- Department of Clinical Pharmacology and Therapeutics, Faculty of Medicine and Surgery, University of Malta, Msida, Malta
Lung cancer remains one of the leading causes of cancer-related mortality worldwide, necessitating innovative research methodologies to improve treatment outcomes and develop novel strategies. The advent of three-dimensional (3D) cell cultures has marked a significant advancement in lung cancer research, offering a more physiologically relevant model compared to traditional two-dimensional (2D) cultures. This review elucidates the various types of 3D cell culture models currently used in lung cancer pharmacology, including spheroids, organoids and engineered tissue models, having pivotal roles in enhancing our understanding of lung cancer biology, facilitating drug development, and advancing precision medicine. 3D cell culture systems mimic the complex spatial architecture and microenvironment of lung tumours, providing critical insights into the cellular and molecular mechanisms of tumour progression, metastasis and drug responses. Spheroids, derived from commercialized cell lines, effectively model the tumour microenvironment (TME), including the formation of hypoxic and nutrient gradients, crucial for evaluating the penetration and efficacy of anti-cancer therapeutics. Organoids and tumouroids, derived from primary tissues, recapitulate the heterogeneity of lung cancers and are instrumental in personalized medicine approaches, supporting the simulation of in vivo pharmacological responses in a patient-specific context. Moreover, these models have been co-cultured with various cell types and biomimicry extracellular matrix (ECM) components to further recapitulate the heterotypic cell-cell and cell-ECM interactions present within the lung TME. 3D cultures have been significantly contributing to the identification of novel therapeutic targets and the understanding of resistance mechanisms against conventional therapies. Therefore, this review summarizes the latest findings in drug research involving lung cancer 3D models, together with the common laboratory-based assays used to study drug effects. Additionally, the integration of 3D cell cultures into lung cancer drug development workflows and precision medicine is discussed. This integration is pivotal in accelerating the translation of laboratory findings into clinical applications, thereby advancing the landscape of lung cancer treatment. By closely mirroring human lung tumours, these models not only enhance our understanding of the disease but also pave the way for the development of more effective and personalized therapeutic strategies.
1 Introduction
Lung cancer is a worldwide leading cause of cancer-related deaths, claiming a global 1.8 million fatalities per year (Sung et al., 2021; Adjei, 2019). It is a heterogeneous disease which has proven difficult to treat, and provides a formidable challenge to healthcare and research workers alike. The two main lung cancer subtypes are non-small cell lung cancer (NSCLC) and small cell lung cancer (SCLC). NSCLC is the more common subtype, comprising about 85% of lung cancers and claiming a 5-year survival rate of only 15%. NSCLC is sub-classified into adenocarcinoma, squamous cell carcinoma (SCC) and large cell carcinoma (LCC). Strongly associated with smoking, SCLC is subdivided into pure small cell carcinoma and combined small cell carcinoma, and is characterized by its rapid growth and early metastasis, having an estimated survival rate of 6.2% (Rudin et al., 2021; Liang et al., 2022).
Treatment of lung cancer has posed a global challenge. The World Health Organization had long advocated for minimizing the incidence rate through the avoidance of risk factors, the main ones being tobacco and workplace related hazards such as chemicals and asbestos (World Health Organization, 2023). Current therapies mainly include surgery, chemotherapy, radiation therapy, targeted therapy (e.g., EGFR tyrosine kinase inhibitors (TKI), KRAS-G12C therapies and ALK gene fusions) and immunotherapy (e.g., anti-PD-L1 immunotherapy). While surgery is limited to early-stage tumours, standard chemotherapy and radiation monotherapies have reached a therapeutic limit resulting from severe side-effects and the development of therapy resistance (Huang et al., 2017; Yegya-Raman et al., 2018). Following their recent emergence, antibody drug conjugates (ADC) and immune checkpoint inhibitors (ICI) enable tumour cell targeting and are currently undergoing clinical trials (Desai et al., 2022; Colombo and Rich, 2022; Genova et al., 2022). However, the survival rates for lung cancer remain exceptionally low, in conjunction with a drug development pipeline which has been repeatedly marked with a very high drug attrition rate at the clinical stage (Huo et al., 2020). These outcomes emphasize the urgent need for safe and effective novel therapies to target lung cancer.
Advancements in lung cancer research have increasingly focused on developing more physiologically relevant models to better understand the complexities of the disease and to improve drug discovery efforts. Traditional 2D cell cultures, while foundational, fall short in replicating the intricate tumour microenvironment (TME) and cellular interactions that characterize lung cancers in vivo. In response, the latest 3D cell culture models, including spheroids, organoids, tumoroids and microfluidic devices, have emerged as powerful tools that more accurately mimic the architecture, gene expression and drug responses observed clinically. In order to develop biomimicking lung cancer models in vitro, it is necessary to understand both the histology and function of the normal human lung and the intricacies of the lung TME consisting of complex interactions and responses between various cell types and the extracellular matrix (ECM). Hence, this article provides a solid foundation to the lung microenvironment and explores cutting-edge 3D lung cancer models, focusing on their potential to revolutionize lung cancer pharmacology research by providing more predictive and reliable platforms for preclinical drug testing and personalized medicine.
2 Normal human lung histology
The normal human respiratory framework comprises various cell types which orchestrate lung functionality by creating an optimal environment for gas exchange and activate several protective mechanisms. One of the most abundant cell types in the human airway epithelium are the pseudostratified ciliated columnar epithelial cells (or ciliated cells). Goblet cells and basal epithelial cells also line the tracheal and bronchial epithelium. This epithelium is covered by a layer of mucus mainly consisting of 95% water, 2%–5% mucins (glycoproteins), salts and other proteins and cell fragments (Murgia et al., 2017). Goblet cells secrete mucin granules at their apical surface and therefore help maintain moistness by supporting the formation of the mucus layer which protects the airway from dust, bacteria and other contaminants via the mucociliary escalator (Ganesan et al., 2013). Basal cells are multipotent stem cells involved in epithelial cell renewal, particularly of ciliated cells, and are located at the basement membrane where they support the attachment of ciliated cells. Additionally, basal cells interact with neurons and immune cells and can also be found in the bronchi and down to the terminal bronchioles in lower numbers (Evans et al., 2001; Rackley and Stripp, 2012).
In the respiratory bronchioles, goblet cells are replaced by non-ciliated club (Clara) cells. Club cells constitute around 9% of the total lung epithelium and are present within the lower parts of the airway beginning at the terminal bronchioles (Khan and Lynch, 2023; Rokicki et al., 2016). Protruding above the level of neighbouring cells, club cells possess secretory granules which secrete various lipoproteins (such as Clara cell secretory protein, CCSP or CC10) and surfactant proteins. Therefore, club cells seem to have a homeostatic role with regards to surfactant fluid (Antunes et al., 2013; Lowe and Anderson, 2015).
Brush cells, present from the nasal cavity to the bronchioles, are thought to act as chemoreceptors, though their exact function is unclear (Brody, 2005; Khan and Lynch, 2023; Hollenhorst et al., 2020). Neuroendocrine cells, containing neurosecretory granules, secrete polypeptide hormones and neuropeptides (trigger immune responses) in response to environmental stimuli. Making up 1%–3% of the epithelial cell layer, neuroendocrine cells are considered to be the only innervated cells in the human lung epithelium, possibly controlling goblet cell and submucosal gland activity (Noguchi et al., 2020; Rokicki et al., 2016; Branchfield et al., 2016; Khan and Lynch, 2023).
Type I and Type II pneumocytes line the alveoli: Type I facilitate gas exchange, while Type II are involved in surfactant production, immune cell modulation and stem cell activity (Ruaro et al., 2021; Glisinski et al., 2020). The lungs are safeguarded by a variety of immune cells such as macrophages and dendritic cells which act by phagocytosing pathogens and presenting antigens, respectively (Khan and Lynch, 2023; Harkema et al., 2018). Lung fibroblasts are integral components of the ECM, modulating tissue architecture and elasticity through ECM synthesis and remodelling (White, 2015; Chua and Laurent, 2006). Histological features of the healthy human lung are illustrated in Figure 1.
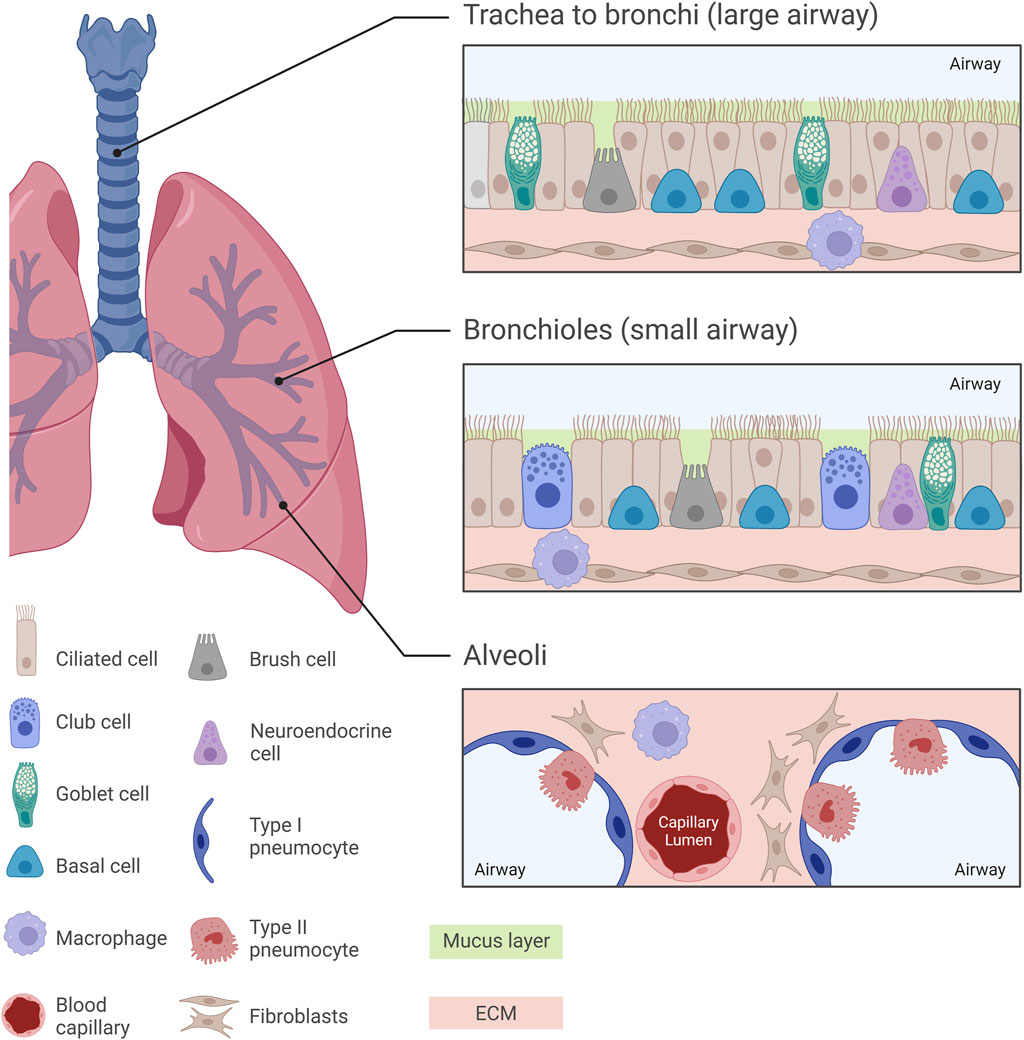
Figure 1. Normal pulmonary histology at different regions of the human lung. Diverse histological features can be observed across different regions of the human lung, from the trachea towards the alveoli, with each region exhibiting specialized cellular arrangements crucial for respiratory function. The trachea, bronchi and bronchioles are characterized by a pseudostratified ciliated columnar epithelium, while the intricate alveolar structures are lined by Type I and Type II pneumocytes. In the small airway, mucus-secreting goblet cells are replaced by surfactant-producing club cells, paralleled with a decrease in the thickness of the mucus layer lining the airway epithelium. Understanding these histological nuances is essential for unravelling the complexities of lung pathology and developing pharmacological in vitro models which replicate the in vivo scenario.
3 The lung tumour microenvironment
The idea of the TME was first coined in 1979 by Lord et al. who studied the interactions between different cells in a tumour (Lord et al., 1979). Apart from tumour cells, the TME consists of several cell types, mainly immune cells, fibroblasts, endothelial cells and cancer stem cells (CSC) (Li and Qiao, 2022; Anderson and Simon, 2020; Wu F. et al., 2021). These cells interact with tumour cells and secrete signalling molecules and ECM components, creating a supportive environment for tumour growth and progression. The ECM consists of both fibrous and multi-adhesive proteins, such as collagen and fibronectin, respectively. It serves to support the spatial arrangement of multiple cell types within the TME all of which communicate with each other and with the ECM to promote tumour growth, immunosuppression and therapy resistance (Labani-Motlagh et al., 2020). Cell-ECM interactions alter structural components to form a physical barrier to the TME, limiting drug accessibility. The lack of homogeneity and limited vasculature penetrability present another barrier to tumour drug delivery (Pinto et al., 2020). In short, the lung TME is a remodelled niche, exploiting every cell type to support tumour growth and metastasis (Altorki et al., 2019). As an area of growing interest, a deep understanding of the TME is crucial for creating accurate biomimetic 3D models. The general cell types and features of the lung TME are illustrated in Figure 2.
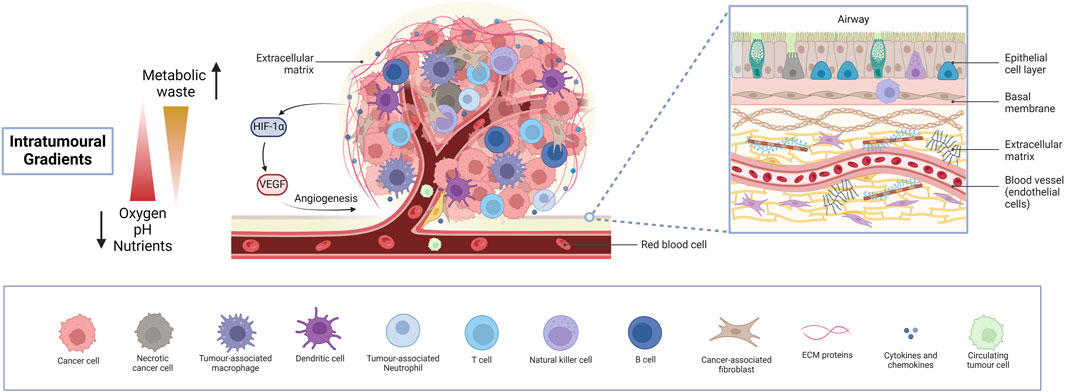
Figure 2. The lung tumour microenvironment. An insight into the intricate heterogeneous cellular and molecular landscape of the lung TME, exhibiting diverse cell types, intra-tumoural gradients and activation of angiogenesis. Cancer cells, together with various immune cells, fibroblasts, and endothelial cells lining blood vessels and several ECM components orchestrate a dynamic interplay driving tumour progression, metastasis and therapy resistance, while limiting drug accessibility. Necrotic areas are fundamentally responsible for the activation of angiogenesis, paving the way for extensive tumour growth. Understanding these complex interactions is crucial for developing lung cancer models for preclinical pharmacological research to effectively target lung cancer and study drug responses. (Abbreviations: TME: tumour microenvironment, ECM: extracellular matrix).
3.1 The lung cancer immune landscape
The immune landscape of lung cancer, particularly non-small cell lung cancer (NSCLC), is a complex and heterogeneous environment that plays a crucial role in the disease’s progression and response to therapies. Indeed, recent studies using single-cell sequencing technologies have identified distinct immune signatures associated with different subtypes of lung cancer, specifically lung adenocarcinoma and lung squamous cell carcinoma (Wang et al., 2022).
A total of 13 tumour immune cell populations have been identified in NSCLC, including macrophages, neutrophils, dendritic cells (DC), natural killer (NK) cells, T cells and B cells, having complex roles and accompanied by immunosuppressive mechanisms (Labani-Motlagh et al., 2020; Madeddu et al., 2022). While the types of NSCLC tumour-infiltrating immune cells are associated with clinical outcomes, strategies aimed at modulating immune cell function are being actively explored (Balážová et al., 2023; Tiwari et al., 2022). Most studies suggest a macrophage-dominant lung cancer tumour-infiltrating immune cell landscape, although other studies exhibited a neutrophil or T cell dominant one (Tamminga et al., 2020; Balážová et al., 2023; Kargl et al., 2017; Liu et al., 2017; Stankovic et al., 2019; Evans et al., 2021). Discrepancies across studies may point towards the extreme heterogeneity of lung cancer amongst different populations. Research on the immune landscape of SCLC is still very lacking.
Tumour-associated macrophages (TAMs), which have been recently described as a unique phenotypic state, play a role in driving the inflammatory response within tumours and are associated with metastatic activation, drug resistance and poor prognosis (Balážová et al., 2023). TAM formation is activated by TME stimuli, including the presence of cancer-associated fibroblasts (CAF) and hypoxia (Tan et al., 2021; Liu J. et al., 2021; Xu et al., 2022; Garnique and Machado-Santelli, 2023; Madeddu et al., 2022).
Macrophages may exist in two major polarisation states: M1 (classically activated; pro-inflammatory) can enhance antitumour immunity (Barrera et al., 2023), while M2 (alternatively activated; anti-inflammatory) secrete immunosuppressive cytokines such as IL-10 and TGF-β and induce Treg expansion, contributing to reduced tumour immunity (Balážová et al., 2023; Bremnes et al., 2011). Additionally, a third undifferentiated subtype, M0, has been recently identified by Hickman et al. (2023). These subtypes have been shown to provide distinct immune signatures that can differentiate lung adenocarcinoma from lung squamous cell carcinoma. Notably, the dominant macrophage subtype in adenocarcinoma was identified as the FABP4-expressing M1 subtype, whereas SPP1-expressing M2 macrophages were predominant in lung squamous cell carcinoma (Wang et al., 2022). While the different subtypes cannot be identified morphologically, a simple immunohistochemistry method based on the high expression of IL12 and CCR7 in M1 and CD163 and ALOX15 in M2 subtypes has been described (Zheng et al., 2020).
T cells constitute a similarly important and highly abundant immune cell type in lung tumours, comprising approximately 47% of all CD45+ immune cells. CD4+ T helper cells and CD8+ cytotoxic T cells are the predominant subsets, followed by a smaller population of double-negative CD4− CD8− T cells (Stankovic et al., 2019). CD8+ T cells are considered the primary effectors of antitumor immunity, capable of directly killing cancer cells upon recognition of tumour antigens presented by MHC class I molecules. CD4+ T cells can differentiate into various helper and regulatory subsets that modulate the immune response. Th1 cells secrete IFN-γ and promote CD8+ T cell cytotoxicity, while Th2 cells support humoral immunity. Regulatory T cells (Tregs) suppress effector T cell function and are associated with a worse prognosis in lung cancer (Bremnes et al., 2011; Zheng et al., 2017).
B cells comprise about 16% of the CD45+ population in lung tumours. They can contribute to antitumor immunity through antibody production, antigen presentation, and cytokine secretion. However, their role in lung cancer is complex, with some studies linking high B cell infiltration to improved survival (Barrera et al., 2023) and others to worse outcomes (Bremnes et al., 2011).
Natural killer (NK) cells make up about 4.5% of the lung tumour immune infiltrate (Stankovic et al., 2019). They can directly lyse tumour cells and secrete cytokines such as IFN-γ that enhance antitumor immunity. Depletion of NK cells has been shown to promote lung tumour growth in mouse models (Barrera et al., 2023), suggesting that they play a protective role.
Dendritic cells are less abundant in lung tumours, comprising only about 2.1% of CD45+ cells. However, they play a crucial role in antigen presentation to T cells. Plasmacytoid dendritic cells secrete type I interferons and promote Th1 responses, while conventional dendritic cells can cross-present tumour antigens to CD8+ T cells (Bremnes et al., 2011).
The spatial organization of immune cells within tumours modulates the interactions between themselves, as well as with the surrounding tissue. This may have implications in tumour progression, proliferative activity, treatment responses and survival, and the challenge to reproduce this environment accurately in a 3D cell culture model may be best addressed by ex vivo 3D setups. For example, Parra et al. (2023) used a 23-marker tumour immunoprofiling panel on NSCLC tumour cells, and reported that CD3+ CD8+ cytotoxic T-cells were the most abundant immune cells in both adenocarcinoma and squamous cell carcinoma, but were relatively distant from malignant cells. In contrast, T-cells expressing PD-L1, B7-H3, B7-H4, IDO-1, and OX40, were less abundant, but were located closer to the malignant cells, suggesting that the distance from malignant cells and distribution patterns both play a role in cancer.
High densities of TAMs are well known to be associated with poor outcomes in many types of cancer. Interestingly, cancer cells undergoing apoptosis have been reported to be located closer to pro-inflammatory M1-polarized macrophages than to anti-inflammatory M2-polarized macrophages, while the reverse was true for tumour cells which were positive for the Ki67 proliferative marker (Zheng et al., 2020). Spatial cellular organisation and immune cell interactions may also influence immunotherapy outcomes. For example, the presence of high spatial niches of T cells, and macrophages in NSCLC adenocarcinomas, increases clonal neoantigen burden, potentially increasing the response of such immune-hot tumours to immunotherapy (Parra et al., 2023).
After studying samples from 120 NSCLC adenocarcinoma patients with disease stages ranging from I to III Barua et al. (2018) reported tumour cell and regulatory T-cell (Treg) interactions to be significantly associated with worse survival, while the co-presence of cytotoxic CD8+ T lymphocytes resulted in better survival. Infiltration of Treg cells into core tumour regions may therefore be an independent predictor of worse overall survival in NSCLC, with the effect being mitigated by co-infiltration of CD8+ cytotoxic T cells. In addition to the influence of immune cells, the tumour vasculature is an important contributor to tumour proliferation rates. This makes the contributory roles of immune cells more difficult to dissect. Enfield et al. (2024) studied the proliferation rates of lung squamous cell carcinoma, based on the differential cell densities of tumour infiltrating lymphocytes (TILs, T cells and B cells), macrophages, and neutrophils within the tumour nest (T) or stroma (S). The lowest proliferative tumours were found to be those with the greatest nest and stromal neutrophil infiltration. Interestingly, these same tumours exhibited the greatest distances between each tumour cell and its nearest endothelial cell, suggesting that the combination of neutrophil activity and reduced oxygen supply jointly contributed to the lower proliferation.
