ginseng extract (AGE) is an efficient and low-toxic adjuvant for type 2 diabetes mellitus (T2DM). However, the metabolic mechanisms of AGE against T2DM remain unknown. In this study, a rat model of T2DM was created and administered for 28 days. Their biological (body weight and serum biochemical indicators) and pathological (pancreatic sections stained with HE) information were collected for further pharmacodynamic evaluation. Moreover, an ultra-performance liquid chromatography–mass spectrometry–based (UHPLC–MS/MS–based) untargeted metabolomics method was used to identify potential biomarkers of serum samples from all rats and related metabolic pathways. The results indicated that body weight, fasting blood glucose (FBG), fasting blood insulin (FINS), blood triglyceride concentration (TG), high-density lipoprotein cholesterol (HDL-C), insulin resistance index (HOMA-IR) and insulin sensitivity index (ISI), and impaired islet cells were significantly improved after the high dose of AGE (H_AGE) and metformin treatment. Metabolomics analysis identified 101 potential biomarkers among which 94 metabolites had an obvious callback. These potential biomarkers were mainly enriched in nine metabolic pathways linked to amino acid metabolism and lipid metabolism. Tryptophan metabolism and glutathione metabolism, as differential metabolic pathways between AGE and metformin for treating T2DM, were further explored. Further analysis of the aforementioned results suggested that the anti-T2DM effect of AGE was closely associated with inflammation, oxidative stress, endothelial dysfunction, dyslipidemia, immune response, insulin resistance, insulin secretion, and T2DM-related complications. This study can provide powerful support for the systematic exploration of the mechanism of AGE against T2DM and a basis for the clinical diagnosis of T2DM.
Introduction
The prevalence of diabetes, a chronic disease that is both serious and common in this day and age, is increasing every year. According to statistics, the global prevalence of diabetes in people aged 20–79 years is estimated at 10.5% in 2021 and will rise to 12.2% by 2045 (Ogurtsova et al., 2022). , It should be noted that type 2 diabetes accounts for more than 90% of patients with diabetes (Chatterjee et al., 2017). It is well known that the typical feature of T2DM is hyperglycemia mainly induced by insulin resistance (Borse et al., 2021). A variety of serious symptoms such as blindness, kidney failure, heart attacks, and so on caused by T2DM can greatly shorten patients’ life (Nasykhova et al., 2020). Most western drugs can bring about great side effects while performing effective anti-T2DM effects (Lee et al., 2021). Surprisingly, many plant extracts have a better anti-T2DM effect and lower side reaction than modern medicines (Tran et al., 2020). Given this, traditional Chinese medicine extracts have wide application prospects in the clinical treatment of T2DM and are promising to develop new drugs.
American ginseng is one of the well-known ginseng species originating from North America, which has multiple medicinal active ingredients such as ginsenosides, polyacetylenes, polyphenolic compounds, and so on (Punja, 2011). Ginsenosides, as its major active component, play a crucial role in treating cancer, type 2 diabetes, cardiovascular diseases, and central nervous system diseases (Qi et al., 2011). Research studies have proven that AGE has an antioxidant effect, reduce glucose levels, and improve insulin sensitization (Yin et al., 2008). AGE can promote insulin production and prevent β-cell loss by inhibiting mitochondrial uncoupling protein-2 (UCP-2), increasing the ATP level and anti-apoptotic factor B-cell lymphoma-2 (Bcl-2), and downregulating pro-apoptotic factor caspase-9 (Luo and Luo, 2006). Furthermore, AGE was acknowledged as an efficient and low-toxic adjunct for T2DM (Vuksan et al., 2019). Consequently, it is of great significance to study the mechanism of AGE against T2DM based on the metabolic level.
Metabonomics is a popular discipline dedicated to detecting all small-molecule metabolites stemming from biological systems based on all kinds of analytical methods (Liggi and Griffin, 2017). Its application in mass spectrometry (MS) analysis is more extensive than genomics and proteomics providing the benefit of higher precision, a lower sample number, etc. In addition, because of its high sensitivity and wide detection range, liquid chromatography–mass spectrometry (LC–MS) was deemed to be a more powerful method to screen potential markers from biological samples (Ma et al., 2018). In addition, metabonomics technology can facilitate the process of discovering and developing novel drugs through the identification of molecular biomarkers.
In our research, a UHPLC–MS/MS-based untargeted metabolomics method was applied to study the mechanism of AGE against T2DM by identifying its potential biomarkers and metabolic pathways.
Materials and methods
Chemicals and reagents
The standards of HPLC grade ginsenoside Rb1, Re, Rg1, Rc, and Rd (Shanghai Yuanye Bio-Technology Co., Ltd.). HPLC grade acetonitrile and methanol (Tianjin Kemio Chemical Reagent Co., LTD.). Ultrapure water (A Milli-Q water purification system; Merck, America). Blood glucose test strips (Roche Diabetes Care GmbH). Glucose assay kit, triglyceride assay kit, and high-density lipoprotein cholesterol assay kit (Nanjing Jiancheng Bioengineering Institute). Rat INS (Insulin) ELISA kit (Elabscience Biotechnology Co., Ltd.).
Preparation of the AGE and quality control
American ginseng (Beijing Tong-Ren-Tang Pharmaceutical Co., Ltd., No. 20190601) was crushed, weighed, and extracted with 70% ethanol by Soxhlet reflux. With the solvent of extract evaporated under a vacuum at 50°C, the concentrates were collected. Applying a freeze dryer lyophilized these concentrates to acquire AGE in a powder form. The yield of the extract was ∼ 39%. Furthermore, the content of ginsenosides from AGE was measured using an Agilent 1200 HPLC System (Bruker, America) and an anti-phase C18 column (Welch, Shanghai). The mobile phase comprised [A] water and [B] acetonitrile. The followings are the gradient elution conditions: 0 min, 17.5%; 0–20 min, 21%; 20–40 min, 26%; 40–42 min, 40%; and 42–50 min, 50%. The flow rate was set to 1.0 ml/min, and the detection wavelength was set at 203 nm.
Induction of experimental diabetes mellitus in mice
Male SD rats (180∼220 g) were offered by Beijing Vital River Co., Ltd. After adaptive feeding for 1 week, the rats were separated into the control group and model group at random. The two groups were, respectively, treated with a common feed and a high-fat feed (comprising 20% sucrose, 10% lard, 5% yolk powder, 2.5% cholesterol, 1% sodium cholate, and 66.5% maintenance feed, purchased from Beijing XiaoshuYoutai Biotechnology Co., Ltd.). After being treated with high-fat feed for 8 weeks, the model rats were injected intraperitoneally with 33 mg/kg of streptozotocin (STZ) (Gentihold, Beijing). The control rats were injected with 1 ml/kg citrate buffer (Suolaibao, Beijing). By taking blood samples from the tail vein, we tested FBG values by applying blood glucose test strips every week. Ultimately, those rats with FBG >16.7 mmol/L were separated into four groups at random, including the model (sodium chloride injection: 5 ml/kg/d) group, metformin (0.2 g/kg/d) group, low-dose American ginseng extract (L_AGE) (1.5 g/kg/d) group, and high-dose American ginseng extract (H_AGE) (3.0 g/kg/d) group. The aforementioned experiment was in accordance with the Animal Ethics Committee of the Institute of Radiation Medicine, Chinese Academy of Medical Sciences and the approval number is IRM-DWLL-2020148.
Histopathological observation and biochemical index detection
The rats were fasted overnight (12 h) after 4 weeks of administration and anesthetized with 10% chloral hydrate. Blood was obtained from the abdominal aorta using a 5 ml vacuum blood collection tube (containing inert separator gel and procoagulant). Then the samples were left to stand for 30 min and centrifuged at 3,000 rpm for 15 min. The supernatant was dispensed into 2 ml lyophilized tubes, snap-frozen in liquid nitrogen for 10 min and placed in a foam box with dry ice, and finally transferred to a −80°C refrigerator for storage. The pancreas of rats was isolated, fixed with 4% paraformaldehyde, dehydrated, translucent, embedded in paraffin, sectioned, and stained. The staining material was hematoxylin–eosin. Pancreatic pathology was observed using an optical microscope (Leica DMI3000B, Germany) with a magnification of ×400. The efficacy of AGE was evaluated by comparing the area and shape of islets between groups.
