- 1Campbell Family Mental Health Research Institute, Centre for Addiction and Mental Health, Toronto, ON, Canada
- 2Department of Psychiatry, University of Toronto, Toronto, ON, Canada
- 3Department of Psychological Clinical Science, University of Toronto, Toronto, ON, Canada
- 4Department of Psychology, University of Toronto Scarborough, Toronto, ON, Canada
- 5Division of Biostatistics, Dalla Lana School of Public Health, University of Toronto, Toronto, ON, Canada
- 6Institute of Mental Health Policy Research, Centre for Addiction and Mental Health, Toronto, ON, Canada
- 7Institute of Medical Science, Temerty Faculty of Medicine, University of Toronto, Toronto, ON, Canada
- 8Department of Pharmacology and Toxicology, Temerty Faculty of Medicine, University of Toronto, Toronto, ON, Canada
- 9Department of Psychology, York University, Toronto, ON, Canada
- 10Moms Stop the Harm, Victoria, BC, Canada
- 11Department of Family and Community Medicine, University of Toronto, Toronto, ON, Canada
- 12Ferkauf Graduate School of Psychology, Yeshiva University, New York, NY, United States
- 13Ontario Canadian Research Initiative in Substance Matters (CRISM) Node Team, Institute for Mental Health Policy Research, Centre for Addiction and Mental Health, Toronto, ON, Canada
- 14Dalla Lana School of Public Health, University of Toronto, Toronto, ON, Canada
- 15The Ontario Network of People Who Use Drugs, Toronto, ON, Canada
- 16Neuroscience and Mental Health, Hospital for Sick Children, Toronto, ON, Canada
- 17Department of Epidemiology and Biostatistics, Western University, London, ON, Canada
Substance use disorders (SUDs), including Alcohol Use Disorder, are pressing global public health problems. Executive functions (EFs) are prominently featured in mechanistic models of addiction. However, significant gaps remain in our understanding of EFs in SUDs, including the dimensional relationships of EFs to underlying neural circuits, molecular biomarkers, disorder heterogeneity, and functional ability. Transforming health outcomes for people with SUDs requires an integration of clinical, biomedical, preclinical, and health services research. Through such interdisciplinary research, we can develop policies and interventions that align with biopsychosocial models of addiction, addressing the complex cognitive concerns of people with SUDs in a more holistic and effective way. Here, we introduce the design and procedures underlying Cognitive Dysfunction in the Addictions (CDiA), an integrative research program, which aims to fill these knowledge gaps and facilitate research discoveries to enhance treatments for people living with SUDs. The CDiA Program comprises seven interdisciplinary projects that aim to evaluate the central thesis that EF has a crucial role in functional outcomes in SUDs. The projects draw on a diverse sample of adults aged 18-60 (target N=400) seeking treatment for SUD, who are followed over one year to identify specific EF domains most associated with improved functioning. Projects 1-3 investigate SUD symptoms, brain circuits, and blood biomarkers and their associations with key EF domains (inhibition, working memory, and set-shifting) and functional outcomes (disability, quality of life). Projects 4 and 5 evaluate interventions for SUDs and their impacts on EF: a clinical trial of repetitive transcranial magnetic stimulation and a preclinical study of potential new pharmacological treatments in rodents. Project 6 links EF to healthcare utilization and is supplemented with a qualitative investigation of EF-related barriers to treatment engagement. Project 7 uses whole-person modeling to integrate the multi-modal data generated across projects, applying clustering and deep learning methods to identify patient subtypes and drive future cross-disciplinary initiatives. The CDiA Program will bring scientific domains together to uncover novel ways in which EFs are linked to SUD severity and functional recovery, and facilitate future discoveries to improve health outcomes in individuals living with SUDs.
Background
Substance use disorders (SUDs)1, including alcohol use disorder (AUD), affect 162 million people worldwide and are associated with substantial morbidity, mortality, and disability (1–4). The socioeconomic and health impacts associated with the use of alcohol, cannabis, and illicit drugs, substantially contribute to the global burden in years living with a disability and lost to premature death (5, 6). In 2020, the World Health Organization heightened their calls for enhanced actions to curb the harmful effects of alcohol use, underscoring the global public health priority of the damaging consequences of alcohol use (7). In the US, 140,000 people die annually from excessive alcohol consumption (i.e., consuming more than 25 grams of ethanol per day) and another 70,000 from drug-related causes, costing the U.S. economy 191.6 billion dollars related to alcohol and 151.4 billion dollars due to use of other substances (8–10). Similarly, in Canada, alcohol and drug use causes almost 20,000 deaths, and costs the Canadian economy nearly 40 billion dollars annually (3). Treatments for SUDs have evidence supporting their efficacy but their availability is limited, highlighting the critical need to increase access to treatments and develop novel and improved interventions for SUD (11).
To advance the understanding of addiction, research on the cognitive and neurobiological factors involved in the development and maintenance of SUD is crucial (12). Contemporary models underscore the centrality of cognition to different phases of SUD development, with a strong emphasis on executive functions (EF) such as response inhibition and decision-making, in conjunction with motivational (e.g., incentive salience, cue reactivity/craving) and affective (e.g., negative emotionality) factors (13, 14). While motivational and affective factors have received extensive attention in the field of SUD research [e.g (15–19)], comprehensive studies of EF and its component processes are relatively scarce, despite the proposed centrality of EF to treatment response and functional outcomes (20, 21).
Further, studies to date have most commonly used relatively “pure” or homogeneous patient groups (e.g., adults with a specific SUD and with no psychiatric comorbidities), with more limited attention to individual differences or within-group variability (22–24). Yet, adults seeking treatment for addiction represent a highly heterogeneous group, with complex substance use histories, often meeting criteria for multiple SUDs, and a high prevalence of psychiatric comorbidities (25–27). Since “pure group” studies have limited generalizability to patient populations seen in real-world clinical settings, there is a critical need to characterize heterogeneity of executive dysfunction in a large inclusive cohort study involving a complex patient population that is characteristic of large tertiary care facilities.
To fill these gaps, we introduce the protocol for the Cognitive Dysfunction in the Addictions (CDiA) program - an integrative team-science and translational research program aiming to evaluate the central thesis that EF is crucial to SUD pathophysiology and functional outcomes. The program aims to aid the discovery of shared versus uniquely affected EF domains (28, 29) across 7 interconnected projects spanning a diverse set of methodologies and perspectives.
While there is ongoing debate about the precise number of “core” processes that define EF, converging evidence suggests that EF comprises at least three factors that are related but distinct on a behavioral and neurobiological level (30–34): 1) inhibition, or the ability to prevent the processing of irrelevant information in working memory and/or inhibit a context-inappropriate behavioral response; 2) working memory updating, or the ability to monitor the contents of working memory for relevance to the current task and to remove from or add information to working memory; and 3) set shifting, reflecting the ability to switch between multiple operations or task sets. These processes are supported by common and dissociable neural substrates within a distributed network of frontoparietal brain regions (35), subserved by known biological and neurotransmitter systems (36, 37), and may be partially genetically influenced (38). EF “deficits” refer to impaired functioning in one or more of these domains, whereas EF “biases” reflect the specific or selective influences of motivational or emotional contexts or materials on EF performance (39).
SUDs can be associated with both deficits and biases in EF accompanied by structural and functional alterations in underlying neural circuitry (40). Problems with EF lead to functional impairment across all stages of addiction. Interventions that bolster EF may mitigate the influence of attentional and behavioral control on drug-seeking and use. For example, computerized cognitive retraining may improve working memory capacity (41–43) and reduce impulsive choice and valuation of specific rewards in addiction (44). Further, non-invasive brain stimulation has exhibited promise across a range of SUDs and appears to impact both craving and decision-making processes (45–47). However, the deficits and biases of EF most linked to functional outcomes, as well as their respective biological bases, remain to be identified and targeted for maximal therapeutic benefit.
By embedding a multilevel assessment of EF in an interdisciplinary research program, CDiA will aid the discovery of shared versus uniquely affected EF domains (28, 29) in a large heterogeneous cohort of adults seeking help for SUDs. Parallel biomarker studies in human participants and translational mechanistic studies in relevant preclinical models will link domains of EF to putative biological underpinnings, together paving the way for the rational design of targeted and individualized therapeutics for treatment and improving functional outcomes in SUDs. Linkages to health care administrative databases will further mobilize knowledge to help shape public health policy and effect change at the societal level.
CDiA consists of seven interconnected projects (P1-7) with the following main objectives, each designed to evaluate and elaborate on the Program’s central tenet that EF is crucial to SUD functional outcomes:
1. To identify domains of EF linked to functional outcomes in adults seeking treatment for SUD (Project 1).
2. To identify imaging biomarkers (MRI, fMRI) associated with domains of EF and those most predictive of functional outcomes in adult outpatients seeking treatment for SUD (Project 2).
3. To identify biomarkers mechanistically associated with EF and functional outcomes in adult outpatients seeking treatment for SUD (Project 3).
4. To assess the impact of repetitive transcranial magnetic stimulation (rTMS) on EF deficits in adult outpatients seeking treatment for comorbid AUD and major depressive disorder (Project 4).
5. To study the impact of alcohol on EF deficits, and their reversal by novel therapeutic interventions, in a preclinical animal model (Project 5).
6. To assess links between EF, treatment-seeking for SUD, and healthcare utilization and costs (Project 6).
7. To identify subtypes of individuals seeking SUD treatment using cross-disciplinary data types from all projects and map the biopsychosocial drivers of cognitive dysfunction in SUDs (Project 7).
Apart for the preclinical project (Project 5), all projects draw on a common sample of adults seeking treatment for SUD, allowing for the triangulation of evidence within the same pool of participants. Alcohol was chosen as the main substance of interest in Projects 4-5 and the qualitative component of Project 6, as those are targeted experimental/qualitative studies that necessitate smaller sample sizes with more homogeneous characteristics. This choice was also dictated by epidemiological estimates, which suggest AUD is the most common type of SUD observed in the US and Canada (48, 49). AUD is therefore also expected to be the most common diagnosis in CDiA. Once established, the proposed research platform can be used to study other types of SUD or more complex populations in the future.
The CDiA Program benefits from the full participation of a Living Expertise Research Advisory (LERA) committee, which is composed of community members who self-identify as having living or lived experience with SUD either directly or as a family member. Incorporating the perspectives of individuals with lived/living experience helps to increase the relevance and impacts of the research on the communities expected to benefit from the findings (50). In conjunction with the scientists leading the program, the LERA committee contributed to the formulation of the program and has offered ongoing insights into its public-facing materials and study procedures. The LERA committee will play a central role in interpreting the CDiA findings and sharing the results with knowledge users in the community. The CDiA Program also consults with an International Scientific Advisory Committee composed of SUD experts who provide ongoing guidance.
An overview of the CDiA Program and synergy between Projects is presented in Figure 1. A detailed description of each project, including the background, methods, and analytical approach, is presented below.
Project 1: to identify domains of EF linked to functional outcomes in adults seeking treatment for SUD
Background
Dual Process models of addiction suggest that SUDs can result from an imbalance between a controlled process that is more intentional (for which EF are central), and an automatic process that is more implicit or involuntary (for which affective states are influential) (51, 52). EFs modulating either appetitive (desirable) or aversive (unpleasant) motivational states are compromised in those with SUDs ( (53); also see the Addiction Neuroclinical Assessment [13, 54, 55)]. Although many studies have investigated how deficits in EFs relate to outcomes among people with SUDs (56), the existing studies are often limited by small samples, very brief (3-6 month) follow up periods, narrow focus on a single substance, the absence of mental health comorbidities, and limited attention to functional outcomes (56). Further, most studies examine either EF deficits (i.e., general impairments in EF performance) or biases (i.e., EF impairments in the context of emotional or motivational material), without examining their relative importance for SUD outcomes. Thus, there is a need for integrative research incorporating all of these factors, and carefully evaluating links with both clinical and functional outcomes in a heterogeneous clinical sample followed prospectively over time.
Objectives
The primary goals of Project 1 (P1) are to characterize EF deficits and biases and to determine which domains of EF are most predictive of functional and treatment outcomes in a complex population of adults seeking treatment for SUD to test the following hypotheses (H1a-e):
H1a
Deficits and biases in the three EF domains will exhibit stronger baseline associations with indicators of functioning, such as disability and quality of life, relative to other components of cognitive functioning.
H1b
EF deficits across all three domains will be most prominent in those with greater (i) severity and (ii) duration of SUD, whereas EF biases will be most prominent in those with concurrent mood disorders and elevated suicidality.
H1c
EF deficits and biases can be used to identify clusters of participants that are associated with distinct clinical features.
H1d
Relatively more severe EF deficits and biases at baseline will predict slower improvements in functional outcomes over and above substance use, treatment engagement, and healthcare utilization.
H1e
EF domains will show improvements from baseline to the one-year follow-up. Improvements in EF will be positively associated with improvements in SUD symptoms and in functional outcomes.
Methods and planned analyses
Design and participants
The CDiA Program aims to recruit a total sample of 400 adults seeking treatment for AUD and/or SUD to participate in this observational, longitudinal study involving clinical and cognitive assessments at baseline and at a one-year follow-up period (see Table 1 for a comprehensive list of clinical and cognitive assessments and the time points of each assessment). Inclusion criteria are: (1) 18 - 60 years of age; (2) meeting diagnostic criteria for a current (past year) AUD and/or SUD (not counting nicotine- or caffeine-related disorders); and (3) seeking support for substance use concerns. Exclusion criteria include: (1) acute intoxication or withdrawal; (2) active psychosis; (3) acute suicidality; and (4) history of severe head injury, dementia, severe neurodevelopmental disorders, and other medical conditions or medications that could severely impair cognition.
Measures
Interview measures will include the Diagnostic Assessment Research Tool (DART (57) to assess SUD and comorbid diagnoses, and the Timeline Follow-Back (TFLB (58) to characterize alcohol and substance use over the past 60 days. Self-report items assessing lifetime history of alcohol and substance use will also be administered, along with measures of severity of alcohol- and substance-related harms (see Table 1). We will measure both (a) general EF deficits and (b) biases in these functions associated with emotional and motivational states; both neutral and emotional stimuli are used in our EF tasks. The stimuli for the EF tasks are a pool of happy, neutral, and sad faces from the Karolinska Emotional Directed Faces database (KEDF (59). Each task is administered with trial blocks with emotional stimuli (i.e., to measure EF biases) and trial blocks with neutral stimuli (i.e., to measure EF deficits). A flanker task (60–63) will be used to assess inhibition, an n-back task (64, 65) will be used to assess working memory updating, and a switch task (66) will be used to assess set-shifting.
Global cognitive function will be evaluated with the Central Nervous System Vital Signs (CNS-VS (67) computerized assessment, which has been validated for use in addiction. To minimize the impact of acute intoxication on cognition and EF, we will verbally confirm participants have not used alcohol/substances 12 hours prior to their cognitive assessment (Last Drug Use Verification Form) and administer a breathalyzer test to confirm zero blood alcohol concentration at the time of testing. We will also administer a urine drug screen and collect self-report data on time since last use of each drug to account for recency of use in the analyses. Other factors relevant to understanding substance use patterns are also assessed, including demographic factors, readiness to change, substance use motives, SUD insight, SUD treatment history, and concurrent mental health difficulties. The complete battery of measures is compiled to provide a fulsome characterization of the sample, consistent with the unique perspectives of each CDiA project and with the intended generativity of the CDiA Program for future research (see Table 1 for a full list of measures).
Power analysis and data analytic plan
Multiple regression will be used with the clinical, functional, and demographic factors entered as simultaneous predictors of each EF domain to test hypotheses about which factors are most strongly associated with specific EF domains (H1a and H1b).
For H1c, we will conduct latent profile analyses using domains of EF as indicators of discrete latent profiles of relative deficits and biases. We will then examine the prediction of membership in these latent profiles from substance use profiles.
For H1d, latent growth curve modeling will be used to model changes in substance use frequency, SUD severity, and functional outcomes over the follow up time points. Next, rate of change will be examined as a function of baseline EF (H1a) by regressing variability in the slopes for the growth factors on baseline EF deficits and biases. These will be included as simultaneous predictors in the model to examine the specific EF domains and biases that are most predictive of change in the clinical and functional outcomes.
For H1e, we will use a generalized linear mixed effects modelling approach. EF deficits and biases will be modelled as dependent variables and time will be entered as a within-subjects factor to determine if there is significant within-person change from baseline to one year follow up. Changes over time in SUD symptoms and functional outcomes will be modelled using the same approach, with changes in EFs included in the models as a time-varying predictor of changes in these outcomes.
Project 2: to identify neuroimaging biomarkers associated with domains of EF and SUD symptoms most predictive of functional outcomes
Background
Whereas Project 1 (P1) will identify EF deficits that may be potential targets of cognitive interventions in future work, Project 2 (P2) will identify neuroimaging biomarkers associated with the same core EF domains and functional outcomes evaluated in P1 to inform innovative brain-based treatments for SUDs. To do so, a complementary mechanistic understanding of neural system dysfunctions that underlie behavioral EF deficits is needed.
Functional magnetic resonance imaging (fMRI) studies in healthy adults suggest that the three dimensions of EF have both common and distinct neural substrates. Studies investigating the three EF domains side by side show overlapping activation in frontoparietal regions, most notably the inferior frontal gyrus, anterior cingulate cortex and bilateral parietal cortices (92). The ability to maintain a task goal stably, shared across all EF domains, in turn relies on areas of the lateral prefrontal cortex (PFC) (30, 93, 94), anterior cingulate cortex (ACC) and frontal operculum (95).
In contrast, inhibition is associated with more extensive recruitment of temporal regions and the cortico-striatal circuit (96). Set-shifting may uniquely involve more posterior dlPFC regions and more extensive parietal areas (97, 98). Working memory updating has been additionally mapped onto fronto-striatal connections and requires input from the basal ganglia and cerebellum (99–101). Similar partially dissociable signatures of the three distinct EF dimensions have been identified in studies using resting-state (i.e., task-free) fMRI (102, 103), structural MRI (104, 105), and diffusion-weighted imaging (DWI) (104).
Meta-analyses of task-based fMRI across SUDs show altered activation in frontoparietal and striatal regions across studies (106). Importantly, however, prior studies have almost universally focused on a single EF domain, providing relatively limited insight into the complex cognitive architecture that may underlie EF impairment in SUDs (107–109). Clinically relevant insight is further limited by a predominance of cross-sectional studies, which do not investigate how neural features supporting EF may contribute to symptom change and functional recovery over time. Characterizing executive dysfunction heterogeneities and underlying neural circuits longitudinally across large naturalistic cohorts of patients seeking treatment for SUDs is likely to provide novel insight into the pathophysiology of addiction and help identify novel targets for brain-based interventions.
