- 1Department of Clinical Chemistry, Faculty of Medicine and Health Technology, Tampere University, Tampere, Finland
- 2Finnish Cardiovascular Research Center Tampere, Faculty of Medicine and Health Technology, Tampere University, Tampere, Finland
- 3Department of Clinical Chemistry, Fimlab Laboratories, Tampere, Finland
- 4Molecular Epidemiology, Faculty of Medicine and Health Technology, Tampere University, Tampere, Finland
- 5Tampere University Hospital, Tampere, Finland
- 6Department of Psychology and Logopedics, Faculty of Medicine, University of Helsinki. Helsinki, Finland
- 7Helsinki University Central Hospital, Adolescent Psychiatry Outpatient Clinic, Helsinki, Finland
- 8Department of Medicine, University of Turku, Turku, Finland
- 9Division of Medicine, Turku University Hospital, Turku, Finland
- 10Department of Paediatrics, Tampere University Hospital, Faculty of Medicine and Health Technology, Tampere University, Tampere, Finland
- 11Department of Clinical Physiology, Tampere University Hospital, Tampere, Finland
- 12Research Centre of Applied and Preventive Cardiovascular Medicine, University of Turku, Turku, Finland
- 13Department of Clinical Physiology and Nuclear Medicine, Turku University Hospital, Turku, Finland
- 14Centre for Population Health Research, University of Turku and Turku University Hospital, Turku, Finland
Background: Studies have shown that cardiovascular health (CVH) is related to depression. We aimed to identify gene networks jointly associated with depressive symptoms and cardiovascular health metrics using the whole blood transcriptome.
Materials and methods: We analyzed human blood transcriptomic data to identify gene co-expression networks, termed gene modules, shared by Beck’s depression inventory (BDI-II) scores and cardiovascular health (CVH) metrics as markers of depression and cardiovascular health, respectively. The BDI-II scores were derived from Beck’s Depression Inventory, a 21-item self-report inventory that measures the characteristics and symptoms of depression. CVH metrics were defined according to the American Heart Association criteria using seven indices: smoking, diet, physical activity, body mass index (BMI), blood pressure, total cholesterol, and fasting glucose. Joint association of the modules, identified with weighted co-expression analysis, as well as the member genes of the modules with the markers of depression and CVH were tested with multivariate analysis of variance (MANOVA).
Results: We identified a gene module with 256 genes that were significantly correlated with both the BDI-II score and CVH metrics. Based on the MANOVA test results adjusted for age and sex, the module was associated with both depression and CVH markers. The three most significant member genes in the module were YOD1, RBX1, and LEPR. Genes in the module were enriched with biological pathways involved in brain diseases such as Alzheimer’s, Parkinson’s, and Huntington’s.
Conclusions: The identified gene module and its members can provide new joint biomarkers for depression and CVH.
1 Background
Depression and cardiovascular disease (CVD) are two key global public health issues with major human suffering and economic costs (1–3). Studies have shown that depression and CVD are associated (3–6). Previous studies have shown that while about 15% of patients with CVD have major depression (7), about two-thirds of patients hospitalized after acute myocardial infarction (AMI) have mild depression (8). In addition, a study by (9) suggests that early treatment for depression, before the development of symptomatic cardiovascular disease, can reduce the risk of CVD by almost half. Based on accumulating evidence, the American Heart Association (AHA) issued a statement in 2015 that clinicians should actively monitor teenage patients with depression and bipolar disorder because they have an increased risk for CVD (10). Therefore, as patients with CVD have an increased risk of developing depression (10) and people with depression have an increased risk for CVD (11), there appears to be a bidirectional relationship between the two conditions. It is, however, unclear whether CVD leads to depression or whether depression leads to CVD due to lifestyle factors that increase the risk for CVD or both. Therefore, as the two conditions can be either comorbid or multimorbid conditions (12), we refer these conditions as potential co/multimorbid conditions in the remainder of the study.
The elevated risk of CVD among people with depression could be at least partly due to lifestyle factors such as cigarette smoking, alcohol use, lack of exercise, unhealthy diet, and medications (13, 14). The biological processes involved in the pathophysiology of depression may also interfere with those linked to cardiovascular health, ultimately leading to CVD. For instance, depressed patients with high stress levels have increased glucocorticoid concentrations, which causes hyperglycemia and can eventually result in CVD (15, 16). The hypothalamic–pituitary–adrenal (HPA) axis, a stress response mechanism involving the central nervous system and endocrine system, increases the risk of CVD by promoting the development of dyslipidemia and hypertension (17). The HPA axis is also upregulated in patients with depression (18). Similarly, depletion of neurotransmitters, such as serotonin, dopamine, and norepinephrine is known to be involved in the pathophysiology of depression (19). These neurotransmitters have also been associated with CVD and its associated risk factors. For example, serotonin is associated with coronary artery disease and cardiac events (20). Dopamine plays an important role in the pathogenesis of hypertension (21). Similarly, norepinephrine acts on the vasavasorum, reducing the flow of oxygen, and ultimately resulting in hypoxia. The restricted oxygen supply plays a vital role throughout the atherosclerotic vulnerable plaque formation process (22). Inflammation, which is a significant contributor to the development and progression of atherosclerotic CVD (23), may also impair the function of these neurotransmitters (24). A chronic low-grade inflammatory response is associated with depression (25). Increased levels of inflammatory markers, such as serum C-reactive protein and interleukin-6, which play roles in CVD pathogenesis, are also known to be associated with depression (26). Beyond the factors that might account for the association between depression and CVD, the biological mechanisms underlying these potential co/multimorbid conditions seem complex and remain largely unknown. Given the burden of these conditions on patients, the development of targeted interventions for depression and CVD is crucial. This requires an understanding of the genetics and biological pathways shared by these two conditions, and the disentanglement of the bidirectional relationship between depression and CVD at the molecular level.
In the present study, we hypothesized that the complexity of the relationship between depression and cardiovascular health (CVH) can be, at least partly, explained by the underlying gene networks. Therefore, the objective of the present study was to identify transcriptomic networks jointly associated with markers of both depression and CVH using a system-level bioinformatics approach. We conducted weighted gene co-expression network analysis (WGCNA) (27) of whole blood transcriptomic data, followed by multivariate analysis of variance (MANOVA) and pathway analysis to identify gene co-expression networks, termed gene modules, enriched biological pathways in the modules, and the most important member genes jointly associated with markers of both depression and CVH. The analysis pipeline has been described in detail elsewhere (28, 29).
2 Materials and methods
2.1 Study participants
This study was based on the Young Finns Study (YFS), one of the largest prospective multicenter follow-up studies assessing cardiovascular risk factors from childhood to adulthood (30). The study began in 1980 with 3,596 children and adolescents aged 3–18 years, randomly selected from five university hospitals in Finland (Turku, Tampere, Helsinki, Kuopio, and Oulu). The participants were regularly followed for over 40 years. The study was approved by the ethics committee of the Hospital District of Southwest Finland on 20 June 2017 (ETMK:68/1801/2017). All participants provided written informed consent and the study was conducted in accordance with the Declaration of Helsinki. Data protection will be handled according to the current regulations. The present study was based on 899 participants (women: 59%), aged 34–49 years, from the 2011 follow-up (Table 1), with ideal CVH metrics as a marker of CVH, Beck’s depression inventory (BDI-II) score as a marker of depression, and transcriptomics data.
2.2 Cardiovascular health metrics
The American Heart Association (AHA) has defined ideal CVH as the simultaneous presence of four favorable health behaviors (nonsmoking, ideal body mass index (BMI), physical activity, and healthy diet) and three favorable health factors (ideal levels of total cholesterol, blood pressure, and fasting glucose) (31). We generated AHA indices for the seven corresponding health behaviors and factors. The ideal CVH metrics simply correspond to the number of ideal health factors and behaviors present during the follow-up period, as described elsewhere (32). The score ranges from 0 to 7, with seven being the best score representing ideal CVH.