In addition, the serum samples of every group were also used to test fasting blood glucose (FBG), fasting blood insulin (FINS), blood triglyceride concentration (TG), and high-density lipoprotein cholesterol (HDL-C). The insulin resistance index (HOMA-IR) and insulin sensitivity index (ISI) were also reckoned to assess the efficacy of AGE. HOMA-IR = (FBG, mmol/L) × (FINS, mIU/L)/22.5; ISI = 1/(FBG, mmol/L) × (FINS, mIU/L).
Sample pretreatment
A measure of 100 µL of the serum sample was accurately weighed into a 1.5-ml centrifuge tube, 400 µL of methanol:acetonitrile (1:1, v/v) solution containing 0.02 mg/ml of internal standard (L-2-chlorophenylalanine) was added, then vortexed and mixed for 30 s, followed immediately by low-temperature sonication for 30 min (5°C, 40 KHz). The samples were left at −20°C temperature for 30 min and centrifuged for 15 min (13000 g, 4°C). The supernatant was removed and blown dry with nitrogen. The residue was reconstituted with 100 μL of the reconstituted solution (acetonitrile: water = 1:1), then vortexed and mixed for 30 s, followed by low-temperature sonication for 30 min (5°C, 40 KHz) and centrifugation for 10 min (13,000 g, 4°C), and the supernatant was pipetted into the injection vial with an internal cannula for analysis. In addition, 20 µL of the supernatant for each sample was mixed as a quality control sample.
UHPLC–MS/MS conditions
Using a Thermo UHPLC system (ACQUITY UPLC HSS T3: 100 mm × 2.1 mm i. d. 1.8 µm; Waters, Milford, United States) for completed chromatographic separation of the metabolites. Gradient elution comprised 0.1% formic acid in water:acetonitrile (95:5,v/v) [A] and 0.1% formic acid in acetonitrile:isopropanol:water (47.5:47.5:5,v/v) [B] at a flow of 0.4 ml/min as follows: 0–3.5 min, 24.5% B; 3.5–5, min 65% B; 5–5.5 min, 100% B; 5.5–7.4 min, 100% B; 7.4–7.6 min, 51.5% B; and 7.6–10 min, 0% B. The sample size was 2 μL, and the column was kept under 40°C temperature. A Thermo UHPLC-Q Exactive HF-X Mass Spectrometer provided with an electrospray ionization (ESI) source was used to obtain the mass spectrometric data. The heater and capillary temperatures were, respectively, set to 425 and 325°C. The sheath gas and aux gas flow rates were, respectively, set to 50 arb and 13 arb. The ion-spray voltage floating (ISVF) in positive and negative patterns was, respectively, set to −3500 and 3500 V. Full MS and MS/MS resolutions were, respectively, set to 60,000 and 7,500 using the data-dependent acquisition (DDA) mode acquisition data. The mass range was set to 70–1,050 m/z during the detection.
Multivariate data processing and analysis
The original data obtained by UHPLC–MS detections were input into the Progenesis QI 2.3 (Nonlinear Dynamics, Waters, United States) for baseline filtering, peak identification, integration, retention time correction, peak alignment, normalization, and integration. The internal standard was used for data QC (reproducibility), while variables with relative standard deviations (RSDs) > 30% of the QC samples were excluded and log transformed data to obtain the final data matrix for subsequent analysis. The MS and MS/MS mass spectrometry information was matched with KEGG (https://www.kegg.jp/kegg/pathway.html), Human Metabolome Database (HMDB) (http://www.hmdb.ca/), and Metlin (https://metlin.scripps.edu/) databases to obtain metabolite information.
The pre-processed data were uploaded on the Majorbio Cloud Platform (https://cloud.majorbio.com) for data analysis. Principal component analysis (PCA), partial squares discriminant analysis (PLS-DA), and orthogonal least squares discriminant analysis (OPLS-DA) were performed using the ropls R package (Version1.6.2, http://bioconductor.org/packages/release/bioc/html/ropls.html). Also, 7-fold cross-validation and 200 permutation tests were used to assess the stability of the OPLS-DA model and to check whether it was overfitted. In addition, Student’s t-test analysis was performed. The selection of significantly different metabolites was determined based on the variable importance in the projection (VIP) obtained from the OPLS-DA model and the p-value of the Student’s t-test, with metabolites with VIP>1 and p < 0.05 being significantly different metabolites. The potential biomarkers were further screened based on KEGG and HMDB databases. Finally, the scipy.stats module in the Python software package was used for pathway enrichment analysis, and Fisher’s exact method was used to obtain the biological pathways most relevant to the experimental processing.
Statistical analysis
The data obtained from the experiment are shown as the mean ± standard deviation (mean ± SD). We applied the GraphPad Prism 8.0.2 statistical analysis software to carry out Student’s t-test and two-way ANOVA. All p-values were two-tailed, and differences with p-values < 0.05 were considered statistically significant.
Results
Ginsenosides from AGE
The HPLC technology was used to determine the content of main ginsenosides from AGE. The following, respectively, represented the content of these ginsenosides (%): Rb1 (8.69), Re (3.34), Rd (1.36), Rc (0.96), and Rg1 (0.58) (Supplementary Figure S1).
Weight observation results
Figure 1 depicts the weight change trend of each group during administration. The rats in the control group and metformin had a gradual increase in weight. The weight of model rats first increased and then slowly decreased. Compared with the model group, the weight change in the L_AGE and H_AGE groups showed a clear opposite trend.
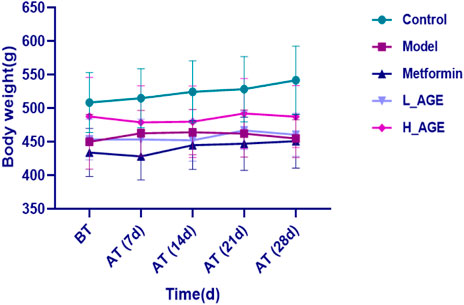
FIGURE 1. Body weight change of control, model, and treatment rats. Note: data are expressed as means ± SD (n = 6); BT: before treatment; AT: after treatment.
Biochemical detection results
The levels of FBG, FINS, TG, and HDL-C in serum samples were evaluated or calculated to reflect the efficacy of administration. Figures 2A–F show that AGE and metformin improved the aforementioned biochemical markers compared with the model rats. Compared with rats in the AGE group, the FBG, FINS, and HOMA-IR index in metformin rats were better regulated; adversely, the HDL-C, TG, and ISI index were better modulated in AGE rats compared with the metformin rats.
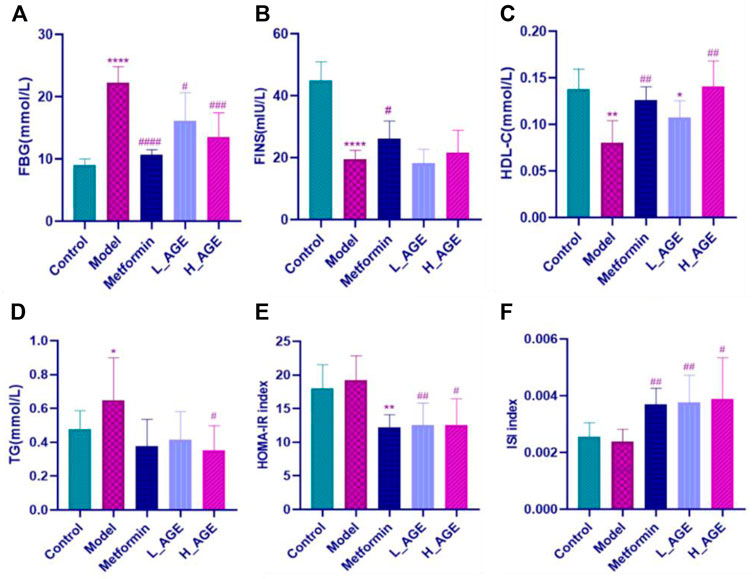
FIGURE 2. Serum biochemical marker levels in each group. (A–F) respectively represent the levels of fasting blood glucose (FBG), fasting blood insulin (FINS), high-density lipoprotein cholesterol (HDL-C), blood triglyceride concentration (TG), insulin resistance index (HOMA-IR) and insulin sensitivity index (ISI) in serum samples. Note: data are expressed as mean ± SD (n = 6); Student’s t-test: compared with the control group, ****p < 0.0001,***p < 0.001, **p < 0.01, and*p < 0.05; compared with the model group, ####p < 0.0001,###p < 0.001,##p < 0.01, and #p < 0.05.
