- 1Clinical lab, Shandong Daizhuang Hospital, Jining, China
- 2Precision Medicine Laboratory, Shandong Daizhuang Hospital, Jining, China
- 3Department of Psychiatry, School of Mental Health, Jining Medical University, Jining, China
- 4Department of Psychiatry, Shandong Daizhuang Hospital, Jining, China
- 5Qingdao Mental Health Center, Qingdao, China
- 6The National Clinical Research Center for Mental Disorders & Beijing Key Laboratory of Mental Disorders, Beijing Anding Hospital & the Advanced Innovation Center for Human Brain Protection, Capital Medical University, Beijing, China
- 7Blood Transfusion Department, Jining First People’s Hospital, Shandong First Medical University, Jining, China
Introduction: With the rising demand for medical services and the associated burden, work-related stress and mental health issue have garnered increased attention among healthcare workers. Anxiety, cognitive impairment, and their comorbidities severely impact the physical and mental health as well as the work status of healthcare workers. The network analysis method was used to identify the anxiety and cognitive impairment among mental healthcare workers using the Generalized Anxiety Disorder Scale (GAD-7) and the Perceived Deficit Questionnaire for Depression (PDQ-D). We sought to identify the core symptoms associated with the comorbidity of anxiety and cognitive impairment in mental healthcare workers.
Methods: The study was conducted by Shandong Daizhuang Hospital and Qingdao Mental Health Center in China from September 13, 2022, to October 25, 2022, involving a total of 680 healthcare workers as participants. GAD-7 and PDQ-D were utilized to assess anxiety and cognitive impairment, respectively. Regularized partial correlation network analysis was employed to examing the expected influence and predictability of each item within the network. Statistical analysis and visualization of the network were performed using R software.
Results: The mean total score for anxiety was 3.25, while the mean total score for cognitive symptoms was 15.89. PDQ17 “Remembering numbers”, PDQ12 “Trouble get started” and PDQ20 “Trouble make decisions” emerged as central symptoms in the anxiety-cognition network. GAD6 “Irritable”, GAD5 “Restlessness” and GAD1 “Nervousness or anxiety” were identified as the most critical bridge symptoms connecting anxiety and cognition. Gender was found to be unrelated to the global strength of the network, edge weight distribution, or individual edge weights.
Conclusion: Utilizing central and bridge symptoms (i.e., Remembering numbers, Trouble get started, Trouble make decisions, Irritable, Restlessness and Nervousness or anxiety) as primary intervention points may aid in mitigating the serious health consequences of anxiety, cognitive impairment, and comorbidities anxiety and cognitive impairment for mental healthcare workers.
1 Introduction
In recent years, the continuous improvement of medical services, coupled with the significant aging of the population has led to an increasing demand for medical care and an accompanying burden on the healthcare system. However, the quantity and quality of healthcare workers have not improved correspondingly, further exacerbating the work pressure of healthcare workers (1, 2). Research shows that the work stress and mental health problems of healthcare workers have become global public health problems, which seriously affect the physical and mental health and career of healthcare workers. Anxiety and cognitive impairment are common psychological problems among healthcare workers (3–5). These symptoms can seriously affect health care workers and their ability to perform their duties effectively. Understanding the impact of anxiety, cognition impairment, and their comorbidities on healthcare providers is critical for developing effective interventions and support systems to address these challenges (6, 7). A 2014 survey examining healthcare workers in China revealed that the prevalence of anxiety among this population was 22.9% (8). Additionally, a national study conducted in 2020 found that the prevalence of cognitive impairment varied between 9.7% and 23.3% (9). Anxiety and cognitive impairment of healthcare workers will not only affect their physical and mental health, but also have a negative impact on the quality of medical services and the safety and health of patients (10). Compared to anxiety or cognitive impairment alone, the occurrence of comorbidities may lead to more serious health problems, such as more severe disease, higher risk of chronic disease, and more severe dysfunction (11). Therefore, to minimize the serious health consequences of comorbidity with both, it is important that we study the specific characteristics of anxiety and cognitive impairment.
Anxiety and cognitive impairment include a series of symptoms, among which the anxiety symptoms are mainly threats, restlessness, irritability, sleep disturbance and nervousness, as well as palpitations, dry mouth and sweating. Cognitive impairment plays an important role in various mental and neurological diseases, mainly manifested as executive function, attention, memory, information processing speed and other functions, affecting people’s work and life quality (12–14). However, most studies evaluate the severity of anxiety and cognitive impairment solely through independent total scores on standardized scale, failing to capture the associations and interrelationships between individual symptoms.
Network analysis is a method employed to simulate complex systems by building a network model (a framework for conceptualizing psychological disorders) to analyze and visually display the relationship between multiple variables (15, 16). Within the context of network analysis, there is an interaction between symptoms in the symptom cluster of psychiatric disorders, where the value of each symptom subscale is a node representing the variable to be observed (for example, each symptom in the anxiety and cognitive impairment scales is a node of information). Different nodes were connected by edges, which represent the relationship between nodes (partial correlation) (17). Node centrality statistical indicators (such as strength and Expected Influence (EI)) are used to measure node characteristics and identify central influence symptoms in the network (15). The central symptom in the network model exhibits the strongest association with other symptoms, and it may activate other symptoms. This symptom could play a crucial role in the onset or maintenance of symptomatic syndromes (18). Therefore, preventive measures and interventions targeting central symptoms may be more effective. In addition, the network model provides a new way to understand the comorbidities of mental illness (19). For example, when a person has anxiety, a symptom in anxiety may increase the risk of cognitive impairment occurring, and a symptom here is seen as a bridge symptom in the network model. Bridge symptoms in the network model may play a key role in the occurrence and continuation of comorbidities of diseases, and also provide ideas for clinicians to prevent and treat comorbidities of mental diseases (20).
A network analysis of depression and anxiety comorbidity among Chinese clinicians revealed that “Impaired motor skills”, “Trouble relaxing” and “Uncontrollable worry” constitute the core symptoms of the overall depression-anxiety network. Additionally, “Irritability”, “Feeling afraid” and “Sad mood” emerged as the most critical bridging symptoms between depression and anxiety. Three symptoms—”Fatigue”, “Trouble relaxing” and “Nervousness”—exhibited the strongest and most negative correlation with quality of life. Notably, studies indicate that more than half of patients with depression experience cognitive dysfunction, which includes deficits in memory, executive function, attention, and reaction time. A substantial body of research supports the notion that cognitive dysfunction serves not only as a state marker of major depressive disorder (MDD) but also as a characteristic marker of MDD. Consequently, we aimed to explore the key symptoms associated with cognitive impairment and anxiety comorbidities for healthcare workers.
