- 1Department of Neurology, Changxing People’s Hospital of Zhejiang Province, Huzhou, China
- 2Department of Neurology, The Second Affiliated Hospital, Zhejiang University School of Medicine, Hangzhou, China
- 3Institute of Brain Science and Department of Physiology, School of Basic Medical Sciences, Hangzhou Normal University, Hangzhou, China
- 4Zhejiang Philosophy and Social Science Laboratory for Research in Early Development and Childcare, Hangzhou Normal University, Hangzhou, China
- 5Zhejiang Key Laboratory for Research in Assessment of Cognitive Impairments, Hangzhou, China
Background: Childhood trauma has been found to have an important impact on mental health. However, little is known regarding the intercorrelations between childhood trauma and mental health during the COVID-19 pandemic. This study aimed to investigate such complex interplay between childhood trauma, depression, anxiety, post-traumatic stress level during the COVID-19 pandemic, and fear of COVID-19 using network analysis.
Methods: A total of 1,247 college students were recruited and were asked to complete a series of questionnaires, including the Childhood Trauma Questionnaire, Patient Health Questionnaire, Generalized Anxiety Disorder Scale, Post-traumatic Stress Checklist—Civilian version, and Fear of COVID-19 Scale. The Gaussian graphical model with the scores of the questionnaires as nodes was estimated. The partial correlations between nodes were calculated as edges. Moreover, network comparison tests were conducted to compare the network patterns between participants with high levels of childhood trauma and low levels of childhood trauma.
Results: Childhood trauma was found to be connected to depression, anxiety, and post-traumatic stress level. The node of childhood trauma exhibited the strongest strength and the highest expected influence in the network. Participants with high levels of childhood trauma and participants with low levels of childhood trauma showed comparable network structure and global strength.
Conclusion: Our findings revealed a complex network pattern between childhood trauma and different mental health problems, indicating that childhood trauma might be a risk factor for mental health during the COVID-19 pandemic.
1. Introduction
The COVID-19 pandemic has become a global health crisis. Apart from the significant challenges to public health, the pandemic has serious impacts on individual’s mental health worldwide (1). The prevalence of psychological distress, depression, anxiety, and post-traumatic stress symptoms has significantly increased during the pandemic (2–5). Importantly, previous studies suggested that individuals with childhood trauma are at higher risk of experiencing more mental health problems during the COVID-19 pandemic (6, 7).
Childhood trauma refers to experiences that are emotionally or physically harmful or distressing, occurring during the developmental period of childhood (8). Previous studies have documented a relatively high prevalence of childhood trauma in college students (9, 10). For example, a survey among 21 countries by the World Mental Health Initiative recruited 51,945 adults and found approximately 40% of the population had adverse childhood experiences (11). Childhood trauma includes various types, including physical and emotional abuse, sexual abuse, or neglect (12). Different types of childhood trauma have distinct impacts on neuropsychological development (13). Physical and emotional abuse or neglect has been found to lead to emotion dysregulation and cognitive decline (14, 15). It has been considered a risk factor contributing to a wide range of psychological difficulties and mental disorders (16, 17). Moreover, individuals who have been exposed to traumatic events in childhood have been found to have higher levels of fear and stress in response to later stressors (18).
Since the end of 2019, the COVID-19 pandemic has become an ongoing and significant stressor for individuals worldwide (6). Recent studies have explored how individuals with childhood trauma experienced such stressor during the pandemic (7, 19–21). Moreover, significant correlations between adverse childhood experiences and mental health problems during the pandemic have been found (19–21). For example, Guo et al. (19) found that individuals with higher levels of adverse childhood experiences (including physical abuse, emotional abuse, sexual abuse, family neglect, and household dysfunctions) had increased levels of anxiety and post-traumatic stress symptoms. Doom et al. (21) found that higher levels of adverse childhood experiences were significantly associated with higher levels of depression during the COVID-19 pandemic. Furthermore, individuals with childhood abuse have been found to have increased levels of COVID-19 fear and post-traumatic stress symptoms (6). Therefore, previous research indicated that individuals with childhood trauma might be more susceptible to heightened anxiety, depression, and post-traumatic stress symptoms during the COVID-19 pandemic. Previous studies on the mental health consequences of the COVID-19 pandemic have found greater increases in depression, anxiety, and post-traumatic stress level in the general population (2–5, 22, 23). Therefore, the present study will focus on the above three mental health problems.
Network analysis, which is a novel method to investigate the relationships and interactions between different symptoms, has been used extensively in psychology and psychiatry (24, 25). In network analysis, symptoms are represented as nodes, and the relationships between symptoms are represented as edges (26, 27). Importantly, an edge linking two nodes represents the independent relationship after controlling for the effects of the rest nodes in the network. The network structure and the network properties such as centrality and betweenness could be examined. Therefore, network analysis can elucidate the interactive pattern of symptoms (28). Some studies have utilized network analysis to explore the network structure of psychological state during the pandemic (29–32). Feng et al. (29) used a network approach to examine the relationships between depression and intolerance of uncertainty in university students. Ge et al. (30) and Ventura-Leon et al. (32) explored the network structure involving depression and anxiety symptoms. However, limited studies examined the network structure between childhood trauma and other mental health problems.
Therefore, this study aimed to use network analysis to investigate the relationships between childhood trauma, depression, anxiety, post-traumatic stress level during the COVID-19 pandemic, and fear of COVID-19. As previous findings using a non-network approach have found that individuals with higher levels of childhood trauma reported more mental health problems during the COVID-19 pandemic (19–21), we hypothesized that (1) childhood trauma would be connected to mental health problems and fear of COVID-19, (2) the node of childhood trauma would have high centrality indices, and (3) compared with participants with low levels of childhood trauma, participants with high levels of childhood trauma would have more mental health problems. The networks of both groups would exhibit different structures.
2. Materials and methods
2.1. Participants and procedure
A total of 1,247 students (361 males; mean age = 19.80 years, SD = 1.48) were recruited from Hangzhou Normal University in Hangzhou, China. The participants who gave consent completed a set of self-report questionnaires online. Each participant received 10 RMB as an incentive. The invalid responses were detected using 5 pairs of validity check items (33, 34). Participants with scores over 3 were excluded due to low-quality responses. This study was approved by the Research Ethics Review Board of the School of Basic Medical Sciences, Hangzhou Normal University.
2.2. Measures
2.2.1. The childhood trauma questionnaire—short form
The CTS-SF is widely used to assess childhood trauma (35, 36). The CTQ-SF comprises 28 items rated from 1 (never true) to 5 (very often true). The higher scores indicate greater severity of childhood trauma the participant experienced. The CTQ-SF had five subscales: emotional abuse (node: CTQ1), physical abuse (node: CTQ2), sexual abuse (node: CTQ3), emotional neglect (node: CTQ4), and physical neglect (node: CTQ5). The Chinese version of the CTQ-SF has been shown to have good psychometric properties (35). The Cronbach’s alpha for the CTQ-SF in the present study was 0.78.
To compare the network pattern between participants with high and low levels of childhood trauma, the CTS-SF was further used to define participants with high levels of childhood trauma and participants with low levels of childhood trauma. A total CTQ score higher than 1 SD above the mean score of the whole sample was defined as “high levels of childhood trauma” (⩾ 54) and a lower total CTQ score (< 54) was classified as “low levels of childhood trauma” (37–39).
2.2.2. The patient health questionnaire
The PHQ-9 is a 9-item questionnaire for assessing depressive levels (40, 41). Each item is rated on a 5-point Likert scale (from 0 as “no day” to 4 as “almost every day”), with higher scores indicating higher levels of depression. The Chinese version of the PHQ-9 has been found to have good reliability and validity (40). The Cronbach’s alpha for the PHQ-9 in this study was 0.86. The total score of the PHQ-9 was added as an indicator of depressive levels (node: PHQ) in the network analysis.
