- 1Department of Dermatovenereology, Chengdu Second People’s Hospital, Chengdu, Sichuan, China
- 2Department of Gynecology, Guang’anmen Hospital, China Academy of Chinese Medical Sciences, Beijing, China
- 3Health Science Center, Yangtze University, Jingzhou, Hubei, China
Background: Rapid changes in attitudes, legality, and patterns of cannabis use (CU) underscore the importance of understanding its impact on mental health. Although links between CU and personality disorders (PDs) are documented, their causality remains uncertain.
Methods: Employing Genome-Wide Association Studies (GWAS) data, this study investigated the causal relationship between cannabis use disorder (CUD) and lifetime cannabis use (LCU) with 9 types of PD risk through Mendelian randomization (MR) analysis. The primary method was the inverse variance weighted (IVW) method, supplemented by multivariable MR to assess direct effects independent of mental, social, and substance use factors, and mediation MR to explore mediating factors.
Results: Corrections for the false discovery rate revealed significant causal associations between CUD and an increased risk of emotionally unstable PD (EUPD; ORIVW = 1.228, 95% CI 1.069–1.411), overall PD (ORIVW = 1.186, 95% CI 1.065–1.321), and schizoid PD (SPD; ORIVW = 1.644, 95% CI 1.131–2.390). Mediation analysis identified schizophrenia (SCZ), major depressive disorder (MDD), neuroticism, and smoking initiation (SmkInit) as shared mediating factors between CUD and both EUPD and overall PD, with an additional mediating factor, household income (HI), specific to the CUD-to-overall PD pathway. In contrast, no mediating factors were found between CUD and SPD. Notably, a bidirectional causal relationship was observed between overall PD and CUD (ORIVW = 1.399, 95% CI 1.033–1.895). Suggestive evidence indicated a causal link between lifetime cannabis use (LCU) and overall PD risk (ORIVW = 1.074, 95% CI 1.008–1.146).
Conclusion: This study offers new insights into the potential impact of CU on the development and progression of various PDs, laying the groundwork for targeted interventions to mitigate its effects on mental health.
Introduction
Personality disorders (PD) are characterized as mental health conditions marked by enduring patterns of maladaptive behavior, cognition, and internal experience (1). Globally prevalent, the average prevalence rate of PD is 7.8%, with higher rates observed in developed countries (2). In general, personality traits represent patterns of thinking, perceiving, reacting, and relating that are relatively stable over time. PD exist when these traits become so pronounced, rigid, and maladaptive that they impair work and/or interpersonal functioning. PD in general are pervasive, enduring patterns of thinking, perceiving, reacting, and relating that cause significant distress or functional impairment, and usually start to become evident during late adolescence or early adulthood, it is shaped through interaction between genes and environment. Individuals with PD frequently encounter interpersonal difficulties, substance use disorder (SUD), self-harming behaviors, diminished quality of life, and often endure significant emotional distress (3), with prevalence rates among psychiatric patients ranging from 40% to 60% (1, 3, 4). The biopsychosocial model of mental disorder takes into account the biological, psychological, and social factors that contribute to the development of the diseases (disorder). It emphasizes the importance of considering the influence of social and behavioral factors on biological disease/condition. This burden not only impacts the individuals diagnosed but also their families and the broader healthcare system. Typically identified during adolescence or adulthood, though sometimes as early as childhood, the behavioral patterns associated with PD pose significant challenges (5, 6). However, prevention remains challenging due to the complex interplay of genetic, environmental, and psychological factors influencing its onset. Consequently, identifying and understanding the risk factors for PD is crucial for developing effective prevention strategies.
Cannabis, primarily composed of Δ-9 tetrahydrocannabinol (THC) and cannabidiol (CBD), is the most widely used illicit drug worldwide (7). In recent years, with rapid changes in attitudes, legal status, and patterns of cannabis use (CU), several countries have legalized recreational CU, which is notably prevalent among adolescents. Adolescence represents a critical period for the development of substance-related psychiatric disorders and other comorbid mental health conditions, such as PD (8). Research indicates that cannabis use disorder (CUD) is the most common SUD among adolescents receiving treatment for SUDs (9). Numerous observational studies have identified an association between CU or CUD and an increased incidence of PD and certain subtypes, where mental health factors, socio-economic factors, and other substance uses may act as mediating factors, creating a bridging effect (10–16). Studies have found that cannabis dependence is associated with higher rates of PD and deficits in social support. The potential interrelationship between interpersonal dysfunction and CUD and the correlation between PD and CUD deserve further study (15). A twin study in the Norwegian general population found that genetics may play a role in CU, CUD, and some PD traits, but not others (12). A study of the association between PD traits and problematic CU in adolescents showed that multiple regression analyses showed that PD traits largely explained the variance in problematic CU symptoms. After adjusting for anxiety and depression symptoms, schizotypic and borderline personality traits were positively associated with symptoms of problematic CU (17). Hasin et al. reported associations between CUD and all PD, with strongest associations reported between CUD and dependent or antisocial PD. Highest proportion of cannabis user were in those with a Cluster B PD (antisocial; borderline; histrionic; narcissistic) compared to Cluster A (paranoid; schizoid; schizotypal), or Cluster C (avoidant; dependent; antisocial) (18). However, there were no strong associations between CU and other later psychiatric disorders, suggesting that cannabis users with a PD may only be at significantly elevated risk for additional substance use disorder. Individuals with any PD are significantly more likely to have a past-year CUD than those without. Borderline PD has the strongest association, compared to antisocial and schizotypal, and these findings are consistent with a twin study, as well as numerous other studies. Genetic and environmental correlations between PD traits and CU suggest that genetic risks in borderline and antisocial PD traits accounted for significant variance in CU, however not for schizoid or dependent PD traits (19). There is no doubt that there is an association between PD and substance use/addiction (CU/addiction). People with PD are at increased risk of other mental disorders, including substance use and addiction. In addition to this, CU in children and adolescents can be a “symptom” of other disorders. However, due to inherent limitations in traditional research, the causal relationship has not been fully established, and there is a lack of systematic research causally linking CU with PD and all its subtypes.
Although randomized controlled trials (RCTs) are considered the gold standard for establishing causal relationships, large-scale RCTs are often hindered by high costs, time and resource intensity, and sometimes impracticality or ethical concerns. Mendelian randomization (MR), a genetic analysis technique akin to RCTs, has emerged as an alternative research method capable of overcoming these limitations. It utilizes genetic variations identified in Genome-Wide Association Studies (GWAS) as instrumental variables (IVs) to determine the causal impact of risk factors (or exposures) on outcomes (20). Given that alleles related to exposures are randomly assigned at birth (21), MR can circumvent issues of confounding and reverse causation, which frequently challenge traditional epidemiological studies (22). This study aims to employ MR analysis to explore the bidirectional causal relationship between CU and PD, offering insights that could inform prevention and intervention strategies.
