- 1Department of Psychiatry, Niigata University Graduate School of Medical and Dental Sciences, Niigata, Japan
- 2Department of Psychiatry, Faculty of Medicine, Mulawarman University, Samarinda, Indonesia
- 3Department of Psychiatry, Uonuma Kikan Hospital, Niigata, Japan
- 4Department of Obstetrics and Gynecology, Niigata University Graduate School of Medical and Dental Sciences, Niigata, Japan
Background: There is a rising interest in perinatal mental health studies, and proper psychometric tools to assess autistic traits among this population in Japan are vital.
Objective: This study aimed to clarify the optimal factor structure of the AQ as part of a perinatal mental health research project.
Methods: We used the Japanese version of the AQ (AQ-J) to measure autistic-like traits in pregnant women. Participants were 4,287 Japanese women who were pregnant or who had given birth within the last month. We performed exploratory factor analysis (EFA) using the first sample group (n = 2,154) to obtain factor structures for the final item selections. We performed confirmatory factor analysis (CFA) using the second sample group (n = 2,133) to obtain a model with good fit, then compared the model to all previously proposed models to determine the best-fitting model.
Results: The EFA analysis identified a model consisting of 25 items distributed across three factors. Cronbach’s alpha for the total 25-item AQ-J, 9-item “Social interaction” factor, 11-item “Non-verbal communication” factor, and 5-item “Restricted interest” factor was 0.829, 0.829, 0.755, and 0.576, respectively. McDonald’s omega and its 95% confidence interval were 0.826 (0.821–0.836), 0.835 (0.821–0.837), 0.755 (0.744–0.766), and 0.603 (0.556–0.596), respectively. CFA confirmed that the three-factor structure had an acceptable fit (goodness of fit index: 0.900, comparative fit index: 0.860, root mean square error of approximation: 0.066). These findings indicated that the three-factor model was better than the 13 existing models.
Conclusion: The findings are discussed in relation to the adequacy of the AQ-J for assessing autistic traits in perinatal women. We recommend the use of this 25-item, three-factor AQ-J model for this population owing to its superiority to all previous models.
1. Introduction
The Autism-Spectrum Quotient (AQ) (1) is a widely used instrument that identifies adult individuals with normal intelligence who may have autistic-like traits. The AQ has frequently been used as a screening instrument for autism spectrum conditions (ASC) or broader phenotypes in the general population (2), as a tool in autism research to explore other, non-clinical traits and behaviors associated with autism spectrum disorder (ASD) (1, 3), and in clinical practice to differentiate between individuals with and without Asperger syndrome (4). Autism is currently considered a state on the continuum from an ASD diagnosis, to ASC, then to normality, and the extent of autistic traits can be quantitatively measured for scientific research into autism etiopathogeneses and for clinical practice to establish early diagnosis and intervention.
Several studies have reported the experiences of mothers with autism during the perinatal period and parenthood (5, 6). One study showed that mothers with autism were more likely to have antepartum and postpartum depression than controls (5). They also tend to experience difficulties and dissatisfaction communicating with healthcare providers during perinatal care and higher rates of difficulty breastfeeding (5). In addition, mothers with autism are more likely to experience higher rates of pregnancy complications, including preterm birth, cesarean and induced delivery, and pre-eclampsia (7). During motherhood, mothers with autism report more parenting difficulties, including lower parenting competence and satisfaction/self-esteem (8), and are more likely to feel isolated and express the desire for increased parenting support (5). Therefore, the AQ could be useful for screening and identifying autistic traits in pregnant women to ensure the provision of more tailored mental health care and support of mothers and children during the perinatal period.
Although the AQ is a widely used measure, its reliability has been questioned (9). After its first publication, several researchers proposed factor structures and models for the AQ (2, 10–20). However, the findings were inconsistent. The proposed models ranged from two- to six-factor structures and the item loadings varied across studies. When the AQ was first developed, a five-factor structure for the measure was proposed that included the factors of social skills, attention switching, attention to detail, communication, and imagination (1). However, this five-factor structure was theoretical rather than empirical, and several studies demonstrated that it had poor fit (2, 10, 11). Some studies generated factor structures using data-driven approaches and a few used theory-driven approaches. Most studies on the structure of the AQ have used statistical analyses featuring only principal component analysis or exploratory factor analysis (EFA), which cannot determine whether the proposed model is a good fit. In addition, the proposed factor structures depend on the extent to which the AQ is used to evaluate the phenomenology related to the autism spectrum, which may or may not be limited to the autism domain.
