- 1Electrical Engineering-ESAT, KU Leuven, Leuven, Belgium
- 2Imec, Leuven, Belgium
- 3Department of Psychiatry, Psychosomatics and Psychotherapy, Goethe University, Frankfurt am Main, Germany
- 4Department of Neurosciences, Psychiatry Research Group, KU Leuven, Leuven, Belgium
- 5University Psychiatric Centre KU Leuven, Leuven, Belgium
- 6OnePlanet Research Center, Wageningen, Netherlands
Background: Chronic stress and depressive symptoms have both been linked to increased heart rate (HR) and reduced HR variability. However, up to date, it is not clear whether chronic stress, the mechanisms intrinsic to depression or a combination of both cause these alterations. Subclinical cases may help to answer these questions. In a healthy working population, we aimed to investigate whether the effect of chronic stress on HR circadian rhythm depends on the presence of depressive symptoms and whether chronic stress and depressive symptoms have differential effects on HR reactivity to an acute stressor.
Methods: 1,002 individuals of the SWEET study completed baseline questionnaires, including psychological information, and 5 days of electrocardiogram (ECG) measurements. Complete datasets were available for 516 individuals. In addition, a subset (n = 194) of these participants completed a stress task on a mobile device. Participants were grouped according to their scores for the Depression Anxiety Stress Scale (DASS) and Perceived Stress Scale (PSS). We explored the resulting groups for differences in HR circadian rhythm and stress reactivity using linear mixed effect models. Additionally, we explored the effect of stress and depressive symptoms on night-time HR variability [root mean square of successive differences (RMSSD)].
Results: High and extreme stress alone did not alter HR circadian rhythm, apart from a limited increase in basal HR. Yet, if depressive symptoms were present, extreme chronic stress levels did lead to a blunted circadian rhythm and a lower basal HR. Furthermore, blunted stress reactivity was associated with depressive symptoms, but not chronic stress. Night-time RMSSD data was not influenced by chronic stress, depressive symptoms or their interaction.
Conclusion: The combination of stress and depressive symptoms, but not chronic stress by itself leads to a blunted HR circadian rhythm. Furthermore, blunted HR reactivity is associated with depressive symptoms and not chronic stress.
Introduction
Depression is associated with pronounced autonomic nervous system abnormalities. Patients with Major Depressive Disorder (MDD) show higher baseline levels of heart rate (HR) and altered heart rate variability (HRV) [e.g., lower Standard Deviation of Normal-to-Normal Intervals (SDNN), Root Mean Square of Successive Differences (RMSSD), low-frequency and high frequency (HF) HRV] (1–3). These baseline differences are paired with blunted reactivity during exposure to acute and repeated psychological stress (2–5). Furthermore, differences of HR and HRV at baseline extend to abnormalities of HR circadian rhythm in severe depression (6, 7). A circadian rhythm is an oscillation of a process over 24 h. HR, for example, rises strongly in the morning, reaches a peak before noon, decreases again and reaches another peak in the afternoon, after which it keeps on lowering during the night until the next morning (8). Abnormalities within this rhythm may be used to discriminate between cases and controls (4, 9). Indeed, it is well known that patients with MDD show altered circadian rhythms and diurnal mood variation (10). Analyzing HR/HRV, particularly during the night period may provide more insight into potential pathological mechanisms.
Interestingly, the association of depressive symptoms and HR/HF-HRV is not as clear-cut. Depressive Recently, Jarczok and colleagues showed that depressive symptoms were associated with reduced 24-h cardiac vagal variation in men, but increased variation in women (11). Interestingly, in their large-scale longitudinal study, Jandackova et al. (12) did not find a cross-sectional association between HR/HRV measures and depressive symptoms at baseline. However, in a predictive analysis, the authors showed that lower HR and higher HF-HRV predicted a lower likelihood of depressive symptoms at follow-up, 10 years later. Inversely, the presence of depressive symptoms at baseline was not associated with HR/HRV measures at follow-up (12). Similar findings have been reported by others: It seems that particularly higher levels of HRV are indicative of a better outcome to treatment [e.g., (13)]. It should also be noted, that resting state HR/HRV alterations do occur in various other psychiatric [e.g., post-traumatic stress disorder (14), anxiety disorders (15)] and somatic [e.g., type 2 diabetes (16), fibromyalgia (17)] disorders and should not be understood as a diagnostic biomarker, but rather as general risk assessment that if combined with other measures, could be helpful in identifying at-risk patients.
While resting state physiological data are informative, functional data provides important information on the capacity to adapt to environmental demands. Stress reactivity studies in healthy people with depressive symptoms are ambiguous: increased (HR) reactivity (18), decreased reactivity (19), or no changed reactivity to a mental stressor (20, 21) have been reported.
A big caveat of the above studies, and possibly a reason for the heterogeneous findings, is that they did not account for the effects of chronic stress. Amongst other factors which can influence HR and HRV readouts, such as age and sex (22), poor cognitive function (23, 24), mental fatigue (25), metabolic syndrome (26), temperature (27), and possibly, other lifestyle factors [e.g., physical activity, alcohol use, smoking (22)], chronic stress may increase HR reactivity and/or decrease HRV (28, 29), although some reports did not find an effect (22). Chronic stress is also a well-known risk factor for psychopathology: the experience of chronic stress (i.e., the real or perceived threat to an individual’s psychological or physiological integrity) (30, 31) has been linked to both the development (32) and the maintenance (33) of several mental disorders, one of which is MDD. In contrast to the blunted stress reactivity observed in MDD, high perceived stress and/or negative life events may increase HR reactivity to stress (34). Changes in HR/HRV may thus represent the consequence of chronic stress, which can precede the development of depressive symptoms [e.g., (12, 29)]. Depression and/or depressive symptoms are often associated with the subjective experience of chronic stress (35); the direction of causality, i.e., whether chronic stress precedes depression or whether depression leads to high subjective stress, is unclear and may vary between individuals.
As initially proposed by McEwen, the identification of subclinical cases before emergence of psychopathology may be key to answer these questions (30). Therefore, we set out to investigate the effects of chronic stress on circadian rhythms and stress reactivity in the Stress in the Work Environment (SWEET) study, which studies a large sample of individuals from the healthy working population. The participants were specifically selected to have (a) no chronic stress and no depressive symptoms, (b) chronic stress but no depressive symptoms, (c) depressive symptoms but no chronic stress, and finally (d) depressive symptoms and high chronic stress.
We aim to investigate whether the effect of chronic stress on HR circadian rhythm is (a) dose dependent and (b) different in participants with, compared to participants without depressive symptoms. We hypothesize that chronic stress and depressive symptoms gradually increase basal HR levels, and that the combination of both, high chronic stress and depressive symptoms results in a biological profile similar to patients with depression. Secondly, we investigated if chronic stress and depressive symptoms alter HR reactivity to an acute stressor. We hypothesize that high depressive symptoms are associated with blunted stress reactivity, while high stress is associated with increased stress reactivity.
Materials and methods
Participants
This study is part of the Stress in the Work Environment study (SWEET-study; n = 1,002) described in Smets et al. (36), which was approved by the Ethics Committee Research UZ/KU Leuven (approval number: S57916). Participants were recruited in 11 technology-oriented, banking, and public sector companies. Participants with heart disease or any psychiatric disorder based on self-report were excluded from analysis. Participants who did not report a psychiatric disease but were currently taking psychotropic medication were also excluded. Cholesterol-lowering supplements or medication was permitted if no heart disease was specified. Participants did not receive any reward for participating in the study apart from having a chance at winning a restaurant or travel voucher.
Procedures
Questionnaires
Before the experiment, participants completed an intake questionnaire which gathered personal information, such as age, sex, health problems, work situation, and lifestyle, and four psychological questionnaires: The Perceived Stress Scale with 10-items (PSS-10), the Pittsburgh Sleep Quality Index (PSQI), and the Depression Anxiety Stress Scale with 21 items (DASS-21).
Ambulatory monitoring
On Thursday morning, participants received a chest patch [hardware system of (37)] which received regulatory approval and measures the electrocardiogram (ECG) and tri-axis acceleration (Acc) at a sampling rate of 256 and 32 Hz, respectively. Though higher sampling rates are preferred in HRV research, a sampling rate of 256 Hz has been reported as sufficient (38) and was preferred in this large-scale ambulatory study to limit memory and storage issues. Participants were asked to wear the chest patch day and night for a total of 5 days (Thursday–Monday morning) and to remove it only during vigorous physical activities. For more details on the data collection, see Smets et al. (36).
Stress task
On the first day (Thursday) of the experiment, participants were asked to complete a modified version of the Montreal Imaging Stress Task (MIST) (39) to induce moderate stress. The MIST (hereafter referred to as stress task) consists of a resting, a training, and an experimental condition. In this study, participants completed the task on a mobile app: a 5-min rest period (relaxing music and images), a 5-min training period, a 5-min stress task and a 5-min recovery period. For details on the task, see Supplementary Information 1.
