- Department of Surgical Sciences, Functional Pharmacology and Neuroscience, Uppsala University, Uppsala, Sweden
Introduction: Depression is a major global burden with unclear pathophysiology and poor treatment outcomes. Diagnosis of depression continues to rely primarily on behavioral rather than biological methods. Investigating tools that might aid in diagnosing and treating early-onset depression is essential for improving the prognosis of the disease course. While there is increasing evidence of possible biomarkers in adult depression, studies investigating this subject in adolescents are lacking.
Methods: In the current study, we analyzed protein levels in 461 adolescents assessed for depression using the Development and Well-Being Assessment (DAWBA) questionnaire as part of the domestic Psychiatric Health in Adolescent Study conducted in Uppsala, Sweden. We used the Proseek Multiplex Neuro Exploratory panel with Proximity Extension Assay technology provided by Olink Bioscience, followed by transcriptome analyses for the genes corresponding to the significant proteins, using four publicly available cohorts.
Results: We identified a total of seven proteins showing different levels between DAWBA risk groups at nominal significance, including RBKS, CRADD, ASGR1, HMOX2, PPP3R1, CD63, and PMVK. Transcriptomic analyses for these genes showed nominally significant replication of PPP3R1 in two of four cohorts including whole blood and prefrontal cortex, while ASGR1 and CD63 were replicated in only one cohort.
Discussion: Our study on adolescent depression revealed protein-level and transcriptomic differences, particularly in PPP3R1, pointing to the involvement of the calcineurin pathway in depression. Our findings regarding PPP3R1 also support the role of the prefrontal cortex in depression and reinforce the significance of investigating prefrontal cortex-related mechanisms in depression.
Highlights
• 92 proteins were analyzed in blood from adolescents assessed for depression using DAWBA score.
• Seven proteins showing different levels between DAWBA risk groups were identified.
• Transcriptomic analyses showed nominally significant replication of PPP3R1, ASGR1 and CD63.
• Platelets may be a peripheral key player mirroring the condition of neurons in the CNS.
Introduction
Depression is a prominent global cause of disability with a lifetime prevalence approaching 20% (1, 2). It can manifest at any stage of life, with the most common period for the first episode typically spanning from adolescence to middle age (1). In its most severe form, depression may lead to suicide attempts, making it one of the leading causes of death among adolescents in Europe (3). It appears to be a multifactorial disease arising from a combination of genetic or metabolic predisposition and environmental factors such as stressful life events and other stressors (4). Currently, the diagnosis of depression relies on clinical assessment, which includes evaluating depressive symptoms like low mood, reduced self-esteem, inappropriate guilt, thoughts of death and suicide, decreased concentration, loss of interest or pleasure in once enjoyable activities, and disturbances in sleep and appetite. Treatment typically involves antidepressant medication, although its efficacy is generally considered mild to moderate (5), with up to 60% of patients experience resistance to treatment (6). Despite major efforts to uncover the pathophysiological mechanisms of depression by analyzing data from genome-wide association studies (7) and RNA gene expression studies (8, 9), our understanding remains limited.
Recent technological advances, particularly within proteomic-based platforms, are providing new insights for better comprehension of depression, going beyond what conventional targeted methods can offer. With the advent of cutting-edge proteomic and transcriptomic techniques allowing simultaneous quantitative assessment of a wide spectrum of proteins or mRNA transcripts, it is now possible to explore not only the functions of individual proteins but also the roles of biochemical pathways and associated metabolites. Proteins serve as the ultimate products of RNA and DNA, often serving as the functional and modifiable units in disease mechanisms. Consequently, proteome analyses hold the potential to elucidate the pathophysiological mechanisms of depression and to identify potential biomarkers for diagnosis, treatment, and monitoring of disease progression. Studies aimed at discovering objective blood-based biomarkers in the fields of psychiatry, neurology, and neuropsychiatry have experienced exponential growth (10–12). However, due to both methodological and clinical challenges associated with psychiatric conditions, such as their inherent complexity and heterogeneous presentation, clinical efficacy remains extremely limited (13–15).
Most studies on risk factors for depression have primarily focused on the adult population. While various biological mechanisms have been explored, no biomarker has been definitively identified or validated for assessing the risk or presence of depression in adolescence and young adulthood (16–20). This is important given that approximately half of depression diagnoses in adults stem from onset in adolescence. Considering the high incidence of depression during the early decades of life and its chronicity throughout life, adolescence presents a unique opportunity to develop effective prevention strategies and alleviate the burden associated with it (21). Furthermore, more research on depression involving youth populations is essential for understanding the natural progression of the disease and identifying factors that may underlie the commonly observed differences in treatment outcomes between adults and children (22). Additionally, developing biomarkers that could predict antidepressant response in the young population would contribute to creating more personalized and effective treatments for young people, a key step in improving the prognosis of depression across the lifespan (23).
In this study, we aimed to analyze protein levels in 461 adolescents assessed for depression as part of the domestic Psychiatric Health in Adolescent Study (PSY cohort) conducted in Uppsala, Sweden, using the Proseek Multiplex Neuro Exploratory panel with Proximity Extension Assay (PEA) technology provided by Olink Bioscience. Subsequently, we conducted transcriptome analyses for the genes corresponding to the significant proteins, using publicly available cohorts, in order to identify differentially expressed transcripts between depressed individuals and controls.
Materials and methods
Ethics declarations
This study uses samples from four human sample cohorts other than PSY published prior to the current study. The PSY cohort was conducted in Uppsala, Sweden and approved by the Regional Ethics Committee of Uppsala. All participants gave their written informed consent for participation in the study. Data from the other four cohorts GSE53987, GSE98793, GSE46743, and GSE64930 was deposited in the Gene Expression Omnibus (GEO) portal in the pseudo-anonymized form. The studies deposited in GEO were approved by corresponding regional ethical committees as detailed in the previous publications (24–27).
PSY subjects
The PSY cohort consists of two phases, including the recruitment phase and the follow-up after approximately one year. The objective of the study was to investigate associations between whole blood biomarkers and different psychiatric phenotypes among school students aged on average between 15-21 years. The phenotypic characterization of the individuals was performed during the visit. Participants self-reported their medications, whereas weight and height were measured. The depressive status of participants was evaluated with a computer-based DAWBA questionnaire (28, 29). The depression band of the questionnaire was used for psychiatric assessment. This band is a numeric score ranging from 0 to 5, where each number corresponds to a probability that an individual has depression: 0 (<0.1%), 1 (~0.5%), 2 (~3%), 3 (~15%), 4 (~50%), and 5 (>70%). We stratified the cohort into high depression risk and low depression risk groups based on the DAWBA score, and individuals with scores 4 and 5 were classified as being depressed. In total, 461 participants successfully completed the DAWBA assessment and had available whole blood and were therefore included in the current study. Among these participants, 334 clearly indicated medications taken, whereas 127 did not fill in the information.
Proximity extension assay
Protein levels from the 461 participants were assessed using Olink Neuro Exploratory Panel involving 92 proteins (Neuro Exploratory Panel of Olink Proteomics AB, Uppsala Sweden) in two batches. The Neuro Exploratory panel was specifically selected due to the exploratory nature of the current study. This panel was deemed suitable because it offers a broad range of neurological biomarkers, allowing for a proteomic exploration of potential pathways. The Olink methodology has been extensively described in the user manual on the company’s website. Briefly, a mixture of 1 µL of EDTA-containing plasma and a 3-µL incubation mix was incubated overnight at 8°C. Then a 96-μL extension mix with PEA enzyme and PCR reagents was added and incubated at room temperature for 5 min. Afterwards, an extension reaction in a thermal cycler was performed followed by 17 cycles of DNA amplification. During these steps, 92 oligonucleotide-labeled pairs of antibodies were allowed to bind to their respective target protein in the sample. Once the antibody probes bound to the targeted protein and the attached DNA oligonucleotides were in close proximity, the oligonucleotides hybridized and were extended by enzymatic polymerization. The oligonucleotide templates were then amplified and quantified using real-time polymerase chain reaction. Protein levels are expressed as Normalized Protein Expression (NPX) values on log2-scale (30). The assay procedures were performed at Affinity Proteomics Uppsala SciLifeLab in Sweden.