Objectives
The first objective of P2 is to identify novel imaging biomarkers (MRI, fMRI) uniquely associated with each of the three domains of EF in SUDs. Its second objective is to identify relationships between these neurostructural and functional markers and SUD severity and day-to-day functioning, both cross-sectionally and over a one-year follow-up. These imaging biomarkers may help guide future development and individualization of biologically informed treatments for SUDs, e.g., through neuromodulation (see P4) or pharmacotherapies (see P5).
H2a
Behavioral performance on the three EF domains will map onto partially dissociable neural substrates overlapping with frontoparietal and corticostriatal circuits. The extent of activation and pattern of brain activity associated with each of the three EFs will be associated with greater SUD severity and lower general functioning at baseline.
H2b
Greater disturbances in EF-associated neural circuits at baseline will be associated with lesser improvement in SUD severity and functional outcomes on follow-up, above and beyond impairments in other neural circuits.
Methods and planned analyses
Participants
All participants included in the total sample are invited to participate in a 1.5-hour MRI protocol. P2-specific exclusion criteria include the presence of MRI-incompatible metal implants, history of stroke, and claustrophobia. We anticipate approximately 50% of the entire sample (n=200) will be eligible and consent to MRI. Eligible participants are invited for a longitudinal scan at one-year follow-up; we anticipate 25% (n=50) being available and consenting to the repeat scan to assess longitudinal stability of our main measures. Given the specific safety requirements of MRI, it is possible that the final P2 sample will not be fully representative of the P1 sample in terms of demographics and clinical characteristics. If this is the case, we will consider this important caveat in interpreting any results using joint P1-P2 and conduct sensitivity analyses where appropriate.
MRI methods
Our research imaging protocol uses state-of-the-art imaging sequences fully harmonized with MRI acquisition protocols used by large-scale population-representative studies (110) and other ongoing CAMH-based cohort studies (111, 112) (see Table 2), which will allow us to leverage our data for comparison or transdiagnostic analyses. To support new research studies, a portion of the scanning time is secured for pilot sequences.
Structural measures
A standard T1-weighted structural scan is acquired to obtain detailed measures of cortical morphology (e.g., thickness, surface area, and volume). Diffusion-weighted imaging (DWI) is acquired to measure white matter tract integrity and tissue microstructure. Neuromelanin-sensitive (NM-MRI) scans are acquired to quantify the integrity of the substantia nigra (113, 114), with partial coverage of the locus coeruleus (115). NM-MRI scans were included to capture metrics related to catecholamine (i.e., dopamine and norepinephrine) signaling implicated in EF (116), and SUDs (113, 117), based on molecular imaging and preclinical models (118). NM-MRI captures the integrity of brain nuclei and neuromodulatory systems that are relatively conserved across species (119, 120). Thus, it also represents a unique bridge to the preclinical component of CDiA as described in Project 5 below.
Resting-state BOLD
Participants are asked to remain awake with their eyes open for 10 minutes while lying restfully in the scanner.
Cognitive fMRI paradigms
We administer three EF tasks for a total duration of ~30 minutes. The tasks assess the same EF domains as in P1 but were specifically selected to be distinct from the paradigms used in P1 to reduce practice effects, which may alter in-scanner performance and brain activation patterns (121, 122). The EF cognitive paradigms were adapted from Rieck et al. (92) and are presented in a pseudorandom order counterbalanced across participants. To minimize visual distraction and permit joint analysis across all EF dimensions, the tasks were designed to be visually simple and consistent across EF domains, using white or light-colored letter stimuli against a dark grey background (Figure 2).
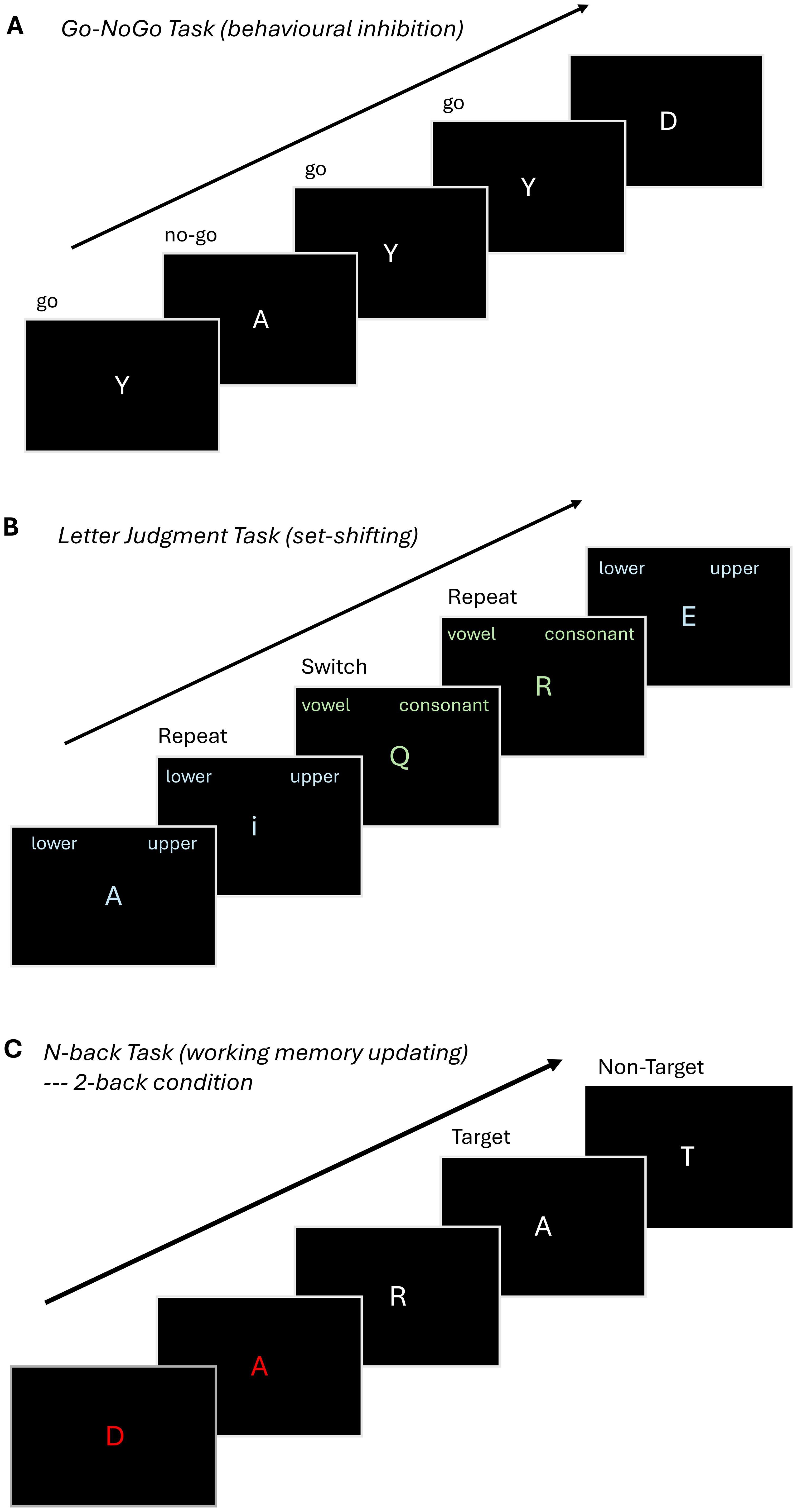
Figure 2. Overview of fMRI tasks. (A) Go-NoGo: Participants are shown a series of letters, one at a time, on a monitor, and are instructed to respond when they see the letter 'Y' (Go Condition) and not to respond (No Go Condition) to other letters. The task comprises two blocks with 90 Go Conditions and 30 No Go Conditions (75% Go and 25% No Go). (B) .Letter Judgement Task: Participants view one of two possible conditions and are instructed to indicate whether a letter presented on the screen is uppercase or lowercase, or whether it is a vowel or a consonant, respectively. The task comprises a single block with 30 switch trials, where the instructions on the current trial are different from the preceeding one and 30 repeat trials in which the instructions on the current trial match the preceeding one. (C) .N-back:The participant is shown a sequence of letters and is instructed to respond when the letter on the monitor matches the letter presented 2 trials earlier (2-back condition). The tasks consists of three blocks of the 2-back condition and three blocks of a 0-back condition, where participants are instructed to press a button when the letter X is presented (not visualized).
Proposed analysis
At baseline, task-based brain activation and brain structure will be investigated in association with SUD symptom severity, functional ability and out-of-scanner EF performance as measured by P1. Multivariate approaches (e.g., Partial Least Squares regression) will be used to investigate relationships between brain structure, function, and performance across the three EF tasks by identifying latent variables (LV) that capture covariance between the set of predictors (microstructure, performance) and outcome variables (functional activation). Individual brain scores reflecting the degree to which participants expressed the LV activation pattern will be correlated with SUD severity, functional ability and out-of-scanner EF performance, at baseline and on follow-up, uncovering neural factors crucial for improved functional outcomes. Age, sex, and primary substance of concern will be used as covariates. We anticipate the EF-domain-specificity of the neuroimaging results will mirror patterns obtained using out-of-scanner EF measures in P1. Although it is challenging to provide a power estimate for studies involving complex multivariate analytic approaches in neuroimaging, our proposed sample size is consistent with recent empirically derived recommendations to enhance reproducibility in task-based fMRI research (123). These studies suggest that a sample size of n=120 is sufficient to obtain a test-retest voxel-wise correlation >0.80 across most paradigms. Thus, our proposed cross-sectional sample size of n=200, is in line with these recommendations. Further, based on standard power analysis tools, a sample size of 200 allows power of >0.80 to detect small effect sizes on the order of r=0.2 or Cohen’s d=0.2.
Project 3: to identify biomarkers mechanistically associated with EF and functional outcomes in adult outpatients seeking treatment for SUD
Background
Identifying neural biomarkers associated with EF in SUDs (P2) will provide valuable insight into neural mechanisms that may be targeted via brain-based therapies. However, imaging is relatively costly, not accessible to everyone (124, 125), and may offer limited insight into molecular mechanisms that may be targetable through pharmacological interventions. To address these limitations, Project 3 (P3) will assess peripheral blood-based biomarkers and provide insight into the molecular mechanisms underlying EF deficits in SUDs. The analysis of peripheral biomarkers has indeed emerged as a promising approach to probe potential mechanisms or inform prognosis for SUDs (126, 127). Establishing the relationship between peripheral biomarkers and cognitive dysfunction in SUDs can help to unravel contributing mechanisms, including domains of EF, and to understand associated risk and mediating factors.
Substance use can trigger the activation of microglia and astrocytes in the brain, driving their function to a neuroinflammatory response and inducing the production of pro-inflammatory biomarkers (128, 129). Several of these molecules can cross the blood-brain barrier, promoting immune activation in peripheral tissues. The persistence of this pro-inflammatory feedback loop between the brain and periphery can lead to reduced neuroplasticity, structural and functional changes in key neural circuits underlying cognitive and emotional processing, leading to executive dysfunction, physical disability, and a higher risk of SUD relapse (130) (Figure 3).
Consistent with this, prior work supports the relationship between SUDs and peripheral markers of pro-inflammatory biological processes. For example, individuals with AUD exhibit an activation pattern characteristic of a pro-inflammatory response that persists even after alcohol withdrawal (131–133). Cocaine abstinence was also associated with higher levels of pro-inflammatory cytokines (IL-2, IL-6, and IL-17) in a sex-specific manner (134). Importantly, higher IL-6 levels have been correlated with worse EF performance (135). In individuals with opioid use disorder, higher levels of pro-inflammatory cytokines (IL-1β, IL-6) were associated with worse episodic memory performance and worse treatment response to methadone (136, 137). A similar pattern of increased pro-inflammatory activation is also observed in patients with cannabis use disorder (138).
Inflammasome activation is frequently reported in different neuropsychiatric conditions (139–141), where it co-occurs with cognitive dysfunction (142–148). However, the relationship between inflammasome activation and cognitive impairment among adults with SUDs remains understudied. The overarching goals of P3 are to investigate pro-inflammatory changes and inflammasome activation as a mechanism associated with executive dysfunction in SUDs.
Objectives
The primary objective of P3 is to investigate the impact of inflammasome activation on EF domains in SUDs. Its secondary objective is to investigate the impact of inflammasome activation on structural and functional brain parameters in SUDs (integration with P2).
H1
Higher inflammasome activation (i.e., high expression of NALP3) will be associated with: (a) worse inhibition (b) lower working memory updating, and (c) reduced set shifting ability.
H2
Higher inflammasome activation will be associated with: (a) lower gray matter in the prefrontal cortex; (b) reduced activation of the default brain network; (c) less connectivity in neural circuits underlying EFs.
Methods and planned analyses
Blood collection, sample preparation, and storage
P3 draws on the same participant pool recruited for P1. Blood is collected into two EDTA (8 cc) and one citrate (4.5 cc) tubes. After processing, the plasma and buffy coat aliquots are immediately stored at -80°C until analysis. Blood samples and extracted DNA are banked for potential future integrative analyses (see Project 7).
Laboratory analysis for plasma biomarkers
We will measure the phosphorylated and non-phosphorylated proteins isoform levels of the NALP3 complex using Western Blot. The ratio between non-phosphorylated and phosphorylated isoforms of NALP3 will be used as a proxy measure of the activation of the inflammasome pathway. Additionally, we will measure the plasma levels of the pro-inflammatory cytokines IL-1β, IL-18, and proCaspase-1 using a multiplex immunoassay (bead-based multiparametric assay).
The immunoassays will be analyzed using the Luminex xMAP technology® (Luminex 200). The assay has very low cross-reactivity, and this technology has a higher sensitivity to detect very low concentrations of different analytes. The intra- and inter-assay coefficient of variance for the assay is below 10% for most of the analytes measured.
Proposed analysis
To test the association of the biomarkers with the three EF domains and neuroimaging outcomes, we will use Pearson correlation and linear multiple regression models. The linear models can be expanded to include potential covariates, if necessary. The total sample size of 400 subjects will be sufficiently powered to detect small effect sizes of correlation coefficients of 0.11 (α=0.05, statistical power of 80%) to 0.14 (α=0.05, statistical power of 95%).
Project 4: to assess the role of repetitive transcranial magnetic stimulation (rTMS) on aberrant EF in the context of major depressive disorder in adult outpatients seeking treatment for AUD (NCT06299787)
Background
Identifying specific neural and molecular mechanisms associated with EF deficits (P2 and P3) may guide future neuromodulatory and pharmacological treatments seeking to boost EF in adults with diverse SUDs and complex clinical presentations. To illustrate the potential utility of brain-based therapies targeting EF, Project 4 (P4) will conduct a clinical trial evaluating the efficacy of neuromodulation on EF in a complex subpopulation of CDiA participants presenting with AUD and Major Depressive Disorder (MDD).
Repetitive Transcranial Magnetic Stimulation (rTMS) of the PFC is FDA-approved for the treatment of MDD and a large body of evidence supports its safety and antidepressant efficacy (149–151). Many cognitive and psychological processes stemming from the PFC, are disrupted in both MDD and AUD (152), which are frequently comorbid (153, 154). Theta Burst Stimulation (TBS) is an efficient form of rTMS associated with both antidepressant effects (155) and alterations in plasticity in the cortex (156). Importantly, TBS takes about 1/6th the time of standard rTMS, translating to a higher impact and consequently more efficient treatment at a population level.
Understanding the mechanisms underlying treatment response to rTMS is crucial to future therapeutic development. Previous studies have demonstrated that both rTMS and TBS target neurophysiological markers of GABA-receptor-mediated inhibition and this mechanism may be related to MDD treatment response (157–160). Although rTMS has shown promise for the treatment of SUDs (E.g (161), there have not been specific neurophysiological investigations into EFs and depressive symptomatology in the context of MDD comorbid with AUD. To address this gap, P4 will conduct a clinical trial evaluating the effects of bilateral TBS on EF in comorbid AUD and MDD. P4 will also investigate the correlation among rTMS treatment, indices of GABAergic inhibition and individual domains of EF (inhibition, updating, and set shifting). To further investigate therapeutic mechanisms, the P4 will incorporate an investigation of theta-gamma coupling (TGC), a neurophysiological index of PFC activity (172), during an N-back working memory task before and after rTMS treatment.
Objectives
The primary objective of P4 is to conduct a double-blind randomized pilot study, consisting of two arms (bilateral TBS and sham) to investigate the effect of bilateral TBS on deficits in EF in the context of comorbid AUD and MDD. We will also measure alterations in TMS-EEG-indexed GABA receptor mediated inhibition and DLPFC TGC as potential mechanisms mediating therapeutic effects. We will also assess the direct associations between improvement in EF domains after bilateral TBS, with improvement in MDD symptoms.
P4 will test six interrelated hypotheses (H1-H6). Specifically, we hypothesize that, compared with sham TBS, 4 weeks of daily bilateral DLPFC TBS will lead to:
H1
Greater improvement in EF task performance;
H2
Greater proportion of individuals remaining abstinent from substance use;
H3
Greater amelioration of depressive symptoms (as indexed by decrease in HRSD-17 score) and suicidal ideation (as indexed by a decrease in Columbia-Suicide Severity Rating Scale score);
H4
Increase in GABA-receptor-mediated inhibition indices;
H5
Increase in TGC proportional to improvements in EF;
H6 (exploratory)
Improvement in all EF domains (inhibition, updating, and set shifting) in conjunction with improvement in MDD symptoms (ie. more amelioration of MDD symptoms will be associated with improved EF).
Methods and planned analyses
A total of 40 participants will be recruited from the CDiA pooled sample within 6 to 12 weeks of the baseline assessment for P1. Participants will be randomized in a 1:1 ratio to one of two different rTMS arms. The first arm will include bilateral TBS, applied as cTBS over the right DLPFC followed by iTBS applied over the left DLPFC. The second arm will be sham TBS. All participants will be asked to participate in 4 weeks of treatment, 5 days/week (i.e. weekdays). TMS-EEG will occur at treatment initiation (week 0) and treatment end (week 4). Baseline and post-treatment clinical measures will be administered to characterize each AUD patient. TBS will be administered using the MagPro X100 stimulator equipped with a Cool-B70 coil and Qooler fluid-cooling device (MagVenture, Farum, Denmark) positioned under MRI guidance using the Brainsight neuronavigation system. With a sample size of 20 participants per group and assumed large effect sizes, we will have 80% of statistical power to detect a significant difference between the active treatment arm and sham at a significance level of 0.05.