Data pre-processing
The mean HR(V) and activity levels were calculated in windows of 5 min with 4 min of overlap, analog to Schiweck et al. (4) and Smets et al. (36), following the minimum window length required to calculate HRV features. The activity level of the participants was calculated as the standard deviation of the magnitude of acceleration (Std Acc) as derived from the accelerometer of the ECG patch. As ambulant physiological recordings may be corrupted by motion artifacts and poor sensor attachment, the acceptability of the recorded ECG was assessed using a quality indicator (QI) derived from Orphanidou et al. (40) and previously implemented by Smets et al. (36). For details see Supplementary Information 2. The HR(V) data was filtered using the QI. All 5 min windows with an average QI below 0.8 were excluded. In addition, all segments of high activity (Std Acc > 0.04) were excluded to obtain a clear result without the confounding effect of physical activity. Finally, all retained windows were averaged into hourly measurements.
The ECG data recorded during the stress task was divided into windows of 1 min, without any overlap, from which the mean HR was calculated. Windows with an average QI below 0.8 were excluded. The retained windows were averaged per condition of the stress task.
Statistical analysis
All statistical analysis was performed in R version 3.6.1 (41). Graphical representations were performed with Python version 3.7. Relevant variables were age, biological sex, hours of physical activity per week, smoking behavior, DASS scores, and PSS scores. For simple group comparisons, the Wilcoxon rank sum test was performed. For multiple group comparisons, the Kruskal-Wallis test was used. If significant, it was followed by Dunn’s test with a Benjamini-Hochberg correction. Missing weight or length data was imputed by the median of the included population. Hours of physical activity per week was enquired as the total hours of sports performed per week with 5 suggested categories: 0, 0–1h, 1–3h, 3–5h, and > 5 h. These values were transformed into a numeric variable ranging from 0 to 4. For circadian variation, recordings were required to include an average HR for at least every hour of a 24 h day (i.e., a minimum of 24 datapoints without constraints for within-day consecutiveness), in which the hourly average was derived from at least 5 data windows. If this requirement was violated, the corresponding participant was excluded. For stress reactivity analyses during the stress task, only participants who completed the MIST and for whom physiological recordings were of sufficient quality were included.
Definition of depression and stress subgroups
To define our population of interest, with and without symptoms of depression and low or high stress, respectively, we used the DASSD [Cronbach’s alpha: 0.81 (42)] and PSS scores [Cronbach’s alpha: 0.78 (43)]. First exploratory dimensional analyses, using HR averages per day and night, indicated the possible importance of second order terms in the PSS and/or DASSD (see Supplementary Table 2).
Since several model terms were already used to model circadian rhythm, we decided against further dimensional analyses, as these would require the inclusion of second order terms for both the PSS and DASSD in interaction with all the harmonic terms. To preserve interpretability and allow for non-linear relationships, we opted for group-based analyses.
The DASSD was used to define groups experiencing no or only low depression scores, and those with high levels, following the official cut-off score of 6 as recommended by Lovibond and Lovibond (44). We opted for a binary distribution due to the expected, non-normal distribution of depression scores in a working population which was confirmed by a skewness of 1.67 and kurtosis of 6.33 within the DASSD (skewness > 1, the distribution is heavily skewed; kurtosis > 3, the distribution has heavier tails than a normal distribution). Regarding the PSS, several cut-offs are mentioned in the literature, but since the PSS is not a diagnostic instrument, no validated cut-offs are given. Given our interest in the difference between low/normal, medium/high, and extreme stress (often present in depression), participants were grouped into three categories using our own, population-derived cut-off values: (1) normal stress levels (lower or equal to the 75th percentile of the PSS scores), (2) high stress levels (between the 75th and the 95th percentile), and (3) very high stress (above the 95th percentile). This corresponded to a PSS value of < 18 for normal stress, between 18 and < 24 for high stress or > 24 for extreme stress. These values are congruent with the values published by Cohen and Janicki-Deverts (45) in their large survey of the normal population using the PSS-10: Mean PSS scores in 2009 were situated around 17 (16.38–17.46) with a standard deviation of around 7 (7.07–7.83) for the population aged 25–54, which is comparable to our sample.
Circadian rhythm
Model development for heart rate
We performed a group-based time-series analysis in which we studied HR, for every group, at consistent time intervals, i.e., 1 h, while modeling time dependencies between the intervals. For this, we used a linear mixed model, predicting HR with the independent variables age, sex, Body Mass Index (BMI), smoking, depressive symptom group, and chronic stress group. The hourly average activity level (Std. Acc), hereafter referred to as the activity index, was also included as a covariate to control for HR recovery following (the excluded) periods of high physical activity. All numerical variables were rescaled to range from 0 to 1. As HR shows diurnal fluctuations (8, 46), we introduced harmonic terms with periods of 24, 12, 8, and 6 h as described in the literature (47, 48). Via interactions with the factor groups, these terms allow for the estimation of group-related diurnal differences. A random intercept was included per subject, as well as random slopes for all harmonic terms. The latter allow us to model person-to-person variability. Due to slight deviations from model assumptions, HR data was log transformed. As the effect of sleep quality was not of primary interest, its relevance was only tested in the final model after the model selection procedure. In a second step, separate models were built for healthy participants with high and low levels of depressive symptomatology to investigate whether differential effects were present.
Model development for heart rate variability
Regarding HR variability, we aimed at studying vagal modulation, as this has been linked with stress vulnerability and reactivity (49, 50) and depression (51). Cardiac vagal tone can be approximated through the HF variations of the RR interval, which primarily reflects the activity of the parasympathetic nervous system (52). The root mean squared successive differences (RMSSD) is a time domain measure that represents short-term variation, and therefore correlates with the power in the HF band. For long-term, ambulatory ECG recordings, it has been recommended to look into RMSSD rather than the HF-HRV (53). Since we were not able to control for respiratory measures and RMSSD is known to be less influenced by respiration than HF-HRV (54), we chose RMSSD as outcome.
Pre-processing revealed that the day-to-day variability within RMSSD was substantially higher than within HR (see Supplementary Table 1 for the comparison). This might have been a consequence of the ambulatory set-up, in which the ECG recording is subject to artifacts and noises: baseline wandering, electromyogram interference, powerline interference, and motion artifacts. As HRV measures require high temporal accuracy for R-wave peak identification, part of the variability may have originated from poor signal quality. Consequently, the circadian model, as explained in the previous section, did not reach good model fit. To reduce the influence of motion, we limited the analysis of RMSSD to night-time analyses (from 10 p.m. to 6 a.m.).
Similar to the HR analysis, we performed a group-based time-series analysis using a linear mixed effects model. In this model, we predicted the hourly average for RMSSD with the independent variables age, sex, BMI, smoking, activity index, depressive symptom group, and chronic stress group. In contrast to the model for HR, we added time directly to the model, without harmonic terms. Interactions between the time variable and the factor groups allowed for the estimation of group-related differences in night-time recovery, i.e., the night-time slope of RMSSD. The variable time ranged from 1 to 8, representing the hours between 10 p.m. and 6 a.m. A random intercept was included per subject, as well as a random slope for time. Due to slight deviations from model assumptions, RMSSD data was log transformed.
Model selection
For HR, a final model was selected using backward stepwise elimination based on the Akaike information criterion (AIC). The AIC is an estimator of prediction error which promotes models with a high goodness-of-fit and penalizes overly complex ones (55). The stepwise elimination process was performed using the R-package “buildmer” with the parameter “crit” set on “AIC” and the parameter “direction” set on the default combination (“order, backward”) to first make sure that the model converges and to then perform backward elimination (56). The retained variables were finally tested in the R-package “lme4.” Importantly, in contrast to the traditional cut-off based on a p-value of 0.05, the AIC-based model selection allows for the inclusion of variables with a p-value above the significance level of 0.05 if these variables improve the goodness-of-fit of the model significantly. With regard of RMSSD, we did not perform a stepwise elimination but estimated the full model.
Stress reactivity
In a linear mixed model, we predicted HR with the independent variables: depressive symptom group, chronic stress group and stress exposure. A random intercept was included per subject. To investigate the effect of depressive symptoms and chronic stress on stress reactivity, both group factors were added in interaction with the stress exposure. No stepwise elimination was performed. Per grouping, the conditions were compared in a pairwise manner while using a Benjamini-Hochberg correction. We did not analyze reactivity in RMSSD for the reasons explained in section “Model development for HR variability.”