Given the importance of autism screening in pregnant women to ensure perinatal health and support healthy motherhood, this study aimed to clarify the optimal factor structure of the AQ as part of a perinatal mental health research project. To the best of our knowledge, no studies have examined the factor structure of the AQ for assessing pregnant women. Using a data-driven approach, we aimed to generate factor structures that specifically identified the autistic traits included in the ASD criteria according to the Diagnostic and Statistical Manual of Mental Disorders (DSM), so that this can be used in future research and clinical practice. We included a large sample of perinatal women and used EFA to obtain factor structures of the final item selections and confirmatory factor analysis (CFA) to obtain a model with good fit. In addition, we compared our factor structure with the AQ factor structures found in 13 previous studies to identify the best-fitting model.
2. Materials and methods
2.1. Participants
This study was part of the Perinatal Mental Health Research Project conducted between March 2017 and March 2021 (21–28). Participants of the present study were 4,287 pregnant Japanese women aged 18 years or older from 34 associated obstetric institutions in Niigata prefecture, Japan. We distributed a large-scale questionnaire to obtain AQ data at the time of project enrollment. We included participants who had returned and completely filled in the AQ questionnaire as part of the mental health project. The AQ data were the same as those used in our previous study (28). We excluded participants with serious physical complications, serious pregnancy complications, and ongoing treatment for psychiatric disorders (e.g., ASD, schizophrenia, depression, bipolar disorder, anxiety disorder, or personality disorder). The present study focused on autistic traits rather than ASD. Although it is possible that women with undiagnosed ASD were inadvertently included in this study, the estimated prevalence of ASD is approximately 1%, and the male-to-female ratio is 3:1 (29). Thus, we assumed that the obstetric sample in the present study was comparable to the general population of women, but not to the general population of men and women.
2.2. Measures
The AQ (1) is a self-administered instrument that assesses autistic traits in adults with normal intelligence. It comprises a 50-item questionnaire. Each item comprises a short statement. The AQ consists of five subscales of 10 statements each: social skills, attention switching, attention to detail, communication, and imagination. Items are rated by participants on a four-point scale: 1 (“definitely agree”), 2 (“slightly agree”), 3 (“slightly disagree”), and 4 (“definitely disagree”). In the original AQ scoring, Baron-Cohen et al. (1) used a 0/1 binary scale in which, for some items, responses of 1 and 2 are scored as 1; for other items, responses of 3 and 4 are scored as 1. The total possible score range is 0–50. However, in this study, we used the four-point scale as we anticipated that this would provide more information and would yield more valid EFA results. The Japanese version of the AQ (AQ-J) has been validated in a previous study (4).
We collected data on obstetric factors, including gestational age (trimester when the participants responded to the AQ), parity (primipara or multipara), type of conception (natural conception or others), and pregnancy (single or multiple).
2.3. Statistical analyses
We randomly divided participants with AQ-J data into two groups. Using the first group (n = 2,154), we performed an EFA with Promax rotation, obtaining the number of factors from a parallel analysis. The parallel analysis indicated that eight factors or fewer were appropriate. Therefore, EFAs were performed, in which the number of factors was specified as eight or less, respectively. The maximum likelihood method and Promax rotation were used for each EFA. Item retention/deletion decisions were made using the following criteria: (a) items with a factor loading >0.40 were retained; (b) items were not retained if they had dual-factor loadings (defined as loadings >0.40 on two or more factors or differences between the loadings on the first two primary factors of <0.20). As a result, factors 4, 5, 6, 7, and 8 were not retained because fewer than three items loaded on them. We reported both Cronbach’s alpha (α) and McDonald’s omega (ω) and its 95% confidence interval (CI) for the whole scale and each subscale to examine internal consistency reliability.
We used the second group (n = 2,133) to perform a CFA using the optimal factor structure as extracted from the EFA. CFAs were performed with two-, three-, and four-factor structures, respectively. The three-factor structure showed the best fit. We used the goodness of fit index (GFI), the comparative fit index (CFI), and the root mean square error of approximation (RMSEA) to identify an acceptable fit (GFI ≥0.90, CFI ≥0.90, and RMSEA ≤0.08) (30) between the models and the data. All statistical analyses were performed using SPSS versions 25 and 29 (IBM Corp., Armonk, NY, United States), and Amos 25.0.0 (IBM Japan, Tokyo, Japan).
3. Results
3.1. Descriptive statistics
We included all data from 4,287 pregnant women who completed the AQ-J questionnaire and had no missing values. We also included questionnaire data from women who had given birth within the last month. Table 1 shows the descriptive statistics of the participants.