Pancreas section results
To verify the protective effect of AGE, we observed the morphology and size of pancreatic islets in pancreatic sections (Figure 3). The islets of the model rats had fewer cells, smaller size, more irregular morphology, and vacuolar degeneration compared with those of the control rats. The islets of the rats in the L_AGE group were not significantly different from those of the model rats. The islets in the metformin and H_AGE groups were more regular in morphology, had a higher number of islet cells, did not have vacuolar degeneration, and tended to be at the level of the control group with a recovery percentage of about 40%–50% compared to the model rats.
Metabolomic study of serum samples
The stability for UHPLC–MS/MS analysis
The total ion chromatograms (TIC) of quality control (QC) samples were obtained in the positive and negative ion modes. Taking sample QC01 as an example, the TIC (Supplementary Figure S2) consistently showed a good peak shape and relatively uniform distribution. It was verified that the LC–MS system was stable in the process of the whole detection.
Multivariate statistical analysis
To better unveil the distinction among samples of different groups, we simultaneously adopted the PCA and PLS-DA approaches. The closer the distance of dots in the diagram, the more similar the metabolite expression pattern of samples. As shown in Figures 4A–D, there was an obvious separation between the samples in the model rats and control rats; the metabolite samples in the treatment groups and model group also had an obvious deviation; the samples in metformin rats were more comparable to the control rats than those to the H_AGE rats, suggesting that the efficacy of metformin was more significant than that of H_AGE; the samples in H_AGE rats were more comparable to the control rats than those to the L_AGE rats, which indicated the more significant efficacy of H_AGE against T2DM than L_AGE.
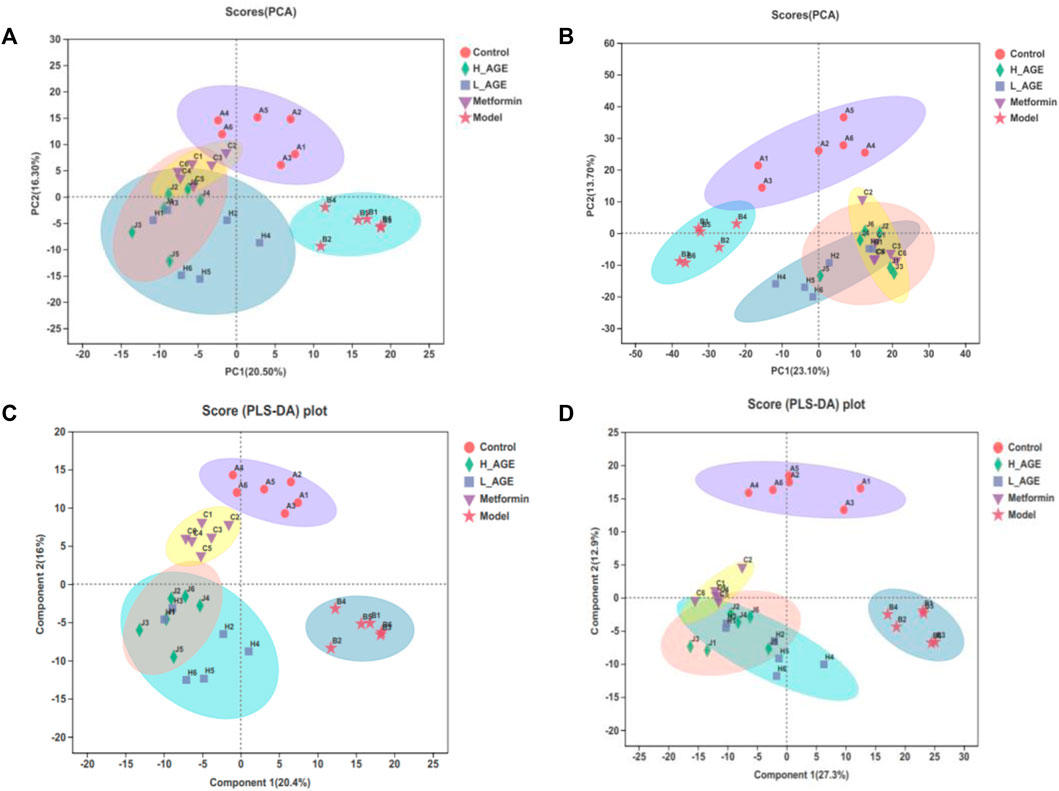
FIGURE 4. PCA score diagrams of the samples from control, model, and treatment rats in ESI + mode (A) or ESI- mode (B). PLS-DA score diagrams of the samples from control, model, and treatment rats in ESI+ (C) or ESI- (D) modes. The ellipse expresses that the confidence interval is 95%.
In contrast with PLS-DA, OPLS-DA can greatly distinguish the differences between the two groups and improve the effectiveness and analytical capability of the model. Therefore, we adopted the OPLS-DA method to analyze the metabolomics data of samples on control vs. model and model vs. H_AGE group. Figures 5A–D and Supplementary Figures S3A–D display the results. The score diagrams for OPLS-DA presented a clear separation between the aforementioned two pairs of groups. Using the 7-fold cross-validation method gained R2X (cum), R2Y (cum), and Q2 (cum) of the OPLS-DA model to verify its reliability. The more these parameters are inclined to 1, the more the model is reliable. Q2 > 0.5 indicated the good predictive ability of the OPLS-DA model. For OPLS-DA models obtained from control and model groups, the three parameters, respectively, are 0.435, 0.994, and 0.91 in the positive ion mode and 0.509, 0.994, and 0.924 in the negative ion mode. Moreover, we used 200 response permutation testing (RPT) to estimate the accuracy of the OPLS-DA model, in which the left R2 and Q2 values were less than the right initial values, suggesting that the model was available.