Results
Sample characteristics
A total of 1,002 participants took part in the SWEET study. Among these participants, 104 did not have any (good quality) ECG data and 181 did not have enough data, i.e., at least one data point for every hour in a day, to perform circadian modeling. This data loss found its origin in several issues inherent to ambulatory data collection such as participant drop-out within 24 h, poor signal quality because of bad sensor adhesion or vigorous motion and sensor failure because of water damage (e.g., while showering). An additional 153 participants did not fill in the questionnaires and 48 participants were removed from the data set based on the exclusion criteria [n = 13 psychiatric disorders (anxiety, ADHD, depression, or other)], n = 2 intake of psychotropic medication without diagnosis, n = 17 heart diseases with medication intake such as beta-blockers, n = 15 heart diseases without medication intake, n = 1 chronic disease (neuropathy), resulting in a total of 516 participants included for analyses [mean age = 39.44 (SD 10.21), mean BMI = 24.20 (SD 3.78), number of women = 243 (47%), mean PSS = 14.21 (SD 6.01), mean DASSD = 2.50 (SD 2.91), mean HR = 67.87 BPM (SD 7.53)]. Participants were mostly highly educated (34.9% graduate school, 7.3% secondary school, 57.8% university). On average, participants had 83.8 (SD 17.7) hourly averages of valid physiological recording spread over the entire period of approximately 104 h (Thursday morning 9 a.m. to Monday evening 5 p.m.). The group of participants who were excluded because of insufficient ECG data after pre-processing (n = 285) did not differ significantly in age, sex, smoking behavior, education, PSS, and DASSD from the final group. However, the final group reported higher levels of physical activity than the excluded group (p-value = 0.035).
Stress subgroups
Three hundred ninety participants (75.59%) had low to normative chronic stress levels (PSS ≤18) (hereafter: normative stress group), 106 (20.54%) had moderate to high chronic stress levels (18 < PSS 24) (hereafter: high stress group) and 20 (3.86%) had extreme chronic stress (PSS > 24) (hereafter: extreme stress group). Post hoc comparison using Benjamini-Hochberg corrections indicated that the high stress group performed fewer hours of physical activity per week and had a significantly higher HR than the normative stress group (z = 2.564, p = 0.031 and z = 2.505, p = 0.037, respectively). Fewer women were in the normative stress group compared to the other groups (normative—high; p = 0.002 normative—extreme; p = 0.011). The normative stress group also indicated better sleep quality than the other groups (normative—high and normative—extreme; p < 0.001). All groups differed in their DASSD scores (high—extreme; p = 0.015, other; p < 0.001). No other variables differed significantly between groups. Sample characteristics and statistical tests for stress subgroups can be found in Supplementary Table 3.
Depression subgroups
Four hundred sixty (89.15%) participants scored below the cut-off for depressive symptoms and 56 (10.85%) scored above the cut-off for depressive symptoms. No significant differences were present for age, sex, BMI, smoking behavior, HR, and Std. Acc. Participants high in depressive symptoms performed significantly less sport per week (W = 14936, p = 0.042), had significantly different PSS scores (W = 4502, p < 0.001) and significantly poorer sleep quality (W = 7204.5, p < 0.001). Of the participants with low depressive symptoms, the majority had normative stress levels (n = 371, 80.65%), a smaller subset had high (n = 79, 17.17%) and few participants had extreme (n = 10, 2.17%) stress levels. In contrast, the majority of the participants with high depression scores also had high stress levels (n = 27, 48.21%). Nineteen participants within this group (33.93%) had normative stress levels and 10 (17.86%) had extreme stress levels. An overview of the sample characteristics for these combined groups can be found in Table 1, sample characteristics and statistical tests for subgroups separately can be found in Supplementary Table 4.
Stress task
A subset of the participants completed the MIST (n = 194). This subset did not differ significantly from the overall sample regarding age, BMI, sex, PSS, and DASSD (p > 0.05) Within the subset, 180 participants had low depression scores and 14 high depression scores. Regarding chronic stress, 152 participants had normative stress levels (PSS 18), while 42 participants had high or extreme stress levels (PSS > 18). Given the low number of participants, we decided against further subgrouping of the high stress group. Sample characteristics of respective subgroups can be found in Supplementary Tables 5, 6.
Circadian rhythm is influenced by chronic stress in healthy participants
Figures 1A,B present circadian variations in HR for participants with low and high depression scores, respectively. The final model (AIC = −84655.2, LogLik = 42396.6, and R2conditional = 0.75) retained several significant covariates (see Supplementary Table 7 for a detailed overview of estimates), a main effect for chronic stress, and a three-way interaction for the 8-h harmonic regression term, chronic stress, and depressive symptoms (main effect stress: F = 2.849, p = 0.059, interaction: F = 2.489, p = 0.084). Given the significant interaction and contrasts (b = 0.018, se = 0.008, t = 2.184, p = 0.030), we opted for split-group analysis by depression category to further examine the effects of chronic stress.
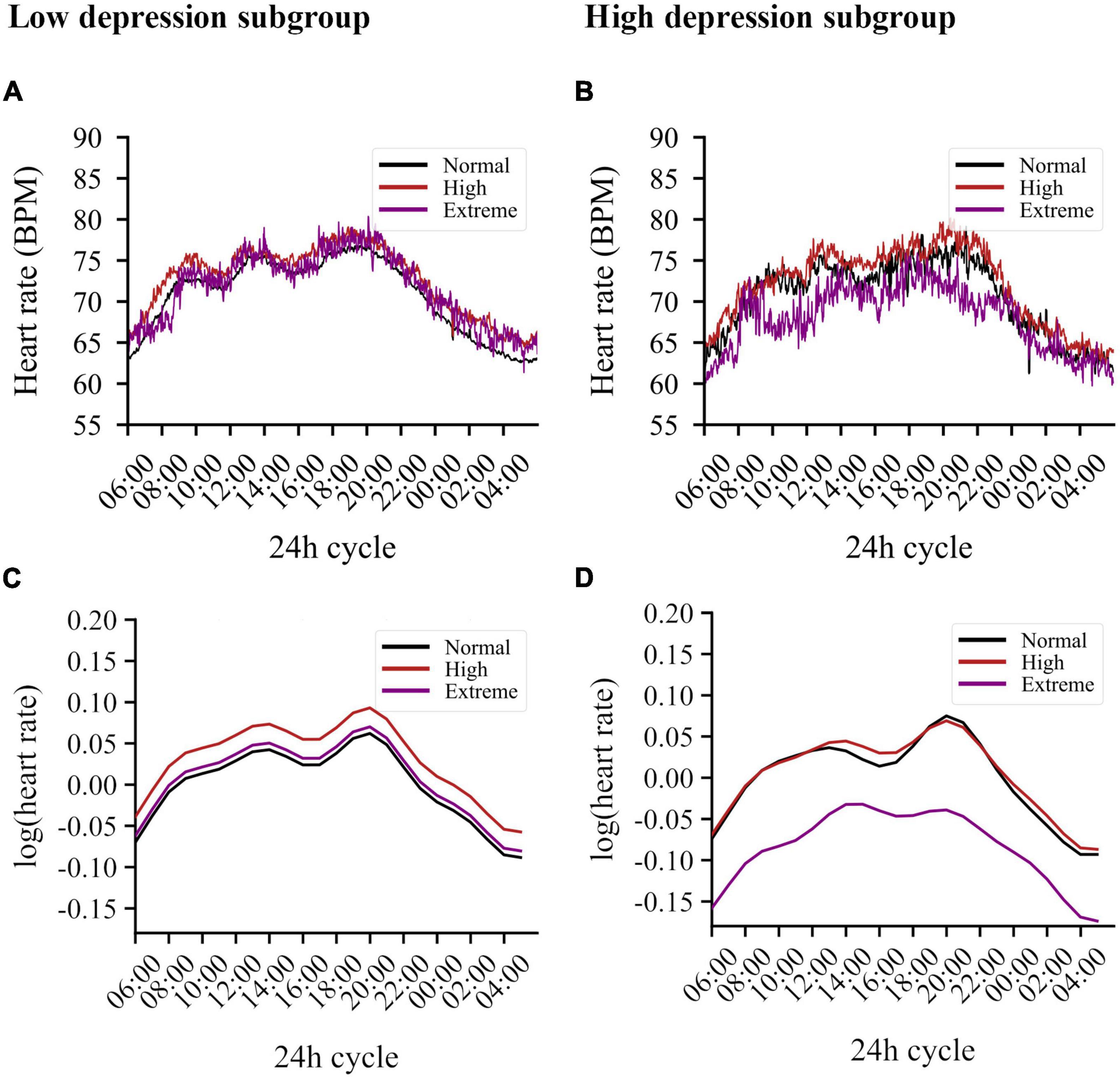
Figure 1. Circadian variations in heart rate averaged per stress subgroup for both low and high depression scores. (A) Averaging results for the low depression subgroup. Chronic stress levels are indicated by color: normative levels in black, high levels in red, extreme levels in purple. (B) Averaging results for the low depression subgroup. (C) Simulation results derived from the model for the low depression subgroup. The graph illustrates the log-transformed HR derived from this model using only the harmonic and stress-related terms, therefore, without the influence of BMI, sex, activity, and smoking behavior. Participants with high levels of chronic stress show the highest increase in HR. (D) Simulation results derived from the model for the high depression subgroup. Whereas the negative main effect of the 12 h-based sine wave causes an increase in the morning and evening, the positive interaction of this wave with extreme stress counteracts this, resulting in a diminished evening peak and an elevated plateau in the afternoon. Similarly, the positive interaction including the 8 h-based cosine wave counteracts the negative main effect of this wave, resulting in, among other effects, an additional reduction of the evening peak in participants with extreme stress.