2.3 Beck’s depression inventory (BDI) score
The BDI score used in this study was based on the revised version of BDI-II (33) of the original BDI (34). Like the original BDI, the BDI-II contains 21 questions measuring the characteristic attitudes and symptoms associated with depression. Each answer is scored on a scale of 0 to 3, with higher scores representing higher levels of depression. Instead of the past week, as in the original BDI, participants were asked to report how they had been feeling over the past two weeks.
2.4 Blood transcriptomics
Whole genome blood transcriptome was profiled from the RNA isolated from whole blood (2.5 ml) collected from YFS participants during the 2011 follow-up in PaXgene Blood RNA Tubes (PreAnalytix, Hombrechtikon, Switzerland). The tubes were inverted 8–10 times and stored at room temperature for at least 2 h. PaXgene tubes were frozen and stored less than one year in −80°C. After thawing, the tubes were stored at room temperature (2 h–12 h), according to the manufacturer’s instructions. RNA was then isolated using the PAXgene Blood RNA Kit (Qiagen) with DNase Set according to the manufacturer’s instructions using the QiaCube.
The concentration and purity of RNA samples were evaluated spectrophotometrically using a NanoDrop spectrophotometer (BioPhotomer, Eppendorf, Wesseling-Berzdorf, Germany). Samples were considered pure if the 260/280 ratio was in the range of 1.8–2.2. The RNA isolation process was validated by analyzing the integrity of the RNA using the RNA 6000 Nano Chip Kit (Agilent). The RNA integrity number (RIN) and shape of the electropherogram of the 26 RNA samples isolated from YFS were evaluated. Visual inspection of the shape of the electropherogram revealed no samples with signs of degradation. The average RIN value was 8.2 with a standard deviation of 0.5.
Expression levels were analyzed using the Illumina HumanHT-12 version 4 Expression BeadChip (Illumina Inc.) containing 47,231 expression and 770 control probes. Briefly, 200 ng of RNA was reverse transcribed into cDNA and biotin-UTP labeled using the Illumina TotalPrep RNA Amplification Kit (Ambion). Next, 1,500 ng of cDNA was hybridized to the Illumina HumanHT-12 v4 Expression BeadChip. BeadChips were scanned using an Illumina iScan system. Samples with fewer than 6,000 significantly detected expression probes (detection p-value <0.01) were discarded. Raw Illumina summary probe-level data were exported from Beadstudio and processed in R (http://www.r-project.org/) using nonparametric background correction, followed by quantile normalization with control and expression probes, with the neqc function in the limma package (35) and a log2 transformation. Samples with a sex mismatch between the recorded sex and predicted sex based on RPS4Y1-2 and XIST mRNA levels on the Y and X chromosomes, respectively, were excluded.
2.5 Biostatistical analysis
All statistical analyses and data processing were performed using the statistical package R version 3.6.0 (36). The overall concept of the co/multimorbidity analysis involved i) identification of gene modules with densely interconnected genes, ii) association of the identified gene modules with markers of both depression and CVH independently, iii) MANOVA test to assess the joint association of the gene modules as well as its member genes with the markers of both depression and CVH, and iv) biological interpretation of the jointly associated gene modules with pathway enrichment analysis of the genes in the modules (Figure 1). The ideal CVH metrics from YFS participants were normally distributed (Supplementary Figure S2). The BDI-II scores were log-transformed to correct for skewness (Supplementary Figure S3).
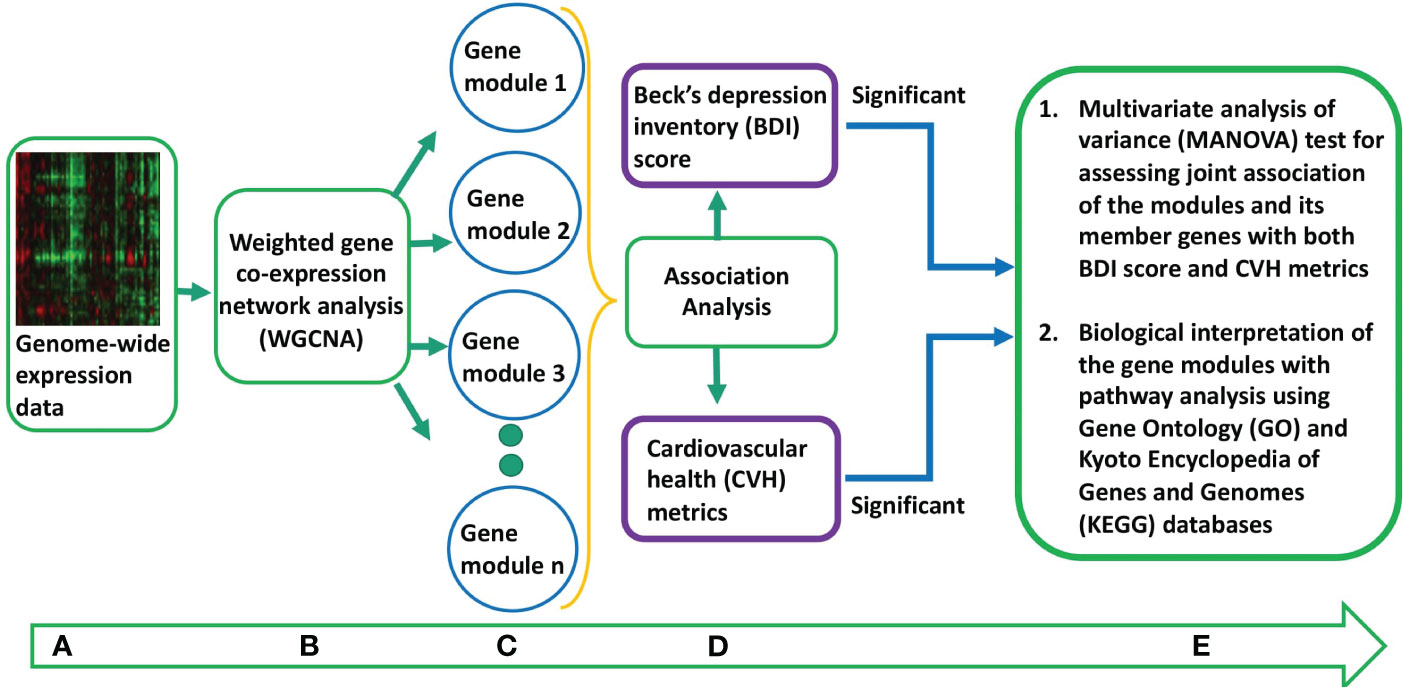
Figure 1 Overall concept of the co/multimorbidity analysis pipeline. (A) Pre-processed genome-wide expression data. (B) Weighted gene co-expression gene network analysis to identify gene co-expression networks or modules. (C) Gene modules identified from step (B). (D) Association analysis of the eigengenes of the gene modules with markers of both depression and cardiovascular health (CVH). The gene modules that are significantly associated with markers of both depression and CVH are considered as candidate gene modules for co/multimorbidity study. (E) Multivariate analysis of variance (MANOVA) test to access joint association of the candidate gene modules as well as its member genes with the markers of both depression and CVH. Biological interpretation of the jointly associated gene modules is done by pathway enrichment analysis of the genes in the modules.
Gene modules, groups of densely interconnected genes, were identified using WGCNA implemented with R statistical software (27). Gene module generation with WGCNA involved multiple steps. The first step involved the calculation of Pearson’s correlation (r) for all pairwise comparisons of genes across all participants, resulting in a correlation matrix. The correlation matrix was then raised to a power of five, chosen using the power function implemented in the WGCNA package, to generate an adjacency matrix to minimize noise and emphasize stronger correlations (Supplementary Figure S1). This approach transforms the correlation matrix into an approximately scale-free topology based on the assumption that most real-world biological networks are scale-free. Next, a topological overlap matrix (TOM), a similarity matrix of genes, was generated from the resulting adjacency matrix to incorporate network topology information into the definition of gene co-expression. TOM was transformed into a dissimilarity matrix (1-TOM). A hierarchical clustering tree of the genes was then generated by average linkage-based hierarchical clustering of the dissimilarity matrix. Gene modules from the hierarchical clusters of genes were identified using a dynamic tree-cutting algorithm. We assessed the quality of the identified gene modules by analyzing the correlation between gene significance (GS) and module membership (MM). GS is defined as the correlation between the module’s individual member gene expression levels and markers of both CVH and depression (ideal CVH metrics and BDI-II score, respectively). MM is defined as the correlation between the summary expression profile of a module (the first principal component, termed the eigengene of the module) and expression levels of its member genes. An ideal gene module is one where GS and MM are highly correlated, suggesting that the genes that are highly correlated with the studied markers are also important members of the analyzed module.
