- 1School of Nursing, Shanghai Jiao Tong University, Shanghai, China
- 2Department of Urology, Renji Hospital, Shanghai Jiao Tong University, Shanghai, China
- 3Department of Sports Medicine, Huashan Hospital, Fudan University, Shanghai, China
- 4Reference Department, Library of Shanghai Jiao Tong University School of Medicine, Shanghai, China
- 5Department of Nursing, Renji Hospital, Shanghai Jiao Tong University, Shanghai, China
Objective: The aim of this article was to review existing documents in the field of mobile-based EMA and EMI, provide an overview of current hot topics, and predict future development trends.
Methods: We conducted a bibliometric study on mobile-based EMA and EMI publications that were collected from the Web of Science Core Collection database. Biblioshiny and CiteSpace were utilized to analyze scientific productions, leading sources, authors, affiliations, documents, research hot topics, keywords, and trend topics.
Results: A total of 2222 documents related to EMA and EMI published between 1992 and 2023 were retrieved. In recent years, scholarly publications have generally increased in mobile-based EMA and EMI research, particularly in the last decade. JMIR mHealth and uHealth (n=86), as well as JMIR (n=73), showed the highest number of publications. The United States (n=1038), Germany (n=218) and Netherlands (n=175) were leading countries. Regarding keyword co-occurrence and trend topics analysis, mental health, health behaviors, and feasibility were hot topics in mobile-based EMA and EMI research. Future research trends included using EMA for tailoring EMI, just-in-time adaptive interventions (JITAI), and digital phenotyping.
Conclusion: This bibliometric study on mobile-based EMA and EMI is a valuable resource for understanding the field’s evolution and future trends. Our analysis indicates that EMA and EMI have great potential in health behaviors and mental health, but implementation should consider feasibility and reactivity issues carefully. Emerging trends include EMA-tailored EMI, JITAI, and digital phenotyping. In the future, strengthening multidisciplinary cooperation will be necessary to promote the continued development of the field.
1 Introduction
Ecological momentary assessment (EMA) involves various research techniques that capture data repeatedly, in real-time, and in real-life experience (1).EMA can measure natural phenomena that occur and change over time, with the strength of improving ecological validity and reducing recall bias (1). Using EMA has led to new insights into the dynamic associations among behaviors, contexts, and psychological states and may help inform the achievement of real-time and tailored interventions and treatments (2).
Ecological momentary intervention (EMI) is an extension of EMA, which facilitates the provision of real-time interventions in real-world settings (3, 4). Participants may receive behavioral or psychological support daily, practicing new behaviors and skills anytime and anywhere (5–7). EMI can also validate the efficacy of clinical interventions or treatment programs using real-world data (4). By leveraging the benefits of EMI, interventions and treatments can be systematically applied in real-world settings, increasing the accessibility and impact, making them more widely accessible, and increasing their overall impact (4).
Mobile technologies are a robust methodology that can support the implementation of EMA and EMI (1). Integrating mobile technology into EMA and EMI can provide researchers and clinicians with a more convenient and flexible way of conducting assessments and interventions. Commonly used mobile technologies, including smartphones and handheld devices, offer the ability to assess and intervene in any location at any time, enabling more comprehensive data collection and treatment implementation (4). Furthermore, mobile technologies can improve the efficiency of assessment, treatment, and care (8). For example, EMA, in combination with ambulatory physiological monitoring devices, can capture ambulatory physiological data, including heart rate and skin conductance, as well as context-sensitive data, which can offer a more accurate and complete view of the participant’s experience (9, 10). Additionally, mobile technologies enable EMI to be tailored to the participant’s characteristics and feedback and can be provided at the specific time when the participant needs the most support, increasing their satisfaction and willingness to engage with the intervention (5). By simplifying repetitive interventions and treatments, mobile technologies can also improve the efficiency of care, allowing medical staff to spend their time more effectively. This reduces duplication of work and improves the quality of treatment and care provided to patients (4).
Over the past few decades, research on mobile-based EMA and EMI has flourished in various fields, including mental health (11), dietary behaviors (12), physical activity (13), substance use (14), and addictive behaviors (15, 16); and across diverse age groups, ranging from children to the elderly (17–21), resulting in a plethora of publications each year (22–24). While there have been significant developments in mobile-based EMA and EMI research, there still needs to be more comprehensive perspectives to synthesize and summarize the current state of the field effectively. The bibliometric analysis provides a quantitative and structured method for reviewing and describing research trends and knowledge structures. Therefore, we conducted a bibliometric study of this field to inform researchers about the latest developments and trends in this rapidly evolving field.
2 Methods
2.1 Data collection
A comprehensive search was conducted on the Web of Science Core Collection database (WOSCC) to retrieve documents in the Science Citation Index Expanded (SCI-EXPANDED). The following terms and search strategies were used: (TS=“mobile” OR TS=“mobile device*” OR TS=“mobile technology” OR TS=“mhealth” OR TS=“ehealth” OR TS=“telehealth” OR TS=“mobile health” OR TS=“smartphone” OR TS=“app” OR TS=“application*” OR TS=“telemedicine” OR TS=“smartphone” OR TS=“phone”) AND (TS=“ecological momentary” OR TS=“ecological momentary assessment” OR TS=“ecological momentary intervention” OR TS=“ambulatory assessment” OR TS=“experience sampling”). The time period of the documents was chosen from the creation of the database to June 30, 2023. We retrieved a total of 2509 articles. After excluding 11 non-English language articles, 129 meeting papers, 78 unspecified, 45 abstracts, 11 books, 7 letters, 3 corrections, and 3 data papers, we finally obtained 2222 articles. The detailed information of all selected documents was downloaded in BibTeX format.
2.2 Bibliometric analysis
A bibliometric analysis of the literature on mobile-based EMI and EMA was conducted using the Bibliometrix package (version 4.0) in R (25). Initially, we extracted five BibTeX files from WOS, each containing “Full Record and Cited References” data. It’s worth noting that WOS limits each file to a maximum of 500 records, and since we had a total of 2222 records, we needed to split them into five separate files. Subsequently, we merged all of these files into a single text file using Apple Terminal Services (2.12.7). Following that, we utilized R Studio software to run the R package “Bibliometrix”. Within the interactive interface, we imported the prepared text file into the “import” section of Biblioshiny to perform Bibliometric analysis. This study examined several key bibliometric indicators, including annual document production, leading journals and authors, leading affiliations, leading countries or regions, international cooperation analysis, leading documents, keywords analysis, and trend topics analysis, to reveal the development and intellectual structure of the field. Relevant bibliometric indicators, such as density, distribution, and centrality, can be referenced for detailed definitions and calculation methods as provided in the Bibliometrix documentation, available at https://www.bibliometrix.org/vignettes/Introduction_to_bibliometrix.html. This source is an invaluable tool for understanding and applying these metrics in bibliometric analysis, offering comprehensive guidance on leveraging Bibliometrix for scholarly research evaluation and trend analysis. Biblioshiny (25), an interactive web-based application that provides a user-friendly interface to Bibliometrix, was used.