Materials and methods
Study design
This study utilizes summary-level data from publicly available GWAS to conduct MR analysis. To investigate the causal relationship between CU and PD, an initial bidirectional univariable MR (UVMR) analysis was performed, followed by a multivariable MR (MVMR) to assess the independence of the causal association from three major confounding factors: mental, social, and substance use. Further mediation MR analysis explored whether confounding phenotypes exhibit a bridging effect. The exposure IVs employed for analysis adhere to the three core assumptions of MR: i) the genetic instruments designated as IVs must be strongly associated with the exposure; ii) these genetic instruments should not be confounded with any potential confounding factors; iii) the influence of the genetic instruments on the outcome must be mediated solely through their interaction with the exposure, not through other outcome phenotypes (23). A flowchart of the MR analysis process is presented in Figure 1.
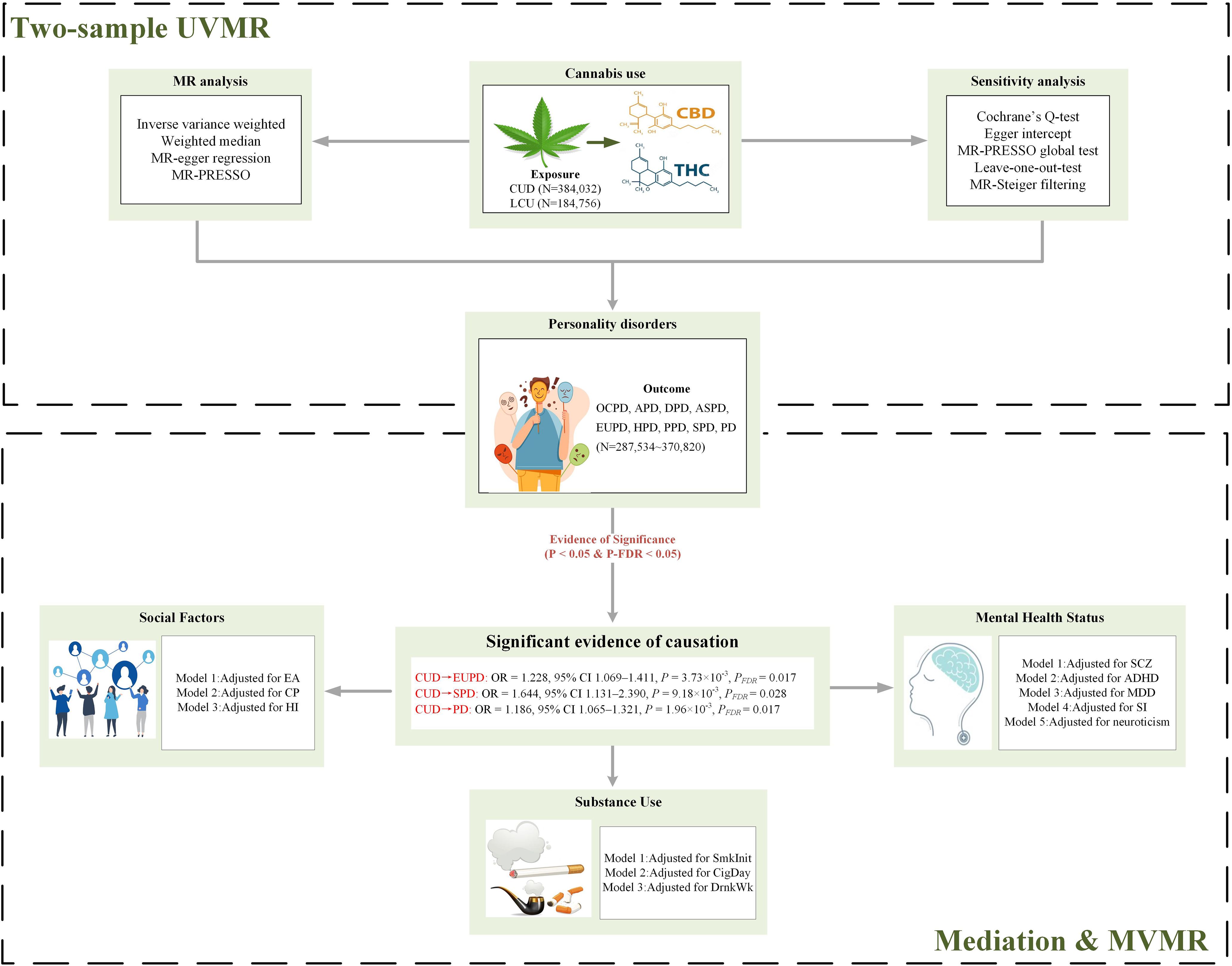
Figure 1. Flowchart of Mendelian randomization analysis. PD, personality disorders; OCPD, anankastic (obsessive–compulsive) personality disorder; APD, anxious personality disorder; DPD, dependent personality disorder; ASPD, antisocial personality disorder; EUPD, emotionally unstable personality disorder; HPD, histrionic personality disorder; PPD, paranoid personality disorder; SPD, schizoid personality disorder; CUD, cannabis use disorder; LCU, lifetime cannabis use; SCZ, schizophrenia; ADHD, attention deficit hyperactivity disorder; CigDay, cigarettes per day; SmkInit, smoking initiation; DrnkWk, alcoholic drinks per week; EA, education attainment; CP, cognitive performance; HI, household income; SI, social Isolation; MDD, major depressive disorder; MR, Mendelian randomization; MVMR, multivariable MR; UVMR, univariable MR; THC, Δ-9 tetrahydrocannabinol; CBD, cannabidiol; FDR, false discovery rate; MR-PRESSO, MR Pleiotropy Residual Sum and Outlier.
Data source
As a secondary analysis of publicly available data, this study did not require further ethical review. Ethical approval, participant informed consent, and eligibility criteria for each original GWAS can be found in their respective publications. CU encompasses CUD and lifetime cannabis use (LCU), derived from the Psychiatric Genomics Consortium (PGC) (24) and the International Cannabis Consortium (ICC) (25), respectively. Nine PD phenotypes were obtained from the FinnGen consortium’s R9 version data (26). Confounding and mediating phenotypes for further analysis were categorized into three major groups: mental health, social factors, and substance use. Schizophrenia (SCZ), attention deficit hyperactivity disorder (ADHD), and major depressive disorder (MDD) were sourced from PGC (27–29); social isolation (SI), neuroticism, and household income (HI) from the UK biobank (UKB) (30); educational attainment (EA) and cognitive performance (CP) from the Social Science Genetic Association Consortium (SSGAC) (31); smoking initiation (SmkInit), cigarettes per day (CigDay), and drinks per week (DrnkWk) from the GWAS and Sequencing Consortium of Alcohol and Nicotine use (GSCAN) (32). All sample populations originated from Europe, with no sample overlap as determined through computation. Table 1 presents all datasets used for analysis, including diagnostic criteria, sample sizes, and sources.