Randomization and blinding
We will randomize participants based on a stratified randomization scheme using a permuted block method with a random number generator, in fixed random sizes. We will use an R30 or X100 with a cool A/P B70-type coil (Magventure Inc.) to ensure blinding of both patient and technician. As such, the clinician, researcher, patient and technician will all be blinded. For both active and sham stimulation, the coil is positioned under MRI guidance using real-time neuronavigation, thus providing technician and participant with visual feedback throughout stimulation.
TBS treatment procedure
TBS will be delivered to the DLPFC bilaterally, with neuronavigation determined through structural MRI. The structural MRI utilized to derive the brain target will have been completed as part of P2. The coil position selection will start by identifying MNI152 stereotaxic coordinates (x, y, z) of (–38, +44, +26) and (26, 38, 44) in the left and right DLPFC, respectively, which we have shown to be effective targets for rTMS in alleviating symptoms of MDD (162). Bilateral TBS will be delivered as follows: R-DLPFC cTBS: 40s uninterrupted bursts (triplet 50 Hz bursts, repeated at 5 Hz, 40s on, 600 pulses total) followed immediately by L-DLPFC iTBS: (triplet 50 Hz bursts, repeated at 5 Hz, 2s on and 8s off, 600 pulses total). For intensity of stimulation we will use 120% RMT. Our work in over 200 people stimulating PFC suggests that 120% RMT is the maximum that should be used for negligible risk of seizure and other serious adverse effects beyond the expected scalp pain and headache during early treatment sessions (163, 164).
Participants
Patients will be included if they meet general inclusion criteria for CDiA and in addition, if they:
(1) have a DSM–5 diagnosis of AUD based on the Mini-International Neuropsychiatric Interview (MINI (165) (2) do not exhibit problematic use of any substances (excluding nicotine and caffeine), including alcohol, for >1 month; (3) screened positive for an MDE based on the MINI without psychotic symptoms (4) are agreeable to keeping their current antidepressant medications and medications for AUD constant during the study; (5) are reliably taking SUD agonist therapies if appropriate and managed by their clinical team; (6) are able to adhere to the study schedule (7) meet the TMS safety criteria (166).
Participants are excluded if they meet general exclusion criteria for CDiA, and in addition, if they: (1) have a concomitant major unstable medical illness or any significant neurological disorder; (2) are pregnant or intend to get pregnant during the study; (3) have failed a course of ECT, due to the lower likelihood of response to rTMS; (4) have an intracranial implant (e.g., aneurysm clips, shunts, cochlear implants) or any other metal object within or near the head, excluding the mouth, that cannot be safely removed; (5) require a benzodiazepine with a dose equivalent to lorazepam 2 mg/day or higher (167).
Participants will be discontinued from the study if they cannot safely continue the study based on any of the following criteria: (1) experience clinically significant worsening of suicidality that requires an involuntary inpatient hospitalization; (2) develop clinically significant hypomanic or manic symptoms; (3) relapse into problematic substance use in the month prior to rTMS treatment or during the rTMS treatment; (4) miss four rTMS treatments (i.e., 20%); or (5) withdraw consent.
If relapse into substance use is suspected during rTMS treatment, for safety purposes (e.g., to manage seizure risk), a physician will be called to assess the participant prior to the initiation of rTMS treatment for the day or as soon as substance use is suspected. Investigations (e.g., urine drug screen or blood draw) will be ordered at the physician’s discretion, with the consent of the participant. The decision to continue with or discontinue rTMS treatment for that day or going forward will be at the discretion of the assessing physician and the participant’s SUD clinical team.
Clinical assessments
Participants will be initially screened with the MINI and C-SSRS. Interested and potentially eligible participants will complete a subsequent interview to address all criteria outlined above to confirm eligibility. The 17-item Hamilton Rating Scale for Depression (HRSD-17) (168) will be our tertiary clinical outcome measure. The tertiary clinical outcome criteria will be a decrease in HRSD-17 score at treatment end compared to baseline (150, 162). The HRSD-17 will be performed at baseline, weekly and at the end of the rTMS treatment course. The Columbia-Suicide Severity Rating Scale (C-SSRS) will be used to evaluate suicidality. This reliable and valid scale has been used in randomized clinical trials and is able to predict completed suicide (169). The C-SSRS has been reported as an effective measure for diagnosis and treatment across several diagnoses (170–172) with high internal consistency, and interrater reliability (172–176). The C-SSRS will be performed at baseline, weekly and at the end of the rTMS treatment course. TMS Adult Safety Screen (TASS) (166) will be used at recruitment and baseline to ensure that participants are safe to participate in rTMS treatment trials. To enhance integration with other projects, we will re-administer the EF cognitive assessments within one week prior to the start of rTMS and at the end of the 4-week course of rTMS.
Neurophysiological indices of GABA receptor mediated inhibition in the DLPFC
To evaluate GABA receptor mediated inhibition indices in the DLPFC, TMS will be administered to the left DLPFC using two Magstim-200 stimulators (Magstim Company Ltd., UK) connected via a Bistim module and electrophysiological data will be collected using dedicated hardware and software (Neuroscan, Compumedics, USA). Each TMS session will include the establishment of the individual threshold for stimulation, followed by GABA receptor mediated inhibition paradigms in the DLPFC according to our published methods (160). Recordings will be acquired through a 64-channel EEG. GABA receptor mediated inhibition indices from the DLPFC will be the dependent variables of interest and derived according to published recommendations (160, 177). Our neurophysiological measures have been established in several of our previous reports and have a high test-retest reliability (i.e., ICC > than 0.9) (178, 179). Data analysis will take place using semi-automated methods developed and validated by our group (160, 178). TMS-EEG will be conducted prior to the start of the course of rTMS and within 48 hours after the last rTMS treatment.
To evaluate TGC, participants will undergo an N-back task (2-back condition) during a 10-minute EEG recording at baseline and after the treatment course as part of the TMS-EEG sessions. EEG signals of TGC are recorded using DC and a low pass filter of 100 Hz at 20-kHz sampling rate, and data processed offline using MATLAB and EEGLAB toolbox, following previously published protocols (180, 181). Theta-Gamma Coupling: The measure of TGC is indexed by the modulation index (MI), calculated for each electrode, followed by an average across the right and left frontal electrodes. The MI for all target trials is analyzed as a weighted average based on the number of correct and incorrect responses, as previously described (180, 181).
Finally, we will aggregate data from other CDiA projects (including imaging, genetics, inflammatory models, preclinical biomarkers and more) to further examine the role of GABA receptor mediated inhibition in the cognitive dysfunction found in SUD. Specific baseline cross-analyses will be performed between EF tasks, imaging variables, inflammatory markers and TMS-EEG indices of GABA receptor mediated inhibition.
Project 5: a preclinical study of the effects of alcohol on EF and the impact of novel therapeutic interventions
Background
Projects 1-3 will provide insight into the behavioral, neural and molecular underpinnings of EF deficits in SUDs. However, these studies are by design correlational, limiting our ability to establish causal relationships among putatively mechanistic factors. In contrast, P4 will test causal contributions of neuromodulation to EF improvement but is limited to a treatment modality with a demonstrated safety profile. In contrast, Project 5 (P5) will seek to causally establish specific molecular mechanisms and create a platform for testing novel pharmacological treatments targeting EF. To accomplish this, P5 will employ a reverse-translational approach using rats to examine EF-like deficits along the same three dimensions measured in P1-P4. To evaluate causal contributions to EF-like function, P5 will experimentally manipulate similar biological systems to those evaluated correlationally throughout the rest of the CDiA Program.
Preclinical research on substance administration has played a critical role in understanding the nature of substance-related cognitive dysfunction. However, studies have typically focused on single cognitive domains, and relatively little is known about the dynamic trajectories of executive dysfunction [i.e. emergence, dependence and potential reversal (182, 183)]. To address these gaps and complement the human studies in CDiA, P5 will measure in rats how EF-like performance in three domains is affected by chronic alcohol consumption (Objective 1), potential reversal via pharmacological intervention (Objective 2), and identification of peripheral and central biomarkers for translation to humans (Objective 3).
This investigation will target specific biological pathways implicated in EF deficits in AUD, and investigated correlationally across the rest of CDiA. Specifically, P5 will focus on GABAergic neurotransmission, the inflammasome system, the noradrenergic system, and the opioid system. The GABAergic system plays a critical role in cognitive functions (184), and will be investigated as a therapeutic mediator of neuromodulation treatment in P4. To complement findings from P4, P5 will enhance GABA activity pharmacologically during withdrawal in rats to investigate its impact on EFs. Similarly, the activity of the inflammasome, implicated in brain disorders (185) and alcohol consumption (186), will be measured in P3 for its translational biomarker value. To complement and extend P3’s correlational findings, P5 will block inflammasome activity pharmacologically (via MCC950), to assess its impact on EFs. The integrity of the noradrenergic system, to be measured through NM-MRI in P2, plays a critical modulatory role in AUDs (187), with complex effects depending on activity at various receptor subtypes (α1 versus α2). P5 will target the α2-noradrenergic receptor, using guanfacine, an α2-receptor agonist that demonstrated efficacy at reducing alcohol consumption in rats (188) and is used for the treatment of attention-deficit/hyperactivity disorder (189).
Finally, naltrexone is a prescribed medication used to manage alcohol dependence, especially consumption, although it appears to have reduced efficacy in certain patients, depending on gender and genetic differences (190). Naltrexone is predicted to be prescribed in a significant proportion of P1 participants. Its effects on EFs are not fully characterized and tend to vary from study to study (191, 192). Here, we propose to systematically investigate the relationship between its known clinical efficacy on consumption and potential improvement of EFs in rats, providing a clinically-relevant comparison to the other interventions.
Objectives
The primary objective of Project 5 (P5) will be to characterize the impact of alcohol on three cognitive domains in rats, identify the impact of novel therapeutic approaches, and delineate novel biomarkers predictive of cognitive deficit emergence or treatment efficacy. We will test the following hypotheses:
H1
Alcohol withdrawal will increase working memory deficits, impulsivity and cognitive flexibility deficits in adult rats.
H2
Cognitive dysfunctions observed will be linked to changes in central biomarker of synaptic functions, changes in various neurotransmitter related activity and peripheral signs of neuroinflammation.
H3a
Pharmacological interventions targeting the GABAergic system will contribute to better working memory function, cognitive flexibility and reduced impulsivity during withdrawal.
H3b
Pharmacological interventions targeting the noradrenergic system will contribute to better cognitive functions and reduced alcohol seeking behaviors.
H3c
Pharmacological interventions targeting the inflammasome will reduce signs of neuroinflammation, indirectly contributing to better cognitive health.
This project will also generate a sample repository (brain, blood, plasma) for investigation and discovery of peripheral and central biomarkers indexing putative mechanisms involved in AUD and executive dysfunction, that could be used for follow-up biomarker analyses.
Methods and planned analyses
Male and female Long Evans rats, 3 months old at study initiation, will be utilized in this study. Rats will be habituated to consume a liquid diet (Nestlé® Chocolate Protein Boost) in which ethanol can be diluted. After baseline assessment of EFs, half of each cohort will be exposed to a diet supplemented with 10% w/v alcohol 5 days per week. Removal on 2 consecutive days per week will induce a withdrawal period. Such cycle will be repeated 4 times, with assessment of cognitive functions during withdrawal after 4 cycles (Figure 4; top panel, orange box).
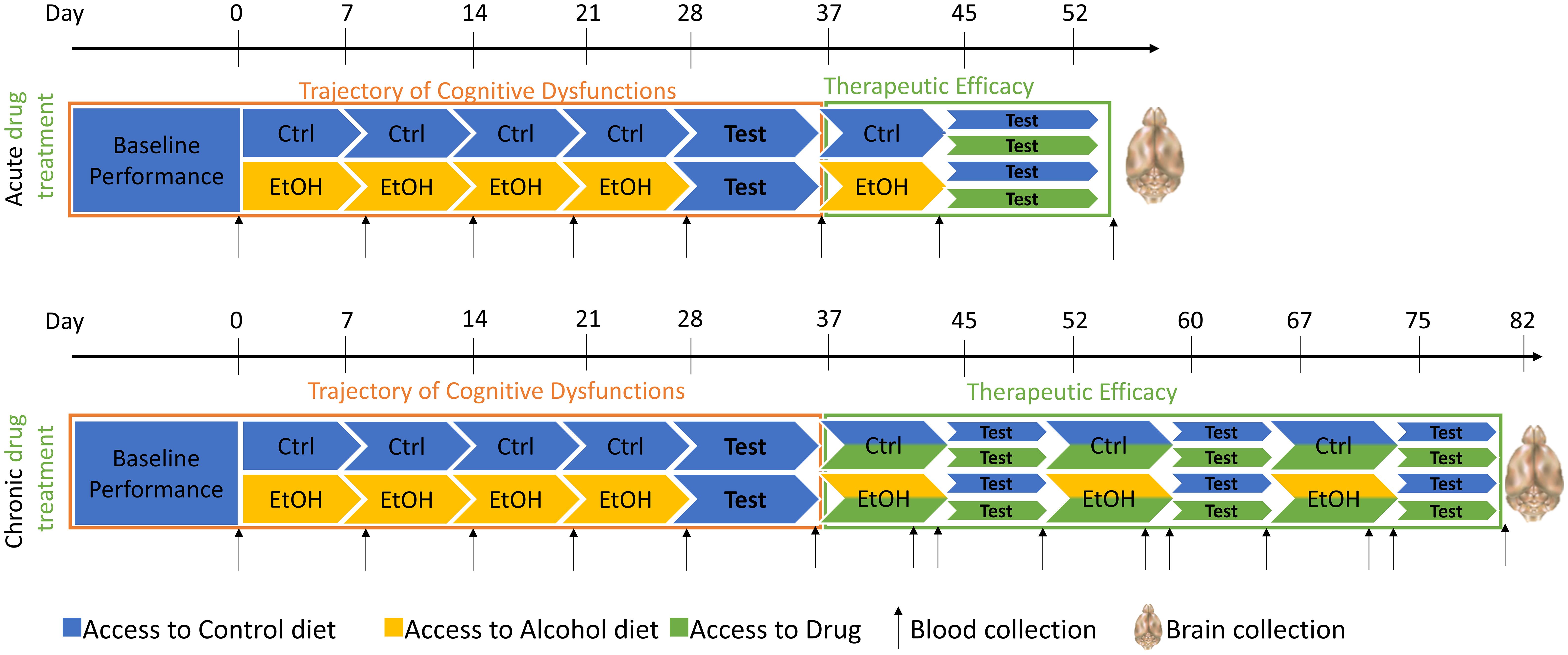
Figure 4. Design of the experiment for cohort of 48 rats. Drugs proposed for this study will be Naltrexone, a5PAM or MC9950. Animals will be tested on different cognitive tasks, depending on their group affiliation (Inhibition/Working Memory or Set-shifting). Blood will be collected on a regular basis to control for the blood alcohol level, and brains will be collected at the end of the study for downstream analysis. Ctrl, control; EtOH, ethanol.
Measures
Three domains of EF (inhibition, working memory, set-shifting) will be assessed to match assessment in P1. Inhibition will be measured using the 5-Choice Serial Reaction Time Task (5-CSRTT). This test is conducted in operant conditioning boxes with 5 apertures featuring integrated light stimuli (193), and a reward dispenser on the opposite wall. Rats are trained to respond to a stimulus among the 5 apertures, earning a reward on a fixed intertrial interval. Lengthening this interval challenges rats to wait longer before responding, with premature responses during the intertrial interval serving as a reliable measure of response inhibition (or impulsivity). In alcohol-exposed rats, premature responses are expected to increase, especially when the intertrial interval is extended.
Working memory will be assessed using a T-maze spontaneous alternation task (194). After 2 days of habituation, rats explore the maze starting in the maze’s initial arm and then choosing between the two goal arms. The chosen arm is closed off, and the rat returns to the start arm for the next trial. This process is repeated for seven trials. Rats naturally alternate between arms, relying on working memory for novel environment exploration. Alcohol-exposed rats tested during withdrawal are expected to show diminished working memory functions.
Set-shifting will be evaluated following Floresco et al.’s protocol (195), in which rats undergo initial training in standard operant conditioning chambers with a house light, two retractable levers, and stimulus lights. Training involves a fixed ratio 1 schedule on each lever, reaching a criterion of 50 rewards or 30 minutes per lever. The second phase introduces a 90-trial session where rats must press a lever within 10 seconds to receive a reward. Visual cue discrimination training follows, with stimulus lights above the levers. Rats respond within 10 seconds to the lit lever for a sucrose reward, while responses on the non-lit lever are punished. Criterion: 10 consecutive correct responses for 30 trials over 4 days. After visual discrimination is learned, rats are tested in a “shift to response discrimination” task, where the rewarded lever is consistent, irrespective of the light stimulus. The number of trials needed to learn this new set serves as a proxy for set-shifting. Rules are modified after each testing session to ensure continual adaptation. Due to the complexity of the inhibition and set-shifting task, these cannot be run in the same animals. Therefore, different animals will be used to run inhibition and working memory vs set-shifting.
Following the assessment of alcohol-induced cognitive function changes, interventions will commence (Objective 2). Treatments involve intraperitoneal administration of drugs targeting α5-GABAA receptors (GL-II-73), the inflammasome (MCC950), the α2-noradrenergic receptor (guanfacine), and the opioid system (naltrexone). Initially, acute dosing will be explored using a Latin square design to test multiple doses (Table 3). Conducting multiple doses in the same animal necessitates several testing sessions, and all employed tests, such as the Y maze and 5-CSRTT, are stable and unaffected by practice and repetition (193, 196–198). The set-shifting task (195) can be adapted to establish new rules at each testing session, enabling regular assessment of set-shifting abilities in the same animals while mitigating the impact of practice on repeated testing.
Blood samples will be drawn weekly and 24 hours after discontinuation of the liquid diet (Figure 4) to verify 1) blood alcohol levels (BALs) exceeding 100mg% during exposure and 2) the absence of alcohol 24 hours after cessation when behavioral testing begins. BALs will be assessed using an ANALOX machine. Serum samples will also be utilized for measuring peripheral inflammasome biomarkers. Inflammasome activity will be determined by the phosphorylated/non-phosphorylated NALP3 ratio in peripheral blood mononuclear cells, along with investigating inflammatory markers (NF-KB, ERK, p38MAPK) and cytokines (IL-1β, IL-18, Caspase-1). Luminex xMAP technology® will be employed for biomarker analyses.