CiteSpace (version 5.7. R3; College of Information Science and Technology, Leisel University, USA), a software tool developed to visualize and analyze trends and structures in scholarly publications, was used to conduct a burst detection analysis to discover the most vigorous and impactful research areas in mobile-based EMI and EMA (26).
3 Results
3.1 Publication output and growth rate
The included documents were published from 1992 to 2023. A total of 2,222 documents were analyzed, written by 8,245 scholars, resulting in an average of 3.71 authors per document. These documents were published across 750 sources (Table 1). Figure 1A shows a broad growth in scientific publications in recent years, particularly within the last ten years. Notably, 2021 and 2022 generated the highest amount of publication output, with 351 and 353 documents published. Furthermore, mean citations per year per document were also calculated, and documents published in 2009 had the most notable mean total citations per year (Figure 1B).
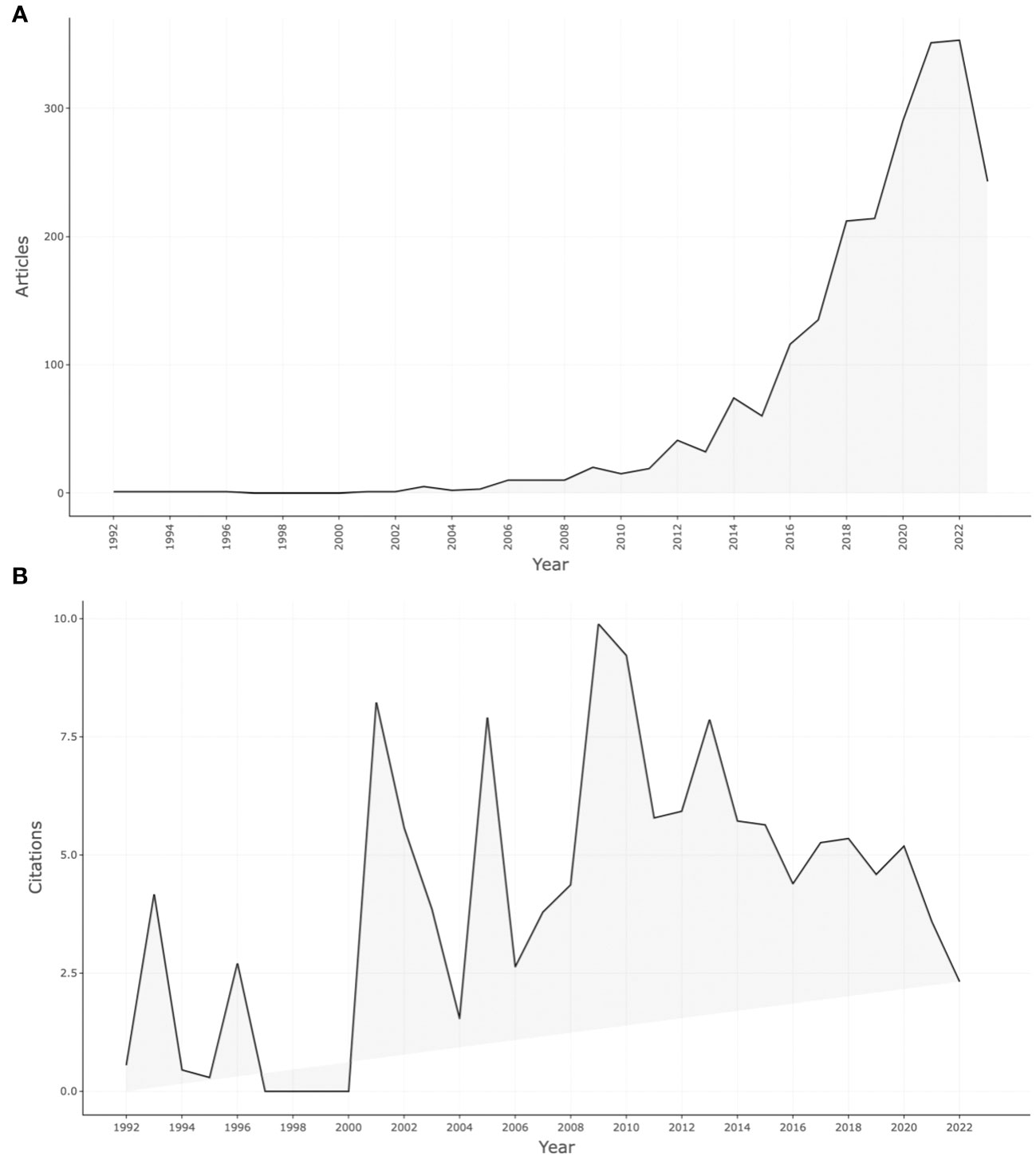
Figure 1 Annual publications of mobile-based EMA and EMI from 1992 to 2023 (A); Average citations per year of documents in mobile-based EMA and EMI (B).
3.2 Leading journals
The journal that has published the most documents in the field was JMIR mHealth and uHealth (n=86), followed by Journal of Medical Internet Research (JMIR) (n=73), and JMIR formative research (n=50), as shown in Figure 2A. The dynamics of the top five sources are displayed in Figure 2B, which revealed that, in the beginning, there were very few sources in this field. However, all sources demonstrated a yearly growth trend. Notably, JMIR mHealth and uHealth started relatively late but exhibited rapid growth, with 86 total documents published between its first publication in 2014 and 2023. Journal of Medical Internet Research also experienced substantial growth, from the first document published in 2006 to 73 documents published in 2023.