3.2 Immune evasion strategies in lung tumours and implications for treatment
Lung tumours employ various molecular mechanisms to evade the immune system, and these mechanisms also influence immunotherapy treatment. There are three overarching mechanisms that tumours bring into play for immune evasion.
Upregulation of immune checkpoint molecules: One of the most well-studied mechanisms of immune evasion in lung cancer is the upregulation of immune checkpoint molecules, such as programmed death-ligand 1 (PD-L1). PD-L1 expressed on lung cancer cells binds to the PD-1 receptor on T cells, triggering an inhibitory signal that leads to T cell exhaustion and dysfunction. High expression of PD-L1 is often associated with poor prognosis in lung cancer patients.
Impairment of antigen presentation: Lung cancer cells can evade immune detection by downregulating or altering the expression of major histocompatibility complex (MHC) class I molecules, which are responsible for presenting tumour-specific antigens to cytotoxic T cells. This process, known as “MHC class I downregulation,” has been observed in up to 90% of lung tumours (Qin et al., 2016). This can be brought about through various mechanisms including; (a) loss of heterozygosity (LOH) at the HLA locus, leading to the complete loss of HLA expression (Anichini et al., 2020), (b) defects in the antigen processing machinery, such as downregulation of the transporter associated with antigen processing (TAP) (Gupta et al., 2023), (c) epigenetic silencing of genes involved in antigen presentation, such as NLRC5, a key regulator of MHC class I-dependent immune responses (Kobayashi and van den Elsen, 2012).
Induction of an immunosuppressive TME: Lung tumours create an immunosuppressive microenvironment by recruiting and activating various immunosuppressive cells, such as Tregs and myeloid-derived suppressor cells (MDSC). These cells secrete inhibitory cytokines such as IL-10 and TGF-β, which suppress the function of effector T cells. Additionally, lung cancer cells can induce the expression of indoleamine 2,3-dioxygenase (IDO), an enzyme that catabolizes tryptophan, leading to T cell anergy and apoptosis (Salehi-Rad and Dubinett, 2019).
The molecular mechanisms of immune evasion in lung cancer have important implications for immunotherapy, the aim of which is to enhance the immune system’s ability to detect and destroy cancer cells. One of the primary therapeutic modalities involves the use of immune checkpoint inhibitors, which block proteins that inhibit immune responses. Drugs such as pembrolizumab and nivolumab which block programmed cell death protein 1 (PD-1), a T cell expressed receptor, have been successfully used in patients with metastatic NSCLC with high PD-L1 expression and have demonstrated increased survival rates. Other therapeutic approaches have targetted the inhibition of PD-L1 which normally interacts with its receptor PD-1 on T cells and signals to inhibit T cell receptor (TCR)-mediated activation of IL-2 production and T cell proliferation. Examples of such drugs are atezolizumab and durvalumab, both of which are used for advanced metastatic NSCLC. Ipilimumab is an inhibitor of cytotoxic T-lymphocyte associated protein 4 (CTLA-4). This protein is a receptor that is constitutively expressed on Tregs and CD4+/CD8+ conventional T cells. When activated by the B7 ligand, it is upregulated in conventional T cells and signals to deactivate them. By inhibiting CTLA-4, ipilimumab enhances T cell activation and proliferation, enhancing the immune response against cancer cells. It is may be used in combination with nivolumab for advanced NSCLC, providing a synergistic effect that improves treatment outcomes (Hellmann et al., 2019).
The contributions of immune cells within a tumour may be further confounded by the mechanical properties of the ECM. For example, changes in stiffness arising from matrix remodelling through the action of various enzymes such as matrix metalloproteinases (MMP) can facilitate immune cell movement or, conversely, contribute to a more immunosuppressive environment. It has been shown that T cells cultured in high-density collagen matrices exhibit diminished cytotoxic activity and reduced production of key cytokines such as IFN-γ, which are vital for an effective immune response (Du et al., 2024). In NSCLC, osteopontin (OPN), a multifunctional extracellular matrix protein, is associated with the amplification of the checkpoint protein PD-L1 via the NF-κB pathway, therefore serving as a tactical manoeuvre employed by the tumour to elude immune surveillance and annihilation (Li et al., 2021a).
Combining immunotherapy with chemotherapy or targeted therapies has become a standard approach in managing lung cancer. For instance, the combination of pembrolizumab with chemotherapy has shown superior efficacy compared to chemotherapy alone in first-line treatment settings. Such combinations leverage the synergistic effects of different treatment modalities, enhancing overall therapeutic outcomes (Dang et al., 2016; Ruiz-Cordero and Devine, 2020; Alexander et al., 2020; Naratornsirakul et al., 2024).
3.3 Cancer-associated fibroblasts
Fibroblasts are one of the most common cell types present in the lung tumour stroma, of which three subtypes have been identified in NSCLC (Hu H. et al., 2021). In the presence of cancer cells, fibroblasts phenotypically switch to cancer-associated fibroblasts (CAF), marked by a decrease in expression and functional pro-tumour alterations of p53 (Arandkar et al., 2018). CAFs seem to increase therapy resistance in NSCLC, including that of EGFR TKIs, by modulating signalling pathways, activating receptors and via cross-talks with cancer cells. CAFs co-cultured with lung cancer cells were found to increase proliferation and tumour cell survival, induce epithelial-mesenchymal transition (EMT), maintain CSC stemness and promote chemoresistance (Nakamura et al., 2019; Kanaji et al., 2017; Wang et al., 2019; You et al., 2019; Meyerholz et al., 2018; Worrell and MacLeod, 2021). Release of chemokines and cytokines by CAFs was shown to promote ECM remodelling, alter the immune landscape and induce autophagy resulting in the recovery of lung cancer cells from radiation-induced damage, together with elevating the tumour metastatic potential via paracrine signalling involving STAT3 (Shien et al., 2017; Wang et al., 2017; Suzuki et al., 2022; Wong et al., 2022; Zhang H. et al., 2021). Their increased expression of hypoxia-inducible factor 1-alpha (HIF-1α) allows CAFs to survive and modulate hypoxic environments, further stimulating NSCLC therapy resistance (Chen et al., 2021). An improved understanding of the TME has initiated the discovery of anti-cancer therapies targeted towards CAFs aiming to minimize lung cancer resistance and other oncogenic properties (Chen et al., 2021; Zhang H. et al., 2021; Papait et al., 2022).
3.4 Tumour endothelial cells
Tumour endothelial cells within the TME orchestrate neo-vascularisation, forming blood vessels for the transport of oxygen, nutrients and metabolic waste. These cells support tumour growth and provide a vascular system for the release of tumour cells in the early stages of metastasis (Hida et al., 2022). Upregulation of HIF-1α in hypoxic conditions promotes increases in pro-angiogenic factors, namely, vascular endothelial growth factor (VEGF), known to be overexpressed in many lung cancers, causing an increase in tumour endothelial cell and tumour cell proliferation and angiogenesis (Wieleba et al., 2022; Frezzetti et al., 2017; Becker et al., 2023; Zhao Y. et al., 2022). Unlike traditional 2D cell cultures, VEGF gene expression was found to be regulated by HIF-1α in lung cancer 3D models, similar to what is observed in vivo (Onodera et al., 2023). Anti-VEGF therapies are emerging as one of the latest trends in targeting lung cancer (Frezzetti et al., 2017; Yang et al., 2017; Jeong et al., 2020; Seitlinger et al., 2022).
3.5 Oxygen, pH and nutrient gradients
A hallmark in all solid tumours is the decreased access of oxygen within deep cell layers due to the lack of neighbouring blood vessels (Hida et al., 2022). Oxygen is known to have a diffusion gradient of approximately 100–200 μm, causing an oxygen gradient in solid tumours including lung cancers. In NSCLC, intra-tumoural oxygen concentrations have been reported to be at around 2%, compared to 5.6% in normal lung tissue. The ability of tumours to sustain hypoxia arises from a phenomenon known as the Warburg effect which induces a metabolic switch in cancer cells from oxidative phosphorylation to aerobic glycolysis (Pinto et al., 2020). This is driven by the upregulation of HIF-1α, promoting survival under hypoxic conditions leading to NSCLC aggressiveness and resistance to target therapy and chemotherapy (Liu C. et al., 2021; Ziółkowska-Suchanek, 2021; Lu et al., 2020). Hypoxic regions of NSCLC are known to be characterized by a unique immune-suppressing landscape (Zhang C. et al., 2021). The potential of hypoxia-activated prodrugs for lung cancer therapy is currently being investigated (Li et al., 2021b; Singleton et al., 2021).
Solid tumours are additionally characterized by nutrient and metabolic waste gradients. The conversion of glucose to lactate causes lactic acid release, with a notable accumulation within tumours. The increase in intra-tumoural lactic acid is accompanied by an increased export to the TME via monocarboxylate transporters. This drop in pH in the TME and within solid tumours causes immune cell-activated oncogenic properties, including angiogenesis and drug resistance, while inhibiting drug permeability by ion trapping (Li and Qiao, 2022; Gao et al., 2022). Furthermore, the distances of cells from blood and lymph vessels gives rise to nutrient gradients, inducing distinct cellular metabolic profiles and increased immunosuppression (García-Cañaveras et al., 2019; Singleton et al., 2021).
4 In vitro lung cancer models used for pharmacological studies
From the development of the first cell line in 1951, the popularity of in vitro cell-based studies has dramatically increased and multiple cell lines have become commercially available, together with their clinical and molecular classifications (Scherer et al., 1953). Although these have provided the basis for in vitro laboratory studies on the molecular pathology and pharmacology of these cancers, 2D culture models have often fallen short of providing a suitable representative model of an in vivo tumour. Consequently, the use of 2D cultures suffers from limitations to translational relevance, known to be a major cause of the low success rate of clinical trials (Huo et al., 2020).
Lung cancer 3D culture models develop other key features which replicate those found within the TME which have already been well reviewed. These include similar cell-cell and cell-ECM contacts through the expression of cadherins, ECM formation, intra-tumoural gradients and cell layer organization. At diameters greater than 400 μm 3D cancer models develop necrotic, quiescent and proliferative cell layers, replicating the in vivo scenario (Pinto et al., 2020; Rozenberg et al., 2021; Shie et al., 2023; Hamilton and Rath, 2019). More complex models can simulate tumour vascularization and perfusion across blood vessels, air exposure and biophysiological characteristics.
3D cultures have been found to better reflect in vivo gene expression profiles, promoting more correct interpretations of results in comparison to monolayer cultures (Tellez-Gabriel et al., 2018). Therefore, the integration of 3D models in drug screening is essential to validate findings from 2D cultures and accurately assess the effects of anti-cancer drugs (Garnique and Machado-Santelli, 2023). Such approaches have proven to be fruitful for cancer drug screening and are emerging as a pivotal tool in shaping the future of lung cancer research and therapeutic development. Additionally, these culture models have a central role in the principle of the “3Rs” as they support the reduction, refinement and replacement of animal models with systems which could potentially better replicate human diseases and pharmacological responses (Lee et al., 2021). A summary of the main types of 3D cultures used in lung cancer pharmacological research is presented in Figure 3.
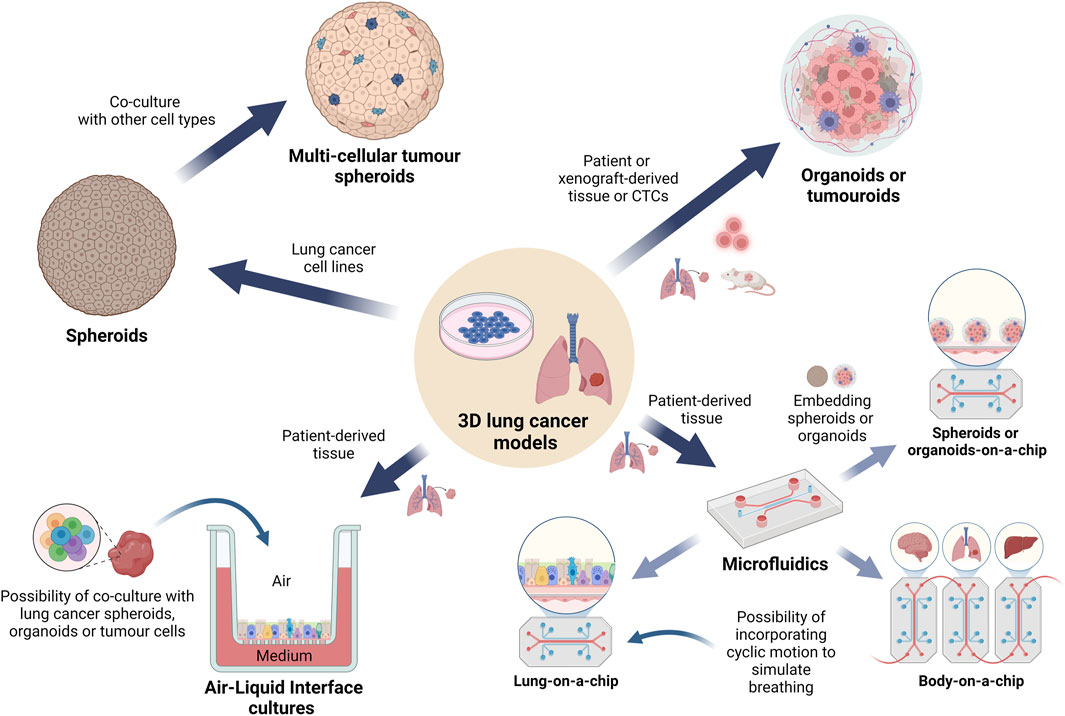
Figure 3. Overview of the 3D cell culture models currently used in lung cancer pharmacology research. A graphical representation depicting the main types of 3D lung cancer models which are being used for in vitro lung cancer pharmacological studies. Spheroids and organoids are the most common types of cell cultures, followed by tumouroids, while the more complex microfluidic models are used to study specific cellular interactions, angiogenesis or metastasis to other organs. These models may incorporate biomaterials generated via bioprinting technologies while maintaining streamlined workflows. Use of ALI conditions has gained increasing interest in simulating the lung environment for drug testing. (Abbreviations: ALI: air-liquid interface).
The objective of this review is to provide a comprehensive overview of the most recent 3D models and methodologies developed for pharmacological investigations aimed at targeting lung cancer, focusing on advancements made within the past 5 years. Additionally, it seeks to highlight potential research gaps to advance preclinical pharmacology of this highly deadly and heterogenous disease. The common types of 3D cell culture models used to study lung cancer pharmacology are summarized in Table 1.
4.1 Lung cancer spheroids
Currently, the term “spheroid” is being used to refer to in vitro 3D cultures derived from commercialized cell lines, although a significant overlap between spheroids and organoids exists (Wieleba et al., 2022). The lung cancer spheroid model offers a versatile platform for studying various aspects of the disease. It accurately replicates key features of patient tumours, including cell composition, hypoxic conditions, extracellular matrix deposition, and immunosuppressive microenvironments. This model enables researchers to explore molecular interactions within the tumour microenvironment, identify drug targets, and test potential therapeutics. Additionally, the spheroid model reflects the clonal heterogeneity observed in patient tumours, providing insights into therapy response and drug resistance mechanisms (Rozenberg et al., 2021). Overall, lung cancer spheroids have been instrumental in evaluating the efficacy of anticancer drugs, particularly in assessing drug penetration and resistance within the 3D TME. Moreover, heterotypic spheroids have further enhanced their utility in studying cell interactions and the influence of the TME on therapeutic responses with the aim of developing improved treatment strategies. Lung cancer spheroids are relatively simple and reproducible tumour-mimicking in vitro models for screening of novel drugs, including immunotherapies (Boucherit et al., 2020).
4.1.1 Homotypic lung cancer spheroids
Spheroids were generated from A549 cells by seeding in 1.5% agarose-coated 96-well plates for 3 days in supplemented high glucose DMEM. 2D cultures and the 3D spheroids were treated with TTA-A2 (a T-type calcium channel antagonist) alone and in combination with paclitaxel (Kumari et al., 2020). Gendre et al. (2021) derived spheroids from three lung mesothelioma cell lines, H2052/484, H2452 and H2052, which were optimized by seeding at different seeding densities (1,000–100,000 cells/spheroid) using 1.5% agarose-coated 96-well plates. Spheroid growth kinetics (diameter) were monitored via brightfield microscopy over 3 days in culture. Following the selection of the 1,000 cells/spheroid density, each growth kinetics of each model was characterized over 28 days in conjunction with a luminescence-based assay to assess intracellular ATP as a measure of cell viability. A549, H460 and H520 NSCLC cell line spheroids have been developed using the agarose micro-mold technique and characterized for pharmacological research. As a proof-of-concept study, spheroids of these NSCLC cell lines were treated with AZD 2014 (a dual mTOR inhibitor) for up to 6 days using concentrations based on IC30 values observed in 2D. AZD2014 was shown to inhibit spheroid growth, shedding light on the increased complexity of these models compared to 2D cultures (Vella et al., 2024).
Spheroids of H1048, H1882, H1876 and DMS53 SCLC cell lines were cultured using the hanging drop method or in ultra-low adhesion (ULA) plates in CSC medium. spheroids were treated with PFK158, a glycolytic inhibitor, and combinations with different chemotherapies to study cytotoxicity (Thirusangu et al., 2022). CSC-enriched spheroids from three lung cancer cell lines, H460, H23 and A549, were formed by culturing in ULA plates for 7 days in glutamine-supplemented DMEM or RPMI medium while lowering the FBS percentage down to 1%. Subsequently, spheroids were dissociated with EDTA and re-seeded into ULA plates for another 14 days. These models were used to study the effects of jorunnamycin A treatment on stem-cell like properties and the resulting chemosensitivity of these spheroids (Sumkhemthong et al., 2021). Vega et al. (2023) developed a novel angle plate adaptor technology for spheroid generation, found to provide similar spheroid formation results to ULA plates. NSCLC spheroids were generated from ten cell lines using this technique and then used to screen 1,280 natural products, resulting in 128 top hits, to potentially identify anti-cancer compounds. A549 transduced with infra-red fluorescent protein (A549-iRFP) have been cultured as spheroids using ULA plates and injected into mice pleura to image tumours in vivo for growth analysis. Interestingly, these xenografts progressed through all the four clinical stages of NSCLC (Huang et al., 2019; Huang et al., 2020). In the same year, Li et al. (2020) presented a large-scale CRISPR screening study on lung cancer spheroids in order to identify oncogenes, tumour suppressor genes and novel anti-cancer compounds, while elucidating the increased biomimicry of 3D spheroids over 2D cultures. In another study published in 2020, spheroids generated from several lung cancer cell lines have been used to identify novel potential therapeutic targets with the aim of developing a high-throughput screening methodology. Genome-wide CRISPR screens were conducted on 3D lung spheroids in parallel with lung cells cultured in 2D and mouse xenografts. Comparing the H23 cells cultured in 2D and those cultured as spheroids to the xenografts, the spheroids were found to more accurately identify growth-related cancer vulnerabilities due to the greater ability of spheroids to mimic tumour biology of the mouse xenografts (Han et al., 2020). Furthermore, lung cancer spheroids of a lung adenosquamous carcinoma cell line, H125, were developed using electrospun poly (ε-caprolactone)-based scaffolds of nanometric and micrometric sizes (González-Martínez et al., 2020).
4.1.2 Lung multi-cellular tumour spheroids
Lung multi-cellular tumour spheroids (MCTS) allow for the co-culturing of several cell types to simulate interactions and cross-talks between different cell types and ultimately improve the TME biomimicry of these in vitro 3D models.
A NSCLC MCTS model was developed using A549 adenocarcinoma cells co-cultured with BEAS-2B normal human bronchial epithelial cells in agarose gel micro-well molds. These MCTS models were used to study tumour cell migration in different matrices while developing an efficient system for investigation by utilizing a specialized real-time cell analysis system. Through this MCTS model, the early onset of cell migration is exhibited, beginning after only 6 h, highlighting the importance of immediate administration of adjuvant therapy targeting migration. Additionally, this study sheds light on the possibility of the automation of cell migration data analysis (Shabalina et al., 2021). Moreover, A549 spheroids formed on agar micro-well molds were used to study cytotoxicity of propolis and propolis-loaded niosomes (Ilhan-Ayisigi et al., 2020). Spheroids of H358 and A549 cell lines co-cultured with WI-38 human lung fibroblasts were developed using 200 μm agarose gel micro-well molds for drug resistance testing (Luan et al., 2024).
Kaur et al. (2021) developed heterotypic spheroids consisting of 60% HCC827 lung adenocarcinoma cells, 25% human umbilical vein endothelial cells (HUVEC) and 15% mesenchymal stem cells in ULA plates. These cell line-derived lung cancer spheroids together with patient tissues were used to compare treatment responses of four EGFR TKIs with respective 2D cultures. Hulo et al. (2024) generated MCTS models by co-culturing ADCA117, H1975 and H1437 NSCLC cell lines with a fibroblast cell line, human foreskin fibroblast-2 (HFF-2), and magnetically-isolated monocytes from blood donors. Following the generation of MCTS cultures over 3 days using ULA plates, spheroids were treated with combinations of three chemotherapies in clinically similar dosing schemes. These 3D cultures were used to investigate chemotherapy combinations with the aim of identifying enhanced combinational treatments, improving second line therapy and reducing cancer relapse. A 3D lung tumour spheroid platform for oncoimmunology assays was developed using the H1650 adenocarcinoma cell line co-cultured with immune cells and fibroblasts and seeded in ULA plates. These served to provide relevant and easy-to-use models for studying tumour-stroma organization, T cell motility, and immune checkpoint blockade regimens. Additionally, this spheroid model was utilized to assess cytotoxic T lymphocyte (CTL)-mediated killing of tumour cells. By introducing CTLs into the spheroids, the authors were able to monitor target cell-specific killing over time using flow cytometry and live cell imaging. This aspect of the model provides a valuable tool for evaluating the efficacy of CTL-based immunotherapies and investigating mechanisms of resistance to CTL-mediated killing (De Ridder et al., 2022). Using a similar method of generation, A549 MCTS models were developed and treated with chemotherapies between one and 5 days post-seeding. Interestingly, to simulate pharmacokinetics, medium was changed accordingly to mimic the drug blood half-life (Pei et al., 2020).