Transcriptome validation cohorts
The validation of the results from the PSY cohort was performed utilizing a publicly-available transcriptome cohort with post-mortem brain tissues (GSE53987) and three cohorts with the whole blood (GSE98793, GSE46743, and GSE64930). The cohort GSE53987 comprises post-mortem brain sample data for major depressive disorder (MDD), schizophrenia, bipolar disorder, and controls. Descriptions of sample collection and preparation are provided in the original publication (27). Transcriptome data from three brain tissues was available and included the associative striatum, hippocampus, and prefrontal cortex (Brodmann area 46) for each of the disorders and controls. We compared transcriptome profiles of MDD patients (n = 16 – 17) versus controls (n = 18 – 19) separately for each of the three brain tissues.
The cohort GSE98793 included 192 participants and is a part of GlaxoSmithKline–High-Throughput Disease-specific target Identification Program. The sample includes 64 individuals with MDD, 64 participants with comorbid MDD and anxiety, and 64 healthy controls. Dataset includes covariables on age, sex, diagnoses (anxiety and MDD), as well as a batch covariable. Please refer to the initial publication for detailed descriptions (26).
The cohort GSE46743 is a part of a large-scale study conducted at the Max Planck Institute of Psychiatry, Munich, Germany. The study objective was to investigate transcriptome reaction to stress in the context of depression. The study investigated whole blood transcriptome profiles before and after exposure to dexamethasone and involved 160 male subjects. Data on age and BMI was collected during the study. Transcriptome profiling was performed with Illumina HumanHT-12 expression beadchip. Further information could be found elsewhere (24). In the present work, we used transcriptome profiles before exposure to dexamethasone to compare the baseline expression in depression patients versus controls.
Lastly, the cohort GSE64930 had a similar design to GSE46743 and investigated transcriptome reaction to stress in the context of depression. This cohort has a partial overlap consisting of 79 participants with GSE46743. However, it included both female and male participants (n=289) (25). The initial deposited phenotypic data contains information only on participant’s sex. Data on age, BMI, RNA integrity number, and Hamilton Depression Rating Scale (HAM-D) scores, as well as three surrogate variables to adjust for cell heterogeneity, was provided by the study investigator after a request. Similar to GSE46743, we used the data before dexamethasone exposure to compare expression in depressed participants versus controls. In total, 286 participants were amenable for analysis as three samples did not have information on RNA integrity and were excluded.
Data preprocessing
Transcriptome data preparation and analysis were performed in the R programming language environment (version 4.2.0). Data for GSE98793 and GSE53987 was available in the form of raw CEL files. The R package affy was used to perform background correction, normalization, and summarization (31). The initial array images were inspected for potential visual artifacts. The initial data had substantial variation due to batching. Data preparation was performed in the expresso framework (affy), where all data curation steps are done sequentially. The background was corrected with a robust multi-array average method. The resulting log2-transformed values were quantile normalized and probe-wise correction was based exclusively on the perfect match intensities (“pmonly”). The resulting expression values were obtained with the “medianpolish” procedure. The final correction for batching was performed with the function ComBat from the R package sva (32). The batch correction was performed with covariates and included subject group, anxiety status, gender, and age to preserve corresponding biological effects.
In the cohorts GSE46743 and GSE64930, we used already preprocessed filtered data. Expression values corrected for background that passed through variance stabilization and normalization (VSN) procedure were downloaded directly from the GEO from the corresponding records.
Data for the PSY cohort comes in the form of analyzable normalized NPX values. We performed additional correction for the batch effect (plate) using the function ComBat from the R package sva. The batch correction was performed with covariates that included DAWBA risk group, age, sex, as well as the antidepressant intake (binary). The antidepressant covariate was only included for the samples subject to differential expression analysis adjusted for antidepressant intake.
Statistical analysis and data visualization
Linear regression models (R implementation) were used to investigate associations between protein/transcript expression and the depression status in all cohorts. The choice of model covariates was based on the available data and biological relevance. In each model, the level of protein/transcript was regressed against the depression status or DAWBA risk group (binary) adjusted for covariates. In the PSY cohort, the covariates included age (numeric) and sex (binary). In the analysis adjusted for antidepressant intake, the antidepressant covariate (binary) was added. The analysis adjusted for antidepressant intake was performed as a “complete case” and all participants that did not report the data on antidepressant intake were excluded. In the GSE53987 cohort, the covariates were sex (binary), ethnic background (binary), age (numeric), tissue pH (numeric), post-mortem interval (numeric), and RNA integrity number (numeric). In the GSE53987 cohort, the covariates included anxiety comorbidity (binary), sex (binary), age (numeric). In the GSE46743 cohort, the only covariate included was age (numeric) as all participants were male. Lastly, sex (binary), age (numeric), RNA integrity number (numeric), and three surrogate variables (numeric) were used as covariates in GSE64930 cohort. A two-tailed nominal p < 0.05 was considered significant and only results reproduced in at least one cohort were deemed as relevant. The Bonferroni-adjusted p-values were calculated along the nominal statistics. Data was visualized using custom R scripts with R packages ggplot2 and visNetwork.
Results
Demographic data
The demographic details of the participants in the PSY cohort are presented in Table 1. The initial dataset included 461 participants, of whom 138 had available proteomic data from recruitment, and 353 had proteomic data from the follow-up. After conducting quality control steps in connection with the proteomic analysis, five samples from the screening and four samples from the recall had quality control warnings and were subsequently removed from the analyses. Out of the 461 participants, 61 were in the high-risk DAWBA group, of which the majority (91.8%) were females. The age was similar in both the high and low-risk DAWBA groups (mean age 16.67 vs. 17.26 years). While five participants in the high-risk DAWBA group reported taking antidepressants, only nine participants reported taking antidepressants in the low-risk DAWBA group. Thus, 121 participants (> 30%) did not answer the drug intake question in the low-risk DAWBA group.
The demographic details of the participants in each of the four publicly available transcriptome validation cohorts are presented in Supplementary Tables S1–4. Each cohort was stratified into two groups based on depression diagnosis, depressed or non-depressed (see methods). The cohort GSE53987 was nearly identical in all characteristics between the depressed and non-depressed groups (Supplementary Table S1). In GSE98793, there was no difference between groups except for a relatively higher proportion of females than males (75% vs. 25%) in both the depressed and non-depressed groups (Supplementary Table S2). In the GSE46743 cohort, the mean age of participants was relatively higher in the depressed group (48.39 vs. 40.18) than in the non-depressed group (Supplementary Table S3). Lastly, for the GSE64930 cohort, there was a higher proportion of males than females (64.3% vs. 35.7%) in the depressed group and (71.9% vs. 28.1%) in the non-depressed group, while the mean age was higher in the depressed group (48.06 vs. 38.55) than in the non-depressed group (Supplementary Table S4).
Differential expression (proteome) analyses in PSY cohort
Out of the 92 protein biomarkers measured using the Proseek Multiplex Neuro Exploratory panel, only 43 proteins were detected in more than 75% of blood samples. Therefore, 49 proteins were excluded from the analysis. We conducted linear regression models, adjusted for age and sex, and both with and without adjustment for antidepressants, to investigate the differences in protein levels between high-risk and low-risk DAWBA participants. Five proteins (RBKS, CRADD, ASGR1, HMOX2, and PPP3R1) showed different levels between DAWBA risk groups at a nominal significance in the linear models non-adjusted for antidepressants (Supplementary Table S5). After adjustment for antidepressants, only three of these proteins (RBKS, CRADD, and PPP3R1) showed nominally significant differences in levels (Supplementary Table S6). The estimated effect sizes of proteins in the depressant-adjusted analysis were slightly larger than in the non-adjusted models for antidepressant intake. A summary of the main results obtained in the PSY cohort and their replications in the transcriptome cohorts is shown in Figure 1.