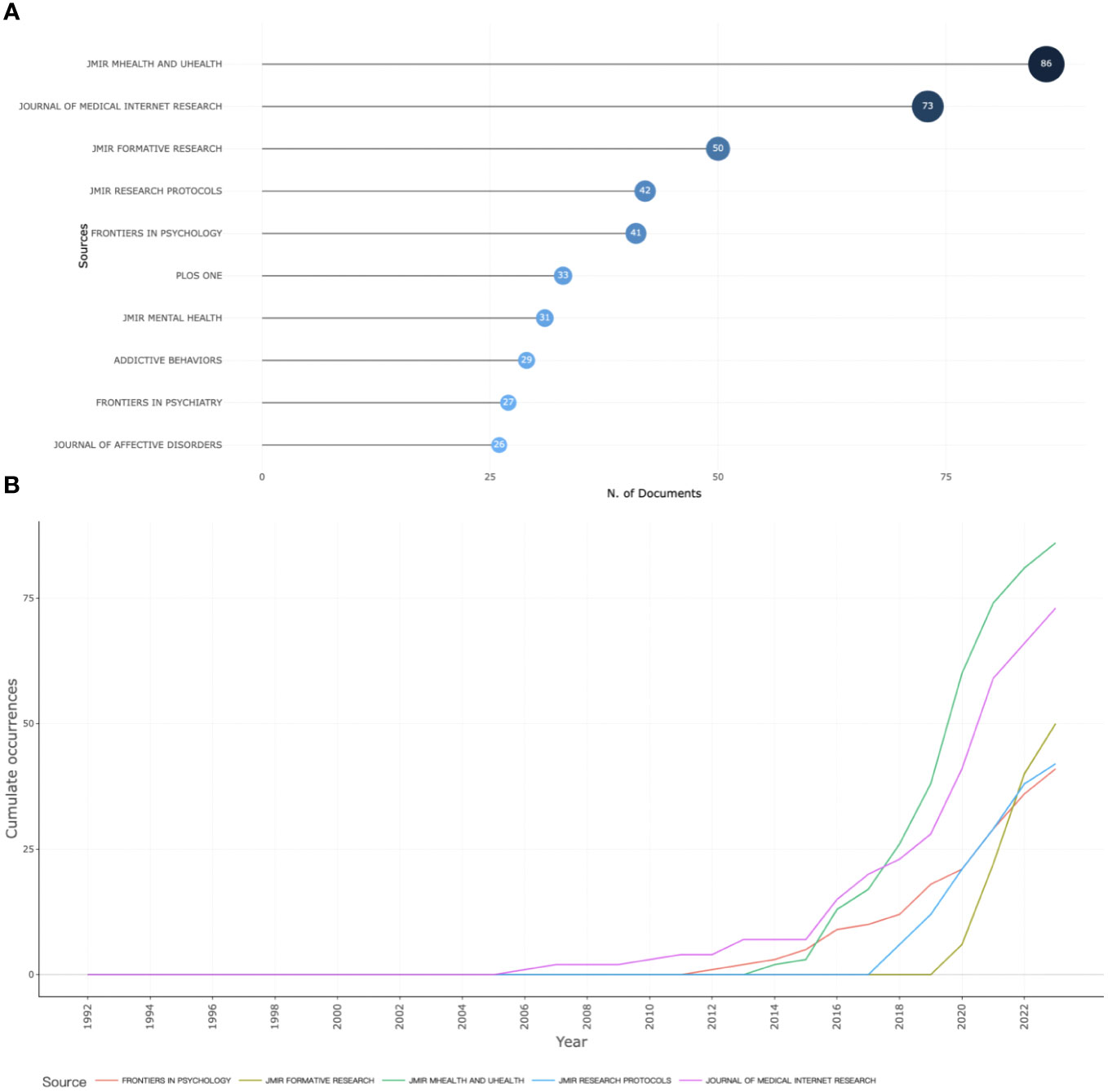
Figure 2 Most relevant sources in mobile technology-based EMA and EMI (A); Dynamics of the top five sources (B).
3.3 Leading authors
As shown in Figure 3, the leading authors publishing the greatest number of documents have been identified. Colin A Depp from the University of California, San Diego, authored the most publications with 35. Genevieve DuntonF, affiliated with the University of Southern California, was the second most prolific author, having published 31 documents. Raeanne C. Moore, associated with University of California, San Diego, was ranked third with 28 publications.
3.4 Leading affiliations
As shown in Figure 4A, the University of Pittsburgh was the top-ranking affiliation for research in this area, with a total of 148 publications. Penn State University followed with 138 publications, and the Maastricht University ranked third with 137 publications. To enhance the understanding of inter-institutional relationships, the methodologies of coupling and clustering are employed in the analysis. In the context of institution-specific investigations, “coupling” delineates the connection established by two or more research institutions through the mutual citation of identical scholarly literature. Conversely, “clustering” involves the aggregation of these research institutions based on the intensity of their coupling, thereby forming distinct research clusters or networks (27). This organization facilitates a comprehensive comprehension of the structure and dynamics underlying research collaborations. Within this analytical framework, each node symbolizes a distinct institution, with the node’s color denoting the cluster to which the institution is affiliated. Figure 4B shows the leading institutions divided into 7 clusters, each represented by a different color. This indicates the presence of connections among the institutions. Furthermore, nodes and links sharing identical colors suggest that a significant proportion of the papers cited by these universities originate from institutions within the same or closely associated clusters. This pattern also intimates that authors tend to collaborate and communicate predominantly within their proximate geographical regions and communities. Kings College London and Maastricht University collaborated vigorously, as did The University of Melbourne and Deakin University.
The dynamics of the top 5 affiliations are displayed in Supplementary Figure 1. Penn State University and the University of Pittsburgh are among the institutions that started early and have seen a swift increase in the number of published articles. Penn State University’s output grew from 3 articles in 2002 to 138 articles by 2023, while the University of Pittsburgh went from publishing 3 articles in 2003 to 148 articles by 2023. Although Maastricht University commenced its publishing activities later than the first two, with its first article published in 2006, its growth has been rapid, reaching a total of 137 publications by 2023.
3.5 Leading countries
Forty-five countries in total were incorporated in the completed documents. Regarding the quantity of publications, the United States ranked first (n=1038), with Germany (n=218) and Netherlands (n=175) coming in second and third. The United States produced the greatest single country publications (n=898), followed by Germany (n=135) and Netherlands (n=108). Multiple country publications were led by the US (n=140), followed by Germany (n=83) and the Netherlands (n=67) (Figure 5A; Supplementary Table 1). In terms of country-to-country cooperation, as shown in Figure 5B and Supplementary Table 2, the collaboration between the Netherlands and the United Kingdom was the strongest (n=51), followed by the United States and the United Kingdom (n=50), and Germany and Netherlands (n=46).
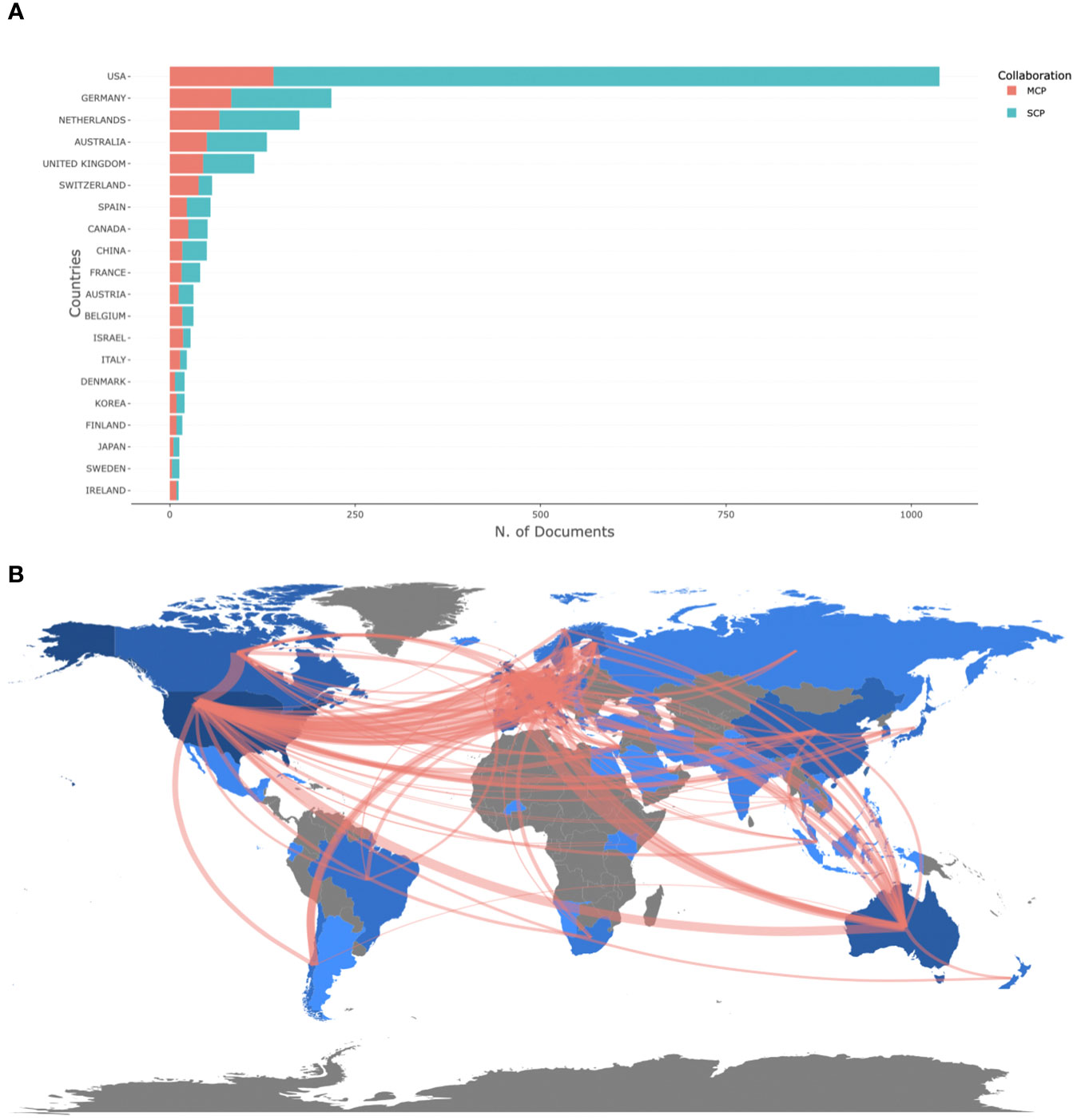
Figure 5 The leading countries according to the amount of documents the author’s country tallied. SCP: Single Country Publications; MCP: Multiple Country Publications (A); The international cooperation network map (B).