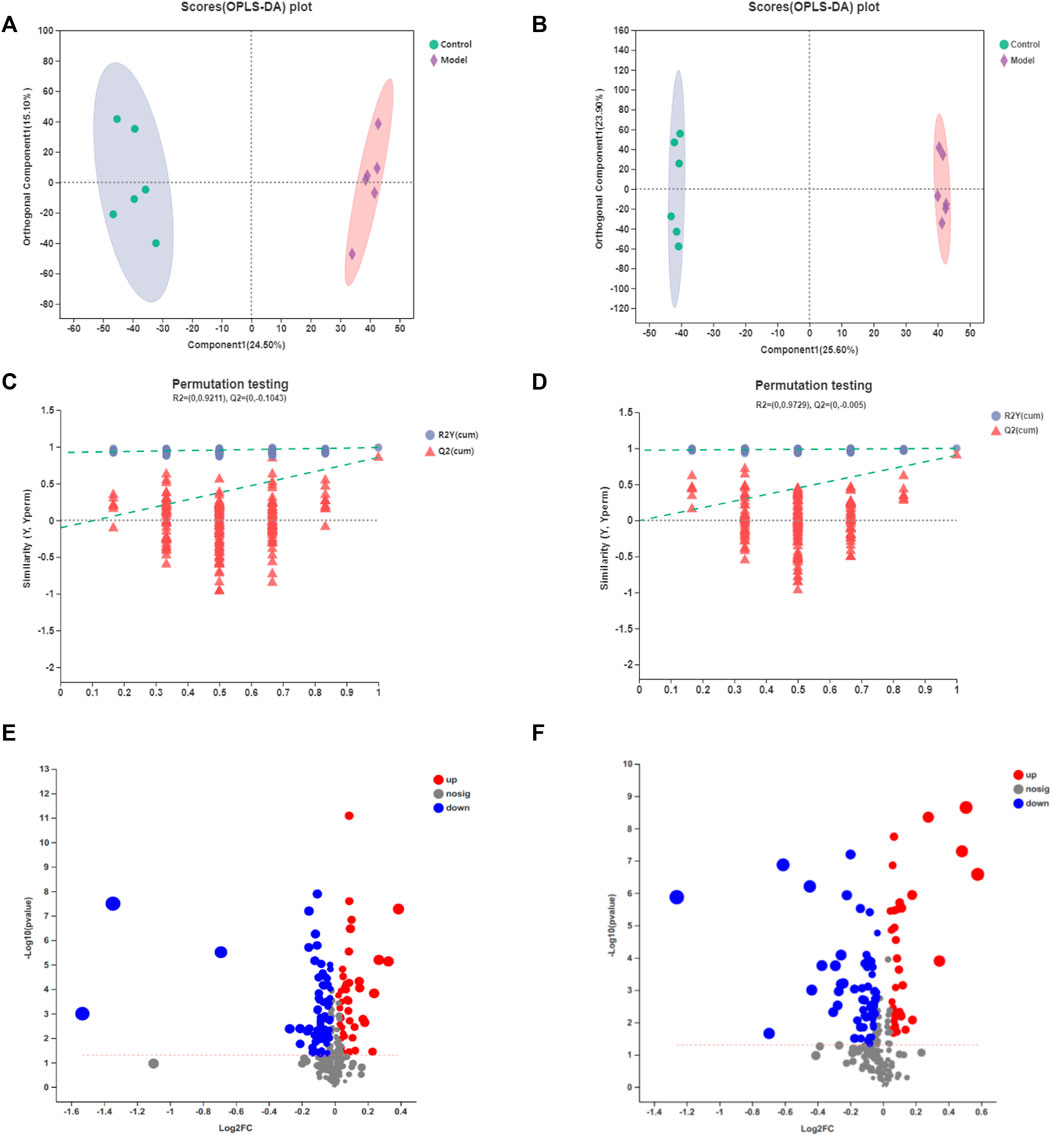
FIGURE 5. OPLS-DA score diagrams of the samples from control vs. model in the positive mode (A) or negative mode (B); 7-fold cross-validation plot of the OPLS-DA model with 200 permutation tests in the positive mode (C) or negative mode (D); volcano plots of the samples from control vs. model in the positive mode (E) or negative mode (F). Ellipse expresses that the confidence interval is 95%.
The volcano plots (as shown in Figures 5E,F) and (Supplementary Figures S3E,F) showed the changing trend of the differential metabolite expression in model vs. control and H_AGE vs. model groups. Each dot represents a specific metabolite and the size of the dot indicates the VIP value. The larger the dot, the larger the VIP value. The red dot refers to the downregulated metabolites and the blue dot refers to upregulated metabolites.
Potential biomarker identification
With a threshold of VIP >1.0, p < 0.05, we identified 204 differential metabolites (model vs. control), 224 differential metabolites (metformin vs. model), 173 differential metabolites (L_AGE vs. model), and 191 differential metabolites (H_AGE vs. model). A total of 111 intersectant metabolites were obtained by overlapping the differential metabolites between model vs. control and metformin vs. model groups. A total of 106 intersectant metabolites were screened by overlapping the differential metabolites between model vs. control and H_AGE vs. model groups. Among these 106 metabolites, 101 significant differential metabolites can be searched by HMDB or KEGG databases and were viewed as potential biomarkers of AGE against T2DM (Supplementary Table S1). Compared with the model group, the expression of 94 potential biomarkers was significantly backregulated in the H_AGE group. Figure 6 intuitively presents the relative expression of 101 potential biomarkers (49 from the ESI + mode and 52 from the ESI- mode) in the serum samples of control, model, H_AGE, and metformin groups in the form of a heatmap.
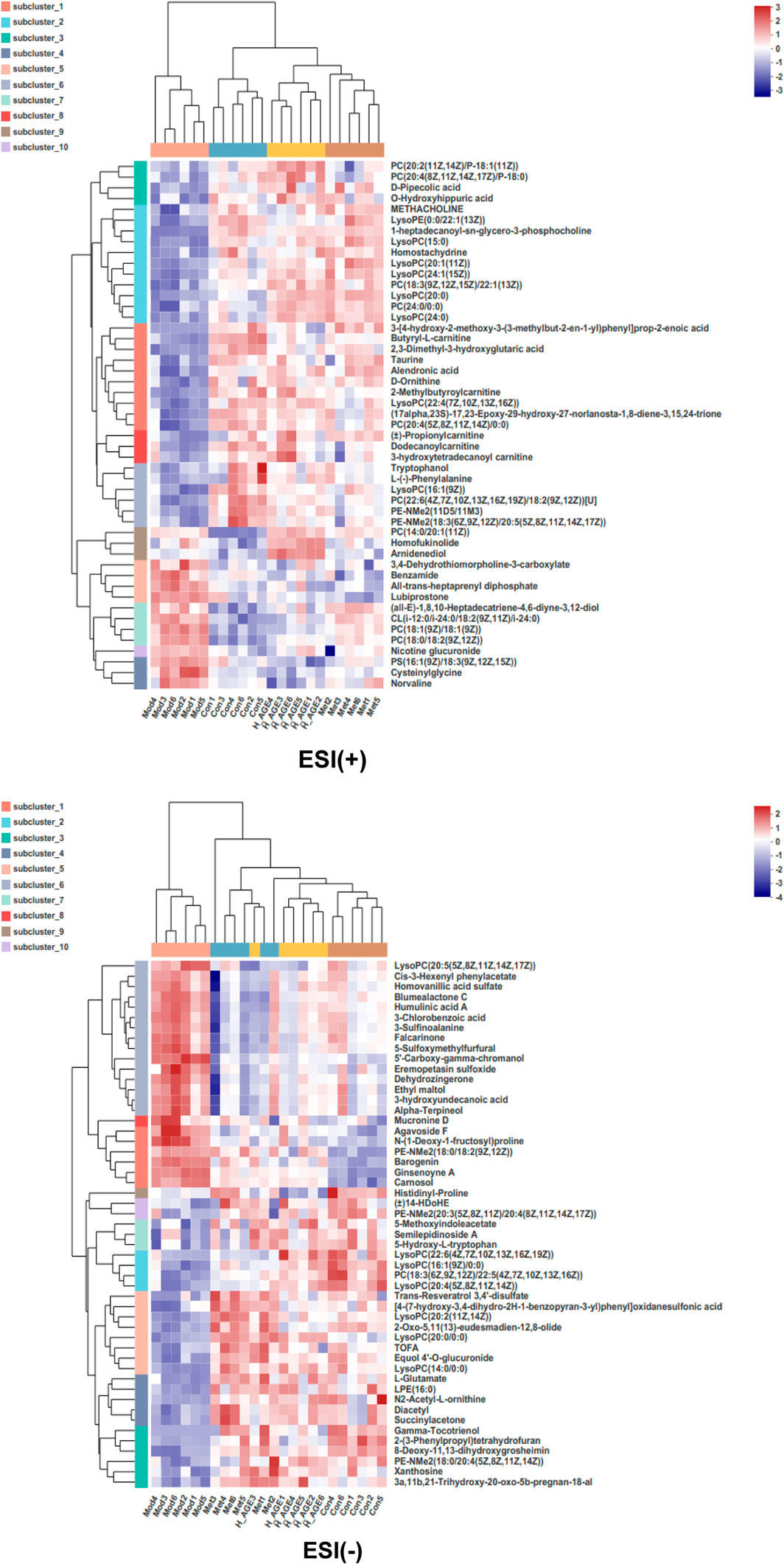
FIGURE 6. Heatmap of potential biomarkers. Rows: biomarkers; columns: samples. The shade in this picture depicts the relative expression size of metabolites in each group sample. Red depicts that the expression of biomarker was increased, blue depicts that the expression of biomarker was decreased (Mod vs. Con; Met vs. Mod; H_AGE vs. Mod).
