- 1Division of Information Science, Nara Institute of Science and Technology, Nara, Japan
- 2Department of Immune Medicine, National Cancer Center Research Institute, Tokyo, Japan
- 3Department of Psycho-Oncology, National Cancer Center Hospital, Tokyo, Japan
- 4Department of Neuropsychiatry, Nippon Medical School, Tama Nagayama Hospital, Tokyo, Japan
- 5Department of Psycho-Oncology, Cancer Institute Hospital of Japanese Foundation for Cancer Research, Tokyo, Japan
- 6Department of Clinical Genomics, National Cancer Center Research Institute, Tokyo, Japan
- 7Department of Pain Control Research, The Jikei University School of Medicine, Tokyo, Japan
- 8Department of Biomedical Data Science, Fujita Health University School of Medicine, Aichi, Japan
Background: Phenotyping analysis that includes time course is useful for understanding the mechanisms and clinical management of postoperative delirium. However, postoperative delirium has not been fully phenotyped. Hypothesis-free categorization of heterogeneous symptoms may be useful for understanding the mechanisms underlying delirium, although evidence is currently lacking. Therefore, we aimed to explore the phenotypes of postoperative delirium following invasive cancer surgery using a data-driven approach with minimal prior knowledge.
Methods: We recruited patients who underwent elective invasive cancer resection. After surgery, participants completed 5 consecutive days of delirium assessments using the Delirium Rating Scale-Revised-98 (DRS-R-98) severity scale. We categorized 65 (13 questionnaire items/day × 5 days) dimensional DRS-R-98 scores using unsupervised machine learning (K-means clustering) to derive a small set of grouped features representing distinct symptoms across all participants. We then reapplied K-means clustering to this set of grouped features to delineate multiple clusters of delirium symptoms.
Results: Participants were 286 patients, of whom 91 developed delirium defined according to Diagnostic and Statistical Manual of Mental Disorders, Fifth Edition, criteria. Following the first K-means clustering, we derived four grouped symptom features: (1) mixed motor, (2) cognitive and higher-order thinking domain with perceptual disturbance and thought content abnormalities, (3) acute and temporal response, and (4) sleep–wake cycle disturbance. Subsequent K-means clustering permitted classification of participants into seven subgroups: (i) cognitive and higher-order thinking domain dominant delirium, (ii) prolonged delirium, (iii) acute and brief delirium, (iv) subsyndromal delirium-enriched, (v) subsyndromal delirium-enriched with insomnia, (vi) insomnia, and (vii) fit.
Conclusion: We found that patients who have undergone invasive cancer resection can be delineated using unsupervised machine learning into three delirium clusters, two subsyndromal delirium clusters, and an insomnia cluster. Validation of clusters and research into the pathophysiology underlying each cluster will help to elucidate the mechanisms of postoperative delirium after invasive cancer surgery.
1. Introduction
Delirium encompasses a variety of symptoms and clinical effects, such as attention disorders, visual hallucinations, thought disorders, disorientation that impairs communication, visuospatial cognitive impairments that contribute to falls and tumbles, motor agitation that threatens medical safety, and motor inhibition that impairs self-care, delays rehabilitation after surgery, and burdens healthcare workers (1–6). Previous work has shown that delirium is a constellation of various symptoms and trajectories that require further investigation to determine a clear etiology and maximize potential reversibility (7, 8).
Although numerous hypotheses have been proposed for the pathogenesis of delirium, the pathophysiology underlying delirium onset has not yet been clarified. Delirium is a psychiatric condition characterized by a wide variety of psychiatric symptoms. Similar to other psychiatric disorders, phenotypic heterogeneity likely plays an important role in delirium pathogenesis. Previous studies (9–15) have assessed delirium symptoms in detail using the Delirium Rating Scale-Revised-98 (DRS-R-98) and introduced three core domains (cognitive, higher-order thinking, and circadian domains) with accessory symptoms, and four motor subtypes (hyperactive, hypoactive, mixed, and normoactive delirium). The cognitive domain comprises orientation, attention, short- and long-term memory, and visuospatial ability; the higher-order thinking domain consists of language and thought processes; the circadian domain comprises the sleep–wake cycle and motor activity alterations.
Meagher et al. have explored delirium clinical subtyping based on motor symptoms (8, 15–18). Hyperactive delirium is characterized by an increase in motor activity and often results in disruptive or potentially harmful behaviors that jeopardize medical compliance and safety. In contrast, hypoactive delirium is characterized by decreased motor activity. Patients who have both features of increased and decreased activity during an episode are categorized as having the mixed delirium subtype. These three main subtypes are currently used for the diagnosis, prevention, and treatment of delirium in older adults (8, 16, 19); however, these motor subtypes do not specify the trajectory of delirium, nor do they address symptoms other than motility.
Trepacz et al. investigated the influence of age and sex, as well as differences in physical illness, on delirium phenotypes (10). They performed factor analysis and found that delirium had a consistent two-factor structure for delirium phenomenology regardless of age and sex. They also identified the following tendencies: The relationship between the core domains and accessory symptoms was similar in younger patients and women, whereas in older adults and men, accessory symptoms were significantly correlated with the circadian domain but not with other core domains. However, in this previous study, the 13-item severity scale of the DRS-R-98 was reduced to three-dimensional composite scores representing the three core domains of delirium. Although this procedure follows the three-core-domain hypothesis and helps to reduce variability in the factor analysis, it is possible that it ignores the relationship among the original 13-item scales, which could contribute to differences in etiology. Thus, a hypothesis-free, data-driven approach could be useful for exploring new possibilities beyond existing hypotheses.
Postoperative delirium remains to be fully phenotyped. The incidence of postoperative delirium has been reported to range from 11.5 to 50%, with a particularly high incidence in highly invasive cancer surgery (20–23). Measures to prevent postoperative delirium have been investigated (24) because systemic inflammation and hypoxia are known to be mechanistically involved in postoperative delirium (25). These responses are triggered by the wound and anesthesia in relatively fit patients undergoing surgery. Phenotyping postoperative delirium could help in the development of methods of prevention and care. However, to our knowledge, only one study has explored the trajectory of delirium severity after cancer resection, and this study did not consider a variety of phenotypes or assess delirium on consecutive days (26). The phenotypic characteristics of postoperative delirium after invasive cancer resection remain to be elucidated. Furthermore, although categorization techniques using time series datasets have been proposed as methods to better understand the heterogeneity of mental illness (27), they have not been adequately examined for delirium.