To date, no studies of comorbidity anxiety and cognitive impairment in clinicians have been published using the network model. Therefore, the purpose of this study was to investigate the network structure of anxiety and cognitive disorder comorbidity among mental healthcare workers in China, and to explore the relationship between anxiety and cognitive disorder symptoms.
2 Method
2.1 Research object
This is a cross-sectional study on the mental health status of healthcare workers, conducted by Shandong Daizhuang Hospital and Qingdao Mental Health Center in China from September 13, 2022 to October 25, 2022. We used “WJX” (a platform providing functions equivalent to Amazon Mechanical Turk, powered by www.wjx.cn) to design questionnaires and form QR codes to conduct surveys through WeChat, a smartphone-based social messaging app widely used in China (21). The inclusion criteria for participants were: 1. Age ≥18 years old; 2. Mental healthcare workers who can understand the assessment. The exclusion criteria were: 1. Mental healthcare workers with mental illnesses or those suffering from mental or physical conditions that may impair cognition; 2. Mental healthcare workers who are taking medications that could potentially affect cognitive function, including glucocorticoids, beta blockers, opioid analgesics, and central stimulants. All participants were required to sign electronic informed consent, and this study was approved by the Ethics Committee of Shandong Daizhuang Hospital (Jining Mental Health Center and the Second Affiliated Hospital of Jining Medical College) (Ethics number: 202208KS-1).
2.2 Measurement tools
Perceived Deficit Questionnaire for Depression (PDQ-D) was used to assess the cognitive impairment of healthcare workers. PDQ-D is a subjective assessment tool of cognitive impairment, which includes 20 items (22, 23). Each item asked how often cognitive deficits were experienced in the past week, scoring them on a scale of 0 (never), 1 (rarely), 2 (sometimes), 3 (often) and 4 (almost always). The PDQ-D score ranges from 0 to 80. The higher the PDQ-D score, the worse the subjective cognition. The method demonstrated excellent internal consistency and test-retest reliability in a study conducted with a Chinese population. The sample comprised not only individuals with major depression but also community volunteers. Consequently, this indicates that the PDQ-D can be effectively applied to the target population of mental health care workers.
The Generalized Anxiety Disorder7 (GAD-7) is commonly used to screen for generalized anxiety disorder disorders and longitudinal monitoring of treatment outcomes, asking suspected cases whether they use alcohol or drugs to reduce anxiety or stress, and screening for depression and suicide risk (22). The scale consists of seven questions on a 21-point scale, with 5–9 indicating mild anxiety, 10–14 indicating moderate anxiety, and 15–21 indicating severe anxiety.
2.3 Statistical methods
2.3.1 Network analysis
Data analysis and visualization of anxiety and cognitive impairment networks in software R (R Core Team; version 4.3.0) (24). In network analysis, each symptom is defined as a node, the line between the two nodes is defined as an edge, the thicker the edge indicates the stronger the correlation, and the green and red edges indicate the positive and negative correlation, respectively. Sparse graph Gaussian Model (GGM) combined with graph minimum absolute contraction and selection operator (LASSO) method was used to estimate the network model. In order to reduce the number of pseudo-edges and improve the interpretable results, LASSO was used to regularize the network model (17). In addition, Extended Bayesian Information Criterion (EBIC) was used to select the optimal fitting model (15). According to previous studies, the optimal parameter of EBIC is 0.5.
2.3.1.1 Central symptoms
In order to identify the most central (influential) symptoms in the network model of anxiety and cognitive impairment, we adopted an intensity centrality estimation approach. Strength centrality is a measure that reveals the strength of the connection between a particular node and other nodes by calculating the sum of the absolute edge weights of that node. For this analysis, we used the bootnet (17) and qgraph (25) in R package.
In addition, we estimate the predictability index of each node (the likelihood that the state of a given node can be explained by the state of its neighboring nodes) (26–28). This estimate is based on the mgm (26, 27) network model in the R package.
Bridge strengths in network models are widely used to identify symptoms that can connect two or more different diseases, such as anxiety and cognitive impairment. Therefore, we also estimated the bridge strength of each node, using the networktools of the R package in this process.
2.3.1.2 Stability and accuracy of the network
The bootnet package is used to test the stability and accuracy of the network. First, the accuracy is evaluated by calculating the edge weight 95% confidence interval (bootstrapped samples = 1000). The narrower the confidence interval, the higher the accuracy (29). Secondly, in order to evaluate the stability of the expected influence, the correlation stability coefficient (CS-C) was calculated through the sample drop bootstrap method (subletting bootstrap=1000). CS-C represents the maximum proportion of samples that can be deleted so that the correlation between the original centrality index can reach at least 0.7 with a 95% probability. According to previous studies, the correlation coefficient should be higher than 0.5 and not lower than 0.25 (17). In order to evaluate whether the difference between the two edge weights or the strength centrality of two nodes was statistically significant, the difference test between the edge weights and the strength centrality of the nodes was conducted (1000 times bootstrap) (significance level α=0.05), and P < 0.05 was considered as statistically significant.
2.3.1.3 Gender network comparison
The differences between the two networks (male vs female) were evaluated using the Network Comparison Test (NCT) package, this package based on permutation tests using 1000 permutation pairs subsamples (male vs female). The overall network strength (sum of absolute values of all edge weights) and network structure (distribution of edge weights) between the two networks were evaluated. In addition, the Holm-Bonferroni method of multiple comparisons was used to compare the edge invariance (30). These tests were performed with the R-package ‘NetworkComparisonTest’ version 2.2.1.
3 Result
3.1 Sample characteristics of comorbidity of anxiety and cognitive impairment
A total of 680 participants were invited to participate in this study, 658 met the study enrollment criteria and completed the assessment, including 462 (70.2%) women and 196 (29.8%) men. 484 (73.6%) with college education or above, and 494 (75.1%) married (Supplementary Table 1).