In the group with low depression scores (n = 460), high chronic stress was associated with higher basal HR levels (b = 0.031, se = 0.013, t = 2.318, p = 0.021) when compared to normative stress. HR in the group with extreme stress did not differ from the normative stress group (see Figure 1C). Important covariates in this model were BMI (p < 0.001), sex (p < 0.001), activity index (p < 0.001), and smoking (p = 0.073). See Supplementary Table 8 for a detailed overview of estimates.
For the group with high depressive symptoms (n = 56), chronic stress also had a significant main effect on the circadian rhythm (F = 3.644, p = 0.026). However, in contrast to the low depressive symptom group, individuals with extreme stress had lower HR (b = −0.084, se = 0.035, t = −2.408, p = 0.016) compared to the normative stress group, while high stress did not change basal HR. In addition, extreme stress altered the circadian rhythm significantly [extreme stress*sin (h), b = 0.022, se = 0.010, t = 2.176, p = 0.030; and extreme stress*cos (h), b = 0.011, se = 0.005, t = 2.136, p = 0.033]. These effects resulted in blunted circadian variability of HR in the extreme stress group, noticeable by the elevated plateau in the afternoon and the diminished evening peak. People with high depressive symptoms and high chronic stress show no difference in HR compared to the normative chronic stress group (with high depressive symptoms), see Figure 1D for the simulated regression line. Several covariates were retained: sex (p < 0.001), activity index (p < 0.001), and smoking (p = 0.025). Age and BMI were not retained in the model. See Supplementary Table 9 for a detailed overview of estimates.
When hours of physical activity performed per week was included, the models differed slightly. The altered models are presented in Supplementary Tables 10–12. In the overall model, the p-value for the main effect of high chronic stress was reduced to a trend for significance (p = 0.081). In the group model for low depressive symptoms, chronic stress was dropped after model selection.
The model for night-time RMSSD reached moderate model fit (AIC = 9796.1, LogLik = −4876.0, R2 = 0.63), but did not show any significant group effects. Only age, BMI, activity index and the time-point were significant predictors (see Supplementary Table 13). Though insignificant, we explored possible trends for the group effects. RMSSD baseline scores showed a positive effect for stress (high stress: b = 0.041, se = 0.047, t = 0.863, p = 0.389; extreme stress: b = 0.177, se = 0.120, t = 1.474, 0.141) and a positive effect for depressive symptoms (b = 0.088, se = 0.088, t = 1.000, p = 0.318). However, the interaction of chronic stress groups (both high and extreme) and depressive symptoms had a reducing effect when combined (high stress × dep.: b = −0.099, se = 0.122, t = −0.814, p = 0.416; extreme stress × dep.: b = −0.210, se = 0.189, t = −1.109, p = 0.268). This suggests that the combination of chronic stress and depressive symptoms has a different effect on RMSSD than their mere summation. Regarding the slope in night-time RMSSD, all group effects had a negative or near-zero positive estimate, and general trends were not established.
Depressive symptoms are associated with lower stress reactivity, chronic stress with higher stress reactivity
For the overall group, reactivity to the stress task (MIST) revealed a significant main effect for the stress task (F = 4.289, p = 0.005) with increased HR during stress compared to baseline (b = 0.028, se = 0.005, t = 5.625, p < 0.001). While the overall ANOVA showed no significance for the interactions of chronic stress or depression group with stress exposure for the overall group (F = 1.868, p = 0.134 and F = 1.886, p = 0.131), the summary output of the model at the factor level showed that chronic stress and depressive symptoms had opposed effects: high chronic stress was associated with slightly higher HR during stress exposure (b = 0.025, se = 0.011, t = 2.274, p = 0.023), while high depressive symptomatology modestly reduced stress reactivity (b = −0.037, se = 0.018, t = 2.104, p = 0.036). See Table 2 for a detailed overview of estimates.
Figure 2 shows the pairwise comparison within depressive symptoms groups (A) and within chronic stress groups (B). Participants with low depressive symptoms had significantly higher HR during stress (pairwise contrasts compared to baseline b = 0.032, p < 0.001; and to the training phase b = 0.024, p < 0.001) and showed significant recovery after the stressor (pairwise contrast: b = −0.031, p < 0.001). However, participants with high depressive symptoms showed no significant reactivity or recovery to the stressor for any period (pairwise contrasts all p > 0.470). The groups did not differ significantly in HR during the baseline condition (Supplementary Table 4). Estimates per model term are presented in Supplementary Table 14, individual results and characteristics can be found in Supplementary Table 15 and Supplementary Figure 1 regarding the participants with depressive symptomatology. Regarding chronic stress, both participants with normative chronic stress and high chronic stress showed a significantly increased HR during the stress task in comparison to baseline and recovery (Supplementary Table 16).
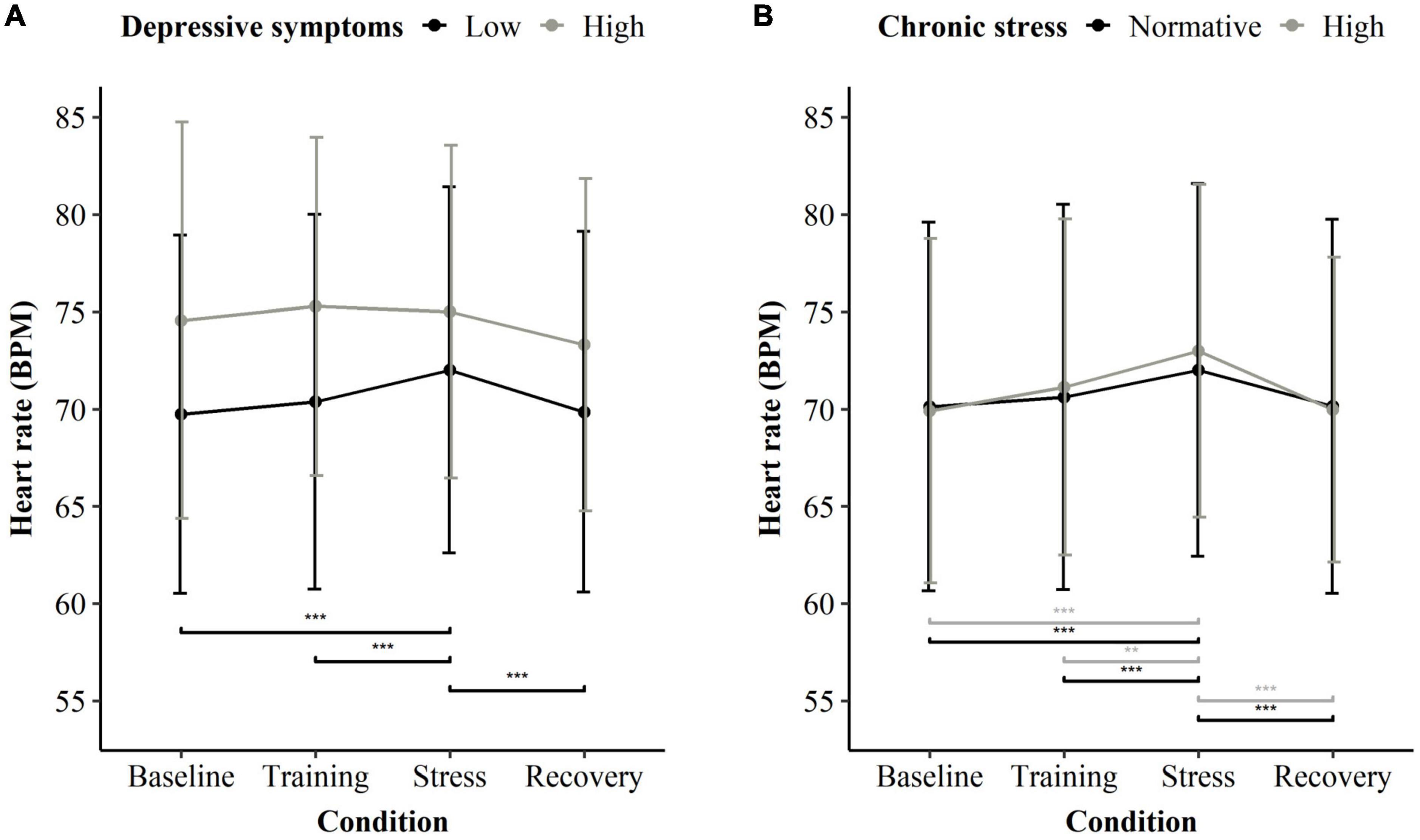
Figure 2. Heart rate averaged per condition of the stress exposure (MIST) and stress/depression subgroup. (A) Mean heart rate for participants low in depressive symptoms (black), n = 180, and high in depressive symptoms (gray), n = 14. All participants, regardless of their chronic stress level, were considered. (B) Mean heart rate for participants with normative chronic stress (black), n = 152, and high chronic stress (gray), n = 42. All participants, regardless of their depressive symptoms, were considered. Significant differences are indicated in a pairwise manner between baseline, stress, recovery, and within subgroups. **p < 0.01 and ***p < 0.001.