3.6 Leading documents
Global citations can represent the impact of documents in the comprehensive database. Figure 6A displays the top 10 most cited documents globally. Notably, Heron’s 2010 article (4) was the most cited global article, with 825 citations, while Nahum-Shani’s 2018 (28) article was the second most cited global document, with 732 citations. Another noteworthy publication is the 2008 article by Shiffman (1), which had the highest number of citations as a reference at 753, according to Figure 6B. These highly cited documents serve as invaluable references for researchers to build upon to advance the field further.
3.7 Keyword analysis
Three thousand nine hundred and forty-six keywords plus from all the selected publications were analyzed in the keyword analysis. The top 10 most widely used keywords in this field were ecological momentary assessment (403), health (219), depression (218), validity (181), behavior (165), stress (141), anxiety (140), physical activity (134), mood (132) and validation (123) (Figure 7A; Supplementary Table 3). To further explore the association between keywords, cluster analysis was further used in the analysis. Cluster analysis of keywords involves grouping keywords by quantifying their co-occurrence frequencies, with keywords in each colored cluster indicating that they are mostly used together (27). The larger the node, the more frequently the keyword is used. The most commonly used keywords were plotted as 3 clusters (Figure 7B). Cluster 1 (red) included depression, validity, stress, anxiety, mood, disorders, schizophrenia, cognitive-behavioral therapy, and depressive symptoms. Cluster 2 (blue) included behavior, physical-activity, time, exercise, consumption, smoking, obesity, and alcohol-use. Cluster 3 (green) included feasibility, interventions, prevalence, reactivity and technology.
3.8 Trend topics
Based on the keyword co-occurrence network, the centrality and density of each cluster are calculated and the theme map is drawn, with each circle representing a cluster of the same color. Centrality indicates the proximity of a topic to other topics, while density indicates the maturity of a topic. In the theme map (Figure 8), the first quadrant (upper right) represents motor themes, which are both significant and fully developed. The second quadrant (upper left) represents niche themes, which are fully developed but have yet to be relevant to the field. The third quadrant (lower left) includes emerging or declining themes, representing marginal ideas that need to be well-developed, may have recently emerged, or are on the verge of disappearing. The fourth quadrant (lower right) includes basic themes that are essential to the field but have yet to be fully explored.
Health-related behaviors such as smoking, substance abuse, alcohol consumption, as well as psychological aspects like depression, anxiety, and stress, along with technology-related assessments and interventions, were important and well-developed topics for mobile-based EMA and EMI. Specifically, the United States also appears in this topic, reflecting the leadership position of the United States with numerous papers published in the field.
Exploring individual differences and personality traits were crucial topics in the current field and needed further development, as they were located in quadrant 4 of the theme map. Although these topics were essential, they were not yet well-developed, and increased research was warranted to explore their potential in various health domains.
In the context of EMA and EMI applications, issues such as engagement and reactivity fell into the emerging or declining categories. These categories represented marginal ideas that required further development and exploration.
Keyword emergence refers to the rapid surge in the frequency of keywords at a certain point, reflecting the research frontier in the field. Figure 9 displays the top 25 keywords with the strongest citation bursts spanning from 2003 to 2023. Since 2020, the terms “digital health”, “digital phenotyping”, “quality”, “sleep”, and “psychometric properties” have received the most attention, indicating possible future frontiers for research in mobile-based EMA and EMI.
Furthermore, an analysis of trending topics was conducted on 164 reviews within the selected literature, as depicted in Supplementary Figure 2. The findings reveal a concentrated focus on psychiatric disorders, notably bipolar disorder and schizophrenia, between 2017 and 2019. This focus shifted towards cognitive-behavioral therapies, anxiety, and depression from 2019 to 2021, among other areas pertinent to psychological disorders. Beyond 2021, the thematic emphasis of the reviews transitioned towards generalized disorders, symptoms, and the reliability of EMI approaches.
4 Discussion
4.1 Publication output and growth rate
Over the course of decades, EMA and EMI have undergone significant development, with their theories and technologies progressively maturing and being applied to an ever-expanding range of disciplines. In 1994, Stone and Shiffman introduced EMA as a method that could be performed using tiny mobile technologies (1). Since then, EMA has gradually evolved, and numerous books and articles have been written to describe the method. In 2008, a more comprehensive review of the rationale, design, methodology, and usage of EMA was provided by Shiffman (1). In 2010, Heron presented the first analysis of the benefits of mobile technology for EMI and reviewed the implementation of mobile-based EMIs across different disciplines. This provided valuable guidance for the future implementation of EMA and EMI using mobile technology and helped advance the use of EMA and EMI to a new stage (4). In the last decades, the utilization of EMA and EMI in areas including substance abuse, mental health, and physical activity began to grow significantly.
Furthermore, dynamically and individually tailored EMI emerged, also referred to as Just-in-Time Adaptive Intervention (JITAI) (4, 28). JITAI leverages mobile and sensing technologies to monitor participants’ ever-changing internal and contextual states in real-time (29). It offers flexible support delivery concerning time and location, providing timely and targeted interventions when they are most likely to be received and effective (29, 30). JITAI has been increasingly used to support health behavior interventions in various domains (28). The explosive growth in mobile-based EMA and EMI documents, fueled by advances like JITAI, demonstrates the enormous potential of this field. A concerted effort from diverse disciplines and professions will be critical to comprehensively grasp the potential of mobile-based EMA and EMI and drive future innovations in research and practice.