4.2 Lung cancer organoids and tumouroids
Lung organoids are self-organized 3D cultures derived from patient lung tumour tissue, lung stem cells or mesenchymal stromal cells which can replicate tumour or organ tissue by maintaining several characteristics observed in vivo (Duzagac et al., 2021; Wieleba et al., 2022; Zhao Z. et al., 2022; Zhu et al., 2023). While 3D models based on cell lines are limited in terms of their biomimicry ability, organoids can replicate tumour or organ structure and heterogeneity, simulating therapeutic responses (Fűr et al., 2024). The first documentation of lung organoids dates back to 1981, while the development of lung cancer organoids (LCOs) for therapeutic screening was first reported in 2019 (Evans and Kaufman, 1981; Kim et al., 2019; Zhu et al., 2023). LCOs are clinically relevant 3D cell cultures, shown to effectively model lung tumours for improved therapeutic investigation and development of precision medicine for specific genetic mutations, potentially minimizing drug failure in the clinical stage and reducing the use of animal models (Lee et al., 2021). Following the first publication of human LCOs, research on LCOs has been drastically increasing since 2016 (Endo et al., 2013; Fűr et al., 2024). The use of LCOs for pharmacological research has gained increasing interest especially over the last 5 years with the number of Pubmed®-indexed publications in 2023 exceeding 150 specifically related articles, approximately half of which involved therapeutic studies.
When culturing LCOs, each laboratory uses different culture media with different growth factors and inhibitor formulations and varying culture protocols. Currently, it is unclear whether or how these differences affect therapeutic responses in vitro. Most LCOs are cultured in a supporting ECM-like material, such as Matrigel or collagen, or otherwise using spinning bioreactors and the air-liquid interface (ALI) method, as discussed in other sections of this review (Huo et al., 2020; Duzagac et al., 2021). Ma et al. (2022) have summarized the latest techniques and growth factors used for culturing LCOs. Currently, partly due to the lack of protocol standardization, LCOs tend to have highly variable success rates ranging from 7% to 87% and risk the overgrowth of normal lung cells. Simpler LCO models are relatively cost-effective, while the more advanced organoid models in combination with animal models, microfluidics and other innovative models tend to be more costly (Kim et al., 2020). Studies involving single-cell RNA sequencing of LCOs have been suggested to better understand the cellular heterogeneity and delve into their tumour-mimicry ability (Lee et al., 2021). A vast range of methodologies have been employed for LCO generation, each one modelling a unique set of characteristics. Figure 4 summarizes different types of lung cancer organoid and tumouroid models with their unique methods of derivation for pharmacological studies.
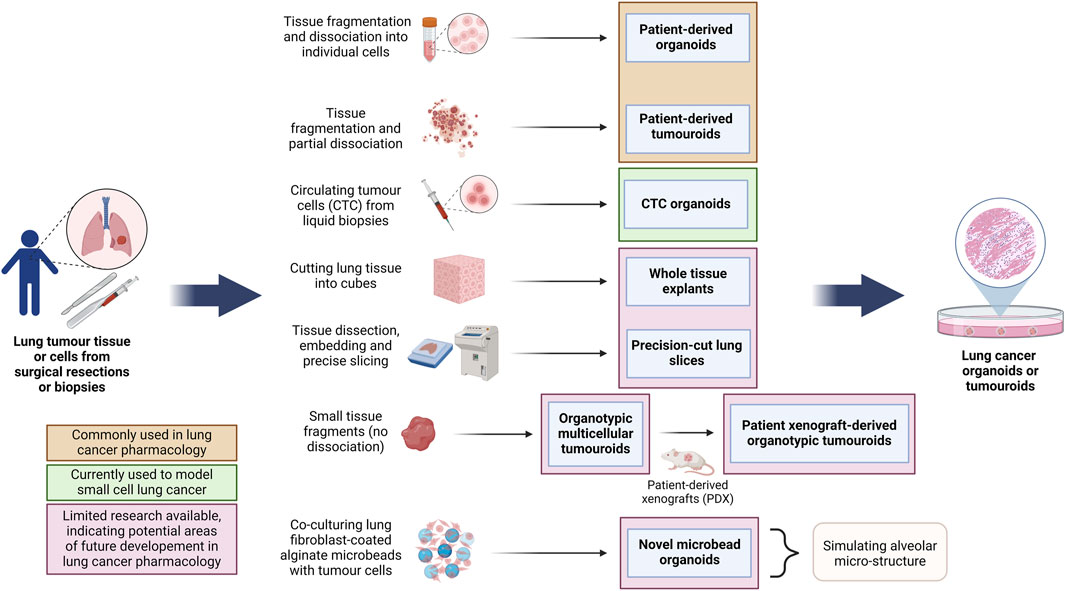
Figure 4. Overview of the methods for the generation of different lung cancer organoids and tumouroids. This diagram illustrates diverse methods employed in the generation of lung cancer organoids and tumouroids, including those derived from patient lung cancer tissue, CTCs from blood biopsies and a novel alveolar-simulating approach. Each method offers unique characteristics which model tumour heterogeneity necessary for accurate drug screening and the development of personalized therapeutics in lung cancer research. Lung cancer PDOs and PDTs have been frequently used in pharmacological studies, while other organoid and tumouroid models represent promising avenues for ongoing advancements in the field. (Abbreviations: CTC: circulating tumour cells, PDO: patient-derived organoids, PDT: patient-derived tumouroids).
Although not always clearly differentiated in literature, here we define the most well-understood differences between tumour organoids and tumouroids. The main differences between these two 3D culture models stems from their method of generation: lung cancer organoids are generated from dissociated tumour tissue, while lung tumouroids are formed from fragmented but only partly-dissociated tumour tissue (patient-derived tumouroids), tumour tissue fragments (organotypic multicellular tumouroids, patient xenograft-derived organotypic tumouroids and whole tissue explants) or tumour tissue slices (precision-cut lung slices). This results in different in vitro tissue microstructures; while organoids are re-generated from individual cells, the lack of complete tissue dissociation of tumouroids allows the latter to maintain the same complex cellular organization as the in vivo tumour from which that specific tumouroid was derived. This preseveration of the in vivo microanatomical structure gives tumouroids an advantage in TME studies and personalized medicine research. Table 2 outlines the main differences between these two types of lung cancer 3D models.
4.2.1 Lung cancer patient-derived organoids
Patient-derived organoids (PDO) are novel in vitro models cultured from patient tissues which have been shown to replicate the original tumour biology, including tissue structure, cellular heterogeneity, CSC-driven regeneration and genetic characteristics (Zeng et al., 2023). Numerous studies are showing how lung cancer PDOs are an ideal tool for pre-clinical drug screening and drug resistance studies with the ability of investigating patient-specific responses, paving the way for the discovery of personalized medicines which are critical for the treatment of this highly heterogenous disease (Kim et al., 2019; Wang et al., 2020; Li Y. et al., 2023; Li H. et al., 2023; Liu et al., 2023; Taverna et al., 2024).
A protocol for culturing of lung cancer PDOs was developed following surgical resection or biopsies of lung cancer tissues cultured in Matrigel with minimum basal medium (MBM). These PDOs were cultured for 6 months, over which organoid proliferation was evident from Ki-67 immunolabelling. After characterization, mouse xenografts were generated by the dissociation and transplantation of these organoids. These organoids, both in vitro and as xenografts, were then used to screen four anti-cancer drugs (Kim et al., 2019). NSCLC PDO models were generated by dissociation of patient tissue slices and plating tumour cells in Matrigel domes for in vitro drug testing (Shi et al., 2020). Choi et al. (2021) developed SCLC PDOs from dissociated tumour tissue biopsies suspended in Matrigel and cultured in supplemented DMEM/F12 which was optimized for long-term SCLC PDO culturing. Moreover, Banda et al. (2020) developed PDOs from homogenized lung adenocarcinoma tissue seeded in Matrigel-coated 24-well plates and characterized for up to 10 days in culture to investigate erlotinib treatment. Lung adenocarcinoma PDO models established by Kim S. K. et al. (2021) were used to predict clinical responses to target therapies and to target specific mutations for the identification of novel molecular targets. Lung tumour tissue was cut and dissociated with collagenase, followed by cell seeding in Matrigel in 48-well plates.
Lung cancer PDO models consisting of tumour cells co-cultured with WI-38 fibroblast cells have been developed with the use of agarose micro-well molds for drug efficacy studies. Co-culturing with fibroblasts was shown to increase resistance to a KRASG12C inhibitor (Luan et al., 2024). Additionally, Sulimanov et al. (2023) cultured PDOs using 96-well plates coated in agarose onto which tumour tissue mixed with a collagen solution was seeded and cultured in supplemented DMEM/F12. Using this culture model, the authors equated a mathematical model describing the interactions of different cell types in NSCLC tumours in order to predict cellular sub-population alterations over 7 days with the aim of translation to the clinic. Yokota et al. (2021) developed PDOs for therapeutic screening from lung adenocarcinoma, lung adenocarcinoma lymph node metastases and EGFR-resistant lung cancer malignant pleural effusion cells using basal membrane extract. The cell culture medium was optimized for cellular purity and long-term expansion (up to 13 months). In parallel, PDOs were cultured from adjacent normal lung tissue (Sachs et al., 2019).
Of interest, lung cancer PDOs in ALI conditions were used to model the lung immune checkpoint blockade for immunotherapy studies. These PDOs were shown to maintain immune cells and their receptors in vitro (Neal et al., 2018). Otherwise, the culturing of lung cancer spheroids or organoids in ALI conditions has been rarely reported.
4.2.2 Circulating tumour cell lung cancer organoids
The concept of using circulating tumour cells (CTCs) for LCO generation has been recently proposed as tools for disease modelling, drug screening and identification of personalized medicine (Yang et al., 2019). Considering the relative difficulty of obtaining SCLC patient tissues, and the ease of CTC collection through liquid biopsies and their abundance in SCLC patients (especially at the advanced stage), this LCO model may pave the way for novel pharmacological research employing SCLC organoids (Huo et al., 2020; Fűr et al., 2024). SCLC CTC LCOs cultured in a polydimethylsiloxane (PDMS)-based microfluidic device in co-culture with CAFs and immune cells have been shown to closely simulate genetic mutations of the primary tumour (Zhang et al., 2014; Tellez-Gabriel et al., 2018). Hamilton and Rath (2019) established nine SCLC CTC cell lines which spontaneously formed LCOs in culture. The authors discuss the role of modelling aggressive metastatic tumours from these cell lines for metastasis and chemoresistance studies. However, in vitro models of SCLC and CTC LCOs require further progress.
4.2.3 A novel organoid formation technique to simulate the alveolar micro-structure
Unique organoid models have been developed by making use of alginate microbeads coated in primary human lung fibroblasts and co-cultured with SCLC cell lines H526, H1963 and H82, forming organoids after 7 days. These were used to study the therapeutic effects, including relapse, of the chemotherapeutic compounds cisplatin and etoposide. Characterization revealed high similarity between these organoids and patient tumours, and were shown to better simulate in vivo SCLC tumours (Sen et al., 2023).
4.2.4 Patient-derived tumouroids
Patient-derived (or tissue-derived) tumouroids (or tumourospheres) (PDT) are generally formed by culturing of fragmented and partly-dissociated tumour tissue (Daunys et al., 2021).
Knelson et al. (2022) generated lung cancer PDT models to study the effects of an agonist of the activation of the stimulator of interferon genes (STING) pathway with the aim of activating innate anti-tumour immunity. Fresh tumour specimens were minced on ice and resuspended in supplemented DMEM, cultured in ULA plates or embedded in collagen (Jenkins et al., 2018). Additionally, PDTs were loaded onto a microfluidic device to generate biomimicry tumour-vascular models.
Other ex vivo spheroid generation protocols involved the processing of NSCLC tissue samples by mincing and enzymatic digestion followed by seeding in Matrigel or in 2.5% agarose gel 256-microwells. PDTs grown in Matrigel were used to study the effects of anti-PD-L1 immunotherapies (atezolizumab and avelumab) and an MEK targeted therapy (selumetinib), while those in agarose microwells were used for studying chemotherapeutic combinations 3 days after seeding (Della Corte et al., 2019; Mueggler et al., 2023). Lung adenocarcinoma primary tissues processed with or without enzymatic digestion were used to develop spheroids by seeding in 1.5% agarose-coated 96-well plates, cultured in complete RPMI. These spheroids were optimized as previously described in the study by Gendre et al. (2021). Di Liello et al. (2019), report the similarity of treatment responses in NSCLC patients and their respective ex vivo PDT models. Lung cancer tissue was dissected, enzymatically digested and seeded in Matrigel. PDTs were able to replicate chemotherapy and immunotherapy responses, while opening up on the possibility to predict patient outcomes and personalized medicine. Seitlinger et al. (2022) developed an in vitro vascularized NSCLC 3D model by cutting and enzymatically digesting patient-derived lung tumour tissue and co-culturing these tumour cells with fibroblasts and endothelial cells in ULA plates. Combined with a pre-vascularized fibrin matrix, the authors note the potential that these PDT models have for incorporation into a microfluidic device for improved anti-cancer drug screening (Lê et al., 2022).
4.2.5 Patient xenograft-derived organotypic tumouroids
Organotypic multicellular spheroids (OMS) are formed by culturing small fragments of tumour tissue without prior dissociation, favouring the maintenance of the in vivo tumour architecture. Ivanova et al. (2020) report the establishment of patient xenograft-derived organotypic tumouroids (PXDOT) from patient-derived exon 19 and exon 20 HER2-mutant NSCLC xenografts (PDX). These PDX tumours were processed and cultured in ULA plates for spheroid formation, followed by transferring spheroids to microfluidic devices and exposed to different drugs and drug combinations (Jenkins et al., 2018).
4.2.6 Lung cancer whole tissue explants
The lung whole tissue explants (WTE) method involves cutting lung tissue into cubes, providing a three-dimensional perspective similar to PCLS. While it may not fully retain the airway’s 3D structure, it has proven effective in studying various stimuli responses in lung tumours. Adapted WTE models were used to investigate human NSCLC chemotherapy and targeted therapy responses, demonstrating the model’s reliability and reproducibility (Karekla et al., 2017). Although WTE requires less preparation than PCLS, comparing different experimental conditions can be challenging due to potential variations in cell type ratios (Pomerenke, 2021). Evans et al. (2021) generated ex vivo models from 1 mm3 NSCLC WTE to demonstrate the advantage of using this model in therapeutic research due to their ability in sustaining an immunosuppressive environment which can mimic the TME better than spheroid co-culture models (Evans et al., 2021). Pharmacological research involving lung cancer WTE is evidently still very lacking.
4.2.7 Lung cancer precision-cut lung slices
Precision-cut lung slices (PCLS) are thin sections of lung tissue that are prepared using precise cutting techniques to maintain the structural and functional integrity of the tissue. These slices typically range from a few hundred micrometres to a few millimetres in thickness and are used as ex vivo models to study lung physiology and disease, including lung cancer pharmacology. Following tissue dissection, embedding in low-melting agarose and precise slicing with specialized equipment, such as a vibratome, these tissues are prepared for culturing. Human PCLS models from tumour samples could be valuable in studying tissue responses in preclinical models of anti-cancer therapy. This organotypic model can be used to simultaneously study various aspects of lung function, including airway reactivity, immune responses, drug metabolism, and toxicology (Alsafadi et al., 2020; Närhi et al., 2018).
Overall, PCLS provide a robust and reproducible model to study the effects of various stimuli in a controlled ex vivo environment. They offer flexibility in testing multiple conditions from the same donor and can be maintained viable for extended periods in vitro. Efforts are ongoing to validate PCLS as a model for drug toxicity testing in preclinical settings (Pomerenke, 2021). Interestingly, NSCLC PCLS ex vivo cultures were found to retain cellular organisation for up to 12 days in culture (Junk et al., 2021). However, Preuß et al. (2022), caution about the changes in cell populations over long-term PCSL culture. Currently, no studies have been published using PCLS models for lung cancer disease progression modelling, and it has been suggested that such studies take into consideration the cutting damage which progresses during cultivation (Alsafadi et al., 2020; Preuß et al., 2022). Alsafadi et al. (2020) discuss the possible applications of PCLS in lung cancer pharmacology, including novel drug discovery and delivery, cancer cell resistance and personalized medicine. However, pharmacological research using models of PCLS from human lung cancers is still lacking and requires a better understanding in order to take advantage of its TME-replicating potential in preclinical lung cancer pharmacology.
4.3 Microfluidics
Microfluidic devices comprise a broad category of micro-fabricated devices designed for 3D cell culture technology. They are commonly referred to as “organ-on-a-chip” or “lab-on-a-chip” systems, and consist of tiny channels and chambers etched into materials such as PDMS or plastics. Cells are cultured in the chamber areas while fluids are pumped at precisely controlled rates through the channels, simulating a circulatory system. The lung-on-a-chip model, conceived over 15 years ago (Huh et al., 2010; Huh, 2015; Francis et al., 2022) has gone through several iterations of improvement and presents a formidable approach towards designing a TME over which a liquid containing nutrients, oxygen, drugs, assay compounds and even other cells, can flow at finely controlled rates. Besides addressing up-scalability and throughput issues, lung-on-a-chip models also allow for the study of the drug effects on lung cancer metastasis.
The mechanical breathing motion occuring within the lungs is thought to alter cellular behaviour leading to changes related to several hallmarks of lung cancer, including progression, angiogenesis and metastasis. Breathing lungs-on-a-chip have been designed to model lung disease and progression and to study drug responses in lung pathologies including lung cancer, using systems design to simulate the biomechanical TME of breathing lungs. This technology involves the use flexible material combined with cyclic motion usually by applying negative pressure via vacuum chambers, simulating the physiological movement of lung alveoli. As in other microfluidic devices, these lung cancer models have been co-cultured with several other cell types such as endothelial cells and fibroblasts (Sontheimer-Phelps et al., 2019; Barros et al., 2021; Das et al., 2022). The growing interest in these multi-dynamic models has the potential to significantly enhance cell culture biomimicry, driving advancements in drug development and personalized medicine research for lung cancer (Shrestha et al., 2020; Francis et al., 2022).
A multi-organ microarray containing three layers of chambers that mimicked the invasive microenvironment of lung cancer, has been used to assess the mechanism of EMT in lung cancer cells which invaded distant tissues and organs, such as the brain, bone, and liver (Xu et al., 2016). This body-on-a-chip multi-organ model was further developed by the same research team, in 2020 (Xu et al., 2020), to construct a microarray model of lung cancer brain metastases to specifically investigate the mechanisms underlying metastatic resistance to chemotherapeutic drugs. One year later, Zheng and co-workers reported a microarray lung cancer model designed to study liver metastasis in hypoxic conditions, focusing on the therapeutic effects of HIF-1 inhibitors on invasion (Zheng et al., 2021; Zhu et al., 2023). More recently, more complex body-on-a-chip models incorporating a broader range of body tissues, and novel micro-biosensors have been developed for lung cancer studies (Jalili-Firoozinezhad et al., 2021; Ding et al., 2021), and the data outputs suggests such models to be a formidable evolving technology with strong applications both in industrial R&D as well as academic research environments.
Termed as the quantum leap in cancer research, efforts are being made to integrate lung cancer spheroids and organoids into microfluidic set-ups (Duzagac et al., 2021; Wu Y. et al., 2021; Carvalho et al., 2022). A549 spheroids were developed using purposely designed microfluidic devices which allow for the co-culturing of HUVEC cells in a spatial arrangement over collagen-embedded spheroids. Hence, the microfluidic channel through which medium passes is lined by a layer of endothelial cells forming biomimicry blood vessels proximal to the A549 spheroids embedded in an ECM-like material. This serves as an ideal model for studying interactions between tumour and endothelial cells, angiogenesis and invasion and how these are affected by drug treatments (Lee et al., 2019). Another drug-screening model was developed by generating triple co-culture spheroids consisting of A549 cells, human lung fibroblasts and HUVECs followed by their incorporation into a microfluidic device with a pro-angiogenic porcine lung decellularized ECM-based hydrogel and a vascularized system (Park et al., 2021; Park et al., 2023). Wan et al. (2023) describe a protocol to enhance vascularization in lung cancer spheroids-on-a-chip by introducing lung fibroblasts after spheroid formation and demonstrate the improved immune responses using this novel model. In this study, spheroids of a mesenchymal derivative of the H69 SCLC cell line (H69M) were co-cultured with fibroblasts, endothelial cells and pericytes in ULA plates and transferred to a PDMS-based microfluidic device having a central gel channel surrounded by two media channels simulating blood vessels. In 2019, a PDMS-based microfluidic device was designed to replicate lung micro-physiology and support the formation of low size variation lung cancer PDOs for drug testing using cells seeded in Matrigel. This is reported to be the first SCLC PDO model for pharmacological research (Jung et al., 2019).
Recent developments in organoid-on-a-chip models have managed to recapitulate lung tumour vascularization, enhancing drug delivery and drug screening research (Kim S. K. et al., 2021; Wu Y. et al., 2021). Organoids-on-a-chip enable high-throughput drug screening, automatic treatment schedules and allow simultaneous monitoring of drug reactivity parameters while highly simulating the TME (Zeng et al., 2023).
4.4 Bioprinting
Cell bioprinting represents an emerging transformative approach in lung cancer research, offering the potential to recreate complex tissue architectures in vitro with a high degree of precision. This innovative technology has the capability to fabricate 3D structures by sequential deposition of bioinks - materials that contain living cells and biomaterials - therefore forming functional living tissues.
In lung cancer research, bioprinting is showing increasing applications, such as the generation of tumour-stroma constructs by co-printing cancer cells with stromal elements, such as fibroblasts and ECM components. This provides a dynamic system to investigate the interactions between cancer cells and the ECM, which are crucial for understanding cancer progression and the impact of the TME (Herreros-Pomares et al., 2021; Falcones et al., 2021). Bioprinted lung models can be leveraged for drug screening and toxicity testing and can potentially contribute to personalized medicine in lung cancer treatment by enabling the production of patient-specific tumour models using the patient’s own cells.
Despite the promising applications, some challenges remain, such as replicating the full complexity of lung tissue, including the vascularization needed for nutrient and oxygen supply to the deeper cell layers, as well as ensuring long-term viability and functionality of the bioprinted constructs. The high financial investments and running costs required for a bioprinting facility also need to be considered and justified, in view of other 3D lung models which are cheaper to generate.
4.5 Decellularized lung matrices
3D lung cancer models supported by decellularized ECM (dECM) mimic important elements of the ECM and cell-ECM interactions. The unique molecular composition and structure of these bioactive scaffolds can significantly improve pre-clinical pharmacological research by taking into account tumour physiological parameters which are frequently overlooked (Hoshiba, 2019; Ferreira et al., 2020; Ferreira et al., 2021; Marques-Magalhães et al., 2022).