In the present study, we aimed to explore the hypothesis-free categorization of patients according to delirium symptoms using an unsupervised machine-learning approach (i.e., an AI technique to find regularity embedded in a data set without desired outputs) to offer new insights into delirium heterogeneity following invasive cancer resection. To identify potential delirium symptom clusters, we applied an unsupervised machine-learning method called K-means clustering to scores obtained from DRS-R-98 assessment over 5 consecutive days after invasive cancer resection.
2. Materials and methods
2.1. Participants
This was a prospective cohort study to measure postoperative delirium symptoms following invasive cancer resection. The present study was conducted in accordance with the Declaration of Helsinki. Participants were recruited from the National Cancer Center Hospital in Japan, and the study was approved by the Institutional Review Board of the National Cancer Center Japan (2017-282, approved on the 27th of March, 2018). Inclusion criteria were patients undergoing invasive cancer resection, which was defined as an operation planned for 6 h or more with postoperative recovery in the intensive care unit; aged 20 years or older; and who provided written informed consent for participation. Participants who were diagnosed with delirium according to the Diagnostic and Statistical Manual of Mental Disorders, Fifth Edition (DSM-5) (28), at enrollment or 1 day before surgery were excluded from the study. All patients were supported for early mobilization by ICU nurses from postoperative day 1.
2.2. Study design
All participants were assessed in the intensive care unit within 2 h of surgery to assess the emergence of delirium according to the DSM-5 (denoted as Day 0) and between 12:00–17:00 on each of the 5 consecutive postoperative days (denoted as Days 1 to 5). In addition, postoperative assessments were performed using the Japanese version of the DRS-R-98 severity scale (20, 29), which assesses delirium severity on 13 items. We defined delirium as positive according to the DSM-5 but used the DRS-R-98 severity scale to investigate various symptoms of delirium following invasive cancer resection and to categorize participants. Diagnostic characteristics were not considered; rather, we focused on symptoms. As an exclusion criterion, positive delirium according to the DSM-5 1 day before surgery enabled us to determine the onset of delirium. All available clinical information was used for the assessment of delirium according to the DSM-5 and DRS-R-98 severity scale. This included witness accounts from nurses and families and recorded assessments using the Confusion Assessment Method for the Intensive Care Unit (30) and the Nursing Delirium Screening Scale (31).
To assess the risk factors for the postoperative delirium cluster, as reported in previous studies (32, 33), we assessed participants’ cognitive function at recruitment using the Mini-Mental State Examination (MMSE) (34) and preoperative anxiety at baseline using the Hospital Anxiety and Depression Scale-Anxiety (HADS-A) (35). We used interviews and medical records to obtain additional information, including daily preoperative use of benzodiazepines (36), anesthesia type (37), and duration of surgery (38) (Supplementary Tables 1, 2).
2.3. Data analysis
2.3.1. Unsupervised K-mean clustering
The K-means clustering algorithm is an unsupervised learning algorithm used to classify a given set of data into K distinct clusters/groups so that internal cohesion within the clusters is optimized. It is widely used in various biomedical fields, such as gene expression analysis, disease prediction, and psychological investigations (further details are provided in Supplementary Methods 1).
2.3.2. Feature grouping and dimension reduction
The matrix included 65 (13 items/day × 5 days) DRS-R-98 score entries (Supplementary Figure 1). To perform hypothesis-free extraction of factors representing core symptoms from all features, we used latent variable modeling and derived factor scores for each participant. Specifically, derivation was implemented using the K-means clustering method (39, 40) to partition all features into different clusters, and a collection of averaged values within the clusters was used as a low-dimensional feature vector in subsequent analyses.
2.3.3. Identification of the participant cluster
To identify the cluster structure of the participants in a data-driven manner, we reapplied the K-means clustering algorithm to the low-dimensional feature vector dataset described in the previous section (further details are provided in Supplementary Methods 2). Of note, this patient clustering was based on delirium-related symptoms during the 5-day postoperative period, grouped in a hypothesis-free manner, and did not lead to a predictive model of patient trajectory.
To characterize the resulting clusters, we also performed post hoc group comparison among the participant clusters using various scores associated with background characteristics and the previously mentioned low-dimensional feature vector. The complete data analysis workflow is summarized in Supplementary Figure 2.
2.3.4. Determination of K for the K-means clustering
Determining the optimal number of clusters (ordinarily denoted as K) is an important aspect of K-means clustering algorithms. In this study, the Akaike information criterion (41, 42) was used as the primary criterion for both dimension reduction and participant clustering (further details are provided in Supplementary Methods 2).
3. Results
Of the 384 consecutively recruited eligible patients, 3 were excluded because of delirium at recruitment and 54 declined to participate, resulting in a total of 327 study participants. The analyses were performed on 286 patients (74.5% of the recruited population) who underwent invasive cancer resection and completed 5 consecutive days of delirium assessments (Supplementary Figure 3). Ninety-one patients were diagnosed with postoperative delirium according to the DSM-5 (Table 1). The delirium group was older, less likely to be working, had poorer cognitive function, had longer surgery and anesthesia duration, and exhibited more severe delirium symptoms as assessed using the DRS-R-98 (Table 1). The characteristics of these variables were analyzed for the identified participant clusters.
3.1. Feature grouping
We first performed feature grouping of the original (286 × 65-dimensional) data matrix using the K-means clustering algorithm. To avoid suboptimal local minima intrinsic to the K-means clustering algorithm, we executed the algorithm 1,000 times with a different initialization for each K and subsequently selected the clustering solution with the lowest Akaike information criterion score as the representative result (Supplementary Figures 4–7). The results showed four feature groups, as shown in Figure 1A (the clustering result is provided in Supplementary Figure 8). Following visual inspection, each feature group was interpreted as follows (Figure 2):
• Mixed motor: This group comprised initial motor retardation with cognitive items and then the trajectory changed to affective lability, motor hyperactivity, and delusion.