4.2 Leading journals
The journals JMIR mHealth and uHealth, JMIR, and JMIR formative research were the primary research sources on mobile-based EMA and EMI. With 209 documents published between 1992 and 2023, these journals offer a wealth of reliable literature. Scholars can use these journals to keep abreast of the latest research trends and findings. Moreover, the journals’ submission guidelines provide valuable references for researchers seeking to publish their work.
4.3 Leading countries and affiliations
The United States had the highest volume of mobile-based EMA and EMI publications, surpassing Germany, the second-highest-ranking country by over four times. The University of Pittsburgh and Penn State University, both leading institutions, are based in the United States, underlining its significant contributions to this field. Among the leading countries with the most scholarly publications in mobile-based EMA and EMI, developed countries played a primary role. It is notable that China, as the only developing country, has also contributed to this field’s development. Furthermore, the publication outputs of cooperative countries were lower compared to individual countries. Collaborative efforts among authors, countries, and institutions are crucial for advancing this research field. Therefore, it is recommended to continue promoting international collaborations among scholars and institutions to facilitate progress.
4.4 Research hotspots and trend topics
The first red cluster was centered around mental health, including depression, validity, stress, anxiety, mood, disorders, schizophrenia, and cognitive-behavioral therapy (CBT). Mental illness is a significant challenge in modern healthcare, with most individuals experiencing mental health problems at some point (31, 32). Mobile technologies’ utility in treating mental disorders is a significant component of the future of mental health management, and smartphones can enable patients to have a positive role in managing mental health syndromes and aiding in their recovery (33–35). By using mobile technology, EMA and EMI delivered through messaging, apps, and phone use can provide patients with much-needed psychological support in their daily lives. EMA is frequently used as a research tool to examine symptoms and potential mechanisms, and the reliability and efficacy have been established among this population (36–38). Additionally, There is an increasing interest in utilizing the EMA method for clinical purposes, including accurately assessing symptoms, identifying and monitoring signs of relapse, and tracking treatment effectiveness (39). CBT is a widely used form of psychotherapy effective in treating mental health conditions (40, 41). EMI using CTB is highly effective, particularly in the areas of depression (42, 43), anxiety (43), and schizophrenia (44). It also increases self-management abilities, satisfaction, positive emotions, and positive well-being and facilitates the development of learning skills (45). Our analysis of the theme map reveals that mental health is a rapidly developing and crucial area in mobile-based EMA and EMI research. It aligns with previous research consistently identifying it as the most prevalent theme (46), representing current and future research directions and hot topics.
The second blue cluster focused on behavior, physical-activity, time, exercise, consumption, smoking, obesity, and alcohol-use, which are associated with the role of mobile-based EMA and EMI in health behaviors. Physical activity, sedentary behavior, dietary behavior, smoking, and excessive alcohol consumption are critical health behaviors that can lead to the onset of diseases and premature death (47, 48). The primary characteristic of healthy behaviors is that they needs to be carried out consistently and regularly, ideally daily or several times per day, and sustained over the long term, even throughout an individual’s lifespan (49). However, maintaining healthy behaviors can be particularly challenging due to daily fluctuations in factors such as mood, social interactions, environmental obstacles, and location, making it challenging to maintain a consistent routine (49). EMA and EMI have emerged as effective tools for monitoring and managing health behaviors. EMA has been used in a broad spectrum of health behaviors (50–53), and study results provide a more accurate insight into how health behaviors change over time context (54). EMI has been implemented in the fields of smoking cessation (55), physical activity (56), alcohol cessation (57), and substance use (14) and has achieved some progress and effectiveness. However, some research results have yet to be significant, and it is hoped that future scholars will continue to explore and study (58, 59). The mobile-based EMA and EMI in health behaviors are promising and vigorously developing themes in the motor domain.
The third green cluster included feasibility, interventions, prevalence, reactivity and technology. Despite the numerous benefits of EMA and EMI, implementing these approaches can present several feasibility challenges. For example, frequent assessments may disrupt participants’ routines, potentially leading to lower adherence and increased dropout rates, particularly among patients with certain diseases (60, 61). In addition to these challenges, cost, participant attitudes, confidentiality concerns, and equipment loss or damage are other factors that must be addressed for successful implementation and patient acceptance (3). Another important consideration is the potential for reactivity or behavioral changes when study participants become aware that they are being assessed or intervened with. Reactivity could negatively impact the validity and accuracy of study results (4). Given these challenges and difficulties, it is important to identify key factors that can improve feasibility and compliance, and reduce reactivity. Future guidelines should systematically address these factors (61). Despite the challenges, advancements in mobile technology offer promise for enhancing the usability and acceptability of EMA and EMI approaches. It is a promising area for further development and exploration (3).
Additionally, the trend topics analysis specifically targeting reviews also uncovered some interesting findings. The initial reviews focused on the application of EMA and EMI within the field of psychiatry, primarily targeting bipolar disorder and schizophrenia. As the treatment of mental illnesses evolved, the application of EMA and EMI in cognitive-behavioral therapy also saw substantial development. Furthermore, EMA and EMI have been applied in the realm of psychological disorders, such as anxiety and depression. In recent years, EMA and EMI have been involved in symptom management across various diseases, thus shifting the review’s keywords towards more broadly defined terms such as “disorders” and “symptoms”. With the widespread application of EMA and EMI, their reliability has also got great attention, giving rise to numerous papers and reviews in this field. Unlike previous reviews that focused on specific areas related to EMA and EMI, this article, as a review utilizing bibliometrics, explores more broadly the development trajectory, application domains, and current state of EMA and EMI, serving as a summary and supplement to existing literature.
Currently, mobile-based EMA and EMI have shown significant progress in multiple fields. They also expand gradually to other health domains (23). Additionally, there has been a consistent emphasis on tailoring EMA and EMI as a trend. Tailoring EMI based on EMA is a new research area that can increase user engagement and intervention effectiveness. However, its efficacy is still to be verified, and robust clinical trials will be needed to test tailoring EMI based on EMA in different populations and settings (46). The era of implementing interventions using Just-In-Time Adaptive Interventions (JITAI) is approaching. A key research direction for the future is to develop sophisticated and intricate health behavior theories to guide the design and delivery of such interventions while implementing them (28). Another crucial trend is the emergence of digital phenotyping, which, aided by machine learning, generates ecological, continuous, and personalized digital phenotypes to enhance diagnostic and evaluative accuracy based on EMA data collected from smartphones, wearables, and human-computer interactions (61–64). The advent of digital tools may pose unprecedented challenges to clinical and subjective assessments by clinicians and questionnaires. It underscores the potential for digital tools to bring about revolutionary breakthroughs and developments in mental health and healthcare. Achieving this will require collaborative efforts from interdisciplinary teams in the future (65).