Selection of genetic instrumental variables
This MR study implemented a rigorous screening process for single nucleotide polymorphisms (SNPs) with the following criteria: (1) SNPs were initially screened at the genome-wide significance level (P < 5×10-8) for association with the exposure. However, due to the absence of genome-wide significant SNPs for CUD, a more relaxed threshold (P < 5×10-7) was adopted based on previous MR studies on CUD (33). In reverse MR analysis, an even more relaxed threshold (P < 1×10-5) was used to ensure a sufficient number of IVs, thereby enhancing statistical power. (2) A stringent linkage disequilibrium (LD) clumping method (r2 < 0.001 within 10,000 kb window base on 1000 Genomes Phase 3) was employed to identify independent SNP loci, minimizing bias due to LD. (3) MR-Steiger filtering was utilized to exclude SNPs with potential reverse causation (34). (4) The F-statistic [F=((n −k−1)/k)(R2/(1− R2))], where R2 is the variance in exposure accounted for by the SNPs, k=1 reflects a single SNP analysis, and N represents the GWAS sample size) for all SNPs was required to be greater than 10 to avoid bias from weak IVs (35). (5) Proxy SNPs (r2 > 0.8) were not used to ensure the precision of the results.
Statistical analyses
Primary MR analysis
For the UVMR study, the Wald ratio test was employed initially for individual IVs. Following this, the multiplicative random-effects inverse-variance-weighted (IVW) method was implemented for the causative assessment of multiple IVs (comprising 2 or more). This approach was further enhanced by the incorporation of both MR-Egger and weighted median techniques. The weightage in IVW bears a direct relation to each SNP’s Wald ratio estimate and an inverse correlation to the variance estimate of each SNP’s Wald ratio (36). When all genetic markers are adjudged valid, IVW provides consistent and efficient estimates. Conversely, the weighted median method stands out when over half of the genetic markers are deemed questionable, and the MR-Egger approach is opted for when the entirety of genetic markers is deemed untenable (37). A stringent adjustment for multiple comparisons was implemented via the false discovery rate (FDR). Following this adjustment, a P-value falling below 0.05 was considered indicative of a significant causal relationship. However, instances where the raw P-value was below 0.05, but the FDR-adjusted P-value exceeded this threshold, were merely regarded as tentative.
Given the potential confounding impact of factors on the path from exposure to outcome, subsequent MVMR analyses were orchestrated. The aim was to accurately quantify the direct causative repercussion of exposure on the result. When juxtaposed with the UVMR paradigm, the primary supposition of MVMR revolves around genetic variability associating with one or more exposures, while the succeeding assumptions harmonize with the UVMR framework (38). To further explore the potentially modifiable mediating factors between cannabis use and PD, we conducted a two-step mediation MR analysis. This approach extends traditional MR by incorporating mediation pathways, leveraging genetic variants as instrumental variables to minimize confounding and reverse causality often encountered in observational mediation studies. In this framework, three core causal effects were considered: (1) the direct effect of cannabis use on PD, independent of the mediator; (2) the indirect effect transmitted through the mediator; and (3) the total effect, representing the combined influence of both direct and indirect pathways. The proportion of mediation was quantified by the ratio of the indirect effect to the total effect, which reflects the extent to which the mediator accounts for the observed association. This strategy enhances causal inference and elucidates biological pathways, offering valuable insights for the development of precise and targeted interventions.
Power calculation
Given that most genetic variants predict only a small fraction of phenotypic variance, statistical power is considered one of the primary challenges in MR study (39). This MR study further calculates R² (the proportion of explained variance) and statistical power. R² was calculated employing the formula 2×MAF×(1-MAF)×beta², wherein MAF represents the minor allele frequency of each specified SNP (40). These cumulative values yielded the coefficient crucial for the estimation of statistical power. The assessment of statistical power was conducted using the mRnd platform, available at (https://shiny.cnsgenomics.com/mRnd/) (39).
Sensitivity analysis
Within the framework of the UVMR analysis, several tests were conducted to validate rigor and authenticity. The heterogeneity of the selected genetic variants was assessed using Cochran’s Q test, wherein a P-value of < 0.05 indicated pronounced discrepancies among the scrutinized SNPs (41). Employing the MR-Egger regression (42), the investigation discerned the potential for directional pleiotropy within the MR context. The MR-Egger’s intercept, bearing a P-value of < 0.05, signified consequential directional pleiotropy (43). However, causal estimates obtained through the MR-Egger method are subject to inherent limitations that could introduce bias and potentially inflate the type I error rate, thereby requiring careful interpretation.The MR Pleiotropy Residual Sum and Outlier (MR-PRESSO) approach was deployed to identify probable outliers and to delve into horizontal pleiotropy, which was inferred when the global P-value was less than 0.05 (44). By excluding such outliers, the refinement of our data correction was realized. An ensuing leave-one-out analysis shed light on the impact exerted by singular SNPs on the collective outcomes (45).
Data analysis software and packages
All statistical analyses were performed using R software (version 4.2.2), TwoSampleMR (version 0.5.6), MR-PRESSO (version 1.0), MVMR (version 0.4), MendelianRandomization (version 0.9.0) were analyzed.
Results
Genetic instrument selection and calculation of power statistics
Regarding the CU phenotype, this study initially acquired 12 SNPs related to CUD from the PGC and 8 SNPs related to LCU from the ICC. Through filtering, the number of IVs utilized in both forward and reverse UVMR analyses ranged from 2 to 47, explaining genetic variance from 0.59% to 36.18% (Supplementary Table S1). All IVs were filtered through the Steiger test, fulfilling the III assumption of MR analysis. Additionally, MR-PRESSO analysis was strictly employed to eliminate outliers, reducing bias introduced by horizontal pleiotropy. The F-statistics calculated for each IV exceeded 10, with averages ranging from 239 to 37,077, significantly minimizing bias due to weak instrumental variables. Moreover, the study possessed sufficient statistical power to detect positive causal associations. Specifically, in forward UVMR analyses, with ORs of 1.228, 1.644, and 1.186, we had statistical powers of 92%, 94%, and 95%, respectively, to detect associations of CUD with emotionally unstable PD (EUPD), schizoid PD (SPD), and PD. With an OR of 1.399, there was an 85% statistical power to detect an association of LCU with PD. In reverse UVMR analyses, an OR of 1.074 provided a 95% statistical power to detect an association of PD with CUD (Supplementary Table S1). This further strengthens the robustness of the causal associations.
Causal association of CU with PD and their subtypes
In the forward UVMR analysis (Figure 2), after correction for FDR, the IVW analysis revealed three significant and one suggestive causal association. Specifically, a genetic predisposition to CUD was significantly associated with an 18.6% increase in the incidence of PD (OR = 1.186, 95% CI 1.065–1.321, P = 1.96×10-3, PFDR = 0.017) per standard deviation (SD) increase. LCU also provided suggestive evidence of an increase in PD incidence (OR = 1.399, 95% CI 1.033–1.895, P = 0.030, PFDR = 0.270). Additionally, significant causal associations were found between CUD and an increase in the incidence rates of EUPD (OR = 1.228, 95% CI 1.069–1.411, P = 3.73×10-3, PFDR = 0.017) and SPD (OR = 1.644, 95% CI 1.131–2.390, P = 9.18×10-3, PFDR = 0.028) by 22.8% and 64.4%, respectively, with the weighted median method providing consistent results (EUPD: OR = 1.327, 95% CI 1.083–1.627, P = 0.006; SPD: OR = 1.767, 95% CI 1.098–2.845, P = 0.019). However, no causal associations were found between CUD or LCU and other PD subtypes (P > 0.05 & PFDR > 0.05) (Supplementary Table S2).