3.2. Exploratory factor analyses
We performed EFA using data from the first group (n = 2,154). Although the parallel analysis indicated that eight factors or fewer were appropriate, the EFA and Promax rotation results indicated that three factors should be retained according to the item retention/deletion criteria. Table 2 shows the EFA results for the AQ-J data. Using the criteria described in the Methods section, we excluded 21 items (1, 2, 4, 7, 15, 18, 21, 24, 25, 26, 28, 29, 30, 33, 34, 35, 40, 41, 43, 49, 50) with factor loadings <0.4, excluded 4 items (5, 12, 20, 23) with dual-factor loadings, and retained 25 items (3, 6, 8, 9, 10, 11, 13, 14, 16, 17, 19, 22, 27, 31, 32, 36, 37, 38, 39, 42, 44, 45, 46, 47, 48) with factor loadings >0.4 without dual-factor loadings. For the 25-item AQ-J, α = 0.829 and ω = 0.826 (95% CI = 0.821–0.836). Factor 1 (“Social interaction”) comprised nine items (10, 13, 17, 22, 38, 44, 46, 47, 48), with α = 0.829 and ω = 0.835 (95% CI = 0.821–0.837). Factor 2 (“Non-verbal communication”) comprised eleven items (3, 8, 11, 14, 27, 31, 32, 36, 37, 42, 45), with α = 0.755 and ω = 0.755 (95% CI = 0.744–0.766). Factor 3 (“Restricted interest”) comprised five items (6, 9, 16, 19, 39), with α = 0.576 and ω = 0.603 (95% CI = 0.556–0.596). Table 3 shows the three-factor structure and the items.
3.3. Confirmatory factor analyses
We performed CFA using data from the second group (n = 2,133). Figure 1 shows the standardized coefficients indicating the association between each item and factor in the CFA. Of the three factors, Restricted interest negatively correlated with Social interaction (r = −0.090) and Non-verbal communication (r = −0.152). Social interaction positively correlated with Non-verbal communication (r = 0.622). The three-factor structure was confirmed to have an acceptable fit for the AQ-J data according to the GFI (0.900) and RMSEA (0.066), but not according to the CFI (0.860).
3.4. Comparison with other models
We performed CFA to compare the factor models of previous studies with our second set of AQ-J data (Table 4). A good-fitting model found in a study by Hoekstra et al. (13) could not be compared with the models found in other studies because it was a single study that used the factors as observable variables in the model, whereas other studies used the factors as latent variables. The data from two studies (18, 20) could not be analyzed using CFA because the items overlapped between factors. Therefore, we excluded these three models from our comparison. Table 4 shows that among the remaining 11 factor models, the six-factor structure of Zhu et al. (2) and the present three-factor structure showed the best fit to the data (GFI = 0.913 and 0.900, CFI = 0.802 and 0.860, RMSEA = 0.059 and 0.066, respectively).
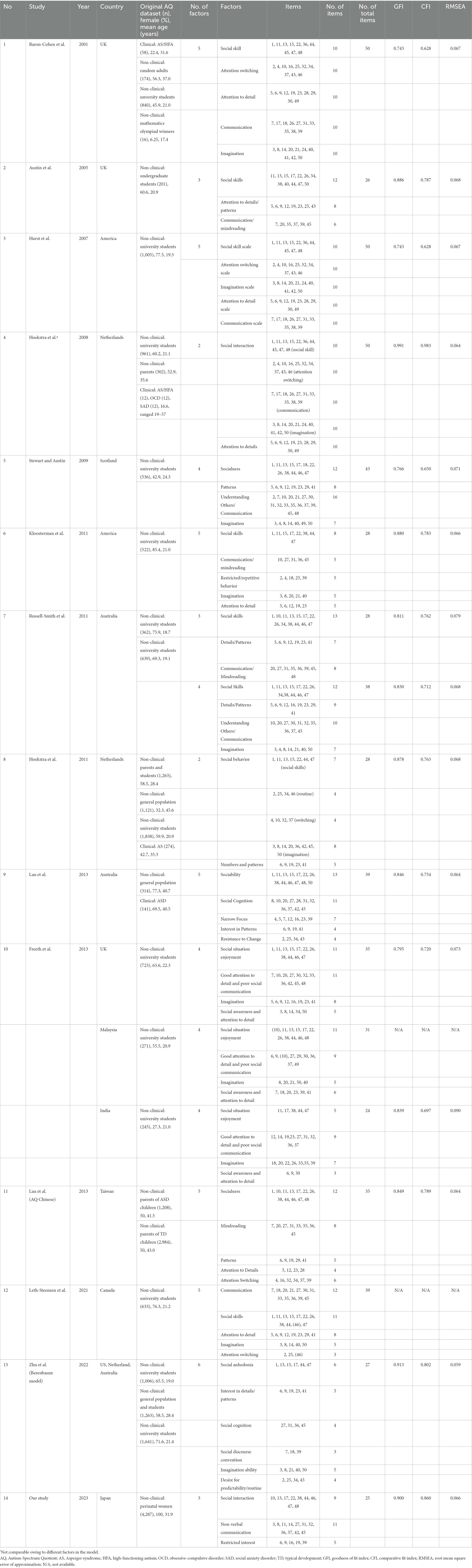
Table 4. Confirmatory factor analysis of the factor models of previous studies with the second set of AQ data (n = 2,133).