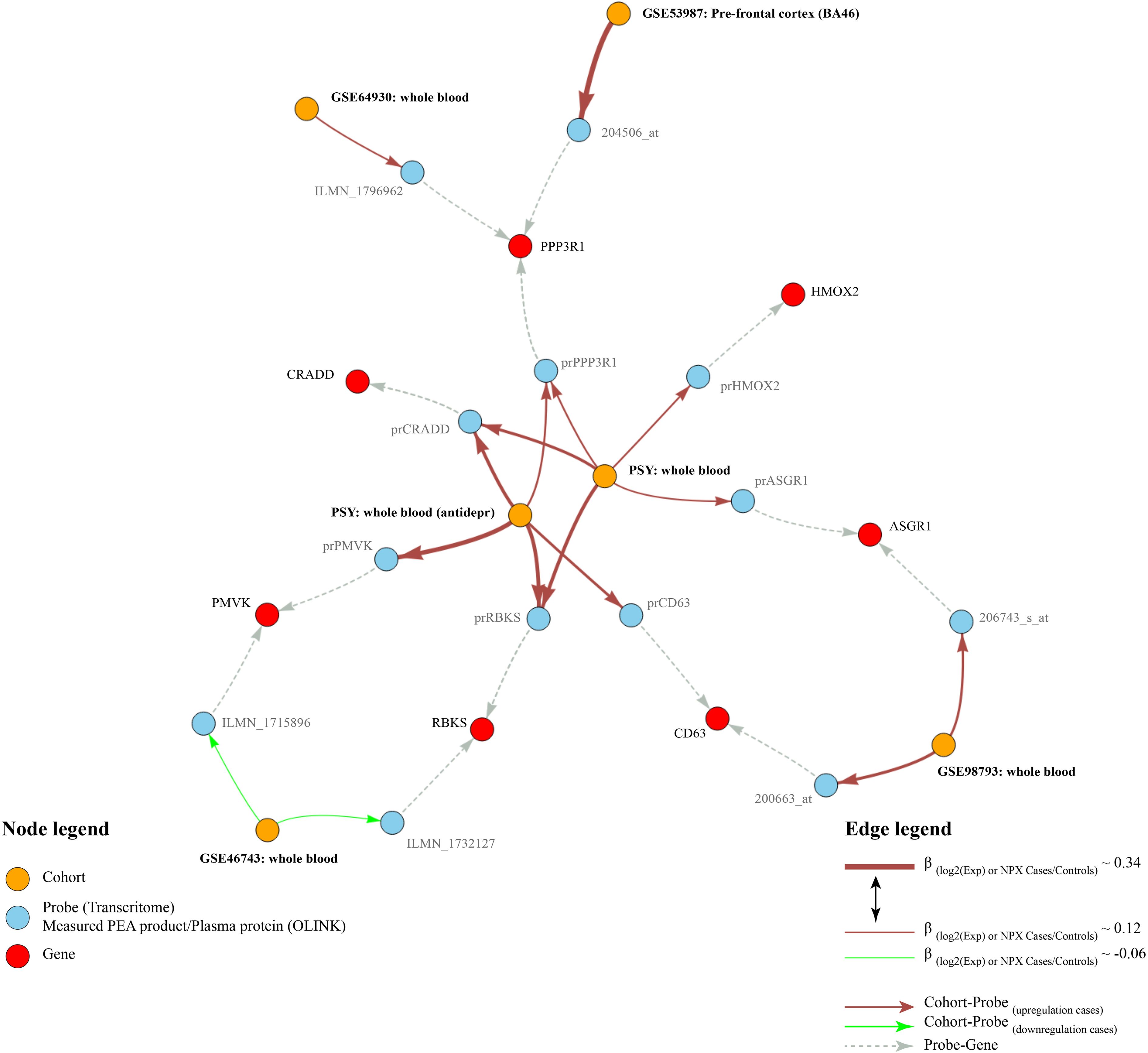
Figure 1 Results graph. This figure shows the main results obtained in the PSY cohort and their replications in the transcriptome cohorts. Red nodes indicate differentially expressed genes, blue nodes indicate probes or plasma proteins, orange nodes indicate the analyses. Arrows indicate relationships between the nodes. Brick-red edges indicate upregulation of the protein/probe in depression, whereas green edges indicate respective downregulation. Gray edges show relationships between gene names and probes. The thickness of the red and green edges edges is proportional to the sizes of β-coefficients in the linear models.
Transcriptome analyses in independent validation cohorts
Transcriptome analyses were conducted for the genes corresponding to the nominally significant proteins using four publicly available cohorts to investigate differentially expressed transcripts. Our differential expression analyses of gene-related probes showed nominal upregulation in depression for PPP3R1, which was replicated in both GSE53987 (prefrontal cortex) (Supplementary Table S7) and GSE64930 (whole blood) (Supplementary Table S8), consistent with the upregulation observed in the PSY cohort. No transcripts were differentially expressed in the hippocampus or associative striatum in GSE53987 (Supplementary Table S7). A summary of the expression levels of PPP3R1 at the proteomic level in the PSY cohort and the transcriptomic level in GSE53987 and GSE64930 is shown in Figure 2, while Supplementary Figure S1 displays PPP3R1 expression across cohorts for better visualization of effect sizes. Moreover, we also observed nominal significance for ASGR1 and Bonferroni-adjusted significance for CD63, both of which were upregulated in depressed cases in GSE98793, aligning with the direction of upregulation observed in the PSY cohort (Supplementary Table S9). Lastly, PMVK and RBKS were nominally significant in GSE46743, but the directions did not match with the PSY cohort (Supplementary Table S10).
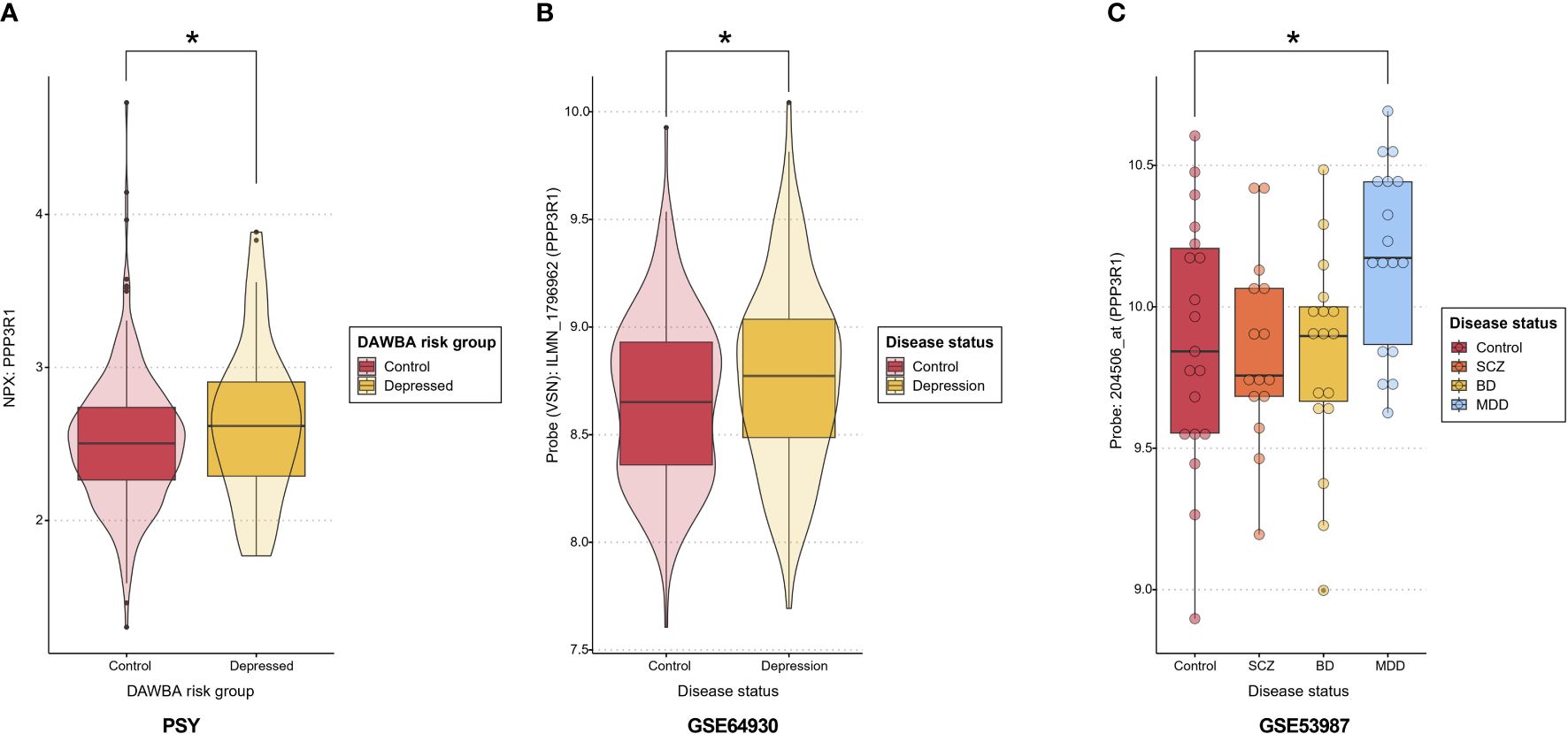
Figure 2 PPP3R1 expression. (A) PPP3R1 plasma NPX in the PSY cohort depending on the DAWBA risk group. Asterisk indicates a nominal p<0.05. (B) The expression levels of PPP3R1-related probe ILMN_1796962 in whole blood in depression versus controls in GSE64930. Asterisk indicates a nominal p<0.05. (C) The expression levels of PPP3R1-related probe 204506_at in prefrontal cortex in several psychiatric conditions and controls in the dataset GSE53987. Asterisk indicates a nominal p<0.05. DAWBA, Development and Well-Being Assessment; NPX, Normalized Protein Expression; SCZ, schizophrenia; BD, bipolar disorder; MDD, major depressive disorder.