4.5 Limitations
Although the research offers valuable perspectives on EMA and EMI in mobile technology literature from 1992 to 2023, several limitations should be considered. Firstly, our study only searched the WOS database, which may not provide a complete picture of all relevant publications. Including additional databases like Scopus and PubMed could yield a more comprehensive view of the literature. Furthermore, despite our best efforts to ensure the precision and comprehensiveness of our search, there may still be omissions in our selection of keywords. Consequently, the results are inevitably influenced by the selected keywords. Additionally, the data on citations may be subject to temporal limitations, with papers published earlier often having a higher citation frequency than those published more recently. This could result in some influential papers not receiving the attention they deserve simply because they were published later. Moreover, the studies included in this article are published in English, which might introduce a language bias into the bibliometric analysis. This could lead to the omission of significant research published in other languages, thereby making the analysis less comprehensive. Finally, our analysis only covers publications until the search date, which may limit the validity of our findings. Future updates may be necessary to capture new developments in the field. Finally, to achieve a deeper overview of the current state and emerging research frontiers, it may be beneficial for future studies to engage and collaborate with experts in the field.
5 Conclusion
Our study employed bibliometric methods to provide a bird’s eye view of mobile-based EMA and EMI research, offering valuable insights for scholars to understand these technologies evolution, development, and future trends. Our analysis of the most productive and influential authors, countries, and core journals has guided researchers in selecting journals to submit to and potential research partners to collaborate with. Furthermore, in the analysis of the hot topics, we found that mobile-based EMA and EMI show great potential in the fields of health behaviors and mental health. However, it is necessary to rigorously and carefully consider the feasibility and reactivity issues during the implementation process. In the future, mobile-based EMA and EMI could be expanded to other health domains, and research on EMA-tailored EMI, JITAI and the incorporation of wearable devices, machine learning, and digital phenotyping are emerging trends. This study offers a valuable reference for scholars to understand the evolution, development, and future trends of EMA and EMI in mobile technology and inform their future research choices.
Data availability statement
The original contributions presented in the study are included in the article/Supplementary Material, further inquiries can be directed to the corresponding author/s.
Author contributions
HY: Conceptualization, Data curation, Methodology, Writing – original draft, Writing – review & editing. HZ: Conceptualization, Methodology, Writing – original draft. JG: Data curation, Writing – original draft. HQ: Data curation, Writing – original draft. WD: Data curation, Methodology, Writing – review & editing. NG: Data curation, Methodology, Supervision, Writing – review & editing. JF: Conceptualization, Data curation, Methodology, Supervision, Writing – review & editing. YY: Conceptualization, Data curation, Methodology, Supervision, Writing – review & editing.
Funding
The author(s) declare financial support was received for the research, authorship, and/or publication of this article. This study was funded by the General Project of National Natural Science Foundation of China in 2021 (No.72174120), Shanghai Jiao Tong University School of Medicine: Nursing Development Program, innovation research team of high-level local universities in Shanghai -SHSMU-ZDCX20212800.
Acknowledgments
We would like to thank Andy Fan from the University of Pennsylvania for his assistance and guidance in this research.
Conflict of interest
The authors declare that the research was conducted in the absence of any commercial or financial relationships that could be construed as a potential conflict of interest.
Publisher’s note
All claims expressed in this article are solely those of the authors and do not necessarily represent those of their affiliated organizations, or those of the publisher, the editors and the reviewers. Any product that may be evaluated in this article, or claim that may be made by its manufacturer, is not guaranteed or endorsed by the publisher.
Supplementary material
The Supplementary Material for this article can be found online at: https://www.frontiersin.org/articles/10.3389/fpsyt.2024.1300739/full#supplementary-material
References
1. Shiffman S, Stone AA, Hufford MR. Ecological momentary assessment. Annu Rev Clin Psychol. (2008) 4:1–32. doi: 10.1146/annurev.clinpsy.3.022806.091415
2. Robbins ML, Kubiak T. Ecological momentary assessment in behavioral medicine. In: The Handbook of Behavioral Medicine Hoboken, NJ, US: Wiley Blackwell (2014). p. 429–46.
3. Bell IH, Lim MH, Rossell SL, Thomas N. Ecological momentary assessment and intervention in the treatment of psychotic disorders: A systematic review. Psychiatr Serv. (2017) 68:1172–81. doi: 10.1176/appi.ps.201600523
4. Heron KE, Smyth JM. Ecological momentary interventions: Incorporating mobile technology into psychosocial and health behaviour treatments. Br J Health Psychol. (2010) 15:1–39. doi: 10.1348/135910709x466063
5. Newman MG, Consoli A, Taylor CB. Computers in assessment and cognitive behavioral treatment of clinical disorders: Anxiety as a case in point. Behav Ther. (1997) 28:211–35. doi: 10.1016/s0005-7894(97)80044-5
7. Kazantzis N, LĽAbate L. Introduction and historical overview. In: Handbook of Homework Assignments In Psychotherapy: Research, Practice, And Prevention. Boston, MA: Springer US (2007). p. 1–15.
8. Newman MG, Kenardy J, Herman S, Taylor CB. Comparison of palmtop-computer-assisted brief cognitive-behavioral treatment to cognitive-behavioral treatment for panic disorder. J Consulting Clin Psychol. (1997) 65:178–83. doi: 10.1037/0022-006x.65.1.178
9. Ben-Zeev D, Wang R, Abdullah S, Brian R, Scherer EA, Mistler LA, et al. Mobile behavioral sensing for outpatients and inpatients with schizophrenia. Psychiatr Serv. (2016) 67:558–61. doi: 10.1176/appi.ps.201500130
10. Wang R, Aung MSH, Abdullah S, Brian R, Campbell AT, Choudhury T, et al. (2016). Crosscheck: Toward passive sensing and detection of mental health changes in people with schizophrenia. Proceedings of the 2016 ACM International Joint Conference on Pervasive and Ubiquitous Computing (Heidelberg, Germany: Association for Computing Machinery) (2016) p. 886–97.