The first principal component of the expression profiles of the member genes in a module, termed the eigengene, was used as a surrogate for the summary expression profile of the module. Pearson’s correlation coefficients (r) were calculated between the eigengenes of the identified gene modules and markers of CVH and depression. Gene modules that were significantly correlated (p-value <0.05) with markers of both CVH and depression were considered candidate modules for testing the joint statistical association with the studied markers of both CVH and depression in multivariate statistical analysis. The joint association of the candidate gene modules with CVH and depression markers were tested using the MANOVA test implemented in the car R package. MANOVA was adjusted for age and sex. Pathway enrichment analysis of the genes in the significant gene modules was performed using biological process Gene Ontology (GO) terms (37) and the Kyoto Encyclopedia of Genes and Genomes (KEGG) (38) using clusterProfiler R/Bioconductor packages (39). The analysis pipeline has been described elsewhere (28).
3 Results
3.1 Study population characteristics
3.2 Association between the markers of cardiovascular health and depression
The CVH metrics had a weak but significant negative correlation (r = −0.10, p-value = 0.01) with the BDI-II score (Supplementary Figure S4).
3.3 Association between the identified gene modules and markers of cardiovascular health and depression
The dynamic tree-cutting algorithm identified 22 gene modules from the hierarchical clustering of the dissimilarity matrix, containing 14–10,367 highly correlated genes (Figure 2). Gene modules were named according to the colors assigned automatically by the WGCNA program. Among the 22 identified gene modules, 15 were associated with CVH metrics with a p-value <0.05, and only two were associated with the BDI-II score with the same p-value threshold. However, the gene module named darkred, containing 256 genes, was correlated with CVH metrics with a correlation coefficient (r) of −0.13, p-value of 6 × 10−5, and BDI-II score with r of 0.09 and p-value of 0.009. Therefore, as the darkred gene module was significantly correlated with markers of both CVH and depression, we considered it a candidate module that is potentially jointly associated with markers of both CVH and depression.
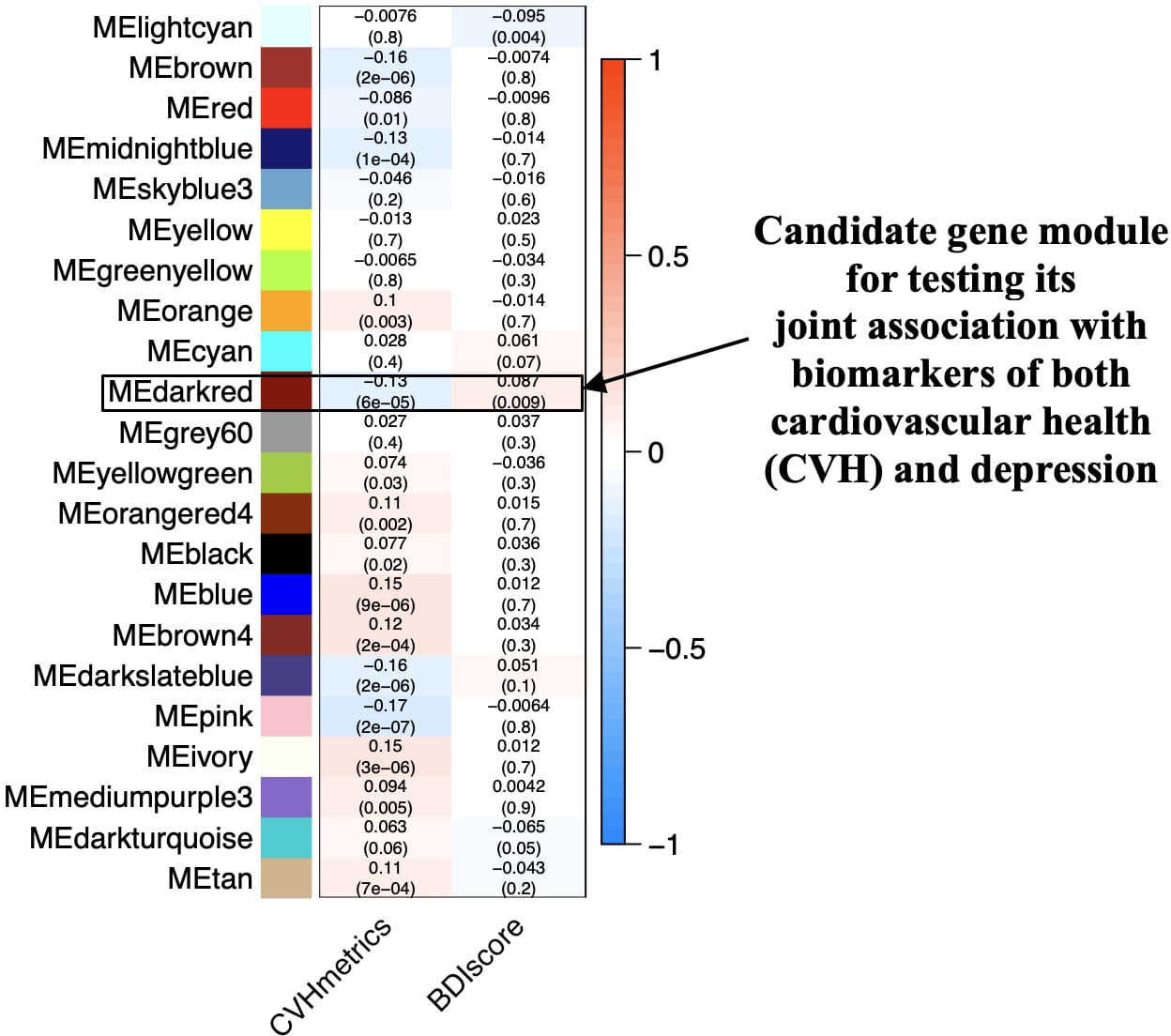
Figure 2 Relationships between gene co-expression modules (x-axis) and markers of cardiovascular health and depression (y-axis). The rows correspond to the different gene modules (named by color) and their summary expression profile; for example, MEdarkred represents the summary expression profile for the module darkred. The columns correspond to the markers of CVH (CVHmetrics) and depression (BDIscore) used in the biostatistical analyses. The values in the cells represent Pearson’s correlation coefficients (r), with the associated p-values in parentheses below the coefficient. The correlation coefficients have a color-coding shown in the color scale (between −1 and + 1) on the right side of the figure. The highlighted darkred module is significantly associated with the markers of both CVH and depression. CVHmetrics, cardiovascular health metrics; BDIscore, Beck’s depression inventory score.
3.4 Gene significance and module membership
GS is defined as the correlation between the module’s member genes and study markers. MM is defined as the correlation between an eigengene and other member genes of the module. An ideal gene module is one where GS and MM are highly correlated, suggesting that the genes that are highly correlated with the study markers are also important members of the gene module (27). The darkred gene module had a highly significant correlation between GS and MM with respect to both CVH metrics (r = 0.39, p-value = 1.0 × 10−10) and BDI-II score (r = 0.62, p-value = 1.4 × 10−28) (Figure 3).