2.2.3. The generalized anxiety disorder scale
The GAD-7 is a 7-item questionnaire used for measuring generalized anxiety (42, 43). Items of the GAD-7 are rated on a 4-point Likert scale (from 0 as “no day” to 3 as “almost every day”). The higher scores indicate higher levels of anxiety. The Chinese version of the GAD-7 has been found to have good test–retest reliability and convergent validity (42). The Cronbach’s alpha for the GAD-7 in this study was 0.90. The total score of the GAD-7 was added as an indicator of anxiety (node: GAD) in the network analysis.
2.2.4. The post-traumatic stress checklist—civilian version
The PCL-C is a 17-item self-report scale for measuring post-traumatic stress symptoms (44, 45). In the present study, the PCL-C was used to measure the post-traumatic stress level during the COVID-19 pandemic. The PCL-C consists of three subscales, including re-experiencing (node: PCL1), avoidance (node: PCL2), and hyperarousal (node: PCL3). Each item is rated on a 5-point Likert scale (from 1 “not at all” to 5 “extremely bothered”), with higher scores indicating more severe symptoms. The Chinese version of the PCL-C has shown good internal consistency and validity (44). The Cronbach’s alpha for the PCL-C in the present study was 0.93.
2.2.5. The fear of COVID-19 scale
The FCV-19S is a valid tool for assessing individuals’ fear of COVID-19 (46, 47). It contains 7 items with a 5-point Likert scale from 1 (strongly disagree) to 5 (strongly agree). The higher scores of the FCV-19S indicate higher levels of fear of COVID-19. The Chinese version of the FCV-19S has been shown to have good reliability and validity (46). The Cronbach’s alpha for the FCV-19S in the present study was 0.87. The total score of the FCV-19S was added as an indicator of fear of COVID-19 (node: FCV).
2.3. Data analyses
2.3.1. Descriptive analyses
All descriptive statistics were computed in IBM SPSS Statistics 21 (IBM Corp., 2012). The mean and standard deviation of age, education duration, and total and subscale scores of all questionnaires were calculated for the entire sample as well as groups of participants with high and low levels of childhood trauma. We examined gender differences in the scores of questionnaires using independent sample t-tests. Pearson correlation analysis and partial correlation analysis were conducted to investigate the relationship between the scores of the CTQ-SF and other questionnaires’ scores in the entire sample (Supplementary Table S1). Independent sample t-tests were conducted to examine the group differences in fear of COVID-19, depression, anxiety, and post-traumatic level. Moreover, we included age, gender, and years of education as covariates to examine the group differences using analysis of covariance (ANCOVA).
2.3.2. Network estimation
Packages of bootnet (48), qgraph (49), and networktools (50) in R studio software (Version: 2023.06.0 + 421, available at https://posit.co/download/rstudio-desktop/) were used to construct networks in our study. We used the Gaussian graphical model as the estimation model. The partial correlation matrix was computed using the Extended Bayesian Information Criterion Graphical Least Absolute Shrinkage and Selection Operator (EBICglasso) procedure to improve the accuracy and interpretability of our network (51, 52). Bonferroni correction was applied to control for a false positive rate (53). Symptoms were represented as the nodes, and correlations between nodes were represented as the edges, ranging from −1 to 1.
2.3.3. Network centrality
R packages of qgraph (49) and networktools (50) were used to calculate the centrality of nodes in the networks. Centrality was a measure used in a network to quantify the significance of nodes within that network based on the connectedness and interactions of each node with other nodes. Centrality indices, including strength, closeness, and betweenness, were computed. Expected influence is referred to evaluate the predicted significance of certain nodes in the entire network. It was argued that in a network with both positive and negative edges, the expected influence is more appropriate to assess the nature and strength of the significance of nodes (54). Hence, we included the expected influence as the fourth centrality index. Results were converted to z-scores to compare different centrality indices on the same scale.
The bridge centrality between clusters of symptoms represented the importance of certain nodes in serving as a connecting link or shared manifestation between various sets of symptoms (55). Subscales of childhood trauma and PTSD were set as two clusters of symptoms. Depression and anxiety were considered a cluster of symptoms. Finally, fear of COVID-19 was set as an independent cluster of the symptoms. Bridge centrality was calculated to identify nodes that connect symptoms of the four different mental disorders.
2.3.4. Network accuracy and stability
R packages of bootnet (Version 1.5) (48) and ggplot2 (56) were used to examine the accuracy and stability of our network. The bootstrapping method was applied with 1,000 iterations. The edge weights accuracy test was conducted to examine the reliability of our network to describe our sample characteristics. The centrality stability test was employed to illustrate the stability of our network across samples, which was quantified by the correlation stability coefficient (CS coefficient). In order to consider a network stable, the coefficient should be at least 0.25 and preferably above 0.5 (48).
2.3.5. Network comparison tests
Network comparison tests (NCTs) were performed using the R package of NetworkComparisonTest to explore the differences between the two networks constructed by samples of participants with high and low levels of childhood trauma as well as between gender-stratified groups (57). A two-tailed permutation test (n = 10,000) with p < 0.05 was used to compare the global strength, centrality invariance, and edge weights of the two networks. Bonferroni correction was applied in multiple comparisons to control for a false-positive rate (53).
3. Results
3.1. Sample characteristics
Demographic information and a descriptive summary of the questionnaires’ scores are shown in Table 1. Based on the scores of the CTS-SF, 125 participants had high levels of childhood trauma and the rest of the participants were grouped as having low levels of childhood trauma (n = 1,122). The independent sample t-test found that participants with high levels of childhood trauma had significantly higher levels of depression, anxiety, fear, and post-traumatic stress symptoms for COVID-19. The results remained unchanged after controlling for age, gender, and years of education (see Supplementary Results and Supplementary Table S2). Male participants had significantly higher levels of childhood trauma and post-traumatic stress symptoms for COVID-19 and lower levels of depression and anxiety than female participants (see Supplementary Table S5).
3.2. Network estimation
The network visualization of the entire sample (n = 1,247) with 11 nodes is shown in Figure 1. As expected, positive correlations were found between the nodes that represented the subscales, indicating relatively strong “within-subscale” edges. As for the “between-scale” edges, the strongest one was the edge between the PHQ (the score of depression) and the GAD (the score of anxiety) (regularized partial correlation = 0.57), followed by the edge between the FCV (the score of fear of COVID-19) and the PCL1 (the score of the re-experiencing subscale) (regularized partial correlation = 0.29). Moreover, the PCL3 (the score of the hyperarousal subscale) was positively connected to the PHQ (the score of depression) and the GAD (the score of anxiety) (the partial correlations were 0.22 and 0.19, respectively). The CTQ1 (the score of emotional abuse subscale) was found to be positively connected to the PHQ (the score of depression), the GAD (the score of anxiety), and the PCL2 (the score of the avoidance subscale), and the CTQ5 (the score of the physical neglect subscale) was found to be positively connected to the PCL3 (the score of the hyperarousal subscale).