Metabolomics pathway results
To explore the connection among potential biomarkers, metabolic pathways, and metabolic mechanisms of AGE against T2DM, the 106 intersectant metabolites between model vs. control and H_AGE vs. model groups carried out pathway enrichment (Figure 7A). The metabolic pathways with p < 0.05 and impact value >0.1 were thought of as the most important pathways, including D-glutamine and D-glutamate metabolism, taurine and hypotaurine metabolism, arginine biosynthesis, tryptophan metabolism, and D-arginine and D-ornithine metabolism (Table 1). Metabolites enriched in these important pathways were probably the most important potential biomarkers, including l-glutamate, taurine, 3-sulfinoalanine, 5-hydroxy-l-tryptophan, 5-methoxyindoleacetate, and D-ornithine . To further explore the differences in the metabolic pathways and mechanisms of AGE and metformin in treating T2DM, we also performed pathway enrichment analysis on 111 intersectant metabolites between model vs. control and metformin vs. model groups (Figure 7B). Notably, unlike metformin, AGE also exerts its anti-T2DM effect by improving disorders of tryptophan metabolism and glutathione metabolism.
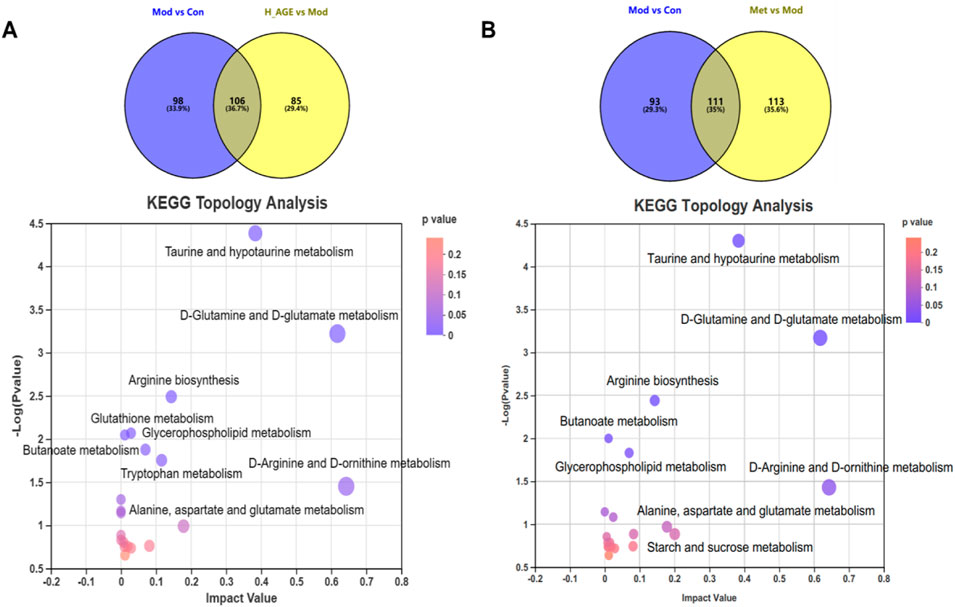
FIGURE 7. Metabolic pathway analysis. (A) refers to the pathway enrichment analysis of the intersectant metabolites between Mod vs Con and H_AGE vs Mod. (B) refers to the pathway enrichment analysis of the intersectant metabolites between Mod vs Con and Met vs Mod. The X-axis represents the impact value and the Y-axis represents–log10(p-value). The size of the bubble represents the impact value. The larger the bubble and higher the–log10(p-value), the greater the importance of the pathway.
Discussion
T2DM is a metabolic syndrome with complex pathogenesis, which primarily results in the disorders of carbohydrate, lipid, and protein metabolism (DeFronzo et al., 2015). Currently, many biologically active traditional Chinese herbal medicines (TCHMs) are widely used in the treatment of type 2 diabetes and are expected to be its alternative therapies (Hasanpour et al., 2020). Metabolomics, as an important component of system biology, helps to confirm the safety and efficacy of these TCHMs, explores their potential mechanisms of action and potential biomarkers, and further identifies the targets of these TCHMs (Shi et al., 2016). Furthermore, metabolomics can unveil metabolic characteristics of the whole system after interventions, which conform to the overall notion of TCHMs and is conducive to its modern research (Wang et al., 2015). Therefore, we attempted to study the metabolic mechanisms of AGE against T2DM by metabolomics technology.
In this study, based on the pharmacodynamic evaluation results, we found that AGE can exert its significant efficacy by improving blood lipid levels and protecting the islets in T2DM rats. The metabolomics results revealed that H_AGE can greatly reverse the metabolic disorder in T2DM rats by regulating 101 potential biomarkers. Furthermore, the pathway enrichment and KEGG topology analysis suggested that anti-T2DM mechanisms of AGE were closely associated with regulating the disorders of amino acid and lipid metabolism. Herein, we aimed to explore the potential mechanisms of AGE against T2DM by investigating some special potential biomarkers and metabolic pathways.
A previous study has shown that American ginseng can increase the content of carnitine and help restore disorders of lipid metabolism in the body (Zhang et al., 2019). However, it was found that butyryl-L-carnitine (BC), as a kind of butyrate ester of carnitine, can produce butyrate and carnitine and is mainly used to alleviate gut inflammation through carnitine transporter OCTN2 and the amino acid transporter ATB0,+ (Srinivas et al., 2007). In addition, propionylcarnitine (PC), is a kind of analog of L-carnitine, can alleviate peripheral vascular disease–related (PAD-related) symptoms and decrease glycemic levels in T2DM patients with PAD (Ragozzino et al., 2004). An experiment also has revealed that PC can improve cardiac function and enhance ischemic tolerance of the diabetic rat heart (Broderick et al., 2004). The expression of BC and PC was upregulated after treatment, indicating that AGE and metformin are beneficial for alleviating inflammation, and improving heart function in diabetic rats. Gamma-tocotrienol is an isomer of unsaturated vitamin E, which can alleviate adipose tissue inflammation and maintain insulin sensitivity by suppressing nuclear factor kappa-B (NF-κB) and Nod-like receptor 3 (NLRP3) inflammasome (Kim et al., 2016). Compared to the model group, the expression of gamma-tocotrienol was upregulated in the H_AGE and metformin group, demonstrating that the anti-T2DM effect of H_AGE and metformin may be closely linked to inflammation.
The metabonomics data presented that the contents of lysophosphatidylcholines (lysoPCs), lysophosphatidylethanolamines (lysoPEs), and phosphatidylcholines (PCs) expect for lysoPC(20:5 (5Z,8Z,11Z,14Z,17Z)), PC(18:1 (9Z)/18:1 (9Z)),PC(14:0/20:1 (11Z)), and PC(18:0/18:2 (9Z,12Z) were downregulated in model rats and upregulated in AGE rats, suggesting that lipid metabolism may play an essential role in AGE against T2DM. A research study also manifested that these lipid levels were downregulated in T2DM patients with cardiovascular disease (CVD) compared with the T2DM patients, indicating that the expression of these lipids is negatively correlated with the risk of cardiovascular disease in T2DM patients (Garcia-Fontana et al., 2016). Moreover, previous studies have shown that lysoPCs and lysoPEs can be used as circulating metabolic intermediates associated with inflammation and oxidative stress to predict the risk of early diabetes (Ha et al., 2012). A previous report revealed that lysoPC can play an important role in modulating vascular endothelial dilation, cellular immune response, and insulin secretion (Yamamoto et al., 2021). Phosphatidylcholine (PC) is the most vital phospholipid enriched in the endoplasmic reticulum, which is involved in lipid storage/secretion, stress response, and the occurrence of diabetes (Lagace and Ridgway, 2013; Wang et al. (2011) suggested that choline, trimethylamine N-oxide (TMAO), and betaine, as the three metabolites of the dietary lipid phosphatidylcholine, were helpful to predict risk for cardiovascular diseases (CVDs).