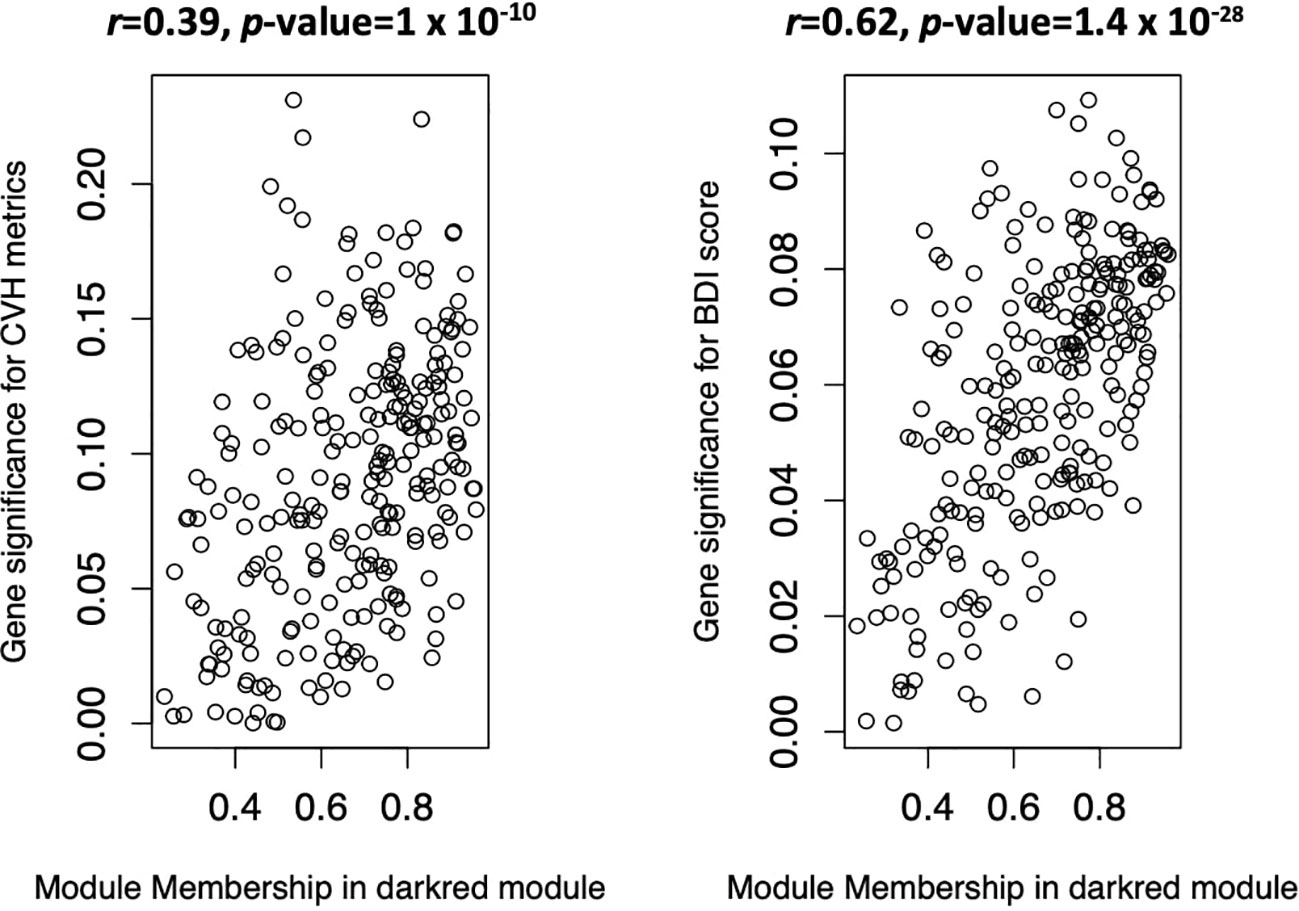
Figure 3 Scatter plots of gene significance (GS) vs module membership (MM) in the darkred gene module. The left panel corresponds to cardiovascular health (CVH) metrics and the right panel to Beck’s depression inventory score, markers of CVH and depression, respectively. CHV metrics, cardiovascular health metrics; BDI-II score, Beck’s depression inventory score.
3.5 Multivariate analysis of the candidate gene module with the markers of cardiovascular health and depression
Based on the MANOVA test adjusted for age and sex of the participants, there was a statistically significant joint association between the eigengene of the darkred gene module and markers of both CVH and depression (Pillai’s Trace = 0.02, p-value = 1.8 × 10−5). The Pillai’s trace statistic ranges from 0 to 1, with higher values indicating a higher effect of the module’s summary expression profile on the study markers.
3.6 Multivariate analysis of the member genes of the gene module jointly associated with the markers of cardiovascular health and depression
We also conducted a MANOVA test for the member genes of the dared-gene module to identify the most significant genes in the module. Ninety-five out of 256 genes had statistically significant joint associations with both CVH metrics and BDI-II scores (p.adj <0.05) (Supplementary Table S1). The 25 most significant member genes from the darkred gene module and their descriptions are presented in Table 2.
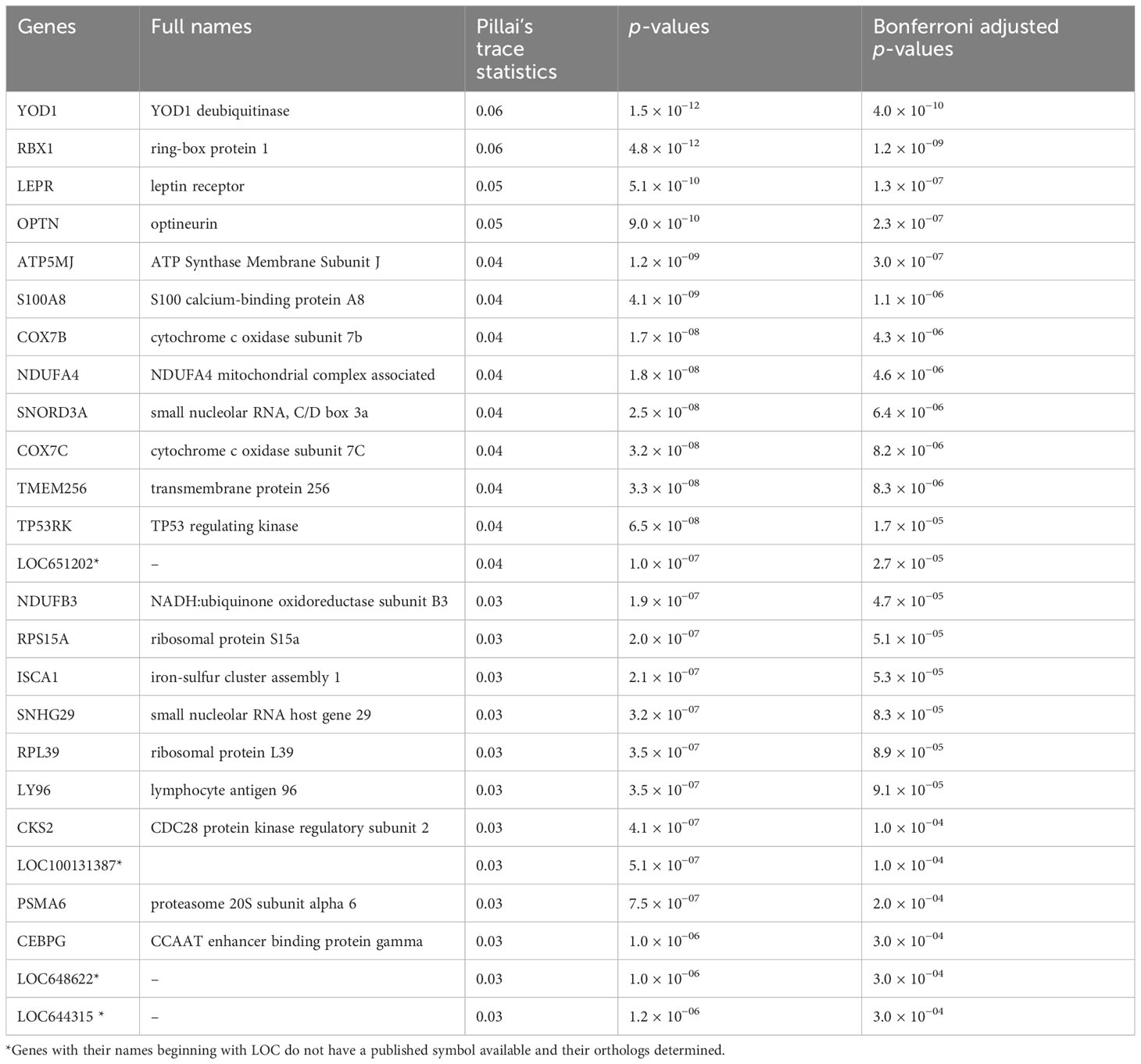
Table 2 List of the top 25 genes that are jointly associated with markers of both cardiovascular health and depression with Bonferroni adjusted p-value <0.05.