After behavioral assessments, rats will be euthanized, and brains dissected for immunofluorescence staining and molecular analyses on regions of interest (prefrontal cortex, VTA, nucleus accumbens and hippocampus). Rostro-caudal coronal sections will be stored at -20°C for neuroinflammation, noradrenergic, and GABAergic marker analysis. RNA and proteins will be extracted from homogenized tissue, allowing for cDNA conversion, quantitative PCR, and Western Blot analyses. The markers examined include, but are not limited to, SST, PV, α5-GABRA, α1 GABRA, BDNF, and other GABAergic markers. Similarly, qPCR, immunostaining, and Western Blot analyses against sterile inflammation biomarkers (NALP3, NF-KB, ERK, p38MAPK, IL-1β, IL-18, Caspase-1) will validate the correlation of peripheral and central biomarkers in a region-specific manner. Investigation of changes in noradrenergic system markers (NET, α1 receptor, α2 receptor) will complement NM-MRI work outlined in P2.
The blood and brain samples collected will form a repository for subsequent biomarker analyses related to executive dysfunction in AUD. As Objective 3 establishes this repository, the analysis of these samples (peripheral or central) will be influenced by findings from other projects. Consequently, additional exploratory investigations of biomarkers will be conducted in these samples when other projects identify relevant pathways or markers in the clinical population.
Proposed analyses
Behavioral data will be examined to assess the impact of alcohol exposure on EFs, utilizing ANOVA (or relevant non-parametric alternatives) followed by Tukey’s post hoc comparisons for statistical significance. In the alcohol-exposed groups, it is possible to observe subgroups of animals with higher susceptibility to alcohol than the others, suggesting existence of high-responders and low-responders.
For data collected weekly, data will be analyzed with repeated measures to identify animals that are more than 50% of the time over the mean, and animals that are more than 50% of the time under the mean to segregate high-responders and low-responders respectively. If such separation does not exist consistently, then analyses will focus on overall alcohol effect. For the second objective involving drug interactions, separate 2-way ANOVA with diet and treatment as factors will be applied, followed by Dunnett’s post-hoc comparisons (198). In Objective #3, blood sample data will be analyzed using Student’s t-test (Objective #1) or 2-way ANOVA (Objective #2) with diet and treatment as factors, followed by Dunnett’s post-hoc test. Brain analyses with qPCR and Western Blot will use 2-way ANOVA with diet and treatment as factors, followed by Dunnett’s post-hoc comparisons. Pearson or Spearman analyses will evaluate correlations between executive dysfunction and peripheral/central biomarker levels, with p-values adjusted for multiple comparisons using the Bonferroni method due to the potential high number of pairwise analyses.
Project 6: to assess links between EF and treatment seeking for addiction and healthcare utilization and costs
Background
P1-P5 will address psychological and biological mechanisms underlying EF and their implications for functional outcomes in SUDs. However, they will not directly evaluate public health impacts and individual experiences with treatment seeking. To complement these mechanistic insights and link them to potential policy implications, Project 6 (P6) will assess the links between domains of EF, treatment seeking and healthcare utilization.
Among individuals with a SUD, EF can affect retention (199–201) and engagement in treatment as well as treatment outcomes (25, 202), including the ability to achieve and maintain abstinence (199–201, 203). Given that SUDs are strongly associated with health care utilization (27), it is also likely that EF, which impacts the success of SUD treatment, is a key factor affecting the extent of health care utilization. In particular, among those with EF deficits, a diminished ability to achieve abstinence or low-risk alcohol use may lead to a higher use of emergency services for the acute consequences associated with SUDs (204). This could result in increased health care costs (205), which is problematic when existing resources dedicated to emergency services are limited (205, 206). Furthermore, a diminished ability to achieve abstinence or low-risk substance use may also lead to a higher use of non-emergency health care services, including primary care visits and hospitalizations, due to acute and chronic health effects associated with substance use (207, 208).
Furthermore, previous research has shown that EF is a factor in engagement in SUD treatment, including more treatment episodes and longer durations of abstinence among those without EF deficits (203). EF deficits lead to worse recognition of problem use (209–211). Therefore, people with EF deficits are more likely to view their symptoms as temporary or not serious, and consequently do not seek treatment (26). These factors may also affect whether patients stay in treatment or remain abstinent after treatment. Accordingly, it is likely that EF deficits affect treatment seeking behaviors, experiences, and barriers among people with SUDs.
Objectives
P6 has two objectives. The first is to qualitatively assess the potential impact of EF on treatment-seeking behaviors, experiences, and barriers, specifically among individuals with AUD. The second objective is to quantitatively assess the association of EF with health care utilization among individuals with SUD, and to estimate the costs of this utilization.
H1
EF deficits will negatively impact treatment seeking and the treatment process as expressed by participants in treatment for AUD.
H2
Emergency and non-emergency health care utilization and related costs will be higher among participants in treatment for SUD with more severe EF deficits than among those with less severe EF deficits.
Methods and planned analyses
Objective 1 (qualitative component)
A semi-structured, one-on-one 90-minute interview with a subset of participants recruited from the pooled CDiA sample will be conducted by a research coordinator trained in qualitative methods including interviewing and analysis, shortly after their baseline data are collected. Given differences in treatment pathways and trajectories and their potential impacts on treatment experiences, this analysis will be limited to participants with AUD. Interview questions will be related to treatment-seeking behaviors and EF. Participants will be asked about their experiences seeking help for substance use, perceived facilitators and barriers to treatment, perceptions and expectations regarding treatment, past health care utilization, and questions related to how EF (e.g., memory, attention, impulsivity, etc.) may have played a potential role in their treatment-seeking behavior and experiences. The interview will include probes to encourage participants to reflect on reasons for seeking treatment, including experiences with and perceptions of stigma. Participants will be asked about their social identities (e.g., age, sex and gender, ethnicities, etc.) and their EF, and whether or to what extent these factors have impacted their decision to seek treatment or their ability to benefit from and remain engaged in treatment. Additionally, in the absence of stigma being explicitly discussed by participants, stigma-related responses will be coded at the time of data analysis to interpretively identify the latent impact and role that stigma may have played on participants’ willingness to seek treatment.
This study will undertake a thematic analysis of the data obtained, examining and identifying common themes that emerge related to the perceived impact of EF on treatment-seeking behaviors, experiences, and barriers across the sample. We expect that thematic data saturation will be met with a sample size of approximately 30 participants.
All interview transcripts will be manually reviewed by a member of the research team, and an initial codebook will be prepared based on preliminary analyses. All transcripts will be coded for the initial set of codebook themes using NVivo software. As we anticipate both inductive and deductive themes to emerge from the data, the codebook will be subject to further development and revision based on ongoing analyses and discussion of emergent themes among members of the research team. Data analysis will be finalized once we have met data saturation, and no new themes emerge. Final results will be informed by common themes that emerged and were expressed by multiple participants. To increase data analysis credibility, a secondary independent coder will code a sub-sample of transcripts using the coding framework to assess inter-coder reliability, and any discrepancies between codes or themes will be discussed.
Subsequent to the interview, participants will be asked to complete the Behavior Rating Inventory for Executive Functioning in Adults (BRIEF-A), a standardized validated assessment that captures self-reported perceptions of an individual’s EFs and self-regulation (212). The results of the BRIEF-A assessment tool will be used to better understand the participants’ executive functioning from their perspective, which will further inform our qualitative analysis.
Objective 2 (quantitative component)
A health systems evaluation will be conducted to assess the association between EF (as measured in P1) and health care utilization, as well as the costs of this utilization. For this analysis, the database constructed in P1 will be linked with data on health care utilization and the associated costs obtained from the Institute for Clinical Evaluative Sciences (ICES). ICES will provide data on the use of publicly funded health services in Ontario. Participant data will be linked through ICES to the Hospital Discharge Abstract Database (DAD), National Ambulatory Care Reporting System (NACRS), Continuing Care Reporting System (CCRS), Ontario Health Insurance Plan Claims Database (OHIP), and Ontario Drug Benefit Claims (ODB) database. Records-level ICES data linkages will be performed using each participant’s unique Ontario Health Insurance Program health card number; the resulting database will be stripped of all direct personal identifiers and each entry will be assigned a confidential code number. All data linkages will be performed by an ICES data analyst. A subanalysis will be performed stratifying by conditions fully attributable and those not fully attributable to SUD.
Analyses will focus on the occurrence of service utilization, stratified by the type of service (i.e., non-emergency services and emergency services). The occurrence of health care service use among the cohort (recurrent time-to-event data) will be analyzed using multiple models, including covariates across multi-disciplinary domains. These models will include the Andersen and Gill model (i.e., generalizes the Cox model), Prentice, Williams and Peterson (PWP) model, marginal means/rates model, and frailty model (i.e., random effects model) (213–216). The final model to be used will be chosen based upon which of the previously used models best describes the data and which model assumptions are upheld. Model assumptions include the occurrence of an event being dependent upon the prior number of events during the follow-up period (PWP), there being no time-dependent covariates (marginal means/rates model), and the occurrence of an event cannot be explained by observed covariates alone (frailty model). Each statistical model will include covariates for the domains of EF, demographics, substance use, and health service utilization prior to study participation.
Project 7: to identify subtypes of individuals seeking addiction treatment using cross-disciplinary data types from all projects to and map the biopsychosocial all-cause drivers of cognitive dysfunction in adult outpatients seeking treatment for SUD
Background
P1-P6 will address targeted hypothesis-driven questions related to EF deficits and health outcomes in SUDs. To complement these hypothesis-driven efforts, Project 7 (P7) will integrate data types collected across all other projects to identify novel data-driven participant profiles and evaluate their utility in predicting outcomes over time.
In SUDs, heterogeneity is the rule rather than the exception (217). This heterogeneity is largely driven by extremely high rates of comorbid psychiatric conditions (218–220) and compounded challenges in the diagnostic process, as emergent cognitive syndromes may be temporary, drug induced, or chronic (221, 222). Even when considering the same SUD, symptoms and prognoses vary wildly between patients due to complex combinations of life experience (223), biology (224), and socio-demographic factors (225, 226). Given this heterogeneity, it is likely that there exist subpopulations, or subtypes (227–229), of individuals with similar diagnostic classifications but different underlying disease mechanisms and degrees of cognitive impairment. Due to these mechanistic differences, interventions aimed at improving deficits in EF (230) would not be expected to work equally well in different subtypes, meaning their identification is a priority for future applications of precision medicine in SUDs.
Attempts to define subgroups within and across diagnoses with relatively homogeneous symptom profiles and outcomes have met with limited success; for example, a five-biotype model of alcohol dependence has been proposed using latent class modeling of family history, age at onset, DSM-IV criteria, and data on comorbid illnesses (231). Others have proposed four- and two-group subtyping schemes based on psychiatric co-morbidity, family history, psychopathy, temperament, and other clinical health measures (232). Despite the strong links between symptom dimensions and executive dysfunction (see P1), subtypes have not been formally evaluated for differences in domains of inhibition, set shifting, or working memory updating, leaving a major gap in the field. To their detriment, most efforts have focused on relatively few domains of interest, not examined changes in cognitive performance (specifically EF) over time, and yielded varying, unstable results. Therefore, it is important to approach the problem from a data-inclusive perspective (233). Using insights from unimodal investigations, such as those proposed in the preceding CDiA projects, it is also possible to assemble optimal parsimonious predictive models at the individual level which could aid in screening efforts and clinical decision making.
Objectives
Within Project 7 (P7), we propose to integrate data collected from each of the proceeding projects – including use of insights from animal experiments in Project 5 – to (1) perform multi-modal subtyping on SUD individuals, (2) evaluate subtypes against known biological risk profiles for cognitive dysfunction and psychiatric illness (such as exploring polygenic risk scores) and other longitudinal outcomes (e.g. TMS treatment outcome, specified in P4), and (3) use machine learning approaches to optimize predictors of cognitive dysfunction over time and to understand the structure of modifiable risk and resilience factors that may present opportunities for intervention. Within this broader framework, P7 will test the following specific hypotheses:
H1
Multi-modal unsupervised and semi-supervised clustering of SUD patients at baseline will reveal transdiagnostic subtypes of SUD with unique risk factor profiles and levels of EF.
H2
Subtypes from Aim 1 will be predictive of changes in EF over one year of follow-up and individuals with evidence for subgroup transition will experience different treatment response rates.
H3
Multivariate predictive models of changes in EF over time, incorporating the available multi-modal feature space of CDiA, will offer better performance than models based on individual modalities.
Methods and planned analyses
Cross-sectional data-driven subtyping
This will involve using subject clustering algorithms to identify relatively homogeneous subgroups of patients. Primary measurements from each of P1-6 will be used as input for clustering (see Table 4 for domain definitions). The similarity network fusion (SNF) method will then be used to combine more fine-detailed, high-dimensional input variables across multiple modalities. To address the key overarching theme of this proposal, subgroups based on EF domains (including and excluding other measures, as in P1 objectives) will be of particular interest, and will be tested for distributional differences in primary prognostic outcomes of functional recovery and sustained health. The semi-supervised association-boosted SNF (abSNF) method will be used to identify subgroups with most significance to EF performance at baseline. Internal validation of group membership will be performed using bootstrap procedures. Socio-demographic, educational, and life experiential characteristics of each subgroup will be described and placed within the context of existing cross-sectional work.
Longitudinal stability of baseline subgroups and subgroup transitions
Within- and across-data type clustering will be performed independently at multiple time points and evaluate the frequencies of individual-level membership transitions. This aim is similar in nature to clustering approaches used by P1 (H1d and H1e). However, the methods are adapted to account for the increased diversity and dimensionality of input data that will be used to identify latent subgroups. In P1, latent profile analysis and then latent growth curve modelling will be used to identify clusters of participants, and to determine if individual trajectories of substance use and functional outcomes are predicted by baseline EFs, respectively. P7 will extend the foundational work of P1 by examining patient trajectories based on data collected across projects 1-6 and determined using different, network-based methodologies. Critically, we will also identify socio-demographic, educational, and life experiential factors determining inconsistency in group membership over time. Subtypes based on integrative data analyses will be evaluated against more traditional clinical subtypes (such as those from P1) to determine if distributions of key demographics and outcomes are modified by the inclusion of multi-disciplinary input features.
Supervised machine learning for prognostication
We will apply machine learning methods on features identified by each project across domains to build optimally predictive models of: (1) EF, (2) social function, (3) suicidality and self-harm, and (4) healthcare system burden (234). Modelling will proceed iteratively, using processes developed by our group (235–237).
Discussion
There is widespread recognition of the need for integrative and longitudinal research incorporating cognitive, biological, and clinical measures of vulnerability and resilience in SUDs. Within the CDiA Program structure, detailed clinical and cognitive characteristics of a treatment-seeking sample will be integrated with biological markers closely linked to both cognitive dysfunction and SUDs, permitting the identification of critical mechanisms and therapeutic targets. Linkages to healthcare utilization and innovative whole-person modeling approaches will further take this initiative from “neuron-to-neighborhood” and foster integrative and translational research consistent with recent calls to action in this field.
A main strength and innovation of the CDiA Program consists of its translational team and unique collaborative research approach on executive dysfunction for functional recovery and sustained health in outpatients seeking treatment for SUDs (Figure 1). Other conceptual advantages of the Program are the adoption of an individual differences approach, appreciation for patient complexity and heterogeneity, and the recognition that transformation of care can only occur when combining expertise from clinical neuroscience, clinical care, clinical research, and epidemiology/health science research perspectives. As depicted in Figure 1, P1-4 are closely linked investigations with a focus on collecting deep data in complex patients seeking treatment for SUDs; these human subjects research projects emphasize ecological validity and will occur in parallel with Project 5, which offers the opportunity to investigate associations between EFs, proposed biological underpinnings, and associated pharmacological therapeutics in a highly controlled and efficient design. P6 and P7 extend the generativity of this work, with a focus on healthcare utilization and costs, as well as lived experience perspectives, and the application of highly sophisticated, advanced computational modeling to integrate data across all other projects. To further demonstrate the intended integrated approach across projects, we provide here selected examples of potential for individual projects benefiting and feeding back on strategy, approach and deliverables of other projects (see Table 5).
Our inclusive approach to participant recruitment will allow us to recruit a large sample representative of the complex clinical populations typically seen in tertiary care settings. We recognize this will also pose analytical challenges related to sample heterogeneity. Our reliance on multi-level measures of transdiagnostic relevance (e.g., EF, functioning, neural circuit function, inflammatory markers) and our state-of-the-art data integrative approaches outlined in P7 place the CDiA Program in a strong position to harness this heterogeneity to improve understanding of “real-world” presentations of SUDs and their cognitive features. This can in turn accelerate impactful discovery science, clinical translation, and societal/policy changes needed to improve outcomes in this population.
It is anticipated that following the recruitment of this initial cohort, subsequent research will investigate the impact of specific interventions (20, 238) on impaired EFs and functional outcomes. Thus, it is the vision to use these results to support an “experimental medicine” approach aimed at remediating cognitive dysfunction. Peripheral and imaging biomarkers identified in our initial cohort will permit such a nuanced investigation of mediators of clinical outcomes, and in conjunction with preclinical investigations, will inform the development of novel therapeutics (239, 240). Although both psychosocial (e.g., cognitive training) and biological (e.g., pharmacotherapy, noninvasive brain stimulation) interventions are forecasted, the first planned intervention will investigate the efficacy of rTMS on outcomes in SUDs. However, participants in the broader CDiA program are expected to be enrolled in a variety of treatment programs across CAMH and beyond. Thus, although Projects 1-3 of the broader CDiA Program are not centered on a specific clinical trial, they are well-positioned to capture symptom changes and recovery across a variety of therapeutic contexts over time. Given the intended generativity of the program, we do anticipate that the current work will give rise to new, larger and potentially more comprehensive treatment trials directed by initial insight from CDiA.
Limitations
This Research Program is not without limitations. First and foremost, the sample in P1-P3 is by design expected to be heterogeneous in terms of specific substance use profiles and comorbid diagnoses. At the same time, given the different eligibility requirements and diversity of methods (e.g., MRI safety requirements in P2), not all Projects’ samples will be representative of the full CDiA cohort. Notably, P4 and P5 represent smaller experimental studies targeting specific biological mechanisms and use narrower clinical phenotypes. Second, we recognize that the 12-hour abstinence window used in P1-P3 may not account for all residual effects of recently used alcohol or other substances on cognition. To account for this residual variability, all analyses will control for data on recency of use, collected through various means such as self-report and urine drug screens (Table 1).