Discussion
In this exploratory study, the Olink Neuro Exploratory panel was used to analyze the expression levels of 92 proteins among adolescents based on their DAWBA risk in the PSY cohort, followed by transcriptome analyses in independent cohorts. As a result, we identified a total of seven proteins showing different levels between DAWBA risk groups at nominal significance, whether adjusted or non-adjusted for antidepressant use, including RBKS, CRADD, ASGR1, HMOX2, PPP3R1, CD63, PMVK. Transcriptomic analyses for these genes showed nominally significant replication of PPP3R1 in two of four cohorts, while ASGR1 and CD63 were replicated in only one cohort.
One protein that drew particular attention due to its differential expression is PPP3R1, as it showed nominal significance both before and after adjustment for antidepressant use, and it was the only protein with consistent directions of change in two transcriptome cohorts. PPP3R1 is a 19 kDa calcium-binding regulatory subunit of the calcium-dependent protein phosphatase, also known as calcineurin. Abundantly expressed in human brain neuronal cell cytosol, PPP3R1 plays a critical role in the calcineurin signaling pathway (33, 34). Studies have observed that mice with a PPP3R1 mutation, eliminating calcineurin activity in somatic cells, exhibited structural defects in anterior neural structures (35). There is increasing evidence suggesting that calcineurin signaling is implicated in the pathophysiology of depression and its treatment. The association between calcineurin activity and psychiatric disorders is based on clinical observations that the rates of anxiety and depression increase in patients treated chronically with the calcineurin inhibitor cyclosporine-A to prevent rejection after organ transplantation (36). Preclinical studies reveal depressive-like behavior induction in mice through amygdala calcineurin inhibition (37). Additionally, chronic stress in rats, a model for depression research, reduces calcineurin activity in the hippocampal CA3 region (38). Notably, calcineurin has also been found to have direct antidepressant-like effects, as the inhibition of calcineurin in the prefrontal cortex of rats induces depressive-like behavior (39). These findings taken together support the role of calcineurin in the pathophysiology of depression.
PPP3R1 was replicated in two transcriptomic cohorts, GSE53987 [prefrontal cortex (PFC)] and GSE64930 (whole blood). The differential expression of PPP3R1 in the prefrontal cortex of depressed adolescents aligns with the existing evidence that the PFC is one of the regions most consistently affected in depression. Extensive literature has strongly implicated PFC dysfunction in MDD, revealing functional, structural, and systems-level abnormalities spanning various PFC regions (40). Evidence of structural changes in the PFC associated with depression mainly comes from secondary measures. For instance, the volume of the PFC is reduced in depressed patients, and this decrease is correlated with the duration of illness (41). In depression, specific changes can be observed in the molecular composition of PFC neurons (42), which modulate their electrical properties (43). Decreases in the volume of the orbitofrontal cortex (44) and pyramidal cell density (45) have also been observed in depression. These studies suggest that PFC neurons undergo widespread physiological and morphological alterations during depression. Other lines of evidence include dysregulation of glutamatergic and GABAergic neurotransmission in the PFC. PFC glutamate metabolites are reduced in depression (46), and postmortem studies demonstrate changes in ionotropic and metabotropic glutamate receptors (47, 48). Medial PFC levels of the GABA synthetic enzyme glutamate decarboxylase-67 are also reduced in postmortem brains of depressed subjects (30), as are markers of the somatostatin/calbindin (SST) GABAergic subtype (49, 50).
Other interesting findings involve two proteins, ASGR1 and CD63, corresponding to genes that may offer new insights into the pathophysiological processes of depression. Both proteins were replicated with consistent changes, but only in one cohort, GSE98793, where CD63 remained significant after Bonferroni correction. CD63 belongs to the tetraspanin family and is characterized by its four transmembrane domains (51). It can associate with interaction partners, including integrins, receptors, kinases, and other tetraspanin proteins, on the cell surface, thus contributing to the regulation of multiple signaling pathways (51–54). CD63 also serves as a platelet dense granule and lysosomal membrane protein. Interestingly, CD63 exhibits high expression in patients with comorbid diabetes and depression compared to those with diabetes alone. This comorbidity results in enhanced platelet hyperactivation and a pro-inflammatory state, increasing susceptibility to vascular complications (55). On the other hand, ASGR1 is the major subunit of the asialoglycoprotein receptor (ASGPR), a liver-specific lectin that plays a role in glycoprotein homeostasis (56). Variants in ASGR1 are associated with lower non-high-density lipoprotein (non-HDL) cholesterol levels and a reduced risk of coronary artery disease (57, 58). Thus, ASGR1 holds clinical potential as a target for lowering blood cholesterol levels. However, there is limited information available regarding the regional or cellular specificity of ASGR1 in the human brain, and its function in the brain remains poorly understood (59).
In the search of an explanatory model for the observed associations between depression and the blood levels of PPP3R1, CD63, and ASGR1, platelets emerge as potentially pivotal players (Figure 3). The presence of free CD63 in plasma strongly suggests its exosomal origin, as CD63 is widely recognized as one of the most specific exosomal markers (62). Notably, activated blood platelets are a major source of exosomes in human blood (63). This finding raises the possibility of a positive association between activated blood platelets and depression, a notion supported by multiple studies linking platelet activation to depression (60, 61). Additionally, it’s worth noting that ASGR1 plays a vital role in platelet clearance by the liver, both in humans and pigs (66, 67). Moreover, genetic variants of ASGR1 has been reported to influence platelet activation (68). Furthermore, PPP3R1, a regulatory component of the calcineurin complex responsible for phosphatase activity, has been observed to bind to platelets and prevent their aggregation (64, 65). Consequently, an increase in PPP3R1 levels may signify disruptions in platelet homeostasis.