Shie et al. (2023) aimed to develop a biomimetic spheroid model derived from HCC827 lung adenocarcinoma cells to study drug responses to several EGFR TKIs. These lung cancer cells were co-cultured with THP-1 (leukaemia monocytes), human pulmonary fibroblasts and HUVECs. Cells were mixed with dECM extracted from bovine lungs and seeded as droplets in 96-well plates via an automated printing needle (5,000 cells/spheroid). A solution of dECM without cells was added to cover the droplets and once gelation was ready, culture medium with HUVECs were added. After 5 days, diameters of most of the spheroids ranged between 300 and 400 μm, allowing for cell layer organization and necrotic core formation. To validate these models for pharmacological research, cytotoxicity with several EGFR TKIs was evaluated in dECM-embedded spheroids of HCC827, H1650, H3255, and GR10 lung cancer cell lines. Two and 5 days post-treatment, cell viability was analysed by live/dead staining using Calcein AM/Ethidium homodimer-1 (EthD-1) and confocal microscopy. In addition, HCC827 models were treated with chemotherapeutic combinations to analyse drug responses (Shie et al., 2023). As previously discussed, the use of lung dECM has been employed in a spheroid-on-a-chip microfluidic device to study angiogenesis (Park et al., 2021; Park et al., 2023).
The ability of such bioactive scaffolds to recapitulate complex TME interactions, brings to light the possibility of utilizing lung tumour dECM based on the stage and location of the lung cancer model being investigated, or using dECM surrounding the same tumour tissue used in culture. Further exploration into the construction of 3D lung cancer models based on lung tumour dECM is highly encouraged due to its potential to significantly improve the advancement of anti-cancer strategies and precision medicine.
4.6 Air-liquid interface
Air-liquid interface (ALI) culturing provides a method of culturing airway epithelial cells, in such a way that they are exposed to the air while still absorbing nutrients from a tissue culture medium. Such cultures are prepared by seeding and culturing airway epithelial cells onto micro-well inserts composed of a collagen-coated polyethylene terephthalate (PET) porous membrane. The apical surface of the cells is exposed to the air, while they absorb nutrients from the tissue culture medium, through the basal side which is adhered to the insert. This mode of culture enables the development of a ciliated morphology and tight junctions similar to that observed in vivo, giving an overall pseudostratified epithelium appearance and better recapitulation of the structural architecture and differentiated functions of the respiratory epithelium (Figure 5). This leads to a more accurate representation of cellular and molecular changes associated with lung cancer (Agraval et al., 2022).
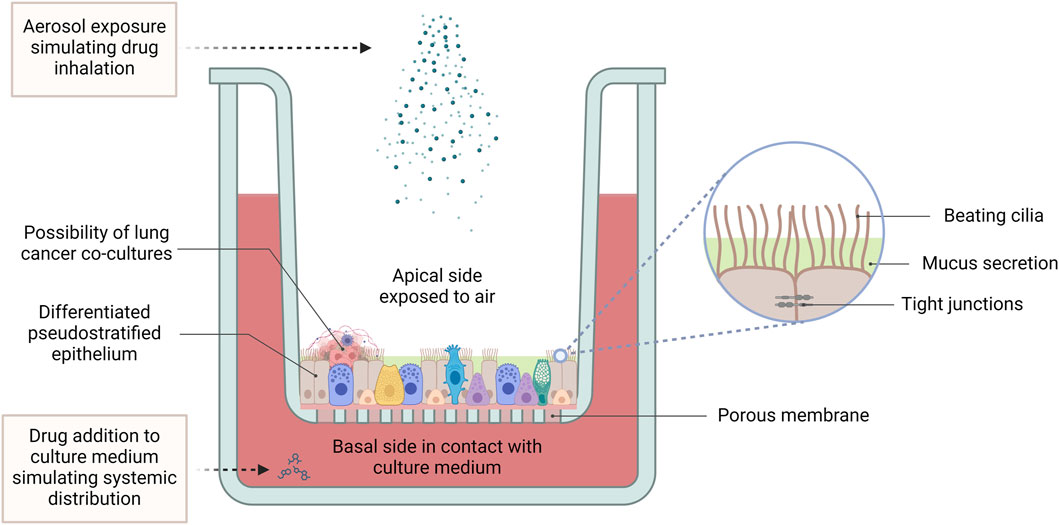
Figure 5. The air-liquid interface culture set-up. In ALI conditions, primary lung cells differentiate into a pseudostratified pulmonary epithelium, featuring beating cilia, mucus production, and tight junctions between epithelial cells. While harbouring the possibility of co-culturing with lung tumour cells, this advanced 3D model provides a physiologically relevant platform for pharmacological studies, facilitating the investigation of lung cancer pathogenesis, drug responses and therapeutic efficacy. ALI cultures allow for application of treatment to the apical side using aerosolized drugs to simulate inhalation therapy, while treatment applied to the culture medium mimics systemic drug distribution. (Abbreviations: ALI: air-liquid interface).
Indeed, Geles and co-workers demonstrated that NSCLC primary cells cultured using the ALI model allows for the examination of gene networks and differentiation potential in a 3D growth environment that mimics the in vivo lung environment. Using this model, the researchers also showed that poorly differentiated cells overexpress RNA processing factors and exhibit a distinct profile of alternatively spliced mRNAs, compared to well differentiated NSCLC cells, suggesting that alternative post-transcriptional mechanisms may be occurring in poorly differentiated lung cancer cells (Geles et al., 2016). More recently, Zhao et al. (2024) used an ALI model generated using tumour tissues from patients with stage IA NSCLC and used it to show that in the presence of low concentrations of interferon-γ, upregulation of the IDO1 gene occurred. This enhanced the viability of cancer cells, while inhibiting the viability of T cells and NK cells, therefore establishing an immune microenvironment conducive to tumour progression.
Another application for which lung cancer ALIs have been used, was to simulate lung inhalation by spraying gene drugs onto the ALI apical surface, in order to assess the effectiveness of administering gene therapy via the inhalation route. This method allowed for the assessment of the effects of inhalable gene transfection on cell proliferation and gene expression under conditions that are more representative of the actual lung environment (Ichikawa et al., 2022).
5 Pharmacological responses in 3D vs. 2D models of lung cancer
5.1 Drug responses in spheroids
HCC827 spheroids treated with erlotinib, afatinib or lapatinib for 96 h exhibited higher sensitivity than the 2D cultures. Furthermore, spheroids were more sensitive to osimertinib than the respective 2D cultures (Kaur et al., 2021). A comparative study using A549 2D cultures and 3D spheroids treated with TTA-A2 monotherapy or in combination with paclitaxel was conducted by Kumari and colleagues. IC50 was reached at 50 nM in 2D A549 cultures, while A549 spheroids exhibited an IC50 of 100 nM. The combinational therapy seemed to be more effectively recapitulated in spheroids, rather than the 2D cultures (Kumari et al., 2020). Other A549 spheroids generated on agar gel micro-wells were treated with propolis for three and 7 days. Fluorescence staining and imaging showed minor cytotoxicity and a slight increase in cell scatter with propolis treatments (Ilhan-Ayisigi et al., 2020). Spheroids generated from wild-type HCC78 cell line and three HCC78 ROS1-mutants, exhibited different responses to five TKIs: lorlatinib, entrectinib, crizotinib, repotrectinib and ceritinib. Interestingly, these differences in therapeutic responses were not observed in treated 2D cultures (Terrones et al., 2024). Three days post-seeding H2052/484 lung mesothelioma cells (1,000 cells/spheroid), spheroids formed were treated with cisplatin (50, 100, or 200 μM) or cisplatin/pemetrexed combination (50/200 μM, 100/400 μM or 200/800 μM) for 3 h, then washed and cultured for 17 days. IC50 was estimated by an ATP-based luminescent assay 24 h post-treatment: cisplatin = 1.21 μM; cisplatin/pemetrexed = 3.18/12.72 μM (Gendre et al., 2021).
H460, H23 and A549 spheroids generated in ULA plates exhibited lower IC50 values compared to normal bronchial cell line, BEAS-2B, spheroids upon treatment with non-toxic concentrations of jorunnamycin A (0.05–0.5 µM) over 7 days. Cytotoxicity was measured using annexin V-fluorescein isothiocyanate (FITC)/propidium iodide (PI) flow cytometry analysis. Spheroid sizes with treatments were analysed and were reduced at a time and dose-dependent manner. Additionally, jorunnamycin A (0.5 µM) was found to sensitize H460 spheroids to cisplatin treatment (25 µM) (Sumkhemthong et al., 2021). ULA-generated A549 MCTS cultures exhibited higher IC50 values in the 3D compared to 2D cultures when treated with cisplatin (20.71 μM vs. 9.73 μM), carboplatin (188.90 μM vs. 131.80 μM), doxorubicin (6.57 μM vs. 0.61 μM) and gemcitabine (>250 μM vs. 0.027 μM) for 72 h (Pei et al., 2020).
SCLC spheroids generated from H1048, H1882, H1876 and DMS53 cells were treated with PFK158 (1, 2.5 and 5 μM) 7 days post-seeding (Mondal et al., 2019). After 24 h post-treatment, an overall decrease in IC50 values was observed in treated spheroids compared to the 2D cultures of the same cell lines. Numbers and sizes of spheroids decreased with treatment, while invasion was inhibited with 5 μM PFK158. In combination studies, PFK158 was found to increase the sensitivity to several conventional chemotherapeutic drugs. Cell viability was assessed via trypan blue dye exclusion assay and spheroid diameters were monitored via inverted microscopy (Thirusangu et al., 2022).
5.2 Drug responses in organoids and tumouroids
Lung cancer PDOs developed by Kim et al. (2019) were used for therapeutic screening of docetaxel (IC50 = 0.08 μM), olaparib (IC50 = 69 μM), erlotinib (IC50 > 100 μM) and crizotinib (IC50 = 3 μM), obtaining IC50 values via a luminescence-based ATP assay after 6 days of treatment. Immunoblotting of lysed PDOs with EGFR and c-MET-related proteins showed changes in expression with erlotinib (1 μM) and crizotinib (1 μM) after 24 h, 48 h and 72 h. In another study, NSCLC PDO models were used to study the targeted therapies trametinib and selumetinib (MEK inhibitors), afatinib (EGFR inhibitor), BKM120 (PI3K inhibitor) and BGJ398 (FGFR inhibitor) targeted therapies at 0.01–10 μM concentrations for 96 h. Cell viability was measured via ATP assays. IC50 values obtained were dependent on the types of mutations present in each PDO model. However, combinations of BGJ398 with BKM120 exhibited some synergistic effects, while BGJ398 with trametinib exhibited strong synergism (Shi et al., 2020). Lung adenocarcinoma PDO models were seeded with four concentrations of erlotinib (0.01, 0.1, 1, or 10 μM) for 2 weeks, followed by treatment with 10 μM for 24 weeks (with several passages and re-platings), always changing the medium every two to 3 days. Confocal and brightfield images were collected over the course of treatment to study morphological characteristics. Moreover, DNA was isolated from the organoids followed by multiplex PCR for mutational analyses (Banda et al., 2020). As reported by Kaur et al. (2021), a PDO model treated with lapatinib exhibited a lower IC50 value than monolayers. In other models, therapy responses of 3D lung adenocarcinoma PDOs and their 2D counterparts were compared after three-day treatments resulting in different responses with different treatments and according to different tumour mutations present in the tumour tissue used. PDOs were treated for up to 15 days and cell viability was measured using an ATP-based luminescent assay (Kim S. Y. et al., 2021). Mueggler et al. (2023) used the agarose micro-well-generated lung adenoid cystic carcinoma PDTs to study therapeutic responses of seven concentrations of cisplatin/etoposide (6.25/4.2–400/270 μM) and cisplatin/paclitaxel (6.25/5–400/320 μM) combinations for 18 days. An ATP-based assay was used to study cell viability, while PDT diameters were monitored via imaging. IC50 was reached at 84/57 µM for cisplatin/etoposide and 103/82 µM for cisplatin/paclitaxel and PDT diameters exhibited a dose-dependent decrease in size.
SCLC PDO models were used for studying the pharmacological effects of cisplatin and etoposide treatment was studied using these SCLC PDO models. 200 single cells were mixed with Matrigel and suspended into 96-well plates. After 2 days, PDOs were treated with cisplatin and etoposide at 0.01, 0.1, 1, 2, 5, or 10 μM for 8 days. Cell viability was assessed by measuring ATP content, attaining IC50 values at 12.39 μM and 0.5382 μM, respectively. Tumour recurrence was studied by withdrawing treatment after 10 days and replacing with fresh medium. SCLC PDOs were able to re-grow to their original size after 2 weeks of 10 μM cisplatin treatment. Cells within the organoids were able to resist 50 μM cisplatin treatment and were shown to grow after 4 weeks of cisplatin withdrawal (Choi et al., 2021). Other SCLC PDOs embedded in a microfluidic device (diameter ˂ 200 μm) were used to test responses to cisplatin (20–80 μM) and etoposide (10–40 μM) over 72 h of treatment. This induced apoptosis which was assessed by staining with Annexin V-FITC/PI and caspase-3 with 4′,6-diamidino-2-phenylindole (DAPI) counterstaining. Cleaved caspase-3 anti-body was used to assess cellular apoptosis with immunofluorescence microscopy. Interestingly, PDO centres were found to survive even at the high concentrations, regardless of the small sizes of the organoids (Jung et al., 2019).
Combinational therapy of MAPK (NVP-BEZ235/dactolisib; 0.5 and 1 μM), PI3K/mTOR (AZD6244/selumetinib; 0.5 μM) and the SRC (saracatinib; 1 μM) inhibitors has been conducted on lung cancer PCLS models. Following 24-h treatments, alterations in signalling pathways and necrotic cell populations were assessed by immunohistochemistry (IHC) and biomarker analysis (Alsafadi et al., 2020; Närhi et al., 2018).
HER2-mutant microfluidics-embedded PXDOT models were used to study therapeutic responses to gefitinib, afatinib and neratinib (all at 0.5 μM) over 96 h using dual immunofluorescent staining to identify live/dead tumour cells. Combinations of neratinib with AZD8055 (1 μM) and trastuzumab (10 μg/mL) showed that the combination of AZD8055 and trastuzumab was ineffective at reducing tumour cell viability. However, trastuzumab and neratinib led to 50% loss of viability, while the combination of AZD8055 (35% decrease) and neratinib (20% decrease) exhibited enhanced therapeutic effects compared to the individual treatments (60% decrease).
5.3 Drug responses in static versus breathing lungs-on-a-chip
Hassell et al. (2018) developed a breathing alveolus lung-on-a-chip involving primary normal lung cells co-cultured with H1975 adenocarcinoma NSCLC cells which were GFP-transfected for microscopic visualisation. When comparing static versus breathing chips, cancer cell growth and invasion decreased significantly with biomimetic breathing. In this study, the presence of cyclic breathing was shown to increase resistance to EGFR therapies, exhibiting almost complete resistance to both 100 and 1,000 nM of rocicletinib over 6 days of treatment. This study suggests that TKI drug responses of such biomechanical orthotopic breathing lung-on-a-chip models parallel drug responses observed in vivo.
6 Common pharmacological assays applicable to lung cancer 3D models
Pharmacological research using 3D cell culture models requires the assessment of a spectrum of parameters arising from the increased complexity of these tumour models. The common laboratory-based assays used for pharmacological studies are described below and summarized in Table 3.
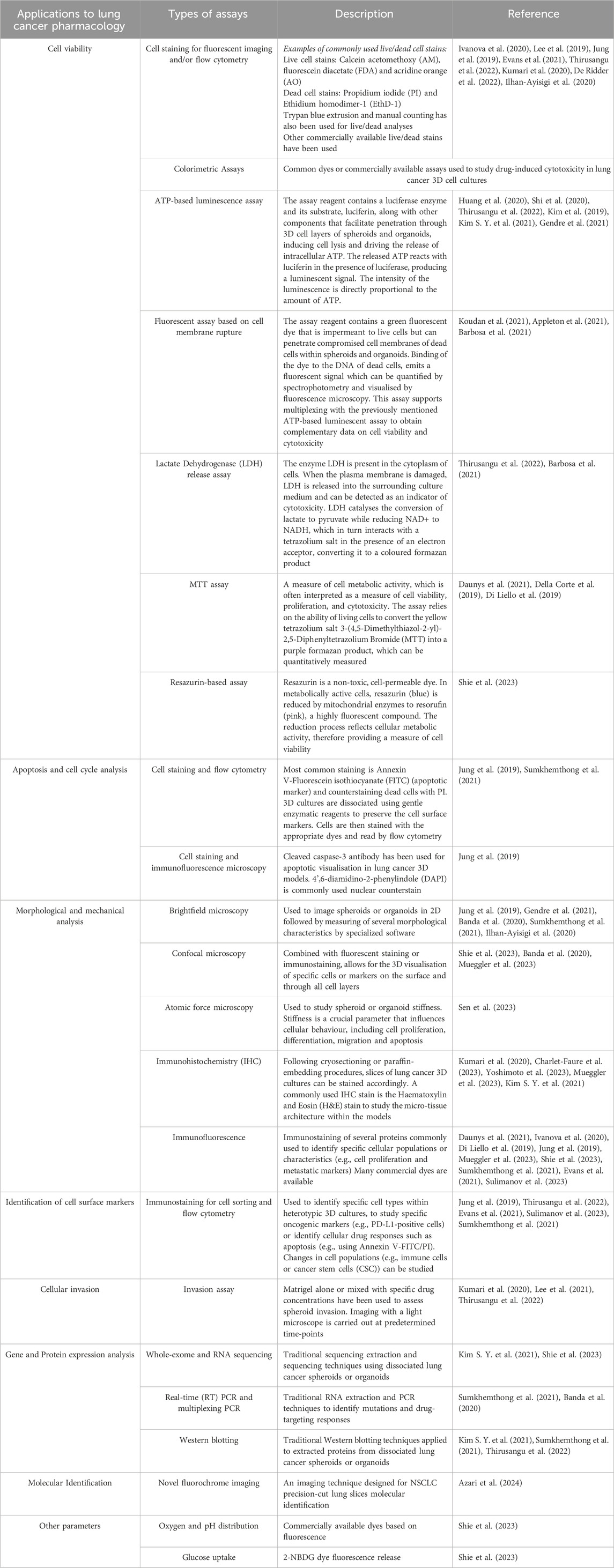
Table 3. Common laboratory-based assays used for pharmacological studies in lung cancer 3D cell culture models.
6.1 Cytotoxicity and apoptosis assays
One of the most widely used techniques in studies involving lung cancer 3D models is flow cytometry. Live cell stains including Calcein acetomethoxy (AM), fluorescein diacetate (FDA) and acridine orange (AO) and dead cell stains such as PI and Ethidium homodimer-1 (EthD-1) are commonly used to analyse cell viability in lung spheroids and organoids (Ivanova et al., 2020). Annexin V-FITC/PI is used to identify apoptotic cells by the binding of annexin V-FITC to phosphatidyl serine moieties on the cell membranes (green) and counterstaining the nucleus (red) with PI (Jung et al., 2019; Sumkhemthong et al., 2021). Cleaved caspase-3 anti-body has been used to visualize apoptotic activity in lung cancer 3D models using immunofluorescence microscopy. DAPI is commonly used as cell nuclear counterstain (Jung et al., 2019). Other brand-specific live/dead cell dyes are often used to analyse live/dead proportions in these 3D cultures (Lee et al., 2019; Jung et al., 2019; Evans et al., 2021). 3D cultures are dissociated for staining and analysis by flow cytometry which is often combined with fluorescence microscopy in order to visually observe fluorescent cells or measure average intensity using software such as ImageJ in lung cancer 3D cultures (Kumari et al., 2020; De Ridder et al., 2022; Ilhan-Ayisigi et al., 2020). Confocal microscopy is commonly used for fluorescent visualization in 3D (Banda et al., 2020; Shie et al., 2023; Mueggler et al., 2023). Thirusangu et al. (2022) report the use of trypan blue extrusion counting as a measure of cell viability in lung cancer spheroids.
Several colorimetric techniques can be used to study cytotoxicity. The 3-(4,5-dimethylthiazol-2-yl)-2,5-diphenyltetrazolium bromide (MTT) assay has been reported to assess cell viability in lung cancer spheroids. Incubation times with MTT can be extended up till 1 day (Daunys et al., 2021). Spheroids grown in Matrigel were extracted using cold PBS-EDTA solution prior to cell viability analysis (Della Corte et al., 2019; Di Liello et al., 2019). Resazurin-based dyes have also been used to study cell viability in lung cancer spheroids (Shie et al., 2023). Commercially available kits involve a simple time-efficient protocol to assess ATP content in spheroids and organoids via a luminescent-based assay, very often used to quantify cell viability in lung cancer 3D models (Huang et al., 2020; Shi et al., 2020; Thirusangu et al., 2022; Kim et al., 2019; Kim S. Y. et al., 2021; Gendre et al., 2021). Other commercially available kits have been developed to study cytotoxicity via fluorescence emission. The dye penetrates ruptured cell membranes, binds to the DNA and gives off a fluorescent signal, which can be quantified by spectrophotometry and imaged with fluorescence microscopy for up to 72 h (Koudan et al., 2021; Appleton et al., 2021; Barbosa et al., 2021). The release of lactate dehydrogenase (LDH) from cells is used to identify cytotoxicity. Many studies employ LDH assays to investigate drug-induced cytotoxicity in different types of lung cancer 3D models (Thirusangu et al., 2022; Barbosa et al., 2021).
6.2 Morphological and mechanical analysis
ImageJ is widely used among scientists to measure spheroid and organoid morphological parameters through light microscopy (Jung et al., 2019). An open-source ImageJ plugin, SpheroidJ, has been released for the analysis of spheroid morphological characteristics (Lacalle et al., 2021). AnaSP (Analysis of SPheroids) has been developed with the intention of facilitating the acquisition of spheroid quantitative morphological data through 2D brightfield imaging. This open-source software allows for automatic, precise and accurate spheroid segmentation which has been optimized in versions 2.0 and above, enabling high-throughput analysis of morphological parameters (Piccinini, 2015; Piccinini et al., 2023). However, till now, no studies involving lung cancer 3D models have been reported to use AnaSP software. These morphological analyses can be used to assess changes in spheroid and organoid growth kinetics (e.g., diameter or cell scattering) with time (Jung et al., 2019; Gendre et al., 2021; Sumkhemthong et al., 2021; Banda et al., 2020; Ilhan-Ayisigi et al., 2020; Mueggler et al., 2023).