Implications and future directions
Limitations aside, the CDiA Program is uniquely positioned to bridge expertise across multiple complementary scientific domains and perspectives that have typically remained siloed in SUDs research to date. Future initiatives may wish to expand this model to other clinical populations, treatment modalities, and experimental systems. Future efforts could also explore alternative qualitative analytical methods (e.g., interpretative phenomenological analysis (241)), as well as further participatory action research and designs. For instance, online photovoice is a recently developed innovative qualitative research method (242–246). Online photovoice gives participants the opportunity to express their own experience with as little manipulation as possible, compared to some traditional quantitative methods (244–246). This may be particularly relevant for the topic of cognitive dysfunction among individuals with SUD and represents a promising direction for future work. Community-based participatory action research (247) and culturally responsive small-group work (248) may further support the needs of this population in an inclusive and equitable way in future studies.
Limitations notwithstanding, with its diverse and complementary projects and a shared focus on EF, the CDiA Program is poised to make groundbreaking strides in understanding the heterogeneity of SUDs. The Program also holds promise to identify novel behavioral and neurobiological mechanisms, along with key experiential factors, that could inspire innovative treatments and policies to improve SUD outcomes.
CDiA program study group
CDiA Program Study Group consists of LQ, ES, DF, SL, TP, YN, AR, JW, EV, DV, Isabelle Boileau, Nikki Bozinoff, DB, Christopher Bowie, Paul Fletcher, PG, AH, Colin Hawco, Yarissa Herman, Pamela Kaduri, BF, OM, LQ, Jürgen Rehm, KS, MS, VT, WW and SW.
Ethics statement
The study was approved by the Research Ethics Board (REB) at the Centre for Addiction and Mental Health (CAMH), REB #: 010/2021, 058/2021 and 108/2021. The participants will provide written informed consent to take part in the study. Animal procedures were reviewed and approved by CAMH Animal Care Committee, in accordance with the guidelines of the Canadian Council for Animal Care.
Author contributions
YN: Conceptualization, Funding acquisition, Methodology, Resources, Visualization, Writing – original draft, Writing – review & editing. AR: Writing – original draft, Writing – review & editing, Conceptualization, Funding acquisition, Investigation, Methodology, Project administration, Supervision. DF: Conceptualization, Data curation, Formal analysis, Funding acquisition, Investigation, Methodology, Resources, Visualization, Writing – original draft, Writing – review & editing. SL: Writing – original draft. TP: Writing – original draft, Writing – review & editing, Conceptualization, Funding acquisition, Investigation, Methodology, Supervision. EV: Investigation, Methodology, Resources, Writing – review & editing. DV: Writing – original draft, Writing – review & editing. JW: Writing – original draft, Writing – review & editing, Conceptualization, Funding acquisition, Investigation, Methodology. DB: Conceptualization, Writing – review & editing. KC: Software, Writing – review & editing. RD: Formal analysis, Investigation, Methodology, Project administration, Resources, Validation, Visualization, Writing – review & editing. PG: Writing – review & editing. AH: Writing – review & editing. SJ: Writing – review & editing. BF: Conceptualization, Investigation, Writing – review & editing. OM: Writing – review & editing. JO: Writing – review & editing, Conceptualization. PP: Project administration, Software, Visualization, Writing – original draft, Writing – review & editing. LQ: Data curation, Methodology, Writing – review & editing. CR: Project administration, Writing – review & editing. KS: Writing – review & editing, Methodology. MS: Writing – review & editing. AS: Writing – review & editing. VT: Methodology, Writing – review & editing. DVC: Conceptualization, Data curation, Formal analysis, Investigation, Methodology, Supervision, Validation, Visualization, Writing – original draft, Writing – review & editing. WW: Writing – review & editing. SW: Conceptualization, Methodology, Writing – review & editing. RW: Data curation, Resources, Software, Validation, Visualization, Writing – review & editing. ES: Project administration, Writing – review & editing, Conceptualization. LQ: Conceptualization, Data curation, Formal analysis, Funding acquisition, Investigation, Methodology, Project administration, Resources, Supervision, Validation, Visualization, Writing – original draft, Writing – review & editing. IH: Writing – review & editing. SI: Writing – review & editing.
Funding
The author(s) declare that financial support was received for the research and/or publication of this article. The CDiA Program is supported by a Discovery Fund grant administered through the Centre for Addiction & Mental Health Foundation. YN is supported by a Koerner New Scientist Award and a Paul Garfinkel Catalyst Award administered by the CAMH Foundation. DF is supported by CIHR, the Koerner Family Foundation and the Krembil Foundation. DB receives research support from CIHR, NIMH (R01MH112815; R21MH128815; R01MH1192850), Brain Canada and the Temerty Family through the CAMH Foundation and the Campbell Family Research Institute. The neurophysiological analysis for Project 4 is partly supported by the Labatt Family Network for Research on the Biology of Depression.
Acknowledgments
The authors gratefully acknowledge the CDiA Program Study Group for their contributions to this research. The authors thank the participants of the CDiA Program. We also thank the CDiA Lived Expertise Research Advisory, CDiA Scientific Advisory Board, and the clinical team members of the CAMH Addictions Program for their contributions and support of this work.
Conflict of interest
DB received research support and in-kind equipment support for an investigator-initiated study from Brainsway Ltd. He was the site principal investigator for three sponsor-initiated studies for Brainsway Ltd. He also received in-kind equipment support from Magventure for two investigator-initiated studies. He received medication supplies for an investigator-initiated trial from Indivior. He is a scientific advisor for Sooma Medical. He is the Co-Chair of the Clinical Standards Committee of the Clinical TMS Society unpaid. TP and ES are listed as inventors on patents covering the use of molecules proposed to be tested in the described study. TP also acts as the Director of Preclinical Research and Development of DAMONA Pharmaceuticals, a spin-off company from CAMH aiming at leading the licensed technology to the clinical for reduction of cognitive deficits in brain disorders. ES acts as the CSO and Founder of DAMONA Pharmaceuticals. BF has obtained funding from Indivior for a clinical trial sponsored by Indivior. BF has in-kind donations of placebo edibles from Indivia. BF has obtained industry funding from Canopy Growth Corporation through research grants handled by the Centre for Addiction and Mental Health and the University of Toronto. BF has participated in a session of a National Advisory Board Meeting Emerging Trends BUP-XR for Indiva Canada and is part of Steering Board for a clinical trial for Indiva. BF has been a consultant for Shinogi and ThirdBridge. BF got travel support to attend an event by Bioprojet. BF is supported by CAMH, Waypoint Centre for Mental Health Care, a clinician-scientist award from the department of Family and Community Medicine of the University of Toronto and a Chair in Addiction Psychiatry from the department of Psychiatry of University of Toronto.
The remaining authors declare that the research was conducted in the absence of any commercial or financial relationships that could be construed as a potential conflict of interest.
The author(s) declared that they were an editorial board member of Frontiers, at the time of submission. This had no impact on the peer review process and the final decision.
Publisher’s note
All claims expressed in this article are solely those of the authors and do not necessarily represent those of their affiliated organizations, or those of the publisher, the editors and the reviewers. Any product that may be evaluated in this article, or claim that may be made by its manufacturer, is not guaranteed or endorsed by the publisher.
Footnotes
- ^ Throughout this protocol paper, we use the term “substance use disorder” or “SUD” broadly to include alcohol use disorder and other substance use disorders, unless noted otherwise.
References
1. Carvalho AF, Heilig M, Perez A, Probst C, Rehm J. Alcohol use disorders. Lancet. (2019) 394:781–92. doi: 10.1016/S0140-6736(19)31775-1
2. Rehm J, Shield KD. Global burden of disease and the impact of mental and addictive disorders. Curr Psychiatry Rep. (2019) 21:10. doi: 10.1007/s11920-019-0997-0
3. Rehm J, Probst C, Falcón LL, Shield KD. Burden of Disease: The Epidemiological Aspects of Addiction. In: el-Guebaly N, Carrà G, Galanter M, Baldacchino AM, editors. Textbook of Addiction Treatment: International Perspectives. Springer International Publishing, Cham (2021). p. 51–64.
4. GBD Results. Institute for Health Metrics and Evaluation . Available online at: https://vizhub.healthdata.org/gbd-results/ (Accessed June 6, 2024).
5. Rehm J, Taylor B, Room R. Global burden of disease from alcohol, illicit drugs and tobacco. Drug Alcohol Rev. (2006) 25:503–13. doi: 10.1080/09595230600944453
6. Connor JP, Stjepanović D, Le Foll B, Hoch E, Budney AJ, Hall WD. Cannabis use and cannabis use disorder. Nat Rev Dis Primers. (2021) 7:16. doi: 10.1038/s41572-021-00247-4
7. World Health Organization. Regional Office for the Eastern Mediterranean. Summary report on the regional technical consultation on the working document for development of the action plan (2022-2030) to effectively implement the global strategy to reduce the harmful use of alcohol as a public health priority, virtual meeting, 23 February 2021 (2021). World Health Organization. Regional Office for the Eastern Mediterranean. Available online at: https://apps.who.int/iris/handle/10665/351532 (Accessed March 3, 2023).
8. ARDI alcohol-attributable deaths, US . Available online at: https://nccd.cdc.gov/DPH_ARDI/Default/Report.aspx?T=AAM&P=612EF325-9B55-442B-AE0C-789B06E3A8D5&R=C877B524-834A-47D5-964D-158FE519C894&M=DB4DAAC0-C9B3-4F92-91A5-A5781DA85B68&F=&D (Accessed March 20, 2023).
9. Peterson C, Li M, Xu L, Mikosz CA, Luo F. Assessment of annual cost of substance use disorder in US hospitals. JAMA Netw Open. (2021) 4:e210242. doi: 10.1001/jamanetworkopen.2021.0242
10. U.S. Department of Health and Human Services. Substance abuse prevention dollars and cents: A cost-benefit analysis. Barking, England: Lulu.com (2013). p. 60.
11. Hendershot CS, Witkiewitz K, George WH, Marlatt GA. Relapse prevention for addictive behaviors. Subst Abuse Treat Prev Policy. (2011) 6:17. doi: 10.1186/1747-597X-6-17
12. Verdejo-Garcia A, Lorenzetti V, Manning V, Piercy H, Bruno R, Hester R, et al. A roadmap for integrating neuroscience into addiction treatment: A consensus of the neuroscience interest group of the international society of addiction medicine. Front Psychiatry. (2019) 10:877. doi: 10.3389/fpsyt.2019.00877
13. Kwako LE, Momenan R, Litten RZ, Koob GF, Goldman D. Addictions neuroclinical assessment: A neuroscience-based framework for addictive disorders. Biol Psychiatry. (2016) 80:179–89. doi: 10.1016/j.biopsych.2015.10.024
14. Brand M, Wegmann E, Stark R, Müller A, Wölfling K, Robbins TW, et al. The Interaction of Person-Affect-Cognition-Execution (I-PACE) model for addictive behaviors: Update, generalization to addictive behaviors beyond internet-use disorders, and specification of the process character of addictive behaviors. Neurosci Biobehav Rev. (2019) 104:1–10. doi: 10.1016/j.neubiorev.2019.06.032
15. McHugh RK, Kneeland ET. Affective vulnerability in substance use disorders. Curr Opin Psychol. (2019) 30:54–8. doi: 10.1016/j.copsyc.2019.01.011
16. Votaw VR, Tuchman FR, Piccirillo ML, Schwebel FJ, Witkiewitz K. Examining associations between negative affect and substance use in treatment-seeking samples: a review of studies using intensive longitudinal methods. Curr Addict Rep. (2022) 9:445–72. doi: 10.1007/s40429-022-00441-8
17. Cheetham A, Allen NB, Yücel M, Lubman DI. The role of affective dysregulation in drug addiction. Clin Psychol Rev. (2010) 30:621–34. doi: 10.1016/j.cpr.2010.04.005
18. Volkow ND, Michaelides M, Baler R. The neuroscience of drug reward and addiction. Physiol Rev. (2019) 99:2115–40. doi: 10.1152/physrev.00014.2018
19. Baskin-Sommers AR, Foti D. Abnormal reward functioning across substance use disorders and major depressive disorder: Considering reward as a transdiagnostic mechanism. Int J Psychophysiol. (2015) 98:227–39. doi: 10.1016/j.ijpsycho.2015.01.011
20. Lechner WV, Sidhu NK, Kittaneh AA, Anand A. Interventions with potential to target executive function deficits in addiction: current state of the literature. Curr Opin Psychol. (2019) 30:24–8. doi: 10.1016/j.copsyc.2019.01.017
21. Blume AW, Alan Marlatt G. The role of executive cognitive functions in changing substance use: what we know and what we need to know. Ann Behav Med. (2009) 37:117–25. doi: 10.1007/s12160-009-9093-8
22. Verdejo-Garcia A, Garcia-Fernandez G, Dom G. Cognition and addiction. Dialog Clin Neurosci. (2019) 21:281–90. doi: 10.31887/DCNS.2019.21.3/gdom
23. Kim-Spoon J, Kahn RE, Lauharatanahirun N, Deater-Deckard K, Bickel WK, Chiu PH, et al. Executive functioning and substance use in adolescence: Neurobiological and behavioral perspectives. Neuropsychologia. (2017) 100:79–92. doi: 10.1016/j.neuropsychologia.2017.04.020
24. Kuhns L, Kroon E, Lesscher H, Mies G, Cousijn J. Age-related differences in the effect of chronic alcohol on cognition and the brain: a systematic review. Transl Psychiatry. (2022) 12:345. doi: 10.1038/s41398-022-02100-y
25. Teichner G, Horner MD, Roitzsch JC, Herron J, Thevos A. Substance abuse treatment outcomes for cognitively impaired and intact outpatients. Addict Behav. (2002) 27:751–63. doi: 10.1016/S0306-4603(01)00207-6
26. Mojtabai R, Olfson M, Mechanic D. Perceived need and help-seeking in adults with mood, anxiety, or substance use disorders. Arch Gen Psychiatry. (2002) 59:77–84. doi: 10.1001/archpsyc.59.1.77
27. Graham K, Cheng J, Bernards S, Wells S, Rehm J, Kurdyak P. How much do mental health and substance use/addiction affect use of general medical services? Extent of use, reason for use, and associated costs. Can J Psychiatry. (2017) 62:48–56. doi: 10.1177/0706743716664884
28. Moustafa AA, Morris AN, Nandrino JL, Misiak B, Szewczuk-Bogusławska M, Frydecka D, et al. Not all drugs are created equal: impaired future thinking in opiate, but not alcohol, users. Exp Brain Res. (2018) 236:2971–81. doi: 10.1007/s00221-018-5355-7
29. Rolland B, D’Hondt F, Montègue S, Brion M, Peyron E, D’Aviau de Ternay J, et al. A patient-tailored evidence-based approach for developing early neuropsychological training programs in addiction settings. Neuropsych Rev. (2019) 29:103–15. doi: 10.1007/s11065-018-9395-3
30. Banich MT. Executive function: the search for an integrated account. Curr Dir Psychol Sci. (2009) 18:89–94. doi: 10.1111/j.1467-8721.2009.01615.x
31. Jurado MB, Rosselli M. The elusive nature of executive functions: a review of our current understanding. Neuropsych Rev. (2007) 17:213–33. doi: 10.1007/s11065-007-9040-z
32. Miller EK, Cohen JD. An integrative theory of prefrontal cortex function. Annu Rev Neurosci. (2001) 24:167–202. doi: 10.1146/annurev.neuro.24.1.167
33. Miyake A, Friedman NP, Emerson MJ, Witzki AH, Howerter A, Wager TD. The unity and diversity of executive functions and their contributions to complex “Frontal Lobe” tasks: a latent variable analysis. Cognit Psychol. (2000) 41:49–100. doi: 10.1006/cogp.1999.0734
34. Hasher L, Lustig C, Zacks R. Inhibitory mechanisms and the control of attention. Variation work Memory. (2007) 321:227–49.
35. Nowrangi MA, Lyketsos C, Rao V, Munro CA. Systematic review of neuroimaging correlates of executive functioning: converging evidence from different clinical populations. J Neuropsych Clin Neurosci. (2014) 26:114–25. doi: 10.1176/appi.neuropsych.12070176
36. Wise RA, Robble MA. Dopamine and addiction. Annu Rev Psychol. (2020) 71:79–106. doi: 10.1146/annurev-psych-010418-103337
37. Urban NBL, Martinez D. Neurobiology of addiction: insight from neurochemical imaging. Psychiatr Clin North Am. (2012) 35:521–41. doi: 10.1016/j.psc.2012.03.011
38. Ibrahim-Verbaas CA, Bressler J, Debette S, Schuur M, Smith AV, Bis JC, et al. GWAS for executive function and processing speed suggests involvement of the CADM2 gene. Mol Psychiatry. (2016) 21:189–97. doi: 10.1038/mp.2015.37
39. Quigley L, Thiruchselvam T, Quilty LC. Cognitive control biases in depression: A systematic review and meta-analysis. Psychol Bull. (2022) 148:662–709. doi: 10.1037/bul0000372
40. Brorson HH, Ajo Arnevik E, Rand-Hendriksen K, Duckert F. Drop-out from addiction treatment: a systematic review of risk factors. Clin Psychol Rev. (2013) 33:1010–24. doi: 10.1016/j.cpr.2013.07.007
41. Hendershot CS, Wardell JD, Vandervoort J, McPhee MD, Keough MT, Quilty LC. Randomized trial of working memory training as an adjunct to inpatient substance use disorder treatment. Psychol Addict Behav. (2018) 32:861–72. doi: 10.1037/adb0000415
42. Houben K, Wiers RW, Jansen A. Getting a grip on drinking behavior: training working memory to reduce alcohol abuse. Psychol Sci. (2011) 22:968–75. doi: 10.1177/0956797611412392
43. Morrison AB, Chein JM. Does working memory training work? The promise and challenges of enhancing cognition by training working memory. Psych Bull Rev. (2011) 18:46–60. doi: 10.3758/s13423-010-0034-0
44. Bickel WK, Yi R, Landes RD, Hill PF, Baxter C. Remember the future: working memory training decreases delay discounting among stimulant addicts. Biol Psychiatry. (2011) 69:260–5. doi: 10.1016/j.biopsych.2010.08.017
45. Dunlop K, Hanlon CA, Downar J. Noninvasive brain stimulation treatments for addiction and major depression. Ann N Y Acad Sci. (2017) 1394:31–54. doi: 10.1111/nyas.2017.1394.issue-1
46. Schluter RS, Daams JG, van Holst RJ, Goudriaan AE. Effects of non-invasive neuromodulation on executive and other cognitive functions in addictive disorders: A systematic review. Front Neurosci. (2018) 12:642. doi: 10.3389/fnins.2018.00642
47. Stein ER, Gibson BC, Votaw VR, Wilson AD, Clark VP, Witkiewitz K. Non-invasive brain stimulation in substance use disorders: implications for dissemination to clinical settings. Curr Opin Psychol. (2019) 30:6–10. doi: 10.1016/j.copsyc.2018.12.009
48. Kranzler HR. Overview of alcohol use disorder. Am J Psychiatry. (2023) 180:565–72. doi: 10.1176/appi.ajp.20230488
49. Government of Canada, Canada S. Mental disorders and access to mental health care (2023). Available online at: https://www150.statcan.gc.ca/n1/pub/75-006-x/2023001/article/00011-eng.htm (Accessed March 24, 2025).