4. Discussion
The present study used a data-driven approach to generate a factor structure for the AQ-J. The findings showed that a three-factor structure is optimal and has an acceptable fit according to the statistical analyses. This three-factor structure comprises the factors of Social interaction, Non-verbal communication, and Restricted interest. These three factors are included in the two primary characteristics of ASD in the DSM-5 diagnostic criteria. Our Factor 1 and Factor 2 are included in domain A of the DSM-5 (persistent deficits in social communication and social interaction, including non-verbal communicative behaviors), and Factor 3 is included in DSM-5 domain B (restricted, repetitive patterns of behavior, interests, or activities) (31). These findings are consistent with those of a previous study, which proposed that a three-factor model comprising “Social skill,” “Patterns/details,” and “Communication/mindreading” subscales was the best way to measure specific types of autistic traits using the AQ (9).
The comparison with previously proposed models showed that almost all previous models identified the three factors found in the present study. However, the number of factors and the composition of the items vary across studies. The validity of a measure or a factor structure likely depends on its intended purpose (2). Previous studies have had different goals in generating proposed factor structures, including assessing the relationship of autistic traits to personality (10), assessing the relationship of autistic traits to schizotypy traits (15), and identifying a range of psychological constructs that may be relevant not only to ASC but to a wide variety of clinical phenomena related to schizophrenia spectrum and anxiety disorders (2). The three-factor structure identified in the present study will elucidate the expression of autistic traits by the general population of pregnant Japanese women.
Previous studies suggest that the dimensions of our three-factor structure align with the mapping of several dimensions found in previous studies of Western populations. Our Factor 1 (Social interaction) is mostly related to Sociability (17) and Social skills (1, 10). Our Factor 2 (Non-verbal communication) is mostly related to Social cognition (2, 17) and Communication/mindreading (10–12, 15), representing the difficulties experienced by people with ASD traits with theory of mind, or the ability to understand the beliefs, desires, and intentions of others (32, 33). Finally, our Factor 3 (Restricted interest) is mostly related to Attention to detail (1, 10, 12, 15) and Patterns (2, 14, 16, 17).
Using our AQ-J data, we performed CFA to compare the factor models of 13 previous studies with our own findings. None of the models, including our own, showed a good fit, despite the fact that we had a large sample of perinatal women and analyzed a substantial amount of AQ-J data. However, our three-factor model is comparable or slightly superior to a previous six-factor model (2) and is better than other previous models. Moreover, the Cronbach’s alpha and McDonald’s omega for the 25-item, three-factor AQ-J solution obtained in this study indicated good internal consistency and reliability. Therefore, we suggest the use of this 25-item, three-factor alternative AQ-J to assess autistic traits in perinatal women owing to its superiority to all previous models. However, our model was generated using data from a large sample of perinatal women aged 27–37 years; thus, there may be various discrepancies between our findings and those of previous studies with different samples. A previous study of the general population showed that men had substantially higher AQ scores than women, but that age had no substantial effect on AQ scores (13, 16). Moreover, clinical samples with ASD diagnosis show considerably higher scores than non-clinical samples (1, 13, 16, 17). Therefore, our findings may reflect the effect of sex and the use of a non-clinical sample. Furthermore, a study by Power et al. (34) demonstrated that individuals with ASD showed a greater reduction in fecundity because few ever married or had children compared with individuals in the general population. Specifically, men with ASD had a lower fertility rate than women with ASD. This sex-specific effect may be because ASD morbidity impairs the ability to find suitable sexual partners or inhibits biological fertility to a greater extent in men. In addition, male siblings of individuals with ASD had fewer children, whereas female siblings of individuals with ASD showed no substantial difference from the general population. This pattern may reflect sexually antagonistic genes or undiagnosed symptoms in male siblings of individuals with ASD. Considering these previous findings (34), and the fact that our sample consisted of only perinatal women, the variance of non-autistic traits in our data may be greater than the variance of autistic traits in the normal population, which includes both sexes regardless of marital status.