3.2 Network structure
The mean total score of anxiety was 3.25 and the mean total score of cognitive impairment was 15.89. No item was lower than 0.25 SD compared to the average amount of information (i.e., SD) of GAD-7 (MSD = 0.66 ± 0.19) or PDQ-D (MSD = 0.83 ± 0.04). In addition, the item redundancy analysis did not find any GAD-7 or PDQ-D items in the measurement redundant with other items.
The descriptive information of cognitive deficits and anxiety symptoms is shown in Table 1, and the estimated network structure is shown in Figure 1. Several nodes are highly connected to the rest of the network, with PDQ17 “Remembering numbers” showing the highest intensity, followed by PDQ12 “Trouble get started” and PDQ20 “Trouble make decisions”.
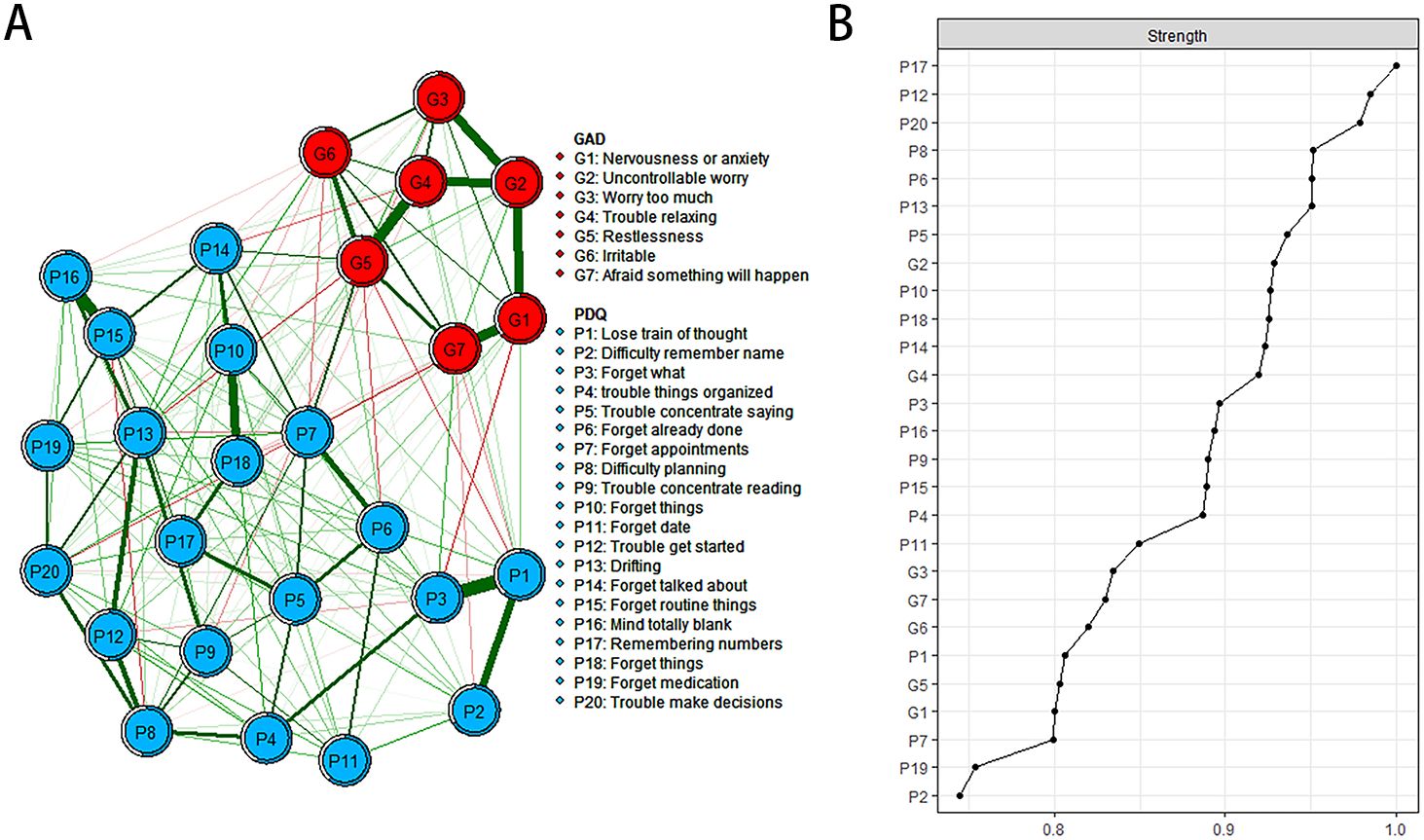
Figure 1. Estimated network structure of anxiety and cognitive impairment and the corresponding centrality of each node. (A) The anxiety and cognitive impairment network structure. The different-size circles represent different strength of the nodes, while the width and saturation of edges indicate the connections and directions (i.e., green: positive correlation; red: negative correlation). The ring around each node indicates the predictability, with a filled ring representing that 100% of the variance is accounted for by the other nodes and an empty ring corresponding to 0% predictability. (B) The corresponding centrality of each node in the anxiety and cognitive impairment network. PDQ-D=Perceived Deficit Questionnaire for Depression, GAD=Generalized Anxiety Disorder.
In the anxiety cognitive network model, PDQ17 “Remembering numbers”, PDQ12 “Trouble get started” and PDQ20 “Trouble make decisions” have the highest intensity centrality. GAD6 “Irritable”, GAD5 “Restlessness” and GAD1 “Nervousness or anxiety” showed the highest bridge strength, linking cognitive deficits and anxiety symptoms in Figure 2 and Supplementary Table 2.