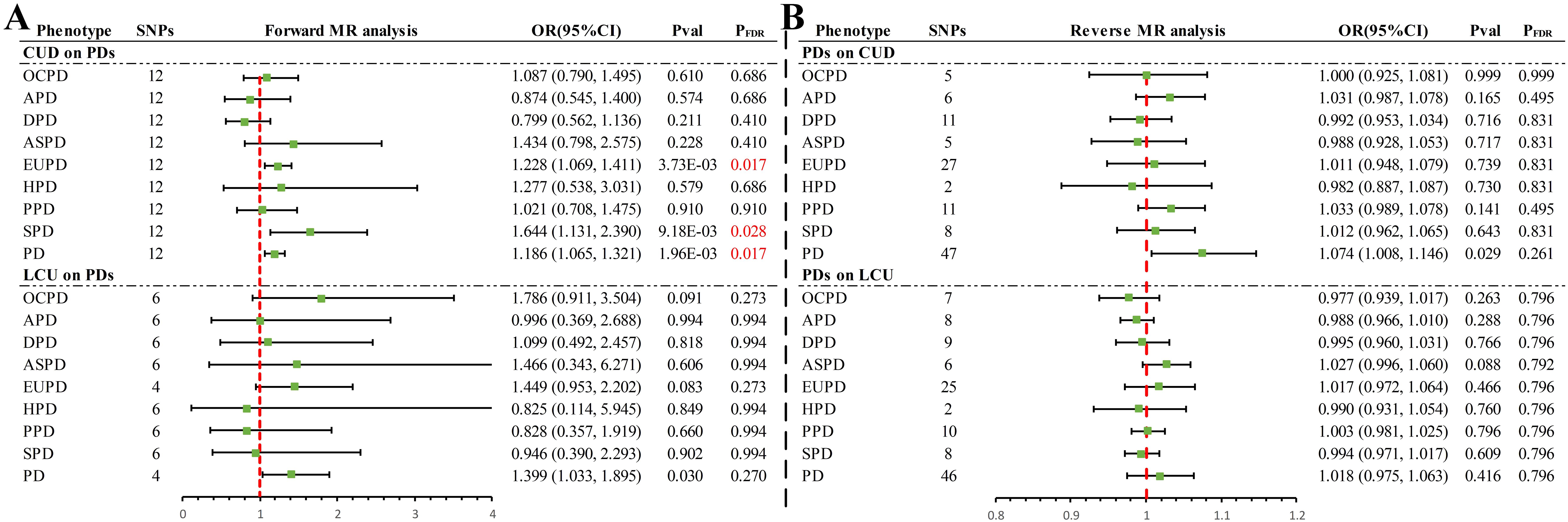
Figure 2. Summary of results of IVW methods for forward and reverse univariate Mendelian randomization analysis. (A) Forward MR analysis (B) Reverse MR analysis. PD, personality disorders; OCPD, anankastic (obsessive–compulsive) personality disorder; APD, anxious personality disorder; DPD, dependent personality disorder; ASPD, antisocial personality disorder; EUPD, emotionally unstable personality disorder; HPD, histrionic personality disorder; PPD, paranoid personality disorder; SPD, schizoid personality disorder; CUD, cannabis use disorder; LCU, lifetime cannabis use; SNP, single nucleotide polymorphisms; MR, Mendelian randomization; FDR, false discovery rate.
In the reverse UVMR analysis (Figure 2), a bidirectional causal association was identified between CUD and PD. Specifically, a genetically predicted increase of one SD in PD was suggestively associated with a 7.4% increase in the incidence of CUD (OR = 1.074, 95% CI 1.008–1.146, P = 0.029, PFDR = 0.261). No causal associations were found between other PD phenotypes and CUD or LCU (P > 0.05 & PFDR > 0.05) (Supplementary Table S2).
Sensitivity analyses revealed no evidence of horizontal pleiotropy via MR-Egger (P > 0.05) (Supplementary Table S3). With few exceptions (Supplementary Table S3), Cochran’s Q statistic detected no heterogeneity (P > 0.05), and MR-PRESSO found no evidence of horizontal pleiotropy (P > 0.05), excluding two outliers (rs10085617, rs9773390) in the analysis of LCU’s effect on EUPD and PD. Leave-one-out analysis indicated that the causal association in the analysis of LCU’s effect on PD was driven by a single SNP (rs2875907) (Supplementary Figure S1), suggesting that the results should be considered suggestive evidence. The remaining causal associations remained robust, unaffected by any single SNP (Supplementary Figure S1–S4). Funnel plots-maintained symmetry (Supplementary Figure S5–S8). Scatter plots clearly illustrated the direction of each analysis (Supplementary Figure S9–S12), while forest plots provided the contribution of each IV (Supplementary Figure S13–S16).
Adjusting for Confounding Factors and Conducting Mediation MR Analysis
In the UVMR analysis, significant causal associations warranted further investigation through MVMR to assess whether the causal effects of CUD on PD and its subtypes were independent of three major confounding factors (Figure 3). Within the realm of mental health factors, evidence was found for a direct impact of CUD genetic predisposition on the incidence of EUPD (P = 0.017), independent of ADHD. Regarding social factors, evidence was discovered for a direct influence of CUD genetic susceptibility on the incidence rates of EUPD and PD, independent of EA and CP (P < 0.05). Additionally, direct evidence of CUD’s impact on EUPD, independent of HI (P = 0.026), was identified. After adjusting in the remaining models, the causal relationship between CUD and PD and its subtypes was no longer significant (P > 0.05).