• Cognitive and higher-order thinking domain with perceptual disturbance and thought content abnormalities: This group was mainly characterized by cognition, language, and thought processes with perceptual disturbances and abnormal thought content after postoperative Day 2. Of note, it is also characterized by agitated behavior feature on postoperative Days 2–4.
• Acute and temporal response: This group indicated a temporal response after postoperative Day 1 following surgery including emergence delirium.
• Sleep–wake cycle disturbance: Insomnia-related features were grouped independently from other features.
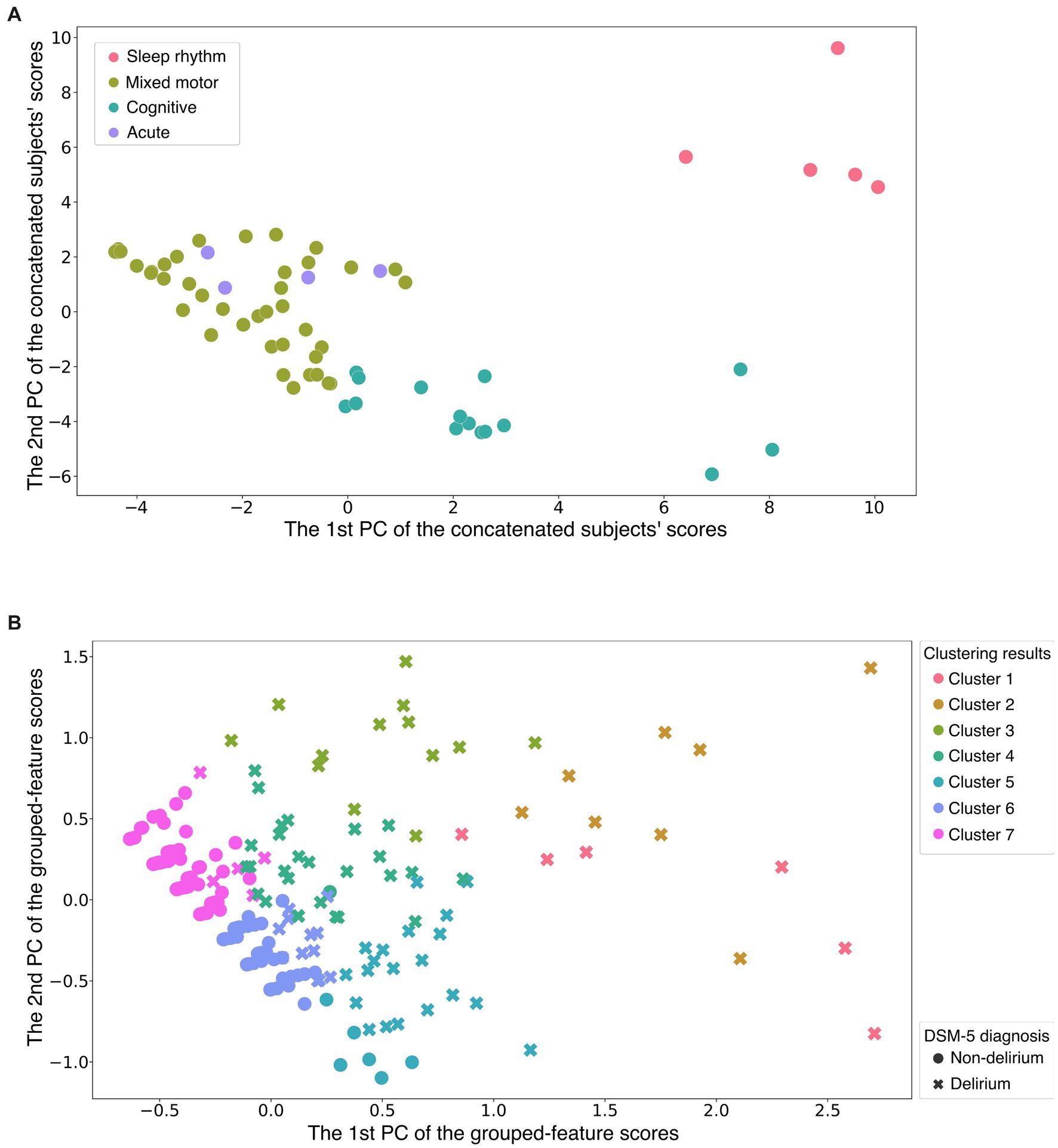
Figure 1. Two-dimensional visualization of feature grouping and participant clustering. (A) The horizontal and vertical axes are the first and second principal component scores of the column vectors of the original data matrix, respectively (Supplementary Figure 2 shows how to create the figure). Each dot indicates a single feature (a DRS-R-98 severity item on a specific day). Color difference shows group difference determined by the first K-means clustering. This suggests that all features assigned to the same group were quite similar to each other. The alternative visualization using the heatmap is shown in Supplementary Figure 8. (B) The horizontal and vertical axes are the first and second principal component scores of the row vectors of the data matrix after dimension reduction processing, respectively. Each point indicates a single participant; the color difference shows the cluster difference determined by the second K-means clustering. Two different markers of the points (crosses and filled circles) indicate whether the corresponding participants were diagnosed as delirium or non-delirium. Participants who belonged to the non-delirium cluster were distributed closely together, whereas the delirium participants were distributed widely. The alternative visualization of participant clusters using the heatmap is shown in Supplementary Figure 9. Sleep rhythm = sleep–wake cycle disturbance; Cognitive = cognitive and higher-order thinking domain with perceptual disturbance and thought content abnormalities; Acute = acute and temporal response; DSM-5 = Diagnostic and Statistical Manual of Mental Disorders, Fifth Edition; PC = principal component (see also Figure 2).