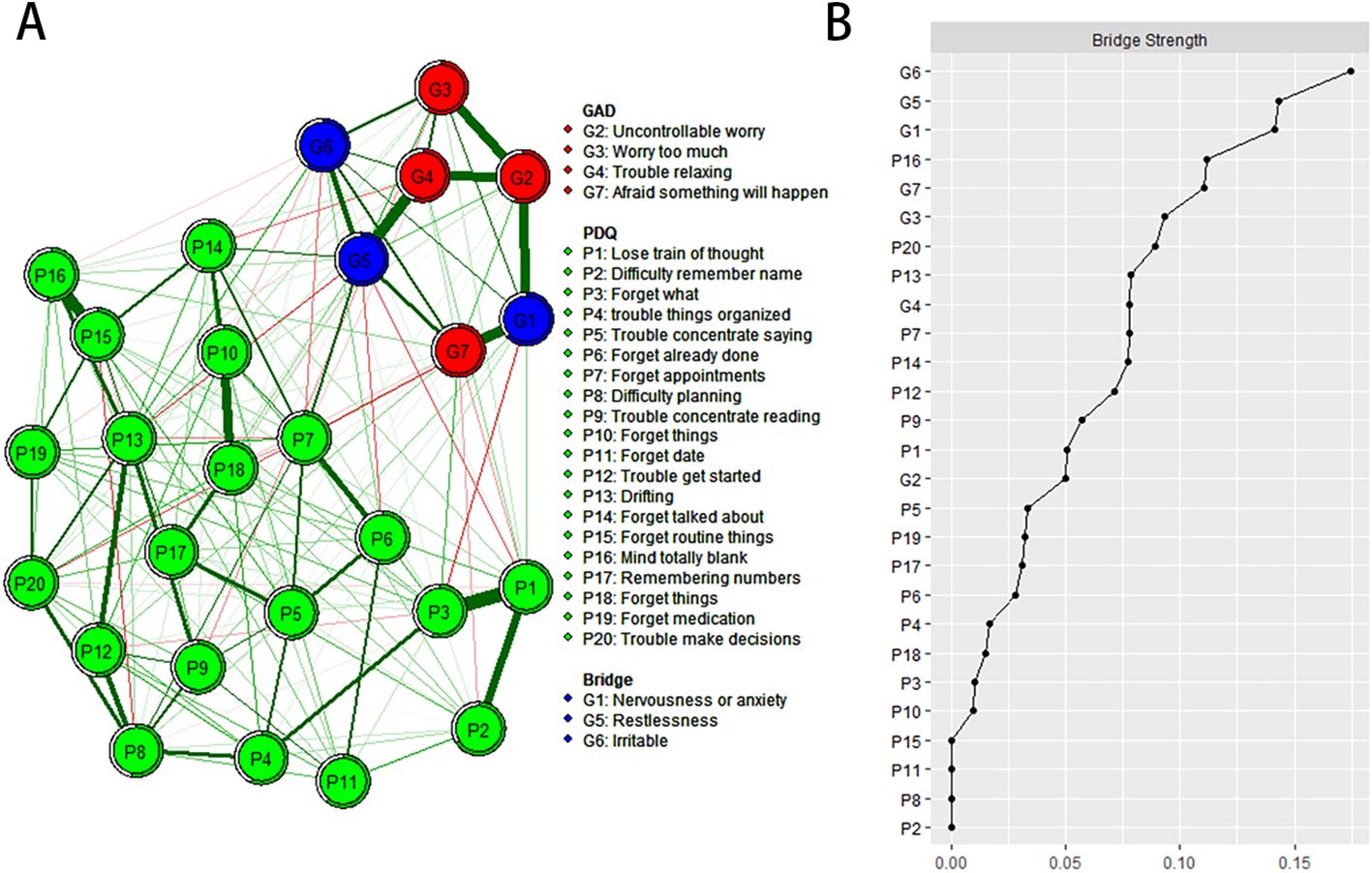
Figure 2. Estimated network structure of anxiety and cognitive impairment and the corresponding bridge symptoms. (A) The anxiety and cognitive impairment network structure highlighting the bridge symptoms. The different-size circles represent different strength of the nodes, while the width and saturation of edges indicate the connections and directions (i.e., green: positive correlation; red: negative correlation). The ring around each node indicates the predictability, with a filled ring representing that 100% of the variance is accounted for by the other nodes and an empty ring corresponding to 0% predictability. (B) The bridge strength of symptoms in the anxiety and cognitive impairment network. PDQ-D=Perceived Deficit Questionnaire for Depression, GAD=Generalized Anxiety Disorder.
3.3 Accuracy and stability of the network
The accuracy of edge weights calculated by bootstrap method shows that the 95% confidence interval with significant edge weights in the current sample is narrow, indicating that the current network has good stability, as shown in Figure 3. The centrality of strength was calculated by subletting bootstrap=1000, and the values of edge strength and strength remained stable even after most samples were dropped (Supplementary Figure 1, CS-C=0.52, i.e., after dropping up to 95% of the sample, the order of the symptoms in strength was still correlated with the original one (r = 0.7)). In addition, the edge and strength difference test based on bootstrap method shows that the strongest edges and nodes are significantly different from other edges and nodes, also reflecting the high precision of the estimated network.
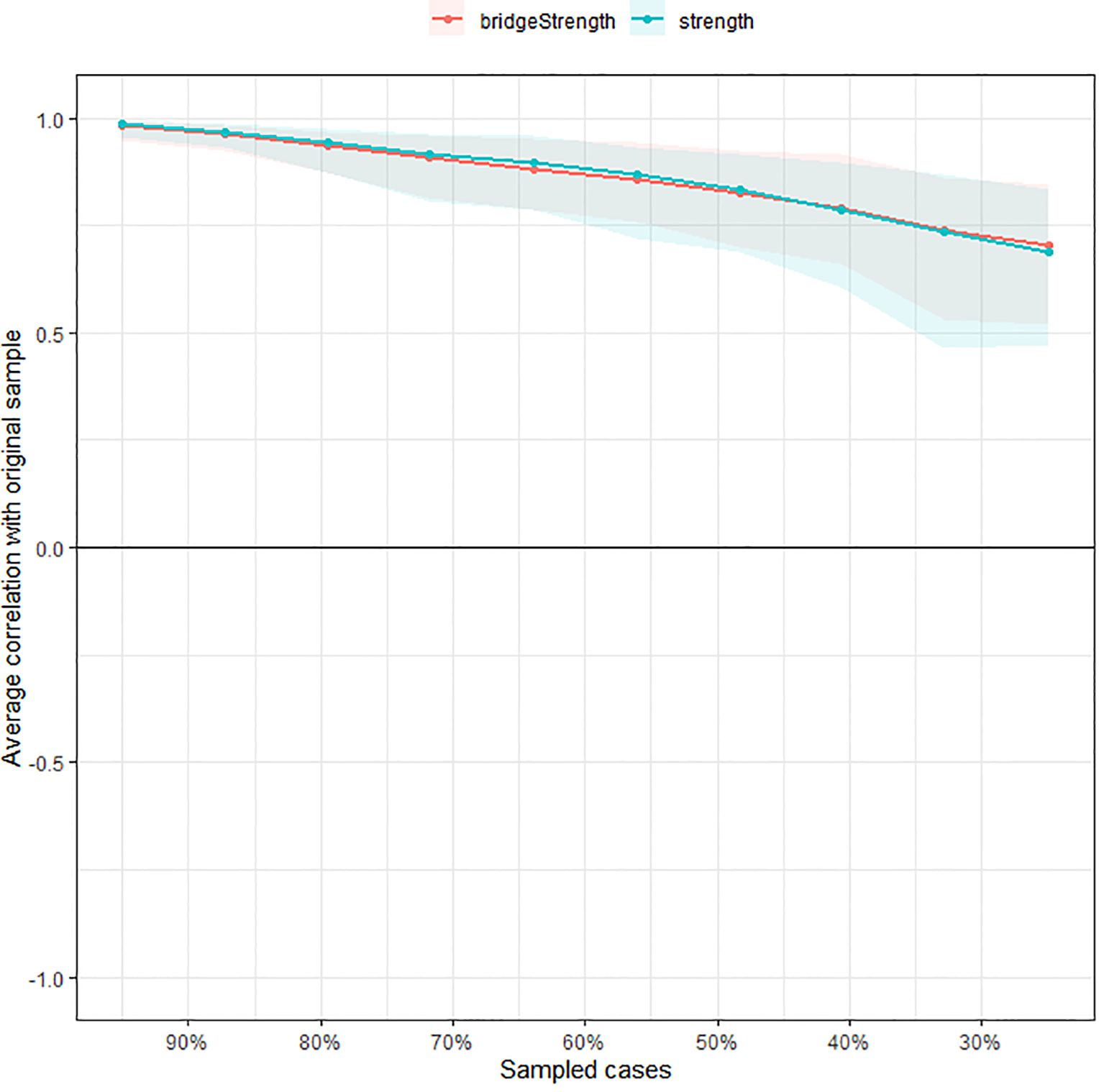
Figure 3. Stability of the estimated network structure of anxiety and cognitive impairment symptoms. The percentage of cases of the original sample used at each step is represented on the x-axis. The average of correlations between the centrality indices from the original network and the centrality indices from the networks that were re-estimated after excluding increasing percentages of cases is represented on the y-axis. Each line indicates the correlations among betweenness, closeness, and strength.
3.4 Gender network comparison
Comparing the network model between male (n = 196) and female (n = 462) healthcare workers, the overall network strength was not statistically significant (male: 11.82; female: 12.67; S=0.85, P=0.62; Supplementary Figure 2), the network difference test had no statistical significance (M =0.29, P= 0.73, Supplementary Figure 3).
4 Discussion
To the best of our knowledge, this study represents the first investigation in China to elucidate the network structure connecting anxiety and cognitive impairment among mental healthcare workers. In terms of network analysis, PDQ17 “Remembering numbers”, PDQ12 “Trouble get started” and PDQ20 “Trouble make decisions” are the most influential central symptoms in the network model. In addition, GAD6 “Irritable”, GAD5 “Restlessness” and GAD1 “Nervousness or anxiety” showed the highest bridge strength linking cognitive impairment and anxiety symptoms, suggesting that these symptoms should be treated first to reduce and prevent comorbidities of anxiety and cognitive impairment.