11. Oorschot M, Kwapil T, Delespaul P, Myin-Germeys I. Momentary assessment research in psychosis. psychol Assess. (2009) 21:498–505. doi: 10.1037/a0017077
12. Engel SG, Crosby RD, Thomas G, Bond D, Lavender JM, Mason T, et al. Ecological momentary assessment in eating disorder and obesity research: A review of the recent literature. Curr Psychiatry Rep. (2016) 18. doi: 10.1007/s11920-016-0672-7
13. Zapata-Lamana R, Lalanza JF, Losilla JM, Parrado E, Capdevila L. Mhealth technology for ecological momentary assessment in physical activity research: A systematic review. Peerj. (2020) 8:e8848. doi: 10.7717/peerj.8848
14. Carreiro S, Newcomb M, Leach R, Ostrowski S, Boudreaux ED, Amante D. Current reporting of usability and impact of mhealth interventions for substance use disorder: A systematic review. Drug Alcohol Depend. (2020) 215:108201. doi: 10.1016/j.drugalcdep.2020.108201
15. Vinci C, Haslam A, Lam CY, Kumar S, Wetter DW. The use of ambulatory assessment in smoking cessation. Addictive Behav. (2018) 83:18–24. doi: 10.1016/j.addbeh.2018.01.018
16. Lukasiewicz M, Fareng M, Benyamina A, Blecha L, Reynaud M, Falissard B. Ecological momentary assessment in addiction. Expert Rev Neurother. (2007) 7:939–50. doi: 10.1586/14737175.7.8.939
17. Bry LJ, Chou T, Miguel E, Comer JS. Consumer smartphone apps marketed for child and adolescent anxiety: A systematic review and content analysis. Behav Ther. (2018) 49:249–61. doi: 10.1016/j.beth.2017.07.008
18. Williams JE, Pykett J. Mental health monitoring apps for depression and anxiety in children and young people: A scoping review and critical ecological analysis. Soc Sci Med. (2022) 297:114802. doi: 10.1016/j.socscimed.2022.114802
19. Grossman JT, Frumkin MR, Rodebaugh TL, Lenze EJ. Mhealth assessment and intervention of depression and anxiety in older adults. Harvard Rev Psychiatry. (2020) 28:203–14. doi: 10.1097/hrp.0000000000000255
20. Beames JR, Kikas K, Werner-Seidler A. Prevention and early intervention of depression in young people: An integrated narrative review of affective awareness and ecological momentary assessment. BMC Psychol. (2021) 9(1):113. doi: 10.1186/s40359-021-00614-6
21. Kim H, Kim S, Kong SS, Jeong YR, Kim H, Kim N. Possible application of ecological momentary assessment to older adults' Daily depressive mood: Integrative literature review. JMIR Ment Health. (2020) 7(6):e13247. doi: 10.2196/13247
22. Hamaker EL, Wichers M. No time like the present: Discovering the hidden dynamics in intensive longitudinal data. Curr Dir psychol Sci. (2017) 26:10–5. doi: 10.1177/0963721416666518
23. Burke LE, Shiffman S, Music E, Styn MA, Kriska A, Smailagic A, et al. Ecological momentary assessment in behavioral research: Addressing technological and human participant challenges. J Med Internet Res. (2017) 19(3):e77. doi: 10.2196/jmir.7138
24. Ginexi EM, Riley W, Atienza AA, Mabry PL. The promise of intensive longitudinal data capture for behavioral health research. Nicotine Tobacco Res. (2014) 16:S73–S5. doi: 10.1093/ntr/ntt273
25. Aria M, Cuccurullo C. Bibliometrix: An R-tool for comprehensive science mapping analysis. J Informetrics. (2017) 11:959–75. doi: 10.1016/j.joi.2017.08.007
26. Synnestvedt MB, Chen C, Holmes JH. (2005). Citespace ii: Visualization and knowledge discovery in bibliographic databases, in: AMIA Annual Symposium proceedings AMIA Symposium, . pp. 724–8.
27. Ezugwu AE, Shukla AK, Agbaje MB, Oyelade ON, José-García A, Agushaka JO. Automatic clustering algorithms: A systematic review and bibliometric analysis of relevant literature. Neural Computing Appl. (2021) 33:6247–306. doi: 10.1007/s00521-020-05395-4
28. Nahum-Shani I, Smith SN, Spring BJ, Collins LM, Witkiewitz K, Tewari A, et al. Just-in-time adaptive interventions (Jitais) in mobile health: Key components and design principles for ongoing health behavior support. Ann Behav Med. (2018) 52:446–62. doi: 10.1007/s12160-016-9830-8
29. Spruijt-Metz D, Nilsen W. Dynamic models of behavior for just-in-time adaptive interventions. IEEE Pervasive Computing. (2014) 13:13–7. doi: 10.1109/mprv.2014.46
30. Kumar S, Nilsen WJ, Abernethy A, Atienza A, Patrick K, Pavel M, et al. Mobile health technology evaluation the mhealth evidence workshop. Am J Prev Med. (2013) 45:228–36. doi: 10.1016/j.amepre.2013.03.017
31. Copeland W, Shanahan L, Costello EJ, Angold A. Cumulative prevalence of psychiatric disorders by young adulthood: A prospective cohort analysis from the great smoky mountains study. J Am Acad Child Adolesc Psychiatry. (2011) 50:252–61. doi: 10.1016/j.jaac.2010.12.014
32. Moffitt TE, Caspi A, Taylor A, Kokaua J, Milne BJ, Polanczyk G, et al. How common are common mental disorders? Evidence that lifetime prevalence rates are doubled by prospective versus retrospective ascertainment. psychol Med. (2010) 40:899–909. doi: 10.1017/S0033291709991036
33. Ben-Zeev D, Davis KE, Kaiser S, Krzsos I, Drake RE. Mobile technologies among people with serious mental illness: Opportunities for future services. Administration Policy Ment Health Ment Health Serv Res. (2013) 40:340–3. doi: 10.1007/s10488-012-0424-x
34. Drake RE, Whitley R. Recovery and severe mental illness: Description and analysis. Can J Psychiatry-Revue Can Psychiatr. (2014) 59:236–42. doi: 10.1177/070674371405900502
35. Gravenhorst F, Muaremi A, Bardram J, Grunerbl A, Mayora O, Wurzer G, et al. Mobile phones as medical devices in mental disorder treatment: An overview. Pers Ubiquitous Computing. (2015) 19:335–53. doi: 10.1007/s00779-014-0829-5
36. Brenner CJ, Ben-Zeev D. Affective forecasting in schizophrenia: Comparing predictions to real-time ecological momentary assessment (Ema) ratings. Psychiatr Rehabil J. (2014) 37:316–20. doi: 10.1037/prj0000105
37. Granholm E, Loh C, Swendsen J. Feasibility and validity of computerized ecological momentary assessment in schizophrenia. Schizophr Bull. (2008) 34:507–14. doi: 10.1093/schbul/sbm113
38. Palmier-Claus JE, Ainsworth J, Machin M, Barrowclough C, Dunn G, Barkus E, et al. The feasibility and validity of ambulatory self-report of psychotic symptoms using a smartphone software application. BMC Psychiatry. (2012) 12(1):172. doi: 10.1186/1471-244x-12-172
39. Trull TJ, Ebner-Priemer UW. Using experience sampling methods/ecological momentary assessment (Esm/ema) in clinical assessment and clinical research: Introduction to the special section. psychol Assess. (2009) 21:457–62. doi: 10.1037/a0017653
40. Butler AC, Chapman JE, Forman EM, Beck AT. The empirical status of cognitive-behavioral therapy: A review of meta-analyses. Clin Psychol Rev. (2006) 26:17–31. doi: 10.1016/j.cpr.2005.07.003
41. David D, Cristea I, Hofmann SG. Why cognitive behavioral therapy is the current gold standard of psychotherapy. Front Psychiatry. (2018) 9:4. doi: 10.3389/fpsyt.2018.00004