In the present study, the total scores on the 25-item AQ-J for our participants ranged from 0 to 25. However, we did not determine a cutoff point owing to the unavailability of case data. A previous study indicated that the cutoff point for AQ-J scores is >33 (out of 50 items) for Asperger syndrome or high-functioning autism, but the cutoff point would likely be higher for individuals diagnosed with autistic disorder (4). Therefore, additional studies that include clinical groups are needed to determine a cutoff for this 25-item AQ-J, which could be used for screening autistic traits in a non-clinical population of perinatal women. For our data, the score ranges for Social interaction, Non-verbal communication, and Restricted interest were 0–9 (out of 9), 0–11 (out of 11), and 0–5 (out of 5), respectively. There is a growing recognition that autism may be a heterogeneous condition with various clinical presentations and subtypes (9, 35). Regarding this, our findings also showed some negative interfactor correlations, as found in a previous study (9). We found that the Restricted interest factor negatively correlated with the Social interaction and Non-verbal communication factors. This suggests that the Restricted interest factor may not directly contribute positively to the AQ-J accumulated total score. Individuals may have a high score on one of the factors and a low score on the other two factors. Thus, the AQ score obtained would be classified as moderate or not exceeding the cutoff point even though it actually masks a specific autistic trait. In clinical practice, all symptoms may positively indicate autism. A possible problem in autism research, however, is the confirmation of autism using statistical analyses of AQ scores, as the presence of autistic traits may be apparent in some factor scores but hardly noticeable in scores on other factors, and vice versa. Thus, a global interpretation of the total AQ score requires a detailed interpretation of each accumulated factor score to obtain a general account of the presence of autistic traits. The total 25-item AQ-J solution, the Social interaction factor, and the Non-verbal communication factor showed good internal consistency. However, the Restricted interest factor showed poor internal consistency. Therefore, the use of the total AQ-J score and scores on each factor are recommended in research. Moreover, additional studies are needed to identify possible distinct autism subtypes that may clarify why analysis of AQ scores suggests that some autistic traits appear to cancel out other autistic traits within the same factor model. In clinical practice, all autistic traits are assumed to comprise a combination of symptoms that contribute positively to each other in characterizing autism.
The present study has several limitations that require further discussion. First, a problem with the model comparisons was the existence of differences in the set of retained items; moreover, the scoring method on some of the previous instruments (2) used a Likert scale that was different from the original binary scoring system (1) that we used in this study. Second, we did not conduct structured diagnostic interviews to confirm ASD diagnosis. Additional studies should be performed to validate the 25-item solution of AQ-J as a screening instrument for autistic traits in perinatal women. The present results showed that a three-factor structure comprising 25 items was the optimal model. However, given that this study was conducted on perinatal women, it is necessary to evaluate the suitability of the model in other populations (unmarried women, men in the general population, and ASD patients) and to confirm whether 25 items are more useful for screening than 50 items.
5. Conclusion
The present findings suggest that our proposed 25-item, three-factor structure of the AQ-J has an acceptable fit and is superior to all other previous models for use with perinatal women. Therefore, it may be the most suitable model to use for perinatal mental health studies of adult populations. Furthermore, we recommend the use of the 25-item AQ-J total score and the scores on each factor in future research.
Data availability statement
The datasets presented in this article are not readily available because all relevant data are provided in the paper. We are not able to make the underlying data available to readers, because we do not have permission from the participating institutions to do so. Requests to access the datasets should be directed to TS, cHN5QG1lZC5uaWlnYXRhLXUuYWMuanA=.
Ethics statement
The studies involving humans were approved by the ethics committee of Niigata University (approval number: 2016–0019) and the ethics committees of the participating obstetric institutions. The studies were conducted in accordance with the local legislation and institutional requirements. The participants provided their written informed consent to participate in this study.
Author contributions
EZ: Conceptualization, Methodology, Formal analysis, Visualization, Writing – original draft. NF: Conceptualization, Data curation, Formal analysis, Funding acquisition, Investigation, Methodology, Writing – original draft. YW: Conceptualization, Methodology, Writing – original draft. KH: Data curation, Writing – review & editing. TM: Data curation, Funding acquisition, Investigation, Writing – review & editing. MO: Data curation, Funding acquisition, Writing – review & editing. JE: Conceptualization, Methodology, Writing – review & editing. KN: Conceptualization, Methodology, Writing – review & editing. TS: Conceptualization, Methodology, Supervision, Writing – review & editing.