We found that PDQ17 “Remembering numbers”, PDQ12 “Trouble get started” and PDQ20 “Trouble make decisions” were central symptoms of the network model of anxiety and cognitive impairment. These symptoms correspond to the entries in PDQ-D, which provides a subjective measure of cognitive dysfunction. A study examining cognitive screening among healthcare workers over the age of 70 revealed an incidence of cognitive impairment of 14.4%. As individuals age, the risk of stroke, neurodegenerative diseases such as Alzheimer’s disease (AD), and other systemic conditions such as cancer increases, all of which can contribute to cognitive decline and elevate the risk of medical errors. This is consistent with previous research (31–36). One study found that “depression,” “anxiety” and “apathy” are common in mild cognitive impairment (MCI). These symptoms can exacerbate cognitive decline and progress to dementia (31). Data show that in patients with AD, the prevalence rate of anxiety is 9.4%-39%, and the core symptom of AD is cognitive impairment and other cognitive deficits (32–36). Other studies, however, have found something different (37–39). For example, studies focusing on cognitive features of attention-deficit/hyperactivity disorder (ADHD) will integrate “the processing speed index” (PSI) and “working memory index” (WMI). It was identified as the most serious symptom of cognitive impairment (37, 38). In a study of 50 patients with Parkinson’s disease, 33 had no anxiety and 17 had anxiety, with the latter’s core cognitive impairment being a decline in “working memory” (39). These inconsistent findings suggest differences in the central symptoms of anxiety and cognitive impairment between different study samples. In addition, the heavy workload and increased workload of healthcare workers persist (40). All these factors may increase the risk of psychomotor emergence, such as “Remembering numbers,” “Trouble get started,” and “Trouble make decisions”. In network theory, central symptoms play an important role in maintaining psychopathological networks (41), so attention and treatment of these symptoms may help improve other symptoms.
In the anxiety and cognitive impairment network model, “Irritable”, “Restlessness”, “Nervousness or anxiety” constituted the most important symptom core connecting the anxiety and cognitive disorder communities. Our study is consistent with many previous studies (42–47). For example, Roberto et al. found that irritable and apathetic patients had similar cognitive decline performance in the two memory domains, and “irritable” proved to be the most serious and fastest neuropsychiatric symptom of cognitive decline in their study (42). Studies have found that the prevalence of anxiety in patients with mild cognitive impairment or dementia ranges from 8% to 71% (43–45). “Restlessness”, “irritability”, “muscle tension”, “fear”, and “respiratory” and other anxiety symptoms reduce their cognitive and behavioral ability and their ability to live independently, resulting in a decline in their quality of life (46, 47). However, previous studies have also had inconsistent findings (48, 49). For example, in a study of 14,578 front-line healthcare workers, “Not being able to stop or control worrying” might be the core symptom and a potential effective intervention target (48). Sun et al. found that “Irritability”, “Nervousness-Uncontrollable worry” and “Excessive worry” were the central symptoms of depression and anxiety network model, and “Sad Mood”, “restlessness” and “Irritability” were the bridge symptoms of depression and anxiety comorbidities (49). We did not find more studies on the comorbidity of anxiety and cognitive impairment, suggesting that we need to fill in the gaps in the field. In conclusion, in network analysis theory, the improvement of bridge symptoms also brings changes in other symptoms of the disease (50). Therefore, interventions targeting these bridging symptoms could reduce symptoms of anxiety and cognitive impairment in healthcare workers.