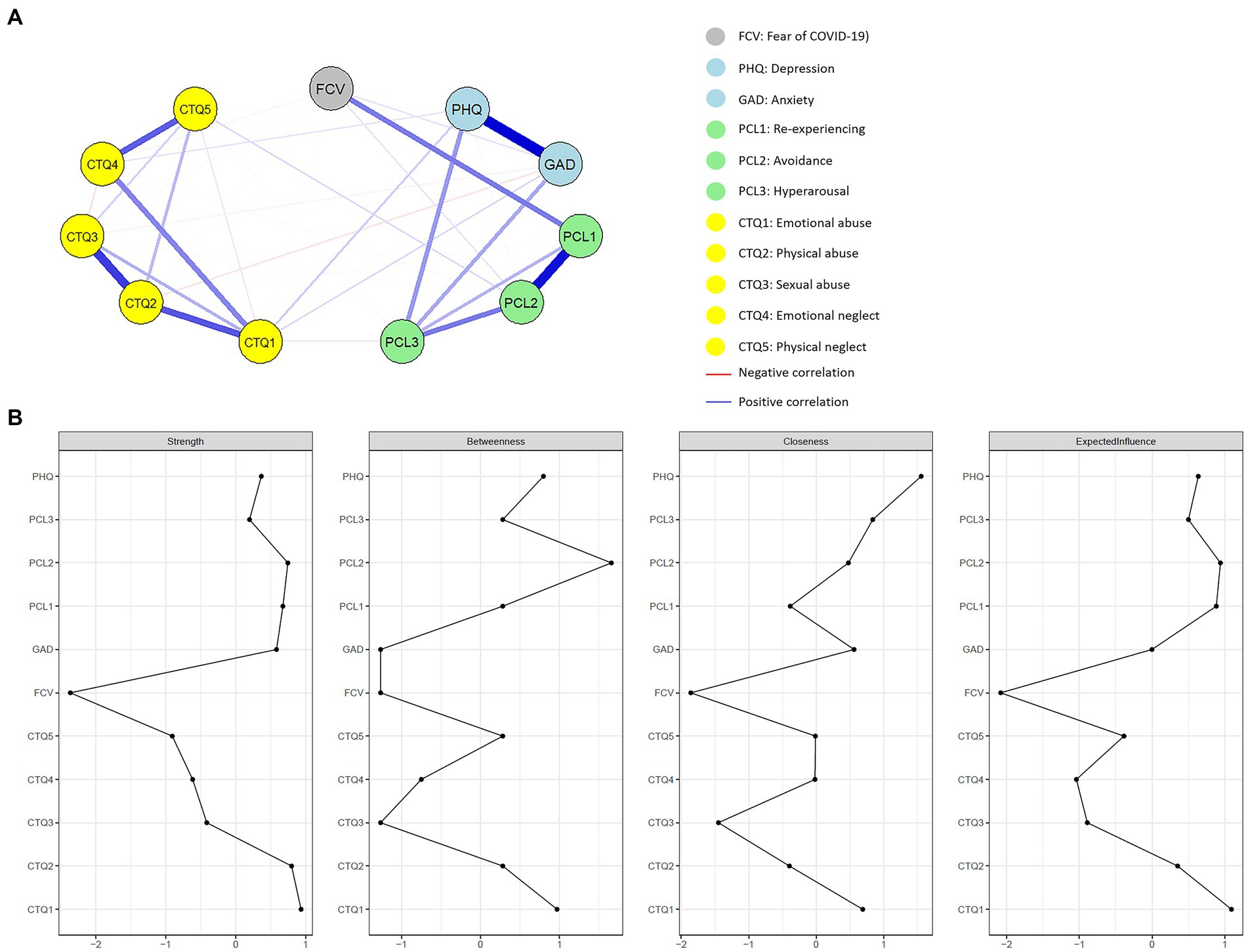
Figure 1. (A) Visualization of the regularized network for the entire sample. Clusters of symptoms are represented by different colors. The gray node represents the fear of COVID-19. The light blue nodes represent depression and anxiety. The green nodes are a combination of traumatic symptoms post-COVID-19. Symptoms of childhood trauma are represented by the yellow nodes. Blue lines mean positive connections between nodes, whereas red lines mean negative connections. Thicker and shorter edges represent larger partial correlations. (B) Centrality indices plot include strength, betweenness, closeness, and expected influence. Values were converted to standardized z-scores. FCV, fear of Coronavirus disease 2019 (COVID-19) scale; PHQ, patient health questionnaire; GAD, generalized anxiety disorder questionnaire; PCL, post-traumatic stress disorder checklist—civilian; CTQ, childhood trauma questionnaire.
3.3. Network centrality
Figure 1 and Table 2 display the four centrality indices of the 11 nodes, which reflect the structural position and significance of each node. The CTQ1 (the score of emotional abuse subscale), the CTQ2 (the score of the physical abuse subscale), and the PCL2 (the score of the avoidance subscale) were the top three nodes in strength centrality, indicating that these nodes had the strongest connections with other nodes in the network. As for the betweenness centrality, the PCL2 (the score of avoidance subscale), the CTQ1 (the score of emotional abuse subscale), and the PHQ (the score of depression) had the most interactions with other nodes in the network. Regarding the closeness centrality, the PHQ (the score of depression), the PCL3 (the score of the hyperarousal subscale), and the CTQ1 (the score of emotional abuse subscale) had the shortest average distance with the other nodes, which suggested that these nodes were more closely connected with the other nodes in the network.
The results of the bridge centrality test are shown in Figure 2 and Supplementary Table S3. The PCL3 (the score of the hyperarousal subscale) had the greatest centrality in both the strength and expected influence, showing the occurrence of hyperarousal in all of the four clusters of symptoms.
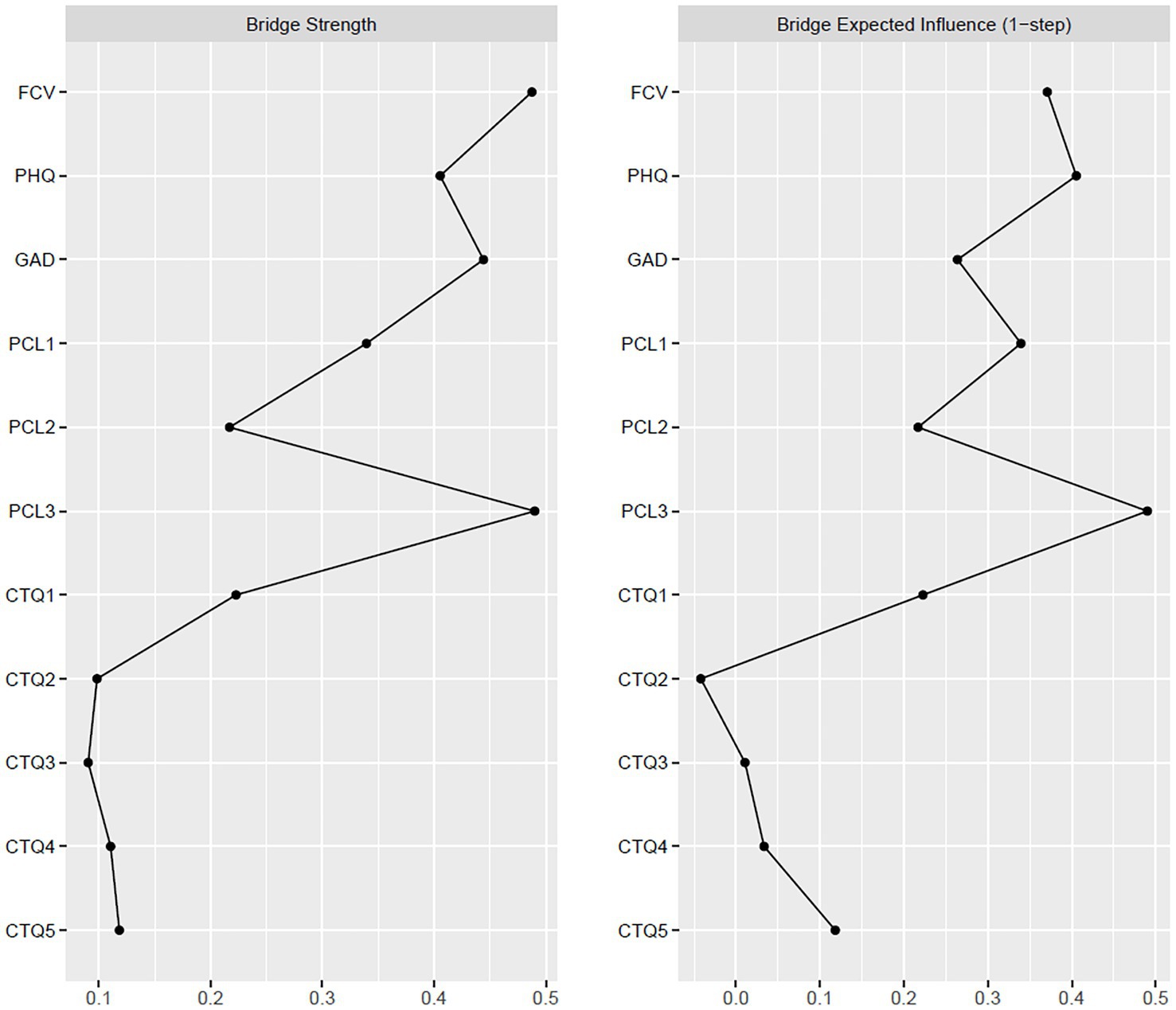
Figure 2. Bridge centrality indices plots of strength (left) and expected influence (right) for the entire sample (n = 1,247). FCV, fear of Coronavirus disease 2019 (COVID-19) scale; PHQ, patient health questionnaire; GAD, generalized anxiety disorder questionnaire; PCL, post-traumatic stress disorder checklist—civilian; PCL1, re-experiencing; PCL2, avoidance; PCL3, hyperarousal; CTQ, childhood trauma questionnaire; CTQ1, emotional abuse; CTQ2, physical abuse; CTQ3, sexual abuse; CTQ4, emotional neglect; CTQ5, physical neglect.