Funding
The author(s) declare financial support was received for the research, authorship, and/or publication of this article. This work was supported by JSPS KAKENHI (grant number JP19K08040 to NF), a grant from the Niigata Medical Association (to TM), a grant from the Medical Association of Niigata City (to MO), and a contract research grant (156195-J15F0001) from the Niigata Prefectural Hospital Bureau to the Department of Community Psychiatric Medicine, Niigata University Graduate School of Medical and Dental Sciences.
Acknowledgments
We are grateful to Takuro Sugai, Ryusuke Tsuboya, Koichi Takakuwa, Masayuki Yamaguchi, Kazufumi Haino, Rie Araki, and Setsuko Mitome for their collaboration during the early stages of this work, and to Atsunori Sugimoto and Kiyohiro Yoshinaga for providing financial support for the publication of the manuscript. We greatly appreciate the help of all participants and staff at the following participating obstetric institutions: Kameda Daiichi Hospital, Niitsu Obstetrics and Gynecology Clinic, Tomita Obstetrics and Gynecology Clinic, Honda Ladies Clinic, Agano City Hospital, Chihara Clinic, Sekizuka Clinic, Hirohashi Obstetrics and Gynecology Clinic, Saiseikai Sanjo Hospital, Saiseikai Niigata Hospital, Itoigawa Sogo Hospital, Niigata Tokamachi Hospital, Takeyama Hospital, Watanabe Kinen Clinic, Kashiwazaki General Hospital and Medical Center, Kido Hospital, Tachikawa Medical Center, Saintpoulia Women’s Clinic, Nagaoka Chuo General Hospital, Arakawa Ladies Clinic, Ueda Ladies Clinic, Uonuma Kikan Hospital, Murakami General Hospital, Sado General Hospital, Tokunaga Ladies Clinic, Nagaoka Red Cross Hospital, Niigata Shibata Hospital, Ladies Clinic Ishiguro, Angel Mother Clinic, Joetsu General Hospital, Niigata City General Hospital, Ojiya General Hospital, Watanabe Clinic, and Niigata University Medical and Dental Hospital. We thank Atsunori Sugimoto for providing guidance about the AQ-J. We thank Diane Williams, from Edanz (https://jp.edanz.com/ac) for editing a draft of this manuscript.
Conflict of interest
The authors declare that the research was conducted in the absence of any commercial or financial relationships that could be construed as a potential conflict of interest.
Publisher’s note
All claims expressed in this article are solely those of the authors and do not necessarily represent those of their affiliated organizations, or those of the publisher, the editors and the reviewers. Any product that may be evaluated in this article, or claim that may be made by its manufacturer, is not guaranteed or endorsed by the publisher.
References
1. Baron-Cohen, S, Wheelwright, S, Skinner, R, Martin, J, and Clubley, E. The autism-spectrum quotient (AQ): evidence from Asperger syndrome/high-functioning autism, males and females, scientists and mathematicians. J Autism Dev Disord. (2001) 31:5–17. doi: 10.1023/a:1005653411471
2. Zhu, Y, Mu, W, Chirica, MG, and Berenbaum, H. Testing a theory-driven factor structure of the autism-spectrum quotient. Autism Res. (2022) 15:1710–8. doi: 10.1002/aur.2763
3. Constantino, JN, and Todd, RD. Autistic traits in the general population: a twin study. Arch Gen Psychiatry. (2003) 60:524–30. doi: 10.1001/archpsyc.60.5.524
4. Wakabayashi, A, Baron-Cohen, S, Wheelwright, S, and Tojo, Y. The autism-Spectrum quotient (AQ) in Japan: a cross-cultural comparison. J Autism Dev Disord. (2006) 36:263–70. doi: 10.1007/s10803-005-0061-2
5. Pohl, AL, Crockford, SK, Blakemore, M, Allison, C, and Baron-Cohen, S. A comparative study of autistic and non-autistic women’s experience of motherhood. Mol. Autism. (2020) 11:11. doi: 10.1186/s13229-019-0304-2
6. McDonnell, CG, and Delucia, EA. Pregnancy and parenthood among autistic adults: implications for advancing maternal health and parental well-being. Autism Adulthood. (2021) 3:100–15. doi: 10.1089/aut.2020.0046
7. Sundelin, HEK, Stephansson, O, Hultman, CM, and Ludvigsson, JF. Pregnancy outcomes in women with autism: a nationwide population-based cohort study. Clin Epidemiol. (2018) 10:1817–26. doi: 10.2147/CLEP.S176910