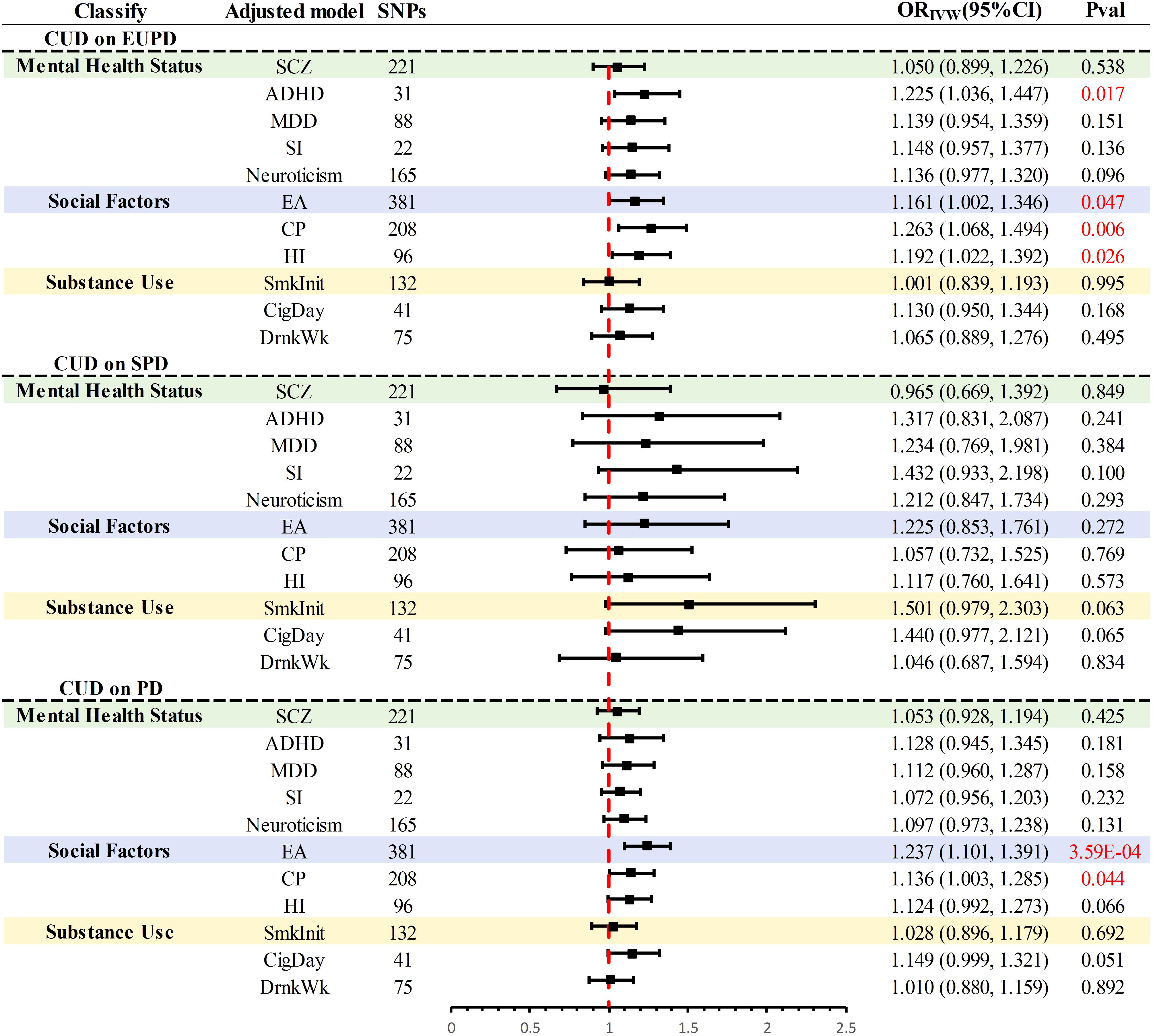
Figure 3. Summary of multivariate MR results for significant causal association. PD, personality disorders; EUPD, emotionally unstable personality disorder; SPD, schizoid personality disorder; CUD, cannabis use disorder; SCZ, schizophrenia; ADHD, attention deficit hyperactivity disorder; CigDay, cigarettes per day; SmkInit, smoking initiation; DrnkWk, alcoholic drinks per week; EA, education attainment; CP, cognitive performance; HI, household income; SI, social Isolation; MDD, major depressive disorder; MR, Mendelian randomization.
Further mediation MR analysis (Supplementary Table S4, Supplementary Figure S17) indicated common mediating factors in the analysis of CUD’s effects on EUPD and PD, including SCZ, MDD, neuroticism, and SmkInit, with neuroticism explaining the largest mediation effect (EUPD: 21.50%; PD:27.95%) (Table 2). Additionally, a mediating effect of HI (Mediation effect: 15.33%) was found in the analysis of CUD’s impact on PD (Table 2).
Discussion
This study conducted a comprehensive MR analysis to explore the genetic predisposition of CU and its causal relationship with various PD risks. The model genetically predicted that CUD would increase the risk of EUPD, SPD, and overall PD. On the contrary, overall PD can increase the risk of CUD, and there was a two-way causal relationship between CUD and overall PD. In addition, genetic evidence suggested that LCU patients get a higher risk of overall PD, while no clear causal relationship has been found between overall PD and LCU. Further analysis pinpointed five mediating factors. The implications of these findings will be discussed from the perspectives of neurobiological mechanisms and social factors.
The primary active component of cannabis, THC, affects key neurotransmitter systems in the brain by acting on cannabinoid receptors, especially CB1 receptors. This action influences emotion regulation, stress response, reward perception, and social behavior, as indicated by extensive research (46, 47). Long-term or heavy CU can lead to dysfunction of these systems, cerebellar hypoactivation, and structural brain changes, particularly in the white matter, prefrontal cortex, hippocampus, and amygdala (48–51). Such changes may trigger issues with emotional stability, social skills, and impulse control, increasing the risk of developing disorders like EUPD and SPD. Further mediation MR analysis revealed several mental health mediating factors. SCZ and MDD reflect a broader spectrum of psychological vulnerability, exhibiting a complex bidirectional relationship with CU. Numerous MR studies have established a causal link between CU and an increased incidence of these psychiatric conditions (52–54), while our reverse MR analysis further reveals a bidirectional causal relationship between CUD and PD. This reciprocal relationship suggests that individuals with these conditions may be more inclined to use cannabis as a form of self-medication. Inappropriate treatment may lead to cannabis dependence, exacerbating mental health issues and creating a vicious cycle. A systematic review by Jerome Sarris’ team provides consistent evidence and emphasizes the need for clinicians to consider a range of prescription and occupational safety factors (55). Additionally, research by Kaeli Zimmermann’s team indicates that individuals with high neuroticism are more likely to use cannabis to alleviate negative emotions (56). Frequent cannabis users show impaired emotional reevaluation and increased neural activity in areas associated with emotion regulation, indirectly increasing the risk of PDs. Therefore, interventions targeting these mediating factors can effectively reduce PD risk from a genetic perspective, promoting mental health and social well-being.
Dongze Chen’s MR analysis has established a bidirectional causal association between CU and education, highlighting the potential adverse effects of cannabis on individual educational and occupational achievements (33). This association suggests an increase in conflicts with family and society, leading to psychological stress and social isolation, particularly during adolescence (57). Notably, the level of HI reflects the socio-economic status of individuals and families, where lower income may limit resources for stress management and increase the psychological burden of living in disadvantaged socio-economic conditions, thereby elevating the risk of developing CUD and PD. Correction of SI and HI factors in MVMR analysis resulted in a non-significant causal relationship between CUD and PD, further validating these factors as critical in the development of PD. Smoking behavior may not only exist as a habit but also reflect deeper psychological and social dynamics. Adolescence, a crucial period for personality formation, social-psychological development, and self-identity exploration, may predispose individuals to experiment with substances such as cannabis and initiate smoking as a means to cope with social pressures, emotional disturbances, or identity exploration. This perspective aligns with Michael Windle’s emphasis on how adolescent delinquency, academic performance, and stressful life events can predict these trajectories, potentially increasing the risk of PD (58). Our mediation analysis underscores the mediating effect of smoking initiation, providing consistent evidence. Thus, smoking behavior and household income levels may be bridges connecting CUD and PD, both by increasing the likelihood of CU and through shared underlying mental health issues.