This study revealed a significant role of anxiety symptoms within the network structure of cognitive impairment and anxiety comorbidity. Notably, both the central and bridge symptoms were predominantly associated with anxiety symptoms, suggesting that these symptoms play a critical role in both the onset and persistence of psychological disorders among healthcare workers. The findings support previous research. A survey of 1,685 healthcare workers revealed that 31% experienced mild anxiety, 33% exhibited clinically significant anxiety, 29% reported mild depression, and 17% faced moderate to severe depressive symptoms. The reason for this phenomenon may be related to fear and uncertainty caused by multiple factors (51). Taken together, our findings suggest the need for monitoring and intervention for specific anxiety symptoms among health care workers.
The strengths of this study include its highly representative sample and the application of network analysis methods for examining the relationship between anxiety and cognitive impairment among healthcare personnel. However, the study also has several limitations. Firstly, as a cross-sectional study, it cannot establish causal relationships between anxiety and cognitive impairment. A longitudinal study would be beneficial for gaining a deeper understanding of the subjects examined. Secondly, the assessment of anxiety and cognitive impairment relies on self-reported scales, which may limit the accuracy of clinical phenomena and introduce recall bias (52). as a cross-sectional study, it cannot establish causal relationships between anxiety and cognitive impairment. A longitudinal study would be beneficial for gaining a deeper understanding of the subjects examined. Secondly, the assessment of anxiety and cognitive impairment relies on self-reported scales, which may limit the accuracy of clinical phenomena and introduce recall bias.
In summary, the central symptoms identified by the network model of anxiety and cognitive impairment include “Remembering numbers,” “Trouble get started,” and “Trouble make decisions”. Additionally, the bridging symptoms comprise “Irritable,” “Restlessness,” and “Nervousness or anxiety”. We fill in the gaps in previous research and help identify the most impactful symptoms of anxiety and cognitive impairment comorbidities. These findings could help develop more effective interventions and prevention strategies for mental healthcare workers.
Data availability statement
The original contributions presented in the study are included in the article/Supplementary Material. Further inquiries can be directed to the corresponding author.
Ethics statement
The studies involving humans were approved by the Ethics Committee of Shandong Daizhuang Hospital (Jining Mental Health Center and the Second Affiliated Hospital of Jining Medical College) (Ethics number: 202208KS-1). The studies were conducted in accordance with the local legislation and institutional requirements. The participants provided their written informed consent to participate in this study.
Author contributions
RC: Formal analysis, Data curation, Writing – original draft, Conceptualization. WY: Writing – review & editing, Formal analysis, Data curation. QS: Writing – review & editing, Formal analysis, Data curation. ML: Formal analysis, Writing – review & editing, Data curation. MC: Writing – review & editing, Supervision, Project administration. JD: Data curation, Writing – review & editing. YW: Writing – review & editing, Formal analysis. XZ: Writing – original draft, Formal analysis, Data curation, Conceptualization. JC: Writing – review & editing, Visualization, Validation, Supervision, Software, Methodology, Investigation, Funding acquisition, Conceptualization, Formal analysis.
Funding
The author(s) declare financial support was received for the research, authorship, and/or publication of this article. Supported by the National Natural Science Foundation of China (82071531), Shandong Medicine and Health Science and Technology Development Plan (202203090679), Key Research and Development Plan of Jining City (2021YXNS096).
Acknowledgments
The authors are grateful to all participants and clinicians involved in this study.
Conflict of interest
The authors declare that the research was conducted in the absence of any commercial or financial relationships that could be construed as a potential conflict of interest.
Publisher’s note
All claims expressed in this article are solely those of the authors and do not necessarily represent those of their affiliated organizations, or those of the publisher, the editors and the reviewers. Any product that may be evaluated in this article, or claim that may be made by its manufacturer, is not guaranteed or endorsed by the publisher.
Supplementary material
The Supplementary Material for this article can be found online at: https://www.frontiersin.org/articles/10.3389/fpsyt.2024.1393598/full#supplementary-material
References
1. Braquehais MD, Vargas-Caceres S. Psychiatric issues among health professionals. Med Clin North Am. (2023) 107:131–42. doi: 10.1016/j.mcna.2022.04.004
2. Yang S, Meredith P, Khan A. Stress and burnout among healthcare professionals working in a mental health setting in Singapore. Asian J Psychiatr. (2015) 15:15–20. doi: 10.1016/j.ajp.2015.04.005
3. Mattingly G, Anderson RH, Mattingly SG, Anderson EQ. The impact of cognitive challenges in major depression: the role of the primary care physician. Postgrad Med. (2016) 128:665–71. doi: 10.1080/00325481.2016.1221318
4. Zhao Y, Tao Y, Bao X, Ding Q, Han C, Luo T, et al. A study on differences about the influencing factors of depressive symptoms between medical staff and residents during 2022 city-wide temporary static management period to fighting against COVID-19 pandemic in Shanghai. Front Public Health. (2022) 10:1083144. doi: 10.3389/fpubh.2022.1083144
5. Luca R, Rifici C, Terranova A, Orecchio L, Castorina MV, Torrisi M, et al. Healthcare worker burnout during the first COVID-19 lockdown in Italy: experiences from an intensive neurological rehabilitation unit. J Int Med Res. (2023) 51:3000605231182664. doi: 10.1177/03000605231182664
6. He Y, Wu C, LeMoult J, Huang J, Zhao Y, Liang K, et al. Exploring symptom-level associations between anxiety and depression across developmental stages of adolescence: a network analysis approach. BMC Psychiatry. (2023) 23:941. doi: 10.1186/s12888-023-05449-6
7. Shang B, Chen R, Luo C, Lv F, Wu J, Shao X, et al. The relationship between alexithymia, depression, anxiety, and stress in elderly with multiple chronic conditions in China: a network analysis. Front Psychiatry. (2023) 14:1209936. doi: 10.3389/fpsyt.2023.1209936
8. Gong Y, Han T, Chen W, Dib HH, Yang G, Zhuang R, et al. Prevalence of anxiety and depressive symptoms and related risk factors among physicians in China: a cross-sectional study. PloS One. (2014) 9:e103242. doi: 10.1371/journal.pone.0103242