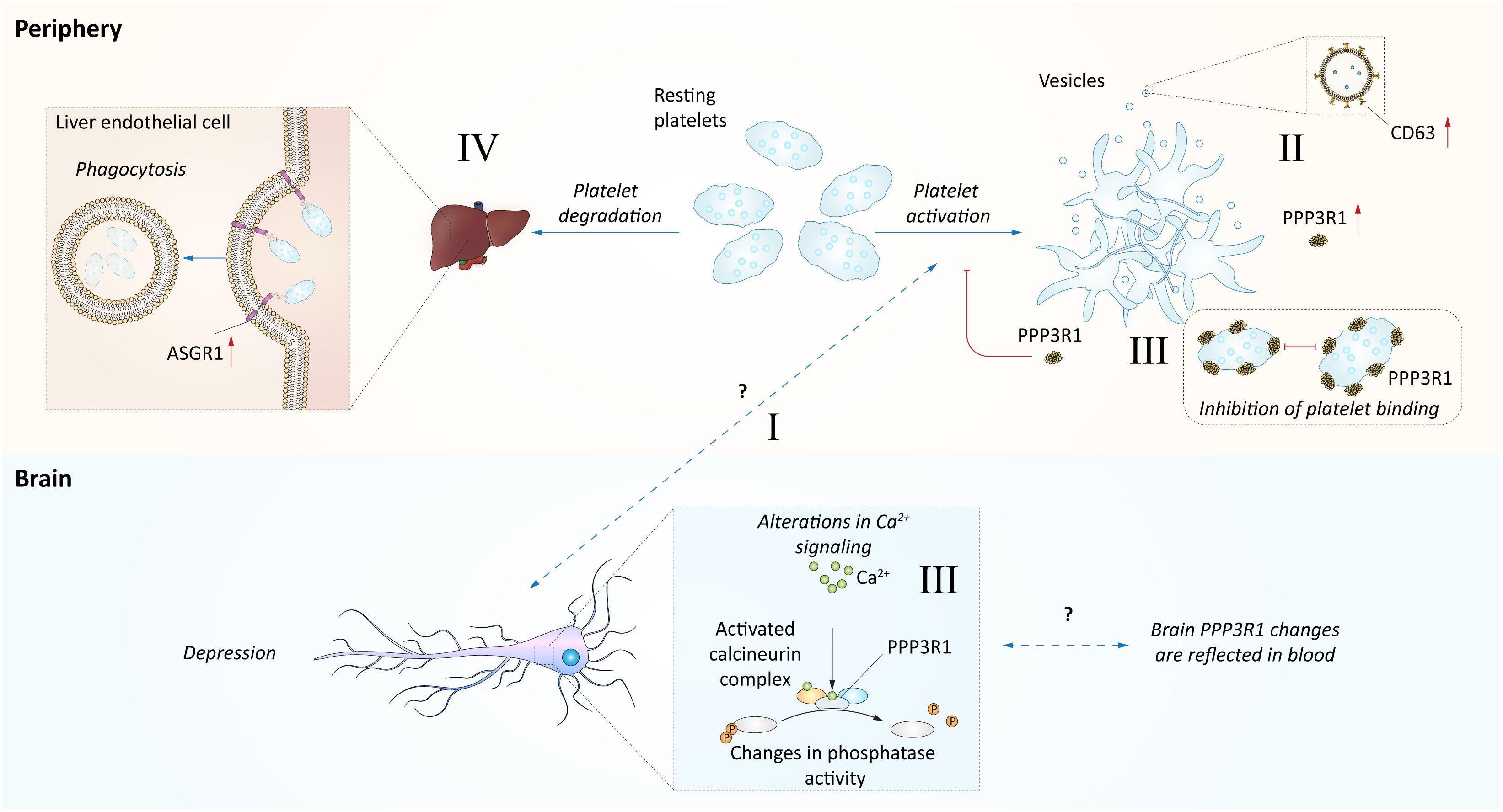
Figure 3 Hypothetical model of blood proteome relation with depression. This figure represents a hypothetical model explaining observed associations between depression and the blood levels of PPP3R1, CD63, and ASGR1. I Several studies indicate links between platelet activation and depression (60, 61). However, this mechanism is not fully established. II. The activation of platelets could be indirectly indicated by increase of CD63 levels, as this protein is abundant in exosomes (62) and release of exosomes could be attributed to platelet activation (63). The levels of CD63 platelets were positively associated with depression (60, 61). III. PPP3R1, a regulatory component of calcineurin complex mediating phosphatase activity, has been shown to bind platelets and prevent their aggregation (64, 65), and thus its increase may indicate disturbances in platelet homeostasis. The disturbances of calcineurin signaling may be indicated by changes in blood and prefrontal cortex as observed in this study. However, we observed increased plasma levels of PPP3R1 in depressed individuals. IV. The ASGR1 contribution to depression is not clear, however it is related to platelet phagocytosis by liver sinusoidal endothelial cells (66, 67).
In summary, while this model may involve some speculation, it is reasonable to suspect that the identification of three proteins related to platelet activation status in depressed adolescents could potentially reflect the neuropathological status of these adolescents. This notion is supported by a substantial body of scientific evidence suggesting that blood platelets may mirror the condition of neurons in the central nervous system (CNS) (69–74). To highlight some specific roles of platelets, studies of the serotonin uptake, storage, and secretion mechanisms in platelets have indicated major similarities to neurons (75). Studies investigating the comorbidity between cardiovascular disease and depression have proposed platelet serotonin signaling as a potential mechanism for the higher incidence of cardiac adverse events in depressed cardiovascular patients (76). Additionally, other studies have suggested that altered platelet reactivity might link stress, depression, and cardiovascular disease through changes in platelet aggregability and their content of bioactive compounds (77, 78). Platelet activation has also been implicated as a contributor to the progression of neurological conditions such as Alzheimer’s disease (79). There is potential for platelet interactions with systemic factors that change concurrently with CNS alterations, underscoring the importance of future studies elucidating the numerous systemic factors that change during depression onset, e.g. in adolescence, and how they interact with platelets.
The study’s results should be interpreted in light of several limitations. Firstly, our proteomic platform covers a rather selective portion of the proteome, potentially missing numerous proteomic pathways related to depression that our methods did not capture. Additionally, a significant number of proteins in the selected panel appear to be unexpressed in whole blood, as only 43 out of 92 were analyzable. This limitation might be attributed to the use of peripheral fluid biomarkers, as concentrations measured in the periphery may not necessarily reflect pathophysiological processes in the CNS. Furthermore, depression is a heterogeneous group of diseases often occurring concurrently with or induced by other conditions. Consequently, it is challenging to select a molecularly homogeneous group of patients or samples.
Additional limitations include the nominal significance observed for almost all proteins, which may provide weak evidence. However, it’s worth noting that nominal replication at the transcriptome level with consistent directions in independent cohorts could be considered stronger evidence. Another limitation is related to the characterization of depression across all cohorts, which is not consistent and may not represent the same phenotype. Furthermore, the populations in the cohorts are diverse and not easily comparable. Despite our efforts to account for some of the differences between the cohorts, such as age, there is still a lack of age overlap between the PSY cohort and the transcriptome cohorts. This necessitates cautious interpretation of the discordant results between the cohorts. However, on the other hand, the current findings in the PSY cohort, which reveal changes associated with depression that differ from those observed in older patients in the transcriptomic cohorts, may offer valuable insights into the etiology of depression onset during adolescence. Lastly, there were many missing values regarding medication in the PSY cohort. Since antidepressant treatment can be a significant confounder, we conducted analyses both with and without adjustment for antidepressants. Adjusting for antidepressants led to a smaller sample size due to the exclusion of missing values. We observed slightly increased effect size in the adjusted analysis that should be interpreted carefully. It could be related to biological effects of antidepressants masking protein effect in non-adjusted models or it may be of random origin as the accuracy of estimates is smaller in the adjusted analysis. Consistently, the observed shifts in the estimates of matching proteins matched between analyses (PPP3R1, RBKS, and CRADD) were less than corresponding standard errors. Lastly, even though the analysis was adjusted for antidepressants, it is possible that the real prevalence of antidepressant use may be underestimated due to underreporting from study participants. However, our estimated prevalence of antidepressant used in the PSY cohort of ~4.2% is comparable to the recent report on antidepressant use in Sweden of ~4.76 (age group 10-19 years) (80).
Conclusion
Our study of adolescent depression revealed protein-level and transcriptomic differences, particularly in PPP3R1, pointing to the involvement of the calcineurin pathway in depression. Our finding with regard to PPP3R1 also support the role of the prefrontal cortex in depression and reinforces the significance of investigating PFC-related mechanisms in depression. However, it’s important to consider these findings within the context of certain limitations, such as the selectivity of our proteomic platform and variations in depression characterization across cohorts. Further research is needed to validate and expand upon these findings, potentially leading to improved diagnostic and therapeutic strategies for adolescent depression.
Data availability statement
The original contributions presented in the study are included in the article/Supplementary Material. Further inquiries can be directed to the corresponding author.
Ethics statement
The studies involving humans were approved by Regional Ethics Committee of Uppsala, Sweden. The studies were conducted in accordance with the local legislation and institutional requirements. Written informed consent for participation in this study was provided by the participants’ legal guardians/next of kin.
Author contributions
AS: Conceptualization, Data curation, Formal analysis, Methodology, Visualization, Writing – review & editing. ML: Conceptualization, Formal analysis, Methodology, Writing – original draft. DN: Methodology, Writing – review & editing. JJ: Conceptualization, Writing – review & editing. HS: Conceptualization, Funding acquisition, Supervision, Writing – review & editing.
Funding
The author(s) declare financial support was received for the research, authorship, and/or publication of this article. This work was supported by the Swedish Brain Foundation.