Discussion
We here present results from the SWEET study, which allowed us to assess the cumulative effect of stress and depressive symptoms on HR circadian rhythm, night-time RMSSD and HR in response to an acute mental stressor.
We show that chronic stress does not simply lead to an increase in HR but is graded and varies in function of depressive symptoms. Without depressive symptoms, high chronic stress modestly increases basal HR but has no effect on circadian rhythm. In contrast, in those with depressive symptoms, high chronic stress does not change basal HR, nor circadian rhythm; however, the few participants experiencing extreme chronic stress and depressive symptoms showed a lower overall HR paired with an atypical, flattened circadian rhythm variation. In the MIST task, depressive symptoms were associated with blunted HR reactivity while chronic stress with higher HR reactivity. Although this finding must be considered preliminary due to the small group size (n = 10), it may have important implications for pathophysiology of depression. In addition, we did not find any significant effects of depressive symptoms and/or stress on RMSSD during the night. Blunted stress reactivity has previously been linked to depression (57), and chronic stress has been hypothesized to be a major factor in the pathogenesis of depression (58). However, given the largely overlapping effect of both, it was previously difficult to show this relationship experimentally. Here, we show that blunted HR reactivity is explained by depressive symptoms and not exposure to high chronic stress when including both in the analysis.
Others have previously shown that 24-h HR is positively correlated with (work-related) stress (29, 59), and have reported associations with various HRV measures (28, 59–61). However, to our knowledge, these studies have mostly used average HR over 24-h intervals and assessed either stress or depressive symptoms, but not both. Few studies specifically assessed circadian rhythm in HR or HRV, some of which assessed chronic stress in shift workers (62, 63), or mood disorders (64), or depressive symptoms (11, 65). In other parameters than HR and HRV, there has been ample research on circadian alterations in depression. The most consistent circadian abnormalities that have been described are changes in daily mood variation, brain activity, core body temperature, hormone secretion, sleep–wake cycle, motor activity, and seasonal mood variation (66). Most studies reported evidence for a blunted amplitude of the circadian rhythm (10, 67). Though, again, no grading by stress levels was examined.
Surprisingly, and somewhat opposed to our hypothesis, in those with depressive symptoms and extreme chronic stress, overall HR was lower, and decreased variability of the HR circadian rhythm was observed, but chronic stress and depressive symptoms were not associated with night-time changes in RMSSD. Regarding the findings on RMSSD, we here only analyzed night-time data and found no difference. Previously, it has been reported that patients with depression had lower RMSSD during sleep compared to those without MDD, although this relationship was partially explained by anti-depressant use (68). The here studied group did not take any anti-depressant medication, and only had depressive symptoms and effects were negligible. As the extreme stress group with depressive symptoms only comprised 10 people, the difference (or lack thereof for RMSSD) might be attributable to this small sample size. It is important to bear in mind that only very few of our healthy participants with extreme stress reported low depressive symptoms (i.e., ∼2%). The co-occurrence of high depressive symptoms and extreme chronic stress is much more common (i.e., ∼18%), be that due to direct relationship or through association with a secondary factor (such as altered cognition). The here analyzed sample of stress and low depressive symptoms is thus quite rare and albeit a small sample, it allowed us to gather preliminary data on the intriguing relationship between stress and depressive symptoms.
The allostatic load model posits that accumulated stress exposure leads to wear and tear of the body (69). Following this model, in depressive illness, there is an exhaustion of the stress system, leading to, among others, a dysregulation of the cardiovascular system (69). The latter potentially results in the observed alterations of circadian HR. It is also possible that depressive symptoms are the consequence of unresolved, chronic stress exposure (in absence of protective factors), over a sustained time-period. It has been suggested that dysregulation of the circadian system increases susceptibility to depression (70, 71). Such dysregulation might be the result of chronic stress exposure, as the circadian system interacts with stress-related neurotransmitter systems, including serotonergic neurotransmission. Therefore, a stress-induced change within the serotonin system may cause circadian dysfunction and increased vulnerability to depression (12, 72). Given that circadian misalignment is associated with decreased cardiovascular health (73), clinicians should thus be on the lookout for depressive symptomatology in patients who report high levels of stress.
It should be noted that adding hours of physical activity to our model reduced the amount of variance explained by high chronic stress. It is well known that higher physical activity is linked to resilience and better mental wellbeing (74) and inversely, that chronic stress may reduce physical activity (75), and thus these results are hardly surprising. Physical activity is also effective in reducing depressive symptoms (76). Our results may thus also point toward this direction, albeit formal analyses for moderating effects of this relationship were not conducted. Additionally, we found that participants with depressive symptoms have lower sleep quality. Sleep quality has been described as an important factor linking chronic stress and depression, potentially by moderating the effect between both (77). In their study, da Estrela et al. (77) showed that lower resting HRV was linked to poorer sleep quality. Furthermore, sleep disturbances after stress exposure have been linked to depressive symptoms (78, 79). It is a known fact that sleep is highly important for regulating physiological functions such as HR (80), and therefore it could also be that the effects we observed here are moderated through sleep quality differences. Although beyond the scope for this article, future studies could elucidate this relationship further. Lastly, in this population, the high stress groups tended to include more women than men. This has been reported before in studies using the PSS (81), as well as using other psychometric scales (82). Recently it was shown that in men, depressive symptoms are associated with lower circadian variation in vagal activity, but an opposite trend was observed in women (11). We attempted to limit sex-related biases by adding sex as a covariate to the baseline of the circadian model, but stratified analyses were not possible due to the small number of participants in the subgroups.
Of particular interest is our finding regarding stress reactivity in this healthy population. Ample research exists on HR stress reactivity in control participants [e.g., (83)], participants with depressive symptoms [see Hamilton and Alloy (57) for an overview] and in depressed patients [see Schiweck et al. (84) for an overview]. Here, depressive symptoms were associated with a blunted reactivity, but chronic stress was linked to higher reactivity. It is of course possible, that other confounding factors play a role in this relationship between blunted reactivity and depressive symptoms: next to the above-mentioned individual factors such as experience of childhood adversity (85), obesity and poor cognitive functioning (23), motivation (86), hours of sleep/sleep quality (87), and preference of “eveningness” may be associated with a blunted cardiovascular response (88). Alternatively, a true association between depressive symptoms and blunted HR reactivity is possible and has been reported in healthy populations before [e.g., (89, 90)], also for other measures: for instance, cortisol reactivity to a naturalistic stressor was abolished in those with high depressive symptoms (91).
With regard to chronic stress, literature has also identified chronic stress to influence HR reactivity to stress [e.g., (92, 93)]. In previous studies it was difficult to disentangle the effects of high chronic stress levels and depressive symptoms, since most patients with depression experience elevated stress levels, and increased stress levels in the population are also associated with an increased likelihood of depression. In light of the findings showing that blunted HR stress reactivity may be associated with motivational dysfunction, or in the case of people with cardiovascular disease even cardiovascular dysfunction (94), our study provides first data on the differential impact of both and can be used as a steppingstone for further research. In the past it has been shown that exercise therapy is highly beneficial for patients with mild to moderate depression and as supplementation even for patients with severe depression (76). Since exercise therapy targets both depressive symptoms and can prevent/improve various health conditions (cardiovascular diseases, type 2 diabetes and metabolic syndrome) (76), this form of intervention should be strongly considered for people with high levels of stress and depressive symptoms.
Conclusion
In this study, we were able to show that high and extreme stress alone did not have any consequence on circadian rhythm, apart from a limited increase in basal HR. Yet, in the presence of depressive symptoms, extreme chronic stress levels did lead to blunted HR circadian rhythm. In addition, blunted reactivity to stressors was associated with depressive symptoms and not chronic stress. Our data suggest that using interventions which target depressive symptoms and cardiovascular health, such as exercise therapy, may be highly relevant for those with high levels of stress and depressive symptoms.
Limitations
A number of limitations need to be mentioned for this study. As this study was cross-sectional, it does not allow to evaluate the question of temporal precedence/causality of either depressive symptoms or high stress levels. The limited sample size of people with extreme stress (a total of 20 people) also shows that this group is rather rare in the healthy population, as expected, consequently power for group comparisons was low. Furthermore, we did not assess cognition in this sample. It may very well be that altered cognition, as often encountered in depression, leads to a higher subjective experience of chronic stress. Additionally, we did not have an objective measure of chronic stress, such as e.g., hair cortisol. Moreover, the study was limited to a single physiological parameter, instead of a combination of multiple parameters. Another limitation was the lack of a clinical assessment for depressive symptoms on intake, and that other parameters which can influence HR/HRV outcomes have not been assessed. Furthermore, our model for HRV circadian analysis did not yield a sufficiently good fit, only RMSSD data during the night provides limited insight. Circadian HRV analyses would be important to draw conclusions for cardiac vagal modulation and thus yield more insight into the biology of chronic stress and depressive symptoms. As there was no psychiatric interview, the sample might include some people with undiagnosed pathology. The sample was also limited to mostly highly educated people, which may not be representative of the complete population. Other limitations related to group differences in sleep quality and sex. These variables were added as covariates to the baseline of the circadian model to reduce possible biases. Particularly sex should be investigated further in future studies since important differences in circadian rhythm abnormalities have been found.