LCU, defined as having used cannabis at least once without implying continuous or frequent use, contrasts with CUD, which represents a severe dependency indicating regular, intensive, and prolonged consumption of cannabis. This distinction explains the differential outcomes of LCU and CUD on PD, where EUPD includes impulsive (F60.30) and borderline (F60.31) PDs. Hence, this MR study is consistent with previous epidemiological research, marking the first identification of a causal association between genetically predicted CUD and increased risk of SPD, highlighting the dose-response relationship previously noted in research on CU and mental illness (59). However, the absence of a positive causal association with other PD subtypes, beyond the nature of LCU versus CUD, may be attributed to three potential reasons: limitations in sample size for different PD subtype causality studies, individual responses to cannabis and PD development influenced by genetic factors, and the association of different PDs with distinct neurotransmitter systems and neural circuits in the brain. Further research and analysis are needed in the future.
Individual differences in the liability to CU and CUD appear to be linked to genetic risks correlated with antisocial and borderline PD traits. A cross-sectional survey of 1,419 twins at the Norwegian Institute of Public Health examined which combination of PDs trait scores best predicted CU and CUD, and estimated the magnitude and significance of genetic and environmental risks in PD traits shared with CU and CUD. The results found that genetic risk for antisocial and borderline PD traits explained 32-60% of the total variance of CU and CUD. Among them, the genetic risk of antisocial PD traits explained CU (56%) and CUD (43%). Borderline PD traits explained CU (32%) and CUD (60%). It can be seen that individual differences in cannabis use susceptibility and cannabis use disorder appear to be associated with genetic risk associated with antisocial and borderline personality disorder traits (12). These phenotypes and genotypes were found to have a strong impact on lifetime alcohol use and alcohol use disorders, suggesting that alcohol and CU and abuse share many of the same genetic and environmental risk factors (12). In results reported by Norwegian twins, we found that borderline and antisocial PD trait scores were also the strongest associations over time and across time for phenotypic and genotypic susceptibility to lifetime drinking and alcohol use disorders (60). This suggests that lifetime use and abuse of alcohol and cannabis are indexed by many of the same genetic and environmental risk factors. It has been hypothesized that early (adolescent) alcohol abuse and co-use of cannabis are causal risk factors for adult antisocial/psychotic personality by altering brain structure and function, as both usually occur simultaneously (61). Our findings appear to provide partial support for this hypothesis, suggesting a two-way relationship between alcohol/cannabis use and antisocial PD.
This study presents several strengths, notably being the first comprehensive MR analysis to establish the causal relationship between CU and PD. This is the first study to investigate all 9 PDs and explore associations with CU and use disorder within the context of genetics. It employs a range of sensitivity analyses to ensure robust results and utilizes MVMR and mediation MR analyses to explore multiple mediating factors, offering a broader perspective. However, the study also has limitations, including a population predominantly from Europe, reducing the diversity of the sample. Additionally, reliance on summary-level GWAS data limits subgroup analysis and further exploration of dose-response relationships.
Conclusion
This MR study offers new insights into the potential impact of CU on the development and progression of various PDs, laying the groundwork for targeted interventions to mitigate its effects on mental health.
Data availability statement
The original contributions presented in the study are included in the article/Supplementary Material. Further inquiries can be directed to the corresponding author/s.
Author contributions
YN: Conceptualization, Data curation, Investigation, Methodology, Software, Writing – original draft, Writing – review & editing. JL: Formal analysis, Supervision, Validation, Writing – original draft, Writing – review & editing. ZT: Visualization, Writing – original draft. YZ: Supervision, Writing – original draft, Writing – review & editing. YF: Funding acquisition, Project administration, Writing – review & editing.
Funding
The author(s) declare that financial support was received for the research and/or publication of this article. Construction of standardized rosacea comprehensive prevention and treatment system based on regional medical model and promotion and application of diagnosis and treatment technology of Science and Technology Department of Sichuan Province (Grant No. 2022YFQ0055).
Acknowledgments
We thank all GWAS participants and investigators for making the summary statistics data publicly available.
Conflict of interest
The authors declare that the research was conducted in the absence of any commercial or financial relationships that could be construed as a potential conflict of interest.
The reviewer LL declared a shared parent affiliation with the author ZT to the handling editor at the time of review.
Publisher’s note
All claims expressed in this article are solely those of the authors and do not necessarily represent those of their affiliated organizations, or those of the publisher, the editors and the reviewers. Any product that may be evaluated in this article, or claim that may be made by its manufacturer, is not guaranteed or endorsed by the publisher.
Supplementary material
The Supplementary Material for this article can be found online at: https://www.frontiersin.org/articles/10.3389/fpsyt.2025.1411587/full#supplementary-material
References
1. Saß H. Personality Disorders. In: Smelser NJ and Baltes PB, editors. International Encyclopedia of the Social & Behavioral Sciences. Pergamon, Oxford (2001). p. 11301–8. doi: 10.1016/B0-08-043076-7/03763-3
2. Winsper C, Bilgin A, Thompson A, Marwaha S, Chanen AM, Singh SP, et al. The prevalence of personality disorders in the community: a global systematic review and meta-analysis. Br J Psychiatry. (2020) 216:69–78. doi: 10.1192/bjp.2019.166
3. Tyrer P, Reed GM, and Crawford MJ. Classification, assessment, prevalence, and effect of personality disorder. Lancet. (2015) 385:717–26. doi: 10.1016/S0140-6736(14)61995-4
4. Beckwith H, Moran PF, and Reilly J. Personality disorder prevalence in psychiatric outpatients: a systematic literature review. Pers Ment Health. (2014) 8:91–101. doi: 10.1002/pmh.1252
5. Association AP. Diagnostic and Statistical Manual of Mental Disorders (DSM-5®). Arlington: American Psychiatric Publishing (2013). p. 1505.
6. Ullrich S, Farrington DP, and Coid JW. Dimensions of DSM-IV personality disorders and life-success. J Pers Disord. (2007) 21:657–63. doi: 10.1521/pedi.2007.21.6.657
7. Urits I, Gress K, Charipova K, Li N, Berger AA, Cornett EM, et al. Cannabis use and its association with psychological disorders. Psychopharmacol Bull. (2020) 50:56–67.