3.4. Network accuracy and stability
Figure 3 presents the network stability and accuracy test of the entire sample. Results showed a strength centrality stability coefficient of 0.75, meaning excellent stability of the network. Additionally, as illustrated in Figure 3, the accuracy test showed small confidence intervals (CIs), indicating a reliable estimation of the edge weights.
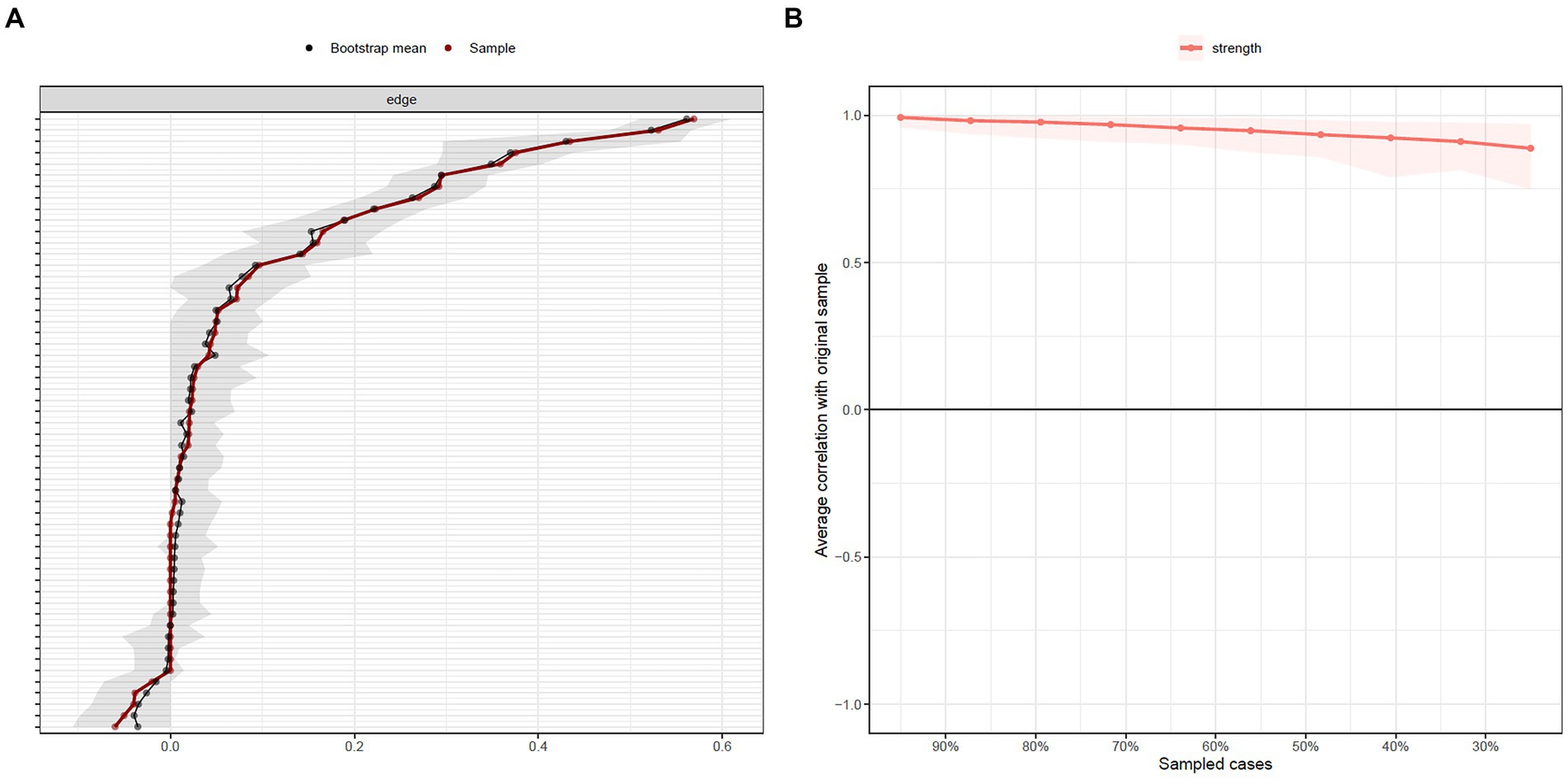
Figure 3. (A) Bootstrapped confidence intervals (CIs) of edge weights for the entire sample (n = 1,247). Dots fitted on the red line are sample edge weights and on the black line are bootstrapped means. The gray area indicates the 95% CIs. (B) Average correlations of strength centrality with the original sample when n % of sample cases were dropped. Lines indicate the means and the areas indicate the range from the 2.5 quantile to the 97.5 quantile.
3.5. Network comparison test
The visualized networks of the sample with high (Figure 4A; n = 125) and low (Figure 4B; n = 1,122) levels of childhood trauma as well as their centrality test are presented in Figure 4. The centrality and expected influence of the network for the two groups are shown in Supplementary Table S4. The global strength invariance test found no significant differences between the two networks (the strength difference was 0.28, p = 0.645), indicating that the two networks shared similar global strength of connections. The network invariance test also found no significant differences between the two networks (M = 0.27, p = 0.309). However, the node with the strongest betweenness for participants with high levels of childhood trauma was the CTQ1 (the score of the emotional abuse subscale), while the PCL3 (the score of the hyperarousal subscale) was the node with the strongest betweenness in the network for participants with low levels of childhood trauma. Moreover, the edge invariance test shows significant differences in FCV-CTQ1, CTQ3-CTQ4, and CTQ4-CTQ5. In addition, the network comparison test did not find a significant difference between the male and the female groups (Supplementary Table S6).