Acknowledgments
This research has been conducted using data from the Psychiatric Health in Adolescent Study (PSY cohort) conducted in Uppsala, Sweden. We thank the participants of the PSY cohort that contributed to this valuable resource. We also thank Diana-Maria Manu at Uppsala University for establishing and facilitating communication with SciLifeLab.
Conflict of interest
The authors declare that the research was conducted in the absence of any commercial or financial relationships that could be construed as a potential conflict of interest.
Publisher’s note
All claims expressed in this article are solely those of the authors and do not necessarily represent those of their affiliated organizations, or those of the publisher, the editors and the reviewers. Any product that may be evaluated in this article, or claim that may be made by its manufacturer, is not guaranteed or endorsed by the publisher.
Supplementary material
The Supplementary Material for this article can be found online at: https://www.frontiersin.org/articles/10.3389/fpsyt.2024.1372106/full#supplementary-material
Supplementary Figure 1 | PPP3R1 expression. This figure shows the PPP3R1 expression across cohorts in the form of bars for better effect size visualization. (A) PPP3R1 plasma NPX in the PSY cohort depending on the DAWBA risk group. Asterisk indicates a nominal p<0.05. Error bar shows the standard error of the mean. (B) The expression levels of PPP3R1-related probe ILMN_1796962 in whole blood in depression versus controls in GSE64930. Asterisk indicates a nominal p<0.05. Error bar shows the standard error of the mean. (C) The expression levels of PPP3R1-related probe 204506_at in prefrontal cortex in several psychiatric conditions and controls in the dataset GSE53987. Error bar shows the standard error of the mean. Asterisk indicates a nominal p<0.05. DAWBA, Development and Well-Being Assessment; NPX, Normalized Protein Expression (NPX); SCZ, schizophrenia; BD, bipolar disorder; MDD, major depressive disorder.
References
2. Liu Q, He H, Yang J, Feng X, Zhao F, Lyu J. Changes in the global burden of depression from 1990 to 2017: Findings from the Global Burden of Disease study. J Psychiatr Res. (2020) 126:134–40. doi: 10.1016/j.jpsychires.2019.08.002
3. Neufeld SAS. The burden of young people’s mental health conditions in Europe: No cause for complacency. Lancet Reg Health Eur. (2022) 16. doi: 10.1016/j.lanepe.2022.100364
4. Heim C, Binder EB. Current research trends in early life stress and depression: review of human studies on sensitive periods, gene-environment interactions, and epigenetics. Exp Neurol. (2012) 233:102–11. doi: 10.1016/j.expneurol.2011.10.032
5. Murrough JW, Charney DS. Is there anything really novel on the antidepressant horizon? Curr Psychiatry Rep. (2012) 14:643–9. doi: 10.1007/s11920-012-0321-8
6. Fava M. Diagnosis and definition of treatment-resistant depression. Biol Psychiatry. (2003) 53:649–59. doi: 10.1016/s0006-3223(03)00231-2
7. Major Depressive Disorder Working Group of the Psychiatric GWAS Consortium, Ripke S, Wray NR, Lewis CM, Hamilton SP, Weissman MM, et al. A mega-analysis of genome-wide association studies for major depressive disorder. Mol Psychiatry. (2013) 18:497–511. doi: 10.1038/mp.2012.21
8. Mostafavi S, Battle A, Zhu X, Potash JB, Weissman MM, Shi J, et al. Type I interferon signaling genes in recurrent major depression: increased expression detected by whole-blood RNA sequencing. Mol Psychiatry. (2014) 19:1267–74. doi: 10.1038/mp.2013.161
9. Jansen R, Penninx BWJH, Madar V, Xia K, Milaneschi Y, Hottenga JJ, et al. Gene expression in major depressive disorder. Mol Psychiatry. (2016) 21:339–47. doi: 10.1038/mp.2015.57
10. Fernandes BS, Williams LM, Steiner J, Leboyer M, Carvalho AF, Berk M. The new field of ‘precision psychiatry. BMC Med. (2017) 15:80. doi: 10.1186/s12916-017-0849-x
11. Gwinn K, David KK, Swanson-Fischer C, Albin R, Hillaire-Clarke CS, Sieber B-A, et al. Parkinson’s disease biomarkers: perspective from the NINDS Parkinson’s Disease Biomarkers Program. biomark Med. (2017) 11:451–73. doi: 10.2217/bmm-2016-0370
12. Lewczuk P, Riederer P, O’Bryant SE, Verbeek MM, Dubois B, Visser PJ, et al. Cerebrospinal fluid and blood biomarkers for neurodegenerative dementias: An update of the Consensus of the Task Force on Biological Markers in Psychiatry of the World Federation of Societies of Biological Psychiatry. World J Biol Psychiatry. (2018) 19:244–328. doi: 10.1080/15622975.2017.1375556
13. Schwarz E, Guest PC, Rahmoune H, Harris LW, Wang L, Leweke FM, et al. Identification of a biological signature for schizophrenia in serum. Mol Psychiatry. (2012) 17:494–502. doi: 10.1038/mp.2011.42
14. Lozupone M, La Montagna M, D’Urso F, Daniele A, Greco A, Seripa D, et al. The role of biomarkers in psychiatry. In: Reviews on Biomarker Studies in Psychiatric and Neurodegenerative Disorders. Springer, Cham (2019). p. 135–62. doi: 10.1007/978-3-030-05542-4_7
15. Carvalho AF, Solmi M, Sanches M, MaChado MO, Stubbs B, Ajnakina O, et al. Evidence-based umbrella review of 162 peripheral biomarkers for major mental disorders. Transl Psychiatry. (2020) 10:1–13. doi: 10.1038/s41398-020-0835-5
16. Lopez-Duran NL, Kovacs M, George CJ. Hypothalamic–pituitary–adrenal axis dysregulation in depressed children and adolescents: A meta-analysis. Psychoneuroendocrinology. (2009) 34:1272–83. doi: 10.1016/j.psyneuen.2009.03.016
17. Dowlati Y, Herrmann N, Swardfager W, Liu H, Sham L, Reim EK, et al. A meta-analysis of cytokines in major depression. Biol Psychiatry. (2010) 67:446–57. doi: 10.1016/j.biopsych.2009.09.033
18. Miller CH, Hamilton JP, Sacchet MD, Gotlib IH. Meta-analysis of functional neuroimaging of major depressive disorder in youth. JAMA Psychiatry. (2015) 72:1045. doi: 10.1001/jamapsychiatry.2015.1376
19. D’Acunto G, Nageye F, Zhang J, Masi G, Cortese S. Inflammatory cytokines in children and adolescents with depressive disorders: A systematic review and meta-analysis. J Child Adolesc Psychopharmacol. (2019) 29:362–9. doi: 10.1089/cap.2019.0015
20. Nikkheslat N, McLaughlin AP, Hastings C, Zajkowska Z, Nettis MA, Mariani N, et al. Childhood trauma, HPA axis activity and antidepressant response in patients with depression. Brain Behavior Immun. (2020) 87:229–37. doi: 10.1016/j.bbi.2019.11.024
21. Whiteford HA, Degenhardt L, Rehm J, Baxter AJ, Ferrari AJ, Erskine HE, et al. Global burden of disease attributable to mental and substance use disorders: findings from the Global Burden of Disease Study 2010. Lancet. (2013) 382:1575–86. doi: 10.1016/S0140-6736(13)61611-6
22. Bylund D, Reed A. Childhood and adolescent depression: Why do children and adults respond differently to antidepressant drugs? Neurochemistry Int. (2007) 51:246–53. doi: 10.1016/j.neuint.2007.06.025
23. Zisook S, Lesser I, Stewart JW, Wisniewski SR, Balasubramani GK, Fava M, et al. Effect of age at onset on the course of major depressive disorder. Am J Psychiatry. (2007) 164:1539–46. doi: 10.1176/appi.ajp.2007.06101757