42. Hur JW, Kim B, Park D, Choi SW. A scenario-based cognitive behavioral therapy mobile app to reduce dysfunctional beliefs in individuals with depression: A randomized controlled trial. Telemedicine E-Health. (2018) 24:710–6. doi: 10.1089/tmj.2017.0214
43. Watts S, Mackenzie A, Thomas C, Griskaitis A, Mewton L, Williams A, et al. Cbt for depression: A pilot rct comparing mobile phone vs. Computer. BMC Psychiatry. (2013) 13(1):49. doi: 10.1186/1471-244x-13-49
44. Ben-Zeev D. Mobile technologies in the study, assessment, and treatment of schizophrenia. Schizophr Bull. (2012) 38:384–5. doi: 10.1093/schbul/sbr179
45. Marciniak MA, Shanahan L, Rohde J, Schulz A, Wackerhagen C, Kobylinska D, et al. Standalone smartphone cognitive behavioral therapy-based ecological momentary interventions to increase mental health: Narrative review. JMIR Mhealth Uhealth. (2020) 8(11):e19836. doi: 10.2196/19836
46. Dao KP, De Cocker K, Tong HL, Kocaballi AB, Chow C, Laranjo L. Smartphone-delivered ecological momentary interventions based on ecological momentary assessments to promote health behaviors: Systematic review and adapted checklist for reporting ecological momentary assessment and intervention studies. JMIR Mhealth Uhealth. (2021) 9:e22890. doi: 10.2196/22890
47. Murray CJ, Aravkin AY, Zheng P, Abbafati C, Abbas KM, Abbasi-Kangevari M, et al. Global burden of 87 risk factors in 204 countries and territories, 1990–2019: A systematic analysis for the global burden of disease study 2019. Lancet. (2020) 396:1223–49. doi: 10.1016/S0140-6736(20)30752-2
48. Perski O, Keller J, Kale D, Asare BYA, Schneider V, Powell D, et al. Understanding health behaviours in context: A systematic review and meta-analysis of ecological momentary assessment studies of five key health behaviours. Health Psychol Rev. (2022) 16:576–601. doi: 10.1080/17437199.2022.2112258
49. Dunton GF. Ecological momentary assessment in physical activity research. Exercise Sport Sci Rev. (2017) 45:48–54. doi: 10.1249/jes.0000000000000092
50. Shiffman S, Stone AA. Introduction to the special section: Ecological momentary assessment in health psychology. Health Psychol. (1998) 17:3. doi: 10.1037/h0092706
51. Dunton GF, Liao Y, Kawabata K, Intille S. Momentary assessment of adults' Physical activity and sedentary behavior: Feasibility and validity. Front Psychol. (2012) 3:260. doi: 10.3389/fpsyg.2012.00260
52. Liao Y, Intille SS, Dunton GF. Using ecological momentary assessment to understand where and with whom adults' Physical and sedentary activity occur. Int J Behav Med. (2015) 22:51–61. doi: 10.1007/s12529-014-9400-z
53. McKee HC, Ntoumanis N, Taylor IM. An ecological momentary assessment of lapse occurrences in dieters. Ann Behav Med. (2014) 48:300–10. doi: 10.1007/s12160-014-9594-y
54. Reichert M, Giurgiu M, Koch ED, Wieland LM, Lautenbach S, Neubauer AB, et al. Ambulatory assessment for physical activity research: State of the science, best practices and future directions. Psychol Sport Exercise. (2020) 50:101742. doi: 10.1016/j.psychsport.2020.101742
55. Rodgers A, Corbett T, Bramley D, Riddell T, Wills M, Lin RB, et al. Do U smoke after txt? Results of a randomised trial of smoking cessation using mobile phone text messaging. Tob Control. (2005) 14:255–61. doi: 10.1136/tc.2005.011577
56. Schwerdtfeger AR, Schmitz C, Warken M. Using text messages to bridge the intention-behavior gap? A pilot study on the use of text message reminders to increase objectively assessed physical activity in daily life. Front Psychol. (2012) 3:270. doi: 10.3389/fpsyg.2012.00270
57. Weitzel JA, Bernhardt JM, Usdan S, Mays D, Glanz K. Using wireless handheld computers and tailored text messaging to reduce negative consequences of drinking alcohol. J Stud Alcohol Drugs. (2007) 68:534–7. doi: 10.15288/jsad.2007.68.534
58. Wright C, Dietze PM, Agius PA, Kuntsche E, Livingston M, Black OC, et al. Mobile phone-based ecological momentary intervention to reduce young adults' Alcohol use in the event: A three-armed randomized controlled trial. JMIR Mhealth Uhealth. (2018) 6:e149. doi: 10.2196/mhealth.9324
59. Riordan BC, Winter T, Carey KB, Conner TS, Moradi S, Jang K, et al. A combined web based intervention and ecological momentary intervention for reducing alcohol use among incoming first-year university students: Results from a three-arm randomised controlled trial. Addict Behav. (2023) 136:107471. doi: 10.1016/j.addbeh.2022.107471
60. Lin XL, Mermelstein RJ, Hedeker D. A 3-level bayesian mixed effects location scale model with an application to ecological momentary assessment data. Stat Med. (2018) 37:2108–19. doi: 10.1002/sim.7627
61. Colombo D, Fernandez-Alvarez J, Patane A, Semonella M, Kwiatkowska M, Garcia-Palacios A, et al. Current state and future directions of technology-based ecological momentary assessment and intervention for major depressive disorder: A systematic review. J Clin Med. (2019) 8(4). doi: 10.3390/jcm8040465
62. Yim SJ, Lui LMW, Lee Y, Rosenblat JD, Ragguett RM, Park C, et al. The utility of smartphone-based, ecological momentary assessment for depressive symptoms. J Affect Disord. (2020) 274:602–9. doi: 10.1016/j.jad.2020.05.116
63. Hirschtritt ME, Insel TR. Digital technologies in psychiatry: Present and future. Focus (American Psychiatr Publishing). (2018) 16:251–8. doi: 10.1176/appi.focus.20180001
64. Dwyer DB, Falkai P, Koutsouleris N. Machine learning approaches for clinical psychology and psychiatry. Annu Rev Clin Psychol. (2018) 14:91–118. In: Widiger T, Cannon TD, editors. Annual Review of Clinical Psychology, Vol 14. doi: 10.1146/annurev-clinpsy-032816-045037
Keywords: mobile technology, ecological momentary assessment, ecological momentary intervention, ambulatory assessment, bibliometric analysis
Citation: Yin H, Zhu H, Gu J, Qin H, Ding W, Guo N, Fu J and Yang Y (2024) Mobile-based ecological momentary assessment and intervention: bibliometric analysis. Front. Psychiatry 15:1300739. doi: 10.3389/fpsyt.2024.1300739
Received: 23 September 2023; Accepted: 07 February 2024;
Published: 26 February 2024.
Edited by:
Uffe Kock Wiil, University of Southern Denmark, DenmarkReviewed by:
Rüdiger Christoph Pryss, Julius Maximilian University of Würzburg, GermanyNikolaos Batalas, Pieriki Energy and Informatics, Greece
Copyright © 2024 Yin, Zhu, Gu, Qin, Ding, Guo, Fu and Yang. This is an open-access article distributed under the terms of the Creative Commons Attribution License (CC BY). The use, distribution or reproduction in other forums is permitted, provided the original author(s) and the copyright owner(s) are credited and that the original publication in this journal is cited, in accordance with accepted academic practice. No use, distribution or reproduction is permitted which does not comply with these terms.
*Correspondence: Yan Yang, eWFuZzIwMjFAc2p0dS5lZHUuY24=; Jingjing Fu, cmVuamlfZmpqQDEyNi5jb20=
†These authors have contributed equally to this work and share first authorship