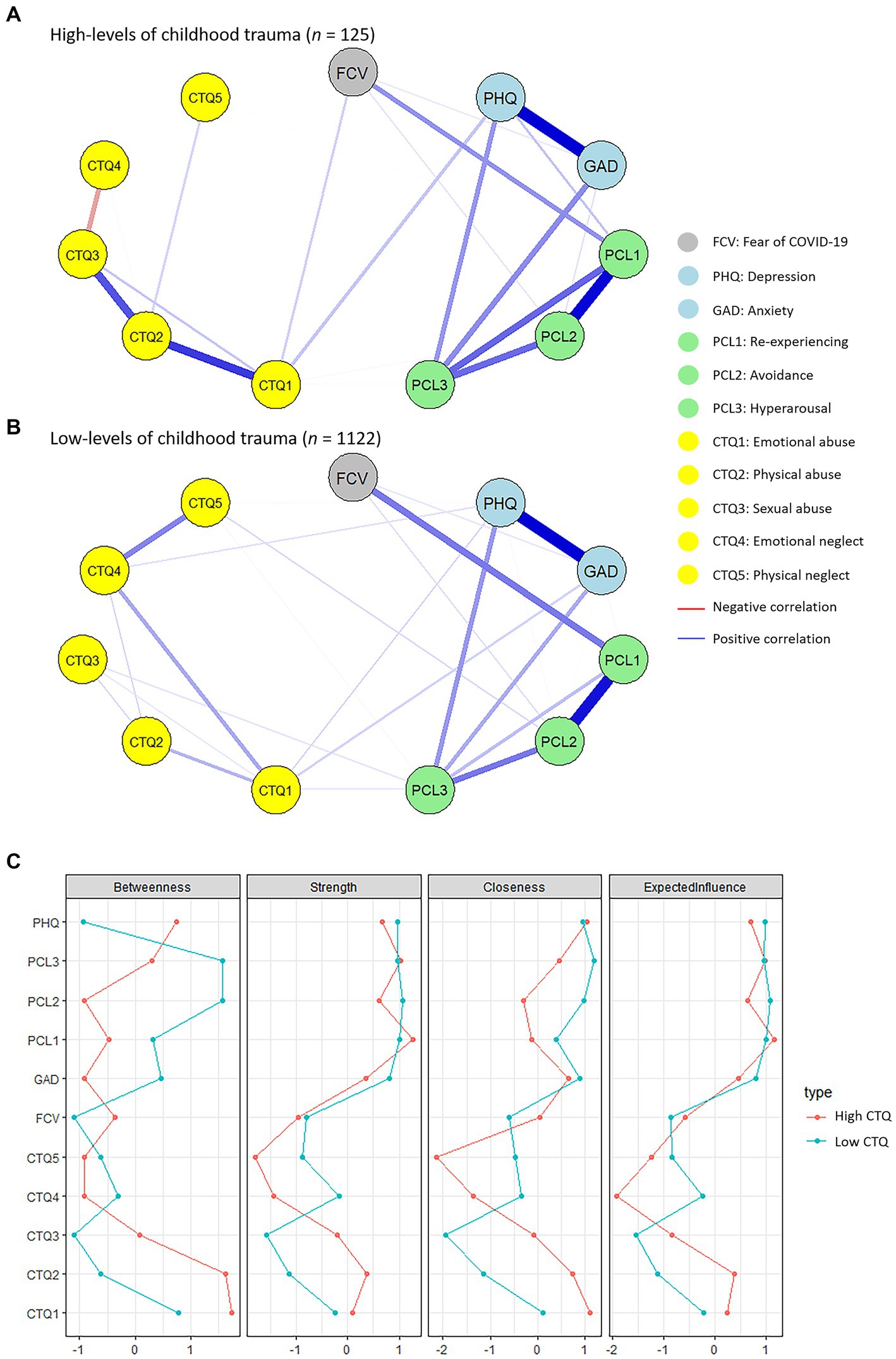
Figure 4. (A) Network visualization of people with high levels of childhood trauma (n = 125). (B) Network visualization of people with low levels of childhood trauma (n = 1,122). The gray nodes represent the fear of COVID-19. The light blue nodes represent depression and anxiety. The green nodes are a combination of traumatic symptoms post-COVID-19. Symptoms of childhood trauma are represented by the yellow nodes. Blue lines are positive correlations between nodes, and red lines are negative correlations. The thicker and shorter the lines, the stronger and closer the connections. FCV, fear of Coronavirus disease 2019 (COVID-19) scale; PHQ, patient health questionnaire; GAD, generalized anxiety disorder questionnaire; PCL, post-traumatic stress disorder checklist—civilian; CTQ, childhood trauma questionnaire. (C) Centrality indices plot, including strength, betweenness, closeness, and expected influence, of the two networks. The red line represents individuals with high levels of childhood trauma and the blue line represents individuals with low levels of childhood trauma. Centrality values are presented in z-scores.
4. Discussion
Applying network analysis, the network structure between various types of childhood trauma and mental health problems during the COVID-19 pandemic was modeled. Consistent with our hypothesis, we found that the nodes of childhood trauma were connected to depression, anxiety, and post-traumatic stress level. Moreover, the nodes of childhood trauma showed the strongest strength centrality, indicating the important role of childhood trauma in the network. The network comparison test between participants with high and low levels of childhood trauma revealed different network structures. Furthermore, compared with participants with low levels of childhood trauma, participants with high levels of childhood trauma showed significantly higher levels of depression, anxiety, fear, and post-traumatic stress for COVID-19. Our findings suggest that individuals who have experienced childhood trauma may be more susceptible to experiencing these mental health difficulties during the COVID-19 pandemic.
Childhood trauma (the CTQ1 node and the CTQ2 node) was found to have the strongest strength centrality in the network based on the whole sample, indicating that childhood trauma played a central role in the network structure and highlighting the significant impact that childhood trauma can have on mental health outcomes during the pandemic. Our findings are consistent with previous studies which found positive correlations between childhood trauma and mental health problems during the COVID-19 pandemic (6, 58). Regarding the betweenness centrality and the closeness centrality, the findings suggested that the post-traumatic stress level (the PCL2 node and the PCL3 node), depression (the PHQ node), and childhood trauma (the CTQ1 node) played an important role in the network. These findings are in line with previous findings (59, 60). Childhood trauma we measured includes emotional abuse (the CTQ1 node), physical abuse (the CTQ2 node), sexual abuse (the CTQ3 node), emotional neglect (the CTQ4 node), and physical neglect (the CTQ5 node). Our findings found that emotional abuse has the highest centrality indexed in the whole network. Notably, individuals with more childhood trauma (especially emotional abuse) have been found to experience more psychological distress during the coronavirus (61). Therefore, interventions on emotion regulation mechanisms (62) may have potential effects on mental health for individuals with childhood trauma during the COVID-19 pandemic. Although studies using network analysis to examine the impacts of childhood trauma on mental health were scarce, some authors have adopted this approach to explore the impacts of the COVID-19 pandemic on mental health (63–66). For example, Zavlis et al. (63) included depression, anxiety, trauma symptoms, COVID-specific anxiety, and viral exposure to construct the network. They found no associations between viral exposure and symptoms, which may support our findings of weak connections between the FCV node with other nodes (63). Furthermore, Ventura-Leon et al. (64) revealed that the symptoms of depression and anxiety were the most central symptoms in their network. Meanwhile, they found that the depressive symptoms bridged the symptoms of stress and anxiety (64), which was similar to our network structure. Bridge centrality is usually used to understand comorbidity in network analysis (55). Hyperarousal had the highest bridge centrality in our study, demonstrating its significance in the development of both fear of the pandemic, depression, anxiety, and PTSD problems and its high co-occurrence with childhood maltreatment. This result was in line with the study, which found that hyperarousal symptoms have a mediation effect between childhood maltreatment and depression (67). Our findings indicate that hyperarousal might be a risk factor for mental health problems.