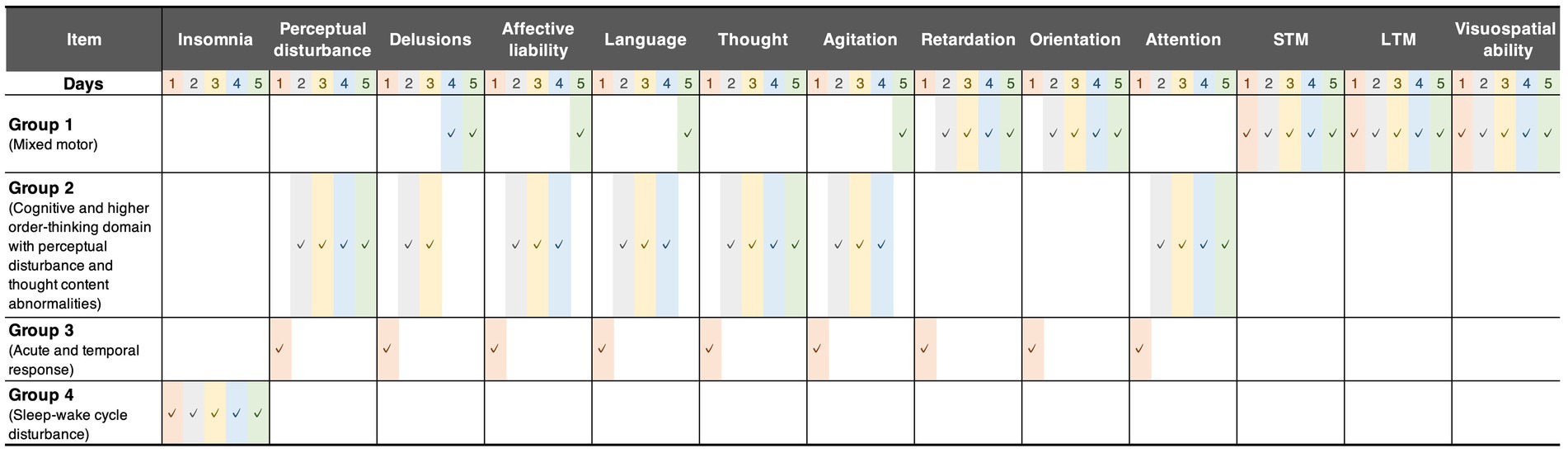
Figure 2. Grouped features. These groups were derived from the first K-means clustering. The checkmarks indicate the group assignment of each feature. Group 1 comprised initial motor retardation with cognitive items and then the trajectory changed to affective lability, motor hyperactivity, and delusion. Group 2 consisted mainly of cognition, language, and thought processes with perceptual disturbances and abnormal thought content after postoperative Day 2. Group 3 indicated temporal response until postoperative Day 1, including the emergence of delirium. Group 4 comprised only sleep–wake cycle disturbance during postoperative 5 days. STM, short-term memory; LTM, long-term memory.
3.2. Participant clustering
As shown in Section 3.1, we extracted four feature groups using K-means clustering. By calculating the average score for each feature group, we transformed the original data matrix X into the dimension-reduced (286 × 4-dimensional) matrix X. By applying K-means clustering to X, we found that the 286 participants could be divided into seven clusters (See the validity of the number of clusters in Supplementary Figures 4–7). Figure 1B illustrates the clusters in reduced two-dimensional space after applying principal component analysis, and shows that participants who belonged to the non-delirium cluster were distributed closely together. In contrast, the delirium participants were distributed widely in the two-dimensional space.
Table 2 shows the demographic and clinical attributes of the participants assigned to each cluster, the order of which was sorted in descending order of the within-cluster average of the maximum DRS-R-98 score over the 5 postoperative days as a measure of delirium symptom severity. Based on the threshold between the number of delirium and non-delirium participants in each cluster, clusters 1 to 5 were treated as delirium clusters, and clusters 6 and 7 were considered non-delirium clusters. Additionally, we used analysis of variance to test the hypothesis that there were no differences between any of the clusters. Our results showed significant differences between two or more clusters for the features of age (p < 0.001), baseline MMSE score (p < 0.005), and the emergence of delirium (p < 0.001). To examine the distribution of each cluster for each grouped feature, we used heatmap visualization, as shown in Supplementary Figure 9. We also generated a radar chart to visualize the mean of each cluster for each of the 13 feature types (Supplementary Figure 10). That clarified that non-delirium participants showed very low scores for all items, whereas delirium participants showed substantial or large scores for one or more items, and the radar shapes varied among delirium clusters.
3.3. Cluster characteristics
To determine how the association between the feature groups and participant clusters should be interpreted, we performed post hoc analyses using one-sample t-tests. The null hypothesis was that the population average in a cluster would be equal to the overall average of all participants for each grouped feature (baseline). We used Bonferroni correction to correct for multiple tests, and set the significance level to a family-wise error rate of 0.05. Table 3 shows the attributes of all seven clusters based on the post hoc tests. The characteristics of each cluster are as follows:
• Cognitive and higher-order thinking domain dominant delirium: This cluster had a feature of “cognitive and higher-order thinking domain with perceptual disturbance and thought content abnormalities” that was significantly higher than baseline.
• Prolonged delirium: This cluster had “mixed motor,” “cognitive and higher-order thinking domain with perceptual disturbance and thought content abnormalities,” and “acute and temporal response” features that were significantly higher than baseline.
• Acute and brief delirium: This cluster is characterized solely by an “acute and temporal response” that is significantly higher than the baseline.
• Subsyndromal delirium-enriched: This cluster had both features of “mixed motor” and “cognitive and higher-order thinking domain with perceptual disturbance and thought content abnormalities” that were significantly higher than baseline.
• Subsyndromal delirium-enriched with insomnia: This cluster had “cognitive and higher-order thinking domain with perceptual disturbance and thought content abnormalities” and “sleep rhythm” features that were significantly higher than baseline.
• Insomnia: This cluster had a “sleep rhythm” feature that was significantly higher than baseline, as well as “mixed motor,” “cognitive and higher-order thinking domain with perceptual disturbance and thought content abnormalities,” and “acute and temporal response” features that were significantly lower than baseline.
• Fit: All features in this cluster were significantly lower than the baseline.