Data availability statement
The data analyzed in this study is subject to the following licenses/restrictions: The data that support the findings of this study are available on request. The data are not publicly available due to them containing information that could compromise research subject privacy. Requests to access these datasets should be directed to CV, Y2hyaXMudmFuaG9vZkBpbWVjLmJl.
Ethics statement
The studies involving human participants were reviewed and approved by the Ethics Committee Research UZ/KU Leuven. The participants provided their written informed consent to participate in this study.
Author contributions
EL designed and performed the analyses and wrote the manuscript. CS reviewed the analyses, performed literature search, and helped with manuscript preparation. JC provided assistance during the trial and during data analysis. WD, AR, and EV provided counsel on statistical analyses and manuscript preparation. SC and CV helped with conception of the trial, counsel on statistical analyses, and manuscript preparation, as well as overall supervision. All authors contributed to the article and approved the submitted version.
Funding
This work was supported by a PhD fellowship from the Research Foundation—Flanders (FWO) awarded to EL (1SB4719N).
Conflict of interest
The authors declare that the research was conducted in the absence of any commercial or financial relationships that could be construed as a potential conflict of interest.
Publisher’s note
All claims expressed in this article are solely those of the authors and do not necessarily represent those of their affiliated organizations, or those of the publisher, the editors and the reviewers. Any product that may be evaluated in this article, or claim that may be made by its manufacturer, is not guaranteed or endorsed by the publisher.
Supplementary material
The Supplementary Material for this article can be found online at: https://www.frontiersin.org/articles/10.3389/fpsyt.2022.1022298/full#supplementary-material
References
1. Carney RM, Freedland KE, Veith RC. Depression, the autonomic nervous system, and coronary heart disease. Psychosom Med. (2005) 67(Suppl. 1):S29–33. doi: 10.1097/01.psy.0000162254.61556.d5
2. Kemp AH, Quintana DS, Gray MA, Felmingham KL, Brown K, Gatt JM. Impact of depression and antidepressant treatment on heart rate variability: a review and meta-analysis. Biol Psychiatry. (2010) 67:1067–74. doi: 10.1016/j.biopsych.2009.12.012
3. Koch C, Wilhelm M, Salzmann S, Rief W, Euteneuer F. A meta-analysis of heart rate variability in major depression. Psychol Med. (2019) 49:1948–57. doi: 10.1017/S0033291719001351
4. Schiweck C, Lutin E, De Raedt W, Cools O, Coppens V, Morrens M, et al. Twenty-Four-Hour heart rate is a trait but not state marker for depression in a pilot randomized controlled trial with a single infusion of ketamine. Front Psychiatry. (2021) 12:696170. doi: 10.3389/fpsyt.2021.696170
5. Schiweck C, Gholamrezaei A, Maxim H, Vaessen T, Vrieze E, Claes S. Exhausted heart rate responses to repeated psychological stress in women with major depressive disorder. Front Psychiatry. (2022) 13:869608. doi: 10.3389/fpsyt.2022.869608
6. Stampfer HG. The relationship between psychiatric illness and the circadian pattern of heart rate. Aust N Z J Psychiatry. (1998) 32:187–98. doi: 10.3109/00048679809062728
7. Taillard J, Lemoine P, Boule P, Drogue M, Mouret J. Sleep and heart rate circadian rhythm in depression: the necessity to separate. Chronobiol Int. (1993) 10:63–72. doi: 10.3109/07420529309064483
8. Guo Y, Stein PK, Louis S. Circadian rhythm in the cardiovascular system : chronocardiology. Am Heart J. (2003) 45:779–86. (02)00088-1 doi: 10.1016/S0002-8703
9. Kuang D, Yang R, Chen X, Lao G, Wu F, Huang X, et al. Depression recognition according to heart rate variability using Bayesian networks. J Psychiatr Res. (2017) 95:282–7. doi: 10.1016/j.jpsychires.2017.09.012
10. Germain A, Kupfer DJ. Circadian rhythm disturbances in depression. Hum Psychopharmacol. (2008) 23:571–85. doi: 10.1002/hup.964
11. Jarczok MN, Aguilar-raab C, Koenig J, Kaess M, Borniger C, Nelson RJ, et al. The Heart’s rhythm ‘n’ blues : sex differences in circadian variation patterns of vagal activity vary by depressive symptoms in predominantly healthy employees. Chronobiol Int. (2018) 35:896–909. doi: 10.1080/07420528.2018.1439499
12. Jandackova VK, Britton A, Malik M, Steptoe A. Heart rate variability and depressive symptoms: a cross-lagged analysis over a 10-year period in the Whitehall II study. Psychol Med. (2016) 46:2121–31. doi: 10.1017/S003329171600060X
13. Balint EM, Daniele V, Langgartner D, Reber SO, Rothermund E, Gündel H, et al. Heart rate variability predicts outcome of short-term psychotherapy at the workplace. Psychophysiology. (2022) 2021:e14150. doi: 10.1111/psyp.14150
14. Schneider M, Schwerdtfeger A. Autonomic dysfunction in posttraumatic stress disorder indexed by heart rate variability: a meta-analysis. Psychol Med. (2020) 50:1937–48. doi: 10.1017/S003329172000207X
15. Cheng YC, Su MI, Liu CW, Huang YC, Huang WL. Heart rate variability in patients with anxiety disorders: a systematic review and meta-analysis. Psychiatry Clin Neurosci. (2022) 76:292–302. doi: 10.1111/pcn.13356
16. Benichou T, Pereira B, Mermillod M, Tauveron I, Pfabigan D, Maqdasy S, et al. Heart rate variability in type 2 diabetes mellitus: a systematic review and meta–analysis. PLoS One. (2018) 13:e0195166. doi: 10.1371/journal.pone.0195166
17. Ying-Chih C, Yu-Chen H, Wei-Lieh H. Heart rate variability in patients with somatic symptom disorders and functional somatic syndromes: a systematic review and meta-analysis. Neurosci Biobehav Rev. (2020) 112:336–44. doi: 10.1016/j.neubiorev.2020.02.007
18. Hughes JW, Stoney CM. Depressed mood is related to high-frequency heart rate variability during stressors. Psychosom Med. (2000) 62:796–803. doi: 10.1097/00006842-200011000-00009
19. Benvenuti SM, Mennella R, Buodo G, Palomba D. Dysphoria is associated with reduced cardiac vagal withdrawal during the imagery of pleasant scripts: evidence for the positive attenuation hypothesis. Biol Psychol. (2015) 106:28–38. doi: 10.1016/j.biopsycho.2014.11.017
20. Gordon JL, Ditto B, D’Antono B. Cognitive depressive symptoms associated with delayed heart rate recovery following interpersonal stress in healthy men and women. Psychophysiology. (2012) 49:1082–9. doi: 10.1111/j.1469-8986.2012.01397.x
21. Silvia PJ, Nusbaum EC, Eddington KM, Beaty RE, Kwapil TR. Effort deficits and depression: the influence of anhedonic depressive symptoms on cardiac autonomic activity during a mental challenge. Motiv Emot. (2014) 38:779–89. doi: 10.1007/s11031-014-9443-0
22. Tegegne BS, Man T, van Roon AM, Riese H, Snieder H. Determinants of heart rate variability in the general population: the lifelines cohort study. Heart Rhythm. (2018) 15:1552–8. doi: 10.1016/j.hrthm.2018.05.006
23. De Rooij SR. Blunted cardiovascular and cortisol reactivity to acute psychological stress: a summary of results from the Dutch Famine Birth Cohort study. Int J Psychophysiol. (2013) 90:21–7. doi: 10.1016/j.ijpsycho.2012.09.011
24. Forte G, Favieri F, Casagrande M. Heart rate variability and cognitive function: a systematic review. Front Neurosci. (2019) 13:710. doi: 10.3389/fnins.2019.00710
25. Matuz A, van der Linden D, Kisander Z, Hernádi I, Kázmér K, Csathó Á. Enhanced cardiac vagal tone in mental fatigue: analysis of heart rate variability in Time-on-Task, recovery, and reactivity. PLoS One. (2021) 16:e0238670. doi: 10.1371/journal.pone.0238670
26. Slušnienë A, Laucevièius A, Navickas P, Ryliškytë L, Stankus V, Stankus A, et al. Daily heart rate variability indices in subjects with and without metabolic syndrome before and after the elimination of the influence of day-time physical activity. Medicina. (2019) 55:1–14. doi: 10.3390/medicina55100700
27. Tang M, He Y, Zhang X, Li H, Huang C, Wang C, et al. The acute effects of temperature variability on heart rate variability: a repeated-measure study. Environ Res. (2021) 194:110655. doi: 10.1016/j.envres.2020.110655
28. Lampert R, Tuit K, Hong K-I, Donovan T, Lee F, Sinha R. Cumulative stress and autonomic dysregulation in a community sample. Stress. (2016) 19:269–79. doi: 10.1080/10253890.2016.1174847
29. Vrijkotte TGM, Van Doornen LJP, De Geus EJC. Effects of work stress on ambulatory blood pressure, heart rate, and heart rate variability. Hypertension. (2000) 35:880–6. doi: 10.1161/01.HYP.35.4.880