Stiffness, as a mechanical property of biological tissues, is a crucial parameter that influences cellular behaviour, including cell proliferation, differentiation, migration, and apoptosis. Atomic force microscopy and has been used to study drug effects on LCO stiffness (Sen et al., 2023).
6.3 Immunohistochemistry and immunofluorescence
Several intra-tumoural parameters can be studied via immunohistochemistry (IHC) of tissue slices. Spheroids can be fixed in 4% formaldehyde or 4% paraformaldehyde, with fixing times varying between different protocols. Cryosectioning or paraffin-embedded (FFPE) sectioning, followed by haematoxylin and eosin (H&E) staining can be used to study micro-tissue anatomy of lung cancer 3D cell cultures, before and after drug treatments (Kumari et al., 2020; Charlet-Faure et al., 2023; Yoshimoto et al., 2023; Mueggler et al., 2023; Kim S. Y. et al., 2021).
Immunostaining of lung 3D cultures with specific antibodies allows for spatial visualization of cells, proteins or targets of interest, both on the surface and inside of the 3D structures allowing for understanding morphological and molecular alterations with drug treatments (Daunys et al., 2021). Anti-EpCAM antibodies can used to identify tumour cells within heterotypic cell populations. In tumour cells not expressing EpCAM other staining methods can be used such as Hoechst staining followed by nuclear morphological analysis. Stromal cell viability can be assessed through EpCAM-negativity (Ivanova et al., 2020). To mention another few, cytokeratin, F-actin, E-cadherin, vimentin and Ki-67 antibodies are commonly used to study structural features or changes in marker expression in lung cancer 3D cultures for pharmacological purposes (Di Liello et al., 2019; Mueggler et al., 2023; Shie et al., 2023).
Cell sorting and flow cytometry provide a platform for the identification of cell surface markers of dissociated 3D cell cultures, most commonly spheroids and organoids (Thirusangu et al., 2022). Flow cytometry is indispensable when dealing with heterotypic cultures for the study of different cell types and sub-populations, such as the identification and characterisation of M1 and M2 TAMs (e.g., through CD64 and CD206 staining, respectively) or CSC markers (e.g., CD133) and the study of specific oncogenic markers (e.g., PDL-1-positive tumour cells) (Evans et al., 2021; Sumkhemthong et al., 2021; Sulimanov et al., 2023).
6.4 Spheroid invasion assay
Being a major culprit responsible for the high mortality of lung cancers, tumour invasion directly impacts our understanding of the lung cancer progression, treatment response and patient outcomes. Spheroids or organoids can be embedded in Matrigel mixed with concentrated drug solutions and imaged using light, phase contrast or confocal microscopy over pre-determined time-points to analyse distance or area of invasion as variables to investigate drug effects on tumour cell invasion (Kumari et al., 2020; Lee et al., 2021; Thirusangu et al., 2022).
6.5 Gene and protein expression analysis and other molecular identification techniques
Several traditional analytical techniques can be used to analyse expression profiles and specific molecules in lung cancer 3D culture cells. Western blotting on proteins extracted from lung cancer spheroids and organoids was carried out to investigate drug-induced alterations in protein expression (Sumkhemthong et al., 2021; Thirusangu et al., 2022). Whole-exome and RNA sequencing were also conducted on lung cancer 3D models (Kim S. Y. et al., 2021; Shie et al., 2023). Recently, Azari et al. (2024) developed and validated a fluorochrome imaging technique for NSCLC PCLS for molecular identification. RNA extraction of lung cancer spheroids followed by real-time (RT) PCR has been described by Sumkhemthong et al. (2021). Banda et al. (2020) have reported the use of multiplexing PCR for mutational analysis in lung cancer PDOs.
6.6 Assessing other parameters
Shie et al. (2023) report the use of commercially available dyes to measure oxygen and pH distribution throughout lung cancer spheroids. Changes in glucose uptake of spheroids exposed to treatment have also been studied using 2-NBDG dye fluorescence release.
7 3D cell culture models in drug development
The application of 3D cell culture models in drug screening and toxicity testing represents a significant enhancement over traditional methods. While animal models have been the gold standard for pre-clinical in vivo studies, they often fail to accurately predict human responses due to species-specific differences. 3D cell cultures bridge this gap by offering a more human-relevant model, and especially when used in tandem with animal studies, they provide a more comprehensive assessment of a drug’s pharmacological profile before progressing to clinical trials (Figure 6). Furthermore, this combined approach also enables the use of less animals during the pre-clinical drug development phases, as well as reduces the likelihood of late-stage failures in clinical trials. The fidelity of such a system can be further enhanced through the application of co-culture approaches.
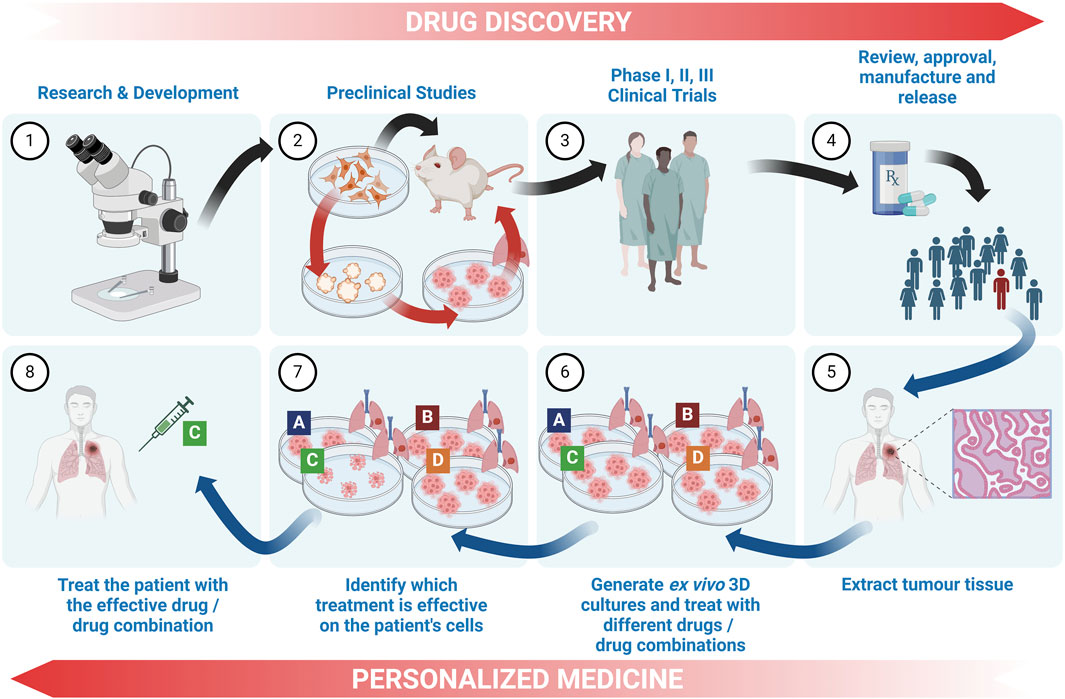
Figure 6. The contribution of 3D cell cultures to drug discovery and personalized medicine in lung cancer. Drug discovery: The drug discovery workflow involves (1) research and development, and moves on to (2) preclinical cell culture and animal model testing, (3) clinical trials and (4) approval, release and post-marketing surveillance. 3D cell culture models bridge the gap between pre-clinical 2D cell culture and animal studies, thus reducing the number of animals required and reducing the risk of therapeutic failure and/or toxicity in the clinical stages. Personalized medicine: 3D cultures can contribute to personalized medicine by (5) extracting tumour tissue from a patient, (6) generating ex vivo 3D cultures and exposing them to a panel of chemotherapeutic drugs or drug combinations (7) identifying the drug or drug combination that is most effective for the patient’s cells, and (8) treating the patient with the identified optimum chemotherapy. (Legend: Black arrows - normal drug development workflow; red arrows - introduction of 3D models into pre-clinical stages; blue arrows - workflow for 3D cell culture applications in personalized medicine).
The incorporation of 3D cultures in drug development, presents several challenges. The diverse types of 3D cultures often presents a dilemma as to which particular model best simulates in vivo conditions for the generation of clinically relevant data for a specific drug or drug class. Furthermore, industrial drug development requires systems that can be used in high-throughput modalities. Such challenges have often hampered their use for large scale primary screening. These have consequently often limited their applications to smaller scale experiments and validation involving single end-point measurements, towards the middle to lower end of the pre-clinical drug development workflow (Booij et al., 2019).
Specifically, 3D models need to be (a) scalable to industrial level requirements. (b) automatable to enable inclusion into a large-scale drug development workflow, and (c) reproducible in order to ensure assay precision and validity of data obtained from testing of different compounds. Furthermore, up-scalability and automation also need to be applicable to any imaging and assay measurements required as experimental endpoints. While automated liquid handling techniques are standard procedures widely adopted in the general industry, these tend to perform poorly when using highly viscous materials such as natural hydrogels. This is further compounded by the inter-batch variability in protein content and composition of these naturally-derived products, often influencing their temperature-dependent consistency and cellular responses (Booij et al., 2019). Notwithstanding such disadvantages, gel-based scaffolds still remain the 3D cell culture models which are most functionally adapted to large-scale drug screening. Although liquid-based models, such as those generated by hanging drop or ULA plates, are easier to automate, the lack of ECM proteins in such models influences their ability to enable the remodelling required to support normal cell adhesion, growth, differentiation and cell-cell communication (Cushing and Anseth, 2007). The choice between natural and synthetic hydrogel use in such systems remains a matter for debate. The former demonstrate higher in vivo mimicry but poorer batch-to-batch reproducibility, while the latter are less accurate at mimicking in vivo tumours, but show higher batch-to-batch consistency and therefore higher experimental precision.
The most promising gel matrices are synthetic hydrogels to which are added functional synthetic peptides which mimic those in natural ECM, such as peptides that mimic fibronectin, laminin–integrin interaction sites and collagen-derived adhesive peptides. Moreover, proteolytically degradable domains can be included in these protein mimics, in order to further allow complex 3D cellular behaviour (Gjorevski et al., 2016; Miller et al., 2010). The synthetic nature of these products ensures batch consistency, however there is still no universal agreement on the profile and nature of functional peptides that should be used in order to best mimic the ECM of different tumours.
High-throughput automated systems capable of handling assay execution for 3D models, especially assays involving histological analysis and imaging, have also proven to be a formidable challenge. Conventional imaging techniques are inadequate to capture 3D data and confocal or multiphoton microscopy is necessary to study the internal micro-tumour core. Moreover, the actual thickness of scaffold-based models, also presents problems with chemical-based endpoint assays, since it is difficult to ensure penetration of assay compounds to the core of the model.
In order to mitigate this, Wu et al. developed an electronic biosensor detection and recording approach to study drug screening in a panel of lung cancer spheroids derived from H549, H1299 and H460 cell lines. Their approach was to use electrical impedance sensing to record the reduction in spheroid size upon the application of anticancer drugs under study. The authors identified a synergistic effect of cisplatin plus etoposide on A549 spheroids. The authors further developed a multi-organ spheroid system, using A549, HL-1 and HepG2-based spheroids, cultured on the same multi-well plate, with interconnected wells such that the different spheroids share the same flow path. This model was used to study the toxicity of lung cancer chemotherapeutic agents on the heart and liver, with simultaneous recording of impedance in multiple wells. This is a promising pathway for high-throughput sensing and recording of drug effects which may be up-scaled to industrial-based anti-cancer drug screening (Wu et al., 2018).
Microfluidic systems offer a promising future for integration into drug development, since they satisfy the requirements of automation and scalability. Moreover, they are largely based on liquid handling engineering technologies, offering precise control of active fluidic transport and content, and making them more amenable to high-throughput adaptation. The possibility of increasing complexity by modelling interactions between different organs on the same chip, sharing the same circulatory environment, greatly increases the potential for these models to serve as industry-level drug screening and testing platforms for new anti-cancer drugs.
8 3D cell culture models and personalized medicine
The National Cancer Institute of the NIH has defined cancer-related personalized (or precision) medicine as an approach that “uses specific information about a person’s tumour to help make a diagnosis, plan treatment, find out how well treatment is working, or make a prognosis” (National Cancer Institute, 2024). It aims to address issues regarding the underlying inter-patient tumour heterogeneity by establishing therapeutic procedures that are tailored to that individual’s unique biochemical, physiological, genetic and behavioural profile (Goetz and Schork, 2018). Within this framework, it is necessary to study live patient material from tumour biopsies or resected tumour tissue within an ex vivo laboratory environment, in order to propose a patient-optimized therapeutic profile (Figure 6). This is particularly useful for rare or aggressive tumours where maximum treatment efficacy within the minimum time is mandatory.
The suitability of 3D cell cultures for personalized medicine has been recently extensively reviewed by El Harane et al. (2023). A key advantage is that patient-tissue derived 3D models carry the same cellular heterogeneity and genomic diversity of the patient’s tumour. Such models afford the ability to interrogate tumour responses to targeted therapies and chemotherapeutic agents within a relevant patient-dependent context, thereby facilitating the identification of patient-specific drug sensitivity and resistance. The models need to mimic the in vivo tumour with enough accuracy in order to enable ex vivo data to be clinically translatable to a patient’s personalized treatment profile. This shifts the emphasis from the industry-required high-throughput and automation, to critical requirements of accurate model mimicry, and clinically translatable treatment data within an actionable timeframe (Cantrell and Kuo, 2015).
PDO models currently occupy centre stage as lung cancer models suitable for the study of personalized chemotherapies (Fong et al., 2017; Law et al., 2021; Li. Y. et al., 2023; Li H. et al., 2023; Liu et al., 2023; Wang H. M. et al., 2023; Gu et al., 2024). PDOs can be generated in a relatively short time, and can be genetically profiled in order to identify tumour-dependent acquired mutations (Hu Y. et al., 2021). There is also ample evidence to show that the cellular composition and protein expression profiles and mutation heterogeneity of the patient’s tumour are retained in PDO models (Kim et al., 2019). Banda et al. showed that chemotherapy-driven enrichment of specific mutant sub-populations of patient-derived 3D models, also mimics what occurs in vivo. For example, erlotinib-treated spheroids derived from patient lung adenocarcinoma, showed a major increase in PIK3CA H1047R mutant subpopulations compared to BRAF V600E, KRAS G12D, KRAS G12V, and PIK3CA H1047R mutant cells, which aligns with what happens in patients. Therefore, ex vivo cultures not only replicate the tumour composition at the time of biopsy, but also project temporal and treatment-induced changes, in the same manner as occurs in patients (Banda et al., 2020; Taverna et al., 2024).
The reported success rates in the generation of ex vivo lung cancer organoids have risen along the years and now generally exceed 75% (Kim S. Y. et al., 2021; Wang M. et al., 2023; Shi et al., 2020; Hu Y. et al., 2021), adding to their suitability for precision medicine. Within NSCLC subtypes, SCC organoids appear to have the greatest variability in success rates between different research groups, which is however still reportedly higher than with other cancers. This places lung cancer as a leading candidate for ex vivo-based precision medicine (Liu et al., 2023; Rossi et al., 2022).
It however remains a challenge to obtain lung cancer tumour tissue, unless the patient is scheduled for surgery. Lung cancer biopsies tend to carry risks and may require strong medical justification in order to be carried out. Pleural effusion aspirate may prove to be an alternative suitable source of tumour cells. The generation of 3D organoids from the drained pleural effusion of lung adenocarcinoma patients has been described by Mazzocchi and co-workers, who showed that the generated organoids contained cancer cells with both immune and stromal components including fibroblasts and haematopoietic cells. Such organoids also produced a drug response profile to a cisplatin/pemetrexed combination, carboplatin/pemetrexed combination and crizotinib alone, that was similar to the responses seen in the patients from whom the pleural effusions were derived. This suggests that drained pleural effusion may be a more suitable and accessible source of lung cancer cells for personalized medicine approaches (Mazzocchi et al., 2019; Mazzocchi et al., 2022).
Zhang and co-workers later developed a novel ex vivo lung cancer model, termed a lung cancer assembloid, that uses droplet microfluidic technology to replicate the three-dimensional architecture and heterogeneous microenvironment of actual lung tumours. This allows for the encapsulation of TME cells and organoids within microgels, leading to an accurate representation of the tumour’s heterogeneity in terms of cell types, genetics, and responses to drugs. The authors utilized this model to study the potential drug resistance that may occur in vivo due to the presence of cancer-associated fibroblasts and other stromal elements, and showed that this model can accurately replicate the clinical outcomes of patients (Zhang et al., 2024).
Challenges which hinder the widespread clinical applications of 3D model-driven personalized therapy for lung cancer remain. The most critical of these is the lack of validated and standardized specific models or protocols that dictate which 3D models should be used for each situation and how such models should be generated. Until there is a general consensus reflected by evidence-based science, applicable to different lung cancer subtypes, there is bound to be data disagreement between different working groups. Furthermore, the inherent complexity and heterogeneity of lung cancer makes it difficult to create ex vivo models that are representative of all aspects of the disease. This coupled to difficulties in establishing the appropriate culture conditions that support their growth and maintenance while preserving the original tumour characteristics, as well as the variable ex vivo model generation success rate reported by different groups, drives some researchers to question the current applicability to precision medicine. More work is needed before attaining a stage where regulatory agencies can be convinced that this is an important step towards improving lung cancer patient treatment efficacy and approve its incorporation into clinical practice.
9 Discussion
The generation of effective pharmacological tools for lung cancer remains a major challenge and this is undoubtedly one reason for its long-standing elevated position in the lethal hierarchy of solid tumours. The use of 3D cell culture models in lung cancer pharmacology research represents a paradigm shift in the approach towards understanding and treating this disease. The scientific community, together with policymakers and funding agencies, need to recognize the value of these advanced research tools and continue to support their development. With continued innovation and investment, 3D cell culture models will undoubtedly play an integral role in shaping the future of lung cancer therapy, offering hope for an increased quality of life to millions of patients worldwide.
Research in this area is active and progressing along two lines. The first is the development of further tumour characteristics that can be modelled, such as vascularization and oxygen tension. The second is the improvement of incorrectly modelled components which actually may contribute to false experimental outcomes. For example, most reported spheroids are smaller than 400 μm in diameter, while passive drug diffusion across cell layers reaches a depth of about 200 μm (Shie et al., 2023; Hamilton and Rath, 2019; Bauleth-Ramos et al., 2020). Therefore, small spheroids do not accurately model intra-tumoural gradients and this could be one factor that contributes to the often-reported discrepancies of drug IC50 values obtained via 2D and 3D culture studies. We suggest that larger spheroids are more amenable to reproduce similar drug diffusion gradients to those which occur in much larger in vivo tumours.
Within the lung cancer research domain, there have been very few reported studies of models which preserve the in vivo lung tissue architecture such as PCLS, WTE and OMS. This may be due to the greater technical difficulties in maintaining such models, with the corresponding low outcome success rates. The lung TME is central to the behaviour and response to treatments and its mimicry is essential for translatable experimental outcomes. As mentioned in this review, the use of dECM in conjunction with 3D lung cancer models has been briefly explored. The dECM used in these models was sourced from animals and the application of human lung dECM remains an area of exploration, offering potential new insights into lung tumour biomimicry. In addition, novel innovative technologies, such as the use of micro-beads to simulate the alveolar architecture and therefore model lung tumours developing in the alveolar region, need to be explored more. ALIs offer a significant promise towards modelling airway epithelial architecture, especially multi-layered co-cultured models. This has been evidenced through morphological, transcriptomic and secretory studies which showed ALIs to behave very similarly to an in vivo epithelial system (Geles et al., 2016). However, although these have been extensively used for other airway conditions such as inflammatory diseases, they have only been marginally applied in lung cancer studies. ALIs provide a unique system where in addition to disease, they can also model both systemic as well as aerosolized drug administration (Meenach et al., 2016; Movia et al., 2018). This makes them an insufficiently tapped resource for 3D lung cancer models and their increased application can provide new data which is of strong pharmacological relevance.
Drug development efforts have been unable to result in levels of effectiveness which are comparable to those achieved for other common cancers. This is further hampered by the long and costly bench to bedside drug development procedures and the minute fraction of screened compounds that actually successfully filter through to the final clinical testing stage. If 3D lung cancer models are expected to generate tangible time and cost-benefits which can translate to improved therapeutic tools, there are several creases in the system that need to be ironed out. The major one is perhaps the lack of agreement on which 3D model best mimics a specific disease. The plethora of variables in 3D cell culture model setups is likely to take multiple years of research before any convergence of agreement on an optimum model or set of models that yields accurate, reproducible and timely results can be established. The possibility of never being able to model the complete TME factors, including interactions of various cell types, tumour cell heterogeneity, vascularization, intra-tumoural oxygen gradients, drug penetration and the influence of other organ systems, has undeniable implications on the data generated by the model. However, the last decades have provided evidence that event and data modelling in areas such as material science are excellent substrates for in silico operation. Digital infrastructures offer the potential of acting in tandem with biological models to create more accurate output models. We propose that this approach could also be applied to biological modelling, in order to electronically compensate for missing or inaccurate components in 3D lung cancer models. Digital enhancement of such biological data can improve human in vivo mimicry and push forward its functional applicability to novel drug development.
The precision medicine academic and clinical areas have been greatly overtaken by genomic and transcriptomic-based treatment personalization. Within this context, ex vivo laboratory disease modelling is still at its infancy. However, genomics and transcriptomics are more adept at predicting disease outcomes due to germline mutations and less effective at addressing the genetic heterogeneity of acquired mutations in tumours. In this regard, 3D cultures currently offer the best ex vivo model for the implementation of personalized medicine and as suggested earlier, are also open to digitally-assisted enhanced modelling in order to direct optimized predictive data.
10 Conclusion
In summary, the 3D lung cancer modelling world is rapidly evolving and its profile of application areas will increase as new developments progressively show greater model-to-human translatability. However, higher in vivo mimicry is generally also associated with more complex model systems requiring higher cost and technical expertise. A balance between attainment of usable translatable data versus cost and time efficiency would likely best drive the way forward. For example, body-on-a-chip microfluidic devices offer excellent academic promise but may be too technically challenging to be implemented within a cost-effective industrial drug development framework. On the other hand, simpler models, such as spheroids, might provide less robust data but are more easily implementable. A balance might be found through the use of computational and perhaps AI modelling systems in tandem with biological models. This would potentially create a complementary digital-biological modelling system that is amenable to a broader application profile with higher throughput and cost efficiency.