8. Dissanayake, C, Richdale, A, Kolivas, N, and Pamment, L. An exploratory study of autism traits and parenting. J Autism Dev Disord. (2020) 50:2593–606. doi: 10.1007/s10803-019-03984-4
9. English, MCW, Gignac, GE, Visser, TAW, Whitehouse, AJO, and Maybery, MT. A comprehensive psychometric analysis of autism-spectrum quotient factor models using two large samples: model recommendations and the influence of divergent traits on total-scale scores. Autism Res. (2019) 13:45–60. doi: 10.1002/aur.2198
10. Austin, EJ. Personality correlates of the broader autism phenotype as assessed by the autism Spectrum quotient (AQ). Pers Individ Differ. (2005) 38:451–60. doi: 10.1016/j.paid.2004.04.022
11. Kloosterman, PH, Keefer, KV, Kelley, EA, Summerfeldt, LJ, and Parker, JDA. Evaluation of the factor structure of the autism-Spectrum quotient. Pers Individ Differ. (2011) 50:310–4. doi: 10.1016/j.paid.2010.10.015
12. Hurst, RM, Mitchell, JT, Kimbrel, NA, Kwapil, TK, and Nelson-Gray, RO. Examination of the reliability and factor structure of the autism Spectrum quotient (AQ) in a non-clinical sample. Pers Individ Differ. (2007) 43:1938–49. doi: 10.1016/j.paid.2007.06.012
13. Hoekstra, RA, Bartels, M, Cath, DC, and Boomsma, DI. Factor structure, reliability and criterion validity of the autism-spectrum quotient (AQ): a study in Dutch population and patient groups. J Autism Dev Disord. (2008) 38:1555–66. doi: 10.1007/s10803-008-0538-x
14. Stewart, ME, and Austin, EJ. The structure of the autism-Spectrum quotient (AQ): evidence from a student sample in Scotland. Pers Individ Differ. (2009) 47:224–8. doi: 10.1016/j.paid.2009.03.004
15. Russell-Smith, SN, Maybery, MT, and Bayliss, DM. Relationships between autistic-like and schizotypy traits: an analysis using the autism Spectrum quotient and Oxford-Liverpool inventory of feelings and experiences. Pers Individ Differ. (2011) 51:128–32. doi: 10.1016/j.paid.2011.03.027
16. Hoekstra, RA, Vinkhuyzen, AAE, Wheelwright, S, Bartels, M, Boomsma, DI, Baron-Cohen, S, et al. The construction and validation of an abridged version of the autism-spectrum quotient (AQ-short). J Autism Dev Disord. (2011) 41:589–96. doi: 10.1007/s10803-010-1073-0
17. Lau, WYP, Kelly, AB, and Peterson, CC. Further evidence on the factorial structure of the autism spectrum quotient (AQ) for adults with and without a clinical diagnosis of autism. J Autism Dev Disord. (2013) 43:2807–15. doi: 10.1007/s10803-013-1827-6
18. Freeth, M, Sheppard, E, Ramachandran, R, and Milne, E. A cross-cultural comparison of autistic traits in the UK, India and Malaysia. J Autism Dev Disord. (2013) 43:2569–83. doi: 10.1007/s10803-013-1808-9
19. Lau, WYP, Gau, SSF, Chiu, YN, Wu, YY, Chou, WJ, Liu, SK, et al. Psychometric properties of the Chinese version of the autism Spectrum quotient (AQ). Res Dev Disabil. (2013) 34:294–305. doi: 10.1016/j.ridd.2012.08.005
20. Leth-Steensen, C, Gallitto, E, Mintah, K, and Parlow, SE. Testing the latent structure of the autism Spectrum quotient in a sub-clinical sample of university students using factor mixture modelling. J Autism Dev Disord. (2021) 51:3722–32. doi: 10.1007/s10803-020-04823-7
21. Fukui, N, Motegi, T, Watanabe, Y, Hashijiri, K, Tsuboya, R, Ogawa, M, et al. Perceived parenting before adolescence and parity have direct and indirect effects via depression and anxiety on maternal–infant bonding in the perinatal period. Psychiatry Clin Neurosci. (2021) 75:312–7. doi: 10.1111/pcn.13289
22. Fukui, N, Motegi, T, Watanabe, Y, Hashijiri, K, Tsuboya, R, Ogawa, M, et al. Exclusive breastfeeding is not associated with maternal-infant bonding in early postpartum, considering depression, anxiety, and parity. Nutrients. (2021) 13:1–8. doi: 10.3390/nu13041184
23. Ogawa, M, Watanabe, Y, Motegi, T, Fukui, N, Hashijiri, K, Tsuboya, R, et al. Factor structure and measurement invariance of the hospital anxiety and depression scale across the peripartum period among pregnant Japanese women. Neuropsychiatr Dis Treat. (2021) 17:221–7. doi: 10.2147/NDT.S294918