Trans-resveratrol 3,4′-disulfate belongs to the class of organic compounds known as stilbenes. Stilbenes are important polyphenolic compounds with a variety of pharmacological activities such as anti-inflammatory, anti-diabetic, and anti-lipidemic and have significant modulating effects on angiogenesis, inflammation, and cell proliferation (Tsai et al., 2017; ShazmeenHaq et al., 2021). The expression of trans-resveratrol 3,4′-disulfate was significantly increased after both H_AGE and metformin treatment, indicating that this compound may play an essential role in AGE and metformin against T2DM.
Tryptophan metabolism is an important metabolic pathway involved in regulating inflammation-related diseases. l-Tryptophan (tryptophan) can decrease blood glucose levels and preserve normal insulin secretion in T2DM rats (Sorgdrager et al., 2019). 5-Hydroxy-l-tryptophan (5-HTP), as the immediate precursor in the biosynthesis of 5-hydroxy-tryptamine (5-HT), can inhibit insulin and glucagon secretion in non-diabetic islet donors and significantly increased the release of insulin in response to glucose in islets isolated from T2D donors (Bennet et al., 2015, (Das et al., 2004). In our study, 5-HTP enriched in the tryptophan metabolism pathway was downregulated in the model rats and upregulated in the H_AGE rats, demonstrating that H_AGE may promote insulin secretion by regulating tryptophan metabolism. Glutathione (γ-glutamyl-cysteine glycine) belongs to a class of low molecular weight thiols synthesized from glutamate, cysteine, and glycine (Wu et al., 2004). Glutathione deficiency is highly likely to lead to the development of T2DM (Hakki Kalkan and Suher, 2013). Glutathione metabolism plays a key role in inhibiting oxidative stress and cysteinyl-glycine can be decomposed into cysteine and glycine under the catalysis of dipeptidase (Lutchmansingh et al., 2018). Our study found that cysteinyl-glycine and l-glutamate were enriched in the glutathione metabolic pathway. The lower cysteinyl-glycine level and higher l-glutamate level in the H_AGE group compared to the model group, indicate that AGE may further synthesize glutathione by promoting the production of cysteine, glycine, and l-glutamate to suppress the process of T2DM.
L-Arginine (arginine), as the substrate for NO production by endothelial cells, is effective in the treatment of endothelial dysfunction triggered by cardiovascular risk factors (Gambardella et al., 2020). It can stimulate insulin production by acting on voltage-gated Ca2+ channels and promote insulin secretion through transforming to l-glutamate (Newsholme and Krause, 2012). l-Glutamate, as a kind of non-essential amino acid abundant in the body and a substrate in metabolism, is derived from the biological synthesis and food intake, as well as enhances insulin secretion (Takahashi et al., 2019). Han, et al. (2021) said that glutamate converted from glutamine is an essential mediator that enhances calcium signaling in the glutamine-amplifying effect on insulin secretion. We observed that the l-glutamate content was downregulated in the model rats and was significantly upregulated after H_AGE and metformin treatment, suggesting that AGE may stimulate insulin secretion by regulating multiple metabolic pathways related to l-glutamate.
Taurine is a type of abundant amino acid that existed in all mammalian tissues, which can improve the disorders of glucose metabolism and lipid metabolism by decreasing serum leptin levels and insulin resistance (Kim et al., 2012). Increasing evidence has shown that taurine can play a beneficial effect on diabetes and its complications through the suppression of oxidative stress and inflammation (Ito et al., 2012; Qaradakhi et al., 2020). Taurine levels increased after H_AGE and metformin treatment, suggesting that H_AGE exerts its efficacy by interfering with the metabolism of taurine and hypotaurine, which is consistent with the past research study (Zhang et al., 2019). Ornithine is an intermediate compound produced by the enzymatic action of arginase and synthesized into urea through the urea cycle (Sivashanmugam and Jaidev 2017). A hospital-based cross-sectional study revealed a negative correlation between T2DM and ornithine levels in the urea cycle, which is consistent with our present results (Cao et al., 2019). A study showed that amino acids involved in the urea cycle are associated with inflammation and oxidative stress (Carracedo et al., 2011). These facts indicated that H_AGE and metformin may mainly exert anti-inflammatory and anti-oxidant effects through the regulation of amino acid metabolism.
TOFA (5-(tetradecyloxy)-2-furoic acid) is an effective hypolipidemic agent that can significantly inhibit fatty acid synthesis, stimulate fatty acid oxidation, and reduce triglyceride synthesis (Halvorson and McCune, 1984; Fukuda and Ontko, 1984). In addition, it was reported that a-terpineol can increase lipid levels by modulating the AMPK/mTOR/SREBP-1 pathway. Compared with the model group, H_AGE and metformin can significantly increase the content of TOFA and decrease the content of a-terpineol, indicating that H_AGE and metformin may exert their anti-T2DM effect by reducing lipid levels. Pipecolic acid is a non-protein amino acid originating from lysine catabolism and is essential for the regulation of human and plant immunity (Wang et al., 2018). According to previous studies, pipecolic acid acts as a key regulator of various plant defense responses and contributes to immune signaling salicylic acid (SA) production, antimicrobial metabolite accumulation, and transcription of defense genes (Zeier, 2013). The higher level of D-pipecolic acid in the H_AGE and metformin rats indicated that AGE and metformin may enhance body immune response by increasing the content of D-pipecolic acid.
Conclusion
Ultimately, the curative effect of AGE and metformin on T2DM was verified by biochemical analysis and pancreas section analysis. Based on UHPLC–MS/MS–based untargeted metabolomics technology and multivariate statistical analysis, we found that AGE can have a significant anti-T2DM effect by modulating 94 potential biomarkers contents. The enrichment analysis revealed that AGE mainly had an impact on the metabolic disturbance of D-glutamine and D-glutamate metabolism, taurine, and hypotaurine metabolism, arginine biosynthesis, and tryptophan metabolism, and D-arginine and D-ornithine metabolism. Further analysis demonstrated that the anti-T2DM mechanisms of AGE were mainly related to suppressing inflammation, oxidative stress, endothelial dysfunction, dyslipidemia, insulin resistance, T2DM-related complications and enhancing immune response, and insulin secretion. Our study systematically elucidated for the first time that the potential biomarkers and mechanisms of AGE against T2DM from a metabolic perspective, which can provide a reference for new drug development and the clinical diagnosis of T2DM.
Data availability statement
The original contributions presented in the study are included in the article/Supplementary Material; further inquiries can be directed to the corresponding authors.
Ethics statement
The animal study was reviewed and approved by the Animal Ethics Committee of the Institute of Radiation Medicine, Chinese Academy of Medical Sciences and the approval number is IRM-DWLL-2020148.
Author contributions
TL: Methodology, data curation, and writing—original draft. DW: Methodology, data curation, writing–review and editing. XZ: Conceptualization, methodology, and data curation. JS: Methodology and data curation. ZY: Data curation, writing–review and editing. CS: Writing–review and editing. RL: Methodology and data curation. YZ: Data curation. JZ: Data curation. JY: Methodology. XZ: Data curation and writing—original draft. YL: Data curation. MG: Data curation. CW: Methodology. CY: Data curation. YC: Methodology, writing—review and editing. XW: Supervision, writing—review and editing.