8. Jordan CJ and Andersen SL. Sensitive periods of substance abuse: Early risk for the transition to dependence. Dev Cognit Neurosci. (2017) 25:29–44. doi: 10.1016/j.dcn.2016.10.004
9. Cousijn J, van Benthem P, van der Schee E, and Spijkerman R. Motivational and control mechanisms underlying adolescent cannabis use disorders: A prospective study. Dev Cognit Neurosci. (2015) 16:36–45. doi: 10.1016/j.dcn.2015.04.001
10. Trovini G, Amici E, Bauco P, Matrone M, Lombardozzi G, Giovanetti V, et al. A comprehensive evaluation of adverse childhood experiences, social-emotional impairments, and neurodevelopmental disorders in cannabis-use disorder: Implications for clinical practice. Eur Psychiatry. (2023) 66:e77. doi: 10.1192/j.eurpsy.2023.2436
11. Low A, Stiltner B, Nunez YZ, Adhikari K, Deak JD, Pietrzak RH, et al. Association patterns of antisocial personality disorder across substance use disorders. medRxiv. (2023). 14(1):346. doi: 10.1101/2023.09.15.23295625
12. Gillespie NA, Aggen SH, Neale MC, Knudsen GP, Krueger RF, South SC, et al. Associations between personality disorders and cannabis use and cannabis use disorder: a population-based twin study. Addiction. (2018) 113:1488–98. doi: 10.1111/add.14209
13. Vaziri-harami R, Sefidgar S, Vaziri-harami S, and Samani N. Borderline personality disorder among cannabis users and its association with demographic variables. Psiquiatr Biol. (2022) 29:100366. doi: 10.1016/j.psiq.2022.100366
14. Brown QL, Shmulewitz D, Sarvet AL, Young-Wolff KC, Howard T, and Hasin DS. Cannabis use, cannabis use disorder and mental health disorders among pregnant and postpartum women in the US: A nationally representative study. Drug Alcohol Depend. (2023) 248:109940. doi: 10.1016/j.drugalcdep.2023.109940
15. Cougle JR, McDermott KA, Hakes JK, and Joyner KJ. Personality disorders and social support in cannabis dependence: A comparison with alcohol dependence. J Affect Disord. (2020) 265:26–31. doi: 10.1016/j.jad.2020.01.029
16. Burke CW, Firmin ES, Lanni S, Ducharme P, DiSalvo M, and Wilens TE. Substance use disorders and psychiatric illness among transitional age youth experiencing homelessness. JAACAP Open. (2023) 1:3–11. doi: 10.1016/j.jaacop.2023.01.001
17. Chabrol H, Melioli T, and Goutaudier N. Association between personality disorders traits and problematic cannabis use in adolescents. Subst Use Misuse. (2015) 50:552–6. doi: 10.3109/10826084.2014.984851
18. Hasin D. Personality disorders and the 3-year course of alcohol, drug, and nicotine use disorders. Arch Gen Psychiatry. (2011) 68:1158. doi: 10.1001/archgenpsychiatry.2011.136
19. Hasin D and Walsh C. Cannabis use, cannabis use disorder, and comorbid psychiatric illness: A narrative review. JCM. (2020) 10:15. doi: 10.3390/jcm10010015
20. Tin A and Kottgen A. Mendelian randomization analysis as a tool to gain insights into causes of diseases: A primer. J Am Soc Nephrol. (2021) 32:2400–7. doi: 10.1681/ASN.2020121760
21. Davies NM and Michael V Holmes GDS. Reading Mendelian randomisation studies: a guide, glossary, and checklist for clinicians. (2018) 362:k601. doi: 10.1136/bmj.k601
22. Tashman KC, Cui R, O’Connor LJ, Neale BM, and Finucane HK. Significance testing for small annotations in stratified LD-Score regression. medRxiv. (2021). doi: 10.1101/2021.03.13.21249938
23. Lawlor DA, Harbord RM, Sterne JAC, Timpson N, and Davey Smith G. Mendelian randomization: Using genes as instruments for making causal inferences in epidemiology. Stat Med. (2008) 27:1133–63. doi: 10.1002/sim.3034
24. Johnson EC, Demontis D, Thorgeirsson TE, Walters RK, Polimanti R, Hatoum AS, et al. A large-scale genome-wide association study meta-analysis of cannabis use disorder. Lancet Psychiatry. (2020) 7:1032–45. doi: 10.1016/S2215-0366(20)30339-4
25. Pasman JA, Verweij KJH, Gerring Z, Stringer S, Sanchez-Roige S, Treur JL, et al. GWAS of lifetime cannabis use reveals new risk loci, genetic overlap with psychiatric traits, and a causal influence of schizophrenia. Nat Neurosci. (2018) 21:1161–70. doi: 10.1038/s41593-018-0206-1
26. Kurki MI, Karjalainen J, Palta P, Sipilä TP, Kristiansson K, Donner KM, et al. FinnGen provides genetic insights from a well-phenotyped isolated population. Nature. (2023) 613:508–18. doi: 10.1038/s41586-022-05473-8
27. Trubetskoy V, Pardiñas AF, Qi T, Panagiotaropoulou G, Awasthi S, Bigdeli TB, et al. Mapping genomic loci implicates genes and synaptic biology in schizophrenia. Nature. (2022) 604:502–8. doi: 10.1038/s41586-022-04434-5
28. Demontis D, Walters RK, Martin J, Mattheisen M, Als TD, Agerbo E, et al. Discovery of the first genome-wide significant risk loci for attention deficit/hyperactivity disorder. Nat Genet. (2019) 51:63–75. doi: 10.1038/s41588-018-0269-7
29. Howard DM, Adams MJ, Clarke T-K, Hafferty JD, Gibson J, Shirali M, et al. Genome-wide meta-analysis of depression identifies 102 independent variants and highlights the importance of the prefrontal brain regions. Nat Neurosci. (2019) 22:343–52. doi: 10.1038/s41593-018-0326-7
30. Conroy MC, Lacey B, Bešević J, Omiyale W, Feng Q, Effingham M, et al. UK Biobank: a globally important resource for cancer research. Br J Cancer. (2023) 128:519–27. doi: 10.1038/s41416-022-02053-5
31. Lee JJ, Wedow R, Okbay A, Kong E, Maghzian O, Zacher M, et al. Gene discovery and polygenic prediction from a genome-wide association study of educational attainment in 1.1 million individuals. Nat Genet. (2018) 50:1112–21. doi: 10.1038/s41588-018-0147-3
32. Liu M, Jiang Y, Wedow R, Li Y, Brazel DM, Chen F, et al. Association studies of up to 1.2 million individuals yield new insights into the genetic etiology of tobacco and alcohol use. Nat Genet. (2019) 51:237–44. doi: 10.1038/s41588-018-0307-5
33. Chen D, Wang X, Huang T, and Jia J. Genetic support of a causal relationship between cannabis use and educational attainment: a two-sample Mendelian randomization study of European ancestry. Addiction. (2023) 118:698–710. doi: 10.1111/add.16090
34. Hemani G, Tilling K, and Davey Smith G. Orienting the causal relationship between imprecisely measured traits using GWAS summary data. PloS Genet. (2017) 13:e1007081. doi: 10.1371/journal.pgen.1007081
35. Teumer A. Common methods for performing mendelian randomization. Front Cardiovasc Med. (2018) 5:51. doi: 10.3389/fcvm.2018.00051
36. Hemani G, Zheng J, Elsworth B, Wade KH, Haberland V, Baird D, et al. The MR-Base platform supports systematic causal inference across the human phenome. Elife. (2018) 7:e34408. doi: 10.7554/eLife.34408