4. Discussion
This is the first study to classify patients by delirium symptoms using hypothesis-free classification with K-means clustering. Although machine-learning approaches have been widely used in psychiatry (43), this study demonstrated their potential utility in the development of delirium research. The K-means clustering analysis of the DRS-R-98 assessment identified four features of delirium symptoms. It was particularly notable that the acute and temporal response feature group was the only group that contained first-day features, whereas the sleep–wake cycle disturbance feature group contained only features from the insomnia item. The participant clustering analysis produced seven clusters with three delirium clusters and two subsyndromal delirium clusters, whereas previous studies have posited only three subtypes of hyperactive delirium, hypoactive delirium, and mixed delirium (11). Although our findings included motor-related features, in line with previous research, we also found additional clusters based on the grouped features. For example, only participants in the subsyndromal delirium-enriched with insomnia cluster and the insomnia cluster had a significantly high value for the sleep rhythm feature. Although these clusters indicated low delirium severity with sleep–wake rhythm disturbance in the circadian domain, they may be a characteristic delirium-related symptom following highly invasive cancer surgery. Drugs to prevent delirium by targeting sleep–wake rhythm disturbances are being developed, of which melatonin, the melatonin analog ramelteon (44, 45), and orexin-receptor antagonists (45, 46) are potential candidates that may also be effective for patients in the subsyndromal delirium-enriched with insomnia cluster following highly invasive cancer surgery. It is also notable that the time course could be divided into an acute and brief delirium cluster and a prolonged delirium cluster. Procedures and complications during the recovery process may affect the time course of postoperative delirium. It is well established that some physical reactions peak immediately after cancer surgery, whereas others peak later and are prolonged (47), which may contribute to the difference between clusters.
This study had several limitations. First, we attempted to classify patients following cancer surgery using delirium-related symptoms in a data-driven manner. However, the statistical approach comprised a group-level analysis, not an individual-level analysis as used in previous research by Meagher et al. (15, 48), and thus did not lead to a predictive model of delirium symptoms. It is unclear whether the clusters found in this study are useful for clinical prediction; for example, the present findings do not suggest that having insomnia the day after surgery predicts insomnia on the fifth postoperative day. However, these findings show that (1) insomnia during 5 postoperative days is more likely to be linked to other delirium symptoms, and (2) sleep rhythm disturbances are a notable feature of the hypothesis-free classification of postoperative patients. Additionally, we did not cluster postoperative delirium patients; rather, we clustered postoperative patients according to delirium symptoms. After validation, this group-level analysis will allow comparison between groups and may help to elucidate the delirium mechanism, taking into account its heterogeneity.
Second, with regard to the data collection process, when we assessed delirium, we could not control the duration between the surgery and the Day 1 assessment. Delirium symptoms fluctuate and tend to be worse at night. Therefore, although performing assessments at night can provide more accurate delirium data, participants are less cooperative than in the daytime. Given this issue, we assessed delirium symptoms from 12:00 to 17:00 using the DRS-R-98 using information from a medical record. This included assessment data from the Confusion Assessment Method for the Intensive Care Unit (30) and the Nursing Delirium Screening Scale (31) obtained from 17:00 the day before the assessment or surgery. Moreover, we used witness accounts of nurses and families to obtain information about patient symptoms until the assessments were performed.
Third, we collected data during the 5 days after surgery and did not analyze symptoms on postoperative Day 6 or later, which may have resulted in missing data for some aspects of delirium. However, our previous study showed that most delirium features manifest by postoperative Day 4 following invasive cancer surgery (49). Therefore, in line with another study (50), we consider that assessing delirium during the 5 days after surgery is appropriate because a longer assessment period increases the probability of delirium being triggered by factors other than surgery.
The fourth limitation is related to the nature of the K-means clustering algorithm. Although the algorithm can be used without prior knowledge of the categorization of features and participants, solutions generally depend on the initial setup and the assumed number of clusters. Thus, it is not feasible to determine a theoretically optimal clustering solution. However, to minimize the dependence, we executed the algorithm 1,000 times, with different initializations for each number of clusters, which varied considerably from 1 to 10. Because we selected the optimal number from all 10,000 clustering solutions based on the Akaike information criterion, our result has high reproducibility as long as the same dataset is analyzed. In addition, we used two-step clustering, in which both DRS-R-98 questionnaire items and participants were categorized in turn, then the items assigned to the same category were summarized using the group average. This aspect of our methodology addresses high within-subject and between-item correlations. In particular, categorization of DRS-R-98 items made it easier to interpret the properties of each cluster (as shown in Figure 2; Supplementary Figure 9).
In related work, Meagher et al. used the generalized estimating equations to analyze the relationship between motor subtypes in delirium and longitudinal phenomenological symptoms assessed by DRS-R-98 (15). This approach is very helpful in characterizing specific subtypes by delirium-related symptoms because it can consider the correlation of within-subject data, which is intrinsic to the type of longitudinal data we collected. However, the generalized estimating equation method itself is not capable of discovering unknown subtypes. Therefore, potential subtypes should be defined based on prior knowledge before applying generalized estimating equations. As the objective of this study was to perform hypothesis-free phenotyping rather than characterize existing subtypes, we used unsupervised K-means clustering. However, a disadvantage of this method is that we had to compromise the nature of the longitudinal data by flattening it to a vector, even though the correlation of within-subject data was partly taken into account by the feature grouping process. We plan to conduct future studies to develop methods to overcome this limitation.
Furthermore, owing to the nature of the data-driven approach, the optimal partition of features can depend on the dataset; thus, we should also investigate whether the structure of the feature grouping is stable over other datasets.
The final limitation is the generalizability of our results to other datasets. The clustering we used in this study constitutes explanatory data analysis to identify a plausible hypothesis. The current findings must now be validated and reproduced using other datasets, especially those that include different anesthesia approaches, cancer sites, and data collection processes. Future validation studies are needed to address these issues.
5. Conclusion
In this study, we used unsupervised machine learning to identify a new phenotype delirium group based on 13 delirium symptoms after invasive cancer surgery. Our data demonstrated seven subgroups of patients with delirium symptoms following surgery: (i) cognitive and higher-order thinking domain dominant delirium, (ii) prolonged delirium, (iii) acute and brief delirium, (iv) subsyndromal delirium-enriched, (v) subsyndromal delirium-enriched with insomnia, (vi) insomnia, and (vii) fit. Future studies should apply these procedures to other participant datasets for validation and to explore the etiology of delirium.