Acknowledgments
The work was supported in part by the National Nature Science Foundation of China (No. 81974521) and the Tianjin natural science foundation key project (No. 19JCZDJC33500).
Conflict of interest
The authors declare that the research was conducted in the absence of any commercial or financial relationships that could be construed as a potential conflict of interest.
Publisher’s note
All claims expressed in this article are solely those of the authors and do not necessarily represent those of their affiliated organizations, or those of the publisher, the editors, and the reviewers. Any product that may be evaluated in this article, or claim that may be made by its manufacturer, is not guaranteed or endorsed by the publisher.
Supplementary material
The Supplementary Material for this article can be found online at: https://www.frontiersin.org/articles/10.3389/fphar.2022.960050/full#supplementary-material
References
Bennet, H., Balhuizen, A., Medina, A., Dekker Nitert, M., Ottosson Laakso, E., Essen, S., et al. (2015). Altered serotonin (5-HT) 1D and 2A receptor expression may contribute to defective insulin and glucagon secretion in human type 2 diabetes. Peptides 71, 113–120. doi:10.1016/j.peptides.2015.07.008
Borse, S. P., Chhipa, A. S., Sharma, V., Singh, D. P., and Nivsarkar, M. (2021). Management of type 2 diabetes: Current strategies, unfocussed aspects, challenges, and alternatives. Med. Princ. Pract. 30, 109–121. doi:10.1159/000511002
Broderick, T. L., Paulson, D. J., and Gillis, M. (2004). Effects of propionyl-carnitine on mitochondrial respiration and post-ischaemic cardiac function in the ischaemic underperfused diabetic rat heart. Drugs R. D. 5, 191–201. doi:10.2165/00126839-200405040-00002
Cao, Y. F., Li, J., Zhang, Z., Liu, J., Sun, X. Y., Feng, X. F., et al. (2019). Plasma levels of amino acids related to urea cycle and risk of type 2 diabetes mellitus in Chinese adults. Front. Endocrinol. 10, 50. doi:10.3389/fendo.2019.00050
Carracedo, J., Merino, A., Briceno, C., Soriano, S., Buendia, P., Calleros, L., et al. (2011). Carbamylated low-density lipoprotein induces oxidative stress and accelerated senescence in human endothelial progenitor cells. FASEB J. 25, 1314–1322. doi:10.1096/fj.10-173377
Chatterjee, S., Khunti, K., and Davies, M. J. (2017). Type 2 diabetes. Lancet 389, 2239–2251. doi:10.1016/S0140-6736(17)30058-2
Das, Y. T., Bagchi, M., Bagchi, D., and Preuss, H. G. (2004). Safety of 5-hydroxy-L-tryptophan. Toxicol. Lett. 150, 111–122. doi:10.1016/j.toxlet.2003.12.070
Defronzo, R. A., Ferrannini, E., Groop, L., Henry, R. R., Herman, W. H., Holst, J. J., et al. (2015). Type 2 diabetes mellitus. Nat. Rev. Dis. Prim. 1, 15019. doi:10.1038/nrdp.2015.19
Fukuda, N., and Ontko, J. A. (1984). Interactions between fatty acid synthesis, oxidation, and esterification in the production of triglyceride-rich lipoproteins by the liver. J. Lipid Res. 25, 831–842. doi:10.1016/s0022-2275(20)37747-6
Gambardella, J., Khondkar, W., Morelli, M. B., Wang, X., Santulli, G., and Trimarco, V. (2020). Arginine and endothelial function. Biomedicines 8, E277. doi:10.3390/biomedicines8080277
Garcia-Fontana, B., Morales-Santana, S., Diaz navarro, C., Rozas-Moreno, P., Genilloud, O., Vicente perez, F., et al. (2016). Metabolomic profile related to cardiovascular disease in patients with type 2 diabetes mellitus: A pilot study. Talanta 148, 135–143. doi:10.1016/j.talanta.2015.10.070
Ha, C. Y., Kim, J. Y., Paik, J. K., Kim, O. Y., Paik, Y. H., Lee, E. J., et al. (2012). The association of specific metabolites of lipid metabolism with markers of oxidative stress, inflammation and arterial stiffness in men with newly diagnosed type 2 diabetes. Clin. Endocrinol. 76, 674–682. doi:10.1111/j.1365-2265.2011.04244.x
Hakki kalkan, I., and Suher, M. (2013). The relationship between the level of glutathione, impairment of glucose metabolism and complications of diabetes mellitus. Pak. J. Med. Sci. 29, 938–942. doi:10.12669/pjms.294.2859
Halvorson, D. L., and Mccune, S. A. (1984). Inhibition of fatty acid synthesis in isolated adipocytes by 5-(tetradecyloxy)-2-furoic acid. Lipids 19, 851–856. doi:10.1007/BF02534514
Han, G., Takahashi, H., Murao, N., Gheni, G., Yokoi, N., Hamamoto, Y., et al. (2021). Glutamate is an essential mediator in glutamine-amplified insulin secretion. J. Diabetes Investig. 12, 920–930. doi:10.1111/jdi.13497
Hasanpour, M., Iranshahy, M., and Iranshahi, M. (2020). The application of metabolomics in investigating anti-diabetic activity of medicinal plants. Biomed. Pharmacother. 128, 110263. doi:10.1016/j.biopha.2020.110263
Ito, T., Schaffer, S. W., and Azuma, J. (2012). The potential usefulness of taurine on diabetes mellitus and its complications. Amino Acids 42, 1529–1539. doi:10.1007/s00726-011-0883-5
Kim, K. S., Oh, D. H., Kim, J. Y., Lee, B. G., You, J. S., Chang, K. J., et al. (2012). Taurine ameliorates hyperglycemia and dyslipidemia by reducing insulin resistance and leptin level in Otsuka Long-Evans Tokushima fatty (OLETF) rats with long-term diabetes. Exp. Mol. Med. 44, 665–673. doi:10.3858/emm.2012.44.11.075
Kim, Y., Wang, W., Okla, M., Kang, I., Moreau, R., and Chung, S. (2016). Suppression of NLRP3 inflammasome by gamma-tocotrienol ameliorates type 2 diabetes. J. Lipid Res. 57, 66–76. doi:10.1194/jlr.M062828
Lagace, T. A., and Ridgway, N. D. (2013). The role of phospholipids in the biological activity and structure of the endoplasmic reticulum. Biochim. Biophys. Acta 1833, 2499–2510. doi:10.1016/j.bbamcr.2013.05.018
Lee, J., Noh, S., Lim, S., and Kim, B. (2021). Plant Extracts for Type 2 Diabetes: From Traditional Medicine to Modern Drug Discovery. Antioxidants (Basel). 10. 81. doi:10.3390/antiox10010081
Liggi, S., and Griffin, J. L. (2017). Metabolomics applied to diabetes-lessons from human population studies. Int. J. Biochem. Cell Biol. 93, 136–147. doi:10.1016/j.biocel.2017.10.011
Luo, J. Z., and Luo, L. (2006). American ginseng stimulates insulin production and prevents apoptosis through regulation of uncoupling protein-2 in cultured beta cells. Evid. Based. Complement. Altern. Med. 3, 365–372. doi:10.1093/ecam/nel026
Lutchmansingh, F. K., Hsu, J. W., Bennett, F. I., Badaloo, A. V., Mcfarlane-Anderson, N., Gordon-Strachan, G. M., et al. (2018). Glutathione metabolism in type 2 diabetes and its relationship with microvascular complications and glycemia. PLoS One 13, e0198626. doi:10.1371/journal.pone.0198626
Ma, Q., Li, Y., Wang, M., Tang, Z., Wang, T., Liu, C., et al. (2018). Progress in metabonomics of type 2 diabetes mellitus. Molecules 23, E1834. doi:10.3390/molecules23071834
Nasykhova, Y. A., Tonyan, Z. N., Mikhailova, A. A., Danilova, M. M., and Glotov, A. S. (2020). Pharmacogenetics of type 2 diabetes-progress and prospects. Int. J. Mol. Sci. 21, E6842. doi:10.3390/ijms21186842
Newsholme, P., and Krause, M. (2012). Nutritional regulation of insulin secretion: Implications for diabetes. Clin. Biochem. Rev. 33, 35–47.