Author contributions
NV: Writing–original draft, Writing–review and editing, Validation. AF: Writing–original draft, Writing–review and editing. VP: Supervision, Writing–review and editing.
Funding
The author(s) declare that no financial support was received for the research, authorship, and/or publication of this article.
Conflict of interest
The authors declare that the research was conducted in the absence of any commercial or financial relationships that could be construed as a potential conflict of interest.
Publisher’s note
All claims expressed in this article are solely those of the authors and do not necessarily represent those of their affiliated organizations, or those of the publisher, the editors and the reviewers. Any product that may be evaluated in this article, or claim that may be made by its manufacturer, is not guaranteed or endorsed by the publisher.
References
Adjei, A. A. (2019). Lung cancer worldwide. J. Thorac. Oncol. 14, 956. doi:10.1016/j.jtho.2019.04.001
Agraval, H., Sharma, J. R., Dholia, N., and Yadav, U. C. S. (2022). Air-liquid interface culture model to study lung cancer-associated cellular and molecular changes. Methods Mol. Biol. 2413, 133–144. doi:10.1007/978-1-0716-1896-7_14
Alexander, M., Kim, S. Y., and Cheng, H. (2020). Update 2020: management of non-small cell lung cancer. Lung 198, 897–907. doi:10.1007/s00408-020-00407-5
Alsafadi, H. N., Uhl, F. E., Pineda, R. H., Bailey, K. E., Rojas, M., Wagner, D. E., et al. (2020). Applications and approaches for three-dimensional precision-cut lung slices. Disease modeling and drug discovery. Am. J. Respir. Cell. Mol. Biol. 62, 681–691. doi:10.1165/rcmb.2019-0276TR
Altorki, N. K., Markowitz, G. J., Gao, D., Port, J. L., Saxena, A., Stiles, B., et al. (2019). The lung microenvironment: an important regulator of tumour growth and metastasis. Nat. Rev. Cancer. 19, 9–31. doi:10.1038/s41568-018-0081-9
Anderson, N. M., and Simon, M. C. (2020). The tumor microenvironment. Curr. Biol. 30, R921–R925. doi:10.1016/j.cub.2020.06.081
Anichini, A., Perotti, V. E., Sgambelluri, F., and Mortarini, R. (2020). Immune escape mechanisms in non small cell lung cancer. Cancers (Basel) 12, 3605. doi:10.3390/cancers12123605
Antunes, M. A., Morales, M. M., Pelosi, P., and Macêdo Rocco, P. R. (2013). “Lung Resident stem cells,” in Resident stem cells and regenerative therapy, 105–122. doi:10.1016/B978-0-12-416012-5.00006-2
Appleton, K., Lassahn, K., Elrod, A., and DesRochers, T. (2021). 281 Measuring immune checkpoint inhibitor efficacy using primary patient-derived 3D spheroids. J. Immunother. Cancer 9, A305. doi:10.1136/jitc-2021-SITC2021.281
Arandkar, S., Furth, N., Elisha, Y., Nataraj, N. B., van der Kuip, H., Yarden, Y., et al. (2018). Altered p53 functionality in cancer-associated fibroblasts contributes to their cancer-supporting features. Proc. Natl. Acad. Sci. USA. 115, 6410–6415. doi:10.1073/pnas.1719076115
Azari, F., Kennedy, G. T., Chang, A., Low, P., Basil, M., Planer, J., et al. (2024). Molecular imaging in precision-cut non-small cell lung cancer slices. Ann. Thorac. Surg. 117, 458–465. doi:10.1016/j.athoracsur.2023.07.037
Balážová, K., Clevers, H., and Dost, A. F. M. (2023). The role of macrophages in non-small cell lung cancer and advancements in 3D co-cultures. Elife 12, e82998. doi:10.7554/eLife.82998
Banda, M., McKim, K. L., Myers, M. B., Inoue, M., and Parsons, B. L. (2020). Outgrowth of erlotinib-resistant subpopulations recapitulated in patient-derived lung tumor spheroids and organoids. PLoS One 15, e0238862. doi:10.1371/journal.pone.0238862
Barbosa, M. A. G., Xavier, C. P. R., Pereira, R. F., Petrikaitė, V., and Vasconcelos, M. H. (2021). 3D cell culture models as recapitulators of the tumor microenvironment for the screening of anti-cancer drugs. Cancers (Basel) 14, 190. doi:10.3390/cancers14010190
Barrera, C., Corredor, G., Viswanathan, V. S., Ding, R., Toro, P., Fu, P., et al. (2023). Deep computational image analysis of immune cell niches reveals treatment-specific outcome associations in lung cancer. NPJ Precis. Oncol. 7, 52. doi:10.1038/s41698-023-00403-x
Barros, A. S., Costa, A., and Sarmento, B. (2021). Building three-dimensional lung models for studying pharmacokinetics of inhaled drugs. Adv. Drug Deliv. Rev. 170, 386–395. doi:10.1016/j.addr.2020.09.008
Barua, S., Fang, P., Sharma, A., Fujimoto, J., Wistuba, I., Rao, A. U. K., et al. (2018). Spatial interaction of tumor cells and regulatory T cells correlates with survival in non-small cell lung cancer. Lung Cancer 117, 73–79. doi:10.1016/j.lungcan.2018.01.022
Bauleth-Ramos, T., Feijão, T., Gonçalves, A., Shahbazi, M. A., Liu, Z., Barrias, C., et al. (2020). Colorectal cancer triple co-culture spheroid model to assess the biocompatibility and anticancer properties of polymeric nanoparticles. J. Control Release. 323, 398–411. doi:10.1016/j.jconrel.2020.04.025
Becker, V., Yuan, X., Boewe, A. S., Ampofo, E., Ebert, E., Hohneck, J., et al. (2023). Hypoxia-induced downregulation of microRNA-186-5p in endothelial cells promotes non-small cell lung cancer angiogenesis by upregulating protein kinase C alpha. Mol. Ther. Nucleic Acids 31, 421–436. doi:10.1016/j.omtn.2023.01.015
Booij, T. H., Price, L. S., and Danen, E. H. J. (2019). 3D cell-based assays for drug screens: challenges in imaging, image analysis, and high-content analysis. SLAS Discov. 24, 615–627. doi:10.1177/2472555219830087
Boucherit, N., Gorvel, L., and Olive, D. (2020). 3D tumor models and their use for the testing of immunotherapies. Front. Immunol. 11, 603640. doi:10.3389/fimmu.2020.603640
Branchfield, K., Nantie, L., Verheyden, J. M., Sui, P., Wienhold, M. D., and Sun, X. (2016). Pulmonary neuroendocrine cells function as airway sensors to control lung immune response. Science 351, 707–710. doi:10.1126/science.aad7969
Bremnes, R. M., Al-Shibli, K., Donnem, T., Sirera, R., Al-Saad, S., Andersen, S., et al. (2011). The role of tumor-infiltrating immune cells and chronic inflammation at the tumor site on cancer development, progression, and prognosis: emphasis on non-small cell lung cancer. J. Thorac. Oncol. 6, 824–833. doi:10.1097/JTO.0b013e3182037b76
Brody, A. R. (2005). The brush cell. Am. J. Respir. Crit. Care Med. 172, 1349. doi:10.1164/ajrccm.172.10.1349
Cantrell, M. A., and Kuo, C. J. (2015). Organoid modeling for cancer precision medicine. Genome Med. 7, 32. doi:10.1186/s13073-015-0158-y
Carvalho, V., Bañobre-López, M., Minas, G., Teixeira, S. F. C. F., Lima, R., and Rodrigues, R. O. (2022). The integration of spheroids and organoids into organ-on-a-chip platforms for tumour research: a review. Bioprinting 27, e00224. doi:10.1016/j.bprint.2022.e00224
Charlet-Faure, C., Thulesen, A. P., and Rogowska-Wrzesinska, A. (2023). Advancements in 3D spheroid imaging: optimised cryosectioning and immunostaining techniques. MethodsX 11, 102415. doi:10.1016/j.mex.2023.102415
Chen, C., Hou, J., Yu, S., Li, W., Wang, X., Sun, H., et al. (2021). Role of cancer-associated fibroblasts in the resistance to antitumor therapy, and their potential therapeutic mechanisms in non-small cell lung cancer. Oncol. Lett. 21, 413. doi:10.3892/ol.2021.12674
Choi, S. Y., Cho, Y. H., Kim, D. S., Ji, W., Choi, C. M., Lee, J. C., et al. (2021). Establishment and long-term expansion of small cell lung cancer patient-derived tumor organoids. Int. J. Mol. Sci. 22, 1349. doi:10.3390/ijms22031349
Choi, Y. M., Lee, H., Ann, M., Song, M., Rheey, J., and Jang, J. (2023). 3D bioprinted vascularized lung cancer organoid models with underlying disease capable of more precise drug evaluation. Biofabrication 15, 034104. doi:10.1088/1758-5090/acd95f
Chua, F., and Laurent, G. J. (2006). “Fibroblasts,” in Encyclopedia of respiratory medicine, 213–219. doi:10.1016/B0-12-370879-6/00156-3
Colombo, R., and Rich, J. R. (2022). The therapeutic window of antibody drug conjugates: a dogma in need of revision. Cancer Cell 40, 1255–1263. doi:10.1016/j.ccell.2022.09.016
Cushing, M. C., and Anseth, K. S. (2007). Materials science. Hydrogel cell cultures. Science 316, 1133–1134. doi:10.1126/science.1140171
Dang, T. O., Ogunniyi, A., Barbee, M. S., and Drilon, A. (2016). Pembrolizumab for the treatment of PD-L1 positive advanced or metastatic non-small cell lung cancer. Expert Rev. Anticancer Ther. 16, 13–20. doi:10.1586/14737140.2016.1123626
Das, P., Najafikhoshnoo, S., Tavares-Negrete, J. A., Yi, Q., and Esfandyarpour, R. (2022). An in-vivo-mimicking 3D lung cancer-on-a-chip model to study the effect of external stimulus on the progress and inhibition of cancer metastasis. Bioprinting 28, e00243. doi:10.1016/j.bprint.2022.e00243
Daunys, S., Janonienė, A., Januškevičienė, I., Paškevičiūtė, M., and Petrikaitė, V. (2021). 3D tumor spheroid models for in vitro therapeutic screening of nanoparticles. Adv. Exp. Med. Biol. 1295, 243–270. doi:10.1007/978-3-030-58174-9_11
Della Corte, C. M., Barra, G., Ciaramella, V., Di Liello, R., Vicidomini, G., Zappavigna, S., et al. (2019). Antitumor activity of dual blockade of PD-L1 and MEK in NSCLC patients derived three-dimensional spheroid cultures. J. Exp. Clin. Cancer. Res. 38, 253. doi:10.1186/s13046-019-1257-1
De Ridder, K., Tung, N., Werle, J.-T., Karpf, L., Awad, R. A., Bernier, A., et al. (2022). Novel 3D lung tumor spheroids for oncoimmunological assays. Adv. Nanobiomed Res. 2, 2100124. doi:10.1002/anbr.202100124
Desai, A., Abdayem, P., Adjei, A. A., and Planchard, D. (2022). Antibody-drug conjugates: a promising novel therapeutic approach in lung cancer. Lung Cancer 163, 96–106. doi:10.1016/j.lungcan.2021.12.002
Di Liello, R., Ciaramella, V., Barra, G., Venditti, M., Della Corte, C. M., Papaccio, F., et al. (2019). Ex vivo lung cancer spheroids resemble treatment response of a patient with NSCLC to chemotherapy and immunotherapy: case report and translational study. ESMO Open 4, e000536. doi:10.1136/esmoopen-2019-000536
Ding, S., Zhang, H., and Wang, X. (2021). Microfluidic-chip-integrated biosensors for lung disease models. Biosens. (Basel). 11, 456. doi:10.3390/bios11110456
Du, W., Xia, X., Hu, F., and Yu, J. (2024). Extracellular matrix remodeling in the tumor immunity. Front. Immunol. 14, 1340634. doi:10.3389/fimmu.2023.1340634
Duzagac, F., Saorin, G., Memeo, L., Canzonieri, V., and Rizzolio, F. (2021). Microfluidic organoids-on-a-chip: quantum leap in cancer research. Cancers (Basel) 13, 737. doi:10.3390/cancers13040737
El Harane, S., Zidi, B., El Harane, N., Krause, K. H., Matthes, T., and Preynat-Seauve, O. (2023). Cancer spheroids and organoids as novel tools for research and therapy: state of the art and challenges to guide precision medicine. Cells 12, 1001. doi:10.3390/cells12071001
Endo, H., Okami, J., Okuyama, H., Kumagai, T., Uchida, J., Kondo, J., et al. (2013). Spheroid culture of primary lung cancer cells with neuregulin 1/HER3 pathway activation. J. Thorac. Oncol. 8, 131–139. doi:10.1097/JTO.0b013e3182779ccf
Enfield, K. S. S., Colliver, E., Lee, C., Magness, A., Moore, D. A., Sivakumar, M., et al. (2024). Spatial architecture of myeloid and T cells orchestrates immune evasion and clinical outcome in lung cancer. Cancer Discov. 14, 1018–1047. doi:10.1158/2159-8290.CD-23-1380
Evans, L., Milward, K., Attanoos, R., Clayton, A., Errington, R., and Tabi, Z. (2021). Macrophage plasticity and function in the lung tumour microenvironment revealed in 3D heterotypic spheroid and explant models. Biomedicines 9, 302. doi:10.3390/biomedicines9030302
Evans, M. J., and Kaufman, M. H. (1981). Establishment in culture of pluripotential cells from mouse embryos. Nature 292, 154–156. doi:10.1038/292154a0
Evans, M. J., Van Winkle, L. S., Fanucchi, M. V., and Plopper, C. G. (2001). Cellular and molecular characteristics of basal cells in airway epithelium. Exp. Lung Res. 27, 401–415. doi:10.1080/019021401300317125
Falcones, B., Sanz-Fraile, H., Marhuenda, E., Mendizábal, I., Cabrera-Aguilera, I., Malandain, N., et al. (2021). Bioprintable lung extracellular matrix hydrogel scaffolds for 3D culture of mesenchymal stromal cells. Polym. (Basel) 13, 2350. doi:10.3390/polym13142350
Ferreira, L. P., Gaspar, V. M., and Mano, J. F. (2020). Decellularized extracellular matrix for bioengineering physiomimetic 3D in vitro tumor models. Trends. Biotechnol. 38, 1397–1414. doi:10.1016/j.tibtech.2020.04.006
Ferreira, L. P., Gaspar, V. M., Mendes, L., Duarte, I. F., and Mano, J. F. (2021). Organotypic 3D decellularized matrix tumor spheroids for high-throughput drug screening. Biomaterials 275, 120983. doi:10.1016/j.biomaterials.2021.120983
Fong, E. L. S., Toh, T. B., Yu, H., and Chow, E. K. (2017). 3D culture as a clinically relevant model for personalized medicine. SLAS Technol. 22, 245–253. doi:10.1177/2472630317697251
Francis, I., Shrestha, J., Paudel, K. R., Hansbro, P. M., Warkiani, M. E., and Saha, S. C. (2022). Recent advances in lung-on-a-chip models. Drug. Discov. Today 27, 2593–2602. doi:10.1016/j.drudis.2022.06.004
Frezzetti, D., Gallo, M., Maiello, M. R., D'Alessio, A., Esposito, C., Chicchinelli, N., et al. (2017). VEGF as a potential target in lung cancer. Expert. Opin. Ther. Targets. 21, 959–966. doi:10.1080/14728222.2017.1371137
Fűr, G. M., Nemes, K., Magó, É., Benő, A. Á., Topolcsányi, P., Moldvay, J., et al. (2024). Applied models and molecular characteristics of small cell lung cancer. Pathol. Oncol. Res. 30, 1611743. doi:10.3389/pore.2024.1611743
Ganesan, S., Comstock, A. T., and Sajjan, U. S. (2013). Barrier function of airway tract epithelium. Tissue Barriers 1, e24997. doi:10.4161/tisb.24997
Gao, Y., Zhou, H., Liu, G., Wu, J., Yuan, Y., and Shang, A. (2022). Tumor microenvironment: lactic acid promotes tumor development. J. Immunol. Res. 2022, 3119375. doi:10.1155/2022/3119375
García-Cañaveras, J. C., Chen, L., and Rabinowitz, J. D. (2019). The tumor metabolic microenvironment: lessons from lactate. Cancer Res. 79, 3155–3162. doi:10.1158/0008-5472.CAN-18-3726
Garnique, A. D. M. B., and Machado-Santelli, G. M. (2023). Characterization of 3D NSCLC cell cultures with fibroblasts or macrophages for tumor microenvironment studies and chemotherapy screening. Cells 12, 2790. doi:10.3390/cells12242790
Geles, K. G., Zhong, W., O'Brien, S. K., Baxter, M., Loreth, C., Pallares, D., et al. (2016). Upregulation of RNA processing factors in poorly differentiated lung cancer cells. Transl. Oncol 9, 89–98. doi:10.1016/j.tranon.2016.01.006
Gendre, D. A. J., Ameti, E., Karenovics, W., Perriraz-Mayer, N., Triponez, F., and Serre-Beinier, V. (2021). Optimization of tumor spheroid model in mesothelioma and lung cancers and anti-cancer drug testing in H2052/484 spheroids. Oncotarget 12, 2375–2387. doi:10.18632/oncotarget.28134
Genova, C., Dellepiane, C., Carrega, P., Sommariva, S., Ferlazzo, G., Pronzato, P., et al. (2022). Therapeutic implications of tumor microenvironment in lung cancer: focus on immune checkpoint blockade. Front. Immunol. 12, 799455. doi:10.3389/fimmu.2021.799455
Gjorevski, N., Sachs, N., Manfrin, A., Giger, S., Bragina, M. E., Ordóñez-Morán, P., et al. (2016). Designer matrices for intestinal stem cell and organoid culture. Nature 539, 560–564. doi:10.1038/nature20168
Glisinski, K. M., Schlobohm, A. J., Paramore, S. V., Birukova, A., Moseley, M. A., Foster, M. W., et al. (2020). Interleukin-13 disrupts type 2 pneumocyte stem cell activity. JCI Insight 5 (1), e131232. doi:10.1172/jci.insight.131232
Goetz, L. H., and Schork, N. J. (2018). Personalized medicine: motivation, challenges, and progress. Fertil. Steril. 109, 952–963. doi:10.1016/j.fertnstert.2018.05.006
González-Martínez, E., Díaz-Águila, C. R., Rodríguez, M. C., Sánchez Ramírez, B., de Lourdes Albor-Aguilera, M., Alfaro, Y. G., et al. (2020). Growing spheroids of lung adenosquamous carcinoma on electrospun poly(ε-caprolactone). Bioinspired, Biomim. Nanobiomaterials 9, 252–256. doi:10.1680/jbibn.20.00054
Gu, Z., Wu, Q., Shang, B., Zhang, K., and Zhang, W. (2024). Organoid co-culture models of the tumor microenvironment promote precision medicine. Cancer Innov. 3, e101. doi:10.1002/cai2.101
Gupta, I., Hussein, O., Sastry, K. S., Bougarn, S., Gopinath, N., Chin-Smith, E., et al. (2023). Deciphering the complexities of cancer cell immune evasion: mechanisms and therapeutic implications. Adv. Cancer Biology-Metastasis 8, 100107. doi:10.1016/j.adcanc.2023.100107
Hamilton, G., and Rath, B. (2019). Role of circulating tumor cell spheroids in drug resistance. Cancer Drug Resist 2, 762–772. doi:10.20517/cdr.2019.47
Han, K., Pierce, S. E., Li, A., Spees, K., Anderson, G. R., Seoane, J. A., et al. (2020). CRISPR screens in cancer spheroids identify 3D growth-specific vulnerabilities. Nature 580, 136–141. doi:10.1038/s41586-020-2099-x
Harkema, J. R., Nikula, K. J., and Haschek, W. M. (2018). “Chapter 14. Respiratory system,” in Fundamentals of toxicologic pathology, 351–393. doi:10.1016/B978-0-12-809841-7.00014-9
Hassell, B. A., Goyal, G., Lee, E., Sontheimer-Phelps, A., Levy, O., Chen, C. S., et al. (2018). Human organ chip models recapitulate orthotopic lung cancer growth, therapeutic responses, and tumor dormancy in vitro. Cell Rep. 21, 508–516. doi:10.1016/j.celrep.2017.09.043
Hellmann, M. D., Paz-Ares, L., Bernabe Caro, R., Zurawski, B., Kim, S. W., Carcereny Costa, E., et al. (2019). Nivolumab plus ipilimumab in advanced non-small-cell lung cancer. N. Engl. J. Med. 381, 2020–2031. doi:10.1056/NEJMoa1910231
Herreros-Pomares, A., Zhou, X., Calabuig-Fariñas, S., Lee, S. J., Torres, S., Esworthy, T., et al. (2021). 3D printing novel in vitro cancer cell culture model systems for lung cancer stem cell study. Mat. Sci. Eng. C. Mat. Biol. Appl. 122, 111914. doi:10.1016/j.msec.2021.111914
Hickman, E., Smyth, T., Cobos-Uribe, C., Immormino, R., Rebuli, M. E., Moran, T., et al. (2023). Expanded characterization of in vitro polarized M0, M1, and M2 human monocyte-derived macrophages: bioenergetic and secreted mediator profiles. PLoS One 18, e0279037. doi:10.1371/journal.pone.0279037
Hida, K., Maishi, N., Takeda, R., and Hida, Y. (2022). “The roles of tumor endothelial cells in cancer metastasis,” in Metastasis. Editor C. M. Sergi (Brisbane (AU): Exon Publications).