Additionally, when comparing the network structures between participants with high and low levels of childhood trauma, different structure was observed. This suggests that the presence and severity of childhood trauma can lead to variations in the network connections and dynamics of mental health problems during the pandemic. In terms of group effect, participants with high levels of childhood trauma displayed significantly more mental health problems compared with those with low levels of childhood trauma. Such findings are consistent with previous findings, which demonstrated more trauma symptoms and mental distress in individuals with childhood abuse (60, 68). Moreover, it has been widely recognized that childhood trauma is a risk factor contributing to the development of mental disorders, such as affective disorders, post-traumatic stress disorder, and schizophrenia (15, 69, 70).
It is important to note that this study has several limitations. First, the data collected relied on self-report measures, which may be subject to recall bias or social desirability bias. Second, the participants recruited in our study were college students. The findings may not be generalizable to other populations. Future research should aim to replicate these findings in diverse samples. Finally, the sample size of the group with high levels of childhood trauma was relatively small. A larger sample size is recommended for future studies to conduct network comparison tests.
In conclusion, the present study applied network analysis to investigate the intercorrelations of childhood trauma, depression, anxiety, fear, and post-traumatic stress levels related to COVID-19. The findings support the specific links between childhood trauma and mental health problems. These findings underscore the critical need for targeted interventions and support for individuals who have experienced childhood trauma, particularly during times of the COVID-19 pandemic.
Data availability statement
The raw data supporting the conclusions of this article will be made available by the authors, without undue reservation.
Ethics statement
The studies involving humans were approved by the Research Ethics Review Board of the School of Basic Medical Sciences, Hangzhou Normal University. The studies were conducted in accordance with the local legislation and institutional requirements. The participants provided their written informed consent to participate in this study.
Author contributions
X-lC and HHei collaboratively designed the study. JX and HHua recruited participants and collected data. LZ analyzed the data. X-lC, LZ, and JX wrote the drafts of the manuscript. PF, MZ, and HHei interpreted the findings and commented on the manuscript critically. All authors contributed to the article and approved the submitted version.
Funding
This study was supported by a grant from the Research Project of Shanghai Science and Technology Commission (20dz2260300), the Fundamental Research Funds for the Central Universities, and the Starting Research Fund from Hangzhou Normal University.
Acknowledgments
The authors would like to thank all the participants who took part in the studies.
Conflict of interest
The authors declare that the research was conducted in the absence of any commercial or financial relationships that could be construed as a potential conflict of interest.
Publisher’s note
All claims expressed in this article are solely those of the authors and do not necessarily represent those of their affiliated organizations, or those of the publisher, the editors and the reviewers. Any product that may be evaluated in this article, or claim that may be made by its manufacturer, is not guaranteed or endorsed by the publisher.
Supplementary material
The Supplementary material for this article can be found online at: https://www.frontiersin.org/articles/10.3389/fpsyt.2023.1251473/full#supplementary-material
References
1. Salari, N, Hosseinian-Far, A, Jalali, R, Vaisi-Raygani, A, Rasoulpoor, S, Mohammadi, M, et al. Prevalence of stress, anxiety, depression among the general population during the COVID-19 pandemic: a systematic review and meta-analysis. Glob Health. (2020) 16:57. doi: 10.1186/s12992-020-00589-w
2. Liu, Y, Zou, L, Yan, S, Zhang, P, Zhang, J, Wen, J, et al. Burnout and post-traumatic stress disorder symptoms among medical staff two years after the COVID-19 pandemic in Wuhan, China: social support and resilience as mediators. J Affect Disord. (2023) 321:126–33. doi: 10.1016/j.jad.2022.10.027
3. Daly, M, and Robinson, E. Acute and longer-term psychological distress associated with testing positive for COVID-19: longitudinal evidence from a population-based study of US adults. Psychol Med. (2023) 53:1603–10. doi: 10.1017/S003329172100324X
4. Fang, X, Zhang, J, Teng, C, Zhao, K, Su, KP, Wang, Z, et al. Depressive symptoms in the front-line non-medical workers during the COVID-19 outbreak in Wuhan. J Affect Disord. (2020) 276:441–5. doi: 10.1016/j.jad.2020.06.078
5. Castro-de-Araujo, LFS, Rodrigues, EDS, Machado, DB, Henriques, CMP, Verotti, MP, Goncalves, AQ, et al. Multimorbidity worsened anxiety and depression symptoms during the COVID-19 pandemic in Brazil. J Affect Disord. (2022) 314:86–93. doi: 10.1016/j.jad.2022.07.005
6. Tsur, N, and Abu-Raiya, H. COVID-19-related fear and stress among individuals who experienced child abuse: the mediating effect of complex posttraumatic stress disorder. Child Abuse Negl. (2020) 110:104694. doi: 10.1016/j.chiabu.2020.104694
7. Gewirtz-Meydan, A, and Lassri, D. A profile analysis of COVID-19 stress-related reactions: the importance of early childhood abuse, psychopathology, and interpersonal relationships. Child Abuse Negl. (2022) 130:105442. doi: 10.1016/j.chiabu.2021.105442
8. Morgan, C, and Fisher, H. Environment and schizophrenia: environmental factors in schizophrenia: childhood trauma--a critical review. Schizophr Bull. (2007) 33:3–10. doi: 10.1093/schbul/sbl053
9. Read, JP, Ouimette, P, White, J, Colder, C, and Farrow, S. Rates of DSM-IV-TR trauma exposure and posttraumatic stress disorder among newly matriculated college students. Psychol Trauma. (2011) 3:148–56. doi: 10.1037/a0021260
10. Strand, EB, Brandt, J, Rogers, K, Fonken, L, Chun, R, Conlon, P, et al. Adverse childhood experiences among veterinary medical students: a multi-site study. J Vet Med Educ. (2017) 44:260–7. doi: 10.3138/jvme.0816-123R
11. Kessler, RC, McLaughlin, KA, Green, JG, Gruber, MJ, Sampson, NA, Zaslavsky, AM, et al. Childhood adversities and adult psychopathology in the WHO world mental health surveys. Br J Psychiatry. (2010) 197:378–85. doi: 10.1192/bjp.bp.110.080499
12. Miller, AB, Esposito-Smythers, C, Weismoore, JT, and Renshaw, KD. The relation between child maltreatment and adolescent suicidal behavior: a systematic review and critical examination of the literature. Clin Child Fam Psychol Rev. (2013) 16:146–72. doi: 10.1007/s10567-013-0131-5
13. McLaughlin, KA, Sheridan, MA, and Lambert, HK. Childhood adversity and neural development: deprivation and threat as distinct dimensions of early experience. Neurosci Biobehav Rev. (2014) 47:578–91. doi: 10.1016/j.neubiorev.2014.10.012