Ogurtsova, K., Guariguata, L., Barengo, N. C., Ruiz, P. L., Sacre, J. W., Karuranga, S., et al. (2022). IDF diabetes Atlas: Global estimates of undiagnosed diabetes in adults for 2021. Diabetes Res. Clin. Pract. 183, 109118. doi:10.1016/j.diabres.2021.109118
Punja, Z. K. (2011). American ginseng: Research developments, opportunities, and challenges. J. Ginseng Res. 35, 368–374. doi:10.5142/jgr.2011.35.3.368
Qaradakhi, T., Gadanec, L. K., Mcsweeney, K. R., Abraham, J. R., Apostolopoulos, V., and Zulli, A. (2020). The anti-inflammatory effect of taurine on cardiovascular disease. Nutrients 12, E2847. doi:10.3390/nu12092847
Qi, L. W., Wang, C. Z., and Yuan, C. S. (2011). Ginsenosides from American ginseng: Chemical and pharmacological diversity. Phytochemistry 72, 689–699. doi:10.1016/j.phytochem.2011.02.012
Ragozzino, G., Mattera, E., Madrid, E., Salomone, P., Fasano, C., Gioia, F., et al. (2004). Effects of propionyl-carnitine in patients with type 2 diabetes and peripheral vascular disease: Results of a pilot trial. Drugs R. D. 5, 185–190. doi:10.2165/00126839-200405040-00001
ShazmeenHaq, I. U., Rajoka, M. S. R., Asim Shabbir, M., Umair, M., Llah, I. U., Manzoor, M. F., et al. (2021). Role of stilbenes against insulin resistance: A review. Food Sci. Nutr. 9, 6389–6405. doi:10.1002/fsn3.2553
Shi, J., Cao, B., Wang, X. W., Aa, J. Y., Duan, J. A., Zhu, X. X., et al. (2016). Metabolomics and its application to the evaluation of the efficacy and toxicity of traditional Chinese herb medicines. J. Chromatogr. B Anal. Technol. Biomed. Life Sci. 1026, 204–216. doi:10.1016/j.jchromb.2015.10.014
Sivashanmugam, M., and Jaidev, J. (2017). Ornithine and its role in metabolic diseases: An appraisal. Biomed. Pharmacother. 86, 185–194. doi:10.1016/j.biopha.2016.12.024
Sorgdrager, F. J. H., Naude, P. J. W., Kema, I. P., Nollen, E. A., and Deyn, P. P. (2019). Tryptophan metabolism in inflammaging: From biomarker to therapeutic target. Front. Immunol. 10, 2565. doi:10.3389/fimmu.2019.02565
Srinivas, S. R., Prasad, P. D., Umapathy, N. S., Ganapathy, V., and Shekhawat, P. S. (2007). Transport of butyryl-L-carnitine, a potential prodrug, via the carnitine transporter OCTN2 and the amino acid transporter ATB(0, +). Am. J. Physiol. Gastrointest. Liver Physiol. 293, G1046–G1053. doi:10.1152/ajpgi.00233.2007
Takahashi, H., Yokoi, N., and Seino, S. (2019). Glutamate as intracellular and extracellular signals in pancreatic islet functions. Proc. Jpn. Acad. Ser. B Phys. Biol. Sci. 95, 246–260. doi:10.2183/pjab.95.017
Tran, N., Pham, B., and Le, L. (2020). Bioactive Compounds in Anti-Diabetic Plants: From Herbal Medicine to Modern Drug Discovery. Biol. (Basel). 9. 252. doi:10.3390/biology9090252
Tsai, H. Y., Ho, C. T., and Chen, Y. K. (2017). Biological actions and molecular effects of resveratrol, pterostilbene, and 3'-hydroxypterostilbene. J. Food Drug Anal. 25, 134–147. doi:10.1016/j.jfda.2016.07.004
Vuksan, V., Xu, Z. Z., Jovanovski, E., Jenkins, A. L., Beljan-Zdravkovic, U., Sievenpiper, J. L., et al. (2019). Efficacy and safety of American ginseng (panax quinquefolius L.) extract on glycemic control and cardiovascular risk factors in individuals with type 2 diabetes: A double-blind, randomized, cross-over clinical trial. Eur. J. Nutr. 58, 1237–1245. doi:10.1007/s00394-018-1642-0
Wang, Z., Klipfell, E., Bennett, B. J., Koeth, R., Levison, B. S., Dugar, B., et al. (2011). Gut flora metabolism of phosphatidylcholine promotes cardiovascular disease. Nature 472, 57–63. doi:10.1038/nature09922
Wang, P., Wang, Q., Yang, B., Zhao, S., and Kuang, H. (2015). The progress of metabolomics study in traditional Chinese medicine research. Am. J. Chin. Med. 43, 1281–1310. doi:10.1142/S0192415X15500731
Wang, C., Liu, R., Lim, G. H., De Lorenzo, L., Yu, K., Zhang, K., et al. (2018). Pipecolic acid confers systemic immunity by regulating free radicals. Sci. Adv. 4, eaar4509. doi:10.1126/sciadv.aar4509
Wu, G., Fang, Y. Z., Yang, S., Lupton, J. R., and Turner, N. D. (2004). Glutathione metabolism and its implications for health. J. Nutr. 134, 489–492. doi:10.1093/jn/134.3.489
Yamamoto, Y., Sakurai, T., Chen, Z., Furukawa, T., Gowda, S. G. B., Wu, Y., et al. (2021). Analysis of serum lysophosphatidylethanolamine levels in patients with non-alcoholic fatty liver disease by liquid chromatography-tandem mass spectrometry. Anal. Bioanal. Chem. 413, 245–254. doi:10.1007/s00216-020-02996-9
Yin, J., Zhang, H., and Ye, J. (2008). Traditional Chinese medicine in treatment of metabolic syndrome. Endocr. Metab. Immune Disord. Drug Targets 8, 99–111. doi:10.2174/187153008784534330
Zeier, J. (2013). New insights into the regulation of plant immunity by amino acid metabolic pathways. Plant Cell Environ. 36, 2085–2103. doi:10.1111/pce.12122
Keywords: American ginseng extract, metabolomics, type 2 diabetes mellitus, potential biomarkers, metabolic pathways
Citation: Liu T, Wang D, Zhou X, Song J, Yang Z, Shi C, Li R, Zhang Y, Zhang J, Yan J, Zhu X, Li Y, Gong M, Wang C, Yuan C, Cui Y and Wu X (2022) Study on the mechanism of American ginseng extract for treating type 2 diabetes mellitus based on metabolomics. Front. Pharmacol. 13:960050. doi: 10.3389/fphar.2022.960050
Received: 02 June 2022; Accepted: 01 August 2022;
Published: 02 September 2022.
Edited by:
Subhash C. Mandal, Directorate of Drugs Control, Government of West Bengal, IndiaReviewed by:
Asis Bala, National Institute of Pharmaceutical Education and Research, IndiaParasuraman S., AIMST University, Malaysia
Copyright © 2022 Liu, Wang, Zhou, Song, Yang, Shi, Li, Zhang, Zhang, Yan, Zhu, Li, Gong, Wang, Yuan, Cui and Wu. This is an open-access article distributed under the terms of the Creative Commons Attribution License (CC BY). The use, distribution or reproduction in other forums is permitted, provided the original author(s) and the copyright owner(s) are credited and that the original publication in this journal is cited, in accordance with accepted academic practice. No use, distribution or reproduction is permitted which does not comply with these terms.
*Correspondence: Yan Cui, Y3VpeWFuYmlvNDVAMTYzLmNvbQ==; Xiaohui Wu, bG9uZ2h1aTgwNEAxNjMuY29t
†These authors have contributed equally to this work