37. Bowden J, Davey Smith G, Haycock PC, and Burgess S. Consistent estimation in mendelian randomization with some invalid instruments using a weighted median estimator. Genet Epidemiol. (2016) 40:304–14. doi: 10.1002/gepi.21965
38. Burgess S and Thompson SG. Multivariable Mendelian randomization: the use of pleiotropic genetic variants to estimate causal effects. Am J Epidemiol. (2015) 181:251–60. doi: 10.1093/aje/kwu283
39. Brion M-JA, Shakhbazov K, and Visscher PM. Calculating statistical power in Mendelian randomization studies. Int J Epidemiol. (2013) 42:1497–501. doi: 10.1093/ije/dyt179
40. Guan W, Steffen BT, Lemaitre RN, Wu JHY, Tanaka T, Manichaikul A, et al. Genome-wide association study of plasma N6 polyunsaturated fatty acids within the CHARGE consortium. Circ Cardiovasc Genet. (2014) 7:321–31. doi: 10.1161/CIRCGENETICS.113.000208
41. Kulinskaya E, Dollinger MB, and Bjørkestøl K. On the moments of Cochran’s Q statistic under the null hypothesis, with application to the meta-analysis of risk difference. Res Synth Methods. (2020) 11:920. doi: 10.1002/jrsm.1446
42. Burgess S and Thompson SG. Interpreting findings from Mendelian randomization using the MR-Egger method. Eur J Epidemiol. (2017) 32:377–89. doi: 10.1007/s10654-017-0255-x
43. Wu F, Huang Y, Hu J, and Shao Z. Mendelian randomization study of inflammatory bowel disease and bone mineral density. BMC Med. (2020) 18:312. doi: 10.1186/s12916-020-01778-5
44. Verbanck M, Chen C-Y, Neale B, and Do R. Detection of widespread horizontal pleiotropy in causal relationships inferred from Mendelian randomization between complex traits and diseases. Nat Genet. (2018) 50:693–8. doi: 10.1038/s41588-018-0099-7
45. Cheng H, Garrick DJ, and Fernando RL. Efficient strategies for leave-one-out cross validation for genomic best linear unbiased prediction. J Anim Sci Biotechnol. (2017) 8:38. doi: 10.1186/s40104-017-0164-6
46. Gu SM, Seo S, Park D, Kim S, Lamichhane S, Han K-M, et al. Cannabinoid receptor type 1 regulates drug reward behavior via glutamate decarboxylase 67 transcription. Int J Mol Sci. (2021) 22:10486. doi: 10.3390/ijms221910486
47. De Gregorio D, Dean Conway J, Canul M-L, Posa L, Bambico FR, and Gobbi G. Effects of chronic exposure to low doses of Δ9- tetrahydrocannabinol in adolescence and adulthood on serotonin/norepinephrine neurotransmission and emotional behaviors. Int J Neuropsychopharmacol. (2020) 23:751–61. doi: 10.1093/ijnp/pyaa058
48. Ashtari M, Avants B, Cyckowski L, Cervellione K, Roofeh D, Cook P, et al. Medial temporal structures and memory functions in adolescents with heavy cannabis use. J Psychiatr Res. (2011) 45:1055–66. doi: 10.1016/j.jpsychires.2011.01.004
49. Lorenzetti V, Solowij N, Whittle S, Fornito A, Lubman D, Pantelis C, et al. Gross morphological brain changes with chronic, heavy cannabis use. Br J Psychiatry: J Ment Sci. (2015) 206:77–8. doi: 10.1192/bjp.bp.114.151407
50. Bava S, Frank LR, McQueeny T, Schweinsburg BC, Schweinsburg AD, and Tapert SF. Altered white matter microstructure in adolescent substance users. Psychiatry Res. (2009) 173:228–37. doi: 10.1016/j.pscychresns.2009.04.005
51. Gruber SA, Rogowska J, and Yurgelun-Todd DA. Altered affective response in marijuana smokers: an FMRI study. Drug Alcohol Depend. (2009) 105:139–53. doi: 10.1016/j.drugalcdep.2009.06.019
52. Hodgson K, Coleman JRI, Hagenaars SP, Purves KL, Glanville K, Choi SW, et al. Major depressive disorder working group of the psychiatric genomics consortium, Lewis CM. Cannabis Use Depression Self-harm: Phenotypic Genet Relationships Addict. (2020) 115:482–92. doi: 10.1111/add.14845
53. Jang S-K, Saunders G, Liu M, 23andMe Research Team, Jiang Y, Liu DJ, et al. Genetic correlation, pleiotropy, and causal associations between substance use and psychiatric disorder. Psychol Med. (2022) 52:968–78. doi: 10.1017/S003329172000272X
54. Johnson EC, Hatoum AS, Deak JD, Polimanti R, Murray RM, Edenberg HJ, et al. The relationship between cannabis and schizophrenia: a genetically informed perspective. Addiction. (2021) 116:3227–34. doi: 10.1111/add.15534
55. Sarris J, Sinclair J, Karamacoska D, Davidson M, and Firth J. Medicinal cannabis for psychiatric disorders: a clinically-focused systematic review. BMC Psychiatry. (2020) 20:24. doi: 10.1186/s12888-019-2409-8
56. Zimmermann K, Walz C, Derckx RT, Kendrick KM, Weber B, Dore B, et al. Emotion regulation deficits in regular marijuana users. Hum Brain Mapp. (2017) 38:4270–9. doi: 10.1002/hbm.23671
57. Lisdahl KM, Wright NE, Kirchner-Medina C, Maple KE, and Shollenbarger S. Considering cannabis: the effects of regular cannabis use on neurocognition in adolescents and young adults. Curr Addict Rep. (2014) 1:144–56. doi: 10.1007/s40429-014-0019-6
58. Windle M and Wiesner M. Trajectories of marijuana use from adolescence to young adulthood: predictors and outcomes. Dev Psychopathol. (2004) 16:1007–27. doi: 10.1017/s0954579404040118
59. Marconi A, Di Forti M, Lewis CM, Murray RM, and Vassos E. Meta-analysis of the association between the level of cannabis use and risk of psychosis. Schizophr Bull. (2016) 42:1262–9. doi: 10.1093/schbul/sbw003
60. Long EC, Aggen SH, Neale MC, Knudsen GP, Krueger RF, South SC, et al. The association between personality disorders with alcohol use and misuse: A population-based twin study. Drug Alcohol Depend. (2017) 174:171–80. doi: 10.1016/j.drugalcdep.2017.01.022
Keywords: cannabis use, Mendelian randomization, personality disorder, causality, mental disease
Citation: Ni Y, Li J, Tang Z, Zhang Y and Feng Y (2025) Psychological, social factors, and smoking behavior mediated the effects of cannabis use on personality disorders: A Mendelian randomization study. Front. Psychiatry 16:1411587. doi: 10.3389/fpsyt.2025.1411587
Received: 03 April 2024; Accepted: 25 April 2025;
Published: 15 May 2025.
Edited by:
Jonna Bobzien, Old Dominion University, United StatesReviewed by:
Fang Fang, RTI International, United StatesLin Li, The First Affiliated Hospital of Yangtze University, China
Copyright © 2025 Ni, Li, Tang, Zhang and Feng. This is an open-access article distributed under the terms of the Creative Commons Attribution License (CC BY). The use, distribution or reproduction in other forums is permitted, provided the original author(s) and the copyright owner(s) are credited and that the original publication in this journal is cited, in accordance with accepted academic practice. No use, distribution or reproduction is permitted which does not comply with these terms.
*Correspondence: Youqian Zhang, MTA4NTkxODk4N0BxcS5jb20=; Yanyan Feng, Znl5bWVkQDE2My5jb20=
†These authors share first authorship