3.7 Pathway analysis of the gene module jointly associated with the markers of cardiovascular health and depression
Genes in the darkred module were enriched with seven biological pathways from the KEGG database and five biological processes from the GO database (p.adj <0.05) (Figure 4).
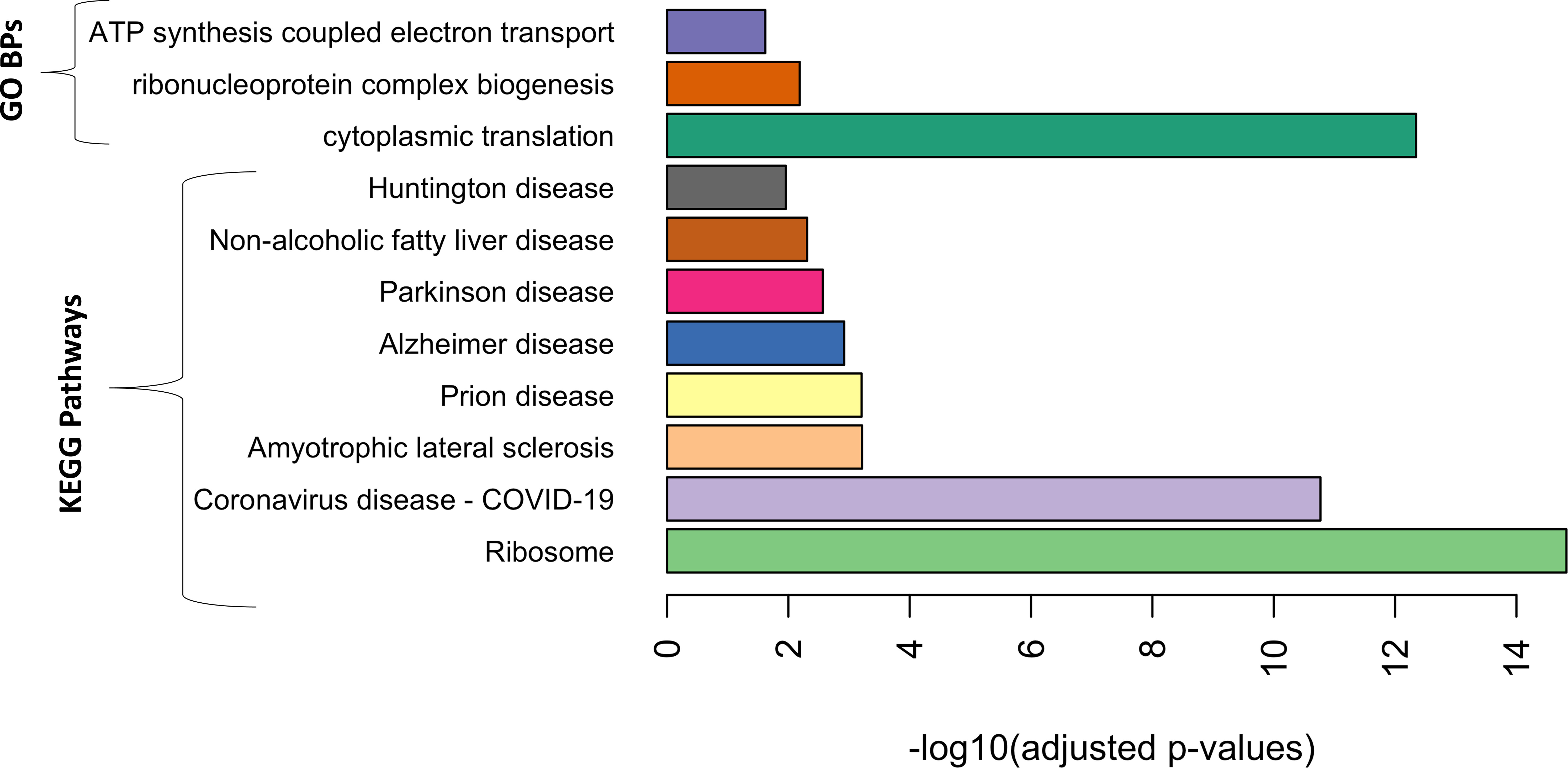
Figure 4 Gene Ontology biological processes (GO BPs) and Kyoto Encyclopedia of Genes and Genomes (KEGG) databases that were significantly enriched in the genes of the darkred gene module with adjusted p-value <0.05.
4 Discussion
We performed a system-level analysis of whole blood transcriptomic data to identify gene modules and enriched biological pathways shared by markers of depression and CVH. We identified a shared gene module that was significantly associated with markers of both depression (BDI-II score) and cardiovascular health (CVH metrics). The biological pathways enriched among the members of the module were related to several mental disorders, ATP synthesis, and protein translation.
Accumulating evidence supports the idea that depression and CVD are co/multimorbid conditions (40). Interestingly, the relationship between these two conditions is bidirectional. For example, after adjusting for all known cardiovascular risk factors in studies of sizable populations free of medical conditions, people with baseline depression were more likely to develop cardiovascular disease (41, 42). Among people who develop coronary artery disease, those with depression, even a milder form, have a significantly higher risk of cardiac morbidity and mortality. Therefore, an important question related to CVD–depression co/multimorbidity is whether addressing depression can lower the risk of developing CVD or vice versa. An in-depth understanding of the underlying molecular mechanisms of the two conditions is of paramount importance to address this question.
The biological processes and pathways enriched in the darkred gene module, identified to be jointly associated with the early markers of both diseases, have been shown in previous studies to be associated with both diseases. For example, genes involved in GO biological process, cytoplasmic translation, and KEGG pathway ribosomes play a role in protein translation. Impairment of protein translation is associated with mental disorders, including major depression (43). Similarly, GO biological process, ATP synthesis coupled electron transport was also enriched among the member genes of the identified module, which is known to regulate depressive-like behavior (44). ATP depletion also plays a role in atherosclerosis (45). Most other pathways enriched in the identified gene module were related to neurodegenerative diseases, such as Huntington’s disease, Parkinson’s disease, and Alzheimer’s disease. The prevalence of depression among patients with neurodegenerative diseases is high (46). A pathway related to non-alcoholic fatty liver disease (NAFLD) was also enriched in this module. Interestingly, a recent study showed a significantly high prevalence of depression among patients with NAFLD (47). NAFLD is an independent risk factor for atherosclerotic CVD (48).
The top three genes of the module, YOD1, RBX1, and LEPR, have been shown previously to be associated with neurodegenerative diseases, bipolar disorder, and depression (49–51). YOD1 belongs to the ubiquitin–proteasome system and plays an important role in protein metabolism. Deubiquitinases stabilize the expression of their target proteins by removing their ubiquitin chains. Alterations in the ubiquitin–proteasome system can lead to inflammation, neurodegeneration and cancer (52). Deubiquitinases are involved in cell death and inflammation (53), and both play major roles in atherosclerosis (54). Similarly, RBX1 is a component of the ubiquitin–proteasome system and is known to play a role in inflammation and neurodegeneration (52). Inflammation is associated with diabetes, obesity, and hypertension (55–57). We speculate that the significant association between the genes and CVH metrics observed in this study was driven, at least partly, by blood glucose level, body mass index, and blood pressure, which are three of the components of the CVH metrics. Therefore, this study uncovered potential genes that may explain the inflammation-based link between depression and CVH. The LEPR protein is present on the cell surfaces of numerous organs and tissues, including the hypothalamus, and regulates body temperature, sleep, mood, hunger, and thirst. LEPR is activated by the adipokine hormone leptin, which is generated and secreted by the white adipose tissue. The attachment of leptin to its receptor in the hypothalamus sets off a cascade of chemical signals that influence hunger and contribute to a sensation of fullness. Neural plasticity, a mechanism of neuronal adaption, is affected by leptin. The onset and progression of depression are influenced by modifications in neural plasticity caused by stress and other adverse stimuli (58). Elevated leptin levels are a major risk factor for atherosclerotic CVD. Leptin exerts proatherogenic effects on a variety of vascular cell types, including macrophages, endothelial cells, and smooth muscle cells via its interaction with LEPR, which is highly expressed in atherosclerotic plaques (59). To the best of our knowledge, this is the first study to identify the role of LEPR in the pathogenesis of both depression and atherosclerotic CVD. A similar transcriptomic study was recently published (60). However, unlike the study by (60), the unique feature of this study is that we focused on the identification of transcriptomic signatures that were jointly associated with markers of both depression and CVH among the same set of participants.