Data availability statement
The original contributions presented in the study are included in the article/Supplementary material, further inquiries can be directed to the corresponding authors.
Ethics statement
The studies involving human participants were reviewed and approved by Institutional Review Board of the National Cancer Center Japan (2017-282). The patients/participants provided their written informed consent to participate in this study.
Author contributions
RS, JY, TY, KA, and YU contributed to the conception and design of the study. RS, SM, SW, and KS collected clinical information and organized the database. PS and JY performed the data analysis and wrote the first draft of the manuscript. RS wrote sections of the manuscript. All authors contributed to the article and approved the submitted version.
Funding
This study was supported by a JSPS KAKENHI grant (no. 18K18468) and the Practical Research for Innovative Cancer Control Project (no. 18ck0106458h0001) from the Japan Agency for Medical Research and Development.
Acknowledgments
We appreciate the considerable efforts of all the surgeons and anesthesiologists who assisted us in conducting this study and the cooperation of all participants. We thank Sarina Iwabuchi and Diane Williams from Edanz (https://jp.edanz.com/ac) for editing a draft of this manuscript.
Conflict of interest
The authors declare that the research was conducted in the absence of any commercial or financial relationships that could be construed as a potential conflict of interest.
Publisher’s note
All claims expressed in this article are solely those of the authors and do not necessarily represent those of their affiliated organizations, or those of the publisher, the editors and the reviewers. Any product that may be evaluated in this article, or claim that may be made by its manufacturer, is not guaranteed or endorsed by the publisher.
Supplementary material
The Supplementary material for this article can be found online at: https://www.frontiersin.org/articles/10.3389/fpsyt.2023.1205605/full#supplementary-material
Abbreviations
DRS-R-98, Delirium rating scale-revised-98; DSM-5, Diagnostic and statistical manual of mental disorders, fifth edition; HADS, Hospital anxiety and depression scale-anxiety; MMSE, Mini-mental state examination.
References
1. Trzepacz, PT, Franco, JG, Meagher, DJ, Lee, Y, Kim, JL, Kishi, Y, et al. Phenotype of subsyndromal delirium using pooled multicultural delirium rating scale—revised-98 data. J Psychosom Res. (2012) 73:10–7. doi: 10.1016/j.jpsychores.2012.04.010
2. Yang, FM, Marcantonio, ER, Inouye, SK, Kiely, DK, Rudolph, JL, Fearing, MA, et al. Phenomenological subtypes of delirium in older persons: patterns, prevalence, and prognosis. Psychosomatics. (2009) 50:248–54. doi: 10.1176/appi.psy.50.3.248
3. Siddiqi, N, House, A, and Holmes, J. Occurrence and outcome of delirium in medical in-patients: a systematic literature review. Age Ageing. (2006) 35:350–64. doi: 10.1093/ageing/afl005
4. Bellelli, G, Speciale, S, Barisione, E, and Trabucchi, M. Delirium subtypes and 1-year mortality among elderly patients discharged from a post-acute rehabilitation facility. J Gerontol A Biol Sci. (2007) 62:1182–3. doi: 10.1093/gerona/62.10.1182
5. Inouye, SK. Delirium in older persons. N Engl J Med. (2006) 354:1157–65. doi: 10.1056/NEJMra052321
6. Breitbart, W, Gibson, C, and Tremblay, A. The delirium experience: delirium recall and delirium-related distress in hospitalized patients with cancer, their spouses/caregivers, and their nurses. Psychosomatics. (2002) 43:183–94. doi: 10.1176/appi.psy.43.3.183
7. Gofton, TE. Delirium: a review. Can J Neurol Sci. (2011) 38:673–80. doi: 10.1017/s0317167100012269
8. Glynn, K, McKenna, F, Lally, K, O’Donnell, M, Grover, S, Chakrabarti, S, et al. How do delirium motor subtypes differ in phenomenology and contributory aetiology? A cross-sectional, multisite study of liaison psychiatry and palliative care patients. BMJ Open. (2021) 11:e041214. doi: 10.1136/bmjopen-2020-041214
9. Meagher, DJ, Moran, M, Raju, B, Gibbons, D, Donnelly, S, Saunders, J, et al. Motor symptoms in 100 patients with delirium versus control subjects: comparison of subtyping methods. Psychosomatics. (2008) 49:300–8. doi: 10.1176/appi.psy.49.4.300
10. Trzepacz, PT, Franco, JG, Meagher, DJ, Lee, Y, Kim, JL, Kishi, Y, et al. Delirium phenotype by age and sex in a pooled data set of adult patients. J Neuropsychiatr Clin Neurosci. (2018) 30:294–301. doi: 10.1176/appi.neuropsych.18020024
11. Franco, JG, Trzepacz, PT, Mejía, MA, and Ochoa, SB. Factor analysis of the Colombian translation of the delirium rating scale (DRS), Revised-98. Psychosomatics. (2009) 50:255–62. doi: 10.1176/appi.psy.50.3.255
12. Leonard, M, Donnelly, S, Conroy, M, Trzepacz, P, and Meagher, DJ. Phenomenological and neuropsychological profile across motor variants of delirium in a palliative-care unit. J Neuropsychiatr Clin Neurosci. (2011) 23:180–8. doi: 10.1176/jnp.23.2.jnp180
13. Franco, JG, Trzepacz, PT, Meagher, DJ, Kean, J, Lee, Y, Kim, JL, et al. Three core domains of delirium validated using exploratory and confirmatory factor analyses. Psychosomatics. (2013) 54:227–38. doi: 10.1016/j.psym.2012.06.010
14. Thurber, S, Kishi, Y, Trzepacz, PT, Franco, JG, Meagher, DJ, Lee, Y, et al. Confirmatory factor analysis of the delirium rating scale revised-98 (DRS-R98). J Neuropsychiatr Clin Neurosci. (2015) 27:e122–7. doi: 10.1176/appi.neuropsych.13110345
15. Meagher, DJ, Leonard, M, Donnelly, S, Conroy, M, Adamis, D, and Trzepacz, PT. A longitudinal study of motor subtypes in delirium: relationship with other phenomenology, etiology, medication exposure and prognosis. J Psychosom Res. (2011) 71:395–403. doi: 10.1016/j.jpsychores.2011.06.001