9. Jia L, Du Y, Chu L, Zhang Z, Li F, Lyu D, et al. Prevalence, risk factors, and management of dementia and mild cognitive impairment in adults aged 60 years or older in China: a cross-sectional study. Lancet Public Health. (2020) 5:e661–e71. doi: 10.1016/S2468-2667(20)30185-7
10. McKenzie EL, Brown PM, Mak AS, Chamberlain P. 'Old and ill': death anxiety and coping strategies influencing health professionals' well-being and dementia care. Aging Ment Health. (2017) 21:634–41. doi: 10.1080/13607863.2016.1144711
11. Liu X, Zhao W, Hu F, Hao Q, Hou L, Sun X, et al. Comorbid anxiety and depression, depression, and anxiety in comparison in multi-ethnic community of west China: prevalence, metabolic profile, and related factors. J Affect Disord. (2022) 298:381–7. doi: 10.1016/j.jad.2021.10.083
12. Yatawara C, Lim L, Chander R, Zhou J, Kandiah N. Depressive symptoms influence global cognitive impairment indirectly by reducing memory and executive function in patients with mild cognitive impairment. J Neurol Neurosurg Psychiatry. (2016) 87:1375–83. doi: 10.1136/jnnp-2016-314191
13. MacQueen GM, Memedovich KA. Cognitive dysfunction in major depression and bipolar disorder: Assessment and treatment options. Psychiatry Clin Neurosci. (2017) 71:18–27. doi: 10.1111/pcn.12463
14. Masala C, Solla P, Liscia A, Defazio G, Saba L, Cannas A, et al. Correlation among olfactory function, motors' symptoms, cognitive impairment, apathy, and fatigue in patients with Parkinson's disease. J Neurol. (2018) 265:1764–71. doi: 10.1007/s00415-018-8913-9
15. Beard C, Millner AJ, Forgeard MJ, Fried EI, Hsu KJ, Treadway MT, et al. Network analysis of depression and anxiety symptom relationships in a psychiatric sample. Psychol Med. (2016) 46:3359–69. doi: 10.1017/S0033291716002300
16. Galderisi S, Rucci P, Kirkpatrick B, Mucci A, Gibertoni D, Rocca P, et al. Interplay among psychopathologic variables, personal resources, context-related factors, and real-life functioning in individuals with Schizophrenia: A network analysis. JAMA Psychiatry. (2018) 75:396–404. doi: 10.1001/jamapsychiatry.2017.4607
17. Epskamp S, Borsboom D, Fried EI. Estimating psychological networks and their accuracy: A tutorial paper. Behav Res Methods. (2018) 50:195–212. doi: 10.3758/s13428-017-0862-1
18. Cai H, Chen MY, Li XH, Zhang L, Su Z, Cheung T, et al. A network model of depressive and anxiety symptoms: a statistical evaluation. Mol Psychiatry. (2024) 29:767–81. doi: 10.1038/s41380-023-02369-5
19. Boschloo L. Zooming in and zooming out: A network perspective on the comorbidity of depression and anxiety. J Am Acad Child Adolesc Psychiatry. (2018) 57:914–5. doi: 10.1016/j.jaac.2018.07.880
20. Jones PJ, Ma R, McNally RJ. Bridge centrality: A network approach to understanding comorbidity. Multivariate Behav Res. (2021) 56:353–67. doi: 10.1080/00273171.2019.1614898
21. Sun D, Yang D, Li Y, Zhou J, Wang W, Wang Q, et al. Psychological impact of 2019 novel coronavirus (2019-nCoV) outbreak in health workers in China. Epidemiol Infect. (2020) 148:e96. doi: 10.1017/S0950268820001090
22. Spitzer RL, Kroenke K, Williams JB, Lowe B. A brief measure for assessing generalized anxiety disorder: the GAD-7. Arch Intern Med. (2006) 166:1092–7. doi: 10.1001/archinte.166.10.1092
23. Fehnel SE, Forsyth BH, DiBenedetti DB, Danchenko N, Francois C, Brevig T. Patient-centered assessment of cognitive symptoms of depression. CNS Spectr. (2016) 21:43–52. doi: 10.1017/S1092852913000643
24. Null RCTR, Team R, Null RCT, Core Writing T, Null R, Team R, et al. R: A language and environment for statistical computing. Computing. (2011) 1:12–21.
25. Epskamp S, Cramer AOJ, Waldorp LJ, Schmittmann VD, Borsboom D. qgraph: network visualizations of relationships in psychometric data. J Stat Software. (2012) 48:367–71. doi: 10.18637/jss.v048.i04
26. Haslbeck JMB, Fried EI. How predictable are symptoms in psychopathological networks? A reanalysis of 18 published datasets. Psychol Med. (2017) 47:2767–76. doi: 10.1017/S0033291717001258
27. Haslbeck JMB, Waldorp LJ. How well do network models predict observations? On the importance of predictability in network models. Behav Res Methods. (2018) 50:853–61. doi: 10.3758/s13428-017-0910-x
28. Dalege J, Borsboom D, van Harreveld F, Waldorp LJ, van der Maas HLJ. Network structure explains the impact of attitudes on voting decisions. Sci Rep. (2017) 7:4909. doi: 10.1038/s41598-017-05048-y
29. Costenbader E, Valente TW. The stability of centrality measures when networks are sampled. Soc Networks. (2003) 25:283–307. doi: 10.1016/S0378-8733(03)00012-1
30. van Borkulo CD, van Bork R, Boschloo L, Kossakowski JJ, Tio P, Schoevers RA, et al. Comparing network structures on three aspects: A permutation test. Psychol Methods. (2023) 28:1273–85. doi: 10.1037/met0000476
31. Ma L. Depression, anxiety, and apathy in mild cognitive impairment: current perspectives. Front Aging Neurosci. (2020) 12:9. doi: 10.3389/fnagi.2020.00009
32. Dubois B, Feldman HH, Jacova C, Hampel H, Molinuevo JL, Blennow K, et al. Advancing research diagnostic criteria for Alzheimer's disease: the IWG-2 criteria. Lancet Neurol. (2014) 13:614–29. doi: 10.1016/S1474-4422(14)70090-0
33. Suarez-Gonzalez A, Crutch SJ, Franco-Macias E, Gil-Neciga E. Neuropsychiatric symptoms in posterior cortical atrophy and Alzheimer disease. J Geriatr Psychiatry Neurol. (2016) 29:65–71. doi: 10.1177/0891988715606229
34. Zhao QF, Tan L, Wang HF, Jiang T, Tan MS, Tan L, et al. The prevalence of neuropsychiatric symptoms in Alzheimer's disease: Systematic review and meta-analysis. J Affect Disord. (2016) 190:264–71. doi: 10.1016/j.jad.2015.09.069
35. Becker E, Orellana Rios CL, Lahmann C, Rucker G, Bauer J, Boeker M. Anxiety as a risk factor of Alzheimer's disease and vascular dementia. Br J Psychiatry. (2018) 213:654–60. doi: 10.1192/bjp.2018.173
36. Connors MH, Seeher KM, Crawford J, Ames D, Woodward M, Brodaty H. The stability of neuropsychiatric subsyndromes in Alzheimer's disease. Alzheimers Dement. (2018) 14:880–8. doi: 10.1016/j.jalz.2018.02.006
37. Raiker JS, Rapport MD, Kofler MJ, Sarver DE. Objectively-measured impulsivity and attention-deficit/hyperactivity disorder (ADHD): testing competing predictions from the working memory and behavioral inhibition models of ADHD. J Abnorm Child Psychol. (2012) 40:699–713. doi: 10.1007/s10802-011-9607-2
38. Thaler NS, Bello DT, Etcoff LM. WISC-IV profiles are associated with differences in symptomatology and outcome in children with ADHD. J Atten Disord. (2013) 17:291–301. doi: 10.1177/1087054711428806
39. Ehgoetz Martens KA, Szeto JY, Muller AJ, Hall JM, Gilat M, Walton CC, et al. Cognitive function in Parkinson's disease patients with and without anxiety. Neurol Res Int. (2016) 2016:6254092.