We speculate that the statistically significant joint association of the genes with depression and CVH metrics observed in this study could be driven by one or both of the following scenarios: i) unhealthy behavior-related components of the CVH metrics such as smoking, unhealthy diet, and lack of physical activity lead to altered expression levels of the genes, which in turn stimulate biological pathways leading to depression; ii) the genes stimulate biological pathways leading to both depression as well as the health factor-related components of the CVH metrics such as total blood cholesterol level, blood pressure, and blood glucose level. We believe that the associations observed in this study can stimulate further research for deeper understanding of the depression–CVD co/multimorbid. For instance, establishing whether the observed associations of the gene network and the genes with the markers of mental and cardiovascular health are causal requires further research involving clinical CVD and depression outcomes in multiethnic cohorts.
This study has several limitations. One limitation was the lack of a clinical CVD endpoint, as the study was based on a relatively young population cohort with an early phase of cardiovascular disease. Instead, CVH metrics have been shown to be associated with subclinical atherosclerosis (61). This study used microarray technology because, at the time of the follow-up (year 2011), RNA-Seq technology was still too expensive for a sizable epidemiological study like the one in this study. Additionally, all the participants in the study were of European descent. Therefore, further research with populations of different ethnicities, age groups, and clinical CVD endpoints is required.
It is imperative to consider depression and cardiovascular disease co/multimorbidity for the best possible care and improved clinical outcomes. However, as the current healthcare systems around the world are built to concentrate on treating specific diseases, the holistic care of individuals with both conditions is challenging. A comprehensive understanding of the joint risk factors, clinical characteristics, and molecular networks underlying these conditions is necessary to develop a holistic treatment approach for patients with these conditions, for example, by identifying key molecules such as genes that can be targeted for intervention, considering their effect on both conditions and potential side effects. The availability of high-throughput biological datasets, such as omics and sophisticated machine learning-based data science methods, makes it feasible to uncover and develop a comprehensive and shared landscape of the molecular networks underlying these conditions. This study aids in transitioning the focus of contemporary biomedical research from a single-disease framework to a spectrum of multiple diseases that may coexist and have a shared biological base.
Overall, our results from the transcriptome-wide multivariate association analysis of mental and cardiovascular health markers support the depression and CVD co/multimorbidity hypothesis. This study identified several genes and biological pathways jointly associated with markers of both conditions, shedding light on the potential molecular mechanisms underlying these two related conditions. The identified gene module and its most significant member genes can provide new joint biomarkers and therapeutic targets for depression and CVH after validation in further studies. Joint biomarkers may facilitate the development of dual-purpose preventative strategies and help predict co/multimorbid disorders. Additionally, this study highlights a system-level multivariate biostatistical approach for analyzing omics data in co/multimorbidity studies.
Data availability statement
The dataset supporting the conclusions of this article were obtained from the Cardiovascular Risk in Young Finns study which comprises health related participant data. The use of data is restricted under the regulations on professional secrecy (Act on the Openness of Government Activities, 612/1999) and on sensitive personal data (Personal Data Act, 523/1999, implementing the EU data protection directive 95/46/EC). Due to these restrictions, the data cannot be stored in public repositories or otherwise made publicly available. Data access may be permitted on a case-by-case basis upon request only. Data sharing outside the group is done in collaboration with YFS group and requires a data-sharing agreement. Investigators can submit an expression of interest to the chairman of the publication committee, Prof OR (Turku University, Finland), Prof MK (Tampere University, Finland) and Prof TL (Tampere University, Finland). Requests to access these datasets should be directed to OR, olli.raitakari@utu.fi; TL, terho.lehtimaki@tuni.fi; MK, mika.kahonen@tuni.fi.
Ethics statement
The studies involving humans were approved by the ethical committee of the Hospital District of Southwest Finland on 20 June 2017 (ETMK:68/1801/2017). The studies were conducted in accordance with the local legislation and institutional requirements. The participants provided their written informed consent to participate in this study.
Author contributions
BHM: Conceptualization, Formal analysis, Investigation, Methodology, Writing – original draft. ER: Writing – review & editing. NM: Writing – review & editing. AS: Writing – review & editing. JV: Writing – review & editing. MJ: Writing – review & editing. NHK: Writing – review & editing. MK: Writing – review & editing. OTR: Resources, Writing – review & editing. TL: Resources, Writing – review & editing. PPM: Conceptualization, Formal analysis, Investigation, Methodology, Writing – original draft.
Funding
The author(s) declare financial support was received for the research, authorship, and/or publication of this article. The Young Finns Study has been financially supported by the Academy of Finland: grants 356405, 322098, 286284, 134309 (Eye), 126925, 121584, 124282, 129378 (Salve), 117797 (Gendi), and 141071 (Skidi); the Social Insurance Institution of Finland; Competitive State Research Financing of the Expert Responsibility area of Kuopio, Tampere and Turku University Hospitals (grant X51001); Juho Vainio Foundation; Paavo Nurmi Foundation; Finnish Foundation for Cardiovascular Research; Finnish Cultural Foundation; The Sigrid Juselius Foundation; Tampere Tuberculosis Foundation; Emil Aaltonen Foundation; Yrjö Jahnsson Foundation; Signe and Ane Gyllenberg Foundation; Diabetes Research Foundation of Finnish Diabetes Association; EU Horizon 2020 (grant 755320 for TAXINOMISIS and grant 848146 for To Aition); European Research Council (grant 742927 for MULTIEPIGEN project); Tampere University Hospital Supporting Foundation; Finnish Society of Clinical Chemistry; the Cancer Foundation Finland; pBETTER4U_EU (Preventing obesity through Biologically and bEhaviorally Tailored inTERventions for you; project number: 101080117); and the Jane and Aatos Erkko Foundation. PPM was supported by the Academy of Finland (Grant number: 349708) and ER (grants: 330809, 338395).
Conflict of interest
BHM, NM, TL and PPM were affiliated with Fimlab Laboratories.
The remaining authors declare that the research was conducted in the absence of any commercial or financial relationships that could be construed as a potential conflict of interest.
Publisher’s note
All claims expressed in this article are solely those of the authors and do not necessarily represent those of their affiliated organizations, or those of the publisher, the editors and the reviewers. Any product that may be evaluated in this article, or claim that may be made by its manufacturer, is not guaranteed or endorsed by the publisher.