16. Meagher, D, Moran, M, Raju, B, Leonard, M, Donnelly, S, Saunders, J, et al. A new data-based motor subtype schema for delirium. J Neuropsychiatr Clin Neurosci. (2008) 20:185–93. doi: 10.1176/jnp.2008.20.2.185
17. Meagher, D, Adamis, D, Leonard, M, Trzepacz, P, Grover, S, Jabbar, F, et al. Development of an abbreviated version of the delirium motor subtyping scale (DMSS-4). Int Psychogeriatr. (2014) 26:693–702. doi: 10.1017/S1041610213002585
18. Adamis, D, Scholtens, RM, de Jonghe, A, van Munster, BC, de Rooij, SE, and Meagher, DJ. Psychometric evaluation of the DMSS-4 in a cohort of elderly post-operative hip fracture patients with delirium. Int Psychogeriatr. (2016) 28:1221–8. doi: 10.1017/S1041610216000065
19. Fong, T, Tulebaev, S, and Inouye, S. Delirium in elderly adults: diagnosis, prevention and treatment. Nat Rev Neurol. (2009) 5:210–20. doi: 10.1038/nrneurol.2009.24
20. Wada, S, Inoguchi, H, Sadahiro, R, Matsuoka, YJ, Uchitomi, Y, Sato, T, et al. Preoperative anxiety as a predictor of delirium in cancer patients: a prospective observational cohort study. World J Surg. (2019) 43:134–42. doi: 10.1007/s00268-018-4761-0
21. Hempenius, L, Slaets, JP, van Asselt, DZ, Schukking, J, de Bock, GH, Wiggers, T, et al. Interventions to prevent postoperative delirium in elderly cancer patients should be targeted at those undergoing nonsuperficial surgery with special attention to the cognitive impaired patients. Eur J Surg Oncol. (2015) 41:28–33. doi: 10.1016/j.ejso.2014.04.006
22. Shah, S, Weed, HG, He, X, Agrawal, A, Ozer, E, and Schuller, DE. Alcohol-related predictors of delirium after major head and neck cancer surgery. Arch Otolaryngol Head Neck Surg. (2012) 138:266–71. doi: 10.1001/archoto.2011.1456
23. Takeuchi, M, Takeuchi, H, Fujisawa, D, Miyajima, K, Yoshimura, K, Hashiguchi, S, et al. Incidence and risk factors of postoperative delirium in patients with esophageal cancer. Ann Surg Oncol. (2012) 19:3963–70. doi: 10.1245/s10434-012-2432-1
24. Mossie, A, Regasa, T, Neme, D, Awoke, Z, Zemedkun, A, and Hailu, S. Evidence-based guideline on management of postoperative delirium in older people for low resource setting: systematic review article. Int J Gen Med. (2022) 15:4053–65. doi: 10.2147/IJGM.S349232
25. Wang, Y, and Shen, X. Postoperative delirium in the elderly: the potential neuropathogenesis. Aging Clin Exp Res. (2018) 30:1287–95. doi: 10.1007/s40520-018-1008-8
26. Shim, EJ, Noh, HL, Lee, KM, Hwang, H, Son, KL, Jung, D, et al. Trajectory of severity of postoperative delirium symptoms and its prospective association with cognitive function in patients with gastric cancer: results from a prospective observational study. Support Care Cancer. (2019) 27:2999–3006. doi: 10.1007/s00520-018-4604-4
27. Nunes, A, Trappenberg, T, and Alda, M. The definition and measurement of heterogeneity. Transl Psychiatry. (2020) 10:299. doi: 10.1038/s41398-020-00986-0
28. American Psychiatric Association. Diagnostic and statistical manual of mental disorders. 5th ed. Washington, DC: American Psychiatric Association (2013).
29. Trzepacz, PT, Mittal, D, Torres, R, Kanary, K, Norton, J, and Jimerson, N. Validation of the delirium rating scale-Revised-98: comparison with the delirium rating scale and the cognitive test for delirium. J Neuropsychiatr Clin Neurosci. (2001) 13:229–42. doi: 10.1176/jnp.13.2.229
30. Kato, M, Kishi, Y, Okuyama, T, Trzepacz, PT, and Hosaka, T. Japanese version of the delirium rating scale, Revised-98 (drs-r98-j): reliability and validity. Psychosomatics. (2010) 51:425–31. doi: 10.1176/appi.psy.51.5.425
31. Koga, Y, Tsuruta, R, Murata, H, Matsuo, K, Ito, T, Ely, EW, et al. Reliability and validity assessment of the Japanese version of the confusion assessment method for the intensive care unit (CAM-ICU). Intensive Crit Care Nurs. (2015) 31:165–70. doi: 10.1016/j.iccn.2014.10.002
32. Gaudreau, J-D, Gagnon, P, Harel, F, Tremblay, A, and Roy, M-A. Fast, systematic, and continuous delirium assessment in hospitalized patients: the nursing delirium screening scale. J Pain Symptom Manag. (2005) 29:368–75. doi: 10.1016/j.jpainsymman.2004.07.009
33. Smith, TO, Cooper, A, Peryer, G, Griffiths, R, Fox, C, and Cross, J. Factors predicting incidence of post-operative delirium in older people following hip fracture surgery: a systematic review and meta-analysis. Int J Geriatr Psychiatry. (2017) 32:386–96. doi: 10.1002/gps.4655
34. Folstein, MF, Folstein, SE, and McHugh, PR. “Mini-mental state”: a practical method for grading the cognitive state of patients for the clinician. J Psychiatr Res. (1975) 12:189–98. doi: 10.1016/0022-3956(75)90026-6
35. Kugaya, A, Akechi, T, Okuyama, T, Okamura, H, and Uchitomi, Y. Screening for psychological distress in Japanese cancer patients. Jpn J Clin Oncol. (1998) 28:333–8. doi: 10.1093/jjco/28.5.333
36. Kassie, GM, Nguyen, TA, Kalisch Ellett, LM, Pratt, NL, and Roughead, EE. Preoperative medication use and postoperative delirium: a systematic review. BMC Geriatr. (2017) 17:298. doi: 10.1186/s12877-017-0695-x
37. Miller, D, Lewis, SR, Pritchard, MW, Schofield-Robinson, OJ, Shelton, CL, Alderson, P, et al. Intravenous versus inhalational maintenance of anaesthesia for postoperative cognitive outcomes in elderly people undergoing non-cardiac surgery. Cochrane Database Syst Rev. (2018) 2018:Cd012317. doi: 10.1002/14651858.CD012317.pub2
38. Zhu, Y, Wang, G, Liu, S, Zhou, S, Lian, Y, Zhang, C, et al. Risk factors for postoperative delirium in patients undergoing major head and neck cancer surgery: a meta-analysis. Jpn J Clin Oncol. (2018) 47:505–11. doi: 10.1093/jjco/hyx029
39. Na, S, Xumin, L, and Yong, G. (2010). “Research on k-means clustering algorithm: an improved k-means clustering algorithm”. in Third International Symposium on Intelligent Information Technology and Security Informatics; 2010 April 2–4. Jinggangshan, China. IEEE p. 63–67.