30. Juster R-P, Bizik G, Picard M, Arsenault-Lapierre G, Sindi S, Trepanier L, et al. A transdisciplinary perspective of chronic stress in relation to psychopathology throughout life span development. Dev Psychopathol. (2011) 23:725–76. doi: 10.1017/S0954579411000289
31. McEwen BS, Seeman T. Protective and damaging effects of mediators of stress. Elaborating and testing the concepts of allostasis and allostatic load. Ann N Y Acad Sci. (1999) 896:30–47. doi: 10.1111/j.1749-6632.1999.tb08103.x
32. Huizink AC, Mulder EJH, Buitelaar JK. Prenatal stress and risk for psychopathology: specific effects or induction of general susceptibility? Psychol Bull. (2004) 130:115–42. doi: 10.1037/0033-2909.130.1.115
33. Harkness KL, Hayden EP, Lopez-Duran NL. Stress sensitivity and stress sensitization in psychopathology: an introduction to the special section. J Abnorm Psychol. (2015) 124:1–3. doi: 10.1037/abn0000041
34. Fleming I, Baum A, Davidson LM, Rectanus E, McArdle S. Chronic stress as a factor in physiologic reactivity to challenge. Health Psychol. (1987) 6:221–37. doi: 10.1037//0278-6133.6.3.221
35. Pruessner M, Hellhammer DH, Pruessner JC, Lupien SJ. Self-reported depressive symptoms and stress levels in healthy young men: associzations with the cortisol response to awakening. Psychosom Med. (2003) 65:92–9. doi: 10.1097/01.PSY.0000040950.22044.10
36. Smets E, Velazquez ER, Schiavone G, Chakroun I, Hondt ED, Raedt W, et al. Large-scale wearable data reveal digital phenotypes for daily- life stress detection. NPJ Digit Med. (2018) 1:67. doi: 10.1038/s41746-018-0074-9
37. BioTelemetry Inc. EPATCH™ Extended Holter Monitoring. (n.d.). Available online at: https://www.gobio.com/heart-monitoring/ (accessed June 15, 2020).
38. Kwon O, Jeong J, Kim HB, Kwon IH, Park SY, Kim JE, et al. Electrocardiogram sampling frequency range acceptable for heart rate variability analysis. Healthc Inform Res. (2018) 24:198–206.
39. Dedovic K, Renwick R, Mahani NK, Engert V, Lupien SJ, Pruessner JC. The montreal imaging stress task : using functional imaging to investigate the effects of perceiving and processing psychosocial stress in the human brain. J Psychiatry Neurosci. (2005) 30:319–25.
40. Orphanidou C, Bonnici T, Charlton P, Clifton D, Vallance D, Tarassenko L. Signal-quality indices for the electrocardiogram and photoplethysmogram : derivation and applications to wireless monitoring. IEEE J Biomed Health Inform. (2015) 19:832–8. doi: 10.1109/JBHI.2014.2338351
41. R Core Team,. R: A Lagnuage and Environment for Statistical Computing. Vienna: R Foundation for Statistical Computing. (2020). Available online at: https://www.r-project.org/ (accessed March 2, 2020).
42. Coker AO, Coker OO, Sanni D. Psychometric properties of the 21-item depression anxiety stress scale (DASS-21). Afr Res Rev. (2018) 12:135–42.
43. Cohen S, Williamson G. Perceived stress in a probability sample of the United States. In: Spacapan S, Oskamp S Editors. The Social Psychology of Health. (Vol. 13), Thousand Oaks, CA: Sage Publications (1988). p. 31–67.
44. Lovibond SH, Lovibond PF. Manual for the Depression Anxiety Stress Scales. Sydney, NS: Psychology Foundation (1995). 2 p.
45. Cohen S, Janicki-Deverts D. Who’s stressed? Distributions of psychological stress in the united states in probability samples from 1983, 2006, and 2009. J Appl Soc Psychol. (2012) 42:1320–34. doi: 10.1111/j.1559-1816.2012.00900.x
46. Veerman DP, Imholz BPM, Wieling W, Wesseling KH, van Montfrans GA. Circadian profile of systemic hemodynamics. Hypertension. (1995) 26:55–9.
47. Cavallari JM, Fang SC, Mittleman MA, Christiani DC. Circadian variation of heart rate variability among welders. Occup Environ Med. (2010) 67:717–9. doi: 10.1136/oem.2010.055210
48. Morelli D, Bartoloni L, Rossi A, Clifton DA. A computationally efficient algorithm to obtain an accurate and interpretable model of the effect of circadian rhythm on resting heart rate a computationally efficient algorithm to obtain an accurate and interpretable model of the effect of circadian rhythm. Physiol Meas. (2019) 40:95001. doi: 10.1088/1361-6579/ab3dea
49. Porges SW. Vagal tone: a physiologic marker of stress vulnerability. Pediatrics. (1992) 90(3 Pt. 2):498–504. doi: 10.1542/peds.90.3.498
50. Thayer JF, Hansen AL, Saus-Rose E, Johnsen BH. Heart rate variability, prefrontal neural function, and cognitive performance: the neurovisceral integration perspective on self-regulation, adaptation, and health. Ann Behav Med. (2009) 37:141–53. doi: 10.1007/s12160-009-9101-z
51. Sgoifo A, Carnevali L, Pico Alfonso MDLA, Amore M. Autonomic dysfunction and heart rate variability in depression. Stress. (2015) 18:343–52. doi: 10.3109/10253890.2015.1045868
52. Stein PK, Bosner MS, Kleiger RE, Conger BM. Heart rate variability: a measure of cardiac autonomic tone. Am Heart J. (1994) 127:1376–81. doi: 10.1016/0002-8703(94)90059-0
53. Camm AJ, Malik M, Bigger JT, Breithardt G, Cerutti S, Cohen RJ, et al. Heart rate variability, standards of measurement, physiological interpretation, and clinical use. Task force of the European society of cardiology and the North American society of pacing and electrophysiology. Circulation. (1996) 93:1043–65.
54. Shaffer F, Ginsberg JP. An overview of heart rate variability metrics and norms. Front Public Health. (2017) 5:258. doi: 10.3389/fpubh.2017.00258
55. Sakamoto Y, Ishiguro M, Kitagawa G. Akaike Information Criterion Statistics. Dordrecht: D. Reidel (1986).
56. Voeten C. Buildmer: Stepwise Elimination and Term Reordering for Mixed-Effects Regression (1.6). (2020). Available online at: https://github.com/cvoeten/buildmer/issues (accessed May 4, 2020).
57. Hamilton JL, Alloy LB. Atypical reactivity of heart rate variability to stress and depression across development: systematic review of the literature and directions for future research. Clin Psychol Rev. (2016) 50:67–79. doi: 10.1016/j.cpr.2016.09.003
58. Tafet GE, Nemeroff CB. The links between stress and depression: psychoneuroendocrinological, genetic, and environmental interactions. J Neuropsychiatry Clin Neurosci. (2016) 28:77–88. doi: 10.1176/appi.neuropsych.15030053
59. Clays E, De Bacquer D, Crasset V, Kittel F, De Smet P, Kornitzer M, et al. The perception of work stressors is related to reduced parasympathetic activity. Int Arch Occup Environ Health. (2011) 84:185–91. doi: 10.1007/s00420-010-0537-z
60. Chandola T, Britton A, Brunner E, Hemingway H, Malik M, Kumari M, et al. Work stress and coronary heart disease: what are the mechanisms? Eur Heart J. (2008) 29:640–8. doi: 10.1093/eurheartj/ehm584
61. Uusitalo A, Mets T, Martinmäki K, Mauno S, Kinnunen U, Rusko H. Heart rate variability related to effort at work. Appl Ergonom. (2011) 42:830–8. doi: 10.1016/j.apergo.2011.01.005
62. Dutheil F, Boudet G, Perrier C, Lac G, Ouchchane L, Chamoux A, et al. JOBSTRESS study: comparison of heart rate variability in emergency physicians working a 24-hour shift or a 14-hour night shift - A randomized trial. Int J Cardiol. (2012) 158:322–5. doi: 10.1016/j.ijcard.2012.04.141
63. Karhula K, Henelius A, Härmä M, Sallinen M, Lindholm H, Kivimäki M, et al. Job strain and vagal recovery during sleep in shift working health care professionals. Chronobiol Int. (2014) 31:1179–89. doi: 10.3109/07420528.2014.957294
64. Carr O, Saunders KEA, Bilderbeck AC, Tsanas A, Palmius N, Geddes JR, et al. Desynchronization of diurnal rhythms in bipolar disorder and borderline personality disorder. Transl Psychiatry. (2018) 8:79. doi: 10.1038/s41398-018-0125-7
65. Verkuil B, Brosschot JF, Marques AH, Kampschroer K, Sternberg EM, Thayer JF. Gender differences in the impact of daily sadness on 24-h heart rate variability. Psychophysiology. (2015) 52:1682–8. doi: 10.1111/psyp.12541
66. Monteleone P, Martiadis V, Maj M. Circadian rhythms and treatment implications in depression. Prog Neuro Psychopharmacol Biol Psychiatry. (2011) 35:1569–74. doi: 10.1016/j.pnpbp.2010.07.028
67. Souêtre E, Salvati E, Belugou J-L, Pringuey D, Candito M, Krebs B, et al. Circadian rhythms in depression and recovery: evidence for blunted amplitude as the main chronobiological abnormality. Psychiatry Res. (1989) 28:263–78. doi: 10.1016/0165-1781(89)90207-2
68. Egeler ME, Bowman M, Thayer JF, Brindle RC, Hall M, Kline CE. 1088 Heart rate variability during sleep in adults with and without a history of depression and the influence of antidepressant use. Sleep. (2020) 43:A414.