Supplementary material
The Supplementary Material for this article can be found online at: https://www.frontiersin.org/articles/10.3389/fpsyt.2024.1345159/full#supplementary-material
References
1. Timmis A, Vardas P, Townsend N, Torbica A, Katus H, De Smedt D, et al. European Society of Cardiology: cardiovascular disease statistics 2021. Eur Heart J. (2022) 43:377–82. doi: 10.1093/ehjqcco/qcac014
2. Wilkins E, Wilson L, Wickramasinghe K, Bhatnagar P. European cardiovascular disease statistics 2017. Eur Heart Network. (2017). Available at: http://www.ehnheart.org/images/CVD-statistics-report-August-2017.pdf
3. Hare DL, Toukhsati SR, Johansson P, Jaarsma T. Depression and cardiovascular disease: A clinical review. Eur Heart J. (2014) 35:1365–72. doi: 10.1093/eurheartj/eht462
4. Nicholson A, Kuper H, Hemingway H. Depression as an aetiologic and prognostic factor in coronary heart disease: A meta-analysis of 6362 events among 146 538 participants in 54 observational studies. Eur Heart J. (2006) 27:2763–74. doi: 10.1093/eurheartj/ehl338
5. Ahto M, Isoaho R, Puolijoki H, Vahlberg T, Kivelä SL. Stronger symptoms of depression predict high coronary heart disease mortality in older men and women. Int J Geriatr Psychiatry. (2007) 22:757–63. doi: 10.1002/gps.1735
6. Rajan S, McKee M, Rangarajan S, Bangdiwala S, Rosengren A, Gupta R, et al. Association of symptoms of depression with cardiovascular disease and mortality in low-, middle-, and high-income countries. JAMA Psychiatry. (2020) 77:1052–63. doi: 10.1001/jamapsychiatry.2020.1351
7. Colquhoun DM, Bunker SJ, Clarke DM, Glozier N, Hare DL, Hickie IB, et al. Screening, referral and treatment for depression in patients with coronary heart disease. Med J Australia. (2013) 198:483–4. doi: 10.5694/mja13.10153
8. Cay EL, Vetter N, Philip AE, Dugard P. Psychological status during recovery from an acute heart attack. J Psychosom Res. (1972) 16:425–35. doi: 10.1016/0022-3999(72)90068-2
9. Stewart JC, Perkins AJ, Callahan CM. Effect of collaborative care for depression on risk of cardiovascular events: Data from the IMPACT randomized controlled trial. Psychosom Med. (2014) 76:29–37. doi: 10.1097/PSY.0000000000000022
10. Goldstein BI, Carnethon MR, Matthews KA, McIntyre RS, Miller GE, Raghuveer G, et al. Major depressive disorder and bipolar disorder predispose youth to accelerated atherosclerosis and early cardiovascular disease. Circulation. (2015) 132:965–86. doi: 10.1161/CIR.0000000000000229
11. Lichtman JH, Froelicher ES, Blumenthal JA, Carney RM, Doering LV, Frasure-Smith N, et al. Depression as a risk factor for poor prognosis among patients with acute coronary syndrome: Systematic review and recommendations: A scientific statement from the american heart association. Circulation. (2014) 129:1350–69. doi: 10.1161/CIR.0000000000000019
12. Harrison C, Fortin M, van den Akker M, Mair F, Calderon-Larranaga A, Boland F, et al. Comorbidity versus multimorbidity: Why it matters. J Multimorbidity Comorbidity. (2021) 11. doi: 10.1177/2633556521993993
13. Holt RIG, Phillips DIW, Jameson KA, Cooper C, Dennison EM, Peveler RC. The relationship between depression, anxiety and cardiovascular disease: Findings from the hertfordshire cohort study. J Affect Disord. (2013) 150:84–90. doi: 10.1016/j.jad.2013.02.026
14. Achttien R, Van Lieshout J, Wensing M, van der Sanden MN, Staal JB. Symptoms of depression are associated with physical inactivity but not modified by gender or the presence of a cardiovascular disease; A cross-sectional study. BMC Cardiovasc Disord. (2019) 19. doi: 10.1186/s12872-019-1065-8
15. Parker KJ, Schatzberg AF, Lyons DM. Neuroendocrine aspects of hypercortisolism in major depression. Hormones Behavior. (2003) 43:60–6. doi: 10.1016/S0018-506X(02)00016-8
16. Clore JN, Thurby-Hay L. Glucocorticoid-induced hyperglycemia. Endocrine Practice. (2009) 15:469–74. doi: 10.4158/EP08331.RAR
17. Le-Ha C, Herbison CE, Beilin LJ, Burrows S, Henley DE, Lye SJ, et al. Hypothalamic-pituitary-adrenal axis activity under resting conditions and cardiovascular risk factors in adolescents. Psychoneuroendocrinology. (2016) 66:118–24. doi: 10.1016/j.psyneuen.2016.01.002
18. Keller J, Gomez R, Williams G, Lembke A, Lazzeroni L, Murphy GM, et al. HPA axis in major depression: Cortisol, clinical symptomatology and genetic variation predict cognition. Mol Psychiatry. (2017) 22:527–36. doi: 10.1038/mp.2016.120
19. Hasler G. Pathophysiology of depression: Do we have any solid evidence of interest to clinicians? World Psychiatry. (2010) 9:155–61. doi: 10.1002/j.2051-5545.2010.tb00298.x
20. Vikenes K, Farstad M, Nordrehaug JE. Serotonin is associated with coronary artery disease and cardiac events. Circulation. (1999) 100:483–9. doi: 10.1161/01.CIR.100.5.483
21. Zeng C, Jose PA. Dopamine receptors: Important antihypertensive counterbalance against hypertensive factors. Hypertension. (2011) 57:11–7. doi: 10.1161/HYPERTENSIONAHA.110.157727
22. Wang JY, Liu K, Wang YB, Deng Y--B, Sun J. Effects of norepinephrine on plaque hypoxia in atherosclerotic rabbits. Front Cardiovasc Med. (2023) 10. doi: 10.3389/fcvm.2023.1121413
23. Sorriento D, Iaccarino G. Inflammation and cardiovascular diseases: The most recent findings. Int J Mol Sci. (2019) 20:3879. doi: 10.3390/ijms20163879
24. Sobolewska-Nowak J, Wachowska K, Nowak A, Orzechowska A, Szulc A, Płaza O, et al. Exploring the heart–mind connection: unraveling the shared pathways between depression and cardiovascular diseases. Biomedicines. (2023) 11. doi: 10.3390/biomedicines11071903
25. Berk M, Williams LJ, Jacka FN, O’Neil A, Pasco JA, Moylan S, et al. So depression is an inflammatory disease, but where does the inflammation come from? BMC Med. (2013) 11. doi: 10.1186/1741-7015-11-200
26. Miller GE, Stetler CA, Carney RM, Freedland KE, Banks WA. Clinical depression and inflammatory risk markers for coronary heart disease. Am J Cardiol. (2002) 90:1279–83. doi: 10.1016/S0002-9149(02)02863-1
27. Langfelder P, Horvath S. WGCNA: An R package for weighted correlation network analysis. BMC Bioinf. (2008) 9. doi: 10.1186/1471-2105-9-559
28. Mishra BH, Mishra PP, Raitoharju E, Marttila S, Mononen N, Sievänen H, et al. Modular genome-wide gene expression architecture shared by early traits of osteoporosis and atherosclerosis in the Young Finns Study. Sci Rep. (2021) 11. doi: 10.1038/s41598-021-86536-0
29. Mishra BH, Mishra PP, Mononen N, Hilvo M, Sievänen H, Juonala M, et al. Lipidomic architecture shared by subclinical markers of osteoporosis and atherosclerosis: The Cardiovascular Risk in Young Finns Study. Bone. (2020) 131. doi: 10.1016/j.bone.2019.115160
30. Raitakari OT, Juonala M, Rönnemaa T, Keltikangas-Järvinen L, Räsänen L, Pietikäinen M, et al. Cohort profile: The cardiovascular risk in young Finns study. Int J Epidemiol. (2008) 37:1220–6. doi: 10.1093/ije/dym225
31. Lloyd-Jones DM, Hong Y, Labarthe D, Mozaffarian D, Appel LJ, Van Horn L, et al. Defining and setting national goals for cardiovascular health promotion and disease reduction. Circulation. (2010) 121:586–613. doi: 10.1161/CIRCULATIONAHA.109.192703
32. Aatola H, Hutri-Kähönen N, Juonala M, Laitinen TT, Pahkala K, Mikkilä V, et al. Prospective relationship of change in ideal cardiovascular health status and arterial stiffness: the Cardiovascular Risk in Young Finns Study. J Am Heart Assoc. (2014) 3. doi: 10.1161/JAHA.113.000532
33. Beck AT, Steer RA, Ball R, Ranieri WF. Comparison of Beck depression inventories -IA and -II in psychiatric outpatients. J Pers Assess. (1996) 67:588–97. doi: 10.1207/s15327752jpa6703_13
34. Beck AT, Ward CH, Mendelson M, Mock J, Erbaugh J. An inventory for measuring depression. Arch Gen Psychiatry. (1961) 4:561–71. doi: 10.1001/archpsyc.1961.01710120031004
35. Smyth GK. Limma: linear models for microarray data. In Bioinformatics and Computational Biology Solutions Using R and Bioconductor (pp. 397–420). Springer. doi: 10.1007/0-387-29362-0_23
36. R Core Team. R Core Team 2021 R: A language and environment for statistical computing Vol. 2. R foundation for statistical computing (2022). Available at: https://www.R-project.org/. R Foundation for Statistical Computing.