40. JB, MacQueen. (1967). “Some methods for classification and analysis of multivariate observations”. in Cam LM, Neyman J, editors. Proceedings of the Fifth Berkeley Symposium on Mathematical Statistics and Probability Vol. 1: Statistics. Berkeley: University of California Press. p. 281–297.
41. Akaike, H. Information theory and an extension of the maximum likelihood principle In: Parzen E, Tanabe K, Kitagawa G, editors. Selected Papers of Hirotugu Akaike: Springer Series in Statistics. New York, NY: Springer (1998), p. 199–213.
42. Kodinariya, TM, and Makwana, PR. Review on determining number of cluster in k-means clustering. Int J Adv Res Comput Sci Manag Stud. (2013) 1:90–5.
43. Bzdok, D, and Meyer-Lindenberg, A. Machine learning for precision psychiatry: opportunities and challenges. Biol Psychiatry Cogn Neurosci Neuroimaging. (2018) 3:223–30. doi: 10.1016/j.bpsc.2017.11.007
44. Han, Y, Wu, J, Qin, Z, Fu, W, Zhao, B, Li, X, et al. Melatonin and its analogues for the prevention of postoperative delirium: a systematic review and meta-analysis. J Pineal Res. (2020) 68:e12644. doi: 10.1111/jpi.12644
45. Hatta, K, Kishi, Y, Wada, K, Takeuchi, T, Hashimoto, N, Suda, K, et al. Real-world effectiveness of ramelteon and suvorexant for delirium prevention in 948 patients with delirium risk factors. J Clin Psychiatry. (2019) 81:19m12865. doi: 10.4088/JCP.19m12865
46. Hatta, K, Kishi, Y, Wada, K, Takeuchi, T, Ito, S, Kurata, A, et al. Preventive effects of suvorexant on delirium: a randomized placebo-controlled trial. J Clin Psychiatry. (2017) 78:e970–9. doi: 10.4088/JCP.16m11194
47. Angka, L, Khan, ST, Kilgour, MK, Xu, R, Kennedy, MA, and Auer, RC. Dysfunctional natural killer cells in the aftermath of cancer surgery. Int J Mol Sci. (2017) 18:1787. doi: 10.3390/ijms18081787
48. Meagher, D, Adamis, D, Trzepacz, P, and Leonard, M. Features of subsyndromal and persistent delirium. Br J Psychiatry. (2012) 200:37–44. doi: 10.1192/bjp.bp.111.095273
49. Sadahiro, R, Wada, S, Matsuoka, YJ, Uchitomi, Y, Yamaguchi, T, Sato, T, et al. Prevention of delirium with agitation by yokukansan in older adults after cancer surgery. Jpn J Clin Oncol. (2022) 52:1276–81. doi: 10.1093/jjco/hyac123
50. Wildes, TS, Mickle, AM, Ben Abdallah, A, Maybrier, HR, Oberhaus, J, Budelier, TP, et al. Effect of electroencephalography-guided anesthetic administration on postoperative delirium among older adults undergoing major surgery: the ENGAGES randomized clinical trial. JAMA. (2019) 321:473–83. doi: 10.1001/jama.2018.22005
Keywords: postoperative delirium, hypothesis-free categorization, K-means clustering, delirium rating scale-revised-98, phenotype, cancer surgery
Citation: Sri-iesaranusorn P, Sadahiro R, Murakami S, Wada S, Shimizu K, Yoshida T, Aoki K, Uezono Y, Matsuoka H, Ikeda K and Yoshimoto J (2023) Data-driven categorization of postoperative delirium symptoms using unsupervised machine learning. Front. Psychiatry. 14:1205605. doi: 10.3389/fpsyt.2023.1205605
Edited by:
Maya Bizri, American University of Beirut, LebanonReviewed by:
Ram J. Bishnoi, University of South Florida, United StatesSemra Bulbuloglu, Istanbul Aydın University, Türkiye
Copyright © 2023 Sri-iesaranusorn, Sadahiro, Murakami, Wada, Shimizu, Yoshida, Aoki, Uezono, Matsuoka, Ikeda and Yoshimoto. This is an open-access article distributed under the terms of the Creative Commons Attribution License (CC BY). The use, distribution or reproduction in other forums is permitted, provided the original author(s) and the copyright owner(s) are credited and that the original publication in this journal is cited, in accordance with accepted academic practice. No use, distribution or reproduction is permitted which does not comply with these terms.
*Correspondence: Junichiro Yoshimoto, anVuaWNoaXJvLnlvc2hpbW90b0BmdWppdGEtaHUuYWMuanA=; Ryoichi Sadahiro, cnNhZGFoaXJAbmNjLmdvLmpw
†These authors have contributed equally to this work