69. McEwen BS. Stress, adaptation, and disease: allostasis and allostatic load. Ann N Y Acad Sci. (1998) 840:33–44. doi: 10.1111/j.1749-6632.1998.tb09546.x
70. Ehlers CL, Frank E, Kupfer DJ. Social zeitgebers and biological rhythms: a unified approach to understanding the etiology of depression. Arch Gen Psychiatry. (1988) 45:948–52. doi: 10.1001/archpsyc.1988.01800340076012
71. McClung CA. Circadian rhythms and mood regulation: insights from pre-clinical models. Eur Neuropsychopharmacol. (2011) 21, (Suppl. 4):S683–93. doi: 10.1016/j.euroneuro.2011.07.008
72. Daut RA, Fonken LK. Frontiers in neuroendocrinology circadian regulation of depression : a role for serotonin. Front Neuroendocrinol. (2019) 54:100746. doi: 10.1016/j.yfrne.2019.04.003
73. Crnko S, Du Pré BC, Sluijter JPG, Van Laake LW. Circadian rhythms and the molecular clock in cardiovascular biology and disease. Nat Rev. Cardiol. (2019) 16:437–47. doi: 10.1038/s41569-019-0167-4
74. Ho FKW, Louie LHT, Chow CB, Wong WHS, Ip P. Physical activity improves mental health through resilience in Hong Kong Chinese adolescents. BMC Pediatrics. (2015) 15:48. doi: 10.1186/s12887-015-0365-0
75. Stults-Kolehmainen MA, Sinha R. The effects of stress on physical activity and exercise. Sports Med. (2014) 44:81–124. doi: 10.1007/s40279-013-0090-5
76. Knapen J, Vancampfort D, Moriën Y, Marchal Y. Exercise therapy improves both mental and physical health in patients with major depression. Disabil Rehabil. (2015) 37:1490–5. doi: 10.3109/09638288.2014.972579
77. da Estrela C, McGrath J, Booij L, Gouin J-P. Heart rate variability, sleep quality, and depression in the context of chronic stress. Ann Behav Med. (2021) 55:155–64. doi: 10.1093/abm/kaaa039
78. Drake CL, Pillai V, Roth T. Stress and sleep reactivity: a prospective investigation of the stress-diathesis model of insomnia. Sleep. (2014) 37:1295–304. doi: 10.5665/sleep.3916
79. Vargas I, Friedman NP, Drake CL. Vulnerability to stress-related sleep disturbance and insomnia: investigating the link with comorbid depressive symptoms. Transl Issues Psychol Sci. (2015) 1:57–66. doi: 10.1037/tps0000015
80. Cajochen C, Pischke J, Aeschbach D, Borbély AA. Heart rate dynamics during human sleep. Physiol Behav. (1994) 55:769–74. doi: 10.1016/0031-9384(94)90058-2
81. Lee EH. Review of the psychometric evidence of the perceived stress scale. Asian Nurs Res. (2012) 6:121–7. doi: 10.1016/j.anr.2012.08.004
82. Matud MP. Gender differences in stress and coping styles. Pers Individ Differ. (2004) 37:1401–15. doi: 10.1016/j.paid.2004.01.010
83. Fredrikson M, Matthews KA. Cardiovascular responses to behavioral stress and hypertension: a meta-analytic review2. Ann Behav Med. (1990) 12:30–9. doi: 10.1207/s15324796abm1201_3
84. Schiweck C, Piette D, Berckmans D, Claes S, Vrieze E. Heart rate and high frequency heart rate variability during stress as biomarker for clinical depression. A systematic review. Psychol Med. (2019) 49:200–11. doi: 10.1017/S0033291718001988
85. Bourassa KJ, Moffitt TE, Harrington HL, Houts R, Poulton R, Ramrakha S, et al. Lower cardiovascular reactivity is associated with more childhood adversity and poorer midlife health: replicated findings from the dunedin and MIDUS cohorts. Clin Psychol Sci. (2021) 9:961–78. doi: 10.1177/2167702621993900
86. Keogh TM, Howard S, O’Riordan A, Gallagher S. Motivational orientation mediates the association between depression and cardiovascular reactivity to acute psychological stress. Psychophysiology. (2021) 58:e13732. doi: 10.1111/psyp.13732
87. Jackowska M, Dockray S, Endrighi R, Hendrickx H, Steptoe A. Sleep problems and heart rate variability over the working day. J Sleep Res. (2012) 21:434–40. doi: 10.1111/j.1365-2869.2012.00996.x
88. Shier AJ, Keogh T, Costello AM, Riordan AO, Gallagher S. Eveningness, depression and cardiovascular reactivity to acute psychological stress: a mediation model. Physiol Behav. (2021) 240:113550. doi: 10.1016/j.physbeh.2021.113550
89. Brindle RC, Ginty AT, Conklin SM. Is the association between depression and blunted cardiovascular stress reactions mediated by perceptions of stress? Int J Psychophysiol. (2013) 90:66–72. doi: 10.1016/j.ijpsycho.2013.06.003
90. Brugnera A, Zarbo C, Tarvainen MP, Carlucci S, Tasca GA, Adorni R, et al. Higher levels of depressive symptoms are associated with increased resting-state heart rate variability and blunted reactivity to a laboratory stress task among healthy adults. Appl Psychophysiol Biofeedback. (2019) 44:221–34. doi: 10.1007/s10484-019-09437-z
91. Burke HM, Fernald LC, Gertler PJ, Adler NE. Depressive symptoms are associated with blunted cortisol stress responses in very low-income women. Psychosom Med. (2005) 67:211–6. doi: 10.1097/01.psy.0000156939.89050.28
92. Chida Y, Hamer M. Chronic psychosocial factors and acute physiological responses to laboratory-induced stress in healthy populations: a quantitative review of 30 years of investigations. Psychol Bull. (2008) 134:829. doi: 10.1037/a0013342
93. Low CA, Salomon K, Matthews KA. Chronic life stress, cardiovascular reactivity, and subclinical cardiovascular disease in adolescents. Psychosom Med. (2009) 71:927–31. doi: 10.1097/PSY.0b013e3181ba18ed
Keywords: heart rate, depressive symptoms, chronic stress, circadian rhythm, stress reactivity
Citation: Lutin E, Schiweck C, Cornelis J, De Raedt W, Reif A, Vrieze E, Claes S and Van Hoof C (2022) The cumulative effect of chronic stress and depressive symptoms affects heart rate in a working population. Front. Psychiatry 13:1022298. doi: 10.3389/fpsyt.2022.1022298
Received: 18 August 2022; Accepted: 26 September 2022;
Published: 13 October 2022.
Edited by:
Panagiotis Ferentinos, National and Kapodistrian University of Athens, GreeceReviewed by:
Elisabeth Maria Balint, Private Clinic Meiringen, SwitzerlandJan Sarlon, University Psychiatric Clinic Basel, Switzerland
Alina Wilkowska, Medical University of Gdańsk, Poland
Copyright © 2022 Lutin, Schiweck, Cornelis, De Raedt, Reif, Vrieze, Claes and Van Hoof. This is an open-access article distributed under the terms of the Creative Commons Attribution License (CC BY). The use, distribution or reproduction in other forums is permitted, provided the original author(s) and the copyright owner(s) are credited and that the original publication in this journal is cited, in accordance with accepted academic practice. No use, distribution or reproduction is permitted which does not comply with these terms.
*Correspondence: Erika Lutin, ZXJpa2EubHV0aW5AaW1lYy5iZQ==
†These authors share first authorship