37. Ashburner M, Ball CA, Blake JA, Botstein D, Butler H, Cherry JM, et al. Gene ontology: Tool for the unification of biology. Nat Genet. (2000) 25:25–9. doi: 10.1038/75556
38. Ogata H, Goto S, Sato K, Fujibuchi W, Bono H, Kanehisa M. KEGG: Kyoto encyclopedia of genes and genomes. Nucleic Acids Res. (1999) 27:29–34. doi: 10.1093/nar/27.1.29
39. Yu G, Wang LG, Han Y, He QY. ClusterProfiler: An R package for comparing biological themes among gene clusters. OMICS. (2012) 16. doi: 10.1089/omi.2011.0118
40. Wulsin LR, Singal BM. Do depressive symptoms increase the risk for the onset of coronary disease? A systematic quantitative review. Psychosomatic Med. (2003) 65:201–10. doi: 10.1097/01.PSY.0000058371.50240.E3
41. Bush DE, Ziegelstein RC, Tayback M, Richter D, Stevens S, Zahalsky H, et al. Even minimal symptoms of depression increase mortality risk after acute myocardial infarction. Am J Cardiol. (2001) 88:337–41. doi: 10.1016/S0002-9149(01)01675-7
42. Lespérance F, Frasure-Smith N, Talajic M, Bourassa MG. Five-year risk of cardiac mortality in relation to initial severity and one-year changes in depression symptoms after myocardial infarction. Circulation. (2002) 105:1049–53. doi: 10.1161/hc0902.104707
43. Laguesse S, Ron D. Protein translation and psychiatric disorders. Neuroscientist. (2020) 26. doi: 10.1177/1073858419853236
44. Lin S, Huang L, Luo Z-c, Li X, Jin S-y, Du Z-j, et al. The ATP level in the medial prefrontal cortex regulates depressive-like behavior via the medial prefrontal cortex-lateral Habenula pathway. Biol Psychiatry. (2022) 92:179–92. doi: 10.1016/j.biopsych.2022.02.014
45. Ekstrand M, Widell E, Hammar A, Akyürek LM, Johansson M, Fagerberg B, et al. Depletion of ATP and glucose in advanced human atherosclerotic plaques. PloS One. (2017) 12. doi: 10.1371/journal.pone.0178877
46. Baquero M. Depressive symptoms in neurodegenerative diseases. World J Clin Cases. (2015) 3:682–93. doi: 10.12998/wjcc.v3.i8.682
47. Xiao J, Lim LKE, Ng CH, Tan DJH, Lim WH, Ho CSH, et al. Is fatty liver associated with depression? A meta-analysis and systematic review on the prevalence, risk factors, and outcomes of depression and non-alcoholic fatty liver disease. Front Med. (2021) 8. doi: 10.3389/fmed.2021.691696
48. Finney AC, Das S, Kumar D, McKinney MP, Cai B, Yurdagul A, et al. The interplay between nonalcoholic fatty liver disease and atherosclerotic cardiovascular disease. Front Cardiovasc Med. (2023) 10. doi: 10.3389/fcvm
49. Bello AI, Goswami R, Brown SL, Costanzo K, Shores T, Allan S, et al. Deubiquitinases in neurodegeneration. Cells. (2022) 11. doi: 10.3390/cells11030556
50. Schubert KO, Thalamuthu A, Amare AT, Frank J, Streit F, Adl M, et al. Combining schizophrenia and depression polygenic risk scores improves the genetic prediction of lithium response in bipolar disorder patients. Transl Psychiatry. (2021) 11. doi: 10.1038/s41398-021-01702-2
51. Strawbridge R, Young AH, Cleare AJ. Biomarkers for depression: Recent insights, current challenges and future prospects. Neuropsychiatr Dis Treat. (2017) 13:1245–62. doi: 10.2147/NDT.S114542
52. Chen D, Dou QP. The ubiquitin-proteasome system as a prospective molecular target for cancer treatment and prevention. Curr. Protein Pept. Sci. (2010) 11(6):459–70. doi: 10.2174/138920310791824057
53. Newton K, Gitlin AD. Deubiquitinases in cell death and inflammation. Biochem J. (2022) 479:1103–19. doi: 10.1042/BCJ20210735
54. Li M, Wang ZW, Fang LJ, Cheng SQ, Wang X, Liu NF. Programmed cell death in atherosclerosis and vascular calcification. Cell Death Disease. (2022) 13. doi: 10.1038/s41419-022-04923-5
55. Tsalamandris S, Antonopoulos AS, Oikonomou E, Papamikroulis G-a, Vogiatzi G. Risk factors and cardiovascular disease prevention the role of inflammation in diabetes : current concepts and future perspectives. Eur Cardiol Review. (2019) 14:50–9. doi: 10.15420/ecr.2018.33.1
56. Hildebrandt X, Ibrahim M, Peltzer N. Cell death and inflammation during obesity: “Know my methods, WAT(son).” Cell Death Differentiation. (2023) 30:279–92. doi: 10.1038/s41418-022-01062-4
57. De Miguel C, Rudemiller NP, Abais JM, Mattson DL. Inflammation and hypertension: new understandings and potential therapeutic targets. Curr Hypertension Rep. (2015) 17. doi: 10.1007/s11906-014-0507-z
58. Ge T, Fan J, Yang W, Cui R, Li B. Leptin in depression: a potential therapeutic target. Cell Death Dis. (2018) 9. doi: 10.1038/s41419-018-1129-1
59. Raman P, Khanal S. Leptin in atherosclerosis: Focus on macrophages, endothelial and smooth muscle cells. Int J Mol Sci. (2021) 22. doi: 10.3390/ijms22115446
60. Huang P, Yan L, Li Z, Zhao S, Feng Y, Zeng J, et al. Potential shared gene signatures and molecular mechanisms between atherosclerosis and depression: Evidence from transcriptome data. Comput Biol Med. (2023) 152. doi: 10.1016/j.compbiomed.2022.106450
Keywords: depressive symptoms, cardiovascular health, comorbidity, multimorbidity, transcriptome, gene networks
Citation: Mishra BH, Raitoharju E, Mononen N, Saarinen A, Viikari J, Juonala M, Hutri-Kähönen N, Kähönen M, Raitakari OT, Lehtimäki T and Mishra PP (2024) Identification of gene networks jointly associated with depressive symptoms and cardiovascular health metrics using whole blood transcriptome in the Young Finns Study. Front. Psychiatry 15:1345159. doi: 10.3389/fpsyt.2024.1345159
Received: 27 November 2023; Accepted: 12 February 2024;
Published: 25 April 2024.
Edited by:
Ming D. Li, Zhejiang University, ChinaReviewed by:
Masaru Tanaka, University of Szeged (ELKH-SZTE), HungaryDavid P. Herzog, Johannes Gutenberg University Mainz, Germany
Copyright © 2024 Mishra, Raitoharju, Mononen, Saarinen, Viikari, Juonala, Hutri-Kähönen, Kähönen, Raitakari, Lehtimäki and Mishra. This is an open-access article distributed under the terms of the Creative Commons Attribution License (CC BY). The use, distribution or reproduction in other forums is permitted, provided the original author(s) and the copyright owner(s) are credited and that the original publication in this journal is cited, in accordance with accepted academic practice. No use, distribution or reproduction is permitted which does not comply with these terms.
*Correspondence: Binisha H. Mishra, binisha.hamalmishra@tuni.fi