50. Quilty LC. Commentary on Verdejo-Garcia et al.: Cognitive training and remediation: identifying priorities for intervention research. Addiction. (2023) 118:952–3. doi: 10.1111/add.v118.5
51. Lindgren KP, Hendershot CS, Ramirez JJ, Bernat E, Rangel-Gomez M, Peterson KP, et al. A dual process perspective on advances in cognitive science and alcohol use disorder. Clin Psychol Rev. (2019) 69:83–96. doi: 10.1016/j.cpr.2018.04.002
52. Wiers RW, Gladwin TE, Hofmann W, Salemink E, Ridderinkhof KR. Cognitive bias modification and cognitive control training in addiction and related psychopathology: mechanisms, clinical perspectives, and ways forward. Clin Psychol Sci. (2013) 1:192–212. doi: 10.1177/2167702612466547
54. Kwako LE, Bickel WK, Goldman D. Addiction biomarkers: dimensional approaches to understanding addiction. Trends Mol Med. (2018) 24:121–8. doi: 10.1016/j.molmed.2017.12.007
55. Kwako LE, Momenan R, Grodin EN, Litten RZ, Koob GF, Goldman D. Addictions Neuroclinical Assessment: A reverse translational approach. Neuropharmacology. (2017) 122:254–64. doi: 10.1016/j.neuropharm.2017.03.006
56. Domínguez-Salas S, Díaz-Batanero C, Lozano-Rojas OM, Verdejo-García A. Impact of general cognition and executive function deficits on addiction treatment outcomes: Systematic review and discussion of neurocognitive pathways. Neurosci Biobehav Rev. (2016) 71:772–801. doi: 10.1016/j.neubiorev.2016.09.030
57. McCabe RE, Milosevic I, Rowa K, Shnaider P, Pawluk EJ, Antony MM. Diagnostic assessment research tool. Psychol Assess. doi: 10.1037/t81500-000
58. Sobell LC, Ontario. Addiction Research Foundation, Sobell MB. Timeline Followback: User’s Guide. Toronto, ON, Canada: Addiction Research Foundation = Fondation de la recherche sur la toxicomanie (1996). p. 109.
59. Lundqvist D, Flykt A, Öhman A. Karolinska Directed Emotional Faces (KDEF) . (1998). doi: 10.1037/t27732-000
60. Eriksen BA, Eriksen CW. Effects of noise letters upon the identification of a target letter in a nonsearch task. Percept Psycho. (1974) 16:143–9. doi: 10.3758/BF03203267
61. Fenske MJ, Eastwood JD. Modulation of focused attention by faces expressing emotion: evidence from flanker tasks. Emotion. (2003) 3:327–43. doi: 10.1037/1528-3542.3.4.327
62. Zetsche U, D’Avanzato C, Joormann J. Depression and rumination: relation to components of inhibition. Cognit Emot. (2012) 26:758–67. doi: 10.1080/02699931.2011.613919
63. Zetsche U, Joormann J. Components of interference control predict depressive symptoms and rumination cross-sectionally and at six months follow-up. J Behav Ther Exp Psychiatry. (2011) 42:65–73. doi: 10.1016/j.jbtep.2010.06.001
64. Levens SM, Gotlib IH. Updating positive and negative stimuli in working memory in depression. J Exp Psychol Gen. (2010) 139:654–64. doi: 10.1037/a0020283
65. Pe ML, Raes F, Kuppens P. The cognitive building blocks of emotion regulation: ability to update working memory moderates the efficacy of rumination and reappraisal on emotion. PloS One. (2013) 8:e69071. doi: 10.1371/journal.pone.0069071
66. Morris N, Jones DM. Memory updating in working memory: The role of the central executive. Br J Psychol. (1990) 81:111–21. doi: 10.1111/j.2044-8295.1990.tb02349.x
67. Gualtieri CT, Johnson LG. Reliability and validity of a computerized neurocognitive test battery, CNS Vital Signs. Arch Clin Neuropsych. (2006) 21:623–43. doi: 10.1016/j.acn.2006.05.007
69. Fernández J, Quiroga MA, Del Olmo I. Is sexual attraction independent of the instrumental and expressive traits? Span J Psychol. (2006) 9:162–70. doi: 10.1017/s1138741600006065
70. Ramsay CE, Abedi GR, Marson JD, Compton MT. Overview and initial validation of two detailed, multidimensional, retrospective measures of substance use: the Lifetime Substance Use Recall (LSUR) and Longitudinal Substance Use Recall for 12 Weeks (LSUR-12) Instruments. J Psychiatr Res. (2011) 45:83–91. doi: 10.1016/j.jpsychires.2010.04.018
71. Saunders JB, Aasland OG, Babor TF, de la Fuente JR, Grant M. Development of the alcohol use disorders identification test (AUDIT): WHO collaborative project on early detection of persons with harmful alcohol consumption-II. Addiction. (1993) 88:791–803. doi: 10.1111/j.1360-0443.1993.tb02093.x
72. Berman AH, Bergman H, Palmstierna T, Schlyter F. The Drug Use Disorders Identification Test-manual. Stockholm: Karolinska Institutet (2003).
73. Heatherton TF, Kozlowski LT, Frecker RC, Fagerström KO. The Fagerström test for nicotine dependence: A revision of the Fagerström tolerance questionnaire. Br J Addict. (1991) 86:1119–27. doi: 10.1111/j.1360-0443.1991.tb01879.x
74. Kim J, Taggar A, Quilty LC, Selby P, Caravaggio F, Ueno F, et al. A measure of illness awareness in alcohol use disorder-Alcohol Use Awareness and Insight Scale (AAS). Drug Alcohol Depend. doi: 10.1016/j.drugalcdep.2021.108813
75. Kim J, Kambari Y, Taggar A, Quilty LC, Selby P, Caravaggio F, et al. A measure of subjective substance use disorder awareness - Substance Use Awareness and Insight Scale (SAS). Drug Alcohol Depend. (2022) 231:109129. doi: 10.1016/j.drugalcdep.2021.109129
76. Gossop M, Darke S, Griffiths P, Hando J, Powis B, Hall W, et al. The Severity of Dependence Scale (SDS): psychometric properties of the SDS in English and Australian samples of heroin, cocaine and amphetamine users. Addiction. (1995) 90:607–14. doi: 10.1046/j.1360-0443.1995.9056072.x
77. Somoza E, Dyrenforth S, Goldsmith J, Mezinskis J, Cohen M. (1995). Search of a universal drug craving scale, in: Paper presented at the Annual Meeting of the American Psychiatric Association, Miami Florida.
78. Miller WR, Tonigan JS. Assessing drinkers’ motivation for change: The Stages of Change Readiness and Treatment Eagerness Scale (SOCRATES). In: Addictive behaviors: Readings on etiology, prevention, and treatment. American Psychological Association, Washington (1997). p. 355–69.
79. Biolcati R, Passini S. Substance use motives measure. PsycTESTS Dataset. (2020). doi: 10.1037/t75863-000
80. Axelsson E, Lindsäter E, Ljótsson B, Andersson E, Hedman-Lagerlöf E. The 12-item self-report World Health Organization Disability Assessment Schedule (WHODAS) 2.0 administered via the Internet to individuals with anxiety and stress disorders: A psychometric investigation based on data from two clinical trials. JMIR Ment Health. (2017) 4:e58. doi: 10.2196/mental.7497
81. Skevington SM, Lotfy M, O’Connell KA, WHOQOL Group. The World Health Organization’s WHOQOL-BREF quality of life assessment: psychometric properties and results of the international field trial. A report from the WHOQOL group. Qual Life Res. (2004) 13:299–310. doi: 10.1023/B:QURE.0000018486.91360.00
82. Kroenke K, Spitzer RL, Williams JB. The PHQ-9: validity of a brief depression severity measure. J Gen Intern Med. (2001) 16:606–13. doi: 10.1046/j.1525-1497.2001.016009606.x
83. Spitzer RL, Kroenke K, Williams JBW, Löwe B. A brief measure for assessing generalized anxiety disorder: the GAD-7: The GAD-7. Arch Intern Med. (2006) 166:1092–7. doi: 10.1001/archinte.166.10.1092
84. Bernstein DP, Stein JA, Newcomb MD, Walker E, Pogge D, Ahluvalia T, et al. Development and validation of a brief screening version of the Childhood Trauma Questionnaire. Child Abuse Negl. (2003) 27:169–90. doi: 10.1016/S0145-2134(02)00541-0
85. Brugha T, Bebbington P, Tennant C, Hurry J. The List of Threatening Experiences: a subset of 12 life event categories with considerable long-term contextual threat. Psychol Med. (1985) 15:189–94. doi: 10.1017/S003329170002105X
86. Carver CS. You want to measure coping but your protocol’s too long: consider the brief COPE. Int J Behav Med. (1997) 4:92–100. doi: 10.1207/s15327558ijbm0401_6
87. Victor SE, Klonsky ED. Validation of a brief version of the difficulties in emotion regulation scale (DERS-18) in five samples. J Psychopathol Behav Assess. (2016) 38:582–9. doi: 10.1007/s10862-016-9547-9
88. Blevins CA, Weathers FW, Davis MT, Witte TK, Domino JL. The Posttraumatic Stress Disorder Checklist for DSM-5 (PCL-5): Development and initial psychometric evaluation: Posttraumatic stress disorder checklist for DSM-5. J Trauma Stress. (2015) 28:489–98. doi: 10.1002/jts.2015.28.issue-6
89. Ward MF, Wender PH, Reimherr FW. The Wender Utah Rating Scale: an aid in the retrospective diagnosis of childhood attention deficit hyperactivity disorder. Am J Psychiatry. (1993) 150:885–90. doi: 10.1176/ajp.150.6.885
90. Krueger RF, Hobbs KA. An overview of the DSM-5 alternative model of personality disorders. Psychopathology. (2020) 53:126–32. doi: 10.1159/000508538
91. MacKillop J, Weafer J C, Gray J, Oshri A, Palmer A, de Wit H. The latent structure of impulsivity: impulsive choice, impulsive action, and impulsive personality traits. Psychopharmacology. (2016) 233:3361–70. doi: 10.1007/s00213-016-4372-0
92. Rieck JR, Baracchini G, Grady CL. Contributions of brain function and structure to three different domains of cognitive control in normal aging. J Cognit Neurosci. (2021) 33:1811–32. doi: 10.1162/jocn_a_01685
93. Braver TS. The variable nature of cognitive control: a dual mechanisms framework. Trends Cognit Sci. (2012) 16:106–13. doi: 10.1016/j.tics.2011.12.010
94. Sakai K. Task set and prefrontal cortex. Annu Rev Neurosci. (2008) 31:219–45. doi: 10.1146/annurev.neuro.31.060407.125642
95. Dosenbach NUF, Fair DA, Cohen AL, Schlaggar BL, Petersen SE. A dual-networks architecture of top-down control. Trends Cognit Sci. (2008) 12:99–105. doi: 10.1016/j.tics.2008.01.001
96. Zhang R, Geng X, Lee TMC. Large-scale functional neural network correlates of response inhibition: an fMRI meta-analysis. Brain Struct Funct. (2017) 222:3973–90. doi: 10.1007/s00429-017-1443-x
97. Derrfuss J, Brass M, Neumann J, von Cramon DY. Involvement of the inferior frontal junction in cognitive control: meta-analyses of switching and Stroop studies. Hum Brain Mapp. (2005) 25:22–34. doi: 10.1002/hbm.20127
98. Wager TD, Jonides J, Reading S. Neuroimaging studies of shifting attention: a meta-analysis. Neuroimage. (2004) 22:1679–93. doi: 10.1016/j.neuroimage.2004.03.052
99. O’Reilly RC, Frank MJ. Making working memory work: a computational model of learning in the prefrontal cortex and basal ganglia. Neural Comput. (2006) 18:283–328. doi: 10.1162/089976606775093909
100. McNab F, Klingberg T. Prefrontal cortex and basal ganglia control access to working memory. Nat Neurosci. (2008) 11:103–7. doi: 10.1038/nn2024
101. Emch M, von Bastian CC, Koch K. Neural correlates of verbal working memory: an fMRI meta-analysis. Front Hum Neurosci. (2019) 13:180. doi: 10.3389/fnhum.2019.00180
102. Reineberg AE, Andrews-Hanna JR, Depue BE, Friedman NP, Banich MT. Resting-state networks predict individual differences in common and specific aspects of executive function. Neuroimage. (2015) 104:69–78. doi: 10.1016/j.neuroimage.2014.09.045
103. Reineberg AE, Banich MT. Functional connectivity at rest is sensitive to individual differences in executive function: A network analysis. Hum Brain Mapp. (2016) 37:2959–75. doi: 10.1002/hbm.23219
104. Bettcher BM, Mungas D, Patel N, Elofson J, Dutt S, Wynn M, et al. Neuroanatomical substrates of executive functions: Beyond prefrontal structures. Neuropsychologia. (2016) 85:100–9. doi: 10.1016/j.neuropsychologia.2016.03.001
105. Yuan P, Raz N. Prefrontal cortex and executive functions in healthy adults: a meta-analysis of structural neuroimaging studies. Neurosci Biobehav Rev. (2014) 42:180–92. doi: 10.1016/j.neubiorev.2014.02.005
106. Klugah-Brown B, Di X, Zweerings J, Mathiak K, Becker B, Biswal B. Common and separable neural alterations in substance use disorders: A coordinate-based meta-analyses of functional neuroimaging studies in humans. Hum Brain Mapp. (2020) 41:4459–77. doi: 10.1002/hbm.25085
107. Navarri X, Afzali MH, Lavoie J, Sinha R, Stein DJ, Momenan R, et al. How do substance use disorders compare to other psychiatric conditions on structural brain abnormalities? A cross-disorder meta-analytic comparison using the ENIGMA consortium findings. Hum Brain Mapp. (2022) 43:399–413. doi: 10.1002/hbm.25114
108. Yan H, Xiao S, Fu S, Gong J, Qi Z, Chen G, et al. Functional and structural brain abnormalities in substance use disorder: A multimodal meta-analysis of neuroimaging studies. Acta Psychiatr Scand. (2023) 147:345–59. doi: 10.1111/acps.v147.4
109. Dugré JR, Orban P, Potvin S. Disrupted functional connectivity of the brain reward system in substance use problems: A meta-analysis of functional neuroimaging studies. Addict Biol. (2023) 28:e13257. doi: 10.1111/adb.13257
110. Casey BJ, Cannonier T, Conley MI, Cohen AO, Barch DM, Heitzeg MM, et al. The Adolescent Brain Cognitive Development (ABCD) study: Imaging acquisition across 21 sites. Dev Cognit Neurosci. (2018) 32:43–54. doi: 10.1016/j.dcn.2018.03.001
111. Dickie EW, Ameis SH, Boileau I, Diaconescu AO, Felsky D, Goldstein BI, et al. Neuroimaging & Biosample collection in the Toronto adolescent and youth (TAY) cohort study: rationale, methods, and early data. Biol Psychiatry Cognit Neurosci Neuroimaging. (2023) (3):275–84. doi: 10.1016/j.bpsc.2023.10.013
112. Agarwal SM, Dissanayake J, Agid O, Bowie C, Brierley N, Chintoh A, et al. Characterization and prediction of individual functional outcome trajectories in schizophrenia spectrum disorders (PREDICTS study): Study protocol. PloS One. (2023) 18:e0288354. doi: 10.1371/journal.pone.0288354
113. Cassidy CM, Carpenter KM, Konova AB, Cheung V, Grassetti A, Zecca L, et al. Evidence for dopamine abnormalities in the substantia nigra in cocaine addiction revealed by neuromelanin-sensitive MRI. Am J Psychiatry. (2020) 177:1038–47. doi: 10.1176/appi.ajp.2020.20010090
114. Cassidy CM, Zucca FA, Girgis RR, Baker SC, Weinstein JJ, Sharp ME, et al. Neuromelanin-sensitive MRI as a noninvasive proxy measure of dopamine function in the human brain. Proc Natl Acad Sci U.S.A. (2019) 116:5108–17. doi: 10.1073/pnas.1807983116
115. Calarco N, Cassidy CM, Selby B, Hawco C, Voineskos AN, Diniz BS, et al. Associations between locus coeruleus integrity and diagnosis, age, and cognitive performance in older adults with and without late-life depression: An exploratory study. NeuroImage Clin. (2022) 36:103182. doi: 10.1016/j.nicl.2022.103182
116. Leh SE, Petrides M, Strafella AP. The neural circuitry of executive functions in healthy subjects and Parkinson’s disease. Neuropsychopharmacology. (2010) 35:70–85. doi: 10.1038/npp.2009.88
117. Ahrens J, Zaher F, Rabin RA, Cassidy CM, Palaniyappan L. Neuromelanin levels in individuals with substance use disorders: A systematic review and meta-analysis. Neurosci Biobehav Rev. (2024) 161:105690. doi: 10.1016/j.neubiorev.2024.105690
118. Foster SL, Weinshenker D. Chapter 15 - The Role of Norepinephrine in Drug Addiction: Past, Present, and Future. In: Torregrossa M, editor. Neural Mechanisms of Addiction. Cambridge, MA United States: Academic Press (2019). p. 221–36.