Hollenhorst, M. I., Jurastow, I., Nandigama, R., Appenzeller, S., Li, L., Vogel, J., et al. (2020). Tracheal brush cells release acetylcholine in response to bitter tastants for paracrine and autocrine signaling. FASEB J. 34, 316–332. doi:10.1096/fj.201901314RR
Hoshiba, T. (2019). Decellularized extracellular matrix for cancer research. Mater. (Basel) 12, 1311. doi:10.3390/ma12081311
Hu, H., Piotrowska, Z., Hare, P. J., Chen, H., Mulvey, H. E., Mayfield, A., et al. (2021). Three subtypes of lung cancer fibroblasts define distinct therapeutic paradigms. Cancer Cell 39, 1531–1547.e10. doi:10.1016/j.ccell.2021.09.003
Hu, Y., Sui, X., Song, F., Li, Y., Li, K., Chen, Z., et al. (2021). Lung cancer organoids analyzed on microwell arrays predict drug responses of patients within a week. Nat. Commun. 12, 2581. doi:10.1038/s41467-021-22676-1
Huang, C. Y., Ju, D. T., Chang, C. F., Muralidhar Reddy, P., and Velmurugan, B. K. (2017). A review on the effects of current chemotherapy drugs and natural agents in treating non-small cell lung cancer. Biomed. (Taipei) 7, 23. doi:10.1051/bmdcn/2017070423
Huang, Y., Lu, Y., Vadlamudi, M., and Guo, X. (2019). Development and characterization of clinically relevant non-small cell lung cancer mouse model with near-infrared fluorescent 3D spheroids. Exp. Biol. 33 (S1), 509–515. doi:10.1096/fasebj.2019.33.1_supplement.509.5
Huang, Y., Lu, Y., Vadlamudi, M., Zhao, S., Felmlee, M., Rahimian, R., et al. (2020). Intrapulmonary inoculation of multicellular spheroids to construct an orthotopic lung cancer xenograft model that mimics four clinical stages of non-small cell lung cancer. J. Pharmacol. Toxicol. Methods 104, 106885. doi:10.1016/j.vascn.2020.106885
Huh, D., Matthews, B. D., Mammoto, A., Montoya-Zavala, M., Hsin, H. Y., and Ingber, D. E. (2010). Reconstituting organ-level lung functions on a chip. Science 328, 1662–1668. doi:10.1126/science.1188302
Huh, D. D. (2015). A human breathing lung-on-a-chip. Ann. Am. Thorac. Soc. 12 (Suppl. 1), S42–S44. doi:10.1513/AnnalsATS.201410-442MG
Hulo, P., Deshayes, S., Fresquet, J., Chéné, A. L., Blandin, S., Boisgerault, N., et al. (2024). Use of non-small cell lung cancer multicellular tumor spheroids to study the impact of chemotherapy. Respir. Res. 25, 156. doi:10.1186/s12931-024-02791-5
Huo, K. G., D'Arcangelo, E., and Tsao, M. S. (2020). Patient-derived cell line, xenograft and organoid models in lung cancer therapy. Transl. Lung Cancer Res. 9, 2214–2232. doi:10.21037/tlcr-20-154
Ichikawa, M., Muramatsu, N., Matsunaga, W., Ishikawa, T., Okuda, T., Okamoto, H., et al. (2022). Effects of inhalable gene transfection as a novel gene therapy for non-small cell lung cancer and malignant pleural mesothelioma. Sci. Rep. 12, 8634. doi:10.1038/s41598-022-12624-4
Ilhan-Ayisigi, E., Ulucan, F., Saygili, E., Saglam-Metiner, P., Gulce-Iz, S., and Yesil-Celiktas, O. (2020). Nano-vesicular formulation of propolis and cytotoxic effects in a 3D spheroid model of lung cancer. J. Sci. Food Agric. 100, 3525–3535. doi:10.1002/jsfa.10400
Ivanova, E., Kuraguchi, M., Xu, M., Portell, A. J., Taus, L., Diala, I., et al. (2020). Use of Ex vivo patient-derived tumor organotypic spheroids to identify combination therapies for HER2 mutant non-small cell lung cancer. Clin. Cancer Res. 26, 2393–2403. doi:10.1158/1078-0432.CCR-19-1844
Jalili-Firoozinezhad, S., Miranda, C. C., and Cabral, J. M. S. (2021). Modeling the human body on microfluidic chips. Trends Biotechnol. 39, 838–852. doi:10.1016/j.tibtech.2021.01.004
Jenkins, R. W., Aref, A. R., Lizotte, P. H., Ivanova, E., Stinson, S., Zhou, C. W., et al. (2018). Ex vivo profiling of PD-1 blockade using organotypic tumor spheroids. Cancer Discov. 8, 196–215. doi:10.1158/2159-8290.CD-17-0833
Jeong, K., Yu, Y. J., You, J. Y., Rhee, W. J., and Kim, J. A. (2020). Exosome-mediated microRNA-497 delivery for anti-cancer therapy in a microfluidic 3D lung cancer model. Lab. Chip. 20, 548–557. doi:10.1039/c9lc00958b
Jung, D. J., Shin, T. H., Kim, M., Sung, C. O., Jang, S. J., and Jeong, G. S. (2019). A one-stop microfluidic-based lung cancer organoid culture platform for testing drug sensitivity. Lab. Chip. 19, 2854–2865. doi:10.1039/c9lc00496c
Junk, D., Krämer, S., Broschewitz, J., Laura, H., Massa, C., Moulla, Y., et al. (2021). Human tissue cultures of lung cancer predict patient susceptibility to immune-checkpoint inhibition. Cell Death Discov. 7, 264. doi:10.1038/s41420-021-00651-5
Kanaji, N., Yokohira, M., Nakano-Narusawa, Y., Watanabe, N., Imaida, K., Kadowaki, N., et al. (2017). Hepatocyte growth factor produced in lung fibroblasts enhances non-small cell lung cancer cell survival and tumor progression. Respir. Res. 18, 118. doi:10.1186/s12931-017-0604-z
Karekla, E., Liao, W. J., Sharp, B., Pugh, J., Reid, H., Quesne, J. L., et al. (2017). Ex vivo explant cultures of non-small cell lung carcinoma enable evaluation of primary tumor responses to anticancer therapy. Cancer Res. 77, 2029–2039. doi:10.1158/0008-5472.CAN-16-1121
Kargl, J., Busch, S. E., Yang, G. H., Kim, K. H., Hanke, M. L., Metz, H. E., et al. (2017). Neutrophils dominate the immune cell composition in non-small cell lung cancer. Nat. Commun. 8, 14381. doi:10.1038/ncomms14381
Kaur, G., Doroshow, J. H., and Teicher, B. A. (2021). Format (2D vs 3D) and media effect target expression and response of patient-derived and standard NSCLC lines to EGFR inhibitors. Cancer Treat. Res. Commun. 29, 100463. doi:10.1016/j.ctarc.2021.100463
Khan, Y. S., and Lynch, D. T. (2023). “Histology, lung,” in StatPearls (Maryland, United States: StatPearls Publishing). Available at: https://www.ncbi.nlm.nih.gov/books/NBK534789.
Kim, J., Koo, B. K., and Knoblich, J. A. (2020). Human organoids: model systems for human biology and medicine. Nat. Rev. Mol. Cell Biol. 21, 571–584. doi:10.1038/s41580-020-0259-3
Kim, M., Mun, H., Sung, C. O., Cho, E. J., Jeon, H. J., Chun, S. M., et al. (2019). Patient-derived lung cancer organoids as in vitro cancer models for therapeutic screening. Nat. Commun. 10, 3991. doi:10.1038/s41467-019-11867-6
Kim, S. K., Kim, Y. H., Park, S., and Cho, S. W. (2021). Organoid engineering with microfluidics and biomaterials for liver, lung disease, and cancer modeling. Acta. Biomater. 132, 37–51. doi:10.1016/j.actbio.2021.03.002
Kim, S. Y., Kim, S. M., Lim, S., Lee, J. Y., Choi, S. J., Yang, S. D., et al. (2021). Modeling clinical responses to targeted therapies by patient-derived organoids of advanced lung adenocarcinoma. Clin. Cancer Res. 27, 4397–4409. doi:10.1158/1078-0432.CCR-20-5026
Knelson, E. H., Ivanova, E. V., Tarannum, M., Campisi, M., Lizotte, P. H., Booker, M. A., et al. (2022). Activation of tumor-cell STING primes NK-cell therapy. Cancer Immunol. Res. 10, 947–961. doi:10.1158/2326-6066.CIR-22-0017
Kobayashi, K. S., and van den Elsen, P. J. (2012). NLRC5: a key regulator of MHC class I-dependent immune responses. Nat. Rev. Immunol. 12, 813–820. doi:10.1038/nri3339
Koudan, E. V., Kudan, S. P., Karshieva, S. S., Khesuani, Y. D., Mironov, V. A., and Bulanova, E. A. (2021). The determination of cytostatic activity on a 3D spheroids-based model in comparison with conventional monolayer culture. Biol 15, 522–531. doi:10.1134/S1990519X21060055
Kumari, N., Bhargava, A., and Rath, S. N. (2020). T-type calcium channel antagonist, TTA-A2 exhibits anti-cancer properties in 3D spheroids of A549, a lung adenocarcinoma cell line. Life Sci. 260, 118291. doi:10.1016/j.lfs.2020.118291
Labani-Motlagh, A., Ashja-Mahdavi, M., and Loskog, A. (2020). The tumor microenvironment: a milieu hindering and obstructing antitumor immune responses. Front. Immunol. 11, 940. doi:10.3389/fimmu.2020.00940
Lacalle, D., Castro-Abril, H. A., Randelovic, T., Domínguez, C., Heras, J., Mata, E., et al. (2021). SpheroidJ: an open-source set of tools for spheroid segmentation. Comput. Methods Programs Biomed. 200, 105837. doi:10.1016/j.cmpb.2020.105837
Law, A. M. K., Rodriguez de la Fuente, L., Grundy, T. J., Fang, G., Valdes-Mora, F., and Gallego-Ortega, D. (2021). Advancements in 3D cell culture systems for personalizing anti-cancer therapies. Front. Oncol. 11, 782766. doi:10.3389/fonc.2021.782766
Lê, H., Seitlinger, J., Lindner, V., Olland, A., Falcoz, P. E., Benkirane-Jessel, N., et al. (2022). Patient-derived lung tumoroids-an emerging technology in drug development and precision medicine. Biomedicines 10, 1677. doi:10.3390/biomedicines10071677
Lee, D., Kim, Y., and Chung, C. (2021). Scientific validation and clinical application of lung cancer organoids. Cells 10, 3012. doi:10.3390/cells10113012
Lee, S. W., Hong, S., Jung, B., Jeong, S. Y., Byeon, J. H., Jeong, G. S., et al. (2019). In vitro lung cancer multicellular tumor spheroid formation using a microfluidic device. Biotechnol. Bioeng. 116, 3041–3052. doi:10.1002/bit.27114
Li, H., Chen, Z., Chen, N., Fan, Y., Xu, Y., and Xu, X. (2023). Applications of lung cancer organoids in precision medicine: from bench to bedside. Cell. Commun. Signal. 21, 350. doi:10.1186/s12964-023-01332-9
Li, S., Zhang, Z., and Han, L. (2020). 3D spheroids propel tumor characterization. Trends Cancer 6, 622–624. doi:10.1016/j.trecan.2020.05.002
Li, T., and Qiao, T. (2022). Unraveling tumor microenvironment of small-cell lung cancer: implications for immunotherapy. Semin. Cancer Biol. 86 (Pt 2), 117–125. doi:10.1016/j.semcancer.2022.09.005
Li, Y., Gao, X., Ni, C., Zhao, B., and Cheng, X. (2023). The application of patient-derived organoid in the research of lung cancer. Cell Oncol. (Dordr) 46, 503–519. doi:10.1007/s13402-023-00771-3
Li, Y., Liu, H., Zhao, Y., Yue, D., Chen, C., Li, C., et al. (2021a). Tumor-associated macrophages (TAMs)-derived osteopontin (OPN) upregulates PD-L1 expression and predicts poor prognosis in non-small cell lung cancer (NSCLC). Thorac. Cancer 12, 2698–2709. doi:10.1111/1759-7714.14108
Li, Y., Zhao, L., and Li, X. F. (2021b). Targeting hypoxia: hypoxia-activated prodrugs in cancer therapy. Front. Oncol. 11, 700407. doi:10.3389/fonc.2021.700407
Liang, M., Chen, M., Singh, S., and Singh, S. (2022). Prognostic nomogram for overall survival in small cell lung cancer patients treated with chemotherapy: a SEER-based retrospective cohort study. Adv. Ther. 39, 346–359. doi:10.1007/s12325-021-01974-6
Liu, C., Jin, Y., and Fan, Z. (2021). The mechanism of Warburg effect-induced chemoresistance in cancer. Front. Oncol. 11, 698023. doi:10.3389/fonc.2021.698023
Liu, J., Geng, X., Hou, J., and Wu, G. (2021). New insights into M1/M2 macrophages: key modulators in cancer progression. Int. 21, 389. doi:10.1186/s12935-021-02089-2
Liu, X., Wu, S., Yang, Y., Zhao, M., Zhu, G., and Hou, Z. (2017). The prognostic landscape of tumor-infiltrating immune cell and immunomodulators in lung cancer. Biomed. Pharmacother. 95, 55–61. doi:10.1016/j.biopha.2017.08.003
Liu, Y., Zhou, Y., and Chen, P. (2023). Lung cancer organoids: models for preclinical research and precision medicine. Front. Oncol. 13, 1293441. doi:10.3389/fonc.2023.1293441
Lord, E. M., Penney, D. P., Sutherland, R. M., and Cooper, R. A. (1979). Morphological and functional characteristics of cells infiltrating and destroying tumor multicellular spheroids in vivo. Virchows Arch. B Cell Pathol. Incl. Mol. Pathol. 31, 103–116. doi:10.1007/BF02889928
Lowe, J. S., and Anderson, P. G. (2015). “Respiratory system,” in Stevens lowes human histology, 166–185. doi:10.1016/B978-0-7234-3502-0.00010-3
Lu, Y., Liu, Y., Oeck, S., Zhang, G. J., Schramm, A., and Glazer, P. M. (2020). Hypoxia induces resistance to EGFR inhibitors in lung cancer cells via upregulation of FGFR1 and the MAPK pathway. Cancer Res. 80, 4655–4667. doi:10.1158/0008-5472.CAN-20-1192
Luan, Q., Pulido, I., Isagirre, A., Carretero, J., Zhou, J., Shimamura, T., et al. (2024). Deciphering fibroblast-induced drug resistance in non-small cell lung carcinoma through patient-derived organoids in agarose microwells. Lab. Chip. 24, 2025–2038. doi:10.1039/d3lc01044a
Ma, H. C., Zhu, Y. J., Zhou, R., Yu, Y. Y., Xiao, Z. Z., and Zhangm, H. B. (2022). Lung cancer organoids, a promising model still with long way to go. Crit. Rev. Oncol. Hematol. 171, 103610. doi:10.1016/j.critrevonc.2022.103610
Madeddu, C., Donisi, C., Liscia, N., Lai, E., Scartozzi, M., and Macciò, A. (2022). EGFR-mutated non-small cell lung cancer and resistance to immunotherapy: role of the tumor microenvironment. Int. J. Mol. Sci. 23, 6489. doi:10.3390/ijms23126489
Marques-Magalhães, Â., Cruz, T., Costa, Â. M., Estêvão, D., Rios, E., Canão, P. A., et al. (2022). Decellularized colorectal cancer matrices as bioactive scaffolds for studying tumor-stroma interactions. Cancers (Basel) 14, 359. doi:10.3390/cancers14020359
Mazzocchi, A., Devarasetty, M., Herberg, S., Petty, W. J., Marini, F., Miller, L., et al. (2019). Pleural effusion aspirate for use in 3D lung cancer modeling and chemotherapy screening. ACS Biomater. Sci. Eng. 5, 1937–1943. doi:10.1021/acsbiomaterials.8b01356
Mazzocchi, A., Dominijanni, A., and Soker, S. (2022). Pleural effusion aspirate for use in 3D lung cancer modeling and chemotherapy screening. Methods Mol. Biol. 2394, 471–483. doi:10.1007/978-1-0716-1811-0_24
Meenach, S. A., Tsoras, A. N., McGarry, R. C., Mansour, H. M., Hilt, J. Z., and Anderson, K. W. (2016). Development of three-dimensional lung multicellular spheroids in air- and liquid-interface culture for the evaluation of anticancer therapeutics. Int. J. Oncol. 48, 1701–1709. doi:10.3892/ijo.2016.3376
Meyerholz, D. K., Suarez, C. J., Dintzis, S. M., and Frevert, C. W. (2018). “Chapter 9: respiratory system,” in Comparative anatomy and histology, 147–162. doi:10.1016/B978-0-12-802900-8.00009-9
Miller, J. S., Shen, C. J., Legant, W. R., Baranski, J. D., Blakely, B. L., and Chen, C. S. (2010). Bioactive hydrogels made from step-growth derived PEG-peptide macromers. Biomaterials 31, 3736–3743. doi:10.1016/j.biomaterials.2010.01.058
Mondal, S., Roy, D., Sarkar Bhattacharya, S., Jin, L., Jung, D., Zhang, S., et al. (2019). Therapeutic targeting of PFKFB3 with a novel glycolytic inhibitor PFK158 promotes lipophagy and chemosensitivity in gynecologic cancers. Int. J. Cancer. 144, 178–189. doi:10.1002/ijc.31868
Movia, D., Bazou, D., Volkov, Y., and Prina-Mello, A. (2018). Multilayered Cultures of NSCLC cells grown at the Air-Liquid Interface allow the efficacy testing of inhaled anti-cancer drugs. Sci. Rep. 8, 12920. doi:10.1038/s41598-018-31332-6
Mueggler, A., Pilotto, E., Perriraz-Mayer, N., Jiang, S., Addeo, A., Bédat, B., et al. (2023). An optimized method to culture human primary lung tumor cell spheroids. Cancers (Basel) 15, 5576. doi:10.3390/cancers15235576
Murgia, X., Yasar, H., Carvalho-Wodarz, C., Loretz, B., Gordon, S., Schwarzkopf, K., et al. (2017). Modelling the bronchial barrier in pulmonary drug delivery: a human bronchial epithelial cell line supplemented with human tracheal mucus. Eur. J. Pharm. Biopharm. 118, 79–88. doi:10.1016/j.ejpb.2017.03.020
Nakamura, H., Sugano, M., Miyashita, T., Hashimoto, H., Ochiai, A., Suzuki, K., et al. (2019). Organoid culture containing cancer cells and stromal cells reveals that podoplanin-positive cancer-associated fibroblasts enhance proliferation of lung cancer cells. Lung Cancer 134, 100–107. doi:10.1016/j.lungcan.2019.04.007
Naratornsirakul, D., Chewaskulyong, B., Kongkarnka, S., and Oranratnachai, S. (2024). Comparison of treatment outcome between first-line combination immunotherapy (anti-PD-L1 or anti-PD1) with or without chemotherapy and chemotherapy alone in advanced non-small cell lung cancer patients in tertiary care hospital. Cancer Med. 13, e70007. doi:10.1002/cam4.70007
Närhi, K., Nagaraj, A. S., Parri, E., Turkki, R., van Duijn, P. W., Hemmes, A., et al. (2018). Spatial aspects of oncogenic signalling determine the response to combination therapy in slice explants from Kras-driven lung tumours. J. Pathol. 245, 101–113. doi:10.1002/path.5059
National Cancer Institute (2024). Dictionary of cancer terms: personalized medicine. Available at: https://www.cancer.gov/publications/dictionaries/cancer-terms/def/personalized-medicine (Accessed April 19, 2024).