14. Hackman, DA, and Farah, MJ. Socioeconomic status and the developing brain. Trends Cogn Sci. (2009) 13:65–73. doi: 10.1016/j.tics.2008.11.003
15. Aas, M, Dazzan, P, Fisher, HL, Morgan, C, Morgan, K, Reichenberg, A, et al. Childhood trauma and cognitive function in first-episode affective and non-affective psychosis. Schizophr Res. (2011) 129:12–9. doi: 10.1016/j.schres.2011.03.017
16. van Nierop, M, Viechtbauer, W, Gunther, N, van Zelst, C, de Graaf, R, Ten Have, M, et al. Childhood trauma is associated with a specific admixture of affective, anxiety, and psychosis symptoms cutting across traditional diagnostic boundaries. Psychol Med. (2015) 45:1277–88. doi: 10.1017/S0033291714002372
17. Pruessner, M, Cullen, AE, Aas, M, and Walker, EF. The neural diathesis-stress model of schizophrenia revisited: an update on recent findings considering illness stage and neurobiological and methodological complexities. Neurosci Biobehav Rev. (2017) 73:191–218. doi: 10.1016/j.neubiorev.2016.12.013
18. Dykshoorn, KL. Trauma-related obsessive-compulsive disorder: a review. Health Psychol Behav Med. (2014) 2:517–28. doi: 10.1080/21642850.2014.905207
19. Guo, J, Fu, M, Liu, D, Zhang, B, Wang, X, and van IJzendoorn, MH. Is the psychological impact of exposure to COVID-19 stronger in adolescents with pre-pandemic maltreatment experiences? A survey of rural Chinese adolescents. Child Abuse Negl. (2020) 110:104667. doi: 10.1016/j.chiabu.2020.104667
20. De Rubeis, V, Gonzalez, A, de Groh, M, Jiang, Y, Erbas Oz, U, Tarride, JE, et al. Obesity and adverse childhood experiences in relation to stress during the COVID-19 pandemic: an analysis of the Canadian longitudinal study on aging. Int J Obes. (2023) 47:197–206. doi: 10.1038/s41366-023-01258-9
21. Doom, JR, Seok, D, Narayan, AJ, and Fox, KR. Adverse and benevolent childhood experiences predict mental health during the COVID-19 pandemic. Advers Resil Sci. (2021) 2:193–204. doi: 10.1007/s42844-021-00038-6
22. Kim, AW, Nyengerai, T, and Mendenhall, E. Evaluating the mental health impacts of the COVID-19 pandemic: perceived risk of COVID-19 infection and childhood trauma predict adult depressive symptoms in urban South Africa. Psychol Med. (2022) 52:1587–99. doi: 10.1017/S0033291720003414
23. Jung, SJ, and Jun, JY. Mental health and psychological intervention amid COVID-19 outbreak: perspectives from South Korea. Yonsei Med J. (2020) 61:271–2. doi: 10.3349/ymj.2020.61.4.271
24. Borsboom, D, and Cramer, AO. Network analysis: an integrative approach to the structure of psychopathology. Annu Rev Clin Psychol. (2013) 9:91–121. doi: 10.1146/annurev-clinpsy-050212-185608
25. Fried, EI, van Borkulo, CD, Cramer, AO, Boschloo, L, Schoevers, RA, and Borsboom, D. Mental disorders as networks of problems: a review of recent insights. Soc Psychiatry Psychiatr Epidemiol. (2017) 52:1–10. doi: 10.1007/s00127-016-1319-z
26. Robinaugh, DJ, Hoekstra, RHA, Toner, ER, and Borsboom, D. The network approach to psychopathology: a review of the literature 2008–2018 and an agenda for future research. Psychol Med. (2020) 50:353–66. doi: 10.1017/S0033291719003404
27. Borsboom, D. A network theory of mental disorders. World Psychiatry. (2017) 16:5–13. doi: 10.1002/wps.20375
28. McNally, RJ. Network analysis of psychopathology: controversies and challenges. Annu Rev Clin Psychol. (2021) 17:31–53. doi: 10.1146/annurev-clinpsy-081219-092850
29. Feng, TW, Ren, L, Liu, C, Li, KL, Wu, L, Wei, XY, et al. The relations between different components of intolerance of uncertainty and symptoms of depression during the COVID-19 pandemic: a network analysis. Front Psych. (2022) 13:993814. doi: 10.3389/fpsyt.2022.993814
30. Ge, FF, Zheng, AN, Wan, MT, Luo, G, and Zhang, J. Psychological state among the general Chinese population before and during the COVID-19 epidemic: a network analysis. Front Psych. (2021) 12:12. doi: 10.3389/fpsyt.2021.591656
31. Jiang, WY, Ren, ZH, Yu, LX, Tan, YF, and Shi, CR. A network analysis of post-traumatic stress disorder symptoms and correlates during the COVID-19 pandemic. Front Psych. (2020) 11:568037. doi: 10.3389/fpsyt.2020.568037
32. Ventura-Leon, J, Caycho-Rodriguez, T, Talledo-Sanchez, K, and Casiano-Valdivieso, K. Depression, COVID-19 anxiety, subjective well-being, and academic performance in university students with COVID-19-infected relatives: a network analysis. Front Psychol. (2022) 13:13. doi: 10.3389/fpsyg.2022.837606
33. Yan, YJ, Hu, HX, Wang, LL, Zhang, YJ, Lui, SSY, Huang, J, et al. Negative schizotypal traits predict the reduction of reward motivation in effort-reward imbalance. Eur Arch Psychiatry Clin Neurosci. (2022) 273:439–45. doi: 10.1007/s00406-022-01419-3
34. Wang, K, Yu, XY, Yu, CR, Liu, YF, Chu, MY, Zhang, RT, et al. Validation of the Chinese version of the body image concern inventory. Eval Health Prof. (2022) 45:204–14. doi: 10.1177/0163278720979651
35. He, J, Zhong, X, Gao, Y, Xiong, G, and Yao, S. Psychometric properties of the Chinese version of the childhood trauma questionnaire-short form (CTQ-SF) among undergraduates and depressive patients. Child Abuse Negl. (2019) 91:102–8. doi: 10.1016/j.chiabu.2019.03.009
36. Bernstein, DP, Stein, JA, Newcomb, MD, Walker, E, Pogge, D, Ahluvalia, T, et al. Development and validation of a brief screening version of the childhood trauma questionnaire. Child Abuse Negl. (2003) 27:169–90. doi: 10.1016/S0145-2134(02)00541-0
37. Roy, A, Gorodetsky, E, Yuan, Q, Goldman, D, and Enoch, MA. Interaction of FKBP5, a stress-related gene, with childhood trauma increases the risk for attempting suicide. Neuropsychopharmacology. (2010) 35:1674–83. doi: 10.1038/npp.2009.236
38. Enoch, MA, Hodgkinson, CA, Yuan, Q, Shen, PH, Goldman, D, and Roy, A. The influence of GABRA2, childhood trauma, and their interaction on alcohol, heroin, and cocaine dependence. Biol Psychiatry. (2010) 67:20–7. doi: 10.1016/j.biopsych.2009.08.019
39. Roy, A, Hodgkinson, CA, Deluca, V, Goldman, D, and Enoch, MA. Two HPA axis genes, CRHBP and FKBP5, interact with childhood trauma to increase the risk for suicidal behavior. J Psychiatr Res. (2012) 46:72–9. doi: 10.1016/j.jpsychires.2011.09.009
40. Wang, W, Bian, Q, Zhao, Y, Li, X, Wang, W, Du, J, et al. Reliability and validity of the Chinese version of the patient health questionnaire (PHQ-9) in the general population. Gen Hosp Psychiatry. (2014) 36:539–44. doi: 10.1016/j.genhosppsych.2014.05.021
41. Kroenke, K, Spitzer, RL, and Williams, JB. The PHQ-9: validity of a brief depression severity measure. J Gen Intern Med. (2001) 16:606–13. doi: 10.1046/j.1525-1497.2001.016009606.x
42. He, X, Li, C, Qian, J, Cui, H, and Wu, W. Reliability and validity of a generalized anxiety disorder scale in general hospital outpatients. Shanghai Arch Psychiatry. (2010) 22:200–3.
43. Spitzer, RL, Kroenke, K, Williams, JB, and Lowe, B. A brief measure for assessing generalized anxiety disorder: the GAD-7. Arch Intern Med. (2006) 166:1092–7. doi: 10.1001/archinte.166.10.1092
44. Yang, X, Yang, H, Liu, Q, and Yang, L. The research on the reliability and validity of PCL-C and influence factors. Chin J Health Psychol. (2007) 15:6–9.