119. Vidal-Gadea AG, Pierce-Shimomura JT. Conserved role of dopamine in the modulation of behavior. Commun Integr Biol. (2012) 5:440–7. doi: 10.4161/cib.20978
120. Vreven A, Aston-Jones G, Pickering AE, Poe GR, Waterhouse B, Totah NK. In search of the locus coeruleus: guidelines for identifying anatomical boundaries and electrophysiological properties of the blue spot in mice, fish, finches, and beyond. J Neurophysiol. (2024) 132:226–39. doi: 10.1152/jn.00193.2023
121. Chen Z, Lei X, Ding C, Li H, Chen A. The neural mechanisms of semantic and response conflicts: an fMRI study of practice-related effects in the Stroop task. Neuroimage. (2013) 66:577–84. doi: 10.1016/j.neuroimage.2012.10.028
122. Weissman DH, Woldorff MG, Hazlett CJ, Mangun GR. Effects of practice on executive control investigated with fMRI. Brain Res Cognit Brain Res. (2002) 15:47–60. doi: 10.1016/S0926-6410(02)00215-X
123. Turner BO, Paul EJ, Miller MB, Barbey AK. Small sample sizes reduce the replicability of task-based fMRI studies. Commun Biol. (2018) 1:62. doi: 10.1038/s42003-018-0073-z
124. Torkamani A, Wineinger NE, Topol EJ. The personal and clinical utility of polygenic risk scores. Nat Rev Genet. (2018) 19:581–90. doi: 10.1038/s41576-018-0018-x
125. Khera AV, Chaffin M, Aragam KG, Haas ME, Roselli C, Choi SH, et al. Genome-wide polygenic scores for common diseases identify individuals with risk equivalent to monogenic mutations. Nat Genet. (2018) 50:1219–24. doi: 10.1038/s41588-018-0183-z
126. Copersino ML. Cognitive mechanisms and therapeutic targets of addiction. Curr Opin Behav Sci. (2017) 13:91–8. doi: 10.1016/j.cobeha.2016.11.005
127. Bough KJ, Pollock JD. Defining substance use disorders: the need for peripheral biomarkers. Trends Mol Med. (2018) 24:109–20. doi: 10.1016/j.molmed.2017.12.009
128. Redwine LS, Pung MA, Wilson K, Chinh K, Duffy AR. Differential peripheral inflammatory factors associated with cognitive function in patients with heart failure. Neuroimmunomodulation. (2018) 25:146–52. doi: 10.1159/000493142
129. Ersche KD, Roiser JP, Lucas M, Domenici E, Robbins TW, Bullmore ET. Peripheral biomarkers of cognitive response to dopamine receptor agonist treatment. Psychopharmacology. (2011) 214:779–89. doi: 10.1007/s00213-010-2087-1
130. Crews FT, Zou J, Qin L. Induction of innate immune genes in brain create the neurobiology of addiction. Brain Behav Immun. (2011) 25 Suppl 1:S4–S12. doi: 10.1016/j.bbi.2011.03.003
131. Umhau JC, Schwandt M, Solomon MG, Yuan P, Nugent A, Zarate CA, et al. Cerebrospinal fluid monocyte chemoattractant protein-1 in alcoholics: support for a neuroinflammatory model of chronic alcoholism. Alcohol Clin Exp Res. (2014) 38:1301–6. doi: 10.1111/acer.2014.38.issue-5
132. Portelli J, Wiers CE, Li X, Deschaine SL, McDiarmid GR, Bermpohl F, et al. Peripheral proinflammatory markers are upregulated in abstinent alcohol-dependent patients but are not affected by cognitive bias modification: Preliminary findings. Drug Alcohol Depend. (2019) 204:107553. doi: 10.1016/j.drugalcdep.2019.107553
133. Girard M, Malauzat D, Nubukpo P. Serum inflammatory molecules and markers of neuronal damage in alcohol-dependent subjects after withdrawal. World J Biol Psychiatry. (2019) 20:76–90. doi: 10.1080/15622975.2017.1349338
134. Zaparte A, Schuch JB, Viola TW, Baptista TAS, Beidacki AS, do Prado CH, et al. Cocaine use disorder is associated with changes in th1/th2/th17 cytokines and lymphocytes subsets. Front Immunol. (2019) 10:2435. doi: 10.3389/fimmu.2019.02435
135. Levandowski ML, Hess ARB, Grassi-Oliveira R, de Almeida RMM. Plasma interleukin-6 and executive function in crack cocaine-dependent women. Neurosci Lett. (2016) 628:85–90. doi: 10.1016/j.neulet.2016.06.023
136. Wang T-Y, Lee S-Y, Chang Y-H, Chen S-L, Chen PS, Chu C-H, et al. Correlation of cytokines, BDNF levels, and memory function in patients with opioid use disorder undergoing methadone maintenance treatment. Drug Alcohol Depend. (2018) 191:6–13. doi: 10.1016/j.drugalcdep.2018.06.024
137. Lu R-B, Wang T-Y, Lee S-Y, Chen S-L, Chang Y-H, See Chen P, et al. Correlation between interleukin-6 levels and methadone maintenance therapy outcomes. Drug Alcohol Depend. (2019) 204:107516. doi: 10.1016/j.drugalcdep.2019.06.018
138. Bayazit H, Selek S, Karababa IF, Cicek E, Aksoy N. Evaluation of oxidant/antioxidant status and cytokine levels in patients with cannabis use disorder. Clin Psychopharmacol Neurosci. (2017) 15:237–42. doi: 10.9758/cpn.2017.15.3.237
139. Liu L, Chan C. The role of inflammasome in Alzheimer’s disease. Ageing Res Rev. (2014) 15:6–15. doi: 10.1016/j.arr.2013.12.007
140. Minkiewicz J, de Rivero Vaccari JP, Keane RW. Human astrocytes express a novel NLRP2 inflammasome. Glia. (2013) 61:1113–21. doi: 10.1002/glia.22499
141. Silverman WR, de Rivero Vaccari JP, Locovei S, Qiu F, Carlsson SK, Scemes E, et al. The pannexin 1 channel activates the inflammasome in neurons and astrocytes. J Biol Chem. (2009) 284:18143–51. doi: 10.1074/jbc.M109.004804
142. Halle A, Hornung V, Petzold GC, Stewart CR, Monks BG, Reinheckel T, et al. The NALP3 inflammasome is involved in the innate immune response to amyloid-beta. Nat Immunol. (2008) 9:857–65. doi: 10.1038/ni.1636
143. Ye T, Meng X, Wang R, Zhang C, He S, Sun G, et al. Gastrodin alleviates cognitive dysfunction and depressive-like behaviors by inhibiting ER stress and NLRP3 inflammasome activation in db/db mice. Int J Mol Sci. (2018) 19. doi: 10.3390/ijms19123977
144. Zhuang J, Wen X, Zhang Y-Q, Shan Q, Zhang Z-F, Zheng G-H, et al. TDP-43 upregulation mediated by the NLRP3 inflammasome induces cognitive impairment in 2 2’,4,4’-tetrabromodiphenyl ether (BDE-47)-treated mice. Brain Behav Immun. (2017) 65:99–110. doi: 10.1016/j.bbi.2017.05.014
145. Forlenza OV, Diniz BS, Talib LL, Mendonça VA, Ojopi EB, Gattaz WF, et al. Increased serum IL-1beta level in Alzheimer’s disease and mild cognitive impairment. Dement Geriatr Cognit Disord. (2009) 28:507–12. doi: 10.1159/000255051
146. Diniz BS, Teixeira AL, Talib L, Gattaz WF, Forlenza OV. Interleukin-1beta serum levels is increased in antidepressant-free elderly depressed patients. Am J Geriatr Psychiatry. (2010) 18:172–6. doi: 10.1097/JGP.0b013e3181c2947f
147. Karagüzel EÖ, Arslan FC, Uysal EK, Demir S, Aykut DS, Tat M, et al. Blood levels of interleukin-1 beta, interleukin-6 and tumor necrosis factor-alpha and cognitive functions in patients with obsessive compulsive disorder. Compr Psychiatry. (2019) 89:61–6. doi: 10.1016/j.comppsych.2018.11.013
148. Tian S, Huang R, Han J, Cai R, Guo D, Lin H, et al. Increased plasma Interleukin-1β level is associated with memory deficits in type 2 diabetic patients with mild cognitive impairment. Psychoneuroendocrinology. (2018) 96:148–54. doi: 10.1016/j.psyneuen.2018.06.014
149. O’Reardon JP, Solvason HB, Janicak PG, Sampson S, Isenberg KE, Nahas Z, et al. Efficacy and safety of transcranial magnetic stimulation in the acute treatment of major depression: a multisite randomized controlled trial. Biol Psychiatry. (2007) 62:1208–16. doi: 10.1016/j.biopsych.2007.01.018
150. George MS, Lisanby SH, Avery D, McDonald WM, Durkalski V, Pavlicova M, et al. Daily left prefrontal transcranial magnetic stimulation therapy for major depressive disorder: a sham-controlled randomized trial. Arch Gen Psychiatry. (2010) 67:507–16. doi: 10.1001/archgenpsychiatry.2010.46
151. O’Reardon JP, Solvason HB, Janicak PG, Sampson S, Isenberg KE, Nahas Z, et al. Reply regarding “efficacy and safety of transcranial magnetic stimulation in the acute treatment of major depression: a multisite randomized controlled trial. Biol Psychiatry. (2010) 67:e15–7. doi: 10.1016/j.biopsych.2007.01.018
152. Goodkind M, Eickhoff SB, Oathes DJ, Jiang Y, Chang A, Jones-Hagata LB, et al. Identification of a common neurobiological substrate for mental illness. JAMA Psychiatry. (2015) 72:305–15. doi: 10.1001/jamapsychiatry.2014.2206
153. Hasin DS, Goodwin RD, Stinson FS, Grant BF. Epidemiology of major depressive disorder: results from the National Epidemiologic Survey on Alcoholism and Related Conditions: Results from the national epidemiologic survey on alcoholism and related conditions. Arch Gen Psychiatry. (2005) 62:1097–106. doi: 10.1001/archpsyc.62.10.1097
154. Grant BF, Stinson FS, Dawson DA, Chou SP, Dufour MC, Compton W, et al. Prevalence and co-occurrence of substance use disorders and independent mood and anxiety disorders: results from the National Epidemiologic Survey on Alcohol and Related Conditions: Results from the national epidemiologic survey on alcohol and RelatedConditions. Arch Gen Psychiatry. (2004) 61:807–16. doi: 10.1001/archpsyc.61.8.807
155. Li C-T, Chen M-H, Juan C-H, Huang H-H, Chen L-F, Hsieh J-C, et al. Efficacy of prefrontal theta-burst stimulation in refractory depression: a randomized sham-controlled study. Brain. (2014) 137:2088–98. doi: 10.1093/brain/awu109
156. Huang Y-Z, Edwards MJ, Rounis E, Bhatia KP, Rothwell JC. Theta burst stimulation of the human motor cortex. Neuron. (2005) 45:201–6. doi: 10.1016/j.neuron.2004.12.033
157. Chung SW, Lewis BP, Rogasch NC, Saeki T, Thomson RH, Hoy KE, et al. Demonstration of short-term plasticity in the dorsolateral prefrontal cortex with theta burst stimulation: A TMS-EEG study. Clin Neurophysiol. (2017) 128:1117–26. doi: 10.1016/j.clinph.2017.04.005
158. Chung SW, Rogasch NC, Hoy KE, Sullivan CM, Cash RFH, Fitzgerald PB. Impact of different intensities of intermittent theta burst stimulation on the cortical properties during TMS-EEG and working memory performance. Hum Brain Mapp. (2018) 39:783–802. doi: 10.1002/hbm.23882
159. Daskalakis ZJ, Möller B, Christensen BK, Fitzgerald PB, Gunraj C, Chen R. The effects of repetitive transcranial magnetic stimulation on cortical inhibition in healthy human subjects. Exp Brain Res. (2006) 174:403–12. doi: 10.1007/s00221-006-0472-0
160. Farzan F, Barr MS, Hoppenbrouwers SS, Fitzgerald PB, Chen R, Pascual-Leone A, et al. The EEG correlates of the TMS-induced EMG silent period in humans. Neuroimage. (2013) 83:120–34. doi: 10.1016/j.neuroimage.2013.06.059
161. Tang VM, Le Foll B, Blumberger DM, Voineskos D. Repetitive transcranial magnetic stimulation for comorbid major depressive disorder and alcohol use disorder. Brain Sci. (2021) 12:48. doi: 10.3390/brainsci12010048
162. Blumberger DM, Vila-Rodriguez F, Thorpe KE, Feffer K, Noda Y, Giacobbe P, et al. Effectiveness of theta burst versus high-frequency repetitive transcranial magnetic stimulation in patients with depression (THREE-D): a randomised non-inferiority trial. Lancet. (2018) 391:1683–92. doi: 10.1016/S0140-6736(18)30295-2
163. Bakker N, Shahab S, Giacobbe P, Blumberger DM, Daskalakis ZJ, Kennedy SH, et al. rTMS of the dorsomedial prefrontal cortex for major depression: safety, tolerability, effectiveness, and outcome predictors for 10 Hz versus intermittent theta-burst stimulation. Brain Stimul. (2015) 8:208–15. doi: 10.1016/j.brs.2014.11.002
164. Dunlop K, Gaprielian P, Blumberger D, Daskalakis ZJ, Kennedy SH, Giacobbe P, et al. MRI-guided dmPFC-rTMS as a treatment for treatment-resistant major depressive disorder. J Vis Exp. (2015) 102:e53129. doi: 10.3791/53129
165. Sheehan DV, Lecrubier Y, Sheehan KH, Amorim P, Janavs J, Weiller E, et al. The Mini-International Neuropsychiatric Interview (M.I.N.I.): the development and validation of a structured diagnostic psychiatric interview for DSM-IV and ICD-10. J Clin Psychiatry. (1998) 59 Suppl 20:22–33.
166. Keel JC, Smith MJ, Wassermann EM. A safety screening questionnaire for transcranial magnetic stimulation. Clin Neurophysiol. (2001) 112:720. doi: 10.1016/S1388-2457(00)00518-6
167. Kaster TS, Daskalakis ZJ, Noda Y, Knyahnytska Y, Downar J, Rajji TK, et al. Efficacy, tolerability, and cognitive effects of deep transcranial magnetic stimulation for late-life depression: a prospective randomized controlled trial. Neuropsychopharmacology. (2018) 43:2231–8. doi: 10.1038/s41386-018-0121-x
168. Hamilton M. Development of a rating scale for primary depressive illness. Br J Soc Clin Psychol. (1967) 6:278–96. doi: 10.1111/j.2044-8260.1967.tb00530.x
169. Arias SA, Miller I, Camargo CA Jr, Sullivan AF, Goldstein AB, Allen MH, et al. Factors associated with suicide outcomes 12 months after screening positive for suicide risk in the emergency department. Psychiatr Serv. (2016) 67:206–13. doi: 10.1176/appi.ps.201400513
170. Ionescu DF, Swee MB, Pavone KJ, Taylor N, Akeju O, Baer L, et al. Rapid and sustained reductions in current suicidal ideation following repeated doses of intravenous ketamine: secondary analysis of an open-label study. J Clin Psychiatry. (2016) 77:e719–25. doi: 10.4088/JCP.15m10056
171. Harvey PD, Posner K, Rajeevan N, Yershova KV, Aslan M, Concato J. Suicidal ideation and behavior in US veterans with schizophrenia or bipolar disorder. J Psychiatr Res. (2018) 102:216–22. doi: 10.1016/j.jpsychires.2018.04.014
172. Legarreta M, Graham J, North L, Bueler CE, McGlade E, Yurgelun-Todd D. DSM-5 posttraumatic stress disorder symptoms associated with suicide behaviors in veterans. Psychol Trauma. (2015) 7:277–85. doi: 10.1037/tra0000026
173. Gwaltney C, Mundt JC, Greist JH, Paty J, Tiplady B. Interactive voice response and text-based self-report versions of the electronic Columbia-suicide severity rating scale are equivalent. Innov Clin Neurosci. (2017) 14:17–23.
174. Hesdorffer DC, French JA, Posner K, DiVentura B, Pollard JR, Sperling MR, et al. Suicidal ideation and behavior screening in intractable focal epilepsy eligible for drug trials. Epilepsia. (2013) 54:879–87. doi: 10.1111/epi.2013.54.issue-5
175. Kerr DCR, DeGarmo DS, Leve LD, Chamberlain P. Juvenile justice girls’ depressive symptoms and suicidal ideation 9 years after Multidimensional Treatment Foster Care. J Consult Clin Psychol. (2014) 82:684–93. doi: 10.1037/a0036521
176. Kerr DCR, Gibson B, Leve LD, Degarmo DS. Young adult follow-up of adolescent girls in juvenile justice using the Columbia suicide severity rating scale. Suicide Life Threat Behav. (2014) 44:113–29. doi: 10.1111/sltb.2014.44.issue-2
177. Sun Y, Farzan F, Mulsant BH, Rajji TK, Fitzgerald PB, Barr MS, et al. Indicators for remission of suicidal ideation following magnetic seizure therapy in patients with treatment-resistant depression. JAMA Psychiatry. (2016) 73:337–45. doi: 10.1001/jamapsychiatry.2015.3097
178. Farzan F, Barr MS, Levinson AJ, Chen R, Wong W, Fitzgerald PB, et al. Reliability of long-interval cortical inhibition in healthy human subjects: a TMS-EEG study. J Neurophysiol. (2010) 104:1339–46. doi: 10.1152/jn.00279.2010
179. Lioumis P, Kicić D, Savolainen P, Mäkelä JP, Kähkönen S. Reproducibility of TMS-evoked EEG responses. Hum Brain Mapp. (2009) 30:1387–96. doi: 10.1002/hbm.20608
180. Brooks H, Goodman MS, Bowie CR, Zomorrodi R, Blumberger DM, Butters MA, et al. Theta-gamma coupling and ordering information: a stable brain-behavior relationship across cognitive tasks and clinical conditions. Neuropsychopharmacology. (2020) 45:2038–47. doi: 10.1038/s41386-020-0759-z
181. Goodman MS, Kumar S, Zomorrodi R, Ghazala Z, Cheam ASM, Barr MS, et al. Theta-gamma coupling and working memory in Alzheimer’s dementia and mild cognitive impairment. Front Aging Neurosci. (2018) 10:101. doi: 10.3389/fnagi.2018.00101
182. Charlton AJ, May C, Luikinga SJ, Burrows EL, Hyun Kim J, Lawrence AJ, et al. Chronic voluntary alcohol consumption causes persistent cognitive deficits and cortical cell loss in a rodent model. Sci Rep. (2019) 9:18651. doi: 10.1038/s41598-019-55095-w
183. Broadwater M, Varlinskaya EI, Spear LP. Chronic intermittent ethanol exposure in early adolescent and adult male rats: effects on tolerance, social behavior, and ethanol intake. Alcohol Clin Exp Res. (2011) 35:1392–403. doi: 10.1111/j.1530-0277.2011.01474.x
184. Prévot T, Sibille E. Altered GABA-mediated information processing and cognitive dysfunctions in depression and other brain disorders. Mol Psychiatry. (2021) 26:151–67. doi: 10.1038/s41380-020-0727-3
185. Lang Y, Chu F, Shen D, Zhang W, Zheng C, Zhu J, et al. Role of inflammasomes in neuroimmune and neurodegenerative diseases: A systematic review. Mediators Inflammation. (2018) 2018:1549549. doi: 10.1155/2018/1549549
186. Lippai D, Bala S, Petrasek J, Csak T, Levin I, Kurt-Jones EA, et al. Alcohol-induced IL-1β in the brain is mediated by NLRP3/ASC inflammasome activation that amplifies neuroinflammation. J Leukoc Biol. (2013) 94:171–82. doi: 10.1189/jlb.1212659
187. Fitzgerald PJ. Elevated Norepinephrine may be a Unifying Etiological Factor in the Abuse of a Broad Range of Substances: Alcohol, Nicotine, Marijuana, Heroin, Cocaine, and Caffeine. Subst Abuse. (2013) 7:171–83. doi: 10.4137/SART.S13019
188. Fredriksson I, Jayaram-Lindström N, Wirf M, Nylander E, Nyström E, Jardemark K, et al. Evaluation of guanfacine as a potential medication for alcohol use disorder in long-term drinking rats: behavioral and electrophysiological findings. Neuropsychopharmacology. (2015) 40:1130–40. doi: 10.1038/npp.2014.294
189. Newcorn JH, Harpin V, Huss M, Lyne A, Sikirica V, Johnson M, et al. Extended-release guanfacine hydrochloride in 6-17-year olds with ADHD: a randomised-withdrawal maintenance of efficacy study. J Child Psychol Psychiatry. (2016) 57:717–28. doi: 10.1111/jcpp.2016.57.issue-6
190. Setiawan E, Pihl RO, Cox SML, Gianoulakis C, Palmour RM, Benkelfat C, et al. The effect of naltrexone on alcohol’s stimulant properties and self-administration behavior in social drinkers: influence of gender and genotype. Alcohol Clin Exp Res. (2011) 35:1134–41. doi: 10.1111/j.1530-0277.2011.01446.x
191. Ralevski E, Balachandra K, Gueorguieva R, Limoncelli D, Petrakis I. Effects of naltrexone on cognition in a treatment study of patients with schizophrenia and comorbid alcohol dependence. J Dual Diagn. (2006) 2:53–69. doi: 10.1300/J374v02n04_05
192. Jaffe A, O’Malley SS, Hickcox M, Chang G, Schottenfeld RS, Rounsaville BJ. Effects of Naltrexone on the Cognitive Functioning of Recently Abstinent Alcohol Dependent Patients. In: Novel Pharmacological Interventions for Alcoholism. Springer New York:Springer (1992). p. 329–34.