24. Arloth J, Bogdan R, Weber P, Frishman G, Menke A, Wagner KV, et al. Genetic differences in the immediate transcriptome response to stress predict risk-related brain function and psychiatric disorders. Neuron. (2015) 86:1189–202. doi: 10.1016/j.neuron.2015.05.034
25. Menke A, Arloth J, Best J, Namendorf C, Gerlach T, Czamara D, et al. Time-dependent effects of dexamethasone plasma concentrations on glucocorticoid receptor challenge tests. Psychoneuroendocrinology. (2016) 69:161–71. doi: 10.1016/j.psyneuen.2016.04.003
26. Leday GGR, Vértes PE, Richardson S, Greene JR, Regan T, Khan S, et al. Replicable and coupled changes in innate and adaptive immune gene expression in two case-control studies of blood microarrays in major depressive disorder. Biol Psychiatry. (2018) 83:70–80. doi: 10.1016/j.biopsych.2017.01.021
27. Lanz TA, Reinhart V, Sheehan MJ, Rizzo SJS, Bove SE, James LC, et al. Postmortem transcriptional profiling reveals widespread increase in inflammation in schizophrenia: a comparison of prefrontal cortex, striatum, and hippocampus among matched tetrads of controls with subjects diagnosed with schizophrenia, bipolar or major depressive disorder. Trans Psychiatry. (2019) 9. doi: 10.1038/s41398-019-0492-8
28. Goodman A, Heiervang E, Collishaw S, Goodman R. The ‘DAWBA bands’ as an ordered-categorical measure of child mental health: description and validation in British and Norwegian samples. Soc Psychiatry Psychiatr Epidemiol. (2011) 46:521–32. doi: 10.1007/s00127-010-0219-x
29. Goodman R, Ford T, Richards H, Gatward R, Meltzer H. The development and well-being assessment: description and initial validation of an integrated assessment of child and adolescent psychopathology. J Child Psychol Psychiatry. (2000) 41:645–55. doi: 10.1111/j.1469-7610.2000.tb02345.x
30. Assarsson E, Lundberg M, Holmquist G, Björkesten J, Bucht Thorsen S, Ekman D, et al. Homogenous 96-plex PEA immunoassay exhibiting high sensitivity, specificity, and excellent scalability. PloS One. (2014) 9:e95192. doi: 10.1371/journal.pone.0095192
31. Gautier L, Cope L, Bolstad BM, Irizarry RA. affy—analysis of Affymetrix GeneChip data at the probe level. Bioinformatics. (2004) 20:307–15. doi: 10.1093/bioinformatics/btg405
32. Leek JT, Johnson WE, Parker HS, Jaffe AE, Storey JD. The sva package for removing batch effects and other unwanted variation in high-throughput experiments. Bioinformatics. (2012) 28:882–3. doi: 10.1093/bioinformatics/bts034
33. Elias-Sonnenschein LS, Helisalmi S, Natunen T, Hall A, Paajanen T, Herukka S-K, et al. Genetic loci associated with alzheimer’s disease and cerebrospinal fluid biomarkers in a finnish case-control cohort. PloS One. (2013) 8:e59676. doi: 10.1371/journal.pone.0059676
34. Karch CM, Jeng AT, Goate AM. Calcium phosphatase calcineurin influences tau metabolism. Neurobiol Aging. (2013) 34. doi: 10.1016/j.neurobiolaging.2012.05.003
35. Cho A, Tang Y, Davila J, Deng S, Chen L, Miller E, et al. Calcineurin signaling regulates neural induction through antagonizing the BMP pathway. Neuron. (2014) 82:109–24. doi: 10.1016/j.neuron.2014.02.015
36. de Groen PC, Aksamit AJ, Rakela J, Forbes GS, Krom RAF. Central nervous system toxicity after liver transplantation. New Engl J Med. (1987) 317:861–6. doi: 10.1056/NEJM198710013171404
37. Mineur YS, Taylor SR, Picciotto MR. Calcineurin downregulation in the amygdala is sufficient to induce anxiety-like and depression-like behaviors in C57BL/6J male mice. Biol Psychiatry. (2014) 75:991–8. doi: 10.1016/j.biopsych.2014.03.009
38. Zhu W-L, Shi H-S, Wang S-J, Wu P, Ding Z-B, Lu L. Hippocampal CA3 calcineurin activity participates in depressive-like behavior in rats. J Neurochemistry. (2011) 117:1075–86. doi: 10.1111/j.1471-4159.2011.07285.x
39. Yu J-J, Zhang Y, Wang Y, Wen Z-Y, Liu X-H, Qin J, et al. Inhibition of calcineurin in the prefrontal cortex induced depressive-like behavior through mTOR signaling pathway. Psychopharmacology. (2013) 225:361–72. doi: 10.1007/s00213-012-2823-9
40. Pizzagalli DA, Roberts AC. Prefrontal cortex and depression. Neuropsychopharmacology. (2022) 47:225–46. doi: 10.1038/s41386-021-01101-7
41. Savitz J, Drevets WC. Bipolar and major depressive disorder: Neuroimaging the developmental-degenerative divide. Neurosci Biobehav Rev. (2009) 33:699–771. doi: 10.1016/j.neubiorev.2009.01.004
42. Shelton RC, Claiborne J, Sidoryk-Wegrzynowicz M, Reddy R, Aschner M, Lewis DA, et al. Altered expression of genes involved in inflammation and apoptosis in frontal cortex in major depression. Mol Psychiatry. (2011) 16:751–62. doi: 10.1038/mp.2010.52
43. Cadwell CR, Palasantza A, Jiang X, Berens P, Deng Q, Yilmaz M, et al. Electrophysiological, transcriptomic and morphologic profiling of single neurons using Patch-seq. Nat Biotechnol. (2016) 34:199–203. doi: 10.1038/nbt.3445
44. Bremner JD, Vythilingam M, Vermetten E, Nazeer A, Adil J, Khan S, et al. Reduced volume of orbitofrontal cortex in major depression. Biol Psychiatry. (2002) 51:273–9. doi: 10.1016/S0006-3223(01)01336-1
45. Rajkowska G, Miguel-Hidalgo JJ, Dubey P, Stockmeier CA, Krishnan KRR. Prominent reduction in pyramidal neurons density in the orbitofrontal cortex of elderly depressed patients. Biol Psychiatry. (2005) 58:297–306. doi: 10.1016/j.biopsych.2005.04.013
46. Moriguchi S, Takamiya A, Noda Y, Horita N, Wada M, Tsugawa S, et al. Glutamatergic neurometabolite levels in major depressive disorder: a systematic review and meta-analysis of proton magnetic resonance spectroscopy studies. Mol Psychiatry. (2019) 24:952–64. doi: 10.1038/s41380-018-0252-9
47. Feyissa AM, Chandran A, Stockmeier CA, Karolewicz B. Reduced levels of NR2A and NR2B subunits of NMDA receptor and PSD-95 in the prefrontal cortex in major depression. Prog Neuropsychopharmacol Biol Psychiatry. (2009) 33:70–5. doi: 10.1016/j.pnpbp.2008.10.005
48. Gray AL, Hyde TM, Deep-Soboslay A, Kleinman JE, Sodhi MS. Sex differences in glutamate receptor gene expression in major depression and suicide. Mol Psychiatry. (2015) 20:1057–68. doi: 10.1038/mp.2015.91
49. Rajkowska G, O’Dwyer G, Teleki Z, Stockmeier CA, Miguel-Hidalgo JJ. GABAergic neurons immunoreactive for calcium binding proteins are reduced in the prefrontal cortex in major depression. Neuropsychopharmacology. (2007) 32:471–82. doi: 10.1038/sj.npp.1301234
50. Fee C, Banasr M, Sibille E. Somatostatin-positive gamma-aminobutyric acid interneuron deficits in depression: cortical microcircuit and therapeutic perspectives. Biol Psychiatry. (2017) 82:549–59. doi: 10.1016/j.biopsych.2017.05.024
51. Pols MS, Klumperman J. Trafficking and function of the tetraspanin CD63. Exp Cell Res. (2009) 315:1584–92. doi: 10.1016/j.yexcr.2008.09.020
52. Doyle EL, Ridger V, Ferraro F, Turmaine M, Saftig P, Cutler DF. CD63 is an essential cofactor to leukocyte recruitment by endothelial P-selectin. Blood. (2011) 118:4265–73. doi: 10.1182/blood-2010-11-321489
53. Kraft S, Jouvin M-H, Kulkarni N, Kissing S, Morgan ES, Dvorak AM, et al. The tetraspanin CD63 is required for efficient igE-mediated mast cell degranulation and anaphylaxis. J Immunol. (2013) 191:2871–8. doi: 10.4049/jimmunol.1202323