40. Cai Q, Feng H, Huang J, Wang M, Wang Q, Lu X, et al. The mental health of frontline and non-frontline medical workers during the coronavirus disease 2019 (COVID-19) outbreak in China: A case-control study. J Affect Disord. (2020) 275:210–5. doi: 10.1016/j.jad.2020.06.031
41. Cramer AO, Waldorp LJ, van der Maas HL, Borsboom D. Comorbidity: a network perspective. Behav Brain Sci. (2010) 33:137–50; discussion 50-93. doi: 10.1017/S0140525X09991567
42. Roberto N, Portella MJ, Marquie M, Alegret M, Hernandez I, Mauleon A, et al. Neuropsychiatric profile as a predictor of cognitive decline in mild cognitive impairment. Front Aging Neurosci. (2021) 13:718949. doi: 10.3389/fnagi.2021.718949
43. Ballard C, Neill D, O'Brien J, McKeith IG, Ince P, Perry R. Anxiety, depression and psychosis in vascular dementia: prevalence and associations. J Affect Disord. (2000) 59:97–106. doi: 10.1016/S0165-0327(99)00057-9
44. Steinberg M, Shao H, Zandi P, Lyketsos CG, Welsh-Bohmer KA, Norton MC, et al. Point and 5-year period prevalence of neuropsychiatric symptoms in dementia: the Cache County Study. Int J Geriatr Psychiatry. (2008) 23:170–7. doi: 10.1002/gps.1858
45. Seignourel PJ, Kunik ME, Snow L, Wilson N, Stanley M. Anxiety in dementia: a critical review. Clin Psychol Rev. (2008) 28:1071–82. doi: 10.1016/j.cpr.2008.02.008
46. Orgeta V, Qazi A, Spector A, Orrell M. Psychological treatments for depression and anxiety in dementia and mild cognitive impairment: systematic review and meta-analysis. Br J Psychiatry. (2015) 207:293–8. doi: 10.1192/bjp.bp.114.148130
47. Teng E, Tassniyom K, Lu PH. Reduced quality-of-life ratings in mild cognitive impairment: analyses of subject and informant responses. Am J Geriatr Psychiatry. (2012) 20:1016–25. doi: 10.1097/JGP.0b013e31826ce640
48. Wu L, Ren L, Li F, Shi K, Fang P, Wang X, et al. Network analysis of anxiety symptoms in front-line medical staff during the COVID-19 pandemic. Brain Sci. (2023) 13:81155. doi: 10.3390/brainsci13081155
49. Sun HL, Chen P, Feng Y, Si TL, Lam MI, Lok KI, et al. Depression and anxiety among Macau residents during the COVID-19 outbreak: A network analysis perspective. Front Psychiatry. (2023) 14:1159542. doi: 10.3389/fpsyt.2023.1159542
50. Jones PJ, Mair P, Riemann BC, Mugno BL, McNally RJ. A network perspective on comorbid depression in adolescents with obsessive-compulsive disorder. J Anxiety Disord. (2018) 53:1–8. doi: 10.1016/j.janxdis.2017.09.008
51. Grupe DW, Nitschke JB. Uncertainty and anticipation in anxiety: an integrated neurobiological and psychological perspective. Nat Rev Neurosci. (2013) 14:488–501. doi: 10.1038/nrn3524
Keywords: anxiety, cognitive impairment, comorbidity, network analysis, mental healthcare workers, PDQ-D, GAD-7
Citation: Chen R, Yan W, Shen Q, Li M, Chen M, Dong J, Wang Y, Zhao X and Cui J (2024) Network analysis of anxiety and cognitive impairment among mental healthcare workers. Front. Psychiatry 15:1393598. doi: 10.3389/fpsyt.2024.1393598
Received: 29 February 2024; Accepted: 01 August 2024;
Published: 21 August 2024.
Edited by:
Carla Masala, University of Cagliari, ItalyReviewed by:
Mohamad Golitaleb, Arak University of Medical Sciences, IranIlenia Pinna, University of Camerino, Italy
Copyright © 2024 Chen, Yan, Shen, Li, Chen, Dong, Wang, Zhao and Cui. This is an open-access article distributed under the terms of the Creative Commons Attribution License (CC BY). The use, distribution or reproduction in other forums is permitted, provided the original author(s) and the copyright owner(s) are credited and that the original publication in this journal is cited, in accordance with accepted academic practice. No use, distribution or reproduction is permitted which does not comply with these terms.
*Correspondence: Jian Cui, em14c3EyMDIzQDEyNi5jb20=; Xianxian Zhao, emhhb3hpYW42NUAxNjMuY29t