193. Fletcher PJ, Tampakeras M, Sinyard J, Higgins GA. Opposing effects of 5-HT(2A) and 5-HT(2C) receptor antagonists in the rat and mouse on premature responding in the five-choice serial reaction time test. Psychopharmacology. (2007) 195:223–34. doi: 10.1007/s00213-007-0891-z
194. Lalonde R. The neurobiological basis of spontaneous alternation. Neurosci Biobehav Rev. (2002) 26:91–104. doi: 10.1016/S0149-7634(01)00041-0
195. Floresco SB, Block AE, Tse MTL. Inactivation of the medial prefrontal cortex of the rat impairs strategy set-shifting, but not reversal learning, using a novel, automated procedure. Behav Brain Res. (2008) 190:85–96. doi: 10.1016/j.bbr.2008.02.008
196. Irimia C, Wiskerke J, Natividad LA, Polis IY, de Vries TJ, Pattij T, et al. Increased impulsivity in rats as a result of repeated cycles of alcohol intoxication and abstinence. Addict Biol. (2015) 20:263–74. doi: 10.1111/adb.2015.20.issue-2
197. Prevot TD, Li G, Vidojevic A, Misquitta KA, Fee C, Santrac A, et al. Novel benzodiazepine-like ligands with various anxiolytic, antidepressant, or pro-cognitive profiles. Mol Neuropsych. (2019) 5:84–97. doi: 10.1159/000496086
198. Fletcher PJ, Rizos Z, Noble K, Higgins GA. Impulsive action induced by amphetamine, cocaine and MK801 is reduced by 5-HT(2C) receptor stimulation and 5-HT(2A) receptor blockade. Neuropharmacology. (2011) 61:468–77. doi: 10.1016/j.neuropharm.2011.02.025
199. Aharonovich E, Hasin DS, Brooks AC, Liu X, Bisaga A, Nunes EV. Cognitive deficits predict low treatment retention in cocaine dependent patients. Drug Alcohol Depend. (2006) 81:313–22. doi: 10.1016/j.drugalcdep.2005.08.003
200. Aharonovich E, Nunes E, Hasin D. Cognitive impairment, retention and abstinence among cocaine abusers in cognitive-behavioral treatment. Drug Alcohol Depend. (2003) 71:207–11. doi: 10.1016/S0376-8716(03)00092-9
201. Copersino ML, Schretlen DJ, Fitzmaurice GM, Lukas SE, Faberman J, Sokoloff J, et al. Effects of cognitive impairment on substance abuse treatment attendance: predictive validation of a brief cognitive screening measure. Am J Drug Alcohol Abuse. (2012) 38:246–50. doi: 10.3109/00952990.2012.670866
202. Allen DN, Goldstein G, Seaton BE. Cognitive rehabilitation of chronic alcohol abusers. Neuropsych Rev. (1997) 7:21–39. doi: 10.1007/BF02876971
203. Katz EC, King SD, Schwartz RP, Weintraub E, Barksdale W, Robinson R, et al. Cognitive ability as a factor in engagement in drug abuse treatment. Am J Drug Alcohol Abuse. (2005) 31:359–69. doi: 10.1081/ADA-200056767
204. Curran GM, Sullivan G, Williams K, Han X, Collins K, Keys J, et al. Emergency department use of persons with comorbid psychiatric and substance abuse disorders. Ann Emerg Med. (2003) 41:659–67. doi: 10.1067/mem.2003.154
205. Dawson H, Zinck G. CIHI Survey: ED spending in Canada: a focus on the cost of patients waiting for access to an in-patient bed in Ontario. Healthc Q. (2009) 12:25–8. doi: 10.12927/hcq.2009.20411
206. Saunders CE. Time study of patient movement through the emergency department: sources of delay in relation to patient acuity. Ann Emerg Med. (1987) 16:1244–8. doi: 10.1016/S0196-0644(87)80232-9
207. Rehm J, Gmel GE Sr, Gmel G, Hasan OSM, Imtiaz S, Popova S, et al. The relationship between different dimensions of alcohol use and the burden of disease-an update. Addiction. (2017) 112:968–1001. doi: 10.1111/add.v112.6
208. Stockwell T. Canadian Substance Use Costs and Harms in the Provinces and Territories: (2007-2014). Ottawa, ON, Canada: Canadian Centre on Substance Abuse (2018). p. 42.
209. Severtson SG, von Thomsen S, Hedden SL, Latimer W. The association between executive functioning and motivation to enter treatment among regular users of heroin and/or cocaine in Baltimore, MD. Addict Behav. (2010) 35:717–20. doi: 10.1016/j.addbeh.2010.02.012
210. Yücel M, Lubman DI. Neurocognitive and neuroimaging evidence of behavioural dysregulation in human drug addiction: implications for diagnosis, treatment and prevention. Drug Alcohol Rev. (2007) 26:33–9. doi: 10.1080/09595230601036978
211. Bruijnen CJWH, Dijkstra BAG, Walvoort SJW, Markus W, VanDerNagel JEL, Kessels RPC, et al. Prevalence of cognitive impairment in patients with substance use disorder. Drug Alcohol Rev. (2019) 38:435–42. doi: 10.1111/dar.2019.38.issue-4
212. Roth RM, Isquith PK, Gioia GA. Assessment of Executive Functioning Using the Behavior Rating Inventory of Executive Function (BRIEF). In: Goldstein S, Naglieri JA, editors. Handbook of Executive Functioning. Springer New York, New York, NY (2014). p. 301–31.
213. Andersen PK, Gill RD. Cox’s regression model for counting processes: A large sample study. Math Centrum Afdeling Math Statistiek. (1981) 10(4):38.
214. Prentice RL, Williams BJ, Peterson AV. On the regression analysis of multivariate failure time data. Biometrika. (1981) 68:373–9. doi: 10.1093/biomet/68.2.373
215. Cai J, Schaubel DE. Marginal means/rates models for multiple type recurrent event data. Lifetime Data Anal. (2004) 10:121–38. doi: 10.1023/B:LIDA.0000030199.23383.45
216. Therneau TM, Grambsch PM. Modeling Survival Data: Extending the Cox Model. New York, NY, USA: Springer Science & Business Media (2013).
217. Epstein EE. Classification of alcohol-related problems and dependence. In: Heather N, editor. International handbook of alcohol dependence and problems, vol. xvii . John Wiley & Sons Ltd, Hoboken, NJ, US (2001). p. 47–70.
218. Houghton DC, Spratt HM, Keyser-Marcus L, Bjork JM, Neigh GN, Cunningham KA, et al. Behavioral and neurocognitive factors distinguishing post-traumatic stress comorbidity in substance use disorders. Transl Psychiatry. (2023) 13:296. doi: 10.1038/s41398-023-02591-3
219. Oliva V, De Prisco M, Pons-Cabrera MT, Guzmán P, Anmella G, Hidalgo-Mazzei D, et al. Machine learning prediction of comorbid substance use disorders among people with bipolar disorder. J Clin Med Res. (2022) 11(14):3935. doi: 10.3390/jcm11143935
220. Long Y, Pan N, Ji S, Qin K, Chen Y, Zhang X, et al. Distinct brain structural abnormalities in attention-deficit/hyperactivity disorder and substance use disorders: A comparative meta-analysis. Transl Psychiatry. (2022) 12:368. doi: 10.1038/s41398-022-02130-6
221. Schuckit MA. Comorbidity between substance use disorders and psychiatric conditions. Addiction. (2006) 101 Suppl 1:76–88. doi: 10.1111/j.1360-0443.2006.01592.x
222. Regier DA, Farmer ME, Rae DS, Locke BZ, Keith SJ, Judd LL, et al. Comorbidity of mental disorders with alcohol and other drug abuse. Results from the Epidemiologic Catchment Area (ECA) Study. JAMA. (1990) 264:2511–8. doi: 10.1001/jama.1990.03450190043026
223. Silveira S, Shah R, Nooner KB, Nagel BJ, Tapert SF, de Bellis MD, et al. Impact of childhood trauma on executive function in adolescence-mediating functional brain networks and prediction of high-risk drinking. Biol Psychiatry Cognit Neurosci Neuroimaging. (2020) 5:499–509. doi: 10.1016/j.bpsc.2020.01.011
224. Deak JD, Johnson EC. Genetics of substance use disorders: a review. Psychol Med. (2021) 51:2189–200. doi: 10.1017/S0033291721000969
225. Connor JP, Haber PS, Hall WD. Alcohol use disorders. Lancet. (2016) 387:988–98. doi: 10.1016/S0140-6736(15)00122-1
226. Niklason GR, Rawls E, Ma S, Kummerfeld E, Maxwell AM, Brucar LR, et al. Explainable machine learning analysis reveals sex and gender differences in the phenotypic and neurobiological markers of Cannabis Use Disorder. Sci Rep. (2022) 12:15624. doi: 10.1038/s41598-022-19804-2
227. Saberi Zafarghandi MB, Khanipour H, Ahmadi SM. Typology of substance use disorder based on temperament dimensions, addiction severity, and negative emotions. Iran J Psychiatry. (2018) 13:184–90.
228. Charron E, Yu Z, Lundahl B, Silipigni J, Okifuji A, Gordon AJ, et al. Cluster analysis to identify patient profiles and substance use patterns among pregnant persons with opioid use disorder. Addict Behav Rep. (2023) 17:100484. doi: 10.1016/j.abrep.2023.100484
229. Drossel G, Brucar LR, Rawls E, Hendrickson TJ, Zilverstand A. Subtypes in addiction and their neurobehavioral profiles across three functional domains. Transl Psychiatry. (2023) 13:127. doi: 10.1038/s41398-023-02426-1
230. Gold AK, Otto MW. Impaired risk avoidance in bipolar disorder and substance use disorders. J Psychiatr Res. (2022) 152:335–42. doi: 10.1016/j.jpsychires.2022.05.019
231. Moss HB, Chen CM, Yi H-Y. Subtypes of alcohol dependence in a nationally representative sample. Drug Alcohol Depend. (2007) 91:149–58. doi: 10.1016/j.drugalcdep.2007.05.016
232. Windle M, Scheidt DM. Alcoholic subtypes: are two sufficient? Addiction. (2004) 99:1508–19. doi: 10.1111/j.1360-0443.2004.00878.x
233. Tapert SF, Eberson-Shumate S. Alcohol and the adolescent brain: What we’ve learned and where the data are taking us. Alcohol Res. (2022) 42:07. doi: 10.35946/arcr.v42.1.07
234. Barr P, Neale Z, Chatzinakos C, Schulman J, Mullins N, Zhang J, et al. Clinical, genomic, and neurophysiological correlates of lifetime suicide attempts among individuals with alcohol dependence. Res Sq. (2024). doi: 10.21203/rs.3.rs-3894892/v1
235. Hueniken K, Somé NH, Abdelhack M, Taylor G, Elton Marshall T, Wickens CM, et al. Machine learning-based predictive modeling of anxiety and depressive symptoms during 8 months of the COVID-19 global pandemic: repeated cross-sectional survey study. JMIR Ment Health. (2021) 8:e32876. doi: 10.2196/32876
236. Tio ES, Misztal MC, Felsky D. Evidence for the biopsychosocial model of suicide: a review of whole person modeling studies using machine learning. Front Psychiatry. (2023) 14:1294666. doi: 10.3389/fpsyt.2023.1294666
237. Felsky D, Cannitelli A, Pipitone J. Whole Person Modeling: a transdisciplinary approach to mental health research. Discovery Ment Health. (2023) 3:16. doi: 10.1007/s44192-023-00041-6
238. Sampedro-Piquero P, Ladrón-de-Guevara-Miranda D, Pavón FJ, Serrano A, Suárez J, Rodríguez de Fonseca F, et al. Neuroplastic and cognitive impairment in substance use disorders: a therapeutic potential of cognitive stimulation. Neurosci Biobehav Rev. (2019) 106:23–48. doi: 10.1016/j.neubiorev.2018.11.015
239. Moeller SJ, Paulus MP. Toward biomarkers of the addicted human brain: Using neuroimaging to predict relapse and sustained abstinence in substance use disorder. Prog Neuropsychopharmacol Biol Psychiatry. (2018) 80:143–54. doi: 10.1016/j.pnpbp.2017.03.003
240. Tanabe J, Regner M, Sakai J, Martinez D, Gowin J. Neuroimaging reward, craving, learning, and cognitive control in substance use disorders: review and implications for treatment. Br J Radiol. (2019) 92:20180942. doi: 10.1259/bjr.20180942
241. Smith JA, Fieldsend M. Interpretative phenomenological analysis.,” Qualitative research in psychology: Expanding perspectives in methodology and design. 2nd ed. Washington: American Psychological Association (2021) p. 147–66.
242. Wang C, Burris MA. Photovoice: concept, methodology, and use for participatory needs assessment. Health Educ Behav. (1997) 24:369–87. doi: 10.1177/109019819702400309
243. Natafgi N, Parris K, Walker E, Gartner T, Coffin J, Mitcham A, et al. Through their eyes: Using photovoice to capture the capacity-building journey of Long Covid Patient Experts. Health Expect. (2024) 27:e70094. doi: 10.1111/hex.70094
244. Tanhan A, Strack RW. Online photovoice to explore and advocate for Muslim biopsychosocial spiritual wellbeing and issues: Ecological systems theory and ally development. Curr Psychol. (2020) 39:2010–25. doi: 10.1007/s12144-020-00692-6
245. Doyumğaç İ, Tanhan A, Kiymaz MS. Understanding the most important facilitators and barriers for online education during COVID-19 through Online Photovoice methodology. Int J High Educ. (2020) 10:166. doi: 10.5430/ijhe.v10n1p166
246. Waalkes PL, Mizutani Y, Kim SR, Napitupulu A, Thompson J, Leiva AN, et al. Professional identity development of counselor educators with international backgrounds through online photovoice. Int J Adv Couns. (2025) 47:150–70. doi: 10.1007/s10447-024-09582-z
247. Collins SE, Clifasefi SL, Stanton J, The Leap Advisory Board, Straits KJE, Gil-Kashiwabara E, et al. Community-based participatory research (CBPR): Towards equitable involvement of community in psychology research. Am Psychol. (2018) 73:884–98. doi: 10.1037/amp0000167
Keywords: alcohol use disorder, substance use disorder, addiction, cognition, executive function, preclinical, translational
Citation: Nikolova YS, Ruocco AC, Felsky D, Lange S, Prevot TD, Vieira E, Voineskos D, Wardell JD, Blumberger DM, Clifford K, Naik Dharavath R, Gerretsen P, Hassan AN, Hope IM, Irwin SH, Jennings SK, Le Foll B, Melamed O, Orson J, Pangarov P, Quigley L, Russell C, Shield K, Sloan ME, Smoke A, Tang V, Valdes Cabrera D, Wang W, Wells S, Wickramatunga R, Sibille E and Quilty LC (2025) Cognitive Dysfunction in the Addictions (CDiA): protocol for a neuron-to-neighbourhood collaborative research program. Front. Psychiatry 16:1455968. doi: 10.3389/fpsyt.2025.1455968
Received: 27 June 2024; Accepted: 31 March 2025;
Published: 19 May 2025.
Edited by:
Ilya Blokhin, University of Pittsburgh, United StatesReviewed by:
Ahmet Tanhan, Economic and Social Research Center (ESAM), TürkiyeAleksandar Jovanoski, University St. Clement of Ohrid, North Macedonia
Copyright © 2025 Nikolova, Ruocco, Felsky, Lange, Prevot, Vieira, Voineskos, Wardell, Blumberger, Clifford, Naik Dharavath, Gerretsen, Hassan, Hope, Irwin, Jennings, Le Foll, Melamed, Orson, Pangarov, Quigley, Russell, Shield, Sloan, Smoke, Tang, Valdes Cabrera, Wang, Wells, Wickramatunga, Sibille and Quilty. This is an open-access article distributed under the terms of the Creative Commons Attribution License (CC BY). The use, distribution or reproduction in other forums is permitted, provided the original author(s) and the copyright owner(s) are credited and that the original publication in this journal is cited, in accordance with accepted academic practice. No use, distribution or reproduction is permitted which does not comply with these terms.
*Correspondence: Lena C. Quilty, bGVuYS5xdWlsdHlAY2FtaC5jYQ==
†These authors have contributed equally to this work