Neal, J. T., Li, X., Zhu, J., Giangarra, V., Grzeskowiak, C. L., Ju, J., et al. (2018). Organoid modeling of the tumor immune microenvironment. Cell 175, 1972–1988. doi:10.1016/j.cell.2018.11.021
Noguchi, M., Furukawa, K. T., and Morimoto, M. (2020). Pulmonary neuroendocrine cells: physiology, tissue homeostasis and disease. Dis. Model Mech. 13, dmm046920. doi:10.1242/dmm.046920
Onodera, R., Jimma, Y., Suzuki, A., Habano, W., Ozawa, S., and Terashima, J. (2023). The regulation pathway of VEGF gene expression is different between 2D cells and 3D spheroids in human lung cancer cells. Biol. Pharm. Bull. 46, 608–613. doi:10.1248/bpb.b22-00772
Papait, A., Romoli, J., Stefani, F. R., Chiodelli, P., Montresor, M. C., Agoni, L., et al. (2022). Fight the cancer, hit the CAF. Cancers (Basel) 14, 3570. doi:10.3390/cancers14153570
Park, S., Kim, T. H., Kim, S. H., You, S., and Jung, Y. (2021). Three-dimensional vascularized lung cancer-on-a-chip with lung extracellular matrix hydrogels for in vitro screening. Cancers (Basel) 13, 3930. doi:10.3390/cancers13163930
Park, S., Kim, Y., Ko, J., Song, J., Lee, J., Hong, Y.-K., et al. (2023). One-step achievement of tumor spheroid-induced angiogenesis in a high-throughput microfluidic platform: one-step tumor angiogenesis platform. Organoid 3, e3. doi:10.51335/organoid.2023.3.e3
Parra, E. R., Zhang, J., Jiang, M., Tamegnon, A., Pandurengan, R. K., Behrens, C., et al. (2023). Immune cellular patterns of distribution affect outcomes of patients with non-small cell lung cancer. Nat. Commun. 14, 2364. doi:10.1038/s41467-023-37905-y
Pei, X., Ling, H., Lu, Y., Vadlamudi, M., Huang, Y., Huang, R., et al. (2020). Abstract 312: multicellular spheroids of A549 cells: a clinically relevant model of lung cancer. Cancer Res. 80, 312. doi:10.1158/1538-7445.AM2020-312
Phogat, S., Thiam, F., Al Yazeedi, S., Abokor, F. A., and Osei, E. T. (2023). 3D in vitro hydrogel models to study the human lung extracellular matrix and fibroblast function. Respir. Res. 5, 242. doi:10.1186/s12931-023-02548-6
Piccinini, F. (2015). AnaSP: a software suite for automatic image analysis of multicellular spheroids. Comput. Methods Programs Biomed. 119, 43–52. doi:10.1016/j.cmpb.2015.02.006
Piccinini, F., Peirsman, A., Stellato, M., Pyun, J.-C., Tumedei, M. M., Tazzari, M., et al. (2023). Deep learning-based tool for morphotypic analysis of 3D multicellular spheroids. J. Mech. Med. Biol. 23, 06. doi:10.1142/S0219519423400341
Pinto, B., Henriques, A. C., Silva, P. M. A., and Bousbaa, H. (2020). Three-dimensional spheroids as in vitro preclinical models for cancer research. Pharmaceutics 12, 1186. doi:10.3390/pharmaceutics12121186
Pomerenke, A. (2021). Organotypic models of lung cancer. Curr. Top. Microbiol. Immunol. 430, 161–181. doi:10.1007/82_2017_79
Preuß, E. B., Schubert, S., Werlein, C., Stark, H., Braubach, P., Höfer, A., et al. (2022). The challenge of long-term cultivation of human precision-cut lung slices. Am. J. Pathol. 192, 239–253. doi:10.1016/j.ajpath.2021.10.020
Qin, A., Coffey, D. G., Warren, E. H., and Ramnath, N. (2016). Mechanisms of immune evasion and current status of checkpoint inhibitors in non-small cell lung cancer. Cancer Med. 5, 2567–2578. doi:10.1002/cam4.819
Rackley, C. R., and Stripp, B. R. (2012). Building and maintaining the epithelium of the lung. J. Clin. Invest. 122, 2724–2730. doi:10.1172/JCI60519
Rokicki, W., Rokicki, M., Wojtacha, J., and Dżeljijli, A. (2016). The role and importance of club cells (Clara cells) in the pathogenesis of some respiratory diseases. Kardiochir Torakochirurgia Pol. 13, 26–30. doi:10.5114/kitp.2016.58961
Roman, V., Mihaila, M., Radu, N., Marineata, S., Diaconu, C. C., and Bostan, M. (2023). Cell culture model evolution and its impact on improving therapy efficiency in lung cancer. Cancers (Basel) 15, 4996. doi:10.3390/cancers15204996
Rossi, R., De Angelis, M. L., Xhelili, E., Sette, G., Eramo, A., De Maria, R., et al. (2022). Lung cancer organoids: the rough path to personalized medicine. Med. Cancers (Basel) 14, 3703. doi:10.3390/cancers14153703
Rozenberg, J. M., Filkov, G. I., Trofimenko, A. V., Karpulevich, E. A., Parshin, V. D., Royuk, V. V., et al. (2021). Biomedical applications of non-small cell lung cancer spheroids. Front. Oncol. 11, 791069. doi:10.3389/fonc.2021.791069
Ruaro, B., Salton, F., Braga, L., Wade, B., Confalonieri, P., Volpe, M. C., et al. (2021). The history and mystery of alveolar epithelial type II cells: focus on their physiologic and pathologic role in lung. Int. J. Mol. Sci. 22, 2566. doi:10.3390/ijms22052566
Rudin, C. M., Brambilla, E., Faivre-Finn, C., and Sage, J. (2021). Small-cell lung cancer. Nat. Rev. Dis. Prim. 14, 3. doi:10.1038/s41572-020-00235-0
Ruiz-Cordero, R., and Devine, W. P. (2020). Targeted therapy and checkpoint immunotherapy in lung cancer. Surg. Pathol. Clin. 13, 17–33. doi:10.1016/j.path.2019.11.002
Sachs, N., Papaspyropoulos, A., Zomer-van Ommen, D. D., Heo, I., Böttinger, L., Klay, D., et al. (2019). Long-term expanding human airway organoids for disease modeling. EMBO J. 38, e100300. doi:10.15252/embj.2018100300
Salehi-Rad, R., and Dubinett, S. M. (2019). Understanding the mechanisms of immune-evasion by lung cancer in the context of chronic inflammation in emphysema. J. Thorac. Dis. 11, 382–385. doi:10.21037/jtd.2019.01.22
Scherer, W. F., Syverton, J. T., and Gey, G. O. (1953). Studies on the propagation in vitro of poliomyelitis viruses. IV. Viral multiplication in a stable strain of human malignant epithelial cells (strain HeLa) derived from an epidermoid carcinoma of the cervix. J. Exp. Med. 97, 695–710. doi:10.1084/jem.97.5.695
Seitlinger, J., Nounsi, A., Idoux-Gillet, Y., Santos Pujol, E., Lê, H., Grandgirard, E., et al. (2022). Vascularization of patient-derived tumoroid from non-small-cell lung cancer and its microenvironment. Biomedicines 10, 1103. doi:10.3390/biomedicines10051103
Sen, C., Koloff, C. R., Kundu, S., Wilkinson, D. C., Yang, J. M., Shia, D. W., et al. (2023). Development of a small cell lung cancer organoid model to study cellular interactions and survival after chemotherapy. Front. Pharmacol. 14, 1211026. doi:10.3389/fphar.2023.1211026
Shabalina, E. Y., Skorova, E. Y., Chudakova, D. A., Anikin, V. B., Reshetov, I. V., Mynbaev, O. A., et al. (2021). The matrix-dependent 3D spheroid model of the migration of non-small cell lung cancer: a step towards a rapid automated screening. Front. Mol. Biosci. 8, 610407. doi:10.3389/fmolb.2021.610407
Shi, R., Radulovich, N., Ng, C., Liu, N., Notsuda, H., Cabanero, M., et al. (2020). Organoid cultures as preclinical models of non-small cell lung cancer. Clin. Cancer Res. 26, 1162–1174. doi:10.1158/1078-0432.CCR-19-1376
Shie, M. Y., Fang, H. Y., Kan, K. W., Ho, C. C., Tu, C. Y., Lee, P. C., et al. (2023). Highly mimetic ex vivo lung-cancer spheroid-based physiological model for clinical precision therapeutics. Adv. Sci. (Weinh). 10, e2206603. doi:10.1002/advs.202206603
Shien, K., Papadimitrakopoulou, V. A., Ruder, D., Behrens, C., Shen, L., Kalhor, N., et al. (2017). JAK1/STAT3 activation through a proinflammatory cytokine pathway leads to resistance to molecularly targeted therapy in non-small cell lung cancer. Mol. Cancer Ther. 16, 2234–2245. doi:10.1158/1535-7163.MCT-17-0148
Shin, S., Ikram, M., Subhan, F., Kang, H. Y., Lim, Y., Lee, R., et al. (2016). Alginate–marine collagen–agarose composite hydrogels as matrices for biomimetic 3D cell spheroid formation. RSC Adv. 6, 46952–46965. doi:10.1039/C6RA01937D
Shrestha, J., Razavi Bazaz, S., Aboulkheyr Es, H., Yaghobian Azari, D., Thierry, B., Ebrahimi Warkiani, M., et al. (2020). Lung-on-a-chip: the future of respiratory disease models and pharmacological studies. Crit. Rev. Biotechnol. 40, 213–230. doi:10.1080/07388551.2019.1710458
Singleton, D. C., Macann, A., and Wilson, W. R. (2021). Therapeutic targeting of the hypoxic tumour microenvironment. Nat. Rev. Clin. Oncol. 18, 751–772. doi:10.1038/s41571-021-00539-4
Sontheimer-Phelps, A., Hassell, B. A., and Ingber, D. E. (2019). Modelling cancer in microfluidic human organs-on-chips. Nat. Rev. Cancer 19, 65–81. doi:10.1038/s41568-018-0104-6
Stankovic, B., Bjørhovde, H. A. K., Skarshaug, R., Aamodt, H., Frafjord, A., Müller, E., et al. (2019). Immune cell composition in human non-small cell lung cancer. Front. Immunol. 9, 3101. doi:10.3389/fimmu.2018.03101
Sulimanov, R., Koshelev, K., Makarov, V., Mezentsev, A., Durymanov, M., Ismail, L., et al. (2023). Mathematical modeling of non-small-cell lung cancer biology through the experimental data on cell composition and growth of patient-derived organoids. Life (Basel) 13, 2228. doi:10.3390/life13112228
Sumkhemthong, S., Chamni, S., Ecoy, G. U., Taweecheep, P., Suwanborirux, K., Prompetchara, E., et al. (2021). Jorunnamycin A suppresses stem-like phenotypes and sensitizes cisplatin-induced apoptosis in cancer stem-like cell-enriched spheroids of human lung cancer cells. Mar. Drugs. 19, 261. doi:10.3390/md19050261
Sung, H., Ferlay, J., Siegel, R. L., Laversanne, M., Soerjomataram, I., Jemal, A., et al. (2021). Global cancer statistics 2020: GLOBOCAN estimates of incidence and mortality worldwide for 36 cancers in 185 countries. CA Cancer J. Clin. 71, 209–249. doi:10.3322/caac.21660
Suzuki, J., Tsuboi, M., and Ishii, G. (2022). Cancer-associated fibroblasts and the tumor microenvironment in non-small cell lung cancer. Expert Rev. anticancer. Ther. 22, 169–182. doi:10.1080/14737140.2022.2019018
Tamminga, M., Hiltermann, T. J. N., Schuuring, E., Timens, W., Fehrmann, R. S., and Groen, H. J. (2020). Immune microenvironment composition in non-small cell lung cancer and its association with survival. Immunology 9, e1142. doi:10.1002/cti2.1142
Tan, Z., Xue, H., Sun, Y., Zhang, C., Song, Y., and Qi, Y. (2021). The role of tumor inflammatory microenvironment in lung cancer. Front. Pharmacol. 12, 688625. doi:10.3389/fphar.2021.688625
Taverna, J. A., Hung, C. N., Williams, M., Williams, R., Chen, M., Kamali, S., et al. (2024). Ex vivo drug testing of patient-derived lung organoids to predict treatment responses for personalized medicine. Lung Cancer 190, 107533. doi:10.1016/j.lungcan.2024.107533
Tellez-Gabriel, M., Cochonneau, D., Cadé, M., Jubellin, C., Heymann, M. F., and Heymann, D. (2018). Circulating tumor cell-derived pre-clinical models for personalized medicine. Cancers (Basel) 11, 19. doi:10.3390/cancers11010019
Tepe, U., Aslanbay Guler, B., and Imamoglu, E. (2023). Applications and sensory utilizations of magnetic levitation in 3D cell culture for tissue Engineering. Mol. Biol. Rep. 50, 7017–7025. doi:10.1007/s11033-023-08585-0
Terrones, M., Deben, C., Rodrigues-Fortes, F., Schepers, A., de Beeck, K. O., Van Camp, G., et al. (2024). CRISPR/Cas9-edited ROS1 + non-small cell lung cancer cell lines highlight differential drug sensitivity in 2D vs 3D cultures while reflecting established resistance profiles. J. Transl. Med. 22, 234. doi:10.1186/s12967-024-04988-0
Thirusangu, P., Ray, U., Sarkar Bhattacharya, S., Oien, D. B., Jin, L., Staub, J., et al. (2022). PFKFB3 regulates cancer stemness through the hippo pathway in small cell lung carcinoma. Oncogene 41, 4003–4017. doi:10.1038/s41388-022-02391-x
Tiwari, A., Trivedi, R., and Lin, S. Y. (2022). Tumor microenvironment: barrier or opportunity towards effective cancer therapy. J. Biomed. Sci. 29, 83. doi:10.1186/s12929-022-00866-3
Vega, V. F., Yang, D., Jordán, L. O., Ye, F., Conway, L., Chen, L. Y., et al. (2023). Protocol for 3D screening of lung cancer spheroids using natural products. SLAS Discov. 28 (2), 20–28. doi:10.1016/j.slasd.2023.01.005
Vella, N., Estêvão, D., Cruz, T., Oliveira, M. J., Fenech, A. G., and Petroni Magri, V. (2024). Abstract LB176: targeting the PI3K/Akt/mTOR pathway in non-small cell lung cancer spheroids. Cancer Res. 84, LB176. doi:10.1158/1538-7445.AM2024-LB176
Wan, Z., Floryan, M. A., Coughlin, M. F., Zhang, S., Zhong, A. X., Shelton, S. E., et al. (2023). New strategy for promoting vascularization in tumor spheroids in a microfluidic assay. Adv. Healthc. Mater 12, e2201784. doi:10.1002/adhm.202201784
Wang, C., Yu, Q., Song, T., Wang, Z., Song, L., Yang, Y., et al. (2022). The heterogeneous immune landscape between lung adenocarcinoma and squamous carcinoma revealed by single-cell RNA sequencing. Signal Transduct. Target Ther. 7, 289. doi:10.1038/s41392-022-01130-8
Wang, H. M., Zhang, C. Y., Peng, K. C., Chen, Z. X., Su, J. W., Li, Y. F., et al. (2023). Using patient-derived organoids to predict locally advanced or metastatic lung cancer tumor response: a real-world study. Cell Rep. Med. 4, 100911. doi:10.1016/j.xcrm.2022.100911
Wang, J., Li, X., and Chen, H. (2020). Organoid models in lung regeneration and cancer. Cancer Lett. 475, 129–135. doi:10.1016/j.canlet.2020.01.030
Wang, L., Cao, L., Wang, H., Liu, B., Zhang, Q., Meng, Z., et al. (2017). Cancer-associated fibroblasts enhance metastatic potential of lung cancer cells through IL-6/STAT3 signaling pathway. Oncotarget 8, 76116–76128. doi:10.18632/oncotarget.18814
Wang, L., Li, X., Ren, Y., Geng, H., Zhang, Q., Cao, L., et al. (2019). Cancer-associated fibroblasts contribute to cisplatin resistance by modulating ANXA3 in lung cancer cells. Cancer Sci. 110, 1609–1620. doi:10.1111/cas.13998
Wang, M., Li, W., Sanchez Flores, R., Cai, L., Garciamendez-Mijares, C. E., Gill, S., et al. (2023). Bioprinted human lung cancer-mimics for tissue diagnostics applications. Tissue Eng. Part A 6, 270–279. doi:10.1089/ten.TEA.2023.0149
White, E. S. (2015). Lung extracellular matrix and fibroblast function. Ann. Am. Thorac. Soc. 12 (Suppl. 1), S30–S33. doi:10.1513/AnnalsATS.201406-240MG
Wieleba, I., Wojas-Krawczyk, K., Krawczyk, P., and Milanowski, J. (2022). Clinical application perspectives of lung cancers 3D tumor microenvironment models for in vitro cultures. Int. J. Mol. Sci. 23, 2261. doi:10.3390/ijms23042261
Wong, K. Y., Cheung, A. H., Chen, B., Chan, W. N., Yu, J., Lo, K. W., et al. (2022). Cancer-associated fibroblasts in nonsmall cell lung cancer: from molecular mechanisms to clinical implications. Int. J. Cancer. 151, 1195–1215. doi:10.1002/ijc.34127
World Health Organization (2023). Lung cancer. Available at: https://www.who.int/news-room/fact-sheets/detail/lung-cancer (Accessed April 19, 2024).
Worrell, J. C., and MacLeod, M. K. L. (2021). Stromal-immune cell crosstalk fundamentally alters the lung microenvironment following tissue insult. Immunology 163, 239–249. doi:10.1111/imm.13319
Wu, F., Fan, J., He, Y., Xiong, A., Yu, J., Li, Y., et al. (2021). Single-cell profiling of tumor heterogeneity and the microenvironment in advanced non-small cell lung cancer. Nat. Commun. 12, 2540. doi:10.1038/s41467-021-22801-0
Wu, Q., Wei, X., Pan, Y., Zou, Y., Hu, N., and Wang, P. (2018). Bionic 3D spheroids biosensor chips for high-throughput and dynamic drug screening. Biomed. Microdevices 20, 82. doi:10.1007/s10544-018-0329-x
Wu, Y., Zhou, Y., Qin, X., and Liu, Y. (2021). From cell spheroids to vascularized cancer organoids: microfluidic tumor-on-a-chip models for preclinical drug evaluations. Biomicrofluidics 15, 061503. doi:10.1063/5.0062697
Xu, M., Wang, Y., Duan, W., Xia, S., Wei, S., Liu, W., et al. (2020). Proteomic reveals reasons for acquired drug resistance in lung cancer derived brain metastasis based on a newly established multi-organ microfluidic chip model. Front. Bioeng. Biotechnol. 8, 612091. doi:10.3389/fbioe.2020.612091
Xu, Y., Wang, X., Liu, L., Wang, J., Wu, J., and Sun, C. (2022). Role of macrophages in tumor progression and therapy (Review). Int. J. Oncol. 60, 57. doi:10.3892/ijo.2022.5347
Xu, Z., Li, E., Guo, Z., Yu, R., Hao, H., Xu, Y., et al. (2016). Design and construction of a multi-organ microfluidic chip mimicking the in vivo microenvironment of lung cancer metastasis. ACS Appl. Mat. Interfaces 8, 25840–25847. doi:10.1021/acsami.6b08746
Yang, C., Xia, B. R., Jin, W. L., and Lou, G. (2019). Circulating tumor cells in precision oncology: clinical applications in liquid biopsy and 3D organoid model. Int. 19, 341. doi:10.1186/s12935-019-1067-8
Yang, M. H., Chang, K. J., Zheng, J. C., Huang, H., Sun, G. Y., Zhao, X. W., et al. (2017). Anti-angiogenic effect of arsenic trioxide in lung cancer via inhibition of endothelial cell migration, proliferation and tube formation. Oncol. Lett. 14, 3103–3109. doi:10.3892/ol.2017.6518
Yegya-Raman, N., Wang, K., Kim, S., Reyhan, M., Deek, M. P., Sayan, M., et al. (2018). Dosimetric predictors of symptomatic cardiac events after conventional-dose chemoradiation therapy for inoperable NSCLC. J. Thorac. Oncol. 13, 1508–1518. doi:10.1016/j.jtho.2018.05.028
Yokota, E., Iwai, M., Yukawa, T., Yoshida, M., Naomoto, Y., Haisa, M., et al. (2021). Clinical application of a lung cancer organoid (tumoroid) culture system. NPJ Precis. Oncol. 5, 29. doi:10.1038/s41698-021-00166-3
Yoshimoto, S., Taguchi, M., Sumi, S., Oka, K., and Okamura, K. (2023). Establishment of a novel protocol for formalin-fixed paraffin-embedded organoids and spheroids. Biol. Open 12, bio059882. doi:10.1242/bio.059882
You, J., Li, M., Cao, L. M., Gu, Q. H., Deng, P. B., Tan, Y., et al. (2019). Snail1-dependent cancer-associated fibroblasts induce epithelial-mesenchymal transition in lung cancer cells via exosomes. QJM 112, 581–590. doi:10.1093/qjmed/hcz093
Zeng, X., Ma, Q., Li, X. K., You, L. T., Li, J., Fu, X., et al. (2023). Patient-derived organoids of lung cancer based on organoids-on-a-chip: enhancing clinical and translational applications. Front. Bioeng. Biotechnol. 11, 1205157. doi:10.3389/fbioe.2023.1205157
Zhang, C., Tang, B., Hu, J., Fang, X., Bian, H., Han, J., et al. (2021). Neutrophils correlate with hypoxia microenvironment and promote progression of non-small-cell lung cancer. Bioengineered 12, 8872–8884. doi:10.1080/21655979.2021.1987820
Zhang, H., Jiang, H., Zhu, L., Li, J., and Ma, S. (2021). Cancer-associated fibroblasts in non-small cell lung cancer: recent advances and future perspectives. Cancer Lett. 514, 38–47. doi:10.1016/j.canlet.2021.05.009
Zhang, Y., Hu, Q., Pei, Y., Luo, H., Wang, Z., Xu, X., et al. (2024). A patient-specific lung cancer assembloid model with heterogeneous tumor microenvironments. Nat. Commun. 15, 3382. doi:10.1038/s41467-024-47737-z
Zhang, Z., Shiratsuchi, H., Lin, J., Chen, G., Reddy, R. M., Azizi, E., et al. (2014). Expansion of CTCs from early stage lung cancer patients using a microfluidic co-culture model. Oncotarget 5, 12383–12397. doi:10.18632/oncotarget.2592
Zhao, W., Wang, H., Zhang, X., Zhang, L., Pu, W., Ma, Y., et al. (2024). Effects of IFN-γ on the immunological microenvironment and TAM polarity in stage IA non-small cell lung cancer and its mechanisms. BMC Pulm. Med. 24, 46. doi:10.1186/s12890-023-02809-6
Zhao, Y., Guo, S., Deng, J., Shen, J., Du, F., Wu, X., et al. (2022). VEGF/VEGFR-targeted therapy and immunotherapy in non-small cell lung cancer: targeting the tumor microenvironment. Int. J. Biol. Sci. 18, 3845–3858. doi:10.7150/ijbs.70958
Zhao, Z., Chen, X., Dowbaj, A. M., Sljukic, A., Bratlie, K., Lin, L., et al. (2022). Organoids. Nat. Rev. Methods Prim. 2, 94. doi:10.1038/s43586-022-00174-y
Zheng, L., Wang, B., Sun, Y., Dai, B., Fu, Y., Zhang, Y., et al. (2021). An oxygen-concentration-controllable multiorgan microfluidic platform for studying hypoxia-induced lung cancer-liver metastasis and screening drugs. ACS Sens. 6, 823–832. doi:10.1021/acssensors.0c01846
Zheng, X., Hu, Y., and Yao, C. (2017). The paradoxical role of tumor-infiltrating immune cells in lung cancer. Intractable Rare Dis. Res. 6, 234–241. doi:10.5582/irdr.2017.01059
Zheng, X., Weigert, A., Reu, S., Guenther, S., Mansouri, S., Bassaly, B., et al. (2020). Spatial density and distribution of tumor-associated macrophages predict survival in non-small cell lung carcinoma. Cancer Res. 80, 4414–4425. doi:10.1158/0008-5472.CAN-20-0069
Zhu, L., Zhang, J., Guo, Q., Kuang, J., Li, D., Wu, M., et al. (2023). Advanced lung organoids and lung-on-a-chip for cancer research and drug evaluation: a review. Front. Bioeng. Biotechnol. 11, 1299033. doi:10.3389/fbioe.2023.1299033
Ziółkowska-Suchanek, I. (2021). Mimicking tumor hypoxia in non-small cell lung cancer employing three-dimensional in vitro models. Cells 10, 141. doi:10.3390/cells10010141
Keywords: 3D cell culture, lung cancer, tumour microenvironment, pre-clinical models, drug screening, drug development, precision oncopharmacology
Citation: Vella N, Fenech AG and Petroni Magri V (2024) 3D cell culture models in research: applications to lung cancer pharmacology. Front. Pharmacol. 15:1438067. doi: 10.3389/fphar.2024.1438067
Received: 24 May 2024; Accepted: 26 August 2024;
Published: 23 September 2024.
Edited by:
Nadia Milad, McMaster University, CanadaReviewed by:
Glaucia Maria Machado-Santelli, University of São Paulo, BrazilGamze Varan, Vaccine Institute of Hacettepe University, Türkiye
Copyright © 2024 Vella, Fenech and Petroni Magri. This is an open-access article distributed under the terms of the Creative Commons Attribution License (CC BY). The use, distribution or reproduction in other forums is permitted, provided the original author(s) and the copyright owner(s) are credited and that the original publication in this journal is cited, in accordance with accepted academic practice. No use, distribution or reproduction is permitted which does not comply with these terms.
*Correspondence: Anthony G. Fenech, YW50aG9ueS5mZW5lY2hAdW0uZWR1Lm10