54. Kobuch J, Cui H, Grunwald B, Saftig P, Knolle PA, Kruger A. TIMP-1 signaling via CD63 triggers granulopoiesis and neutrophilia in mice. Haematologica. (2015). doi: 10.3324/haematol.2014.121590
55. R S, Saharia GK, Patra S, Bandyopadhyay D, Patro BK. Flow cytometry based platelet activation markers and state of inflammation among subjects with type 2 diabetes with and without depression. Sci Rep. (2022) 12. doi: 10.1038/s41598-022-13037-z
56. Ashwell G, Harford J. Carbohydrate-specific receptors of the liver. Annu Rev Biochem. (1982) 51:531–54. doi: 10.1146/annurev.bi.51.070182.002531
57. Nioi P, Sigurdsson A, Thorleifsson G, Helgason H, Agustsdottir AB, Norddahl GL, et al. Variant ASGR1 associated with a reduced risk of coronary artery disease. New Engl J Med. (2016) 374:2131–41. doi: 10.1056/NEJMoa1508419
58. Ali L, Cupido AJ, Rijkers M, Hovingh GK, Holleboom AG, Dallinga-Thie GM, et al. Common gene variants in ASGR1 gene locus associate with reduced cardiovascular risk in absence of pleiotropic effects. Atherosclerosis. (2020) 306:15–21. doi: 10.1016/j.atherosclerosis.2020.07.001
59. Oren RL, Kim EJ, Leonard AK, Rosner B, Chibnik LB, Das S, et al. Age-dependent differences and similarities in the plasma proteomic signature of postoperative delirium. Sci Rep. (2023) 13. doi: 10.1038/s41598-023-34447-7
60. Morel-Kopp M-C, McLean L, Chen Q, Tofler GH, Tennant C, Maddison V, et al. The association of depression with platelet activation: evidence for a treatment effect. J Thromb Haemost. (2009) 7:573–81. doi: 10.1111/j.1538-7836.2009.03278.x
61. Izzi B, Tirozzi A, Cerletti C, Donati MB, de Gaetano G, Hoylaerts MF, et al. Beyond haemostasis and thrombosis: platelets in depression and its co-morbidities. Int J Mol Sci. (2020) 21. doi: 10.3390/ijms21228817
62. Khushman M, Patel GK, Laurini JA, Bhardwaj A, Roveda K, Donnell R, et al. Exosomal markers (CD63 and CD9) expression and their prognostic significance using immunohistochemistry in patients with pancreatic ductal adenocarcinoma. J Gastrointest Oncol. (2019) 10:695–702. doi: 10.21037/jgo.2018.07.02
63. Saumell-Esnaola M, Delgado D, García Del Caño G, Beitia M, Sallés J, González-Burguera I, et al. Isolation of platelet-derived exosomes from human platelet-rich plasma: biochemical and morphological characterization. Int J Mol Sci. (2022) 23. doi: 10.3390/ijms23052861
64. Su Z, Xin S, Li J, Guo J, Long X, Cheng J, et al. A new function for the calcineurin b subunit: antiplatelet aggregation and anticoagulation. IUBMB Life. (2011) 63:1037–44. doi: 10.1002/iub.562
65. Khatlani T, Pradhan S, Da Q, Gushiken FC, Bergeron AL, Langlois KW, et al. The β isoform of the catalytic subunit of protein phosphatase 2B restrains platelet function by suppressing outside-in αII b β3 integrin signaling. J Thromb Haemost. (2014) 12:2089–101. doi: 10.1111/jth.12761
66. Paris LL, Estrada JL, Li P, Blankenship RL, Sidner RA, Reyes LM, et al. Reduced human platelet uptake by pig livers deficient in the asialoglycoprotein receptor 1 protein. Xenotransplantation. (2015) 22:203–10. doi: 10.1111/xen.12164
67. Paris LL, Chihara RK, Reyes LM, Sidner RA, Estrada JL, Downey SM, et al. ASGR1 expressed by porcine enriched liver sinusoidal endothelial cells mediates human platelet phagocytosis in vitro. Xenotransplantation. (2011) 18:245–51. doi: 10.1111/j.1399-3089.2011.00639.x
68. Ali L, Boogert MAW vd, Stroes EGS. ASGR1 - Targeting three aspects of atherosclerosis. Atherosclerosis. (2018) 275:e114. doi: 10.1016/j.atherosclerosis.2018.06.322
69. Dukhinova M, Kuznetsova I, Kopeikina E, Veniaminova E, Yung AWY, Veremeyko T, et al. Platelets mediate protective neuroinflammation and promote neuronal plasticity at the site of neuronal injury. Brain Behav Immun. (2018) 74:7–27. doi: 10.1016/j.bbi.2018.09.009
70. Leiter O, Walker TL. Platelets: The missing link between the blood and brain? Prog Neurobiol. (2019) 183:101695. doi: 10.1016/j.pneurobio.2019.101695
71. Amadio P, Zarà M, Sandrini L, Ieraci A, Barbieri SS. Depression and cardiovascular disease: the viewpoint of platelets. Int J Mol Sci. (2020) 21. doi: 10.3390/ijms21207560
72. Wassmer SC, Humpel C, Orian JM. Editorial: platelets as players in neuropathologies: novel diagnostic and therapeutic targets. Front Immunol. (2021) 12:772352. doi: 10.3389/fimmu.2021.772352
73. Burnouf T, Walker TL. The multifaceted role of platelets in mediating brain function. Blood. (2022) 140:815–27. doi: 10.1182/blood.2022015970
74. Wei Y, Feng J, Ma J, Chen D, Xu H, Yin L, et al. Characteristics of platelet-associated parameters and their predictive values in Chinese patients with affective disorders. BMC Psychiatry. (2022) 22:150. doi: 10.1186/s12888-022-03775-9
75. Jedlitschky G, Greinacher A, Kroemer HK. Transporters in human platelets: physiologic function and impact for pharmacotherapy. Blood. (2012) 119:3394–402. doi: 10.1182/blood-2011-09-336933
76. Williams MS, Ziegelstein RC, McCann UD, Gould NF, Ashvetiya T, Vaidya D. Platelet serotonin signaling in patients with cardiovascular disease and comorbid depression. Psychosomatic Med. (2019) 81:352. doi: 10.1097/PSY.0000000000000689
77. Halaris A. Comorbidity between depression and cardiovascular disease. Int Angiol. (2009) 28:92–9.
78. Hüfner K, Koudouovoh-Tripp P, Kandler C, Hochstrasser T, Malik P, Giesinger J, et al. Differential changes in platelet reactivity induced by acute physical compared to persistent mental stress. Physiol Behav. (2015) 151:284–91. doi: 10.1016/j.physbeh.2015.07.021
79. Gowert NS, Donner L, Chatterjee M, Eisele YS, Towhid ST, Münzer P, et al. Blood platelets in the progression of Alzheimer’s disease. PloS One. (2014) 9:e90523. doi: 10.1371/journal.pone.0090523
Keywords: depression, proteome, transcriptome, adolescents, psychiatry
Citation: Sokolov AV, Lafta MS, Nordberg DOT, Jonsson J and Schiöth HB (2024) Depression proteomic profiling in adolescents with transcriptome analyses in independent cohorts. Front. Psychiatry 15:1372106. doi: 10.3389/fpsyt.2024.1372106
Received: 17 January 2024; Accepted: 26 April 2024;
Published: 15 May 2024.
Edited by:
Keming Gao, Case Western Reserve University, United StatesReviewed by:
Magdalena Sowa-Kucma, University of Rzeszow, PolandEric B. Dammer, Emory University, United States
Srinath Jagarlapudi, Pfizer, New York, United States, in collaboration with reviewer ED
Copyright © 2024 Sokolov, Lafta, Nordberg, Jonsson and Schiöth. This is an open-access article distributed under the terms of the Creative Commons Attribution License (CC BY). The use, distribution or reproduction in other forums is permitted, provided the original author(s) and the copyright owner(s) are credited and that the original publication in this journal is cited, in accordance with accepted academic practice. No use, distribution or reproduction is permitted which does not comply with these terms.
*Correspondence: Helgi B. Schiöth, aGVsZ2kuc2NoaW90aEBuZXVyby51dS5zZQ==
†These authors have contributed equally to this work