45. Weathers, FW, Litz, BT, Herman, DS, Huska, JA, and Keane, TM. (eds.). The PTSD checklist (PCL): reliability, validity, and diagnostic utility. In annual convention of the international society for traumatic stress studies. Vol. 462. San Antonio, TX (1993).
46. Chi, X, Chen, S, Chen, Y, Chen, D, Yu, Q, Guo, T, et al. Psychometric evaluation of the fear of COVID-19 scale among Chinese population. Int J Ment Health Addict. (2022) 20:1273–88. doi: 10.1007/s11469-020-00441-7
47. Ahorsu, DK, Lin, CY, Imani, V, Saffari, M, Griffiths, MD, and Pakpour, AH. The fear of COVID-19 scale: development and initial validation. Int J Ment Health Addict. (2022) 20:1537–45. doi: 10.1007/s11469-020-00270-8
48. Epskamp, S, Borsboom, D, and Fried, EI. Estimating psychological networks and their accuracy: a tutorial paper. Behav Res Methods. (2018) 50:195–212. doi: 10.3758/s13428-017-0862-1
49. Epskamp, S, Cramer, AO, Waldorp, LJ, Schmittmann, VD, and Borsboom, D. Qgraph: network visualizations of relationships in psychometric data. J Stat Softw. (2012) 48:1–18. doi: 10.18637/jss.v048.i04
50. Jones, PJ. Networktools: tools for identifying important nodes in networks. R package version (2018) 1:10–1155.
51. Foygel, R, and Drton, M. Extended Bayesian information criteria for Gaussian graphical models. Adv Neural Inf Proces Syst. (2010) 23:604–12.
52. Chen, J, and Chen, Z. Extended Bayesian information criteria for model selection with large model spaces. Biometrika. (2008) 95:759–71. doi: 10.1093/biomet/asn034
53. Armstrong, RA. When to use the Bonferroni correction. Ophthalmic Physiol Opt. (2014) 34:502–8. doi: 10.1111/opo.12131
54. Robinaugh, DJ, Millner, AJ, and McNally, RJ. Identifying highly influential nodes in the complicated grief network. J Abnorm Psychol. (2016) 125:747–57. doi: 10.1037/abn0000181
55. Jones, PJ, Ma, R, and McNally, RJ. Bridge centrality: a network approach to understanding comorbidity. Multivariate Behav Res. (2021) 56:353–67. doi: 10.1080/00273171.2019.1614898
56. Wickham, H., Chang, W, and Wickham, MH. (2016). Package ‘ggplot2’. Create elegant data visualisations using the grammar of graphics. Version. 2:1–189.
57. van Borkulo, CD, van Bork, R, Boschloo, L, Kossakowski, JJ, Tio, P, Schoevers, RA, et al. Comparing network structures on three aspects: a permutation test. Psychol Methods. (2022). doi: 10.1037/met0000476
58. Zhu, Z, Li, P, and Hao, L. Correlation of childhood psychological abuse and neglect with mental health in Chinese college students during the COVID-19 pandemic. Front Psych. (2021) 12:770201. doi: 10.3389/fpsyt.2021.770201
59. Zhao, YJ, Zhang, C, Guo, T, Sha, S, Su, Z, Cheung, T, et al. Associations between post-traumatic stress symptoms and quality of life among psychiatric healthcare personnel in China during the COVID-19 pandemic: a network approach. Front Psych. (2023) 14:975443. doi: 10.3389/fpsyt.2023.975443
60. Asmundson, GJ, and Katz, J. Understanding the co-occurrence of anxiety disorders and chronic pain: state-of-the-art. Depress Anxiety. (2009) 26:888–901. doi: 10.1002/da.20600
61. Janiri, D, Moccia, L, Dattoli, L, Pepe, M, Molinaro, M, De Martin, V, et al. Emotional dysregulation mediates the impact of childhood trauma on psychological distress: first Italian data during the early phase of COVID-19 outbreak. Aust N Z J Psychiatry. (2021) 55:1071–8. doi: 10.1177/0004867421998802
62. Fava, GA, Cosci, F, and Sonino, N. Current psychosomatic practice. Psychother Psychosom. (2017) 86:13–30. doi: 10.1159/000448856
63. Zavlis, O, Butter, S, Bennett, K, Hartman, TK, Hyland, P, Mason, L, et al. How does the COVID-19 pandemic impact on population mental health? A network analysis of COVID influences on depression, anxiety and traumatic stress in the UK population. Psychol Med. (2021) 52:3825–33. doi: 10.1017/S0033291721000635
64. Ventura-Leon, J, Lopez-Jurado, R, Porturas, E, Leon-Mostacero, I, and Canchanya-Balbin, SE. Anxiety, depression, stress, worry about COVID-19 and fear of loneliness during COVID-19 lockdown in Peru: a network analysis approach. Front Public Health. (2022) 10:946697. doi: 10.3389/fpubh.2022.946697
65. Cha, EJ, Jeon, HJ, and Chung, S. Central symptoms of insomnia in relation to depression and COVID-19 anxiety in general population: a network analysis. J Clin Med. (2022) 11:3416. doi: 10.3390/jcm11123416
66. Jin, Y, Sha, S, Tian, T, Wang, Q, Liang, S, Wang, Z, et al. Network analysis of comorbid depression and anxiety and their associations with quality of life among clinicians in public hospitals during the late stage of the COVID-19 pandemic in China. J Affect Disord. (2022) 314:193–200. doi: 10.1016/j.jad.2022.06.051
67. Kwon, A, Lee, HS, and Lee, SH. The mediation effect of hyperarousal symptoms on the relationship between childhood physical abuse and suicidal ideation of patients with PTSD. Front Psych. (2021) 12:613735. doi: 10.3389/fpsyt.2021.613735
68. Tsur, N, Shahar, G, Defrin, R, Lahav, Y, and Ginzburg, K. Torturing personification of chronic pain among torture survivors. J Psychosom Res. (2017) 99:155–61. doi: 10.1016/j.jpsychores.2017.06.016
69. Yehuda, R, Halligan, SL, and Grossman, R. Childhood trauma and risk for PTSD: relationship to intergenerational effects of trauma, parental PTSD, and cortisol excretion. Dev Psychopathol. (2001) 13:733–53. doi: 10.1017/S0954579401003170
Keywords: COVID-19, childhood trauma, mental health, pandemic, network analysis
Citation: Xia J, Zhu L, Huang H, Fan P, Zhou M, Cai X-l and He H (2023) Relationships between childhood trauma and mental health during the COVID-19 pandemic: a network analysis. Front. Psychiatry. 14:1251473. doi: 10.3389/fpsyt.2023.1251473
Edited by:
Juehua Yu, The First Affiliated Hospital of Kunming Medical University, ChinaReviewed by:
Kui Wang, Institute of Psychology Chinese Academy of Sciences, ChinaYing Lin, Illumina, United States
Copyright © 2023 Xia, Zhu, Huang, Fan, Zhou, Cai and He. This is an open-access article distributed under the terms of the Creative Commons Attribution License (CC BY). The use, distribution or reproduction in other forums is permitted, provided the original author(s) and the copyright owner(s) are credited and that the original publication in this journal is cited, in accordance with accepted academic practice. No use, distribution or reproduction is permitted which does not comply with these terms.
*Correspondence: Xin-lu Cai, Y2FpeGlubHVAaHpudS5lZHUuY24=; Hui He, empoZWh1aTEyMzRAMTYzLmNvbQ==
†These authors have contributed equally to this work