24. Motegi, T, Watanabe, Y, Fukui, N, Ogawa, M, Hashijiri, K, Tsuboya, R, et al. Depression, anxiety and primiparity are negatively associated with mother-infant bonding in Japanese mothers. Neuropsychiatr Dis Treat. (2021) 16:3117–22. doi: 10.2147/NDT.S287036
25. Motegi, T, Fukui, N, Hashijiri, K, Tsuboya, R, Sugai, T, Egawa, J, et al. Identifying the factor structure of the mother-to-infant bonding scale for post-partum women and examining its consistency during pregnancy. Psychiatry Clin Neurosci. (2019) 73:661–2. doi: 10.1111/pcn.12920
26. Hashijiri, K, Watanabe, Y, Fukui, N, Motegi, T, Ogawa, M, Egawa, J, et al. Identification of bonding difficulties in the peripartum period using the mother-to-infant bonding scale-Japanese version and its tentative cutoff points. Neuropsychiatr Dis Treat. (2021) 17:3407–13. doi: 10.2147/NDT.S336819
27. Fukui, N, Watanabe, Y, Hashijiri, K, Motegi, T, Ogawa, M, Egawa, J, et al. Factor structure of the parental bonding instrument for pregnant Japanese women. Sci Rep. (2022) 12:19071. doi: 10.1038/s41598-022-22017-2
28. Fukui, N, Watanabe, Y, Motegi, T, Hashijiri, K, Ogawa, M, Egawa, J, et al. Relationships among autistic traits, depression, anxiety, and maternal-infant bonding in postpartum women. BMC Psychiatry. (2023) 23:463. doi: 10.1186/s12888-023-04970-y
29. Sun, X, Allison, C, Auyeung, B, Baron-Cohen, S, and Brayne, C. Parental concerns, socioeconomic status, and the risk of autism spectrum conditions in a population-based study. Res Dev Disabil. (2014) 35:3678–88. doi: 10.1016/j.ridd.2014.07.037
30. Schermelleh-Engel, K, Moosbrugger, H, and Müller, H. Evaluating the fit of structural equation models: tests of significance and descriptive goodness-of-fit measures. Methods Psychol Res. (2003) 8:23–74. doi: 10.23668/psycharchives.12784
31. American Psychiatric Association. Diagnostic and statistical manual of mental disorders. 5th Edn. American Psychiatric Association. (2013).
32. Brown, HM, and Klein, PD. Writing, asperger syndrome and theory of mind. J Autism Dev Disord. (2011) 41:1464–74. doi: 10.1007/s10803-010-1168-7
33. Peterson, CC, Wellman, HM, and Slaughter, V. The mind behind the message: advancing theory-of-mind scales for typically developing children, and those with deafness, autism, or Asperger syndrome. Child Dev. (2012) 83:469–85. doi: 10.1111/j.1467-8624.2011.01728.x
34. Power, RA, Kyaga, S, Uher, R, MacCabe, JH, Långström, N, Landen, M, et al. Fecundity of patients with schizophrenia, autism, bipolar disorder, depression, anorexia nervosa, or substance abuse vs their unaffected siblings. JAMA Psychiatry. (2013) 70:22–30. doi: 10.1001/jamapsychiatry.2013.268
Keywords: Autism-Spectrum Quotient (AQ), autism spectrum disorder, autism spectrum conditions (ASC), perinatal women, factor structure, factor analysis
Citation: Zain E, Fukui N, Watanabe Y, Hashijiri K, Motegi T, Ogawa M, Egawa J, Nishijima K and Someya T (2023) The three-factor structure of the Autism-Spectrum Quotient Japanese version in pregnant women. Front. Psychiatry. 14:1275043. doi: 10.3389/fpsyt.2023.1275043
Edited by:
Ernesto González-Mesa, University of Malaga, SpainReviewed by:
Juko Ando, Keio University, JapanShinsuke Hidese, Teikyo University, Japan
Mark Andrew Stokes, Deakin University, Australia
Copyright © 2023 Zain, Fukui, Watanabe, Hashijiri, Motegi, Ogawa, Egawa, Nishijima and Someya. This is an open-access article distributed under the terms of the Creative Commons Attribution License (CC BY). The use, distribution or reproduction in other forums is permitted, provided the original author(s) and the copyright owner(s) are credited and that the original publication in this journal is cited, in accordance with accepted academic practice. No use, distribution or reproduction is permitted which does not comply with these terms.
*Correspondence: Toshiyuki Someya, cHN5QG1lZC5uaWlnYXRhLXUuYWMuanA=